- 1Geospatial Ecology of Marine Megafauna Lab, Marine Mammal Institute, Department of Fisheries and Wildlife, Hatfield Marine Science Center, Oregon State University, Newport, OR, United States
- 2Cooperative Institute for Marine Resources Studies, Oregon State University and Pacific Marine Environmental Laboratory, National Oceanic and Atmospheric Administration, Newport, OR, United States
During traditional boat-based surveys of marine megafauna, behavioral observations are typically limited to records of animal surfacings obtained from a horizontal perspective. Achieving an aerial perspective has been restricted to brief helicopter or airplane based observations that are costly, noisy, and risky. The emergence of commercial small unmanned aerial systems (UAS) has significantly reduced these constraints to provide a stable, relatively quiet, and inexpensive platform that enables replicate observations for prolonged periods with minimal disturbance. The potential of UAS for behavioral observation appears immense, yet quantitative proof of utility as an observational tool is required. We use UAS footage of gray whales foraging in the coastal waters of Oregon, United States to develop video behavior analysis methods, determine the change in observation time enabled by UAS, and describe unique behaviors observed via UAS. Boat-based behavioral observations from 53 gray whale sightings between May and October 2016 were compared to behavioral data extracted from video analysis of UAS flights during those sightings. We used a DJI Phantom 3 Pro or 4 Advanced, recorded video from an altitude ≥25 m, and detected no behavioral response by whales to the UAS. Two experienced whale ethologists conducted UAS video behavioral analysis, including tabulation of whale behavior states and events, and whale surface time and whale visible time (total time the whale was visible including underwater). UAS provided three times more observational capacity than boat-based observations alone (300 vs. 103 min). When observation time is accounted for, UAS data provided more and longer observations of all primary behavior states (travel, forage, social, and rest) relative to boat-based data, especially foraging. Furthermore, UAS enable documentation of multiple novel gray whale foraging tactics (e.g., headstands: n = 58; side-swimming: n = 17; jaw snapping and flexing: n = 10) and 33 social events (nursing and pair coordinated surfacings) not identified from boat-based observation. This study demonstrates the significant added value of UAS to marine megafauna behavior and ecological studies. With technological advances, robust study designs, and effective analytical tools, we foresee increased UAS applications to marine megafauna studies to elucidate foraging strategies, habitat associations, social patterns, and response to human disturbance.
Introduction
With the decreasing cost of commercial small unmanned aerial systems (UAS; also known as ‘drones’) and increasing accessibility and reliability of platforms, there has been a rapid expansion of UAS applications to marine megafauna research throughout the 2010s. In this period, UAS has been applied to a range of marine megafauna, including studies on body condition of cetaceans (Christiansen et al., 2016a; Dawson et al., 2017; Burnett et al., in press) and pinnipeds (Goebel et al., 2015; Krause et al., 2017) through photogrammetry techniques; population, density and distribution estimates of pinnipeds (Goebel et al., 2015; Johnston et al., 2017), cetaceans (Hodgson et al., 2017), seabirds (Goebel et al., 2015), sirenia (Hodgson et al., 2013), and turtles (Sykora-Bodie et al., 2017); photo-identification studies of pinnipeds (Pomeroy et al., 2015) and cetaceans (Koski et al., 2015); and exhalent sample collection of cetacean blows (Acevedo-Whitehouse et al., 2010; Pirotta et al., 2017). Prior to application of UAS technology, collection of many of these data types was limited and/or reliant on costly, noisy and risky helicopter or fixed wing platforms (e.g., Perryman and Lynn, 2002; Torres et al., 2005; Brower et al., 2017). UAS has the potential to significantly reduce the human risk associated with aerial marine megafauna surveys by eliminating the need for manned flights. Furthermore, UAS provides significantly increased repeatability of data and sample collection relative to traditional methods, allowing for increased sample size of datasets. Importantly, the reduced noise level of UAS compared to planes and helicopters greatly minimizes behavioral impact on study species (Christiansen et al., 2016b; Smith et al., 2016). Although the potential of UAS technology to enhance marine megafauna behavioral studies has been recognized (Nowacek et al., 2016; Fiori et al., 2017; Rees et al., 2018), few behavioral studies have been conducted, with the exception of sea turtles (Bevan et al., 2016; Schofield et al., 2017), sharks (Rieucau et al., 2008; Gallagher et al., 2018), and assessments of disturbance response to UAS by marine mammals (Pomeroy et al., 2015; Arona et al., 2018; Domínguez-Sánchez et al., 2018) and seabirds (Rümmler et al., 2016; Weimerskirch et al., 2018). To date, no study has applied UAS to investigate the behavioral ecology of marine mammals.
Undoubtedly, novel UAS applications to study marine megafauna are on the horizon as we are only beginning to tap into the media, payload, sensor, and mechanical capabilities of UAS. While UAS offer exciting new perspectives and data collection capabilities, there is a simultaneous need for robust study design and data analysis to ensure that UAS-based studies produce rigorous, repeatable, and reliable results. For instance, although video or image data collection via UAS is relatively easy, robust analysis of these data to produce reliable and objective results requires thoughtful, time intensive, quantitative approaches that account for image and analysis bias or error, and variable detection probability (Hodgson et al., 2013, 2017; Dawson et al., 2017; Krause et al., 2017; Sykora-Bodie et al., 2017; Burnett et al., in press). Transferable methods of data collection and analysis that foster comparability of UAS-based research across study populations and systems are needed. Without such systematic methods, the full potential of UAS to marine megafauna research will not be achieved because the greatest insights are often achieved through comparable, longitudinal, and collaborative studies. For example, the immense and enduring contribution of photo-identification methods (Wursig and Wursig, 1977) to marine megafauna studies and knowledge is a testament to the importance of method simplicity, transferability, and rigor. UAS may provide a unique aerial perspective of marine megafauna behavior, with minimal noise (Christiansen et al., 2016b), safety risk, and cost, but methods for robust quantification are needed, as well as proof of utility as an observational tool.
Improved knowledge of a species’ behavioral ecology can enhance the understanding of evolutionary systems, communication patterns, physiological requirements, reproductive cycles, habitat use and the roles of competition and predation, response to disturbance, and much more. All of which help to improve conservation management efforts. Behavioral observations of marine animals are challenging, not least because many species, like cetaceans, spend the majority of their lives below the surface (often >90%; Lagerquist et al., 2000; Berta et al., 2005). Like most marine megafauna, cetaceans are capable of moving large distances in short periods, often behave unpredictably, and demonstrate individuality, all of which restrict many forms of stationary or remote behavioral aquatic observation techniques that are applied for observation of other marine animals [e.g., camera traps, Remotely Operated Vehicle (ROVs), scuba]. Hence, behavioral observation of cetaceans is traditionally conducted from a boat-based horizontal perspective of animals during their surface periods. Boat-based observers use prior experience, species knowledge, and intuition to interpret surface observations as behavior states, patterns or events. Despite efforts to reduce and account for potential bias in these methods through standardized behavior definitions (e.g., ethograms), describing and classifying the behavior of an animal during ∼90% of its life based on surface observation during ∼10% of its life remains a difficult task. In addition to only providing a snapshot of animal behavior, boat-based observations also limit the ability to assess the context of behavioral choices by animals, including intra- or inter-specific interactions, habitat associations, or human influence. Focal animal follows are a useful method of behavioral data collection frequently applied to cetacean studies (Mann, 1999). With this approach, a behavioral sample is collected at predetermined temporal intervals, in order to develop an objective behavioral budget that avoids sampling bias toward unique or short-term behaviors. Despite this robust data collection method, behavioral evaluation primarily remains limited to brief surface observations.
Here, we suggest that traditional boat-based cetacean behavioral documentation during sighting events and focal follows could be enhanced through the application of UAS, particularly small vertical take-off and landing (VTOL) UAS aircraft. Multicopter VTOL UAS are well-suited for cetacean behavioral observation because they are generally lightweight, transportable, easy to launch and retrieve from a small research vessel, record altitude and geolocation metadata at high sample rates, and use a small and intuitive flight ground control station allowing the pilot real-time remote flight control and review of high-definition video. Moreover, these UAS typically use a brushless gimbal and can hover with high flight stability allowing high quality imagery. The main drawback to these small VTOL UAS is limited battery life, which is frequently between 15 and 30 min (depending on model). While VTOL UAS could revolutionize the capacity for behavioral observation of cetaceans, it is prudent to first evaluate behavioral impacts of UAS on cetaceans, develop quantitative methods of video and data analysis, and evaluate the added value of UAS as an observational tool.
In this study we examine VTOL UAS video footage of gray whales (Eschrichtius robustus) collected off the Oregon, United States coast for the primary purpose of photogrammetry (Burnett et al., in press). Upon review of the footage, it quickly became apparent that previously undocumented behaviors could be described through these data as well. Therefore, we conducted a behavioral assessment of the UAS video footage in comparison to our boat-based observed and recorded behaviors. This case study examines the behavior patterns of the Pacific Coast Feeding Group (PCFG) of gray whales, which is a sub-population of the larger Eastern North Pacific (ENP) gray whale population (Calambokidis et al., 2002, 2012). PCFG whales forage in coastal waters (<3 km from shore) from June to October between northern California (United States) and southern British Columbia (Canada), which is a crucial life-history stage as it replenishes their energy stores to sustain migration, fasting and reproductive phases (Rice and Wolman, 1971; Sumich, 1984).
