- 1Sorbonne Université, CNRS, Laboratoire d'Océanographie de Villefranche, LOV, F-06230 Villefranche-sur-Mer, France
- 2ACRI-ST, Sophia-Antipolis, France
- 3Remote Sensing and Satellite Research Group, School of Earth and Planetary Sciences, Curtin University, Perth, WA, Australia
- 4School of Marine Sciences, University of Maine, Orono, ME, United States
- 5Department of Oceanography, Dalhousie University, Halifax, NS, Canada
An efficient system to produce in situ high quality radiometric measurements is compulsory to rigorously perform the vicarious calibration of satellite sensors dedicated to Ocean Color Radiometry (OCR) and to validate their derived products. This requirement is especially needed during the early stages of an OCR satellite activity or for remote areas poorly covered by oceanographic cruises with possible bio-optical anomalies. Taking advantage of Argo's profiling float technology, we present a new autonomous profiling float dedicated to in situ radiometric measurements. The float is based on the Provor CTS5 (manufacturer NKE) with an added novel two protruding arm design allowing for sensor redundancies, shading mitigation and near-surface data. Equipped with two identical radiometers on each arm that measure downward irradiance and upwelling radiance at seven wavelengths, the ProVal float generates both redundant radiometric profiles as well as an estimate of Remote Sensing Reflectance. Results from 449 profiles obtained in the NW Mediterranean Sea and in the Indian sector of the Southern Ocean are presented to illustrate the ProVal float technical maturity. Analysis of the behavior of the profiling float, including tilting and ascent speeds is presented. The vertical stability of the ProVal exhibits 85% of surface data of the Mediterranean Sea with a tilt smaller than 10 degrees. This percentage is 40% in the Southern Ocean due to rougher seas. Redundant sensors provide a characterization of the relative drift between sensors over the deployment which is found to be <0.15% per month over a year. Post-cruise calibration of a recovered float revealed no significant drift. As an example of the utility of ProVal floats, a match-up of Remote Sensing Reflectance measured with the European Space Agency Ocean and Land Color Imager (OLCI onboard Sentinel-3A) is shown. It follows that profiling floats, such as ProVal, could provide a significant contribution to an upcoming global System Vicarious Calibration of space-based radiometers.
Introduction
Since the first satellite sensor dedicated to Ocean Color Radiometry (OCR) was launched in 1978, the “Coastal Zone Color Scanner” (CZCS, NASA) (Hovis et al., 1980), the number and types of satellites observing the color of the world's ocean has greatly increased. In addition, the variety of applications and products derived from their observations has as well dramatically increased. To deliver OCR data with the required level of uncertainties, the spectral imagers of the satellite sensors are calibrated through a specific procedure called “System Vicarious Calibration” (SVC). SVC consists of adjusting the sensor's spectral gains (g-factors) by comparing reflectances derived from the satellite observations to reflectances derived from highly accurate in situ radiometric measurements over the mission lifetime (Gordon, 1987, 1998). The primary marine products (i.e., spectral water-leaving radiance Lw) as well as those derived from these products (e.g., Chlorophyll-a concentration) must also be validated through comparison with in situ observations.
Only two in situ moorings are routinely used for SVC: the Marine Optical Buoy (MOBY) offshore of Lanai, Hawaii (Clark et al., 2003), and the Bouée pour l'acquisition de Séries Optiques à Long Terme (BOUSSOLE) in the Northwestern Mediterranean Sea (Antoine et al., 2008a,b). Deployed in deep-water areas, these moorings provide long-term time-series that are needed for the inter-comparison and merging of data acquired by different generations of satellite sensors (IOCCG, 2004; Gregg, 2007). Radiometric in situ measurements are also performed by coastal automatic stations as part of the AERONET-OC network (Zibordi et al., 2009), as well as from ships by using in-water freefalling profilers or above-water radiometers (Hooker and Maritorena, 2000; Hooker et al., 2002b). Nevertheless, the scarcity of in situ data still remains an issue for the realization of the full potential and capabilities of OCR satellite missions. This is especially true for remote areas that have been poorly explored by oceanographic cruises and/or areas with known bio-optical anomalies such as the Southern Ocean (Mitchell and Holm-Hansen, 1991; Organelli et al., 2017). The lack of real time in situ data is also particularly critical at the beginning of the lifetime of an OCR satellite. Operational ocean color missions must provide validated and accurate measurements and products within a short delay after launch. Typically, data are expected after the commissioning phase which generally lasts between 6 and 12 months. Using current systems (i.e., moorings), several years can go by before a sufficient number of concurrent in situ and satellite measurements bearing the required quality levels can be reached to achieve accurate and stable g-factors (Franz et al., 2007; Bailey et al., 2008; Zibordi and Mélin, 2017). As an example, to perform the first SVC, an operational mission such as Ocean Land Color Instrument (OLCI) on board the Sentinel-3A satellite has relied on climatological averages derived from other satellite missions, in addition to in situ measurements (Sentinel-3A Product Notice–OLCI Level-2 Ocean Colour, 2018). Thus, proper calibration, validation and interpretation of satellite OCR data on a global basis could benefit greatly from an extensive observational record of real time in situ radiometric measurements particularly early in the mission.
Since 1999, the international Argo program has revolutionized modern oceanography by operating more than 4,000 autonomous profiling floats measuring temperature and salinity profiles across most of the global ocean. Argo is now the main source of CTD data for the world's oceans (Roemmich, 2009). Thanks to the miniaturization of sensors, in particular optical devices, Argo floats have progressively acquired the capability to monitor additional seawater properties, relevant, in particular, for biogeochemical and bio-optical studies. The anticipated high spatio-temporal coverage resulting from such a program thus represents an opportunity for ocean color validation activities. In 2011, the International Ocean Color Coordinating Group (IOCCG) recommended the utilization of several types of profiling floats and provided recommendation for their use in synergy with ocean color (IOCCG, 2011). In the lightest configuration, the measurement of the downward Photosynthetically Available Radiation (PAR), Chlorophyll-a fluorescence and the backscattering coefficient as a proxy for suspended particulate matter was recommended. An outcome of this evolution is the Biogeochemical Argo (BGC-Argo) program, started in 2016, which aims to organize, coordinate and valorize the Argo scientific activity related to the new capability for biogeochemical and bio-optical studies. The BGC-Argo community recommended the global implementation of 1000 floats measuring 6 core variables (Biogeochemical-Argo Planning Group, 2016, see table 1) including aforementioned variables recommended by the IOCCG. Some BGC-Argo floats additionally measure downward irradiance at 380, 412, and 490 nm in addition to PAR (Leymarie et al., 2013; Organelli et al., 2016). In its report, the IOCCG also recommended to develop a “Val float” which would be able to measure upwelling radiances and downward irradiances at several wavelengths between 412 and 665 nm and, if possible, up to 870 nm, as well as Chlorophyll-a fluorescence. A first “Val type” float was reported on by Gerbi et al. (2016). This float measured upwelling radiances and downward irradiances at four wavelengths (412, 443, 490, and 555 nm). The authors concluded that “autonomous floats can be used for in situ validation of satellite estimates of remote sensing reflectance in the ocean” and showed that the comparison between floats and satellite are consistent in time over at least 1 year. More recently, off-the-shelf floats and sensors were used to measure upwelling radiances and downward irradiances at the same wavelengths in the Indian ocean (Wojtasiewicz et al., 2018).
Going a step further, we present here an autonomous profiling float, called ProVal, which is dedicated to the validation of OCR data. This float is based on a two-arm design that allows for the measurement of upwelling radiances and downward irradiances at seven wavelengths with sensor redundancy and shading mitigation. In this paper, the rationale of the platform design is described, its navigation behavior (velocity in the water column and tilt) is reported, and initial results that confirm the benefit of the sensor redundancy are presented. Finally, a first comparison of Remote Sensing Reflectance with the OLCI sensor (Sentinel-3A) is presented followed by a discussion about the place of ProVal floats, or equivalent autonomous platforms, in a global validation array. The possible future utilization of such platforms as part of a global System Vicarious Calibration is also presented.
Methods
ProVal Mission Requirements
The ProVal project aimed to create an autonomous platform for cost-effective in situ radiometric measurements in the open ocean for OCR validation. Off-the-shelf radiometers were selected to measure multispectral in-water radiances and irradiances, and the development focus was on the platform itself. This general objective resulted in three main mission requirements.
The first requirement was to develop an autonomous platform able to operate in remote areas of the ocean, without human supervision, and with a long-enough lifetime to allow for large numbers of profiles to be acquired, yet within the acceptable limits of calibration drift.
The second requirement was to obtain the same radiometric data quality as that presently achieved with manual freefall profilers which are generally used with similar radiometers. This requirement includes addressing issues such as shading, sensor tilt and sensor drift. Shading could be produced by the equipment itself (radiometer and profiler) or by external structures (ship, buoy) resulting in a biased measurement. A low tilt is mandatory to fulfill radiometric acquisition geometry and could be influenced by unbalanced profiler, waves or external constraint (cable connected to a ship). For this requirement, a threshold of 10° for the tilt was chosen (Mueller et al., 2003) even though 5° could be a target with favorable weather conditions. Finally, measurements long-term drift may be caused by sensor aging or biofouling. As recovery is not always possible, post-deployment calibration of radiometer cannot be done systematically for an autonomous system. In this case, sensor redundancy helps to monitor degradation over the system lifetime by performing sensor inter-comparison.
Finally, the third requirement was to deliver final radiometric products in near-real-time for ocean color data validation. This point deals with possible co-located satellite measurements (in time and space), as well as a sampling strategy adapted to the production of relevant products such as water leaving radiance or remote sensing reflectance. In addition, for an accurate extrapolation of radiometric quantities to the sea surface to be performed, radiometric data must be acquired as close as possible to the sea surface, at least above 1 m depth (Li et al., 2016) and with a targeted depth resolution of 10 cm for the upwelling radiance (Zibordi et al., 2004).
The ProVal Float
The Argo and BGC-Argo programs have demonstrated that autonomous profiling floats are cost-effective and flexible platforms for performing rigorous in situ measurements. Profiling floats are weakly impacted by biofouling because they spend most of the time in the dark deep (>500 m) ocean (Boss et al., 2008; Organelli et al., 2016). They have also several advantages when compared, for example, to other autonomous platforms such as gliders. The slow motion of a float increases data density per meter while its design and operational principle ensure the required orientation of radiometers. In addition, floats can easily accommodate complex sensor structures because they do not have strong requirements as to their hydrodynamic shape. Finally, floats are very well adapted for long term deployment (several months) in open ocean. For the ProVal application, a new PROVOR float (NKE instrumentation) named “CTS5,” was developed as part of a collaboration between NKE and the Laboratoire d'Océanographe de Villefranche (LOV). As for any float of the PROVOR family, the large hull size ensures good stability (large separation of center of buoyancy from center of gravity), a substantial reserve of buoyancy, and a large battery capacity. The CTS5 offers extended capacities thanks to novel hardware and software (see Supplementary Material for details).
