- Research Department, Phillip Island Nature Parks, Cowes, VIC, Australia
Technical advances in monitoring devices, specifically drones, are allowing managers and scientists to obtain quality information on ecosystem health with minimal disturbance to ecosystems and the wildlife they support. Temporal and spatial indicators of ecosystem health, such as population size and/or abundance estimates of marine mammals are the basis for our understanding and prediction of ecosystem change. This is critical for the achievement of conservation goals and sustainable natural resources use. Performing surveys to obtain abundance estimates can be logistically demanding and expensive particularly in offshore marine environments, and can cause significant disturbance to wildlife. These constraints may lead to sub-optimal monitoring programs that reduce the frequency and/or precision of surveys at the cost of data quality and confidence in the resulting analyses. Using Remote Piloted Aircraft (RPA) can be a solution to this challenge. With appropriate testing and ethical consideration; for many situations, RPAs can perform surveys with increased frequency, higher data resolution and less disturbance than typical methods that involve people being present on the ground, thereby enabling more robust programs for monitoring. We demonstrate the process of testing images from RPAs for estimating the abundance of Australian fur seals (Arctocephalus pusillus doriferus) at one of their largest colonies on Seal Rocks, Australia. Two sizes of multirotor (1,400 and 350 mm) with different imaging equipment were tested at 40, 60, and 80 m altitude above sea level. We assessed wildlife disturbance levels and optimized a methodology for effective and economical monitoring of this site. We employed commercially available and open-source software for programming survey flights (Drone Deploy), image processing (Agisoft Photoscan and Autopano Giga), data collation and analyses (R and Python). An online portal “SealSpotter” was developed to facilitate data collection, with the ultimate goal being the engagement of the public as citizen scientists in fur seal counts from RPA images. Preliminary comparisons show that a small RPA at 40 m altitude can produce pup counts 20–32% higher than corresponding ground counts without observable disturbance. The benefits and disadvantages of the RPA trials are discussed, as well as important considerations for those looking to incorporate similar methodologies in their research.
Introduction
Recent technological and legislative advances for Remote Piloted Aircraft (RPAs) have provided opportunities to survey seal colonies with greater control and reproducibility than allowed by piloted aerial surveys, as well as better image resolution and (when performed in an optimal fashion) reduced disturbance, logistics and cost (Nilssen et al., 2014; Pomeroy et al., 2015; Hodgson and Koh, 2016; Adame et al., 2017). Such technology may facilitate the collection of more frequent surveys; for example, every year and even several times per breeding season, instead of every 3–5 years (Kirkwood et al., 2010). More frequent estimates are more valuable in identifying changes in population trends and understanding the effects of environmental variability on a population (Kirkman et al., 2011).
RPAs have been proven to be effective at performing wildlife monitoring surveys and have several advantages including: providing data at high spatial and temporal resolution; providing systematic, permanent data with statistical integrity due to simple replication; having low operational costs and simple logistical requirements particularly in marine environments; and being low-risk for the operators (Jones et al., 2006; Gonzalez et al., 2016). However, RPAs have some constraints, such as short flight endurance, regulatory restrictions, post-survey processing and image storage requirements (Linchant et al., 2015). The post-processing stage can be particularly time consuming and managing the workflow, storage and volume of data becomes an issue. This has led to automatic object identification techniques, machine learning (Gonzalez et al., 2016; Seymour et al., 2017) and the use of citizen scientists to economize data collection (Cox et al., 2015; Lukyanenko et al., 2016; Swanson et al., 2016).
Australian fur seal (Arctocephalus pusillus doriferus) abundance and population trends underpin ecosystem management because they are top predators and provide an important top-down role in the ecosystem (Goldsworthy et al., 2003). They can be the prime attraction of tourism ventures and can negatively impact the commercial fishing and aquaculture industries (Hamer and Goldsworthy, 2006; Robinson et al., 2008). As marine mammals, they are protected by law in Australia under the Environmental Protection and Biodiversity Conservation Act 1999 (EPBC Act) and the location of breeding colonies are relevant to emergency response planning in cases such as oil spills. For traditional on-ground or aerial piloted surveys, availability of external funds is often unpredictable, making long-term monitoring plans difficult. Long delays between successive surveys may also mean that survey methodologies change, and it is therefore difficult to compare data and assess trends accurately (Kirkman et al., 2011).
Like any monitoring or survey method, no single approach will ever be 100% accurate and precise. The goal is to obtain the best method for the task, specifically an index of abundance that is as precise as possible and can be accurately replicated given available resources, such that population trends over time can be ascertained.
It is also important to attempt to calibrate a new method such as RPA survey with the old survey method it replaces, such that the datasets can be accurately compared. This can take more replicates than expected (Hodgson et al., 2016), which may be irreconcilable. If the RPA method is preferred because of other parameters such as disturbance or cost, datasets can be analyzed separately although this is not ideal. In the case of fur seals, population estimates are usually based on the number of pups, which are confined to the breeding colony for the first few weeks of life (Wickens and Shelton, 1992). They are easy to distinguish from older seals, and are born in a single period each year. Typical methods of estimating Australian fur seal abundance include ground counts, piloted aerial surveys and capture-mark-resight surveys (CMR) (Kirkwood et al., 2010). Ground counts and piloted aerial surveys can be considered comparable to a count obtained at the same time from a RPA survey because they are counting what is present and can be seen at that point in time (Hodgson et al., 2016). CMR surveys, however use a marked proportion of the population to estimate the total population at a point in time, including those that cannot be seen; for example those under rocks, in caves, and underwater (Cormack, 1964; Seber, 1982), and should therefore not be considered comparable to counts (McIntosh et al., 2006). While piloted aerial surveys may be performed with less disturbance and logistics than ground counts and CMR, they have been considered highly variable and less reliable (Littnan and Mitchell, 2002). RPA surveys can provide a precise and simple alternative to these methods.
Seal Rocks is the largest Australian fur seal colony, located at the western end of Summerland Peninsula, Phillip Island in Victoria, Australia. The most recent census in 2013–14 identified the first reduction in pup abundance at Seal Rocks, after a period of population recovery (McIntosh et al., 2014). However, 2013–14 results may have been affected by poor environmental conditions and reduced food supply (Alderman, 2014; Peter and Dooley, 2014; Berlincourt and Arnould, 2015). It had been 5 years between population censuses and the frequency reduced the ability to interpret the result. McIntosh et al. (2014) highlighted the problems associated with infrequent abundance estimates and anomalous results for interpreting trends. The major benefits of using RPAs are the reduced effort, cost, and disturbance compared to typical methods. It should be noted here that the reduction in animal disturbance is provisional on height limits and disturbance issues being carefully tested (Hodgson and Koh, 2016).