While most ENP gray whales are benthic suction feeders that exploit thick mats of ampeliscid amphipods in the Bering and Chukchi seas (Coyle et al., 2007; Brower et al., 2017), PCFG gray whales display flexible foraging habitats and prey items. In their northern range along the coasts of Vancouver Island (Canada) and Washington (United States), PCFG gray whales have been documented benthic feeding on amphipods and ghost shrimp (Callianassa californiensis) (Oliver et al., 1984; Weitkamp et al., 1992; Dunham and Duffus, 2001) and consuming epibenthic and pelagic mysid (Mysidae Dana, 1850), herring eggs/larvae (Clupu barengus pallasi), and crab larvae (Family Porcellanidae) (Darling et al., 1998; Dunham and Duffus, 2001; Nelson et al., 2008; Feyrer and Duffus, 2011). Comparatively little is known about the foraging ecology of PCFG gray whales in Oregon, except documentation of feeding on two mysid species (Holmesimysis sculpta and Neomysis rayi) (Newell and Cowles, 2006; Newell, 2009) and foraging in association with kelp forests (Nereocystis luetkeana) and reef habitats (Iddings, 2017; Sullivan, 2017). While Stelle et al. (2008) documented the broad behavioral budgets of gray whales near Vancouver Island, there is minimal description of PCFG gray whale foraging strategies or tactics that facilitate prey detection, capture, and handling. Improved information of behavior patterns of PCFG whales could help inform management efforts to reduce the impact of a growing whale watch industry (Sullivan and Torres, 2018) and increased fisheries entanglements (Scordino et al., 2017).
New technology frequently brings exciting potential to scientific fields. Among other applications, UAS may increase observational capacity of marine megafauna, and therefore an improved understanding of their biology, ecology, physiology, and susceptibility to anthropogenically driven impacts (Hunt et al., 2013; Nowacek et al., 2016; Torres, 2017). The main goals of this gray whale case study are to determine the change in observation time enabled by UAS, assess the increased resolution of behavioral data provided by UAS-based observations, and describe unique gray whale behaviors observed via UAS. We examine the potential benefit of UAS to cetacean behavioral studies and describe limitations, pitfalls, and methodological pathways to encourage the development of the robust application of UAS to expand our understanding of cetacean behavior.
Materials and Methods
Data Collection
Boat-based surveys for gray whales were conducted near Newport (44.60765, -124.08162) and Port Orford (42.73828, -124.50004), OR, United States between late May and October 2016. A crew of three used a small (∼6 m) rigid hull inflatable boat (RHIB) to identify and observe gray whales and collect data in favorable weather conditions (wind <12 knots, swell <5 ft, minimal to no fog or rain). After detection of a whale, the vessel slowly approached the whale(s), GPS location and time were recorded, and the vessel maintained a balanced distance that allowed effective photo-identification effort while also minimizing behavioral disturbance (approximately 30 to 80 m). After collection of right and left sided whale body photographs, UAS operations commenced when weather conditions and whale behavior were favorable. Although behavioral focal follows were not conducted during sightings, behavioral observation of whale(s) and notation were conducted throughout the sighting period including during photo-identification and UAS effort. After each sighting, gross behavior state(s) was assigned as travel, forage, social, rest, and/or unknown using the following definitions.
Travel: whale shows directed travel in a consistent direction, with regular surfacing intervals. Speed of travel can vary (slow or fast).
Forage: whale shows no consistent directional movement, but rather stays in a general area. Surfacings may be at irregular temporal intervals and durations, and often culminate with a high arch terminal dive to promote a more vertical body angle. Observations may also include defecation, sharking (vertical profile of half the fluke above the water surface) and bubble blasts (underwater release of air that rises to surface and forms a circle/puka). The Forage behavior state comprises both “searching” and “feeding” behavior states.
Social: whales demonstrate interaction with a conspecific either through observed tactile action, mother/calf nursing, or coordinate surface activity (e.g., racing and splashing).
Rest: logging type behavior observed where whale remains in same location, lying at or just below the surface, and with minimal to no active fluking to promote movement. Surfacings are generally slow and at regular intervals.
Unknown: no recognizable or classifiable behavior patterns are observed.
In addition to identification of gross behavior state(s) for each whale sighting, group size and composition were recorded, and observation of specific behavior events were noted, including bubble blasts, sharking, travel speed, breaching, and defecation.
UAS operations were conducted when conditions were favorable: wind <10 knots, no fog or rain, cloud ceiling >1000 ft. A DJI Phantom 3 Pro or 4 Advanced was piloted using manual remote flight control of the aircraft and real-time camera output through an AppleTM IPAD MiniTM tablet ground station operating the DJI Go application. The cameras on these aircraft have a 3.61 mm focal length and are stabilized by a 3-axis brushless gimbal. The video output was recorded at 4 K and a 1080 p downsample was transmitted real-time to the pilot at 30 Hz.
A trained and licensed pilot in command operated the aircraft under a United States Federal Aviation Administration (FAA) Certificate of Authorization (CoA) issued to Oregon State University. The aircraft was manually released and caught from the RHIB using gloves and a face shield to prevent injury in case of aircraft malfunction or flight error. All flights were logged including launch and retrieval GPS location and time. Given the limited battery life of the UAS (∼15 min) it was important to promote quick acquisition of the whale into the camera’s field of view. Therefore, while waiting for the whale to surface, the UAS hovered at a higher altitude (∼45 m). When the whale surfaced, the boat crew immediately communicated the whale’s location to the aircraft pilot to assist localization of the whale, and film its behavior and capture photogrammetry images (Burnett et al., in press). Once located by the UAS pilot in the video screen, the UAS approached the whale and hovered above it between 25 and 40 m altitude. The UAS followed the whale at the surface and underwater as enabled by water clarity and whale behavior. The boat stayed with the whale and aircraft during flight operations at a safe distance (∼80 m) to minimize impact on whale behavior. Depending on group size and data capture success, multiple flights were sometimes conducted during a sighting. A GPS location and time position was recorded at the end of each sighting.
Data Analysis
UAS video behavior analysis began by clipping video to only periods where a whale or a blow was visible. Next, these edited video clips were grouped by sighting, but were not linked with any sighting behavior information to avoid bias in behavioral assignment. Two experienced whale behavioral ecologists (LGT and SLN) thoroughly reviewed the video clips independently to identify sub-behaviors and behavior events and categorize broad behavior states (Table 1). Initial steps in this process included tabulation of group size and composition (e.g., mother-calf pair), total time the whale was at the surface (whale surface time), and total time the whale was visible including underwater (whale visible time; Figure 1). Whale surface time was defined as anytime a whale’s body part broke the water’s surface; this metric was used to capture the amount of time a whale could be observed from a boat-based horizontal viewpoint. Whale visible time was defined as anytime the whale’s body was discernable, either at the surface or underwater. If two or more whales were recorded at the same time, time summaries and behavioral classification was conducted separately for each individual. Unusable video clips, due to limited whale observation time or poor viewing conditions (glare), were identified and removed from the dataset during this initial pass.
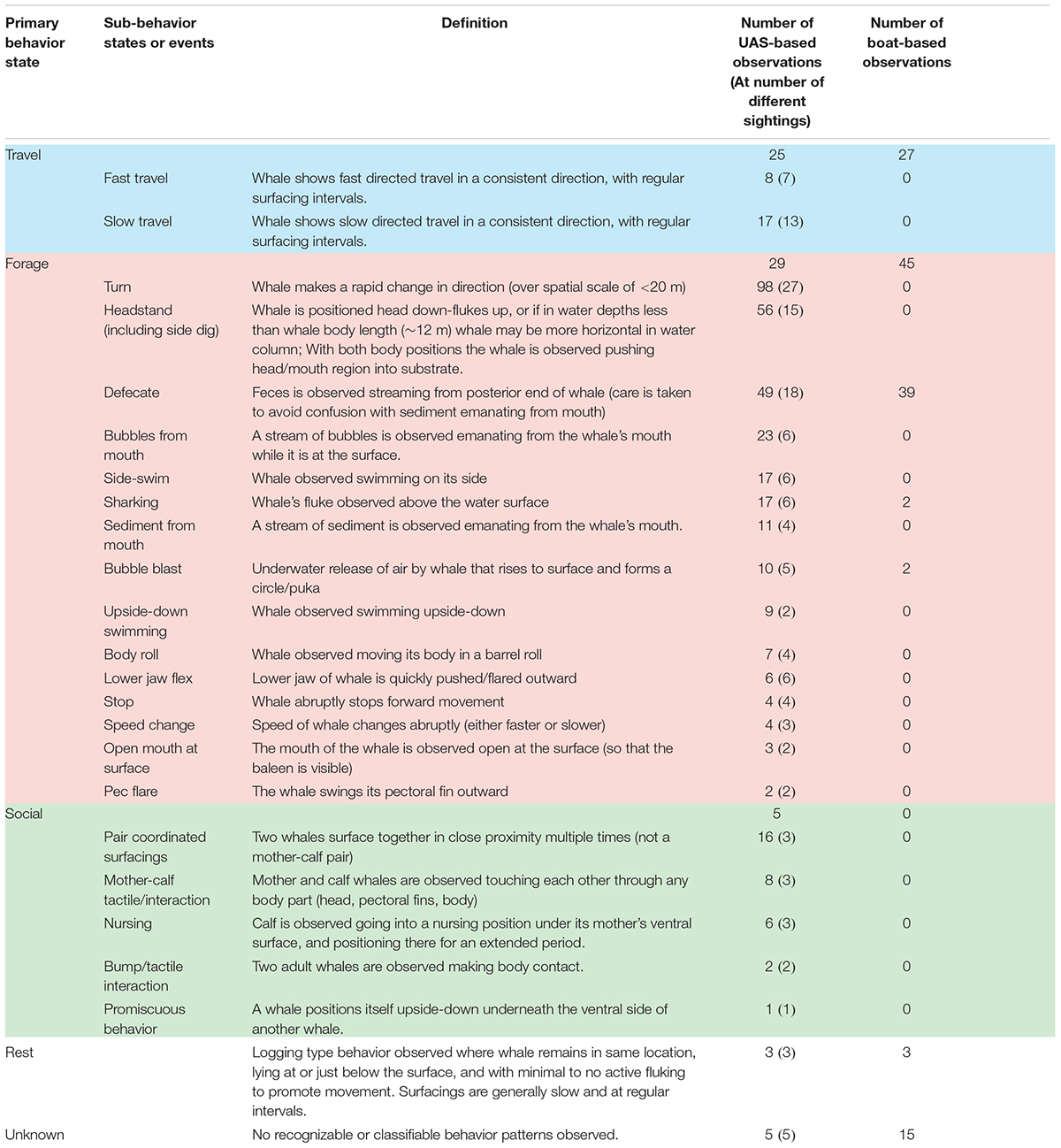
TABLE 1. Description of whale sub-behavior states and events that were identified in Unmanned Aerial System (UAS) video analysis, and used to classify Primary behavior state of each sighting. No sub-behavior states or events were identified for the primary behavior states of Rest and Unknown. The right columns tabulate the number of times each behavior was observed in UAS videos (multiple observations can occur in one sighting), the number of sightings each behavior was observed at, and the number of times the behavior was observed from the boat.
