- 1Biology Department, Dalhousie University, Halifax, NS, Canada
- 2Marine Affairs, Dalhousie University, Halifax, NS, Canada
- 3Science Branch, Fisheries and Oceans Canada, Gulf Fisheries Centre, Moncton, NB, Canada
Globally, the production of marine bivalves has been steadily increasing over the past several decades. As the effects of human population growth are magnified, bivalves help provide food security as a source of inexpensive protein. However, as climate change alters sea surface temperatures (SST), the physiology, and thus the survival, growth, and distribution of bivalves are being altered. Challenges with managing bivalves may become more pronounced, as the uncertainty associated with climate change makes it difficult to predict future production levels. Modeling techniques, applied to both climate change and bivalve bioenergetics, can be used to predict and explore the impacts of changing ocean temperatures on bivalve physiology, and concomitantly on aquaculture production. This study coupled a previously established high resolution climate model and two dynamic energy budget models to explore the future growth and distribution of two economically and ecologically important species, the eastern oyster (Crassotrea virginica), and the blue mussel (Mytilus edulis) along the Atlantic coast of Canada. SST was extracted from the climate model and used as a forcing variable in the bioenergetic models. This approach was applied across three discreet time periods: the past (1986–1990), the present (2016–2020), and the future (2046–2050), thus permitting a comparison of bivalve performance under different temporal scenarios. Results show that the future growth is variable both spatially and interspecifically. Modeling outcomes suggest that warming ocean temperatures will cause an increase in growth rates of both species as a result of their ectothermic nature. However, as the thermal tolerance of C. virginica is higher than M. edulis, oysters will generally outperform mussels. The predicted effects of temperature on bivalve physiology also provided insight into vulnerabilities (e.g., mortality) under future SST scenarios. Such information is useful for adapting future management strategies for both farmed and wild shellfish. Although this study focused on a geographically specific area, the approach of coupling bioenergetic and climate models is valid for species and environments across the globe.
Introduction
With a growing human population expected to surpass 9 billion by 2050, the cultivation of bivalves helps to provide food security as a source of inexpensive protein (Shumway et al., 2003; Godfray et al., 2010). Globally, the production of bivalves has been steadily increasing over the past several decades and is expected to continue to do so (FAO, 2016). Considered ecosystem engineers as well as keystone species (Gutiérrez et al., 2003; Zippay and Helmuth, 2012; Han et al., 2017; Sorte et al., 2017), bivalves interact with their environment through both top-down and bottom-up processes (e.g., Coen et al., 2007; Rice, 2008). Top-down control via filter-feeding may significantly curtail phytoplankton populations (Cranford et al., 2003; Newell, 2004; Forsberg et al., 2017) potentially affecting bivalve performance itself (Dame and Prins, 1998; Bacher et al., 2003; Strohmeier et al., 2005), but also impacting other filter-feeders and grazers (Kluger et al., 2017). Filtration activity can also play an important role in regulating water quality and depth of light penetration (Gallardi, 2014; Guyondet et al., 2015; Petersen et al., 2016). Bivalves can also impose bottom-up control on plankton communities by altering fluxes of nutrients (Menge, 1992; Newell, 2004). Bivalves change geographical patterns in energy transfers from the pelagic environment to the benthos, since feces and pseudofaeces contribute to augmenting organic matter directly below farms (Newell, 2004; Cranford et al., 2007, 2009) and filtration acts in reducing organic matter deposition elsewhere in the system (Guyondet et al., 2015).
Interactions between bivalves and their environment are bidirectional, meaning the abiotic environment also imposes effects on bivalves. Temperature plays an important role in physiology, gene expression, distribution, and fitness of bivalves (Zippay and Helmuth, 2012; Shelmerdine et al., 2017). The internal body temperature of bivalves usually matches external water temperatures, except when intertidal species are subject to aerial exposure (Zippay and Helmuth, 2012). Although the effect of temperature on physiology is species-specific, generally as temperature increases, physiological rates will increase until a threshold is met, at which point performance will decline (Kooijman, 2010). Due to this relationship between physiological functions (e.g., filtration rates and oxygen consumption), short-term changes (days/weeks) in temperature will predictably impact their survival (e.g., Malham et al., 2009; Rinde et al., 2016) and long-term changes (years) will impact their reproductive timing and effort, and consequently their spatial distribution (Bayne et al., 1976; Philippart et al., 2003; Kittner and Riisgård, 2005; Toupoint et al., 2012; Zippay and Helmuth, 2012; Filgueira et al., 2014). Accordingly, the relationship between temperature and physiology of bivalves is particularly relevant within the context of climate change.
The Intergovernmental Panel on Climate Change (IPCC) has reported that since 1971 global ocean surface temperatures have increased on average 0.11°C (±0.02 SD) per decade (Pachauri and Mayer, 2015). Although temperature will not be the only change to impact bivalves, it has been shown to be the most deterministic factor influencing shellfish growth and distribution, and is one of the most widely studied abiotic factors related to climate change (Zippay and Helmuth, 2012; Rodrigues et al., 2015; Buckley and Huey, 2016; Filgueira et al., 2016). Warming oceans are modifying current natural ranges of many marine species (e.g., Diederich et al., 2005; Jones et al., 2009, 2010; Rinde et al., 2016; Shelmerdine et al., 2017). Despite inherent uncertainty, modeling techniques which incorporate both climate data and organismal bioenergetics are the only tools available to explore the effects of future climate change scenarios on animal populations.
Climate models are quantitative representations of natural processes that make up Earth's conditions, and are often used to predict the effects of climate change (Pachauri et al., 2014). Currently, global emissions of CO2 are the best predictor of Earth's surface warming, and are directly related to both human population and economic growth (Pachauri et al., 2014). Outputs from these models include estimated surface, air, and water warming, ice cover, and change in circulation patterns (Pachauri et al., 2014). Refining the scale of global climate models promotes understanding how climate processes and conditions will change on local scales at highly detailed spatial resolutions. This has been done for the Northwest Atlantic shelf region of Canada (Brickman and Drozdowski, 2012), integrating atmospheric and oceanic information to estimate future sea surface temperature (SST) and salinity of the Scotian Shelf and Gulf of Saint Lawrence (Long et al., 2016).
Regarding bioenergetics, Dynamic Energy Budgets (DEB) provide a mathematical method for modeling energetic flows through individual organisms (Kooijman, 2010). DEB models breach interdisciplinary boundaries by merging the principles of thermodynamics, physiology, and theoretical biology. The mechanistic nature of DEB models permits its application to a wide range of environmental conditions. DEB has been parameterized for several bivalve species (e.g., Pouvreau et al., 2006; Crassostrea gigas; van der Veer et al., 2006; Macoma balthica, Mya arenaria, Cerastoderma edule, Mytilus edulis, Crassostrea gigas; Filgueira et al., 2014; Crassostrea virginica) and used to predict their growth (e.g., Lavaud et al., 2017; Crassostrea virginica), and reproductive effort (Montalto et al., 2016; Brachidontes pharaonis, Mytilaster minimus, Mytilus galloprovincialis). The coupling of climate and growth models is being used under the context of climate change to explore the effect of predicted temperatures on the performance and distribution of organisms (e.g., Sarà et al., 2011, 2013; Brachidontes pharaonis; Thomas et al., 2011, 2015; Mytilus edulis, Crassostrea gigas; Klinger et al., 2017).
The development of the bivalve aquaculture industry is contextualized by climate change and its concomitant effects on the oceans, given its reliance on natural environmental conditions. Climate change is generating uncertainty around future production levels of bivalves, which makes creating resilient government policies increasingly difficult (e.g., Rodríguez-Rodríguez and Roberto, 2017). On the Atlantic coast of Canada, it has been recognized that SST is increasing at a rate higher than the global average (IPCC, 2013; Saba et al., 2016). As these changes are predictable, impacts to cultivated bivalve species can be anticipated, and their effects could be mitigated with management plans. In the present study, the future growth of two widely cultivated bivalve species in Atlantic Canada (Mytilus edulis and Crassostrea virginica) are estimated by coupling their bioenergetics to a high resolution climate model. In this way, bivalve growth and performance can be predicted for the coming decades, to prepare for the impacts of climate change on the industry. The findings of this study are relevant for planning bivalve farming, in terms of both site- and species-selection.
Methods
Description of Study Area
The study area for this research is the Scotian Shelf and Gulf of Saint Lawrence, the bodies of water surrounding Nova Scotia (NS) and Prince Edward Island (PEI) (Latitude: 42.7130–49.0416, Longitude: −67.1065 to −59.0403). The study area contains widely varying temperatures both seasonally and spatially. Colder waters in the Bay of Fundy reach an average summer high of 13°C, however water temperatures in the sheltered Northumberland Strait (e.g., the body of water between NS and PEI) may exceed 20°C (Feindel et al., 2013). Furthermore, this area is extensively used for bivalve aquaculture, primarily the eastern oyster (Crassostrea virginica), and the blue mussel (Mytilus edulis). Culture methods consist primarily of long-lines, floating bags, and oyster bottom culture (DFO, 2003a,b).