Mechanical Design and Sensors Properties
The ProVal float uses a “two-arm configuration” inspired by the BOUSSOLE mooring (Antoine et al., 2008b) and allows for two identical radiometers to be hosted (OCR507-IR, Satlantic Inc., Canada), one on each side of the float at a distance of 0.65 m (inter-axial) from the float hull (0.5 m from the syntactic foam, Figure 1). Radiometers are maintained parallel to the float hull by three rigid polyester tubes and their correct alignments are checked in laboratory, prior to deployment, by using a spirit level. Both sensors measure the downward irradiance (Ed) and the upwelling nadir radiance (Lu) at seven customizable wavelengths with a 10 nm bandwidth and with a field of view of 10° (half-angle) for the radiance sensor. This “two-arm configuration” has several advantages in relation to previously cited requirements: when the float is at the surface (“buoy” mode) the sensors are at 21 and 45 cm depth, respectively, for irradiance and radiance sensors. Platform shading issues are minimized by always having at least one sensor being outside of the float's shadow. This configuration also provides redundancy of data, which is helpful in monitoring the relative behavior of the instruments over time and for quality control.
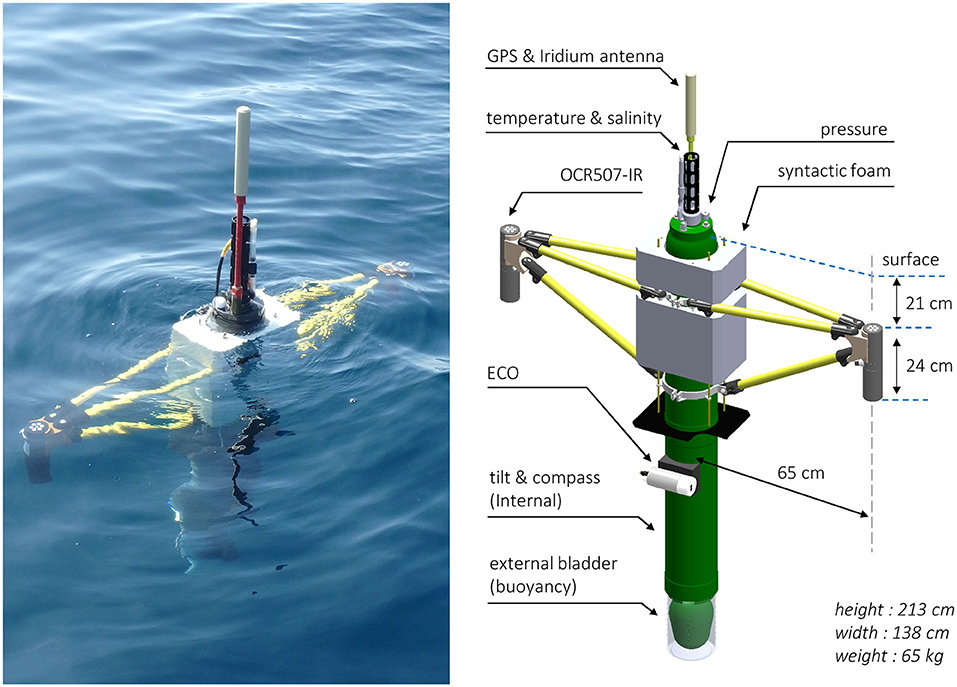
Figure 1. Left, ProVal float at surface during deployment in the Mediterranean Sea (photo courtesy of N. Mayot). Right, technical drawing. Radiometers are mounted at 65 cm (inter-axial) from the float hull. In “buoy” mode, radiometers are at 21 and 45 cm depth, respectively, for irradiance and radiance sensors.
The characteristics of the two radiometers integrated on floats presented here are reported in Table 1. A channel for downward PAR (Photosynthetically Available Radiation) has been included to ensure compatibility with other floats deployed by the BGC-Argo program (Biogeochemical-Argo Planning Group, 2016), leaving six channels on each arm for irradiance measurements. As a result, irradiance at 380 and 412 nm are only measured on one arm (see Table 1. Note that space-borne measurements apply an irradiance model to derive reflectance while on the ProVal it is directly calculated from measurements).
For a proper use of radiometric data, knowledge of the tilt and orientation of the float is required. For this purpose, the float is equipped with a tilt and compass sensor (HMR 3300, Honeywell Electronics). Some ProVal floats also host Chlorophyll A (Chla) and Colored Dissolved Organic Matter (CDOM) fluorometers combined with an optical backscattering measurement at 700 nm (sensor ECO FLbbCD2k, WetLabs). The sensor suite mounted on the ProVal float is summarized in Table 1.
Data Sampling Strategy
Sampling rates of sensors onboard the float are too high for transmitting all data using iridium communication at a reasonable cost. The software on the acquisition board used on the ProVal float enables a flexible sampling strategy in order to optimize the resolution and volume of data. Several configurations have been tested to study the behavior of the platform. Here a description is given of the most appropriate configuration in which an initial dataset can be collected for data quality assessment (Table 2). No data are acquired during the descent of the float due to the variable speed during this phase (not shown). Some data are acquired during the parking phase at about 500 m depth to monitor the dark currents. Vertical profiles are acquired during the float's ascending phase with increasing resolution (decreasing depth intervals) from bottom to surface. For example, radiometers (OCR507IR) are powered on at 250 m depth and data frames are recorded every 1 m up to 60 m then every 10 cm up to the surface. Finally, 1 min of radiometric data and tilt are acquired at the surface in “buoy” mode. This configuration can be modified remotely with a tradeoff between the amount of data to be transmitted and the available energy and data transmission costs. The configuration described here results in a data volume of nearly 140 kBytes per profile (average transmission time of 12 min) and provides a theoretical lifetime of more than 300 profiles.
Data Processing
For the purpose of this paper, which is mainly focused on a description of the platform, classical ocean optics protocols have been applied to process radiometric data. These are summarized in this section along with aspects concerning other measured variables (i.e., tilt and depth). Example of data are shown in Figure 2.
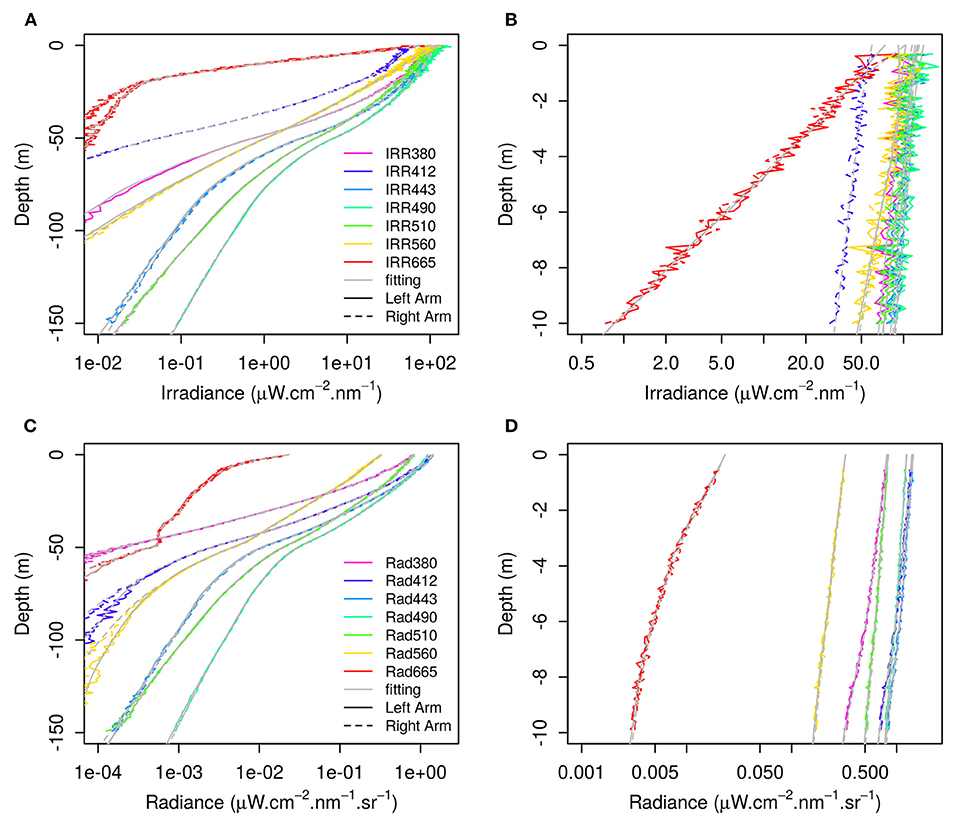
Figure 2. Example of data, float lovapm006f (profile 57, 2017–08–02). Data (color) and fitting functions (gray), for irradiance (A,B), and radiance (C,D) data, for the whole profile (A,C) and close to the surface (B,D). Data with tilt >10° are flagged. A Deep Chlorophyll Maximum (not shown) is present at 50 m depth and explains the local maximum of radiance at 665 nm at the same depth. Data from left arm (solid lines) and from right arm (dashed lines) are as expected mostly superimposed except at depth where dark correction issues appear.
Basic Processing
Digital counts from the radiometers are converted into physical units using the manufacturer calibration coefficients. These coefficients include the dark counts which are, by nature, sensitive to temperature (Kuusk, 2011). During a standard cruise operation with shipboard optical profilers, dark counts are measured just before and/or after the deployment and subtracted from measurements. Here, we use the median of data measured during a one-night profile (sampled at the beginning of the deployment) as an in situ dark correction. This in situ dark correction is typically <0.05% of the signal at the surface and is therefore only significant at depths where signals are small. Depths in units of pressure are converted to meters by using the swDepth function of the oce R-package (Kelley, 2017) and the distance offsets between the pressure gauge and the optical collectors are taken into account. The tilt and heading values associated with each radiometric measurement are calculated by interpolating measurements near the time of the radiometric measurement. On average, the time difference is 0.5 s between tilt/heading and radiometric measurements. An offset is added to have heading values relative to North. This offset is determined at sea by assuming that the maximum shading effect on radiance measurements is obtained when one arm is aligned with the sun (see section in situ Shading Estimation).
Ascent and Surface Processing
Data from the ascending profiles are processed to extrapolate Lu and Ed measurements to just below the surface (the “0−” level). First, data with a tilt above a threshold of 10° are discarded (Mueller et al., 2003). This threshold can possibly be reduced to 5° in calm seas such as the Mediterranean Sea. To extrapolate data to “0−,” we use a local polynomial regression fitting (Cleveland et al., 1992). This method was preferred to an exponential fitting to allow for a profile to be fitted even with a local maximum as can be found in the red due to inelastic scattering (Figure 2C) related to the presence of a deep chlorophyll maximum (not shown). These fitting functions are noted fEdi(z, λ) and fLui(z, λ) where i is the arm number (1 or 2) and their values for z = 0 provide an estimate of the irradiance and radiance at “0−” noted and . Fitting functions are also be used to derive irradiance and radiance diffuse attenuation coefficients [noted, respectively, Kdi(z, λ) and KLui(z, λ)] as follows:
where Δz, equal to 1 m here, is the depth increment chosen to compute diffuse attenuation coefficients (first value at Δz/2 = 0.5 m).