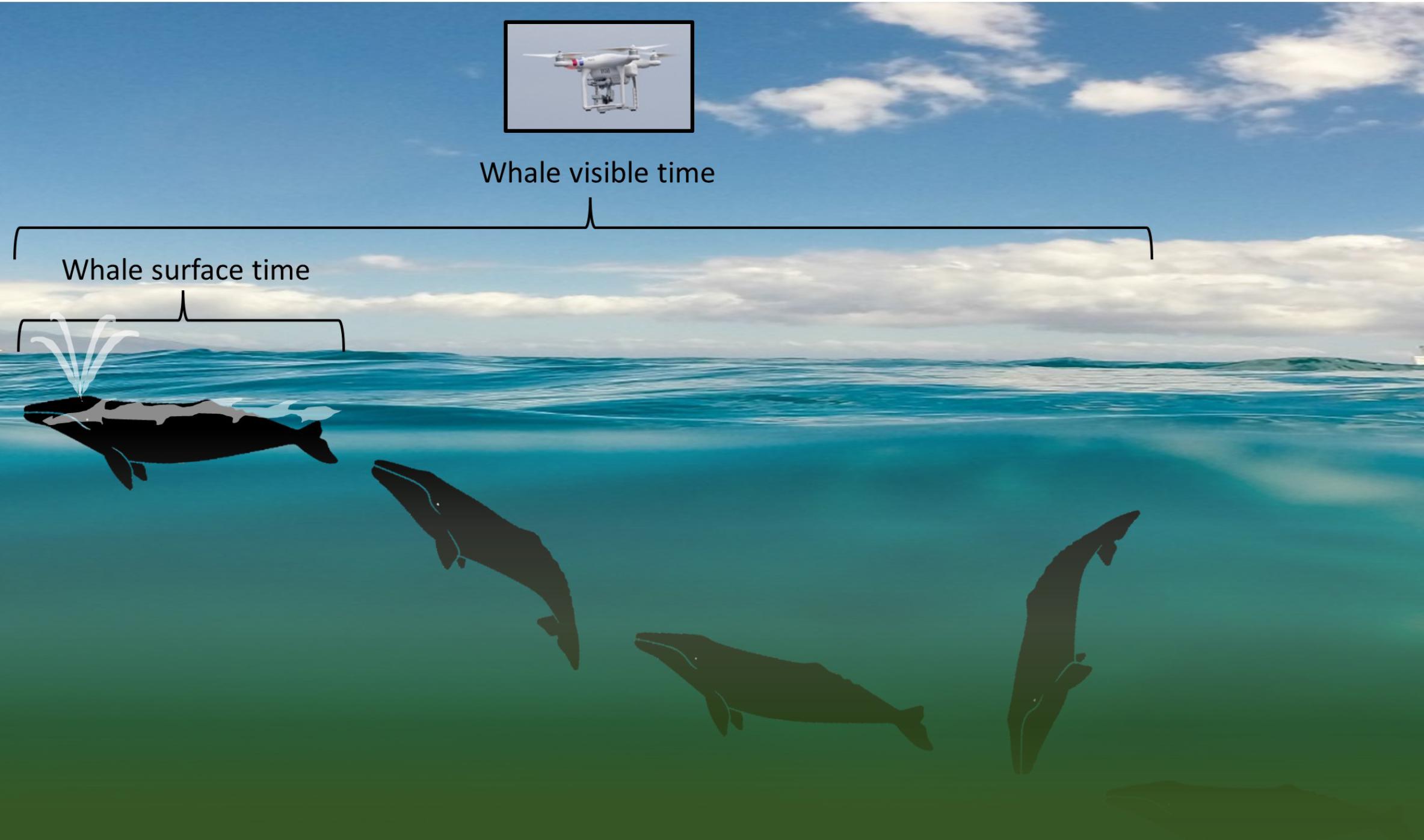
FIGURE 1. Diagram illustrating “whale surface time” relative to “whale visible time” data as collected from an unmanned aerial systems (UAS) aircraft flying over a gray whale as it moves sequentially (from right to left) from “headstand” foraging to surfacing. Decreased visibility through the water column due to turbidity is illustrated by shading. Background image provided by Matt Flores on Unsplash with permission for use; gray whale images by iStock.com/KBelka with permission for use; UAS photo of a DJI Phantom 3 Pro by L. Torres.
The UAS video clips were then reviewed multiple times by the behavioral ecologists, who identified all sub-behavior states or events (Table 1) that were subsequently used to classify each clip into a primary behavior state of travel, forage, social, rest and/or unknown. The duration of each behavior state was summed by sighting. All sub-behavior states or events were tabulated and descriptive comments provided. Behavioral assessment by the two behavioral ecologists were compared; if behavioral classification differed for a sighting, the ecologists jointly reviewed the video clips and conferred until an agreement was reached on whale behavior (n = 2). To assess the improved resolution of the UAS-based behavior observations relative to boat-based data, and to describe how these UAS location data may enhance the understanding of fine-scale whale habitat use patterns, the locations of two boat-based gray whale sightings were plotted in conjunction with the locations of UAS-based sub-behavior events (headstands and bubble blasts; see Table 1) recorded at those sightings (ESRI®, ArcGIS v 10.1 implemented for mapping).
UAS clip data were then collapsed by sighting to describe the UAS derived primary whale behavior state(s) and any sub-behavior states or events. Each sighting was then linked with the corresponding boat-based sighting data including total duration of sighting (start time–end time), broad behavior state classification, and documentation of any behavior events in the field notes. Only sightings where the UAS was deployed and recorded usable data were included in this analysis. These boat-based and UAS-based behavior data were then compared to assess the added value of UAS behavioral observation in terms of time and improved behavioral classification and description. ANOVAs were applied to determine the relationship between the duration of sightings or the amount of UAS whale visible time with the number of primary behavior states or sub-behavior events recorded.
UAS behavioral analysts also assessed potential behavioral response of the whale to the UAS by noting any sudden behavioral change with UAS approach to the whale or rolling of the head or body to visually inspect the aircraft.
Results
Behavioral data from 53 gray whale sightings were compared to UAS flights during those sightings. Neither UAS video analyst detected a behavioral response of a whale to the UAS overflight. Total time spent with whales at these sightings was 57:54:00 (594 min). Gray whales were visible (above or below water) in UAS video recorded at these sightings for 5:00:36 (300.6 min). A whale was at the surface in these video clips for 1:40:52 (104.8 min), representing 34.9% of the whale visible time. Hence, the UAS added three times more observational capacity than boat-based horizontal whale observation alone.
Whale sightings assessed via boat-based observation and UAS video analysis were assigned one, two, or three different primary behavior classes. The number of primary behavior classes recorded during boat-based observations was not related to the duration of the sighting (ANOVA, F2,50 = 0.62, P = 0.54), but the number of identified primary behavior states in the UAS video clips was correlated with the amount of whale visible time (ANOVA, F2,50 = 24.94, P < 0.0001). Furthermore, the number of sub-behavior states and events was also correlated with the amount of whale visible time (ANOVA, F17,35 = 49.44, P < 0.0001). Underlying assumptions of data implemented in these ANOVAs were met (normality, independence, and homoscedasticity).
The percent of observation time that whales were observed in each primary behavior state (activity budgets) was very similar between UAS observed whale surface time and UAS observed whale visible time (Figure 2). When the absolute counts of primary behavioral states are compared between boat-based observations and UAS video analysis (Figure 3A), there were more observations of foraging and unknown behavior states from the boat, more identification of social behaviors from UAS data, and approximately the same number of observations of travel and rest behaviors from either platform. However, when absolute counts of behavioral observations are related to sighting duration (boat-based observations) or whale visible time (UAS based observations), the distribution of these proportional primary behavior states differs (Figure 3B): UAS data provided greater observational capacity of travel, forage, social, and rest behavior states compared to boat-based observations, given the amount of observational time. Furthermore, when the duration of behavioral observation time is compared between whale surface time and whale visible time (Figure 3C), it is clear that the UAS provides increased observational capacity of all behavior states relative to what could be seen from a horizontal boat-based perspective, especially foraging, which increased by three times.