Climate Change Model
The climate change model used in this study was produced by the Department of Fisheries and Oceans Canada, as a part of the Canadian Government's Aquatic Climate Change Adaptation Services Program. Unlike other Global Climate Models (GCMs), this is a high resolution (1/12°) model of regional climate dynamical downscaling system of the Gulf of Saint Lawrence, Scotia Shelf, and Gulf of Maine (Long et al., 2016). The model domain, for the use in this research was restricted to the waters surrounding NS and PEI (Latitude: 42.7130–49.0416, Longitude: −67.1065 to −59.0403). The model is constructed of the atmospheric Canadian Regional Climate Model (CRCM), and the oceanic model the Canadian Océan PArallélisé (CANOPA) model. The CANOPA model was developed at the Bedford Institute of Oceanography (Brickman and Drozdowski, 2012), based on the Océan PArallélisé model, version 9 (OPA 9.0; Madec et al., 1998), and the Louvain-la-Neuve ice model, version 2 (LIM2; Fichefet and Morales Maqueda, 1997; Bouillon et al., 2009). The model covers the time period from 1970 to 2100 under the A1B and Representation Concentration Pathway (RCP) 8.5 scenario (Long et al., 2016). The output is produced in grid cells with dimensions of 5–6 km horizontally. River inputs are included in the model; however tidal forcing is not used. A full description of the model can be found in Long et al. (2016)
Bioenergetic Model: Dynamic Energy Budget (DEB)
The bivalve models used in this study are based on DEB theory (Kooijman, 1986, 2010). DEB models define how energy is moved through an individual by describing them with three standard state variables: reserves, structure, and maturity/reproduction (depending on the life stage). Energy moves as organisms assimilate food, which is first stored as reserves. Subsequently, a fraction of reserves (κ) is directed toward growth and maintenance of the structure of the individual, and the rest (1 − κ) is allocated to reproductive maturity in juveniles, and reproduction in adults (specifically, gametes). The notations and symbols used in this text are consistent with Kooijman (2010) where square brackets [] denote quantities per unit structural volume, braces {} denote quantities per unit surface-area of the structural volume, and rates are defined by dots above their symbol. The model equations are described briefly in Table 1, and further details of the model can be found in Pouvreau et al. (2006). The models were run for a full year, beginning on January 1st, and were initialized with the same length for both species (2 cm) and dry flesh mass (DFM), including structural weight only, of 0.013 g and 0.02 g for C. virginica and M. edulis respectively.
The parameterization of the DEB models (Table 2) followed existing studies, Lavaud et al. (2017) for C. virginicia and Rosland et al. (2009) and Saraiva et al. (2011) for M. edulis. The models were calibrated using the scaled functional response (f) as a simplified proxy for food availability. This parameter originates from the Holling Type II response used in DEB theory (Kooijman, 2010):
Where X is food density (or availability) and Xk is the half-saturation coefficient, which represents the time and energy an individual allocates to searching for food. As the value of f moves from 0 to 1, increasingly less time and energy is spent looking for food. Using this relationship, f was simplified as a proxy ranging between 0 and 1 for food availability where low values of f (i.e., f = 0) reflect low food availability, and high values of f (i.e., f = 1) reflect food availability at saturation (Kooijman, 2010). This proxy does not incorporate potential negative effects of high food availability. This proxy was used to calibrate the growth rates of both C. virginica and M. edulis. Calibration was completed to ensure that growth rates were within biologically reasonable ranges. This was done by comparing C. virginica and M. edulis growth from the literature with model outputs (Table 3). Growth rates were calculated following Clausen and Riisgård (1996):
Where Wt and W0 are the average dry weight or shell length on Day 0 and Day t respectively.
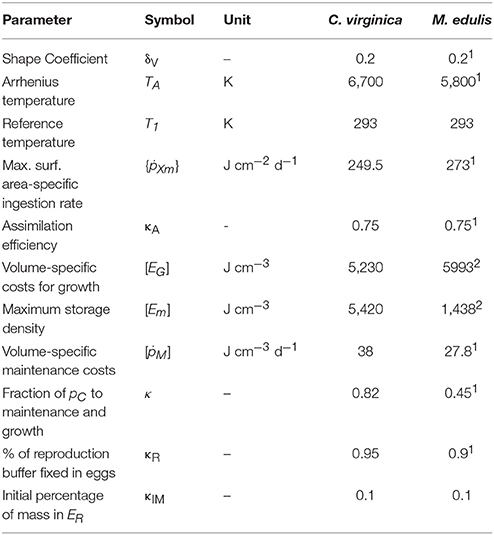
Table 2. Standard DEB parameters for Crassostrea virginica (from Lavaud et al., 2017) and Mytilus edulis (from Rosland et al., 20091; Saraiva et al., 20112).
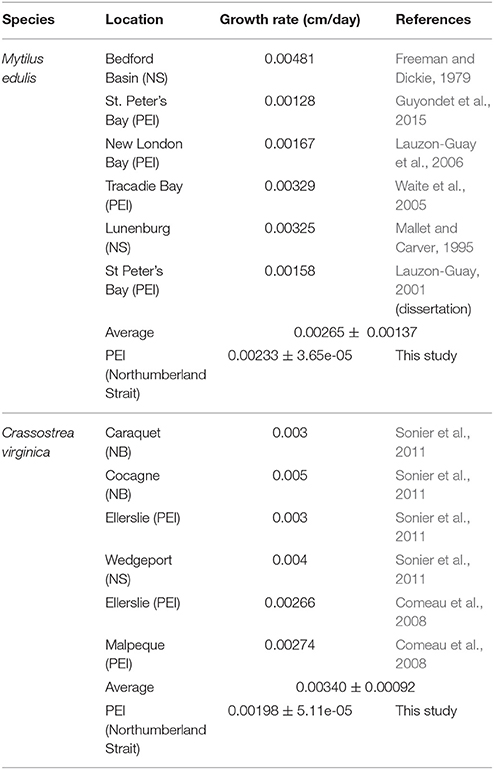
Table 3. Review of observed growth rates of Mytilus edulis and Crassostrea virginica used to validate the DEB model output.
Coupled Model
The DEB models for C. virginica and M. edulis were coupled to the high resolution ocean climate model (Long et al., 2016) following an off-line scheme. The forcing variable used from the climate model was seawater temperature. Temperature data was extracted from the model between the years of 1980 and 2050 for each grid square in the region of interest, and then the top two depth measurements (0 and 12 m) were daily-averaged. Model outputs were available twice a month, and linear interpolation was used to estimate data points between observations. Three periods were studied by averaging 5 year periods, 1985–1990, 2016–2020, 2046–2050, representing the past, present, and future scenarios respectively. Averages were used to minimize the impact of potential outliers.
Data Analysis and Numerical Experiments
To determine how temperature changed both spatially and temporally, past SST data (1986–1990) was subtracted from future SST data (2046–2050), for the entire region being analyzed. This was done for two periods during the year, by averaging the temperature for January and August, the coldest and warmest months, respectively. To determine how thermal stress may change over time, the number of consecutive days exceeding physiologically relevant thermal thresholds (°C) were calculated for comparison between the three times periods. This was done for warm temperatures relevant for the upper thermal threshold of M. edulis and cold temperatures relevant for the lower thermal threshold for C. virginica. The upper thermal threshold used for M. edulis in this DEB model is 23°C (Rosland et al., 2009), however increased mortality rates have been observed in laboratory conditions at 22°C (Clements et al., 2018). Similarly, the lower thermal threshold of C. virginica used for this model was 2°C (Lavaud et al., 2017), however behavioral changes (e.g., filtration rates decrease by 50%) have been observed at 9°C (Comeau et al., 2008). This was done for the warmest and coldest areas relevant for bivalve aquaculture contained in the model domain. For this, only coastal areas were considered. To explore the species-specific effects of SST changes, growing degree days (GDD) were calculated for both C. virginica and M. edulis across the three time periods, in the warmest area within the model domain relevant for bivalve aquaculture. GDD were calculated as follows:
Where Ti is the SST (°C) on day i, and TTL and TTH are the predetermined lower and upper threshold temperatures respectively (°C), and was a determine time-step (1 day). GDD is in units of °C × day, and was calculated for 1 year (January 1–December 31). Lower and upper thermal tolerances for C. virginica were 2°C and 35°C (Lavaud et al., 2017), respectively, and −1.8°C and 23°C (Saraiva et al., 2011) were used for M. edulis.
To estimate the physiological performance of both species, shell length (SL), dry weight (DW), and gonadosomatic index (GSI), were estimated for the present time period after 1 year of growth, for the warmest and coldest areas relevant for bivalve aquaculture within the model domain. GSI was calculated as a ratio of reproductive tissue to total dry weight. To determine how these indices changed spatially and temporally, percent change was calculated for both SL and GSI in the entire model domain as follows:
To estimate changes in phenology across the model domain, the earliest spawning dates of both species were estimated using a combination of temperature and GSI thresholds, wherein both conditions must be met for a spawning event to occur. The temperature thresholds for C. virginica and M. edulis were 17°C (DFO, 2003a), and 14°C (Newell and Moran, 1989), respectively. Both of these temperature thresholds reflect relatively conservative estimates. Additionally, GSI thresholds of 0.2 (Choi, 1992) and 0.28 (Troost et al., 2010) for C. virginica and M. edulis, respectively. On the first day that both thresholds were met, spawning was triggered in the model by emptying reproductive reserves.
All statistical analyses were performed in Rstudio version 3.1.2. For all parametric analyses, tests for normality and homogeneity of variance were performed with Shapiro-wilk and Barlett's tests respectively. No data transformations were required; all parametric assumptions were met. For GDD comparisons, 2-way ANOVAs were run. When factors yielded significant effects, post-hoc testing was done using a Tukey test. All α levels were 0.05. No significant interactions between factors were found.
Results
Climate Model
The climate model indicates that SST warming will be spatially dependent within the model domain. Differential warming rates were observed between the past (1986–1990) and the future (2046–2050) scenarios (Figure 1). For example, some areas experienced average August temperatures of 2.5°C higher in the future, than in the past (Figure 1). Smaller changes were observed in January, with the highest absolute change being 1.5°C. SST warming may be more impactful to the upper thermal limits of M. edulis than the lower thermal limits of C. virginica. In the warmest coastal area within the model domain, the number of consecutive days exceeding the upper thermal threshold of M. edulis (23°C; Rosland et al., 2009) increased over the three time periods (between past and future for all temperatures p < 0.05) (Figure 2A). In the past (1986–1990), 6 days in a row exceeding 22°C were expected, compared to the future, where 39 consecutive days could exceed this temperature (Figure 2A). For temperatures closet to the lower thermal threshold of C. virginica (2°C; Lavaud et al., 2017), no significant changes were observed in the number of consecutive days falling below these temperatures (between past and future for all temperatures, p > 0.05; Figure 2B). These were calculated for the coldest coastal area relevant for bivalve farming within the model domain. Further linking temperature and physiology, in the warmest coastal area GDD increased between the past and the future for both species (p < 0.05, Figure 3). In the coldest coastal location, no changes in GDD were observed for either species (Figure 3).