Data from the surface “buoy” mode are filtered according to the tilt by using the same thresholds. Even in “buoy” mode, irradiances and radiances values are sampled slightly underwater at a given depth z estimated according to the pressure sensor. Shallowest estimated diffuse attenuation coefficients are used to extrapolate these measurements to “0−” following:
The mean of all irradiance and radiance values obtained during the “buoy” mode are processed to obtain and . In addition to and four estimates of irradiance and radiance below the surface are obtained.
Computing in situ Remote Sensing Reflectance
The nadir remote sensing reflectance (Rrs(λ)) is defined as the ratio between the water-leaving radiance (Lw(λ)) and the downward irradiance just above water (ES(λ)):
Lw is derived from according to Gordon and Clark (1981) :
where ρ ≅ 0.021 is the Fresnel reflectance at the air sea interface (Austin, 1974) and nw ≅ 1.34 is the refractive index of seawater relative to air (Quan and Fry, 1995). Downward irradiance just above water is derived from:
Where α = 0.043 is the Fresnel reflection albedo for sun and sky Gordon et al. (1988).
Finally, we have:
For this study, data acquired during ascent and surface “buoy” modes and from both arms are used, allowing for the estimation of four remote sensing reflectance spectra per profile, noted and with i∈(1, 2), respectively for ascent and surface measurements and for arms one and two, respectively. While the seven radiance channels are redundant, only six irradiance channels are available on both arms to accommodate the PAR measurement (Table 1). The first arm does not measure Ed(412) whereas Ed(380) is missing on the second. To overcome this difficulty and benefit from Rrs spectra with seven wavelengths for both arms, the missing irradiance channel is replaced by the value measured on the other arm. Thus, Rrs spectra of the first arm use Ed(412) measured by the second arm and similarly with Ed(380) for the Rrs spectra of the second arm. This processing should have a negligible impact due to the weak impact of shading on irradiance sensors.
Shading Estimation
An existing body of literature on shading estimation and correction (Gordon and Ding, 1992; Zibordi et al., 1999; Leathers et al., 2004; Piskozub, 2004) emphasizes the leading role of absorption. As in situ measurements of absorption and other optical properties are seldom available in conjunction with radiometry measurements, absorption and scattering coefficients derived from radiometric measurements (Morel and Maritorena, 2001) may need to be employed for applying shading corrections with associated uncertainties. ProVal floats are designed to minimize shading, and therefore correction uncertainties, by spacing the sensors away from the main float axis. In addition to shading reduction, this configuration allows for at least one sensor to be at the well-lit side of the float. To evaluate the performance of the design in terms of shading avoidance, a 3D Monte Carlo code, named SimulO, was used. This code has been previously used to evaluate the performance of optical instrumentations (Leymarie et al., 2010; Babin et al., 2012; Doxaran et al., 2016) but can also serve to perform shading estimation (Gerbi et al., 2016) based on a 3D backward Monte Carlo approach (see section E in the Supplementary Material for details). In this study, a direct sun in a black sky has been used to simulate sky radiances. This is a first acceptable approximation for evaluating shading (Leathers et al., 2004; Gerbi et al., 2016) even if this leads to an overestimation of the true shading effect when the float is shading the sensor and an underestimation on the opposite direction. Simulations for the Lu sensor only are presented because shading issues on Ed measurements are much weaker (Not shown). Inelastic scattering is not modeled within SimulO while these processes could have a non-negligible impact especially in the red (Li et al., 2016).
The simulated shading over one Lu sensor at 2 m depth, for sun zenith angles of 30, 45 and 60 degrees, and IOPs (including the volume scattering function) derived for a Chla concentration of 0.1 μg.l−1 (Morel and Maritorena, 2001) is shown on Figure 3. The shading varies from 8% in the blue to 65% in the red, when the instrument is in the area of maximum shading by the float (azimuth 90° ± 15°), whereas it is <1% in the blue and up to only 6% at 665 nm for other azimuth angles.
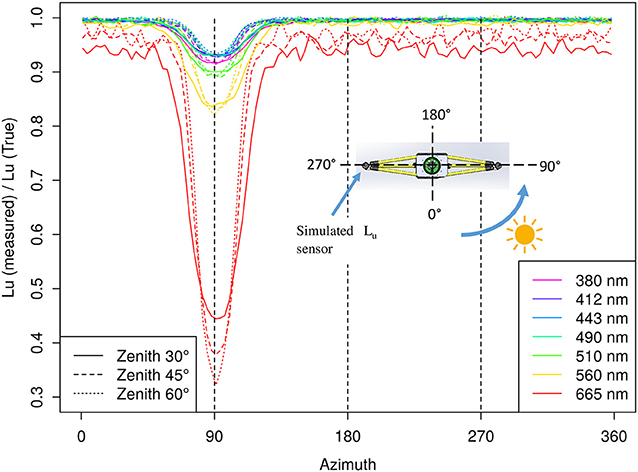
Figure 3. Shading estimated by Monte Carlo simulations expressed as ratio between measured and true Lu. Simulation realized at 2 m depth with sun zenith angles of 30°, 45°, and 60° in a black sky. IOPs derived from [Chla] = 0.1 μg/L. Azimuth of 90° corresponds to the maximum shade of the float over the simulated Lu sensor.
While these results are interesting to estimate the benefit, in terms of shading mitigation, of the two-arm design, it is not straightforward to use this approach for correcting data. Different aspects have to be carefully quantified such as the variation of the shading with depth or the impact of the sky radiance distribution which is different for each profile. In addition, the azimuth speed of rotation of the float could be fast, around 10° per ascending meter (not shown), making correction even more difficult. Consequently, no shading correction is applied in this study as our main focus is the platform design.
Assessing Radiometric Quality Degradation
The two-arm configuration enables the drift of one sensor relative to another to be estimated. To do so, the sensor Unbiased Percent Difference (UPD) is calculated for all measurements below 10 m depth for irradiances and radiances by using equation 9, where Si represents the radiometric quantities to compare from arms one and two.
The upper threshold of 10 m was chosen to limit relative differences at the surface due to wave focusing effects and only values above 0.1μWcm−2nm−1 for irradiance and 10−3μWcm−2nm−1sr−1 for radiance were retained to avoid differences dominated by dark current issues.
In addition, in regions close enough to shore and with ships of opportunity, it is possible to recover a float at sea, thanks to the two-way communication of Iridium telemetry providing the ability to instruct a float to stay at the surface and obtain its GPS position frequently. This is particularly feasible over the whole Mediterranean Sea (Taillandier et al., 2017) and circulation models could be used in other regions to determine deployment locations where the probability of recovery is high. These recoveries allow for data quality to be estimated over a deployment, for possible biofouling to be evaluated and for post-deployment calibrations to be performed, possibly before and after cleaning of the instruments. Two methods are proposed here to assess radiometric performance after recovery: One referred to as the “bucket inter-comparison” and another, more traditional, post-deployment calibration.
For the “bucket inter-comparison,” radiometers are immediately unmounted after float recovery and placed without any cleaning in a bucket filled with sea water and stored on board in a cold and dark place. Less than 24 h later, a third irradiance sensor, used as a reference, is immersed into the same bucket with the other radiometers and placed outside in an area with an unobstructed sky view. The three irradiance sensors are maintained vertically with their collectors just beneath the water surface. Irradiance measurements are collected simultaneously during 1 min. This experiment favors a comparison of irradiance sensors after deployment and recovery with, in a first approximation, a preservation of the state of the possible biofouling film over the collector. For post-deployment calibration, sensors are left to gently desalt in miliQ water (frequently replaced) for several days. Then sensors are left to dry out and no mechanical action is carried out on the optical elements. Following the methodology described in Hooker et al. (2002a), a new calibration of dry sensors is performed at the radiometric laboratory of the Laboratoire d'Océanographe de Villefranche (LOV) and compared to the calibration performed before deployment with the same protocols.
OLCI Remote Sensing Reflectance
OLCI (Ocean and Land Color Instrument) is the new European ocean color imager launched on February 16th, 2016 aboard the Sentinel-3A satellite. It was designed to continue the ENVISAT MERIS data sampling with increased capabilities (in particular more wavebands and global full resolution coverage). The deployment of ProVal floats during the commissioning phase of the OLCI instrument is a unique opportunity to demonstrate the capabilities of such platforms. OLCI remote sensing reflectance were provided by the Sentinel-3 Mission Performance Center (led by ACRI-ST). Macropixels centered on the ProVal position at the surface were provided using the v2.23 processing version including SVC and subsequently filtered using level-2 products flags (Sentinel-3A Product Notice–OLCI Level-2 Ocean Colour, 2018). In the Southern Ocean, where 70% of our profiles took place, acquisition geometry and atmospheric conditions are known to be particularly complex. Consequently, to maximize the number of match-ups for this area, two aspects were relaxed. First of all, the list of flags used for this validation exercise was not as strict as what is usually recommended by the user community. The following flags were ignored: HiSolZen, OC4Me_Fail, ANNOT_TAU06, Annot_Drout and Annot_MixR1. While the first three flags were not relevant in our case, the last two flags were raised frequently for our Southern Ocean data (20% for Annot_MixR1 and 12% for Annot_Drout). But most notably, the size of the macropixel has been increased from 5*5 (usually used for validation) to 19*19 (5.7*5.7 km2). A minimum of 18 valid (i.e. not flagged) pixels out of the 361 pixels in a macropixel were required to retain the OLCI match-up and a mean of these pixels were used for comparison with the ProVal measurements. With this constraint we managed to obtain seven match-ups in the Southern Ocean (see section Match-up Analysis). For the consistency, the same constraint was applied for the Mediterranean Sea data resulting in 25 match-ups. 22 out of the 25 match-ups in the Mediterranean meet the recommended flags and constraints for validation including smaller macropixel and higher proportion of valid pixels.
Results
Test Deployments
ProVal prototypes were tested in the NW Mediterranean Sea, close to the BOUSSOLE mooring. Floats were recovered after several weeks of operation. The first operational and long-term deployments were then carried out in the framework of the SOCLIM project (doi: 10.17600/16003300) in the Indian sector of Southern Ocean, in the vicinity of the Kerguelen plateau. Information concerning the deployments is summarized in Table 3 and trajectories are depicted in Figure 4.
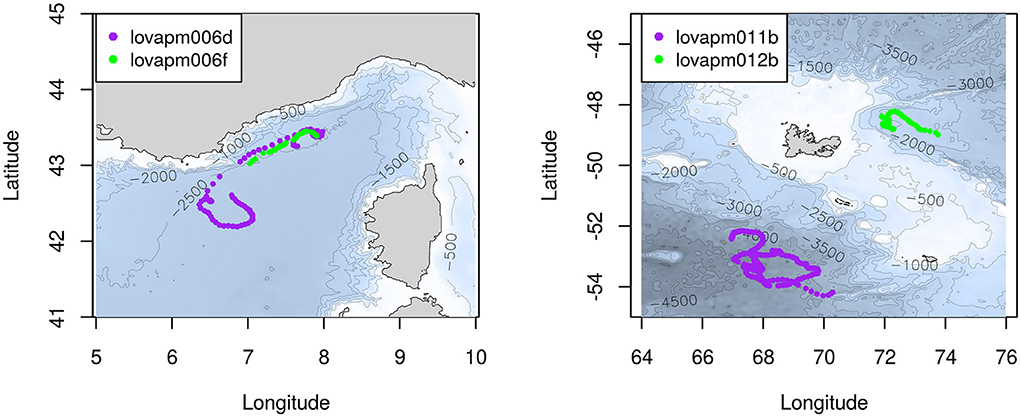
Figure 4. Maps and float trajectories of the deployments in the NW Mediterranean Sea (left) and Southern Ocean (right).