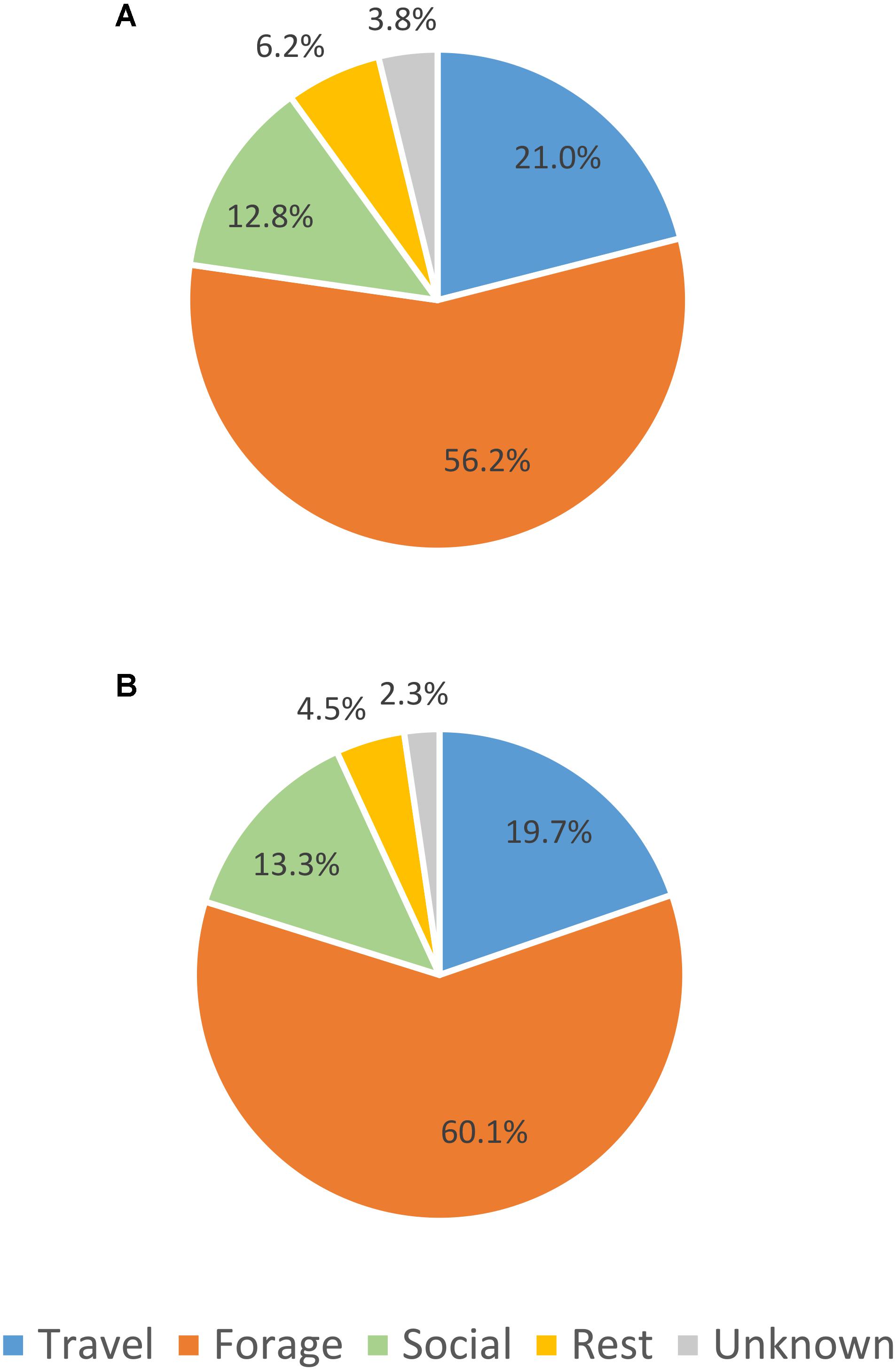
FIGURE 2. Comparison of percent of activity budget for each primary gray whale behavior state between (A) UAS observed whale surface time and (B) UAS observed whale visible time.
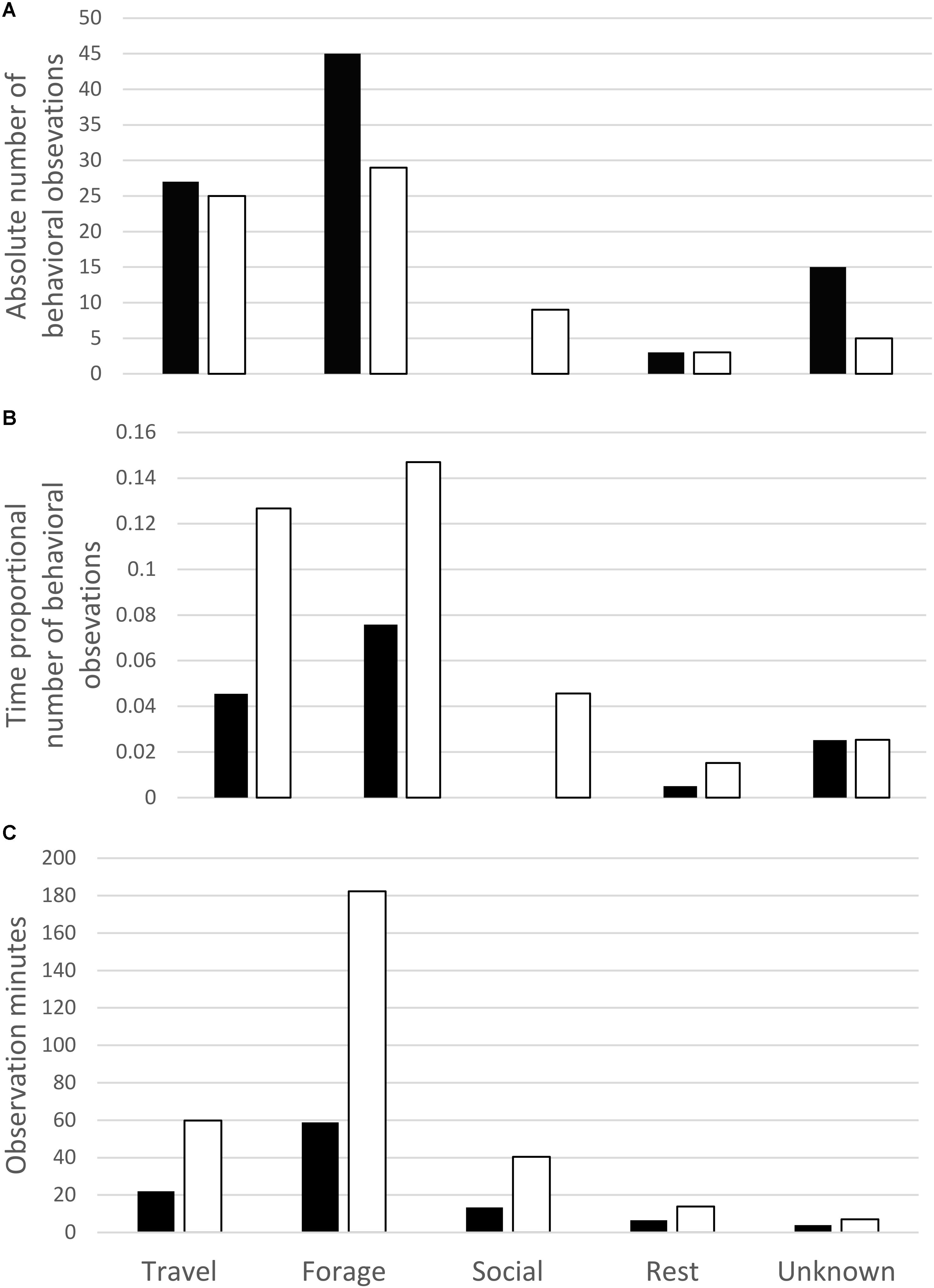
FIGURE 3. Comparison of (A) absolute count, (B) time proportional, and (C) total observational minutes of primary gray whale behavior states recorded during boat-based field observations (black bars) and those identified in review of UAS video (white bars).
While specific behavior events of sharking (n = 2) and bubble blasts (n = 2) were occasionally observed at sightings by the boat-based team, multiple unique and interesting sub-behavior states and events were identified through the UAS video analysis only (Table 1, Figure 4 and Supplementary Material). These observations include behaviors not previously documented for a gray whale, including headstands, swimming upside-down or on its side, lower jaw flexing, a stream of bubbles coming from the whale’s mouth followed by a stream of sediment, and opening its mouth at the surface (Figure 4 and see Supplementary Material for video clips of UAS recorded behaviors). The UAS data enabled greater description of the forage primary behavior state through the identification of 13 added sub-behavior states or events (not including sharking or bubble blasts). Additionally, five added behavioral descriptions were identified in the social behavior class that were not described in boat-based field notes, including nursing, bump/tactile interaction between adult whales, and coordinated surfacings of adults (Figure 4). This increased identification and description of behaviors highlights the added value of UAS-based behavior analysis to traditional boat-based behavioral observations.