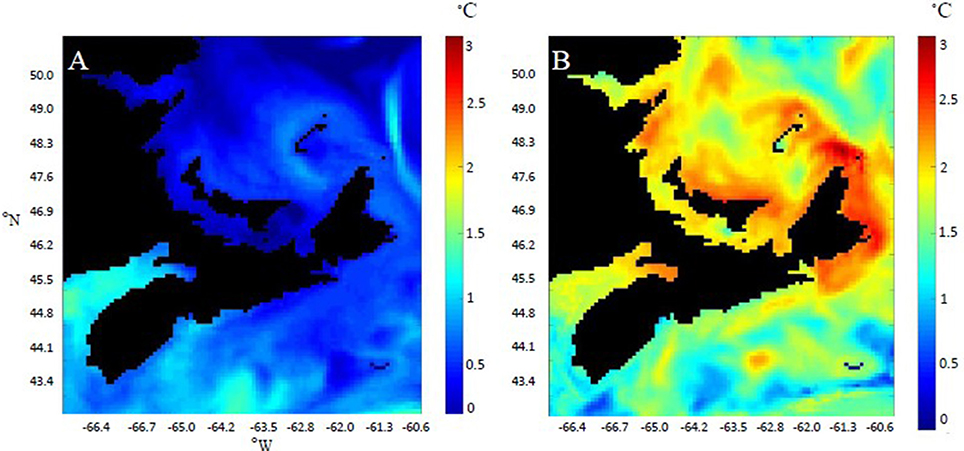
Figure 1. Difference in average temperature (°C) between the past (1986–1990) and the future (2046–2050) for the Gulf of Saint Lawrence and Scotian Shelf areas within the model domain. Temperatures were calculated by averaging the SST values for the month of January (A) and August (B), the coldest and warmest months, respectively.
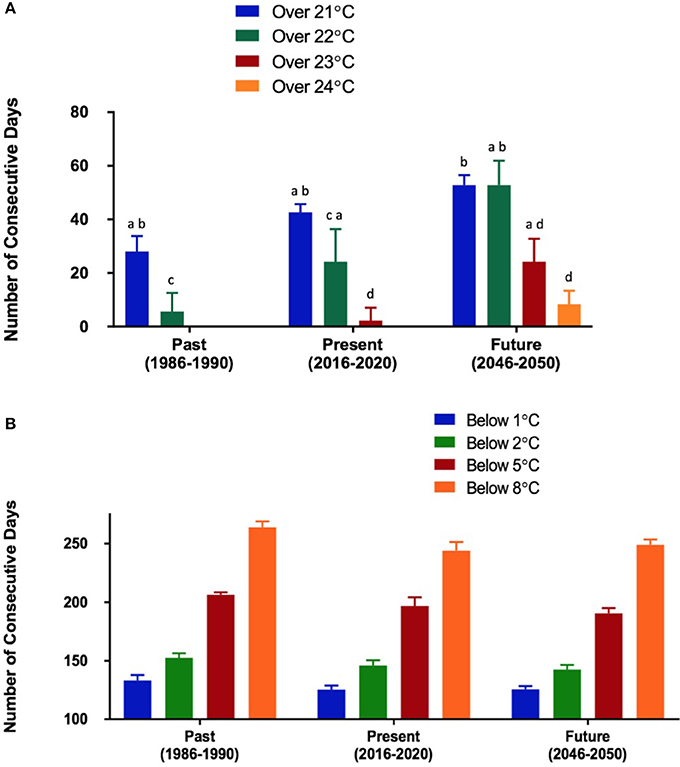
Figure 2. (A) Number of consecutive days that exceeded M. edulis' upper thermal threshold (21°C) (Rosland et al., 2009). Temperature data was taken from the warmest coastal pixel within the model domain. Bars not sharing the same letter indicate significantly different groups (p < 0.05). (B) Number of consecutive days falling below C. virginica's lower thermal threshold (8°C) (Lavaud et al., 2017). Temperature data was taken from the coldest coastal pixel in the model domain. Within each temperature group, no significant effect of time was found (p > 0.05).
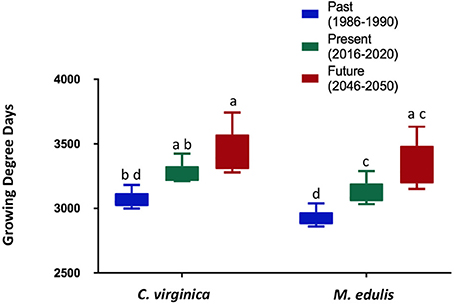
Figure 3. Growing-degree days for C. virginica and M. edulis for each time period analyzed. Upper thermal tolerances were 35 and 23°C, and lower thermal tolerances were 2 and −1.8°C, respectively. This was done for the warmest coastal pixel within the area analyzed. Bars not sharing the same letters indicate significantly different groups (p < 0.05).
DEB Model Validation
The performance of the DEB models for both M. edulis and C. virginica were assessed by comparing growth rates (calculated using shell length) to those observed in the literature for similar geographic regions as those used in this study (Table 3). Using the present output (2016–2020), M. edulis growth rates (0.00233 ± 3.65 × 10−5 cm/day; mean ± SD) matched those observed in the literature (0.00265 ± 0.00137 cm/day) (Table 3). For C. virginica, growth rates produced for the present (0.00198 ± 5.1 × 10−5 cm/day) were below those observed in the literature (0.00340 ± 9.2 × 10−4 cm/day) (Table 3).
Coupled DEB-Climate Model
The performance, as measured by DW, SL, and GSI of both species varied by both speices and location (Figures 4A–H). Generally, the performance of both species was greater (i.e., higher growth rates) in the area with warmer temperatures. Performance as measured by SL and GSI of both species was also visualized for the entire geographic region (Figure 5). The predicted temperature increases are likely to have more observable effects on C. virginica than M. edulis in terms of both length and GSI, over 1 year of growth (Figure 5). In the first year of growth, it was found that C. virginica did not meet the GSI thresholds needed to spawn (Figures 4D,H) either in the present or the future. For M. edulis, the spawning date occurred earlier in the future than in the past, with the largest differential being observed in the Bay of Fundy region (Figure 6).
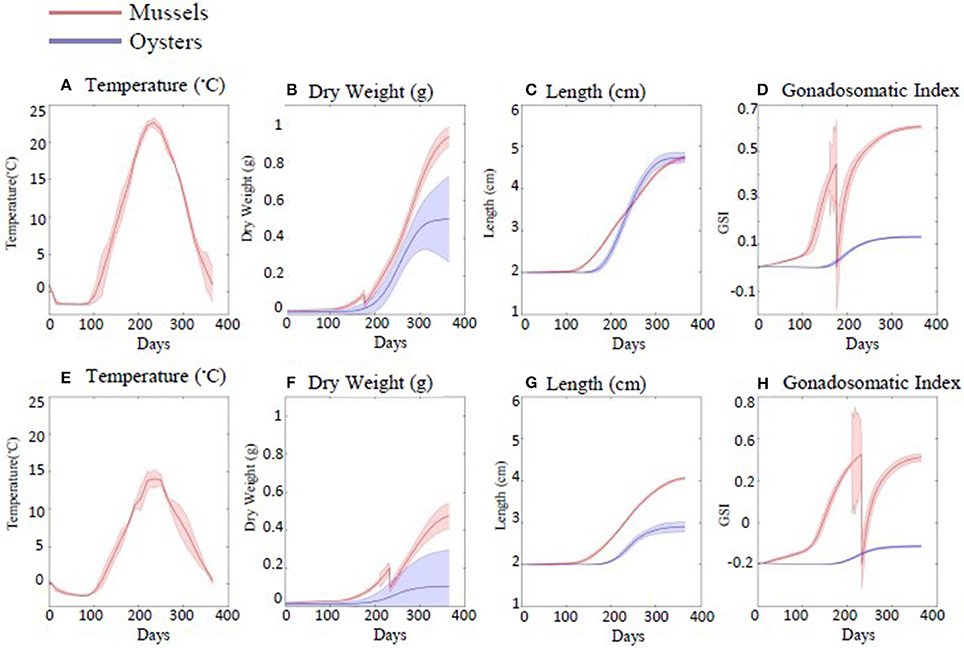
Figure 4. Plots of dynamic energy budget (DEB) output indicating C. virginica and M. edulis growth in dry weight (B,F), length (C,G), and gonadosomatic index (D,H). Over 1 year (beginning on January 1st). This was replicated for areas with two differing thermal regimes, where (A–D) are model outputs from an area with higher average water temperatures than (E–H).