To perform ground counts, teams of people need to access the colony and this cannot be achieved until after the mate-guarding harems have dissolved in late December (Kirkwood et al., 2010), missing the peak pupping time when such counts would be most accurate. RPAs can perform a survey at any time during the breeding season without significant disturbance (provided the surveys are performed with best practice), meaning that the survey can take advantage of this peak pupping time. Different wildlife species and locations may involve specific risks that result from RPA use. A key consideration for seal colonies includes the bird species that also use the site that may be more likely to negatively interact with RPAs than the seals being surveyed. The type of RPA, its size, shape and noise levels can also be important considerations for ensuring the ethical treatment of wildlife (Hodgson and Koh, 2016; Radiansyah et al., 2017).
Several factors influence the configuration of the RPA system used: the research question is the most important, followed by the localized environmental conditions, the habitat, the color of the target subjects (contrast or camouflage against the habitat), impact of shadows and time of day, as well as tidal amplitude for marine environments. Choosing a system that is the least likely to cause disturbance is also a priority. Finally, the behavior of the subject is important: their movement rate and whether they may be captured in the image more than once, and whether they hide or take refuge in caves and under rocks (for example).
In this study our aims were to trial and compare two different system configurations at Seal Rocks to optimize RPA survey methodology: a large RPA platform carrying a high resolution 35 mm camera, and a small <2 kg RPA with integrated camera and gimbal. We determined the system that provided the best image quality for counting pups with as little disturbance as possible to the seals and the birds that use the site. As a secondary aim, given the presence of seals entangled in marine debris at Seal Rocks (McIntosh et al., 2015), we were also interested in whether RPA surveys could detect entangled seals. Simple comparisons between ground counts and the RPA surveys were used to confirm successful method development. Our goal was to provide the methodology for a simple and cost effective RPA system that can provide an index of abundance suitable for monitoring the trends of wildlife population with fewer disturbances than currently used methods. Readers will be able to use this guide to rapidly implement and troubleshoot their own RPA monitoring system.
Methods
Ground and Airborne RPA System
The RPA used were multirotors: Gryphon Dynamics X8-1400 (large, 1,400 mm diagonal size); and DJI Phantom 4, and 4 Pro (small, 350 mm diagonal size) (Table 1). The Gryphon was flown by contracted professionals, adhering to Civil Aviation Safety Authority (CASA) regulations in all cases. The Phantoms, being <2 kg, were flown by a local provider and by Phillip Island Nature Parks' staff using a ground system that consisted of an iPad™ for flight automation, telemetry, and display; range extenders for improving signal reliability; spare batteries; telescopes and binoculars to observe wildlife and RPA at closer range; and a laptop computer to download and check images and survey success onsite. Prior to the survey, notifications to CASA were in place and all regulations ratified. Landings were performed under manual control to minimize safety risks.
The Gryphon and DJI Phantoms were programmed to take a photo every 2 s, capturing <300 images/flight at a speed aimed at 7 m/s. For the Phantom 4 and 4 Pro we used Drone Deploy (https://www.dronedeploy.com/) to pre-program the flight paths. Importantly, autonomous control enabled a specific, repeatable, programmed flight path (Jones et al., 2006). For the Phantom 4, image frontlap was set to ~70% to control ground speed during image capture, and sidelap was set at 50% to ensure adequate image overlap to facilitate image stitching during processing. The width/height image ratio was 3:2 for all systems.
Study Area
Seal Rocks consists of three rock areas separated by water channels, Seal Rock, East Reef, and Black Rock (Figure 1). Raised plateau areas reach a maximum elevation of 15 meters above sea level. Two rocky outcrops closely situated to the north west of Seal Rock are frequently inundated by waves and not used by the fur seals. Black Rock is characterized by more complex terrain than Seal Rock that has wide open expanses of flat basalt and cobble beaches (Kirkwood et al., 2010).
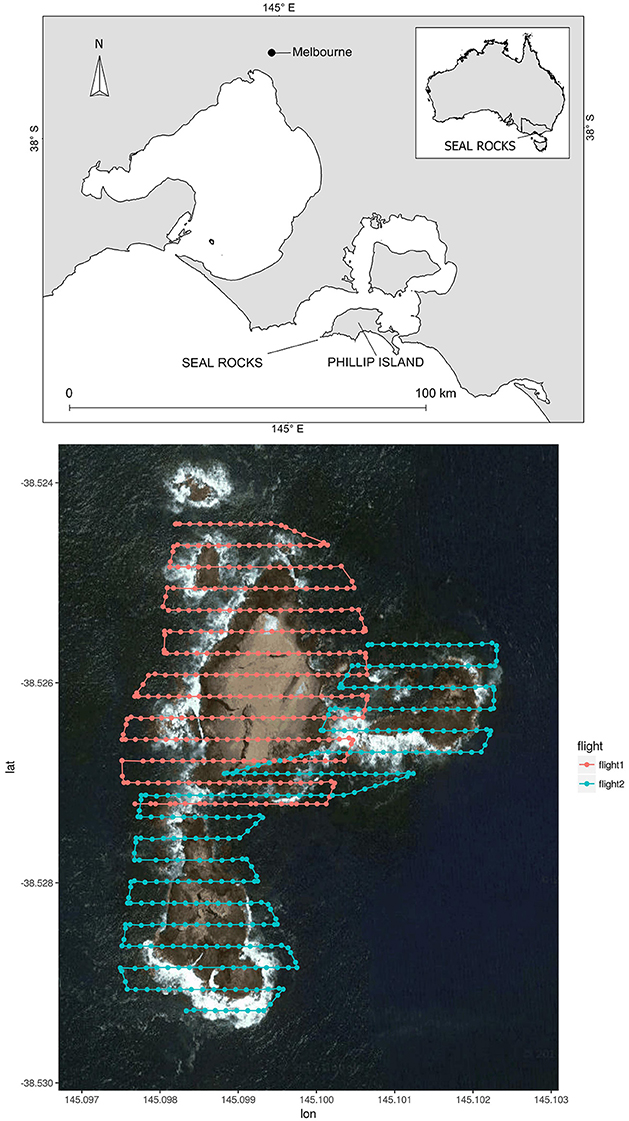
Figure 1. Location of Seal Rocks in Victoria, Australia, and satellite image of Seal Rocks with flight transect lines (for DJI Phantom 4 Pro at 40 m ASL) overlayed. Flight 1 covered Seal Rock and flight 2 covered East Reef and Black Rock. The points on the transect lines identify the locations where photographs were taken, once every 2 s (>300 images/flight). Ground speed during capture is maintained at ~7 m/s. Seal Rocks covers approximately eight hectares in area.