Navigation Behavior
ProVal floats are equipped with pressure, tilt and compass sensors that record their motion.
Figure 5A illustrates the percentage of data with a tilt below 5° and 10° as a function of depth for the Mediterranean and Southern Ocean floats, respectively. Unsurprisingly, the tilt is lower in the calmer Mediterranean Sea than in Southern Ocean. In this latter region, characterized by rough seas, the effect of the state of the sea surface on the vertical orientation of the float only becomes visible at a depth of about 20 m but remains acceptable toward the surface with about 40% of data points with a tilt below the 10°requirement. These values are notably higher for profiles used for OLCI match-ups (see section Match-up Analysis) for which weather conditions are assumed to be better (see section B in the Supplementary Material for details). In the Mediterranean Sea about 85% of the data points have a tilt below 10° and 55% below 5° at the surface. This latest value could be compared to the tilt of one of the most recent manual freefall profiler, the C-OPS (Biospherical Instrument Inc.), for which the tilt is estimated to be “regularly <5° from the vertical” (Morrow et al., 2010). For one deployment at the BOUSSOLE site, we take advantage of a meteorological mooring (Bouée Côte d'Azur, Météo-France) close to BOUSSOLE to study the behavior of the float in different weather conditions. Figure 5B shows the median value of the tilt in the upper five meters as a function of significant wave height (H1/3) measured by a wave motion sensor on the meteorological mooring. The relationship is linear with a slope of 2° per meter.
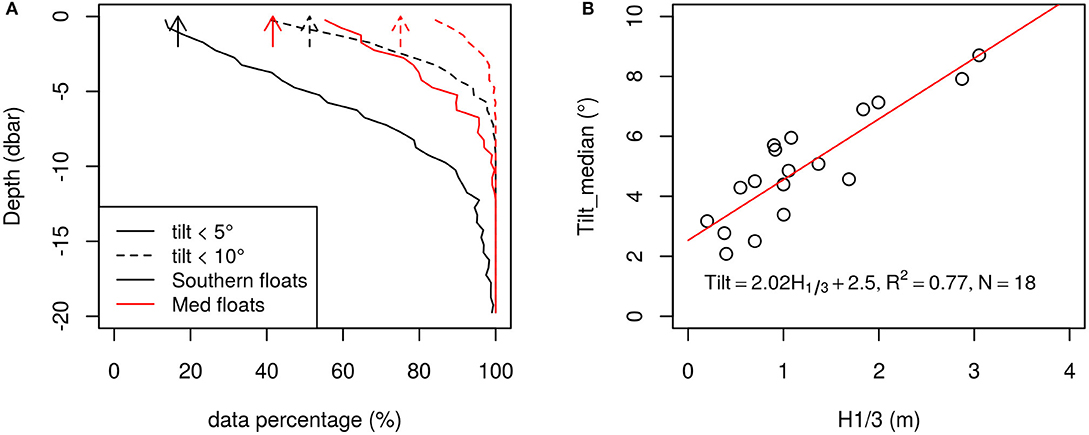
Figure 5. (A) Percentage of the data point below 5° (solid lines) and 10° (dashed lines) as a function of depth for the Southern Ocean (black) and the Mediterranean Sea (red). Arrows represent values during “buoy” mode. (B) median of the tilt within the shallowest 5 m as a function of waves height derived from a meteorological mooring (Mediterranean Sea only, float lovapm006d).
The profile of the median ascending speed is illustrated in Figure 6 for both Mediterranean Sea and Southern Ocean floats. Ascension speed in the Southern Ocean is stable in deep water, varying around 9 cm s−1 and progressively decreases to 4.5 cm s−1 above 15 m depth. In the Mediterranean Sea, comparable velocities are observed at depth (8.5 cm s−1) but there is a significant decrease in the velocity near the surface, with a local minimum at 10 m depth. This effect is likely due to density stratification with a steep gradient at ~20 m in the Mediterranean Sea during summer compared to a 100 m mixed layer in the Southern Ocean (data not shown). Once again, it is possible to compare these results to the C-OPS instrument characteristics for which velocities are about 3 cm s−1 near the surface, 12 cm s−1 at 1 m, and 25 cm s−1 below 5 m depth (Morrow et al., 2010). In all cases, the ProVal float travels more slowly, except perhaps when very near the surface and if the C-OPS is well balanced. This slow speed allows the float to collect a higher density of data if both platforms carried the same radiometer and if the electronics and telemetry of the float enabled the acquisition and transfer of these data.
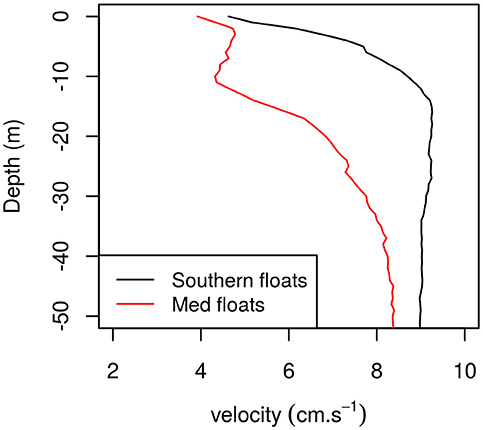
Figure 6. Median profile of ascent velocity for the Mediterranean Sea (red) and Southern Ocean (black) floats. Only shallowest 50 m are shown as velocity at depth is stable.
With the ProVal float, a surfacing time can be scheduled with a theoretical precision of 1 min. In practice, the reproducibility of the time of surfacing was found to be <5 min which is well within the ± 3 h requirement for a good satellite match-up in the open ocean (Mazeran, 2017). Several profiles in a same day can be performed with this float, although it takes about 3 h to perform an additional 100 m profile. This duration could be reduced by disabling the Iridium and GPS sessions of the first profile (data transmission, including emerging and diving, takes about 45 min).
Sensor in situ Comparison and Drift
For autonomous platforms, and especially when no recovery is planned, degradation of the radiometric accuracy of the measurement over time is a major concern (see section ProVal Mission Requirements). Yearly radiometer calibrations are usually recommended by manufacturers. Although this is acceptable for instruments deployed aboard oceanographic ships (i.e., freefalling profilers that typically spend most of their time switched off inside their cover), it may not be the case for the more intensively used radiometers aboard profiling floats. Biofouling is another factor that could progressively degrade the quality of radiometers on board floats. Previous studies have demonstrated how biofouling development on float radiometers is mitigated by the fact that they spend most of their time in dark and cold waters (Gerbi et al., 2016; Organelli et al., 2016). Biofouling is also expected to affect irradiance sensors more rapidly than radiance sensors as falling particles might settle on the surface of the sensors and accelerate bacterial colonization and subsequently algal colonization. These in situ degradation processes can be assessed, to a certain degree, by comparison between redundant sensors.
In situ Sensor Comparison
The Sensor Unbiased Percent Difference (UPD) (section Assessing Radiometric Quality Degradation, Equation 9 and depth constraints) at five wavelengths for irradiance and seven wavelengths for radiance are shown on Figure 7 as a function of time, for the entire deployment (480 days) of the float in the Southern Ocean (lovapm011b).
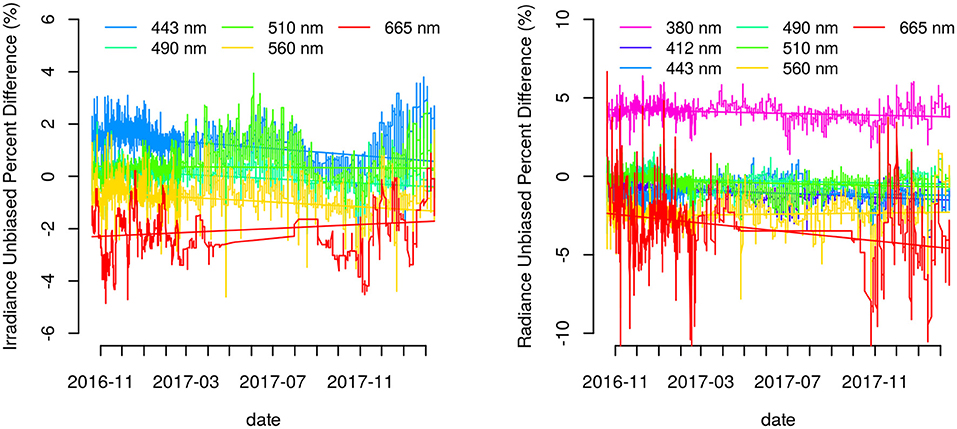
Figure 7. Running median of the Unbiased Percent Difference for Irradiance (left) and Radiance (Right) for the lovapm011b float over 480 days and 247 profiles. The float profiling cycle was daily at the beginning, then every three days starting in early 3/2017. Linear trends are shown.
UPD Values at the deployment (intercept), slopes (i.e., instrument drift) of the linear regressions vs. time and UPD at the end of the mission are reported in Table 4. Two channels, Ed(665) and Lu(380) show significant UPD since the deployment, with values of−2.4 and 4.3%, respectively. These differences are still within the absolute calibration uncertainties which are estimated around ±3 to ±5% (Hooker et al., 2002a). This is especially true for radiance at 380 nm for which the calibration uncertainty is higher due to the weak irradiance from calibrated FEL lamps in the blue.
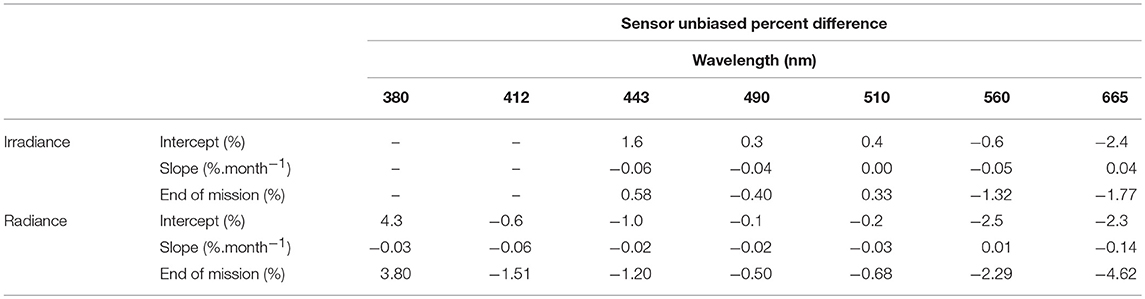
Table 4. Intercept (initial values), slope and final values of all UPD over 480 days (float lovapm011b).