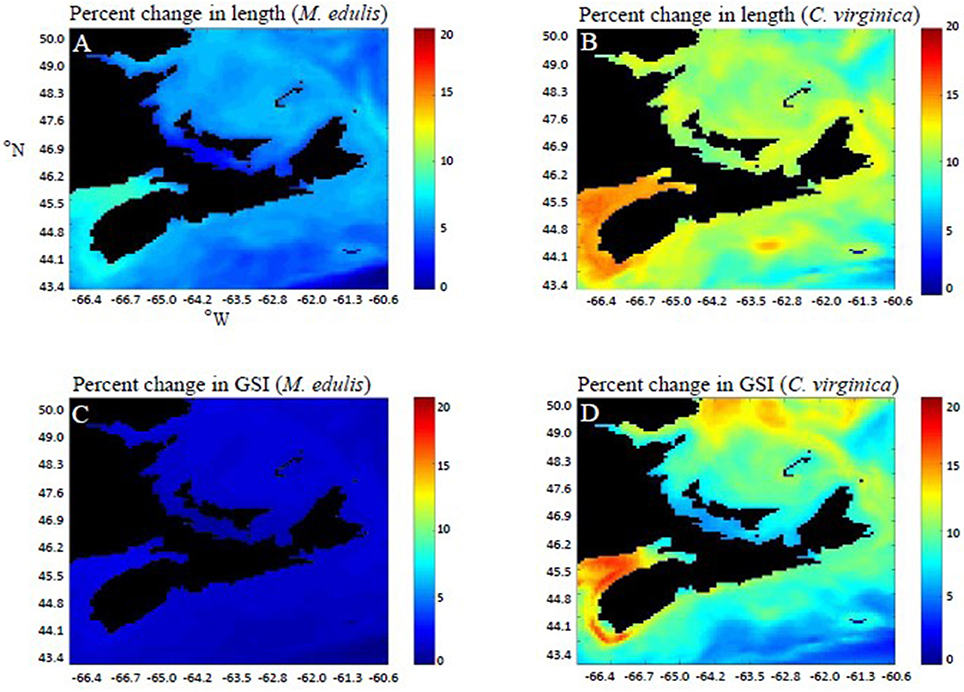
Figure 5. Difference (%) in length (SL) (A,B) and gonadosomatic index (GSI) (C,D) of C. virginica and M. edulis between the past (1986–1990) and the future (2046–2050), calculated after 1 year of growth.
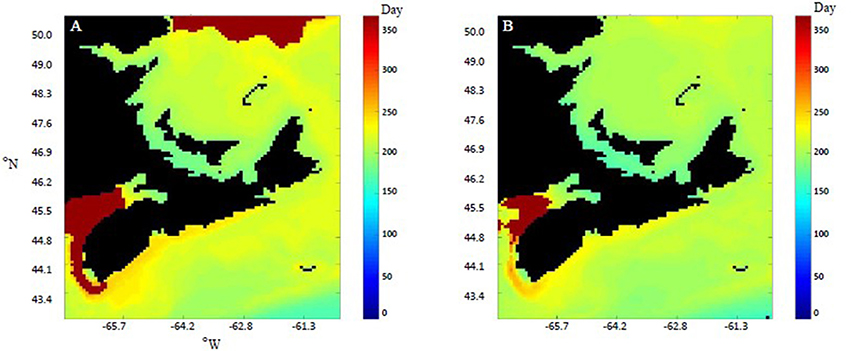
Figure 6. The spawn date of M. edulis indicated in Julian day for the past (A) (1986–1990) and the future (B) (2046–2050). Spawn date was calculated as combination of temperature (14°C) (Newell and Moran, 1989) and GSI (0.28) (Troost et al., 2010) thresholds. Spawn dates above day 365 indicate that spawning did not occur in the first year of growth.
Discussion
Water temperature impacts the physiology of bivalves in species specific ways for C. virginica and M. edulis. Downstream effects of temperature changes on these species have the potential to impact distribution, performance, and phenology (Zippay and Helmuth, 2012; Shelmerdine et al., 2017). In this study, a high resolution climate model was coupled to bioenergetic models of C. virginica and M. edulis to explore the differential effects of predicted SST changes on these commercial species in the coming decades. By simulating the growth of both of these species over a year, throughout different time periods, the effect of temperature on performance in terms of dry weight, shell length, and reproductive effort was examined for both species.
Climate Model
Results from the climate model indicated variable rates of warming within the model domain. Temporally, between the past (1986–1990) and the future (2046–2050), SST warmed for all areas. For seasonal extremes (January and August) in terms of absolute warming, larger changes are predicted for the summer compared to the winter (Figure 1). Spatially, SST increased differentially; in January, the southern Scotian Shelf region experienced the most warming, and in August higher rates of absolute warming were observed in coastal areas around northern NS and the northern shore of PEI (Figure 1). These results match predictions for Canada's mid-high latitude waters in the Scotian Shelf and Gulf of Saint Lawrence (Feindel et al., 2013; Bush et al., 2014). The climate model used in this research has a high spatial resolution, however it does not capture inner coastal bays where aquaculture is carried out. Although it is difficult to create climate change models at high enough resolution to capture the temperatures in these bays (e.g., Stobart et al., 2016), adding 1°C to coastal temperatures in PEI may provide a closer estimation to current bay/inlet temperatures (Filgueira et al., 2015). This variability in SST warming both spatially and temporally indicates that climate change, in terms of ocean warming, will have variable impacts on bivalve bioenergetic processes.
DEB Model
DEB models, as mechanistic tools, currently require calibration to the local environmental conditions where they are applied (e.g., Bernard et al., 2011; Picoche et al., 2014). The application of DEB to future scenarios is then limited to the availability of projected climate data, namely temperature and food availability. Inherently, these estimations are impacted by uncertainties as outlined by Skogen et al. (2014): scenario uncertainty (the unknown future socioeconomic climate), model uncertainty (flaws within model estimations), and internal uncertainty (inability to detect change until variability of a signal flattens out). Although these uncertainties have been shown to decrease as models are applied to more local scales (Hawkins and Sutton, 2009), they still limit the ability to integrate environmental data into locally calibrated DEB models. This may explain the lower growth rates produced by the C. virginica model in this study, compared to those observed in the literature (Table 3). The DEB model used in this study was originally calibrated using field data from the Gulf of Mexico (Lavaud et al., 2017). The scaled functional response was the only parameter calibrated in this study. Potential ecophysiological (Dittman et al., 1998) or genetic (Murray and Hare, 2006) variability from oyster populations from different latitudes could be missed in the current parameterization of the model, and consequently explain these lower growth rates. This lower growth may also be exacerbated by the conservative temperature estimate of coastal bays, as discussed above.
Although methods for estimating some environmental variables (e.g., temperature) are clearly defined, others (e.g., food availability) are less clear. Defining food availability for bivalves is frequently done using proxies such as phytoplankton concentration (e.g., Riisgård et al., 2012), often represented by chlorophyll (e.g., Lesser et al., 2010). These methods for defining food availability are associated with inherent model uncertainty for current conditions (Smith, 1980), and become even more uncertain when estimating future chlorophyll a concentrations (Elliott et al., 2005). Filgueira et al. (2016), using a spatially explicit model combining the physical environment, aquaculture practices, and climate change drivers, have suggested that climate change (increased SST) may cause a decrease in chlorophyll a concentration in coastal embayments, where bivalve aquaculture is present. There is also potential for the abundance of specific size classes of phytoplankton to shift temporally (Agirbas et al., 2015), indicating the possibility for both the quantity and quality of food availability to change over time. Although temperature has been suggested to be the most deterministic factor for bivalve performance under climate change conditions (Filgueira et al., 2016), forcing predicted food availability onto the bioenergetic model would provide a more comprehensive understanding of how bivalve performance will change over time. As predicting chlorophyll a in climate change modeling is inherently difficult, food availability was held ad libitum for the entire year in this model, following the methods of Lavaud et al. (2017). By limiting the forcing variables in the model to temperature, the results of this study are restricted to the impact of temperature on bivalve physiology.
Coupled Climate-DEB Model
Predicted changes in SST coupled to bivalve physiology have shown to benefit to C. virginica over M. edulis in terms of growth, due to their differing thermal physiologies. For M. edulis a maximum SL increase of 8.7% was predicted for the future (2046–2050), compared to 16.0% for C. virginica (Figure 5). Growth rates of these ectothermic species are highly temperature dependent (Zippay and Helmuth, 2012; Feindel et al., 2013), and although growth rates are predicted to increase, thermal stress associated with ocean warming must be considered. The upper thermal limit of M. edulis used in this model was 23°C (Saraiva et al., 2011), but temperatures above 20°C are associated with increases rates of mortality (Newell and Moran, 1989; Mallet and Myrand, 1995), and significantly reduced growth rates (Gonzalez and Yevich, 1976). Specifically, in the southern Gulf of St Lawrence, mortality associated with summer heat stress has recently been observed (Myrand et al., 2000). Although mortality is not predicted by the model, an analysis of predicted temperature could be used as an indicator of mortality risk. For mussels, the number of consecutive days per year at temperatures above 22°C would increase from ~7 in the past (1986–1990) up to ~50 in the future (2046–2050) (Figure 2A). The potential for increased mortality rates at this temperature is highly relevant; in a recent laboratory experiment, mussels held at 22°C for a 30 day period experienced significantly higher mortality rates than a control group held at 16°C (Clements et al., 2018). This suggests significantly increased mortality rates for M. edulis in the future, compared to past conditions (Figure 2A). Note that, as discussed above, mortality risk would become even higher in aquaculture areas, that is, sheltered bays which cannot be captured with the spatial resolution of the climate model. In this way, these predictions are a conservative estimate of mortality risk in highly coastal, sheltered bays.
Contrastingly, C. virginica has the potential to grow to market size at an expedited rate under future scenarios (Figure 5), with no adverse effects from high temperatures due to their high thermal tolerance. Although there was no significant decrease observed in the number of consecutive days below temperatures relevant to the lower thermal threshold of C. virginica (Figure 2B), increased growth rates are still likely due to warming summer temperatures. C. virginica growth becomes observable in water temperatures around 9°C (Shumway, 1996), a temperature threshold which is likely to be met earlier in the year in the future. Food availability (recorded as timing of phytoplankton blooms) is currently not temporally synced to the seasonal initiation of C. virginica growth. In waters contained within the model domain, spring phytoplankton blooms occur in water temperatures between 4 and 9°C, creating a mismatch between the timing of peak food availability and the seasonal initiation of C. virginica growth (Pernet et al., 2007; Comeau et al., 2008; Feindel et al., 2013). As the temporal match between food availability (phytoplankton blooms) and temperatures suitable for growth could become more closely linked under climate change scenarios, the production potential for C. virginica would be further benefitted.