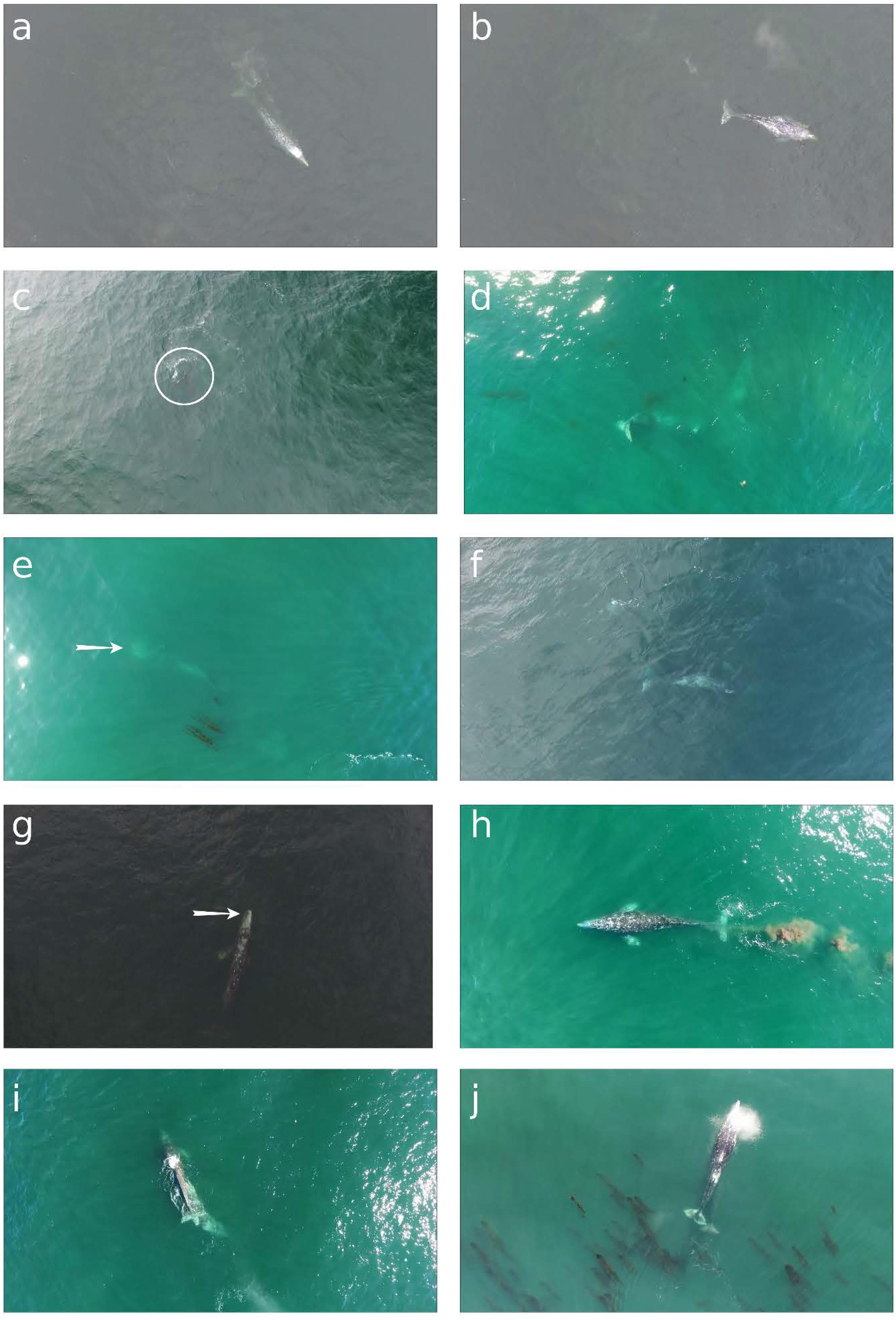
FIGURE 4. Still images captured from unmanned aerial system (UAS) overflights of gray whales off the Oregon, United States coast illustrating different behavior events (see Table 1 for descriptions): (a) “bubbles from mouth” can be seen streaming from left side of mouth, which was followed 39 s later by (b) “sediment from mouth” that is gray/brown steam of small clouds coming from left side of mouth; (c) white circle highlights fluke of whale performing a “headstand”; (d) two whales engaged in “headstand” foraging together; (e) whale engaged in “side-swim”, arrow points to open mouth; (f) whale swimming “upside-down”, which persisted for 3 min; (g) arrow points to “open mouth at surface” of whale as it swims forward; (h) “defecation” event; (i) a “bump/tactile interaction” event as one whale pushes with its head into the underside of another whale’s caudal region; (j) nursing behavior observed as calf aligns under mother’s ventral area (see Supplementary Material of UAS video clips of behavior events).
The two example plots of boat-based gray whale sighting locations relative to the locations of UAS-based behavior observation at those sightings (Figure 5) demonstrate the increased resolution of spatially explicit behavior data and the potential for enhanced knowledge of functional habitat use patterns relative to benthic substrate. In both cases, the original sighting location (black star) was recorded in benthic habitat that is different from the substrate where the actual behavior (headstands and bubble blasts) was exhibited and recorded by the UAS. The boat-based data alone indicated that foraging occurred at these sightings in boulder and sand habitat. Yet, with the UAS-based behavior observations these behavioral associations are resolved to seven headstands and five bubble blasts occurring in rock, and three headstands and two bubble blasts occurring in sand habitat.
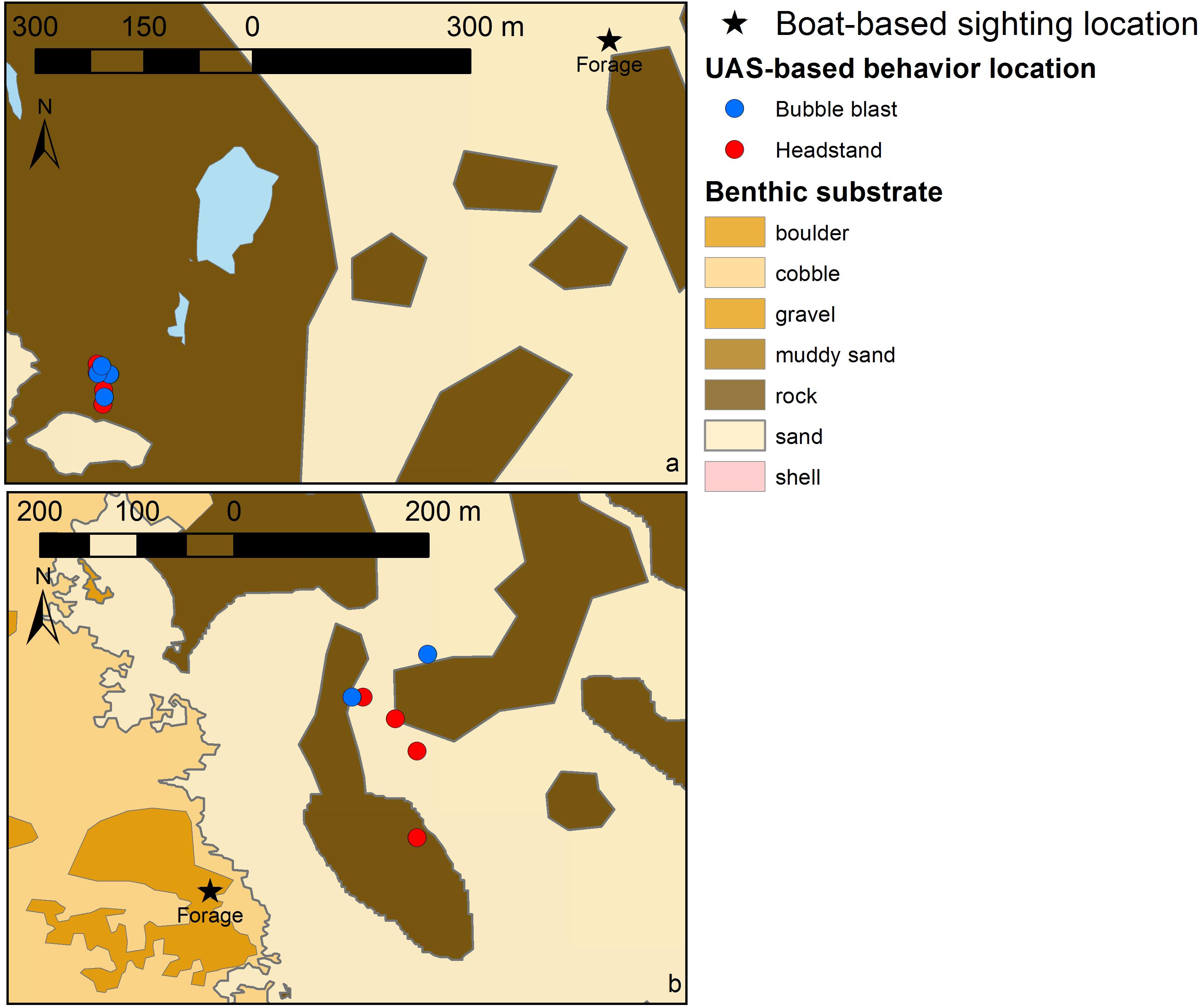
FIGURE 5. Two examples of the boat-based location of a gray whale sighting (black star; labeled by recorded behavior state) and the UAS-based locations of sub-behavior events (see Table 1 for descriptions) recorded at these sightings (blue and red circles). (a) June 22, 2016 near Newport, OR, United States; blue areas indicate unclassified benthic substrate; (b) August 28, 2016 near Port Orford, OR, United States. Benthic substrate layer collected by multibeam in 2010 and provided by C. Goldfinger and C. Romsos, Active Tectonics and Seafloor Mapping Lab, Oregon State University.
Discussion
Behavioral observations lend critical information to the ecological and biological understanding of wild animals. In this case study, we demonstrate that UAS provided three times the observational capacity of gray whale behavior compared to possible boat-based observations (whale surface time) during the same time periods. Furthermore, through UAS analysis multiple sub-behavior states and events are newly described (Table 1 and Figure 4), which provide greater insight into their foraging ecology, habitat use patterns, and social interactions. However, UAS operations are limited by battery life, making boat-based observations critical to obtain greater temporal context of behaviors. Yet, as technology and battery capacity improve, the duration of UAS flights will improve, offering more capacity for an aerial perspective on marine animals, as indicated by the correlations between the number of behavior states and events observed and whale visible time. Clearly, the amount of whale visible time is highly dependent on ocean conditions, particularly water clarity. However, even in the worst water clarity conditions, UAS-based behavioral observations can confirm or clarify boat-based observations, and potentially help describe behavior from a new perspective. We anticipate that multicopter VTOL UAS technology with a real-time pilot can be used successfully in future marine mammal focal follow studies by using multiple aircraft that alternate data collection periods (swap in and swap out) to allow battery replacement. Such UAS-based focal follows could allow enhanced data collection and behavioral insights. However, UAS video analysis is labor intensive, requiring significant post-processing time for video review, assessment, and tabulation of marine mammal behavior data. Hence, we encourage the development of thoughtful field and data analysis protocols prior to data collection to facilitate this process and robust results through efficient processing. Additionally, the development of automated or semi-automatic detection algorithms of animals and behavior states in images and videos, such as those applied in acoustic monitoring of cetaceans (Baumgartner and Mussoline, 2011; Klinck and Mellinger, 2011), would significantly decrease video analysis time (i.e., Maire et al., 2015; Karnowski et al., 2016).