RPA Survey Design
In this study, one survey represents the whole of Seal Rocks being covered once by RPA transects and one flight represents the activity that occurs between take-off and landing. For the larger RPA, one survey was completed as one flight. For the Phantoms, two flights completed one survey: flight one covered Seal Rock and flight two covered East Reef and Black Rock (Figure 1). Given the short 20 min duration of each flight (40 min total), few seals were expected to move between the three areas of Seal Rocks during the survey.
Survey design was a balance of battery power, RPA specification and distance traveled, which was easily explored using Drone Deploy. The total area of Seal Rocks is approximately eight hectares and the maximum distance of a single transect was ~300 m. The RPAs were fully capable of traveling the 1.8 km distance from Summerland Peninsula to Seal Rocks and performing a survey. Considerations here, given the distance traveled, include battery power and remote control signal strength, as well as the limitation of maintaining visual contact with the RPA. In some cases it was necessary to conduct flights from a boat to ensure all of these requirements were fulfilled, however this carried additional inherent risks.
We employed the precautionary principle and started trials from higher altitudes where no disturbance was expected: the goal was to determine the highest altitude for the RPA while obtaining images of applicable quality. Finding the lower altitude limit tolerated by the seals and birds to improve image resolution was considered unnecessary because this would force an increased transect density to get complete coverage of the site, which would result in greater exposure of the RPA to the seals and birds, and may exceed the flight time capacity of the RPA. We worked with the Phillip Island Nature Parks animal ethics committee (AEC) to ensure our methods were appropriate. Operations were aborted when excessive disturbance was observed (see levels of disturbance defined below).
Gryphon flights were performed at 80 and 60 m above sea level (ASL) and the Phantom Pros were flown at 60 and 40 m ASL (Table 2). Because the Drone Deploy software takes the programmed altitude as above ground level (AGL) relative to the take-off point, we selected a take-off site as close to sea level as possible to avoid any discrepancy between ASL and AGL altitudes.
Disturbance Observations and Ground Counts
For four surveys, a team of three to five people were taken to Seal Rocks via mother vessel and landed on site using an inflatable rubber boat (IRB). The researchers camped overnight in a stone hut on Seal Rock where their presence did not cause any noticeable disturbance, allowing the fur seals to recover from the disturbance caused at landing. They performed observations of the fur seals and birds while concealed inside the hut during the RPA flights to record levels of disturbance caused by the RPA. Ground counts of fur seal pups and fur seals with marine debris entanglements were performed on Seal Rock after the RPA survey was completed, in order to compare ground counts with the counts obtained from the RPA survey. Ground counts were not performed on Black Rock or East Reef because of the poor visibility (oblique angle) when counting those areas from Seal Rock and the logistical difficulties in landing on Black Rock after performing the ground count on Seal Rock.
To perform the ground count, experienced researchers worked individually to produce four independent counts; disturbance was minimized by moving slowly and cautiously around the plateau areas, which provided a higher ground vantage point for the counts. Binoculars and hand counters were used to count the fur seals.
Disturbance observations recorded whether wildlife looked at the RPA, moved toward or away from the RPA, or attacked the RPA, and whether wildlife altered their behavior at the time of the RPA survey: e.g., whether they were flushed from a nest or roost, and whether they stood up, walked, ran or stampeded away from point of origin. The observations included an estimate of the number of fur seals or birds affected. Observations were also taken using binoculars and a telescope at the take-off/landing site for any disturbance to birds or other wildlife in that area and the flight path to Seal Rocks.
Disturbance for birds and seals was ranked as: none, low, moderate, high and extreme. For seals, no disturbance was attributed when there was no change observed in behavior or location of the seals that could be attributed to the RPA; low level disturbance was not moving, but looking at the RPA; moderate disturbance was sitting up and looking at the RPA; high disturbance was moving away from the point of origin; and extreme disturbance was a stampede caused by the RPA. We also recorded the number of seals disturbed, if suckling between mothers and pups was disturbed, and estimated distances moved from point of origin should the disturbance cause such movement.
For birds, no disturbance indicated no change observed in behavior or change of position that could be attributed to the RPA; low level disturbance was not moving, but looking at the RPA; moderate level disturbance was individuals flushing from point of origin; high level disturbance was a flock of birds flushing, or being displaced from a nest; extreme disturbance was endangerment to a bird or flock of birds after being flushed or from closely approaching the RPA. We recorded species of bird, approximate height and distances flown of flushed birds and the number of birds in each disturbance category. This included birds present at the site of take-off and touch-down, such as a resident peregrine falcon (Falco peregrinus) pair at the end of Summerland Peninsula that also forage at Seal Rocks.
In the event of an extreme disturbance, the RPA was to be landed at a safe and suitable location and the survey ceased. We expected sound and vision to be important parameters affecting disturbance. The AEC regularly assessed the progress of these RPA surveys and determined continuation or abortion of the project.
Image Processing and Portal Development
Agisoft Photoscan Professional (Agisoft 2017, version 1.3.2, http://www.agisoft.com, accessed 8 August 2017) and Autopano Giga (Kolor 2017, version 4.4, http://www.kolor.com/autopano/ accessed 8 August 2017) were used for image stitching to create a single “total site” mosaic. Python (Python Software Foundation, version 2.7.12, https://www.python.org, accessed 8 August 2017) and R (R-Development-Core-Team, 2005) were then used to slice the mosaic into individual subsection images for manual classification.