In addition to the effects on growth and mortality, SST can also affect phenology, in terms of both reproductive timing and distribution (Thackeray et al., 2010; Feindel et al., 2013). Warming ocean temperatures and the expanded northward distribution of the oyster Crassostrea gigas has been extensively studied in European waters (Laugen et al., 2015; Shelmerdine et al., 2017). Spawn dates were estimated in this study using a combination threshold of both temperature and GSI, a common method to trigger spawning in DEB modeling (e.g., Bourlès et al., 2009). The application of this threshold suggested that in the future M. edulis will spawn earlier in the year (Figure 6B), and there is potential for oysters to demonstrate a similar trend, however they did not spawn in the first year (Figure 5). For all areas in which spawning occurs, the dates fall within the currently observed spawning times for the Gulf region (between May and August) (DFO, 2003b). GSI calculations (Figure 5) indicate that for both species, proportionally more energy will be allocated to reproduction in the future, although a greater increase was observed in C. virginica.
Limitations
The predictions of these DEB models are forced by temperature, the most significant variable for bivalve ecophysiology (Filgueira et al., 2016); however, the effects of other drivers such as ocean acidification, food availability and ecosystem dynamics should not be ignored (Feindel et al., 2013). Ocean acidification, although difficult to predict, has been incorporated into DEB models (e.g., Klok et al., 2014). The waters included within this model have experienced changes in their pH, showing an average pH decline of 0.1–0.2 units since the 1930s (Stewart and White, 2001). Gledhill et al. (2015) have also suggested that the coastal waters of NS have a reduced buffering capacity due to significant freshwater inputs. Acidification has the potential to negatively affect fertilization, larval settlements, and spat shell formation (Curren and Azetsu-Scott, 2013; Gurney-Smith, 2015). Negative impacts have been reported on M. edulis larvae in terms of slow growth rates and shell deformities (Gazeau et al., 2010, 2013), and also for recruitment (Brown et al., 2016). For C. virginica and M. edulis in North America, negative impacts to shell calcification are expected under marine pH conditions predicted for 2050 and 2100, respectively (Gazeau et al., 2007; Whitman-Miller et al., 2009). Despite this, most studies show few impacts of elevated CO2 on growth or mortality rates of adult bivalves (Keppel et al., 2015; Clements et al., 2018). The impacts of acidification on larval mortality may be particularly important for the aquaculture industry in NS and PEI, where spat are primarily harvested from the wild and therefore rely on unbuffered water (Feindel et al., 2013). However, rearing spat in hatcheries may help to mitigate the impact of acidification on bivalve aquaculture (Clements and Chopin, 2016).
Culture conditions, where bivalves are grown at high densities are also not represented in these models, which are individual based. Local effects caused by high culture density, such as competition, can have significant impacts on bivalve performance (Cubillo et al., 2012). The primary culture method of bivalves in NS and PEI is in-shore, which increases the risk of thermal stress, and requires cultivation at high densities due to limiting space. Off-shore aquaculture avoids high summer temperatures experienced in coastal bays, potentially reducing thermal stress on M. edulis (Myrand et al., 2000; DFO, 2017). Additionally, less competition for space could permit cultivating bivalves at lower stocking densities. Although off-shore aquaculture lacks the physical protection of sheltered bays, by lowering long-lines several meters into the water column, abrasive wave action can be avoided, and more stable temperatures can be achieved (DFO, 2017; Klinger et al., 2017). Preliminary results from M. edulis grown off-shore in Newfoundland indicated that off-shore growth rates were comparable to in-shore growth, and that spawning occurred less often, but was more predictable (DFO, 2017). Deeper cooler waters have the potential to avoid thermal stress for M. edulis under climate change conditions, compared to sheltered inlets (Figure 2A). Compounding stressors such as low food availability, reproduction, and summer heatwaves can act to simultaneously increase mortality rates. For example, previous summer mortalities of M. edulis has been explained by the combined effects of high water temperatures, low food availability, and high reproductive output (Tremblay et al., 1998; Myrand et al., 2000). For C. virginica, as mentioned, it should also be considered that this model was calibrated in the Gulf of Mexico (Lavaud et al., 2017), with a differing thermal regime to the one in the model domain. Not accounting for possible differences in the ecophysiology of C. virginica latitudinally may introduce error into the model.
Conclusions
Results of this study, although subject to the uncertainties previously discussed, indicate that SST in coastal waters will undergo differential rates of warming both spatially and seasonally. Due to the different thermal physiologies of C. virginica and M. edulis, these predicted changes in SST will create species specific risks and opportunities in terms of growth and phenology. Summer heat stress may pose an increased threat to M. edulis, due to their lower thermal tolerance. This can impact performance in terms of growth, but also potentially increase the risk of mortality. C. virginica, with a higher thermal tolerance, is unlikely to experience negative impacts to growth rates, but instead is likely to show an increase in performance. Additionally, as SST warms there may be an increased temporal match between food availability and the growing period of C. virginica, which may in turn impact growth and phenology. When planning for the future of cultivating bivalves in NS and PEI, these results provide information on which areas will become stressful for M. edulis, and concomitantly where opportunities will arise to cultivate C. virginica more effectively. The continued success of this industry is dependent upon the careful selection of species and farming sites. To avoid increased temperature related mortality of M. edulis from thermal stress, off-shore aquaculture could be considered as a cultivation method. Furthermore, C. virginica could be opportunistically grown in areas where warming SST could promote increased growth rates compared to colder areas. To build upon these results, and reduce uncertainties, future studies should incorporate environmental variables such as seasonal food availability and ocean acidification. Factors such as these can be explored under climate change scenarios, and further integrated into bioenergetic models. bioenergetic models.
Author Contributions
Climate model data was provided by JC, and DEB modeling expertise provided by RF. All authors contributed to the improvement of the manuscript.
Conflict of Interest Statement
The authors declare that the research was conducted in the absence of any commercial or financial relationships that could be construed as a potential conflict of interest.
Funding
This work was prepared as part of a graduate research project at Dalhousie University, Canada. Funding was provided through a graduate NSERC scholarship to LS, and an NSERC discovery grant to RF.
References
Agirbas, E., Martinez-Vicente, V., Brewin, R. J. W., Racault, M.-F., Airs, R. L., and Llewellyn, C. A. (2015). Temporal changes in total and size-fractioned chlorophyll-a in surface waters of three provinces in the Atlantic Ocean (September to November) between 2003 and 2010. J. Mar. Syst. 150, 56–65. doi: 10.1016/j.jmarsys.2015.05.008
Bacher, C., Grant, J., Hawkins, A., Fang, J., Zhu, P., and Duarte, P. (2003). Modeling the effect of food depletion on scallop growth in Sungo Bay (China). Aquat. Living Resour. 16, 10–24. doi: 10.1016/S0990-7440(03)00003-2
Bayne, B. L., Widdows, J., and Thompson, R. J. (1976). “Physiological integrations,” in Marine Mussels, ed B. L Bayne (Cambridge: Cambridge University Press), 261–299.
Bernard, I., de Kermoysan, G., and Pouvreau, S. (2011). Effect of phytoplankton and temperature on the reproduction of the Pacific oyster Crassostrea gigas: investigation through DEB theory. J. Sea. Res. 6, 349–360. doi: 10.1016/j.seares.2011.07.009
Bouillon, S., Morales Maqueda, M. A., Legat, V., and Fichefet, T. (2009). An elastic-viscous-plastic sea ice model formulated on Arakawa B and C grids. Ocean Model. 27, 174–184. doi: 10.1016/j.ocemod.2009.01.004
Bourlès, Y., Alunno-Bruscia, M., Pouvreau, S., Tollu, G., Leguay, D., Arnaud, C., et al. (2009). Modelling growth and reproduction of the Pacific oyster Crassostrea gigas: advances in the oyster-DEB model through application to a coastal pond. J. Sea. Res. 62, 62–71. doi: 10.1016/j.seares.2009.03.002
Brickman, D., and Drozdowski, A. (2012). Development and Validation of a Regional Shelf Model for Maritime Canada Based on the NEMO-OPA Circulation Model. Canadian Technical Report of Hydrography and Ocean Sciences. Available online at: http://www.dfo-mpo.gc.ca/Library/347377.pdf
Brown, N. E., Therriault, T. W., and Harley, C. D. (2016). Field-based experimental acidification alters fouling community structure and reduces diversity. J. Anim. Ecol. 85, 1328–1339. doi: 10.1111/1365-2656.12557
Buckley, L. B., and Huey, R. B. (2016). Temperature extremes: geographic patterns, recent changes, and implications for organismal vulnerabilities. Glob. Change Biol. 22, 3829–3842. doi: 10.1111/gcb.13313
Bush, E. J., Loder, J. W., James, T. S., Mortsch, L. D., and Cohen, S. J. (2014). “An overview of Canada's changing climate,” in Canada in a Changing Climate: Sector Perspectives on Impacts and Adaptation, eds J. Warren and D. S. Lemmen (Ottawa, ON: Government of Canada), 23–64.
Choi, K. (1992). A Study on the Reproduction of Oysters, Crassostrea virginica (Pelecypoda; Mollusca) in the Galveston Bay area, Texas, Using Immunological Techniques. Dissertation, Texas, Texas University.