While intercept at deployment are essentially related to absolute calibration uncertainties, the slope describing the variation of the UPD with time is related to a difference in the drift of the sensors. The slope remains below 0.05% per month for most wavelengths whereas it is about −0.15% per month for Lu(665). This implies that assuming no systematic simultaneous drift of the two sensors (for example due to an homogenuous biofouling developing on both sensors), relative degradation of the sensors within a year is comparable to the initial absolute calibration uncertainty at all wavelengths and will therefore introduce a comparable source of uncertainty.
Post Cruise Calibration
After two months in the Mediterranean Sea, the float lovapm006d was recovered. Neither biofouling nor degradation were visible on the float nor on the sensors (Figure 8). The experiments described in section Assessing Radiometric Quality Degradation to assess radiometric performance after recovery were carried out. Figure 9A illustrates the relative difference between irradiances measured by sensors used on the lovapm006d float (SN 279 and 280) and a reference sensor (SN220) during the so called “bucket inter-comparison.” Unfortunately, as irradiance at 380 nm was missing on the reference sensor, comparison began at 412 nm. Results present an average absolute difference of 4% with a minimum at −7%. Nevertheless, in this case, it is difficult to quantify to which extent the observed differences might be due to inhomogeneity of the local radiance field generated by the experiment itself.
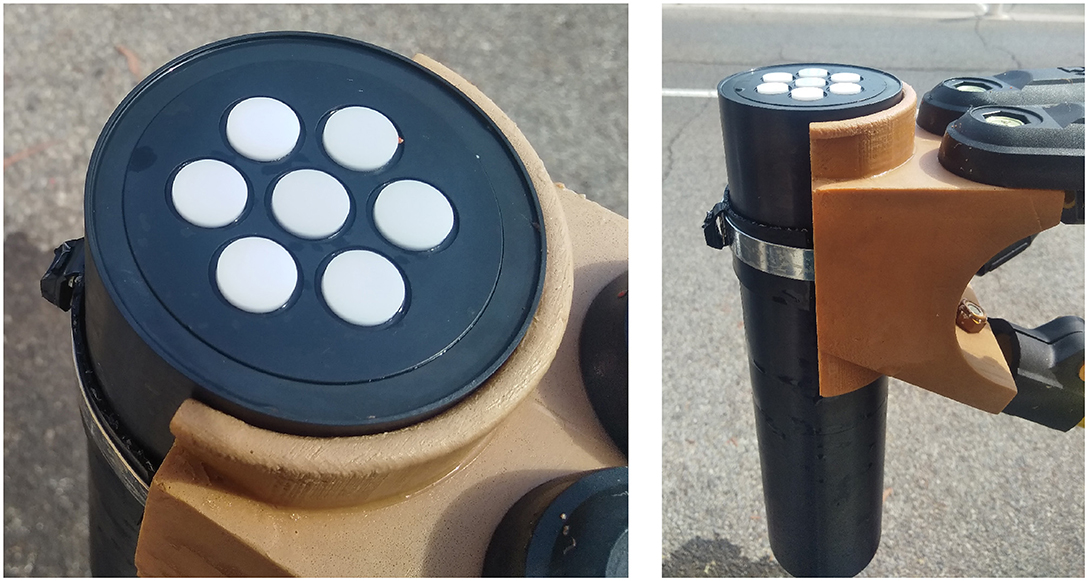
Figure 8. Pictures of the sensor just after the recovery and without any cleaning after 2 months at sea in summer in the Mediterranean Sea.
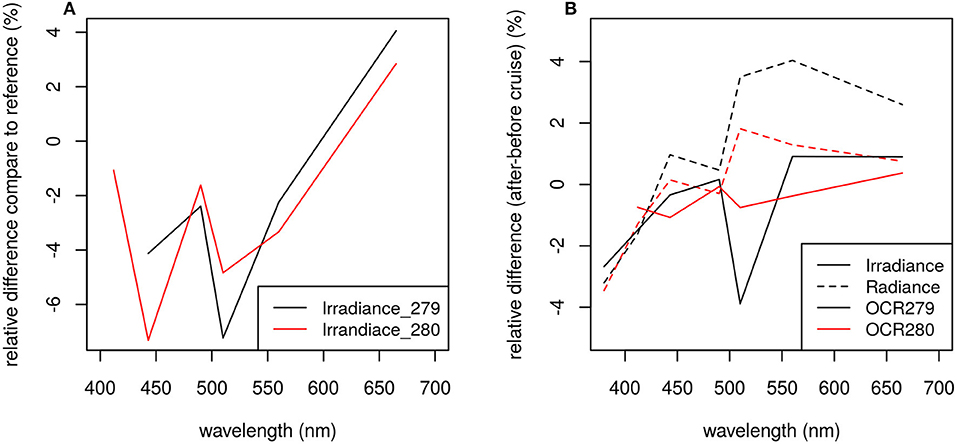
Figure 9. (A) Relative difference of irradiance from OCR SN 279 and 280 used on lovapm006d float with respect to a reference sensor (bucket inter-comparison). (B) Relative difference of calibration coefficients determined at the LOV calibration laboratory before and after deployment.
Differences between the two post cruise calibrations are illustrated on Figure 9B for radiance and irradiance of both float sensors. Results point to an average difference close to zero with maxima of ± 4% which is globally within the expected uncertainties for this kind of experiment (Hooker et al., 2002a). To conclude, following the two experiments described in Figure 9, the possible degradation of the data quality cannot be distinguished from the uncertainties of these experiments.
In situ Shading Estimation
In this section, the UPD, as defined in equation 9, is employed to estimate the shading effect for the float lovapm006f deployed in summer 2017 in the Mediterranean Sea. The UPD was calculated for radiance measurements taken close to the surface (depth <10 m), where the shading effect is expected to be greater than at depth, for 31 profiles selected over a total of 81 collected during the deployment period. Selection was based on the measured PAR at surface which must be between 85 and 100% of the theoretical PAR (Bird and Riordan, 1986) to retain only sunny profiles. In this section, UPD was not calculated from individual measurements but from the local polynomial regression fitting of the radiance as computed in section Ascent and Surface Processing to remove the fast fluctuations of the radiant field and specifically address the trend of the average radiance close to the surface. These data are presented in Figure 10 as a function of the solar azimuth angle measured by the embedded compass. They originate from 31 profiles distributed over 100 days of deployment and are by chance very well distributed over the azimuth axis even though the first peak (at 90°) is sampled during the second half of the deployment while the second peak (at 270°) is sampled mostly at the beginning (Figure 10, last panel). As pointed out in section Basic Processing, an offset is simply added to the compass heading output in order to obtain the maximum of UPD for a sun's azimuth of 90° (same definition of azimuth than in Figure 3).
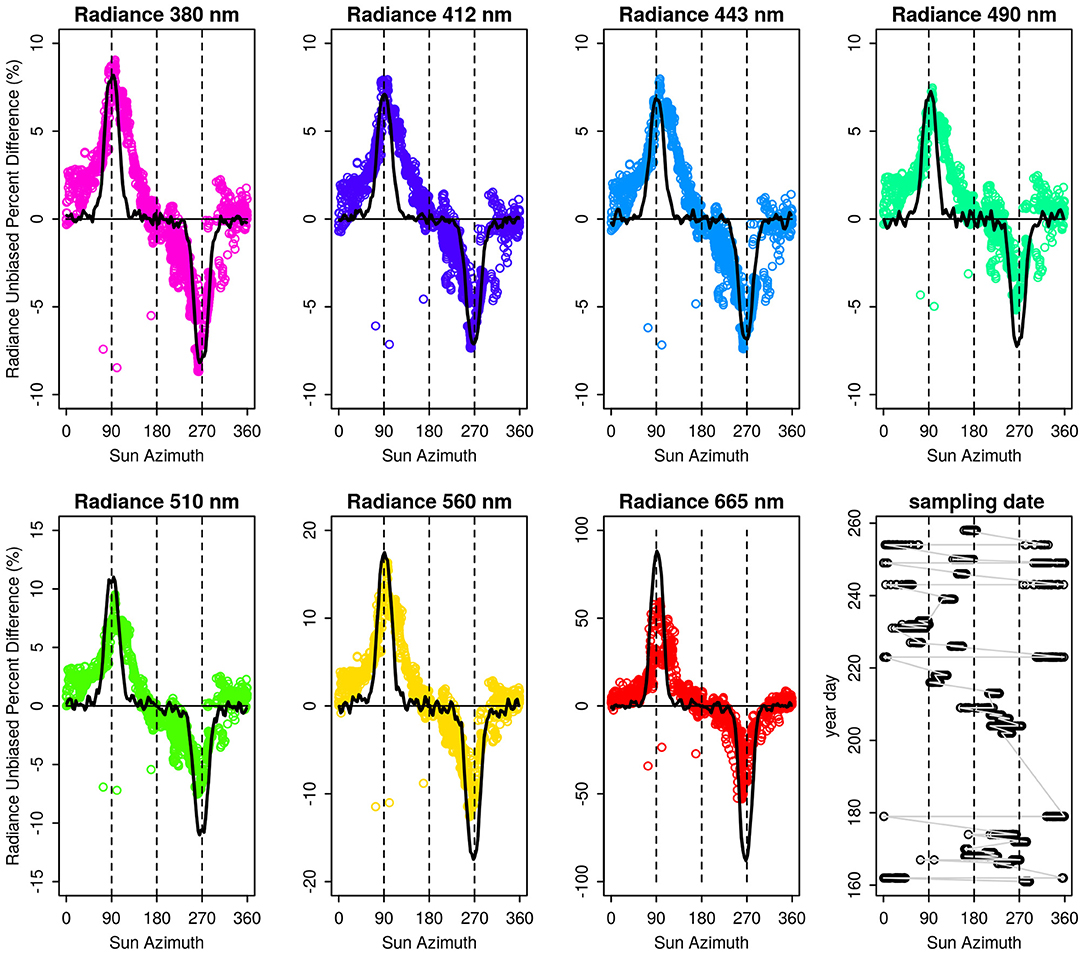
Figure 10. UPD calculated from local polynomial regression fitting of radiance data measured above 10 m depth for 31 profiles of the float lovapm006f as a function of the solar azimuth angle. The black line represents the theoretical effect of shading estimated by the SimulO software at 2 m depth and for a sun zenith angle of 45 degrees. See Figure 3 for sun azimuth definition. Bottom right, day of the acquisition for each sun azimuth angles.
At all wavelengths, the variation of the UPD is clearly linked to the sun azimuth angle with a change in the sign of the UPD and a clear symmetry around an azimuth of 180°. Theoretical UPD values are processed according to self-shading estimated by Monte Carlo at 2 m depth and for a sun zenith angle of 45 degrees (see section Shading Estimation) and plotted in black on Figure 10. A good agreement, especially at short wavelengths, is observed between modeled and in situ UPD, which gives confidence in the self-shading modeling. Nevertheless, in situ UPD peaks are found to be wider than the ones obtained by modeling. This difference is likely due to the fact that our simulation ignore skylight. In practice, diffuse sky radiance around the direct sun beam should increase the range of azimuth angles impacted by shading while decreasing shading when directly in the float's shade. Self-shading might also be overestimated by the model at 665 nm. This is likely due to inelastic scattering (Raman, chlorophyll fluorescence) which has a stronger impact at the highest wavelengths (Li et al., 2016).