The subsection images were then uploaded to an online portal hosted by Phillip Island Nature Parks titled “SealSpotter” (http://natureparksresearch.com.au/sealSpotter/), developed to facilitate rapid manual processing. An observer (counter) logged into the site with their name and clicked to mark the pups and entangled fur seals in each image. For each fur seal marked, x and y coordinates, as well as a precise timestamp, were recorded. The data for each classification of each image was saved as a csv file on the server. This allowed us not only to count the fur seals, but also to map the data and isolate areas, for example, to access the Seal Rock count separately to Black Rock and East Reef. Images were archived once counted to maximize efficiency. For the purpose of this paper, RPA surveys were counted by a single individual (RM). The portal can be programmed to allow multiple replicates of images from multiple individuals to obtain measures of precision around the abundance estimates. The goal is to engage citizen collaborators globally in data collection and facilitate communication and education between citizens and scientists.
Validation Tests and Data Integrity
Flights were planned to balance trade-offs between weather conditions (lower wind speeds typical in morning), angle of the sun and potential differences in seal detection with tidal amplitude. Optimum conditions were expected to include low wind speed to reduce drag and camera movement, and midday sun to maintain consistent image brightness across the site. Tidal amplitude was expected to affect the counts: dark colored pups on dark wet rocks at low tide were expected to be harder to count. Also, at high tide, seals may be expected to be more mobile from having to move away from the incoming tide. RPA surveys were prioritized for the sun at its greatest height to minimize shadows and when wind velocities were lower than 15 kt. We did not have sufficient replication of surveys to test effects of tidal amplitude on the results. This paper provides the methodology for surveying a site with RPAs, comparative pup counts, and counts of fur seals entangled in marine debris are provided to identify that the method is effective.
Addressing Biases for RPA Surveys and Ground Counts
All survey methods have inherent biases and assumptions that vary by method. The three main biases for counts include:
1) Availability bias: that all individuals are equally observable—pups under rocks and in the water will be less observable than those on the flat-open terrain. The probability of an animal being observed depends on its behavior, which changes with age, and the observation window. This is longer for ground counts and shorter for RPA image surveys. Results can also be affected by weather conditions, shadow length and tidal amplitude. Whenever possible, we prioritized the effect of shadow length and standardized the time of day for RPA surveys to close to midday to minimize shadows (Table 2).
2) Perception or observer bias—different people count different numbers, this can be accounted for using multiple counts. However, performing multiple ground counts can result in greater disturbance caused by more people or greater duration of exposure to the people. We used multiple people for the ground counts and we used the same observer for each RPA count to minimize this bias.
3) Movement bias–whether seals are likely to move between locations during the same survey and be counted more than once. The probability of animal duplication (where a single animal appears in the processed image more than once) is dependent on the specific survey technique and equipment used. Ground counts are likely to increase movement bias because disturbance can herd animals away from the observer counting on the ground. The RPA surveys were performed in a small time window (20 min) and it was considered unlikely that seals would move between locations and appear in more than one image.
Results
Disturbance Observations and Counts
Flights using the large Gryphon RPA at 80 m resulted in low disturbances for small numbers of seals, however flying at 60 m did cause disturbance to seals at an unacceptable level (Table 3). Hovering above seals was observed to cause the most disturbance and should be avoided at all times. With the larger RPA, the point at which the noise became noticeable was the first point of awareness for the seals, which triggered general restlessness, as well as several to look, and at times sit up, then move away from the point of origin. Silver gulls (Chroicocephalus novaehollandiae) on nests appeared aware of the large RPA, but did not leave their nests or flush from their point of origin. The smaller RPA did not appear to be detected by the seals or birds above background noise levels and did not cause observable disturbance at as low as 40 m ASL (Table 3). The quality of the Phantom images at 40 m was satisfactory for counting and classifying seals, and it was considered unnecessary to attempt to fly at a lower altitude.
To perform ground counts, the team was required to land in a zodiac on the site; this resulted in high level disturbance classified by large numbers of fur seals moving rapidly into the water around the landing site. The fur seals returned to shore within 2 h of the team retiring to the hut. Ground counts performed from the plateau did not result in disturbance provided the team remained undetected by smell or sight. With repeated counts, counters were typically detected at some point by the fur seals resulting in low to high disturbance levels. Upon leaving the site, large numbers of fur seals were displaced similar to the high level of disturbance caused upon arrival.
RPA Survey Design
RPA survey methods were adjusted depending on image quality and disturbance levels of wildlife (Tables 2, 3). A process of trouble shooting was undertaken after each survey to improve the methodology (Table 3). Image resolution was not clear enough from the Gryphon RPA at 80 or 60 m ASL (Figures 2A,B) until a 28 mm lens was employed (Figure 2C); however, this was not an appropriate system because the resulting increase in number of transects per survey increased exposure to the fur seals and caused a high level of disturbance that was not acceptable. A higher resolution capture setup could possibly solve this, but the Phantom was able to produce high quality images (Figures 2D,E) with no disturbance and was considered a better and more user-friendly system for the task.
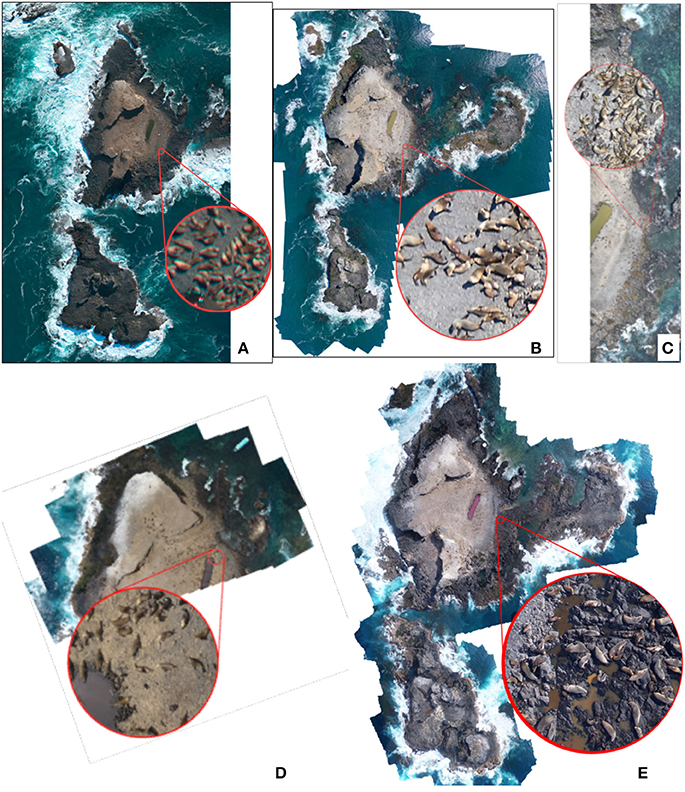
Figure 2. Stitched images from trials (Left to right: upper A–C and lower; D,E), with inset zooms to show resolution and clarity achieved. (A) Gryphon Dynamics RPA survey 1, using a 16 mm lens and 42 MP sensor at 80 m ASL. Resolution is not high enough to be useful for research purposes. (B) Gryphon Dynamics RPA Survey 2 using a 16 mm lens and 42 MP sensor at 60 m ASL. Resolution is higher with this configuration than from the first survey (A) due to lower flight altitude. (C) Gryphon Dynamics RPA survey 3 (part survey only), flown at 60 m ASL using a 28 mm lens and 42 MP sensor. Detail of the seals is of a higher resolution than earlier surveys (A,B) due to narrower field of view. (D) Phantom 4 RPA survey 4, flown at 60 m ASL, using 20 mm equiv. lens and 12.4 MP sensor. (E) Phantom 4 Pro RPA, flown at 40 m ASL. Twenty-four millimeters equivalent lens, 20 MP sensor. Resolution and image clarity here are at a level where identifying individual animals, even when clustered and on dark rocks, is possible. Image is of similar resolution to the larger RPA at 60 m with 28 mm lens (C).