Our derived activity budgets for whale primary behavior states, based both on UAS observed whale surface time and UAS observed whale visible time, were very similar to those determined by Stelle et al. (2008) for the same gray whale sub-population (PCFG) foraging in similar habitat in British Columbia, Canada, which indicates that UAS observations are not behaviorally biased. It is the increased observational minutes (Figures 3B,C) and the refinement of primary behavior states into sub-behavior states and events that forms a strength of UAS-based behavioral observations. While Stelle et al. (2008) stopped their analysis at the description of primary behavior states, the UAS based observations allow us to describe and identify 15 more foraging-associated events, five more social behavior events, and two different travel speeds. Furthermore, although Stelle et al. (2008) describe a “tailswish” as when “part or all of the tail breaks the water’s surface without concurrent surfacing of the entire animal (i.e., no respiration is possible),” which is likely the same behavior as our “headstand” (Figures 4c,d), through our UAS observations we can thoroughly describe the whale’s underwater behavior during these events, better surmise the functional purpose of this sub-behavior, and likely identify many more of these events. Furthermore, the relative spatial distribution of boat-based and UAS-based behavior data from the same sightings (Figures 5a,b) illustrates how the fine-scale UAS-based behavior data improves the resolution of behaviorally mediated habitat use patterns. Due to embedded GPS data in the UAS video, all observed behaviors include high-resolution spatial data that can be further investigated to better understand the animals’ spatial behavioral ecology.
Our case study here on gray whale behavior demonstrates that with improved behavioral data we can gain insight into this population’s foraging flexibility, functional habitat use patterns, temporal and spatial context of behaviors, and social relationships. We documented that gray whales use multiple foraging tactics, highlighting their adaptability to prey availability and various habitats. This foraging plasticity may help explain the rapid population increase of Northeast Pacific gray whales since whaling (Alter et al., 2007), their broad spatial distribution across the northeast Pacific during their foraging season (Calambokidis et al., 2002; Moore et al., 2003; Brower et al., 2017), and their potential to respond to multiple scales of habitat change, from seismic surveys (Bröker et al., 2015) to climate change (Alter et al., 2015; Salvadeo et al., 2015). This new information can be applied to refine management plans so that mitigation of human induced threats, such as vessel strikes, noise pollution, and fisheries entanglement, is targeted and effective. We believe that UAS-based behavioral observation can also be successfully applied to other surface-associated marine megafauna species, such as other whales, delphinids, pinnipeds, sirenians, and sharks, to improve knowledge of their behavioral repertoire and apply this information gained toward spatially explicit management efforts. Like all field work, methodology and logistics depend on context, and successful use of UAS in other systems will be a function of species’ surface times and speed, water clarity and ability to track the animal(s) while underwater by UAS, and habitat conditions that affect UAS operations such as swell, fog, hazards (i.e., bridges, mangrove islands, and rocky headlands), and air and vessel traffic.
Given the retrospective nature of this study, we were not able to directly compare the behavioral budgets of UAS whale visible time with a budget derived for whales based on boat-based observations because time-stamped behavioral data were not collected (focal follows were not conducted). Therefore, we estimated the potential boat-based observation time through the calculation of UAS whale surface time. This approach assumes that anytime the whale’s body is above the surface the boat-based observers can identify the behavior state from their horizontal perspective. Therefore, whale surface time provides a near-maximum estimate of boat-based observation capacity. However, we did not account for any “through the water column” whale behavioral observations by the field crew. These boat-based underwater observations are rare (LGT estimate 1% of sighting time) but can inform behavioral classification of sightings while in the field. A study that compares independent boat-based and UAS-based focal follow data of a marine mammal would be valuable to directly compare the added value of each perspective.
More primary behavior states were attributed to the whale sightings based on boat-based observations (Figure 3A) because twice as much observational time was available to boat observers relative to battery-limited UAS video analysis. Once behavior distribution was assessed relative to time observed (Figures 4b,c), foraging was the clear dominant primary behavior state. More travel time was observed in UAS video analysis because this data is more temporally refined. For example, when a whale moved between kelp patches to feed, this behavior was marked as travel in the UAS analysis. However, at a larger spatial and temporal perspective of boat-based observations this movement would still be considered part of a foraging or searching behavior.
Social behaviors are difficult to discern during boat-based observations, particularly if they do not occur at the surface, as exemplified by the lack of any social behavior states identified by boat-based observation for the 53 sightings examined, and by the low social behavior activity budget (8%) documented by Stelle et al. (2008). In contrast, through UAS video analysis we observed 33 social events that were undetected by the field team. It is often assumed that while on their foraging grounds gray whales are primarily engaged in solitary foraging activities and are not very social, except for mother-calf pairs (Sumich, 2014). Yet, the UAS data illustrate a number of social behaviors that increased in frequency toward the autumn months as the breeding season approached, which aligns with results from Stelle et al. (2008). When observations of mother-calf interactions (n = 8) and nursing (n = 6) are excluded from our UAS behavior dataset, the remaining 19 social events all occurred in the latter half of the field season (between August 22 and September 03, 2016). This increased knowledge of gray whale social interactions on the “foraging grounds” achieved through UAS-based observations can help clarify this population’s reproductive cycles and habitats used.
In the current developmental stages of UAS applications to marine megafauna research, both the added value of the technology and the potential for disturbance should be assessed. Here, we did not find any gray whale behavioral response to the UAS overflights, which corresponds with findings from other assessments of UAS impacts on baleen whales (Koski et al., 2015; Christiansen et al., 2016a; Durban et al., 2016; Domínguez-Sánchez et al., 2018). However, disturbance may not manifest behaviorally, but rather physiologically as was demonstrated with American black bears (Ursus americanus) that showed minimal behavioral response to UAS but had significantly elevated heart rates (Ditmer et al., 2015). Additionally, although acoustic signals of UAS are not expected to penetrate the water column significantly (Christiansen et al., 2016b), we must maintain caution in our applications of UAS to marine megafauna research and follow established guidelines (Vas et al., 2015; Hodgson and Koh, 2016; Smith et al., 2016). For instance, cetaceans may hear the UAS when their hearing canal is above the water’s surface, or the shadow of a UAS overhead may illicit disturbance. Vigilance of disturbance is particularly important during UAS close approaches, such as for blow sample collection. Domínguez-Sánchez et al. (2018) found no difference in behavioral response of blue whales to UAS close approaches for blow sample collection when the UAS approached the animal from behind. However, a behavioral response was noted when the UAS accidentally approached head-on, which demonstrates that flight characteristics (i.e., height, approach direction, aircraft type) are important to consider (Domínguez-Sánchez et al., 2018). In our case study, the UAS was never flown less than 25 m above the whale, which we hope limits potential disturbance. Furthermore, given the high-resolution video output of most UAS and the large size of baleen whales, higher flight altitudes can provide increased context for behavioral observations due to a larger field of view of habitat and conspecific interactions. However, this height may be constrained in studies with dual objectives, such as body condition or photo-id catalogs.
Analysis of our UAS video has provided immense insight into the behavioral repertoire of gray whales that are summer residents along the Oregon coast (Table 1, Figure 4 and Supplementary Material). Subsequently we have adjusted our flight protocol to not only collect photogrammetry data but also film whales for a longer duration to collect behavioral data. As we continue to build our dataset of behaviors we will assess the fine-scale functional habitat use patterns of these whales by comparing behavioral data with environmental conditions, thus improving the ecological understanding of this population. This example is just one of the many ways UAS behavioral observation data can be extended to better understand marine megafauna ecology (Torres, 2017) and human disturbance (Nowacek et al., 2016). Even without large datasets, UAS can provide unique perspectives on marine megafauna behaviors allowing even brief observations to enhance the understanding of animal ecology (e.g., Barlow et al., 2018). However, it is imperative to acknowledge the limitations, biases, and potential harm of UAS technology in marine megafauna studies. UAS is not a panacea for marine megafauna research, but rather another useful tool to complement traditional field study methods. Added equipment and logistics needed to conduct quantitative behavioral observations of marine megafauna include a UAS and ancillary gear such as batteries, a base station, and SD cards, additional permits (location specific airspace authority and research oversight), a certified and trained UAS pilot and aircraft observer, a hard drive for large data storage, video analyst time, and video viewing software (free options include VLC Media Player and Media Player Classic). Development of a robust study design prior to data collection is essential to ensure minimal disturbance, appropriate data collection and sample size to answer targeted questions, field team safety, acquisition of all necessary permits, and analysis success. While the capacity for undisturbed behavioral observation of marine megafauna via UAS is great, this technology cannot replace perceptive in situ field observations by context-aware researchers. Yet, integration of a UAS perspective with boat-based field studies on marine megafauna behavior (e.g., Nowacek et al., 2001; Nowacek, 2002) can be highly valuable.
Author Contributions
LT conceived the project idea, obtained funding to support the research, and led data analysis and writing. LT and SN conducted video analysis. TC was the primary unmanned aerial systems pilot and facilitated video data collection. All authors contributed to data collection during fieldwork and reviewed the manuscript.
Funding
Funding for this project was supplied by the NOAA/NMFS Ocean Acoustics Program, The Marine Mammal Institute at Oregon State University, Oregon Sea Grant, and the Pacific Marine Environmental Lab. L. Lemos was supported by Brazil’s Science Without Borders program, Brazil’s CNPq, and the Harvard Laspau Institute.
Conflict of Interest Statement
The authors declare that the research was conducted in the absence of any commercial or financial relationships that could be construed as a potential conflict of interest.
Acknowledgments
Data were collected under NOAA/NMFS permit #16111 to John Calambokidis and Oregon State University’s FAA Certificate of Authorization. We are grateful to Joe Haxel, Dawn Barlow, Lauren Roche, and Florence Sullivan for field assistance, to Jonathan Burnett and Ashley Leopold for data analysis support, and to the reviewers of this manuscript for useful comments and revision. This paper represents Pacific Marine Environmental Lab contribution #4780.