In situ Remote Sensing Reflectance Comparison
Uncertainties in the evaluation of in situ remote sensing reflectance arise from many sources and are partly related to the data processing procedure, which is not the focus of this work. The ProVal setup and acquisition scheme allow for four Rrs spectra to be derived from the two arms and from ascent and surface phases. A comparison of these measurements provides inputs to a first attempt to estimate the uncertainties of the ProVal measurements. For each profile p, we note the mean value of the four Rrs spectra obtained for this profile.
Firstly, to compare Rrs from the two acquisition modes, the average normalized difference between ascent and surface measurements (ΔRn(λ)) is defined for N profiles:
Secondly, to quantify the general agreement between the four values, the standard deviation (σ(λ)) of Rrs values normalized to the average value is estimated:
Results from these statistical descriptors are provided in Table 5 for all profiles (i.e., without any profile selection) from floats in Mediterranean Sea (lovapm006f) and in the Southern Ocean (lovapm011b).
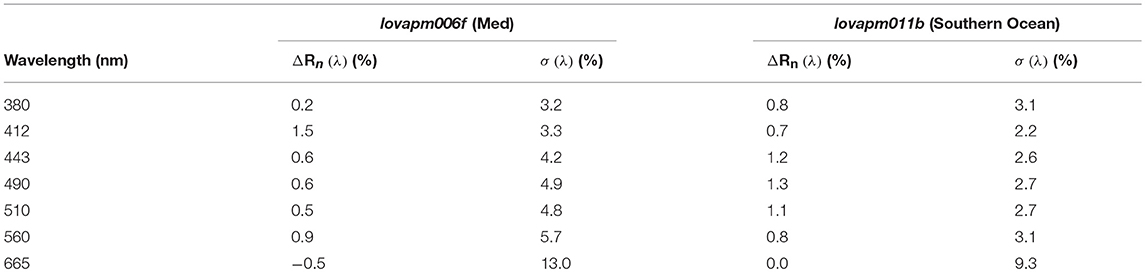
Table 5. Average of the normalized Rrs difference and standard deviation of Rrs normalized values for all profiles of floats lovapm006f and lovapm011b.
According to the average normalized difference ΔRn, Rrs values from surface measurements are in average, and for both floats, always slightly larger (around 1%) than ascent values, except at 665 nm for the Mediterranean float (−0.5%). Standard deviations are larger in the red and for the Mediterranean float. This suggests that dispersion of Rrs values is probably dominated by shading effects and associated Raman, which are more important in the red and under clear skies. To go a step further in this hypothesis, we assume that downward irradiance is weakly impacted by shading. In this case, the normalized variation of the remote sensing reflectance should be equal to the normalized variation of the water-leaving radiance. Then, estimation of the standard deviation of the Radiance Unbiased Percent Difference (UPD) data as used in Figure 10 to estimate in situ shading provides values around 4% for the lowest wavelengths, 5.7% for 560 nm and 17% for 665 nm which is in a good agreement with values found in Table 5 for the Mediterranean float. A careful selection of profiles and a good use of the double-arm configuration to reduce shading effects is likely to minimize this dispersion.
Match-Up Analysis
A match-up is defined as a concomitant and collocated occurrence of measurements from a satellite-borne sensor and an in situ device (Mazeran, 2017). Both measurements should pass their own Quality Control procedure. Here, the quality control on OLCI data is defined in section OLCI Remote Sensing Reflectance. The concomitance of OLCI and ProVal measurements was, in average, within 25 min (max 1 h 10 min). For the comparison presented below, both OCLI and ProVal reflectance data were fully normalized (i.e., corrected for bi-directionality) as defined by Morel et al. (2002) using Chla concentrations estimated from reflectance spectra. In the Mediterranean Sea, 25 valid OLCI measurements were retained over the 81 days of possible match-ups, i.e., a data availability of 31%. In the Southern Ocean, during the operating window and with 2 floats, a total of 254 days of OLCI images were collected, of which only 7 passed the quality control (data availability of 2.7%). For these 32 ProVal profiles collected in both areas, verifications were made that radiometric data were not affected by passing cloud shadows in the upper 25 meters, and that the polynomial fit, as described in section Ascent and Surface Processing, visually retrieved the correct surface value. In the present case, 30 ProVal profiles were retained for the comparison (one rejected in both area) showing that OLCI flags (including cloud and White Caps) provided a good indication of their quality. Corresponding irradiance and radiance profiles are shown in Supplementary Material (section C). The number of match-ups for the Southern Ocean, 6, for 254 available satellite overpasses may appear low. However, as a comparison, the match-up analyses for the whole Southern Ocean (> 40°S) over the full MERIS archive (10 years) using the MERMAID data base (http://mermaid.acri.fr) on similar criteria (5 x 5 macropixel, i.e., 36 km2, equivalent flags and rejection conditions), resulted in only 8 match-ups.
Normalized remote sensing reflectance (ρwN) spectra from OLCI, processed according to section OLCI Remote Sensing Reflectance, were compared to the median of the four spectra provided by a ProVal float for each profile while the minimum and maximum values were used for providing an uncertainty estimate. Results are presented in Figure 11 for the 30 match-ups obtained in both the Southern Ocean and the Mediterranean Sea. No significant bias was observed between the in situ and satellite data, with a linear regression slope of 0.968 (± 0.015) for all gathered wavelengths. The median Relative Percent Difference (RPD) and the median Absolute Percent Difference (APD) were calculated using in situ data as reference values. RPD varies between −2 and −7% except for 510 and 665 nm (−12 and 12% respectively) whereas the APD varies between 9 and 15% except for the 665 nm band (25%). A regional difference is found for the slope value with 0.93 and 1.11 for the Mediterranean Sea and the Southern Ocean, respectively. See section D in the Supplementary Material for details.
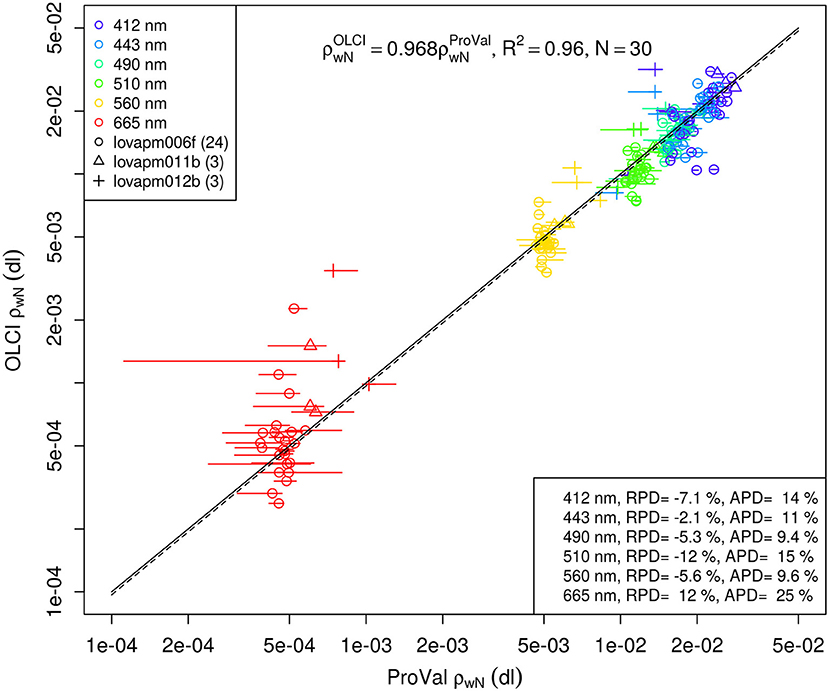
Figure 11. Normalized reflectance ρwN from OLCI and ProVals for both Mediterranean (lovapm006f) and Southern Ocean floats (lovapm011b and lovapm012b). Error bars on the ProVal data are given by the minimum and maximum of the four normalized reflectance values. The solid line represents the 1:1 line while the dashed line is the linear regression. The number of match-ups for each float are given in brackets in the top-left legend.
Discussion
The ProVal float was designed to respond to the need of a “Val float” as defined by the IOCCG (IOCCG, 2011) and follows first attempts by Gerbi et al. (2016) and Wojtasiewicz et al. (2018). It has now become part of a more general effort to investigate new approaches to SVC which include also the Sea-Bird HYPERNAV project (A. Barnard, Sea-Bird Scientific, personal communication). While the benefits of a float-based approach for “Val” activities have been well demonstrated, the question of the use of these platforms for “Cal” activities has not received much attention. Results from this study and from previous works (Gerbi et al., 2016; Wojtasiewicz et al., 2018) and published requirements for SVC (Bailey et al., 2008; Zibordi et al., 2015; Mazeran, 2017) could be used to review the advantages and disadvantages of floats for “Cal” activities.
This study demonstrates that a profiling float can measure radiometric quantities in a very efficient way with favorable tilt and ascent speed values, with mitigation of shading effects, with redundant sensors, and suffering relatively (to calibration uncertainties) low drift in time. In addition, highly resolved vertical data can be collected all the way to the surface; data collection in surface “buoy” mode, with upwelling radiance sensors situated a few cm from the surface, also minimizes uncertainties in extrapolation to the surface especially in a context of inelastic scattering (Li et al., 2016). As the float spends most of its time in cold dark waters under high hydrostatic pressures, the good quality of the data is maintained during several months thanks to the absence of visible biofouling effects. Moreover, this quality can even be monitored at sea based on the sensor redundancy and by recovering the float after deployment. Float recovery has become feasible with the availability of the two-way iridium telemetry and the use of circulation models that can help determine the deployment positions which optimize the probability of retrieval. Such recovery has now been organized for BGC-floats over the entire Mediterranean Sea (Taillandier et al., 2017) and has also been performed near Hawaii (HYPERNAV team). The recovery and subsequent post-calibration, ensure that sensor drift can be constrained, a necessary input for SVC.
Site information and ancillary measurements are required in addition to in-water radiometry for “Cal” activities. Especially, the knowledge of the atmospheric composition at the site is essential and it is challenging to imagine in situ and concomitant atmospheric measurements for autonomous floats. However, although they can be deployed in remote regions characterized by some of the most pristine atmospheres, space-born LIDARs (e.g., CALIOP) and radiometers, can also be used for characterizing the state of the atmosphere on a global basis (e.g., amounts and types of aerosols). The small size of the profiling floats, the absence of a fixed surface structure, and the possibility of deployment far offshore, all minimize the identified issues such as the attraction of fish, boaters, and fishermen (and associated issues) which generally introduce optical artifacts for fixed moorings (the artificial reef effect).