During the Phantom surveys, we experienced some flights returning empty SD cards or dramatic errors in capture settings (white balance changes in the order of thousands of Kelvin, i.e., blue images). Through careful testing, we now theorize that these malfunctions were caused by the DJI communications system jumping channels automatically, causing a break in the functionality of Drone Deploy. By manually selecting a channel with low traffic, and locking the system to that, a continuous connection was maintained for the duration of the surveys, and the problem has not been experienced since adding this step to our pre-flight process; DJI signal boosters were also used to enhance the reliability of communications. Flight 1 (Seal Rock) and Flight 2 (Black Rock and East Reef) respectively, return with ~38 and 50% battery capacity remaining.
Performing flights with the midday sun (central position in the sky) was identified through trials to be more critical to seal detection in RPA images than tidal amplitude. It is possible; however, that seals are more mobile at maximum high tide than at other times because of displacement from the water. Seal movement made it more difficult to stitch images together using Autopano Giga, but Agisoft Photoscan Professional achieved good results (Table 4).
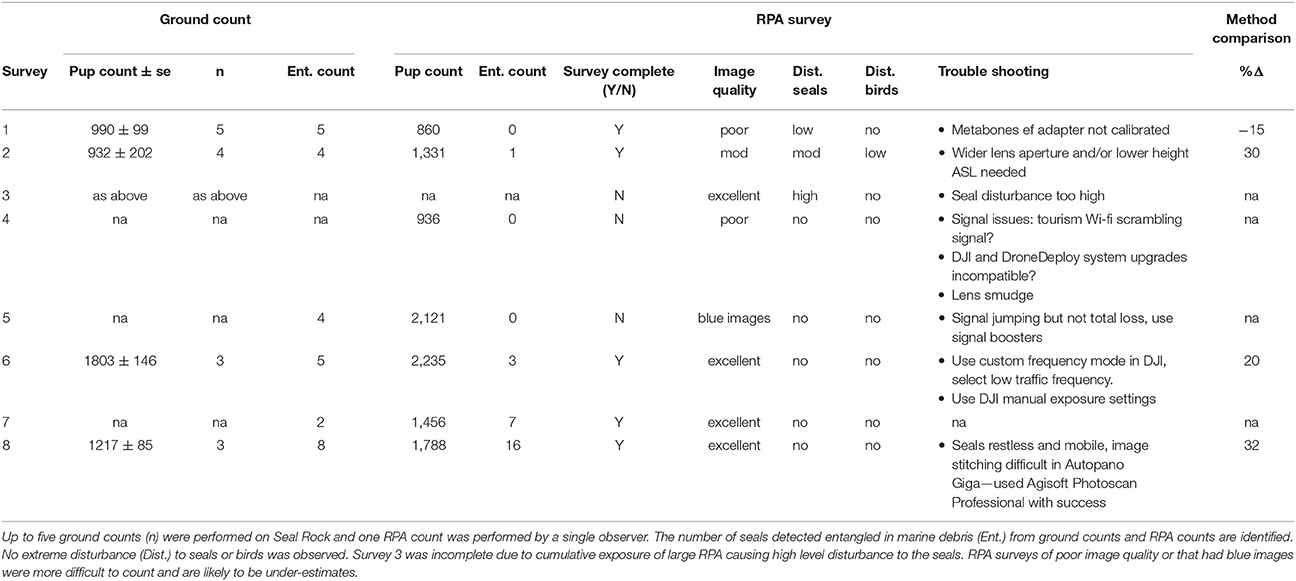
Table 4. Comparison of number of pup detections from the average ground count at Seal Rock (± the standard error, se) and the number of seals counted by an expert from RPA images (RPA survey) of Seal Rock, including the method comparison as the difference between the counts (%Δ).
Ground Counts vs. RPA Counts
Ground counts of pups were only performed for Seal Rock, whereas RPA pup counts were obtained for Seal Rock, Black Rock, and East Reef. To allow comparisons of RPA counts with ground counts, we extracted the Seal Rock RPA count from the Total count (Table 4). Once troubleshooting had resulted in high quality image resolution, the RPA counts of pups were between 20 and 32 % higher than the ground counts of pups and there were more counts of seals with marine debris entanglements in the high quality RPA images (Table 4). For Survey 1 using the Gryphon (Table 4), the ground pup and entanglement counts were higher than the RPA count because the resolution of the RPA images was too poor to count the pups or identify entanglements in the images.
Discussion
The best system to use for surveying Australian fur seals at Seal Rocks was a DJI Phantom Pro 4. This system included a small (<2 kg) and unobtrusive RPA in terms of noise and visibility. Using the smaller system allowed transects to be flown at a lower altitude and tighter transect configuration: this resulted in greater overall exposure to the RPA than a larger RPA flown at higher altitude, however the seals and birds did not respond measurably despite this. Additional benefits were that the smaller weight class exposes operators to fewer regulatory requirements and it is extremely economical in contrast to contracting licensed operators for larger RPA systems and more technical gear. Given the lack of observable disturbance caused by this system, we recommend that providers planning on using RPAs for seal censuses use these trials as a starting point for their own surveys to fast-track their success. A generalized conclusion was that larger systems should remain a minimum of 80 m altitude ASL and smaller systems can provide the required image resolution at 40 m altitude ASL for surveying Australian fur seals. Other fur seal species and wildlife may be more or less sensitive to these stimuli, and localized testing with appropriate permits should be undertaken to ensure best practice.
