- 1Gulf of Maine Research Institute, Portland, ME, United States
- 2MIT-WHOI Joint Program in Biological Oceanography, Woods Hole, MA, United States
The fishery for American lobster is currently the highest-valued commercial fishery in the United States, worth over US$620 million in dockside value in 2015. During a marine heat wave in 2012, the fishery was disrupted by the early warming of spring ocean temperatures and subsequent influx of lobster landings. This situation resulted in a price collapse, as the supply chain was not prepared for the early and abundant landings of lobsters. Motivated by this series of events, we have developed a forecast of when the Maine (USA) lobster fishery will shift into its high volume summer landings period. The forecast uses a regression approach to relate spring ocean temperatures derived from four NERACOOS buoys along the coast of Maine to the start day of the high landings period of the fishery. Tested against conditions in past years, the forecast is able to predict the start day to within 1 week of the actual start, and the forecast can be issued 3–4 months prior to the onset of the high-landings period, providing valuable lead-time for the fishery and its associated supply chain to prepare for the upcoming season. Forecast results are conveyed in a probabilistic manner and are updated weekly over a 6-week forecasting period so that users can assess the certainty and consistency of the forecast and factor the uncertainty into their use of the information in a given year. By focusing on the timing of events, this type of seasonal forecast provides climate-relevant information to users at time scales that are meaningful for operational decisions. As climate change alters seasonal phenology and reduces the reliability of past experience as a guide for future expectations, this type of forecast can enable fishing industry participants to better adjust to and prepare for operating in the context of climate change.
Introduction
Societies have long been structured around typical seasonal and interannual cycles. The ability to anticipate the types, timing, and magnitude of variability—even in an intuitive manner—has afforded the ability to plan activities in ways that are compatible with environmental conditions. For example, farming relies on timing activities such as tilling, planting, growth, and harvesting of crops to typical annual rainfall and temperature cycles. However, this tight link between human activities and seasonal cycles can also disrupt societies when large, abrupt events or unexpected changes occur. Climate change is now pushing environments beyond conditions that have come to be intuitively expected based on personal experiences. As such, shifts in the timing of seasonal events and magnitudes of extremes (Mora et al., 2013; Poloczanska et al., 2013; Thomas et al., 2017; Alexander et al., in press) can pose substantial challenges for and require adaptation of coupled ecological and social systems (Mills et al., 2013; Alexander et al., 2017).
While climate change will require new capacities to adapt to changing environmental conditions, societies are becoming more prepared and capable of confronting these challenges. Increases in observational and computing power are greatly expanding our ability to understand and predict changes in environmental conditions as well as their effects on ecosystem features and human activities. Indeed, forecasting—in a variety of forms and at multiple time scales—has become an essential part of modern life. The ability to accurately predict weather conditions days in advance has improved daily life, generating over US$30 billion in benefits to weather forecast users in the United States alone (Lazo et al., 2009). Seasonal climate forecasts support decision-making and help avoid major disruptions across many natural resources sectors, such as agriculture (Hansen et al., 2011; Ramírez-Rodrigues et al., 2016) and water resources (Hamlet et al., 2002; Kwon et al., 2009). In addition, long-term projections enable the consideration of environmental change over a range of decisions. For example, projecting the consequences of anthropogenic carbon dioxide emissions allows communities to plan for expected impacts of warming and sea level rise and to weigh the costs and benefits of different adaptation strategies (Shepard et al., 2012).
Forecasts have long been central to the operation and management of marine fisheries. The quota-setting process at the heart of fisheries management involves projecting the expected performance of the stock and the yield that can be obtained from it. Similarly, longer-term projections of the growth of stocks that are at low biomass levels are used to determine rebuilding plans and timelines. While environmental variability has been recognized as exerting a substantial influence on the recruitment and productivity of fish stocks (Vert-pre et al., 2013; Szuwalski et al., 2014), this information has not been widely incorporated into fish population models and projections. However, recent examples have demonstrated that population projections used to set catch quotas and rebuilding plans can be more reliable for some stocks if environmental factors, particularly temperature, are considered in projections (Jacobson and McClatchie, 2013; Hill et al., 2014; Pershing et al., 2015; Tommasi et al., 2017).
These types of stock projections establish the general harvest constraints under which fisheries are prosecuted, and within this context, fishing industry participants make a variety of shorter-term operational decisions. Fishermen decide on a day-to-day basis when and where to fish, which species to target given their suite of permits and gear, and how to manage their operational costs. Dealers face decisions about which species to purchase given storage options, transportation capacity, and supply chain demand. And processors determine the type, amount, and timing of products to produce as well as how to adjust factory operations for different production activities. Forecasting efforts that are directed at industry-based operating decisions on seasonal or shorter time scales are now emerging; for example, Hobday et al. (2016) showcase several seasonal forecasts that support operations in both wild harvest fisheries and aquaculture.