The lack of above surface downward irradiance measurements (Es) at the same spectral resolution as the radiance (Lu) measurements and over the whole profile still needs to be discussed. This data is not used for SVC but is useful for estimating the cloud cover and sky clarity for data quality control. In addition to information from the OCR satellite itself, short time-scale modifications of the cloud cover is readily apparent in the vertical profile of radiometry (Organelli et al., 2016) as well as in the PAR value at the surface especially during the “buoy” mode of the float.
We find that the ProVal float meets at least 7 of the eleven “Current Recommended Requirements on Sea-Truth Data for Vicarious Calibration Activities” published by Bailey et al. (2008, Table 1), (“clear maritime atmosphere,” “clear-water site,” “horizontally homogeneous water mass,” “daily-to-weekly monitoring of derived Lwn,” “avoidance of platform perturbation,” “cloud-free site,” “atmosphere free of terrestrial influence”) and partially to the requirement “free from biofouling.” Two additional requirements “hyperspectral instrumentation” and “extraordinary calibration” are met by the HYPERNAV float and could be met by the ProVal float in the near future. The only problematic requirement not fulfilled is “coincident aerosol measurements” which is also not fulfilled by the MOBY mooring and for which atmospheric remote-sensing could potentially be used. In fact, ProVal floats meet these requirements in a very similar way to freefall profilers which already demonstrated their capabilities for SVC as part of the NOMAD data set (Bailey et al., 2008; Zibordi et al., 2015).
Autonomous floats have the ability to be deployed in many regions, thus decreasing potential systematic biases associated with having the SVC assets deployed in one region and vastly increasing the degrees of freedom in developing robust statistical predictors. The use of several floats during the commissioning phase of a new OCR sensor has a clear advantage for producing the sufficient number of match-ups needed to achieve accurate and stable g-factors within a relatively short time frame. The use of multiple platforms in a SVC framework involves a rigorous management of the system, i.e., sensors and platforms, with knowledge and traceability of uncertainties. For this latter concern, the legacy of Argo and BGC-Argo programs, which both provide a high level of data quality control and assurance, demonstrate that such management is feasible and may provide a SVC data base characterized by high consistency and fidelity to international standards.
Platform improvements are possible and probably necessary in the future. Radiometers with higher spectral resolution and sampling frequency should increase the utility and data quality of ProVal floats. Two developments should be necessary to manage the large amount of data resulting from these improvements. New electronic boards with enhanced embedded CPUs will have to be used for advanced on-board data treatment and reduction. The amount of data transmitted to shore will also be increased in the very near future by the implementation of the new IRIDIUM NEXT® constellation which promises faster communication for the benefit of all Argo float applications including cal/val floats. Nevertheless, the biggest effort necessary to demonstrate the utility of floats for SVC, is to provide a precise uncertainty budget which take all the specificities of these platforms into account.
Summary and Conclusions
The ProVal float was designed to respond to the need of a “validation float” as defined by the (IOCCG, 2011). Its conception was guided by three major requirements (see section ProVal Mission Requirements). The first one is to provide yearlong accurate and precise radiometric data autonomously in the open ocean. The 14 months of a ProVal float mission deployed in the Southern Ocean have demonstrated this capability. The second requirement was to create a platform providing radiometric data similar to or of better quality than traditional freefall profilers. This is supported by several of our results. Regarding navigation behavior, a ProVal float shows a tilt that is mostly within the recommended range (<10°) even in cases of rough sea states when it is not possible to deploy radiometers from research vessels. The velocity of the float is often slower and more stable than that of freefall radiometer systems. This advantage could be used to increase the number of samples per meter if higher sampling frequency can be achieved. Data quality is also related to the degradation and drift of radiometers. After 2 months in the Mediterranean Sea a significant drift of the sensors could not be detected and after 1 year in the Southern Ocean, the relative differences between the two redundant sensors were lower than calibration uncertainties. Moreover, there are now sufficient examples of float recovery after deployment, so that post-calibration of radiometers can be done if deemed necessary. Thus, one of the principal objections to the use of autonomous platforms for SVC, e.g., the lack of post-cruise calibration (Mazeran, 2017), can be mitigated. Recovered floats should also help to estimate the uncertainties in radiometric data for the unrecovered floats deployed in remote areas. Shading is also relevant for the quality of radiometric data. The two-arm configuration of the ProVal minimizes shading thanks to its design; this allows for at least one arm to be out of the shade of the float hull and provides an in situ estimation of shading by comparing both arms. Our last requirement was to deliver in almost real time final radiometric products for ocean color data validation. Results presented in this study clearly demonstrate this capability.
Autonomous floats have proven to provide routine validation-quality radiometric data, although dedicated floats such as ProVal or HYPERNAV, may in a near future even provide routine calibration-quality radiometric data. They can be operated for at least 1 year in remote areas that are currently poorly covered by oceanographic cruises. As an example, the same number of match-ups has been obtained in the Southern Ocean in 1 year with ProVal floats as during the 10 years of activity of MERIS validation efforts. Deployments in many regions insure that possible regional biases (in the optics of atmosphere, ocean or both) do not bias measurements worldwide. Lastly, ProVal floats could also be used in synergy with permanent moorings such as BOUSSOLE, or in conjunction with research cruises, to explore spatial heterogeneity or increase sampling capabilities.
Author Contributions
EL developed the general ProVal concept with inputs from all authors. EL finalized the mechanical design and CP realized the adaptation of the embedded software to host ProVal's sensors. CP, EL, and VV carried out field tests and operational deployments. EL processed float data with inputs from all authors. CL processed OLCI data and performed the normalization of ProVal reflectances. All authors provided feedback on data quality and their interpretation. EB and ML provided arguments for the discussion section. EL wrote the draft. All authors contributed to the writing of the manuscript.
Funding
The authors acknowledge spatial agencies for funding: CNES (TOSCA) for the initial funding, ESA, and NASA. Following projects also provide support and funding: NAOS (Investissements d'avenir ANR-10-EQPX-40), SOCLIM (BNP Paribas Foundation, DOI:10.17600/16003300), remOcean (ERC advanced grant N°246777), BOUSSOLE (CNES TOSCA and ESA/ESRIN/Contract N°4000119096/17/I-BG). Support of EB was provided by NASA OBB program (NNX14AP86G).
Conflict of Interest Statement
The authors declare that the collaboration between the Laboratoire d'Océanographe de Villefranche (LOV) and the manufacturer of the float NKE was conducted in the absence of any commercial or financial relationships that could be construed as a potential conflict of interest. The authors declare that the past affiliation of ML with Satlantic LP, does not preclude the scientific independence of this work which meets the standard of academic research. The authors did not receive any benefits from NKE nor Satlantic for the publication of this article. CL is employed by company ACRI-ST.
The remaining authors declare that the research was conducted in the absence of any commercial or financial relationships that could be construed as a potential conflict of interest.
Acknowledgments
The authors acknowledge Météo-France and HyMeX for supplying the Bouée côte d'Azur data. We thank all the administrative staff of the LOV and in particular Linda Féré, Corrine Poutier, Anne Silbermann and Isabelle Maire. We also thank the crew of R/V Sagitta III and Téthys II as well as Melek Golbol and Guillaume De Liège. Sabine Marty is kindly acknowledged for his help and expertise for the calibration of ProVal's radiometers. Finally, we are grateful to Joséphine Ras for proofreading and to the two external reviewers and the editor for providing constructive comments to the manuscript.
Supplementary Material
The Supplementary Material for this article can be found online at: https://www.frontiersin.org/articles/10.3389/fmars.2018.00437/full#supplementary-material
References
Antoine, D., d'Ortenzio, F., Hooker, S. B., Becu, G., Gentili, B., Tailliez, D., et al. (2008a). Assessment of uncertainty in the ocean reflectance determined by three satellite ocean color sensors (MERIS, SeaWiFS and MODIS-A) at an offshore site in the Mediterranean Sea (BOUSSOLE project). J. Geophys. Res. Oceans 113:C07013. doi: 10.1029/2007JC004472
Antoine, D., Guevel, P., Desté, J.-F., Bécu, G., Louis, F., Scott, A. J., et al. (2008b). The “BOUSSOLE” buoy—A new transparent-to-swell taut mooring dedicated to marine optics: Design, tests, and performance at sea. J. Atmospheric Ocean. Technol. 25, 968–989. doi: 10.1175/2007JTECHO563.1
Austin, R. W. (1974). “The remote sensing of spectral radiance from below the ocean surface,” in Optical Aspects of Oceanography, eds N. G. Jerlov and E. S. Neilsen (London; New York, NY: Academic Press), 317–344.
Babin, M., Stramski, D., Reynolds, R. A., Wright, V. M., and Leymarie, E. (2012). Determination of the volume scattering function of aqueous particle suspensions with a laboratory multi-angle light scattering instrument. Appl. Opt. 51, 3853–3873. doi: 10.1364/AO.51.003853
Bailey, S. W., Hooker, S. B., Antoine, D., Franz, B. A., and Werdell, P. J. (2008). Sources and assumptions for the vicarious calibration of ocean color satellite observations. Appl. Opt. 47, 2035–2045. doi: 10.1364/AO.47.002035
Biogeochemical-Argo Planning Group, (2016). The Scientific Rationale, Design and Implementation Plan for a Biogeochemical-Argo Float Array.
Bird, R., and Riordan, C. (1986). Simple solar spectral model for direct and diffuse irradiance on horizontal and tilted planes at the earths surface for cloudless atmospheres. J. Clim. Appl. Meteorol. 25, 87–97.
Boss, E., Swift, D., Taylor, L., Brickley, P., Zaneveld, R., Riser, S., et al. (2008). Observations of pigment and particle distributions in the western North Atlantic from an autonomous float and ocean color satellite. Limnol. Oceanogr. 53, 2112–2122. doi: 10.4319/lo.2008.53.5_part_2.2112
Clark, D. K., Yarbrough, M. A., Feinholz, M., Flora, S., Broenkow, W., Kim, Y. S., et al. (2003). MOBY, a radiometric buoy for performance monitoring and vicarious calibration of satellite ocean color sensors: measurement and data analysis protocols. Ocean Opt. Protoc. Satell. Ocean Color Sens. Valid. Revis. 4, 3–34.
Cleveland, W. S., Grosse, E., and Shyu, W. M. (1992). Local regression models. Stat. Models S 2, 309–376.