Success for the RPA method being tested was dependent on obtaining high quality images that could be used to count the seals. Pup counts can vary by time of year: being lowest at the start of the pupping period, reaching a peak peaking when most pups are born at the end of the pupping period and then typically reducing and becoming more variable from mortality and increased pup mobility with age (McIntosh et al., 2012). The highest pup counts obtained from the RPA surveys were achieved by a combination of image quality and timing in relation to the fur seal breeding season. The highest quality image resolution and the highest pup counts were obtained on 16 and 28 Dec 2016 (Surveys 5 and 6, Tables 2, 4) by the Phantom 4 flown at 40 m ASL and after peak pupping when maximum pups are available for counting (Gibbens and Arnould, 2009). The two lowest RPA pup counts were Survey 1 that had low image resolution and Survey 4 that occurred at the start of the breeding season (2 Dec 2016) when few pups were available to count. Entangled seals were detected in the RPA images and at a greater number than the ground count in Surveys 7 and 8 (Table 4), when image quality was the highest. These preliminary results for RPA surveys are highly encouraging.
The weather was also an important consideration for survey performance. An overcast day at noon (or when the sun is central in the sky), with low wind speed and no rain provided the best conditions for standardizing light and image quality. Tidal amplitude was another important consideration for terrestrial sites in marine systems. Originally we thought that low tide might be the worst time to obtain a survey because the black pups would be placed against wet black rocks and have limited contrast for detection in RPA images. We prioritized reducing the effect of shadows on the trials to maximize image resolution and therefore did not have adequate replication of surveys to test the impact of tide on the counts. However, pups could be counted at all tidal amplitudes, provided the image quality was high. Testing of individual systems for the target species will be required to understand the ideal conditions specific to that system.
The time taken to count pups on a ground count was ~2 h. It took ~3 h to just count pups from the RPA images (Table 4). However, the benefits of the RPA counts are that we can revisit the images to gain additional data (count other age classes), the counts are likely to be more accurate and more precise and they are obtained with less disturbance to wildlife, as well as reduced logistics and personal accident risk.
The portal titled “Seal Spotter” used for obtaining data counts was accessible, flexible and efficient. It was easy to navigate through and count fur seals. Any number of categories could be designated by name and color for counting (Figure 3) and the data was easily stored and manipulated to summarize the statistics and visualize the data (Figure 4). In this study, only one replicate of images was counted; however, any number of independent replicate image counts can be programmed into the portal.
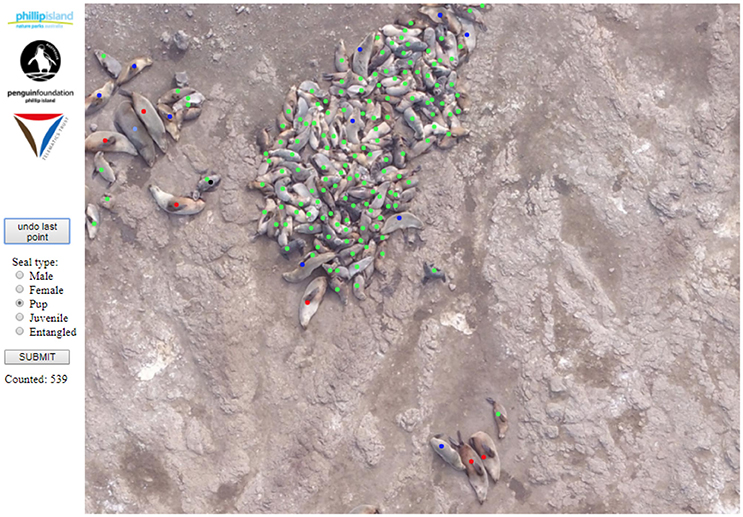
Figure 3. Screenshot of “SealSpotter” web portal. Users select animal types using radio buttons in the left side panel, then mark animals on the image before submitting the data for storage on the server, and subsequent collation and analysis. User name, precise click timestamps, and user comments, are also collected as part of this process.
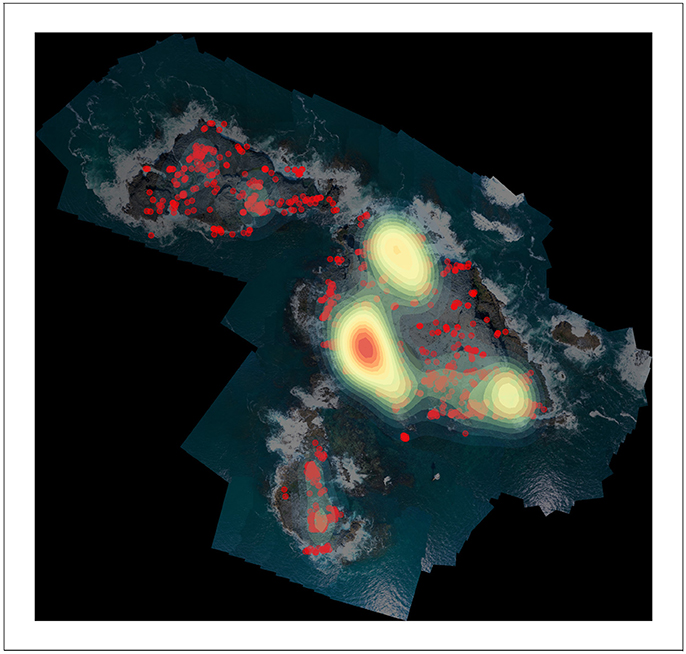
Figure 4. Mapped x and y co-ordinate data (red dots) for Australian fur seal pups at Seal Rocks counted from Unpiloted Aerial Vehicle (RPA) images obtained on Survey 3, 12 October 2016. Overlayed heat map shows the density of aggregated pups from less dense (yellow) to more dense (red).