Clausen, I., and Riisgård, H. (1996). Growth, filtration and respiration in the mussel Mytilus edulis: no evidence for physiological regulation of the filter-pump to nutritional needs. Mar. Ecol. Prog. Ser. 141, 37–45. doi: 10.3354/meps141037
Clements, J. C., and Chopin, T. (2016). Ocean acidification and marine aquaculture in North America: potential impacts and mitigation strategies. Rev. Aquacult. 9, 326–341. doi: 10.1111/raq.12140
Clements, J. C., Hicks, C., Tremblay, R., and Comeau, L. (2018). Elevated seawater temperature not pCO2 negatively affects post-spawning adult mussels (Mytilus edulis) under food limitation. Conserv. Physiol. 6:cox078. doi: 10.1093/conphys/cox078
Coen, L. D., Brumbaugh, R. D., Bushek, D., Grizzle, R., Luckenbach, M. W., Posey, M. H., et al. (2007). Ecosystem services related to oyster restoration. Mar. Ecol. Prog. Ser. 341, 303–307. doi: 10.3354/meps341303
Comeau, L. A., Pernet, F., Tremblay, R., Bates, S. S., and LeBlanc, A. (2008). Comparison of eastern oyster (Crassostrea virginica) and blue mussel (Mytilus edulis) filtration rates at low temperatures. Can. Tech. Rep. Fish. Aquat. Sci. 2810, 1–17. Available online at: http://epe.lac-bac.gc.ca/100/200/301/dfo-mpo/cdn_technical_report/comparison_eastern_oyster/Fs97-6-2810E.pdf
Cranford, P. J., Dowd, M., Grant, J., Hargrave, B., and McGladdery, S. (2003). Ecosystem level effects of marine bivalve aquaculture. Sci. Rev. Potential Environ. Effects Aquacult. Aqua. Ecosyst. 1, 51–95.
Cranford, P. J., Hargrave, B. T., and Doucette, L. I. (2009). Benthic organic enrichment from suspended mussel (Mytilus edulis) culture in Prince Edward Island, Canada. Aquaculture 292, 189–196. doi: 10.1016/j.aquaculture.2009.04.039
Cranford, P., Strain, P., Dowd, M., Hargrave, B., Grant, J., and Archambault, M. (2007). Influence of mussel aquaculture on nitrogen dynamics in a nutrient enriched coastal embayment. Mar. Ecol. Prog. Ser. 347, 61–78. doi: 10.3354/meps06997
Cubillo, A. M., Peteiro, L. G., Fernández-Reiriz, M. J., and Labarta, U. (2012). Influence of stocking density on growth of mussels (Mytilus galloprovincialis) in suspended culture. Aquaculture 342–343, 103–111. doi: 10.1016/j.aquaculture.2012.02.017
Curren, K., and Azetsu-Scott, K. (2013). “Ocean acidification,” in State of the Scotian Shelf Report, Fisheries and Oceans Canada, Dartmouth, Nova Scotia, Canadian Technical Report on Fisheries and Aquatic Sciences, eds M. MacLean, H. Breeze, J. Walmsley, and J. Corkum (Nova Scotia: Dartmouth), 164–182.
Dame, R. F., and Prins, T. C. (1998). Bivalve carrying capacity in coastal ecosystems. Aquat. Ecol. 31, 409–442. doi: 10.1023/A:1009997011583
DFO (Department of Fisheries and Oceans Canada) (2003b). Profile of the Blue Mussel (Mytilus edulis) Gulf Region. Moncton, NB: Policy and Economics Branch.
DFO (Department of Fisheries, and Oceans Canada). (2003a). Profile of the American oyster (Crassostrea virginica) Gulf region. Moncton, NB: Policy and Economics Branch.
DFO (Department of Fisheries Oceans Canada) (2017). A Study of the Reproductive Patterns of the Blue Mussel, Mytilus edulis Grown in Deep and Shallow Water Sites in Notre Dame Bay, Newfoundland and Labrador. Aquaculture Collaborative Research and Development Program. Available online at: http://www.dfo-mpo.gc.ca/aquaculture/rp-pr/acrdp-pcrda/projects-projets/15-1-N-03-eng.html
Diederich, S., Nehls, G., van Beusekom, J. E. E., and Reise, K. (2005). Introduced Pacific oysters (Crassostrea gigas) in the northern Wadden Sea: invasion accelerated by warm summers? Helgoland Mar. Res. 59, 97–106. doi: 10.1007/s10152-004-0195-1
Dittman, D. E., Ford, S. E., and Haskin, H. H. (1998). Growth patterns in oysters, Crassostrea virginica, from different estuaries. Mar. Biol. 132, 461–469. doi: 10.1007/s002270050412
Elliott, A. J., Thackeray, S. J., Huntingford, C., and Jones, R. G. (2005). Combining a regional climate model with a phytoplankton community model to predict future changes in phytoplankton in lakes. Freshwater Biol. 50, 1404–1411. doi: 10.1111/j.1365-2427.2005.01409.x
FAO (Food and Agricultural Organization of the United Nations) (2016). The State of World Fisheries and Aquaculture 2016: Contributing to Food Security and Nutrition for All. Rome.
Feindel, N., Cooper, I., Tripple, E., and Blair, T. (2013). “Climate change and marine aquaculture in Atlantic Canada and Quebec,” in Climate Change Impacts, Vulnerabilities and Opportunities Analysis of the Marine Atlantic Basin, eds N. L. Shackell, B. J. W. Greenan, P. Pepin, D. Chabot, and A. Warburton (Canadian Technical Report of Fisheries and Aquatic Sciences), 195–240.
Fichefet, T., and Morales Maqueda, M. A. (1997). Sensitivity of a global sea ice model to the treatment of ice thermodynamics and dynamics. J. Geophys. Res. 102, 609–646. doi: 10.1029/97JC00480
Filgueira, R., Brown, M. S., Comeau, L. A., and Grant, J. (2015). Predicting the timing of the pediveliger stage of Mytilus edulis based on ocean temperature. J. Mollus. Stud. 81, 269–273. doi: 10.1093/mollus/eyu093
Filgueira, R., Guyondet, T., Comeau, L. A., and Grant, J. (2014). A fully-spatial ecosystem-DEB model of oyster (Crassostrea virginica) carrying capacity in the Richibucto Estuary, Eastern Canada. J. Mar. Syst. 136, 42–54. doi: 10.1016/j.jmarsys.2014.03.015
Filgueira, R., Guyondet, T., Comeau, L. A., and Tremblay, R. (2016). Bivalve aquaculture-environment interactions in the context of climate change. Glob. Change Biol. 22, 3901–3913. doi: 10.1111/gcb.13346
Forsberg, P. L., Lumborg, U., Bundgaard, K., and Ernstsen, V. B. (2017). Impact of mussel bioengineering on fine-grained sediment dynamics in a coastal lagoon: a numerical modelling investigation. J. Mar. Syst. 176, 1–12. doi: 10.1016/j.jmarsys.2017.07.008
Freeman, K. R., and Dickie, L. M. (1979). Growth and mortality of the blue mussel (Mytilus edulis) in relation to environmental indexing. J. Fish Res. Board Can. 26, 1238–1249. doi: 10.1139/f79-178
Gallardi, D. (2014). Effects of bivalve aquaculture on the environment and their possible mitigation: a review. Fish. Aquacult. J. 5:105. doi: 10.4172/2150-3508.1000105
Gazeau, F., Gattuso, J.-P., Dawber, C., Pronker, A. E., Peene, F., Peene, J., et al. (2010). Effect of ocean acidification on the early life stages of the blue mussel Mytilus edulis. Biogeosciences 7, 2051–2060. doi: 10.5194/bg-7-2051-2010
Gazeau, F., Parker, L. M., Comeau, S., Gattuso, J.-P., O'Connor, W. A., Martin, S., et al. (2013). Impacts of ocean acidification on marine shelled molluscs. Mar. Biol. 160, 2207–2245. doi: 10.1007/s00227-013-2219-3
Gazeau, F., Quibler, C., Jansen, J. M., Gattuso, J. P., Middelburg, J. J., and Heip, C. H. R. (2007). Impact of elevated CO2 on shellfish calcification. Geophys. Res. Let. 34:L07603. doi: 10.1029/2006GL028554
Gledhill, D., White, M., Salisbury, J., Thomas, H., Misna, I., et al. (2015). Ocean and coastal acidification off New England and Nova Scotia. Oceanography 25, 182–197. doi: 10.5670/oceanog.2015.41
Godfray, H. C., Beddington, J. R., Crute, I. R., Haddad, L., Lawrence, D., Muir, J. F., et al. (2010). Food security: the challenge of feeding 9 billion people. Science 327, 812–818. doi: 10.1126/science.1185383
Gonzalez, J. G., and Yevich, O. (1976). Responses of an estuarine population of the blue mussel Mytilus edulis to heated water from a steam generating plant. Mar. Biol. 34, 177–189. doi: 10.1007/BF00390760
Gurney-Smith, H. J. (2015). “The changing oceans: causes, effects, and mitigation for shellfish in acidifying times,” in Aquaculture Canada 2014: Proceedings of Contributed Papers; Bulletin of the Contributed Papers: Bulletin of the Aquaculture Association of Canada 2015 (Saint Andrews, NB), 45–58.