Our interest in seasonal forecasting to support fisheries decision-making was motivated by the experience of the Maine lobster fishery during a marine heat wave in 2012 (Mills et al., 2013). American lobster supports the most valuable commercial fishery in the United States at present (>US$620 million in 2015; National Marine Fisheries Service, 2016), and over 80% of its landings occur in the state of Maine. The Maine lobster fishery is highly seasonal, with low landings rates during the winter and spring, followed by an abrupt shift into a high landings period in early summer. The high landings period is driven by lobsters migrating into nearshore waters where they become accessible to the large small-boat fleet, becoming more active and entering traps, and molting into a harvestable size class. These three processes are all tightly related to water temperature (Cooper and Uzmann, 1971; Aiken, 1973; Aiken and Waddy, 1975; Ennis, 1984; Crossin et al., 1998). During 2012, sea surface temperatures (SST) on the northeast U. S. continental shelf were 1–3°C warmer than the 1982–2011 average, the highest documented in 150 years of measurements (Friedland, pers. comm.) and on par with the mean SST change that climate models project for the region by the end of the century (Mills et al., 2013). The heatwave led to spring temperatures warming 3 weeks ahead of the typical schedule, and lobster landings also rose sharply 3 weeks earlier than normal. The supply chain was not prepared for the rapid uptick and high volume of landings, which outstripped holding, transportation, and processing capacity as well as market demand (Mills et al., 2013). While record high landings volume was reported in 2012 (National Marine Fisheries Service, 2016), an ensuing price collapse left many fishermen struggling to break even.
The events of 2012 highlighted the potential usefulness of seasonal forecasts to support decision-making at multiple points in the supply chain. Prior to 2012, the entire production process for American lobster—from harvest to processing to tables—was based on a historically reliable and intuitively understood seasonal cycle. Advance notice that the high landings period would begin much earlier than usual may have enabled dealers to increase storage and transportation capacity, processors to prepare equipment and hire seasonal staff, and marketers to intensify efforts to expand markets for the product. Alternatively, a forecast of an early season could have enabled harvesters or managers to adopt strategies that better aligned supply with demand, with a goal of avoiding a price collapse. While the 2012 heat wave demonstrated weaknesses of relying on past experiences, the chain of events that occurred also showcased the tight and lagged coupling between water temperature and the Maine lobster fishery.
At the request of members of Maine's lobster fishery, we set out to develop a forecast system to provide advanced warning of unusual conditions in the seasonality of the Maine lobster fishery. The forecast focuses on the timing of when the fishery shifts into its high-landings summer period, and it is based on real-time temperature measurements provided by NERACOOS (Northeastern Regional Association of Coastal and Ocean Observing Systems), the regional contribution to the U.S. Integrated Ocean Observing System. Our results demonstrate the potential for using a simple process to forecast features of the human system (i.e., fishery landings) from temperature months in advance. Similar forecasts that are targeted to inform specific decisions faced by users will become increasingly valuable as climate change inhibits reliance on familiar past patterns and as multiple actors in a range of fishing industries seek to adapt to unfamiliar extremes and new levels of variability that are associated with climate change.
Methods
The goal of our analysis is to assess the potential to predict the phenology of the Maine lobster fishery using temperature data. Our approach involves characterizing seasonal patterns of lobster landings and then developing statistical models to explain changes in landing rates as a function of temperature. Results are conveyed through a probabilistic forecast of when the rate of landings is expected to increase. This forecast is updated weekly from early March through mid-April and is served via a public website to interested users.
Temperature Data
Temperature data are accessed from buoys B, E, F, and I of the Northeastern Regional Association of Coastal and Ocean Observing Systems (NERACOOS; www.neracoos.org, Pettigrew et al., 2011). These buoys are all located in coastal waters of the Gulf of Maine, USA. Buoy B is representative of the Western Maine Shelf; Buoy E is representative of the Central Maine Shelf; Buoy F is located at the mouth of Penobscot Bay; and Buoy I is representative of the Eastern Maine Shelf (Figure 1). All four buoys became active during July 2001 and record water temperature on an hourly basis at the surface (1 m), 2, 20, and 50 m. Temperatures for 2002–2016 were used for analyses presented herein.