Final Protocol for RPA Survey of the Fur Seals on Seal Rocks
The final time requirement for a Phantom Pro 4 survey of Seal Rocks was ~60 min from arrival at the take-off and landing site to departure. Each flight included ~18 min total flying time, during which the RPA was over the colony area for ~12–13 min, spread evenly over the colony area. After flight one, the SD card was removed and checked onsite for image quality and completion, the battery changed and the second flight performed and checked. Battery power, site topography and RPA flight regulations are the main constraints for survey design and this will be unique for different sites.
Due to our experiences of technical glitches resulting in incomplete or compromised surveys, researchers would be well-advised to allow for occasional repeat flights in order to troubleshoot any problems as they arise. Once we had determined the suitable height limitations and equipment that avoided disturbance, the AEC authorized up to four attempts per survey (equalling a maximum of four possible exposures to the RPA per survey) should first or subsequent attempts fail and there be no observed high to extreme disturbance of the seals and birds. If a problem could not be solved within the four attempts, it was considered undesirable to continue testing over wildlife and testing was to be performed elsewhere.
A basic protocol using a <2 kg Phantom 4 Pro with Drone deploy and DJI software is:
• 40 m above sea level—this is the height where there is no measurable disturbance to seals or birds at Seal Rocks
• Flight speed of 7 ms−1 with a photo captured every 2 s and ~70% frontlap
• 50% sidelap—enables adequate overlap areas for stitching
• Signal boosters can be used to help maintain signal reliability (not required if take-off and landing point is close to survey location)
• Manual camera settings in DJI Go app to ensure exposure consistency
• “Custom frequency” mode in DJI to avoid channel switching:
◦ Check frequencies for background noise/traffic
◦ Select frequency with least noise
• Camera positioned at nadir (i.e.: angled vertically downward).
Conclusion
We have successfully developed a protocol to survey an Australian fur seal colony with undetectable disturbance to the fur seals and the birds at the site. When image resolution allows for identification of pups, the RPA counts were higher than the ground counts and seals entangled in marine debris were able to be detected. We now plan on examining the precision of these estimates compared to typical methods and applying the methodology to different sites and for different wildlife species. The methods provided here are easily replicated, cost effective, and safe for personnel and wildlife. It is expected that researchers and managers will be able to use the explained methodology to develop their own RPA surveys under best practice principles and with minimal disturbance to wildlife.
Ethics Statement
This study was carried out in accordance with the recommendations of Department of Land Water and Planning, Victoria, Australia under permit number 10007974. The protocol was approved by the Phillip Island Nature Parks Animal Ethics Committee (reference number 2.2016).
Author Contributions
RM developed the central ideas, performed the seal counts and led the writing process. Unless otherwise indicated, RM and RH conducted all RPA fieldwork. RH developed the image-processing code and extracted the data. PD provided management support and all authors contributed critically to the drafts and gave final approval for publication.
Conflict of Interest Statement
The authors declare that the research was conducted in the absence of any commercial or financial relationships that could be construed as a potential conflict of interest.
The reviewer BP and handling Editor declared their shared affiliation.
Acknowledgments
This research was performed under the Animal Ethics Approval 2.2016 and DELWP Research Permit 10007974. Thank you to The Penguin Foundation, Phillip Island Nature Parks and Telematics Trust for funding the project. Thanks to Richard Reina (Monash University) and Rohan Clarke (Monash University) for discussions relating to the project. Thanks to George Pappas Media (Phillip Island) for his availability and enthusiasm and Guava Insights for their time and resources. We thank two reviewers for their contributions to this manuscript.
References
Adame, K., Pardo, M. A., Salvadeo, C., Beier, E., and Elorriaga-Verplancken, F. R. (2017). Detectability and categorization of California sea lions using an unmanned aerial vehicle. Mar. Mam. Sci. 33, 913–925. doi: 10.1111/mms.12403
Alderman, R. (2014). Shy Albatross in Australia: Population and Conservation Assessment. Report for the 2013/14 field season. Department of Primary Industries, Parks, Water and Environment: Hobart, TAS.
Berlincourt, M., and Arnould, J. P. Y. (2015). Influence of environmental conditions on foraging behaviour and its consequences on reproductive performance in little penguins. Mar. Biol. 162, 1485–1501. doi: 10.1007/s00227-015-2685-x
Cormack, R. M. (1964). Estimates of survival from the sighting of marked animals. Biometrika 51, 429–438. doi: 10.1093/biomet/51.3-4.429
Cox, J., Oh, E. Y., Simmons, B., Lintott, C., Masters, K., Greenhill, A., et al. (2015). Defining and measuring success in online citizen science: a case study of Zooniverse projects. Comput. Sci. Eng. 17, 28–41. doi: 10.1109/MCSE.2015.65
Gibbens, J., and Arnould, J. P. Y. (2009). Interannual variation in pup production and the timing of breeding in benthic foraging Australian fur seals. Mar. Mam. Sci. 25, 573–587. doi: 10.1111/j.1748-7692.2008.00270.x
Goldsworthy, S. D., Bulman, C., He, X., Larcombe, J., and Littnan, C. (2003). “Trophic interactions between marine mammals and Australian fisheries: an ecosystem approach,” in Marine Mammals: Fisheries, Tourism and Management Issues, eds. N. Gales, M. Hindell, and R. Kirkwood (Melbourne, VIC: CSIRO Publishing), 62–99.