Doxaran, D., Leymarie, E., Nechad, B., Dogliotti, A., Ruddick, K., Gernez, P., et al. (2016). Improved correction methods for field measurements of particulate light backscattering in turbid waters. Opt. Express 24, 3615–3637. doi: 10.1364/OE.24.003615
Franz, B. A., Bailey, S. W., Werdell, P. J., and McClain, C. R. (2007). Sensor-independent approach to the vicarious calibration of satellite ocean color radiometry. Appl. Opt. 46, 5068–5082. doi: 10.1364/AO.46.005068
Gerbi, G. P., Boss, E., Werdell, P. J., Proctor, C. W., Haentjens, N., Lewis, M. R., et al. (2016). Validation of Ocean color remote sensing reflectance using autonomous floats. J. Atmosph. Ocean. Technol. 33, 2331–2352. doi: 10.1175/JTECH-D-16-0067.1
Gordon, H. (1987). Calibration requirements and methodology for remote sensors viewing the Ocean in the visible. Remote Sens. Environ. 22, 103–126. doi: 10.1016/0034-4257(87)90029-0
Gordon, H., and Ding, K. (1992). Self-shading of in-water optical-instruments. Limnol. Oceanogr. 37, 491–500. doi: 10.4319/lo.1992.37.3.0491
Gordon, H. R. (1998). In-orbit calibration strategy for ocean color sensors. Remote Sens. Environ. 63, 265–278. doi: 10.1016/S0034-4257(97)00163-6
Gordon, H. R., Brown, O. B., Evans, R. H., Brown, J. W., Smith, R. C., Baker, K. S., et al. (1988). A semianalytic radiance model of ocean color. J. Geophys. Res. Atmosph. 93, 10909–10924. doi: 10.1029/JD093iD09p10909
Gordon, H. R., and Clark, D. K. (1981). Clear water radiances for atmospheric correction of coastal zone color scanner imagery. Appl. Opt. 20, 4175–4180. doi: 10.1364/AO.20.004175
Gregg, W. W. (2007). Ocean-Colour Data Merging. Dartmouth, MA: International Ocean-Colour Coordinating Group.
Hooker, S. B., Firestone, E. R., McLean, S., Sherman, J., Small, M., Lazin, G., et al. (2002a). The Seventh SeaWiFS Intercalibration Round-Robin Experiment (SIRREX-7), March 1999. Available online at: https://ntrs.nasa.gov/search.jsp?R=20020045342
Hooker, S. B., Lazin, G., Zibordi, G., and McLean, S. (2002b). An evaluation of above- and in-water methods for determining water-leaving radiances. J. Atmospheric Ocean. Technol. 19, 486–515. doi: 10.1175/1520-0426(2002)019<0486:AEOAAI>2.0.CO;2
Hooker, S. B., and Maritorena, S. (2000). An evaluation of oceanographic radiometers and deployment methodologies. J. Atmospheric Ocean. Technol. 17, 811–830. doi: 10.1175/1520-0426(2000)017<0811:AEOORA>2.0.CO;2
Hovis, W. A., Clark, D. K., Anderson, F., Austin, R. W., Wilson, W. H., Baker, E. T., et al. (1980). Nimbus-7 coastal zone color scanner - system description and initial imagery. Science 210, 60–63. doi: 10.1126/science.210.4465.60
IOCCG (2004). “Guide to the creation and use of ocean-colour, level-3, binned data products,” in Reports of the International Ocean-Colour Coordinating Group, No. 4. ed. D. Antoine. Dartmouth, NS: IOCCG. Available online at: http://ioccg.org/wp-content/uploads/2015/10/ioccg-report-04.pdf
IOCCG (2011). “Bio-optical sensors on argo floats,” in Reports of the International Ocean-Colour Coordinating Group, No. 11. ed H. Claustre. Dartmouth, NS: IOCCG. Available online at: http://ioccg.org/wp-content/uploads/2015/10/ioccg-report-11.pdf
Kelley, D. (2017). Package ‘oce’, Analysis of Oceanographic Data. Available online at: https://cran.r-project.org/web/packages/oce/oce.pdf (Accessed November 7, 2017).
Kuusk, J. (2011). Dark signal temperature dependence correction method for miniature spectrometer modules. J. Sens. 2011:608157. doi: 10.1155/2011/608157
Leathers, R., Downes, T., and Mobley, C. (2004). Self-shading correction for oceanographic upwelling radiometers. Opt. Express 12, 4709–4718. doi: 10.1364/OPEX.12.004709
Leymarie, E., Doxaran, D., and Babin, M. (2010). Uncertainties associated to measurements of inherent optical properties in natural waters. Appl. Opt. 49, 5415–5436. doi: 10.1364/AO.49.005415
Leymarie, E., Poteau, A., Andre, X., Besson, F., Brault, P., Claustre, H., et al. (2013). Development and validation of the new ProvBioII float. Mercat. Ocean-Quaterly Newsl. 48, 26–30. Available online at: https://archimer.ifremer.fr/doc/00219/32989/
Li, L., Stramski, D., and Reynolds, R. A. (2016). Effects of inelastic radiative processes on the determination of water-leaving spectral radiance from extrapolation of underwater near-surface measurements. Appl. Opt. 55, 7050–7067. doi: 10.1364/AO.55.007050
Mazeran, C. (2017). Requirements for Copernicus Ocean Colour Vicarious Calibration Infrastructure. EUMETSAT. Available online at: https://www.eumetsat.int/website/wcm/idc/idcplg?IdcService=GET_FILE&dDocName=PDF_COP_OCEAN_COL_CAL&RevisionSelectionMethod=LatestReleased&Rendition=Web.
Mitchell, B., and Holm-Hansen, O. (1991). Biooptical properties of antarctic peninsula waters - differentiation from temperate ocean models. Deep-Sea Res. Part -Oceanogr. Res. Pap. 38, 1009–1028. doi: 10.1016/0198-0149(91)90094-V
Morel, A., Antoine, D., and Gentili, B. (2002). Bidirectional reflectance of oceanic waters: accounting for Raman emission and varying particle scattering phase function. Appl. Opt. 41, 6289–6306. doi: 10.1364/AO.41.006289
Morel, A., and Maritorena, S. (2001). Bio-optical properties of oceanic waters: a reappraisal. J. Geophys. Res.-Oceans 106, 7163–7180. doi: 10.1029/2000JC000319
Morrow, J. H., Hooker, S. B., Booth, C. R., Bernhard, G., Lind, R. N., and Brown, J. W. (2010). Advances in measuring the apparent optical properties (AOPs) of optically complex waters. NASA Tech Memo 215856, 42–50.
Mueller, J. L., Fargion, G. S., McClain, C. R., Pietras, C., Hooker, S. B., Austin, R., et al. (2003). Ocean Optics Protocols For Satellite Ocean Color Sensor Validation, Revision 4, Volume II: Instrument Specifications, characterization and Calibration. Goddard Space Flight Space Center Greenbelt, Maryland: National Aeronautical and Space Administration. Available online at: https://oceancolor.gsfc.nasa.gov/docs/technical/protocols_ver4_volii.pdf (Accessed November 7, 2017).
Organelli, E., Claustre, H., Bricaud, A., Barbieux, M., Uitz, J., D'Ortenzio, F., et al. (2017). Bio-optical anomalies in the world's oceans: an investigation on the diffuse attenuation coefficients for downward irradiance derived from Biogeochemical Argo float measurements. J. Geophys. Res.-Oceans 122, 3543–3564. doi: 10.1002/2016JC012629
Organelli, E., Claustre, H., Bricaud, A., Schmechtig, C., Poteau, A., Xing, X., et al. (2016). A novel near-real-time quality-control procedure for radiometric profiles measured by bio-argo floats: protocols and performances. J. Atmospheric Ocean. Technol. 33, 937–951. doi: 10.1175/JTECH-D-15-0193.1
Piskozub, J. (2004). Effect of 3-D instrument casing shape on the self-shading of in-water upwelling irradiance. Opt. Express 12, 3144–3148. doi: 10.1364/OPEX.12.003144
Quan, X., and Fry, E. (1995). Empirical-equation for the index of refraction of seawater. Appl. Opt. 34, 3477–3480. doi: 10.1364/AO.34.003477
Roemmich, D. (2009). Argo the challenge of continuing 10 years of progress. Oceanography 22, 46–55. doi: 10.5670/oceanog.2009.65
Sentinel-3A Product Notice–OLCI Level-2 Ocean Colour (2018). Available online at: https://www.eumetsat.int/website/wcm/idc/idcplg?IdcService=GET_FILE&dDocName=PDF_S3A_PN_OLCI_L2_REP&RevisionSelectionMethod=LatestReleased&Rendition=Web
Taillandier, V., Wagener, T., D'Ortenzio, F., Mayot, N., Legoff, H., Ras, J., et al. (2017). Hydrography in the Mediterranean Sea during a cruise with RV Tethys 2 in May 2015. Earth Syst. Sci. 10, 627–41. doi: 10.5194/essd-10-627-2018
Wojtasiewicz, B., Hardman-Mountford, N. J., Antoine, D., Dufois, F., Slawinski, D., and Trull, T. W. (2018). Use of bio-optical profiling float data in validation of ocean colour satellite products in a remote ocean region. Remote Sens. Environ. 209, 275–290. doi: 10.1016/j.rse.2018.02.057
Zibordi, G., D'Alimonte, D., and Berthon, J. F. (2004). An evaluation of depth resolution requirements for optical profiling in coastal waters. J. Atmospheric Ocean. Technol. 21, 1059–1073. doi: 10.1175/1520-0426(2004)021<1059:AEODRR>2.0.CO;2
Zibordi, G., Doyle, J. P., and Hooker, S. B. (1999). Offshore tower shading effects on in-water optical measurements. J. Atmospheric Ocean. Technol. 16, 1767–1779. doi: 10.1175/1520-0426(1999)016<1767:OTSEOI>2.0.CO;2
Zibordi, G., Holben, B., Slutsker, I., Giles, D., D'Alimonte, D., Melin, F., et al. (2009). AERONET-OC: a network for the validation of ocean color primary products. J. Atmospheric Ocean. Technol. 26, 1634–1651. doi: 10.1175/2009JTECHO654.1
Zibordi, G., and Mélin, F. (2017). An evaluation of marine regions relevant for ocean color system vicarious calibration. Remote Sens. Environ. 190, 122–136. doi: 10.1016/j.rse.2016.11.020
Keywords: autonomous profiling floats, remote sensing reflectance, ocean color, validation, system vicarious calibration, austral ocean, Mediterranean Sea, radiometric measurement
Citation: Leymarie E, Penkerc'h C, Vellucci V, Lerebourg C, Antoine D, Boss E, Lewis MR, D'Ortenzio F and Claustre H (2018) ProVal: A New Autonomous Profiling Float for High Quality Radiometric Measurements. Front. Mar. Sci. 5:437. doi: 10.3389/fmars.2018.00437
Received: 16 July 2018; Accepted: 30 October 2018;
Published: 27 November 2018.
Edited by:
Gilles Reverdin, Centre National de la Recherche Scientifique (CNRS), FranceReviewed by:
Christoph Waldmann, University of Bremen, GermanyShinya Kouketsu, Japan Agency for Marine-Earth Science and Technology, Japan
Copyright © 2018 Leymarie, Penkerc'h, Vellucci, Lerebourg, Antoine, Boss, Lewis, D'Ortenzio and Claustre. This is an open-access article distributed under the terms of the Creative Commons Attribution License (CC BY). The use, distribution or reproduction in other forums is permitted, provided the original author(s) and the copyright owner(s) are credited and that the original publication in this journal is cited, in accordance with accepted academic practice. No use, distribution or reproduction is permitted which does not comply with these terms.
*Correspondence: Edouard Leymarie, leymarie@obs-vlfr.fr