Gutiérrez, J. L., Jones, C. G., Strayer, D. L., and Iribarne, O. O. (2003). Mollusks as ecosystem engineers: the role of shell production in aquatic habitats. Oikos 101, 79–90. doi: 10.1034/j.1600-0706.2003.12322.x
Guyondet, T., Comeau, L. A., Bacher, C., Grant, J., Rosland, R., Sonier, R., et al. (2015). Climate change influences carrying capacity in a coastal embayment dedicated to shellfish aquaculture. Estuar. Coast. 38, 1593–1618. doi: 10.1007/s12237-014-9899-x
Han, D., Chen, Y., Zhang, C., Ren, Y., Xue, Y., and Wan, R. (2017). Evaluating impacts of intensive shellfish aquaculture on a semi-closed marine ecosystem. Ecol. Model. 359, 193–200. doi: 10.1016/j.ecolmodel.2017.05.024
Hawkins, E., and Sutton, R. (2009). The potential to narrow uncertainty in regional climate predictions. B. Am.Meteorol. Soc. 90, 1095–1107. doi: 10.1175/2009BAMS2607.1
IPCC (Intergovernmental Panel on Climate Change) (2013). “Summary for policymakers,” in Climate Change 2013: The Physical Science Basis (Contribution of Working Group I to the Fifth Assessment Report of the Intergovernmental Panel on Climate Change), eds T. F. Stocker, D. Qin, G.-K. Plattner, M. Tignor, S. K. Allen, J. Boschung, A. Nauels, et al. (Cambridge; New York, NY: Cambridge University Press), 3–29.
Jones, S. J., Lima, F. P., and Wethey, D. S. (2010). Rising environmental temperatures and biogeography: poleward range contraction of the blue mussel, Mytilus edulis L., in the western Atlantic: Mytilus edulis poleward range contraction. J. Biogeog. 37, 2243–2259. doi: 10.1111/j.1365-2699.2010.02386.x
Jones, S. J., Mieszkowska, N., and Wethey, D. S. (2009). Linking thermal tolerances and biogeography: Mytilus edulis (L.) at its southern limit on the East coast of the United States. Biol. Bull. 217, 73–85. doi: 10.1086/BBLv217n1p73
Keppel, E. A., Scrosati, R. A., and Courtenay, S. C. (2015). Interactive effects of ocean acidification and warming on subtidal mussels and sea stars from Atlantic Canada. Mar. Biol. Res.11, 337–348. doi: 10.1080/17451000.2014.932914
Kittner, C., and Riisgård, H. (2005). Effect of temperature on filtration rate in the mussel Mytilus edulis: no evidence for temperature compensation. Mar. Ecol. Prog. Ser. 305, 147–152. doi: 10.3354/meps305147
Klinger, D. H., Levin, S. A., and Watson, J. R. (2017). The growth of finfish in global open-ocean aquaculture under climate change. P. Roy. Soc. B Biol Sci. 284:20170834. doi: 10.1098/rspb.2017.0834
Klok, C., Wijsman, J. W. M., Kaag, K., and Foekema, E. (2014). Effects of CO2 enrichment on cockle shell growth interpreted with a dynamic energy budget model. J. Sea. Res. 94, 111–116. doi: 10.1016/j.seares.2014.01.011
Kluger, L. C., Filgueira, R., and Wolff, M. (2017). Integrating the concept of resilience into an ecosystem approach to bivalve aquaculture management. Ecosystems 20, 1–19. doi: 10.1007/s10021-017-0118-z
Kooijman, S. (1986). Energy budgets can explain body size relations. J. Theor. Biol. 121, 269–282. doi: 10.1016/S0022-5193(86)80107-2
Kooijman, S. A. L. M. (2010). Dynamic Energy Budget Theory for Metabolic Organization, 3rd Edn. Cambridge: Cambridge University Press.
Laugen, A. T., Hollander, J., Obst, M., and Strand, A. A. (2015). The Pacific Oyster (Crassostrea gigas) Invasion in Scandinavian Coastal Waters: Impact on Local Ecosystem Services. Available online at: https://www.degruyter.com/downloadpdf/books/9783110438666/9783110438666-015/9783110438666-015.pdf
Lauzon-Guay, J. S. (2001). Dynamics of Cultivated Populations of Blue Mussels (Mytilus edulis) in Prince Edward Island, Canada. Dissertation, Montréal Université du Québec. 137.
Lauzon-Guay, J. S., Barbeau, M. A., Watmough, J., and Hamilton, D. J. (2006). Model for growth and survival of mussels Mytilus edulis reared in Prince Edward Island, Canada. Mar. Ecol. Prog. Ser. 323, 171–183. doi: 10.3354/meps323171
Lavaud, R., La Peyre, M. K., Casas, S. M., Bacher, C., and La Peyre, J. F. (2017). Integrating the effects of salinity on the physiology of the eastern oyster, Crassostrea virginica, in the northern Gulf of Mexico through a dynamic energy budget model. Ecol. Mod. 363, 221–233. doi: 10.1016/j.ecolmodel.2017.09.003
Lesser, M. P., Bailey, M. A., Merselis, D. G., and Morrison, J. R. (2010). Physiological response of the blue mussel Mytilus edulis to differences in food and temperature in the Gulf of Maine. Comp. Biochem. Physiol. A. 156, 541–551. doi: 10.1016/j.cbpa.2010.04.012
Long, Z., Perrie, W., Chassé, J., Brickman, D., Guo, L., Drozdowski, A., et al. (2016). Impacts of climate change in the Gulf of St. Lawrence. Atmosphere-Ocean. 54, 337–351. doi: 10.1080/07055900.2015.1029869
Madec, G., Delecluse, P., Imbard, M., and Lévy, C. (1998). OPA 8.1 Ocean General Circulation Model Reference Manual, No. 11. Note du Pôle de Modélisation, Institut Pierre-Simon Laplace (IPSL), France.
Malham, S. K., Cotter, E., O'Keeffe, S., Lynch, S., Culloty, S. C., King, J. W., et al. (2009). Summer mortality of the Pacific oyster, Crassostrea gigas, in the Irish Sea: the influence of temperature and nutrients on health and survival. Aquaculture. 287, 128–138. doi: 10.1016/j.aquaculture.2008.10.006
Mallet, A. L., and Carver, C. E. (1995). Comparative growth and survival patterns of Mytilus trossulus and Mytilus edulis in Atlantic Canada. Can. J. Fish. Aquat. Sci. 52, 1873–1880. doi: 10.1139/f95-780
Mallet, A., and Myrand, B. (1995). “The culture of the blue mussel in Atlantic Canada,” in Cold-Water Aquaculture in Atlantic Canada, 2nd Edn., ed A. D. Borden (Moncton, NB: Donald J. Savoie Institute), 35–81.
Menge, B. (1992). Community regulation: under what conditions are bottom-up factors important on rocky shores? Ecology 73, 755–765. doi: 10.2307/1940155
Montalto, V., Helmuth, B., Ruti, P. M., Dell'Aquila, A., Rinaldi, A., and Sarà, G. (2016). A mechanistic approach reveals non-linear effects of climate warming on mussels throughout the Mediterranean sea. Clim. Change. 139, 293–306. doi: 10.1007/s10584-016-1780-4
Murray, M. C., and Hare, M. P. (2006). A genomic scan for divergent selection in a secondary contact zone between Atlantic and Gulf of Mexico oysters, Crassostrea virginica: genomic differentiation in Oysters. Molec. Ecol. 15, 4229–4242. doi: 10.1111/j.1365-294X.2006.03060.x
Myrand, B., Guderley, H., and Himmelman, J. H. (2000). Reproduction and summer mortality of blue mussels Mytilus edulis in the Magdalen Islands, southern Gulf of St. Lawrence. Mar. Ecol. Prog. Ser. 197, 193–207. doi: 10.3354/meps197193
Newell, R. I. (2004). Ecosystem influences of natural and cultivated populations of suspension-feeding bivalve molluscs: a review. J. Shellfish Res. 23, 51–62.
Newell, R. I., and Moran, D. (1989). Species Profiles: life histories and environmental requirements of coastal fishes and invertebrates (North and Mid-Atlantic) – blue mussel U.S. Fish. Wildl. Serv. Biol. Rep. 82, 11–102.
Pachauri, R. K., and Mayer, L. Intergovernmental Panel on Climate Change (eds.). (2015). Climate change 2014: Synthesis Report. Geneva: Intergovernmental Panel on Climate Change.
Pachauri, R. K., Allen, M. R., Barros, V. R., Broome, J., Cramer, W., Christ, R., et al. (2014). Climate Change 2014: Synthesis Report. Contribution of Working Groups I, II and III to the Fifth Assessment Report of the Intergovernmental Panel on Climate Change. Bremerhaven: Alfred-Wegener-Inst.