Gonzalez, L. F., Montes, G. A., Puig, E., Johnson, S., Mengersen, K., and Gaston, K. J. (2016). Unmanned Aerial Vehicles (UAVs) and artificial intelligence revolutionizing wildlife monitoring and conservation. Sensors 16:E97. doi: 10.3390/s16010097
Hamer, D. J., and Goldsworthy, S. D. (2006). Seal-fishery operational interactions: identifying the environmental and operational aspects of a trawl fishery that contribute to by-catch and mortality of Australian fur seals (Arctocephalus pusillus doriferus). Biol. Conserv. 130, 517–529. doi: 10.1016/j.biocon.2006.01.014
Hodgson, J. C., Baylis, S. M., Mott, R., Herrod, A., and Clarke, R. H. (2016). Precision wildlife monitoring using unmanned aerial vehicles. Sci. Rep. 6:22574. doi: 10.1038/srep22574
Hodgson, J. C., and Koh, L. P. (2016). Best practice for minimising unmanned aerial vehicle disturbance to wildlife in biological field research. Curr. Biol. 26, R404–R405. doi: 10.1016/j.cub.2016.04.001
Jones, G. P., Pearlstine, L. G., and Percival, H. F. (2006). An assessment of small unmanned aerial vehicles for wildlife research. Wildlife Soc. Bull. 34, 750–758. doi: 10.2193/0091-7648(2006)34[750:AAOSUA]2.0.CO;2
Kirkman, S. P., Oosthuizen, W. H., Meýer, M. A., Seakamela, S. M., and Underhill, L. G. (2011). Prioritising range-wide scientific monitoring of the Cape fur seal in southern Africa. Afr. J. Mar. Sci. 33, 495–509. doi: 10.2989/1814232X.2011.637354
Kirkwood, R., Pemberton, D., Gales, R., Hoskins, A. J., Mitchell, A., Shaughnessy, P. D., et al. (2010). Continued population recovery by Australian fur seals. Mar. Freshw. Res. 61, 695–701. doi: 10.1071/MF09213
Linchant, J., Lisein, J., Semeki, J., Lejeune, P., and Vermeulen, C. (2015). Are unmanned aircraft systems (UASs) the future of wildlife monitoring? A review of accomplishments and challenges. Mam. Rev. 45, 239–252. doi: 10.1111/mam.12046
Littnan, C. L., and Mitchell, A. T. (2002). Australian and New Zealand fur seals at the Skerries, Victoria: recovery of a breeding colony. Aust. Mam. 24, 57–64. doi: 10.1071/AM02057
Lukyanenko, R., Parsons, J., and Wiersma, Y. F. (2016). Emerging problems of data quality in citizen science. Conserv. Biol. 30, 447–449. doi: 10.1111/cobi.12706
McIntosh, R. R., Goldsworthy, S. D., Shaughnessy, P. D., Kennedy, C. W., and Burch, P. (2012). Estimating pup production in a mammal with an extended and aseasonal breeding season, the Australian sea lion (Neophoca cinerea). Wildlife Res. 39, 137–148. doi: 10.1071/WR10222
McIntosh, R. R., Kirkwood, R., Sutherland, D. R., and Dann, P. (2015). Drivers and annual estimates of marine wildlife entanglement rates: a long-term case study with Australian fur seals. Mar. Pollut. Bull. 101, 716–725. doi: 10.1016/j.marpolbul.2015.10.007
McIntosh, R. R., Shaughnessy, P. D., and Goldsworthy, S. D. (2006). “Mark-recapture estimates of pup production for the Australian sea lion (Neophoca cinerea) at Seal Bay Conservation Park, South Australia,” in Sea Lions of the World, eds. A.W. Trites, S.K. Atkinson, D.P. Demaster, L.W. Fritz, T.S. Gelatt, L.D. Rea, and K.M. Wynne (Fairbanks, AK: Alaska Sea Grant College Program, University of Alaska), 353–367.
McIntosh, R., Sutherland, D., Dann, P., Kirkwood, R., Thalman, S., Alderman, R., et al. (2014). Pup Estimates for Australian and New Zealand fur Seals in Victoria, Tasmania and New South Wales between 2007 and 2013. Report to the Australian Marine Mammal Centre, Department of the Environment. (Phillip Island, VIC: Phillip Island Nature Parks).
Nilssen, K. T., Johansen, K.-S., Storvold, R., Stødle, D., Poltermann, M., Solbø, S. A., et al. (2014). Testing UAVs to Perform Aerial Photographic Survey of Harp and Hooded Seals in the West Ice Area. Survey Report – KV “Svalbard” 16-26 March 2014. Institute of Marine Research Report, 15.
Peter, J., and Dooley, S. (2014). The fatal shore. Aust. Birdlife 3, 24–27. Available Online at: https://en.calameo.com/read/0041078952d9be0708d3e
Pomeroy, P., O'connor, L., and Davies, P. (2015). Assessing use of and reaction to unmanned aerial systems in gray and harbor seals during breeding and molt in the UK1. J. Unmanned Vehicle Syst. 3, 102–113. doi: 10.1139/juvs-2015-0013
Radiansyah, S., Kusrini, M. D., and Prasetyo, L. B. (2017). “Quadcopter applications for wildlife monitoring,” in IOP Conference Series: Earth and Environmental Science (Bogor), 54.
R-Development-Core-Team (2005). R: A Language and Environment for Statistical Computing. Vienna: R Foundation for Statistical Computing. Available online at: http://www.R-project.org.
Robinson, S., Terauds, A., Gales, R., and Greenwood, M. (2008). Mitigating fur seal interactions: relocation from Tasmanian aquaculture farms. Aqu. Conserv. 18, 1180–1188. doi: 10.1002/aqc.971
Seber, G. A. F. (1982). The Estimation of Animal Abundance and Related Parameters. New York, NY: MacMillan.
Seymour, A. C., Dale, J., Hammill, M., Halpin, P. N., and Johnston, D. W. (2017). Automated detection and enumeration of marine wildlife using unmanned aircraft systems (UAS) and thermal imagery. Sci. Rep. 7:45127. doi: 10.1038/srep45127
Swanson, A., Kosmala, M., Lintott, C., and Packer, C. (2016). A generalized approach for producing, quantifying, and validating citizen science data from wildlife images. Conserv. Biol. 30, 520–531. doi: 10.1111/cobi.12695
Keywords: citizen science, disturbance, drone, ecosystem health, marine debris entanglement, fur seals, population abundance, wildlife monitoring
Citation: McIntosh RR, Holmberg R and Dann P (2018) Looking Without Landing—Using Remote Piloted Aircraft to Monitor Fur Seal Populations Without Disturbance. Front. Mar. Sci. 5:202. doi: 10.3389/fmars.2018.00202
Received: 14 December 2017; Accepted: 18 May 2018;
Published: 06 June 2018.
Edited by:
Rob Harcourt, Macquarie University, AustraliaReviewed by:
Benjamin James Pitcher, Macquarie University, AustraliaMaritza Sepulveda, Universidad de Valparaíso, Chile
Copyright © 2018 McIntosh, Holmberg and Dann. This is an open-access article distributed under the terms of the Creative Commons Attribution License (CC BY). The use, distribution or reproduction in other forums is permitted, provided the original author(s) and the copyright owner are credited and that the original publication in this journal is cited, in accordance with accepted academic practice. No use, distribution or reproduction is permitted which does not comply with these terms.
*Correspondence: Rebecca R. McIntosh, cm1jaW50b3NoQHBlbmd1aW5zLm9yZy5hdQ==