Supplementary Material
The Supplementary Material for this article can be found online at: https://www.frontiersin.org/articles/10.3389/fmars.2018.00319/full#supplementary-material; https://oregonstate.box.com/s/t6vkgpjzc3cl61i87bt61hs3n9zdm1ty
References
Acevedo-Whitehouse, K., Rocha-Gosselin, A., and Gendron, D. (2010). A novel non-invasive tool for disease surveillance of free-ranging whales and its relevance to conservation programs. Anim. Conserv. 13, 217–225. doi: 10.1111/j.1469-1795.2009.00326.x
Alter, S. E., Meyer, M., Post, K., Czechowski, P., Gravlund, P., Gaines, C., et al. (2015). Climate impacts on transocean dispersal and habitat in gray whales from the Pleistocene to 2100. Mol. Ecol. 24, 1510–1522. doi: 10.1111/mec.13121
Alter, S. E., Rynes, E., and Palumbi, S. R. (2007). DNA evidence for historic population size and past ecosystem impacts of gray whales. Proc. Natl. Acad. Sci. U.S.A. 104, 15162–15167. doi: 10.1073/pnas.0706056104
Arona, L., Dale, J., Heaslip, S. G., Hammill, M. O., and Johnston, D. W. (2018). Assessing the disturbance potential of small unoccupied aircraft systems (UAS) on gray seals (Halichoerus grypus) at breeding colonies in Nova Scotia. Canada. Peer J. 6:e4467. doi: 10.7717/peerj.4467
Barlow, D. R., Torres, L. G., Hodge, K. B., Steel, D., Baker, C. S., Chandler, T. E., et al. (2018). Documentation of a New Zealand blue whale population based on multiple lines of evidence. Endanger. Species Res. 36, 27–40. doi: 10.3354/esr00891
Baumgartner, M. F., and Mussoline, S. E. (2011). A generalized baleen whale call detection and classification system. J. Acoust. Soc. Am. 129, 2889–2902. doi: 10.1121/1.3562166
Berta, A., Sumich, J. L., and Kovacs, K. M. (2005). “Respiration and Diving Physiology,” in Marine Mammals: Evolutionary Biology, ed. J. L. Sumich (New Yorkm, NY: Elsevier).
Bevan, E., Wibbels, T., Navarro, E., Rosas, M., Najera, B., Sarti, L., et al. (2016). Using Unmanned Aerial Vehicle (UAVs) technology for locating, identifying, and monitoring courtship and mating behavior in the green sea turtle (Chelonia mydas). Herpetol. Rev. 47, 27–32.
Bröker, K., Gailey, G., Muir, J., and Racca, R. (2015). Monitoring and impact mitigation during a 4D seismic survey near a population of gray whales off Sakhalin Island, Russia. Endanger. Species Res. 28, 187–208. doi: 10.3354/esr00670
Brower, A. A., Ferguson, M. C., Schonberg, S. V., Jewett, S. C., and Clarke, J. T. (2017). Gray whale distribution relative to benthic invertebrate biomass and abundance: northeastern Chukchi Sea 2009–2012. Deep Sea Res. II Top. Stud. Oceanogr. 144, 156–174. doi: 10.1016/j.dsr2.2016.12.007
Burnett, J. D., Lemos, L., Barlow, D. R., Wing, M. G., Chandler, T. E., Torres, L. G., et al. (in press). Estimating morphometric attributes of baleen whales with photogrammetry from small UAS: a case study with blue and gray whales. Mar. Mammal Sci.
Calambokidis, J., Darling, J. D., and Deecke, V. (2002). Abundance, range and movements of a feeding aggregation of gray whales (Eschrichtius robustus) from California to southeastern Alaska in 1998. J. Cetacean Res. Manag. 4, 267–276.
Calambokidis, J., Laake, J. L., and Klimek, A. (2012). Updated Analysis of Abundance and Population Structure of Seasonal Gray Whales in the Pacific Northwest, 1998-2010. Technical Report SC/M12/AWMP2-Rev. Seattle, WA: NOAA.
Christiansen, F., Dujon, A. M., Sprogis, K. R., Arnould, J. P. Y., and Bejder, L. (2016a). Noninvasive unmanned aerial vehicle provides estimates of the energetic cost of reproduction in humpback whales. Ecosphere 7:e01468. doi: 10.1002/ecs2.1468
Christiansen, F., Rojano-Doñate, L., Madsen, P. T., and Bejder, L. (2016b). Noise Levels of multi-rotor unmanned aerial vehicles with implications for potential underwater impacts on marine mammals. Front. Mar. Sci. 3:277. doi: 10.3389/fmars.2016.00277
Coyle, K. O., Bluhm, B., Konar, B., Blanchard, A., and Highsmith, R. (2007). Amphipod prey of gray whales in the northern bering sea: comparison of biomass and distribution between the 1980s and 2002–2003. Deep Sea Res. II Top. Stud. Oceanogr. 54, 2906–2918. doi: 10.1016/j.dsr2.2007.08.026
Darling, J. D., Keogh, K. E., and Steeves, T. E. (1998). Gray whale (Eschrichtius robustus) habitat utilization and prey species off Vancouver Island, BMarine, C. Mamm. Sci. 14, 692–720. doi: 10.1111/j.1748-7692.1998.tb00757.x
Dawson, S. M., Bowman, M. H., Leunissen, E., and Sirguey, P. (2017). Inexpensive aerial photogrammetry for studies of whales and large marine animals. Front. Mar. Sci. 4:366. doi: 10.3389/fmars.2017.00366
Ditmer, M. A., Vincent, J. B., Werden, L. K., Tanner, J. C., Laske, T. G., Iaizzo, P. A., et al. (2015). Bears show a physiological but limited behavioral response to unmanned aerial vehicles. Curr. Biol. 25, 2278–2283. doi: 10.1016/j.cub.2015.07.024
Domínguez-Sánchez, C. A., Acevedo-Whitehouse, K. A., and Gendron, D. (2018). Effect of drone-based blow sampling on blue whale (Balaenoptera musculus) behavior. Mar. Mamm. Sci. 34, 841–850. doi: 10.1111/mms.12482
Dunham, J. S., and Duffus, D. A. (2001). Foraging patterns of gray whales in central clayoquot sound, British Columbia, Canada. Mar. Ecol. Prog. Ser. 223, 299–310. doi: 10.3354/meps223299
Durban, J. W., Moore, M. J., Chiang, G., Hickmott, L. S., Bocconcelli, A., Howes, G., et al. (2016). Photogrammetry of blue whales with an unmanned hexacopter. Mar. Mamm. Sci. 32, 1510–1515. doi: 10.1111/mms.12328
Feyrer, L., and Duffus, D. (2011). Predatory disturbance and prey species diversity: the case of gray whale (Eschrichtius robustus) foraging on a multi-species mysid (family Mysidae) community. Hydrobiologia 678, 37–47. doi: 10.1007/s10750-011-0816-z
Fiori, L., Doshi, A., Martinez, E., Orams, M. B., and Bollard-Breen, B. (2017). The use of unmanned aerial systems in marine mammal research. Remote Sens. 9:543. doi: 10.3390/rs9060543
Gallagher, A. J., Papastamatiou, Y. P., and Barnett, A. (2018). Apex predatory sharks and crocodiles simultaneously scavenge a whale carcass. J. Ethol. 36, 205–209. doi: 10.1007/s10164-018-0543-2
Goebel, M., Perryman, W., Hinke, J., Krause, D., Hann, N., Gardner, S., et al. (2015). A small unmanned aerial system for estimating abundance and size of Antarctic predators. Polar Biol. 38, 619–630. doi: 10.1007/s00300-014-1625-4
Hodgson, A., Kelly, N., and Peel, D. (2013). Unmanned Aerial vehicles (UAVS) for surveying marine fauna: a dugong case study. PLoS One 8:e79556. doi: 10.1371/journal.pone.0079556
Hodgson, A., Peel, D., and Kelly, N. (2017). Unmanned aerial vehicles for surveying marine fauna: assessing detection probability. Ecol. Appl. 27, 1253–1267. doi: 10.1002/eap.1519
Hodgson, J. C., and Koh, L. P. (2016). Best practice for minimising unmanned aerial vehicle disturbance to wildlife in biological field research. Curr. Biol. 26, R404–R405. doi: 10.1016/j.cub.2016.04.001
Hunt, K. E., Moore, M. J., Rolland, R. M., Kellar, N. M., Hall, A. J., Kershaw, J., et al. (2013). Overcoming the challenges of studying conservation physiology in large whales: a review of available methods. Conserv. Physiol. 1:cot006. doi: 10.1093/conphys/cot006
Iddings, K. (2017). How Gray Whales Find Food: A Novel Approach Offers Insight Into the Factors That Drive Gray Whale Foraging at a Local Scale Ph.D. thesis, Duke University, Durham, N C.