Pernet, F., Tremblay, R., Comeau, L., and Guderley, H. (2007). Temperature adaptation in two bivalve species from different thermal habitats: energetics and remodelling of membrane lipids. J. Exp. Biol. 210, 2999–3014. doi: 10.1242/jeb.006007
Petersen, J. K., Saurel, C., Nielsen, P., and Timmermann, K. (2016). The use of shellfish for eutrophication control. Aquac. Int. 24, 857–878. doi: 10.1007/s10499-015-9953-0
Philippart, C. J., van Aken, H. M., Beukema, J. J., Bos, O. G., Cadée, G. C., and Dekker, R. (2003). Climate-related changes in recruitment of the bivalve Macoma balthica. Limnol. Oceanog. 48, 2171–2185. doi: 10.4319/lo.2003.48.6.2171
Picoche, C., Le Gendre, R., Flye-Sainte-Marie, J., Françoise, S., Maheux, F., Simon, B., et al. (2014). Towards the determination of Mytilus edulis food preferences using the dynamic energy budget (DEB) theory. PLoS ONE 9:e109796. doi: 10.1371/journal.pone.0109796
Pouvreau, S., Bourles, Y., Lefebvre, S., Gangnery, A., and Alunno-Bruscia, M. (2006). Application of a dynamic energy budget model to the Pacific oyster, Crassostrea gigas, reared under various environmental conditions. J. Sea. Res. 56, 156–167. doi: 10.1016/j.seares.2006.03.007
Rice, M. A. (2008). Environmental effects of shellfish aquaculture in the Northeast. University of Maryland, Maryland. NRAC Publication Fact Sheet 105, 1–6. Available online at: https://agresearch.umd.edu/sites/agresearch.umd.edu/files/_images/uploaded/105-EnvironmentalEffects.pdf
Riisgård, H. U., Lundgreen, K., and Larsen, P. S. (2012). Field data and growth model for mussels Mytilus edulis in Danish waters. Mar. Biol. Res. 8, 683–700. doi: 10.1080/17451000.2012.678857
Rinde, E., Hjermann, D. Ø., and Staalstrøm, A. (2016). Larvae Drift Simulations of the Pacific Oyster in Skagerrak–Influence of Climate Change on Larvae Development, Survival and Dispersal. Available online at: https://brage.bibsys.no/xmlui/handle/11250/2386876
Rodrigues, L. C., Bergh, J. C. J. M. V. D., Massa, F., Theodorou, J. A., Ziveri, P., and Gazeau, F. (2015). Sensitivity of mediterranean bivalve mollusc aquaculture to climate change, ocean acidification, and other environmental pressures: findings from a producer survey. J. Shellfish Res. 34, 1161–1176. doi: 10.2983/035.034.0341
Rodríguez-Rodríguez, G., and Roberto, B. R. (2017). Market driven management of climate change impacts in the spanish mussel sector. Mar. Policy 83, 230–235. doi: 10.1016/j.marpol.2017.06.014
Rosland, R., Strand, Ø., Alunno-Bruscia, M., Bacher, C., and Strohmeier, T. (2009). Applying dynamic energy budget (DEB) theory to simulate growth and bio-energetics of blue mussels under low seston conditions. J. Sea. Res. 62, 49–61. doi: 10.1016/j.seares.2009.02.007
Saba, V. S., Griffies, S. M., Anderson, W. G., Winton, M., Alexander, M. A., Delworth, T. L., et al. (2016). Enhanced warming of the Northwest Atlantic Ocean under climate change: Northwest Atlantic enhanced warming. J. Geophys. Res. Oceans 121, 118–132. doi: 10.1002/2015JC011346
Sarà, G., Kearney, M., and Helmuth, B. (2011). Combining heat-transfer and energy budget models to predict thermal stress in Mediterranean intertidal mussels. Chem. Ecol. 27, 135–145. doi: 10.1080/02757540.2011.552227
Sarà, G., Palmeri, V., Rinaldi, A., Montalto, V., and Helmuth, B. (2013). Predicting biological invasions in marine habitats through eco-physiological mechanistic models: a case study with the bivalve Brachidontes pharaonis. Divers. Distrib. 19, 1235–1247. doi: 10.1111/ddi.12074
Saraiva, S., der Meer, J., van Kooijman, S. A. L. M., and Sousa, T. (2011). DEB parameters estimation for Mytilus edulis. J. Sea. Res. 66, 289–296. doi: 10.1016/j.seares.2011.06.002
Shelmerdine, R., Mouat, B., and Shucksmith, R. (2017). The most northerly record of feral Pacific oyster Crassostrea gigas (Thunberg, 1793) in the British Isles. Bioinvasions Rec. 6, 57–60. doi: 10.3391/bir.2017.6.1.09
Shumway, S. A. (1996). “Natural environmental factors,” in The Eastern Oyster Crassostrea virginica, eds V. S. Kennedy, C. R. Newell and A. F. Eble (College Park, MD: Maryland Sea Grant College), 467–513.
Shumway, S. E., Davis, C., Downey, R., Karney, R., Kraeuter, J., Parsons, J., et al. (2003). Shellfish aquaculture–in praise of sustainable economies and environments. World Aquacult. 34, 8–10. Available online at: http://www.edc.uri.edu/temp/ci/ciip/FallClass/Docs_2008/Shumway_etal2003.pdf
Skogen, M. D., Eilola, K., Hansen, J. L. S., Meier, H. E. M., Molchanov, M. S., and Ryabchenko, V. A. (2014). Eutrophication status of the North Sea, Skagerrak, Kattegat, and the Baltic Sea in present and future climates: a model study. J. Mar. Sys. 132, 174–184 doi: 10.1016/j.jmarsys.2014.02.004
Smith, R. A. (1980). The theoretical basis for estimating phytoplankton production and specific growth rate from chlorophyll, light and temperature data. Ecol. Model. 10, 243–264. doi: 10.1016/0304-3800(80)90062-9
Sonier, R., LeBlanc, K., Hardy, M., Ouellette, M., Comeau, L. A., and Landry, T. (2011). Shellfish Monitoring Network in Atlantic Canada 1996–2001: Temperature, Growth, Condition and Survival. Canadian Data Report of Fisheries and Aquatic Sciences 1234.
Sorte, C. J. B., Davidson, V. E., Franklin, M. C., Benes, K. M., Doellman, M. M., Etter, R. J., et al. (2017). Long-term declines in an intertidal foundation species parallel shifts in community composition. Global Change. Biol. 23, 341–352. doi: 10.1111/gcb.13425
Stewart, P. L., and White, L. (2001). A Review of contaminants on the Scotian Shelf and in adjacent coastal waters: 1970 to 1995. Canadian Technical Report of Fisheries and Aquatic Sciences 2351.
Stobart, B., Mayfield, S., Mundy, C., Hobday, A. J., and Hartog, J. R. (2016). Comparison of in situ and satellite sea surface-temperature data from South Australia and Tasmania: how reliable are satellite data as a proxy for coastal temperatures in temperate southern Australia? Mar. Freshwater Res. 67:612. doi: 10.1071/MF14340
Strohmeier, T., Aure, J., Duinker, A., Castberg, T., Svardal, A., and Strand, Ø. (2005). Flow reduction, seston depletion, meat content and distribution of diarrhetic shellfish toxins in a long-line blue mussel farm. J. Shellfish Res. 24, 15–24. doi: 10.2983/0730-8000(2005)24[15:FRSDMC]2.0.CO;2
Thackeray, S. J., Sparks, T. H., Frederiksen, M., Burthe, S., Bacon, P. J., Bell, J. R., et al. (2010). Trophic level asynchrony in rates of phenological change for marine, freshwater, and terrestrial environments. Global Change Biol. 16, 3304–3313. doi: 10.1111/j.1365-2486.2010.02165.x
Thomas, Y., Mazurié, J., Alunno-Bruscia, M., Bacher, C., Bouget, J.-F., Gohin, F., et al. (2011). Modelling spatio-temporal variability of Mytilus edulis (L.) growth by forcing a dynamic energy budget model with satellite-derived environmental data. J. Sea. Res. 66, 308–317. doi: 10.1016/j.seares.2011.04.015
Thomas, Y., Pouvreau, S., Alunno-Bruscia, M., Barillé, L., Gohin, F., Bryère, P., et al. (2015). Global change and climate-driven invasion of the Pacific oyster (Crassostrea gigas) along European coasts: a bioenergetics modelling approach. J. Biogeography. 43, 568–579. doi: 10.1111/jbi.12665
Toupoint, N., Gilmore-Solomon, L., Bourque, F., Myrand, B., Pernet, F., Olivier, F., et al. (2012). Match/mismatch between the Mytilus edulis larval supply and seston quality: effect on recruitment. Ecology 93, 1922–1934. doi: 10.1890/11-1292.1
Tremblay, R., Myrand, B., Sevigny, J.-M., Blier, P., and Guderley, H. (1998). Bioenergetic and genetic parameters in relation to susceptibility of blue mussels, Mytilus edulis (L.) to summer mortality. J. Exp. Mar. Biol. Ecol. 221, 27–58. doi: 10.1016/S0022-0981(97)00114-7
Troost, T. A., Wijsman, J. W. M., Saraiva, S., and Freitas, V. (2010). Modelling shellfish growth with dynamic energy budget models: an application for cockles and mussels in the Oosterschelde (southwest Netherlands). Philos. T. R. Soc. B. 365, 3567–3577. doi: 10.1098/rstb.2010.0074
van der Veer, H. W., Cardoso, J. F. M. F., and van der Meer, J. (2006). The estimation of DEB parameters for various Northeast Atlantic bivalve species. J. Sea. Res. 56, 107–124. doi: 10.1016/j.seares.2006.03.005
Waite, L., Grant, J., and Davidson, J. (2005). Bay-scale spatial growth variation of mussels Mytilus edulis in suspended culture, Prince Edward Island, Canada. Mar. Ecol. Prog. Ser. 297, 157–167. doi: 10.3354/meps297157
Whitman-Miller, A., Reynolds, A. C., Sobrino, C., and Riedel, G. F. (2009). Shellfish face uncertain future in high CO2 world: influence of acidification on oyster larvae calcification and growth in estuaries. PLoS ONE 4:e5661. doi: 10.1371/journal.pone.0005661
Keywords: aquaculture, bivalves, climate change, bioenergetics, dynamic energy budget modeling
Citation: Steeves LE, Filgueira R, Guyondet T, Chassé J and Comeau L (2018) Past, Present, and Future: Performance of Two Bivalve Species Under Changing Environmental Conditions. Front. Mar. Sci. 5:184. doi: 10.3389/fmars.2018.00184
Received: 19 March 2018; Accepted: 07 May 2018;
Published: 25 May 2018.
Edited by:
Nesar Ahmed, University of Manitoba, CanadaReviewed by:
Nor Azman Kasan, Universiti Malaysia Terengganu, MalaysiaGabriel Cardenete, Universidad de Granada, Spain
Copyright © 2018 Steeves, Filgueira, Guyondet, Chassé and Comeau. This is an open-access article distributed under the terms of the Creative Commons Attribution License (CC BY). The use, distribution or reproduction in other forums is permitted, provided the original author(s) and the copyright owner are credited and that the original publication in this journal is cited, in accordance with accepted academic practice. No use, distribution or reproduction is permitted which does not comply with these terms.
*Correspondence: Laura E. Steeves, laura.steeves@dal.ca