Johnston, D. W., Dale, J., Murray, K. T., Josephson, E., Newton, E. W., Wood, S., et al. (2017). Comparing occupied and unoccupied aircraft surveys of wildlife populations: assessing the gray seal (Halichoerus grypus) breeding colony on Muskeget Island, USA. J. Unmanned Vehicle Syst. 5, 178–191. doi: 10.1139/juvs-2017-0012
Karnowski, J., Johnson, C., and Hutchins, E. (2016). Automated video surveillance for the study of marine mammal behavior and cognition. Anim. Behav. Cogn. 3, 255–264. doi: 10.12966/abc.05.11.2016
Klinck, H., and Mellinger, D. K. (2011). The energy ratio mapping algorithm: a tool to improve the energy-based detection of odontocete echolocation clicks. J. Acoust. Soc. Am. 129, 1807–1812. doi: 10.1121/1.3531924
Koski, W. R., Gamage, G., Davis, A. R., Mathews, T., LeBlanc, B., Ferguson, S. H., et al. (2015). Evaluation of UAS for photographic re-identification of bowhead whales, Balaena mysticetus. J. Unmanned Veh. Syst. 3, 22–29. doi: 10.1139/juvs-2014-0014
Krause, D. J., Hinke, J. T., Perryman, W. L., Goebel, M. E., and LeRoi, D. J. (2017). An accurate and adaptable photogrammetric approach for estimating the mass and body condition of pinnipeds using an unmanned aerial system. PLoS One 12:e0187465. doi: 10.1371/journal.pone.0187465
Lagerquist, B., Stafford, K., and Mate, B. (2000). Dive characteristics of satellite-monitored blue whales (Balaenoptera musculus) off the central California coast. Mar. Mamm. Sci. 16, 375–391. doi: 10.1111/j.1748-7692.2000.tb00931.x
Maire, F., Alvarez, L.M., and Hodgson, A. (2015) “Automating marine mammal detection in aerial images captured during wildlife surveys: A deep learning approach,” in Proceedings of the AI 2015: Advances in Artificial Intelligence, eds B. Pfahringer and J. Renz, Vol. 9457. Cham: Springer International Publishing, 379–385. doi: 10.1007/978-3-319-26350-2_33
Mann, J. (1999). Behavioral sampling methods for cetaceans: a review and critique. Mar. Mamm. Sci. 15, 102–122. doi: 10.1111/j.1748-7692.1999.tb00784.x
Moore, S. E., Grebmeier, J. M., and Davies, J. R. (2003). Gray whale distribution relative to forage habitat in the northern Bering Sea: current conditions and retrospective summary. Can. J. Zool. 81, 734–742. doi: 10.1139/z03-043
Nelson, T. A., Duffus, D. A., Robertson, C., and Feyrer, L. J. (2008). Spatial-temporal patterns in intra-annual gray whale foraging: characterizing interactions between predators and prey in Clayquot Sound, British Columbia, Canada. Mar. Mamm. Sci. 24, 356–370. doi: 10.1111/j.1748-7692.2008.00190.x
Newell, C. (2009). Ecological Interrelationships Between Summer Resident Gray Whales (Eschrichtius robustus) and Their Prey, Mysid Shrimp (Holmesimysis sculpta and Neomysis rayi) along the Central Oregon Coast Ph.D. thesis, Oregon State University Corvallis, OR, 107.
Newell, C. L., and Cowles, T. J. (2006). Unusual gray whale Eschrichtius robustus feeding in the summer of 2005 off the central Oregon Coast. Geophys. Res. Lett. 33:l22S11.
Nowacek, D. P. (2002). Sequential foraging behavior of bottlenose dolphins, Tursiops truncatus, in Sarasota Bay, Fl. Behaviour 139, 1125–1145. doi: 10.1007/s00442-015-3241-6
Nowacek, D. P., Christiansen, F., Bejder, L., Goldbogen, J. A., and Friedlaender, A. S. (2016). Studying cetacean behaviour: new technological approaches and conservation applications. Anim. Behav. 120, 235–244. doi: 10.1016/j.anbehav.2016.07.019
Nowacek, D. P., Tyack, P. L., and Wells, R. S. (2001). A platform for continuous behavioral and acoustic observation of free-ranging marine mammals: overhead video combined with underwater audio. Mar. Mamm. Sci. 17, 191–199. doi: 10.1111/j.1748-7692.2001.tb00992.x
Oliver, J. S., Slattery, P. N., Silberstein, M. A., and O’Connor, E. F. (1984). Gray whale feeding on dense ampeliscid amphipod communities near Bamfield, British Columbia. Can. J. Zool. 62, 41–49. doi: 10.1139/z84-009
Perryman, W. L., and Lynn, M. S. (2002). Evaluation of nutritive condition and reproductive status of migrating gray whales (Eschrichtius robustus) based on analysis of photogrammetric data. J. Cetacean Res. Manag. 4,155–164.
Pirotta, V., Smith, A., Ostrowski, M., Russell, D., Jonsen, I. D., Grech, A., et al. (2017). An economical custom-built drone for assessing Whale Health. Front. Mar. Sci. 4:425. doi: 10.3389/fmars.2017.00425
Pomeroy, P., O’Connor, L., and Davies, P. (2015). Assessing use of and reaction to unmanned aerial systems in gray and harbor seals during breeding and molt in the U K. J. Unmanned Veh. Syst. 3, 102–113. doi: 10.1139/juvs-2015-0013
Rees, A. F., Avens, L., Ballorain, K., Bevan, E., Broderick, A. C., Carthy, R. R., et al. (2018). The potential of unmanned aerial systems for sea turtle research and conservation: a review and future directions. Endanger. Species Res. 35, 81–100. doi: 10.3354/esr00877
Rice, D. W., and Wolman, A. A. (1971). The Life History and Ecology of the Gray Whale (Eschrichtius robustus). Stillwater Ok: American Society of Mammalogists.
Rieucau, G., Kiszka, J. J., Castillo, J. C., Mourier, J., Boswell K. M., and Heithaus, M. R. (2018). Using unmanned aerial vehicle (UAV) surveys and image analysis in the study of large surface-associated marine species: a case study on reef sharks Carcharhinus Melanopterus Shoaling Behaviour. J. Fish Biol. 93, 119–127. doi: 10.1111/jfb.13645
Rümmler, M.-C., Mustafa, O., Maercker, J., Peter, U. H., and Esefeld, J. (2016). Measuring the influence of unmanned aerial vehicles on Adélie penguins. Polar Biol. 39, 1329–1334. doi: 10.1007/s00300-015-1838-1
Salvadeo, C. J., Gómez-Gallardo, U. A., Nájera-Caballero, M., Urbán-Ramirez, J., and Lluch-Belda, D. (2015). The effect of climate variability on gray whales (Eschrichtius robustus) within their wintering areas. PLoS One 10:e0134655. doi: 10.1371/journal.pone.0134655
Schofield, G., Papafitsoros, K., Haughey, R., and Katselidis, K. (2017). Aerial and underwater surveys reveal temporal variation in cleaning-station use by sea turtles at a temperate breeding area. Mar. Ecol. Prog. Ser. 575, 153–164. doi: 10.3354/meps12193
Scordino, J., Carretta, J., Cottrell, P., Greenman, J., Savage, K., Scordino, J., et al. (2017). Ship Strikes and Entanglements of Gray Whales in the North Pacific Ocean. Cambridge: International Whaling Commission, 1924–2015.
Smith, C. E., Sykora-Bodie, S. T., Bloodworth, B., Pack, S. M., Spradlin, T. R., and Leboeuf, N. R. (2016). Assessment of known impacts of unmanned aerial systems (UAS) on marine mammals: data gaps and recommendations for researchers in the United States. J. Unmanned Veh. Syst. 4, 31–44. doi: 10.1139/juvs-2015-0017
Stelle, L. L., Megill, W. M., and Kinzel, M. R. (2008). Activity budget and diving behavior of gray whales (Eschrichtius robustus) in feeding grounds off coastal British Columbia. Mar. Mamm. Sci. 24, 462–478. doi: 10.1111/j.1748-7692.2008.00205.x
Sullivan, F. A. (2017). Fine Scale Foraging Behavior of Gray Whales in Relation to Prey Fields and Vessel Disturbance Along the Oregon Coast. Ph.D. thesis, Oregon State University, Corvallis, OR.
Sullivan, F. A., and Torres, L. G. (2018). Assessment of vessel disturbance to gray whales to inform sustainable ecotourism. J. Wildl. Manag. 82, 896–905. doi: 10.1002/jwmg.21462
Sumich, J. L. (1984). Gray whales along the oregon coast in summer, 1977-1980. Murrelet 65, 33–40. doi: 10.2307/3535288
Sumich, J. L. (2014). E. Robustus: The Biology and Human History of Gray Whales. Corvallis, OR: Whale Cove Marine Education.
Sykora-Bodie, S. T., Bezy, V., Johnston, D. W., Newton, E., and Lohmann, K. J. (2017). Quantifying nearshore sea turtle densities: applications of unmanned aerial systems for population assessments. Sci. Rep. 7:17690. doi: 10.1038/s41598-017-17719-x
Torres, L. G. (2017). A sense of scale: foraging cetaceans’ use of scale-dependent multimodal sensory systems. Mar. Mamm. Sci. 33, 1170–1193. doi: 10.1111/mms.12426
Torres, L. G., McLellan, W. A., Meagher, E., and Pabst, D. A. (2005). Seasonal distribution and relative abundance of bottlenose dolphins, Tursiops truncatus, along the US mid-Atlantic coast. J. Cetacean Res. Manag. 7, 153–161.
Vas, E., Lescroel, A., Duriez, O., Boguszewski, G., and Grémillet, D. (2015). Approaching birds with drones: first experiments and ethical guidelines. Biol. Lett. 11:20140754. doi: 10.1098/rsbl.2014.0754
Weimerskirch, H., Prudor, A., and Schull, Q. (2018). Flights of drones over sub-Antarctic seabirds show species- and status-specific behavioural and physiological responses. Polar Biol. 41, 259–266. doi: 10.1007/s00300-017-2187-z
Weitkamp, L. A., Wissmar, R. C., Simenstad, C. A., Fresh, K. L., and Odell, J. G. (1992). Gray whale foraging on ghost shrimp (Callianassa californiensis) in littoral sand flats of Puget Sound, U.S. A . Can. J. Zool. 70, 2275–2280. doi: 10.1139/z92-304
Keywords: unmanned aerial systems (UAS), behavior, gray whale, foraging, cetacean
Citation: Torres LG, Nieukirk SL, Lemos L and Chandler TE (2018) Drone Up! Quantifying Whale Behavior From a New Perspective Improves Observational Capacity. Front. Mar. Sci. 5:319. doi: 10.3389/fmars.2018.00319
Received: 07 May 2018; Accepted: 21 August 2018;
Published: 10 September 2018.
Edited by:
Rob Harcourt, Macquarie University, AustraliaReviewed by:
David W. Johnston, Duke University, United StatesPhil J. Bouchet, The University of Western Australia, Australia
Copyright © 2018 Torres, Nieukirk, Lemos and Chandler. This is an open-access article distributed under the terms of the Creative Commons Attribution License (CC BY). The use, distribution or reproduction in other forums is permitted, provided the original author(s) and the copyright owner(s) are credited and that the original publication in this journal is cited, in accordance with accepted academic practice. No use, distribution or reproduction is permitted which does not comply with these terms.
*Correspondence: Leigh G. Torres, Leigh.torres@oregonstate.edu