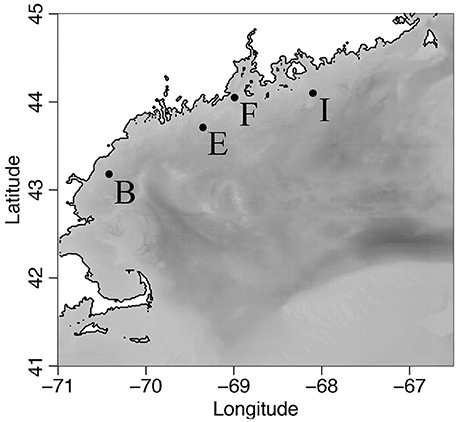
Figure 1. Site map of NERACOOS buoys from which water temperatures at 50 m depth were derived. Buoys B, E, and I are representative of the Western, Central, and Eastern Maine Shelf, respectively. Buoy F, at the mouth of Penobscot Bay, is influenced by river outflow.
To process the temperature data, a daily average is first computed, and then an 8-day centered moving average is applied. When data are missing at depth (0.1–3.2% of the data points at each buoy) and available at the surface, a linear regression is used to fill the gaps in the temperature record at depth, by using the 8-day smoothed surface temperature as the independent variable. This generates smoothed time series of temperatures at 50 m for each of the four buoys.
For each buoy, a mean annual temperature cycle is generated by averaging daily temperatures between 2002 and 2011. This mean annual cycle is then subtracted from the smoothed daily temperatures (2002–2016) to produce a daily temperature anomaly time series for each buoy. These are also averaged over the four buoys to produce one time series of region-wide anomalies. For simplicity, we will only present models built using data from the 50 m sensors. This depth provides the best available approximation to bottom temperatures for Maine's coastal waters where the bulk of the lobster fishery occurs. Models built using data from other depths produce similar results.
We assess temperature persistence at the four buoys and for the 4-buoy average to understand its potential influence on the forecast. This analysis is conducted by computing the Pearson product moment correlation between temperatures at 50 m on the forecast day and daily 50 m temperatures for the remainder of the year. For this analysis, we use only direct temperature measurements, not those derived from surface-depth regressions.
Lobster Landings Phenology
Although regulations enable the Maine lobster fishery to remain open year-round, for practical purposes there is a strong seasonality to its operation. Landing rates are low during the winter but increase abruptly in the early summer as lobsters move into nearshore waters, become more active, and molt to larger sizes. The annual cumulative landings show a sharp change in slope between late June and mid-July (Figure 2). We define the day when this change occurs as the “start day” for the high-landings period.
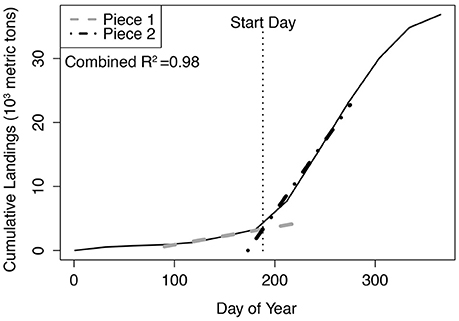
Figure 2. Cumulative landings (103 metric tons) in 2009, demonstrating the piecewise regression method for defining start day. Landings data between day-of-year 90 and day-of-year 275 are split into two pieces. These are fitted by simple linear regression, shown by the gray and black dashed lines, and their intersection defines the start day, shown by the vertical dotted line. Landings in 2009 would indicate a start day on day-of-year 188 (July 7).
Statewide landings data are provided by the Maine Department of Marine Resources. From 1990 to 2007, they are reported as monthly totals; from 2008 to 2016, they are available as daily totals. Because daily data are not available prior to 2008, we use a procedure to find the start day using only monthly data. For years in which only monthly data are available, the cumulative landings over the year are computed to obtain the total biomass of landings at the end of each month. We then linearly interpolate between months to approximate daily cumulative landings, whereby we implicitly assume that the landing rate is constant within each month. For 2008–2016, we compared the start days defined by the monthly vs. daily landings and found a tight correlation (Pearson's product moment: r = 0.985, p < 0.01). Subsequently, we apply a standard procedure across all years that uses the daily interpolation of monthly landings to determine the start day.
To define the start day for each year, piecewise linear regression is used to fit two lines to the cumulative daily landings. The first portion incorporates data from March 31 to day d; the second piece uses data from day d to October 2 (Figure 2). The model fitting procedure is repeated for values of d between March 31 and October 2. We quantify the performance of the model for each break day d by taking the weighted average of the R2-values of the two linear components.
where n1 is the number of days between March 31 and d, n2 is the number of days between d and October 2, and R12 and R22 are the R2-values of the corresponding linear models. The piecewise regression model with the highest weighted average R2 is selected, and the date on which the two lines intersect is recorded as the start day for that year. Similar methods have been employed to identify transition dates in studies of vegetation phenology (e.g., Zhang et al., 2013; Sweet et al., 2015).
Temperature-Start Day Relationships
After the annual start days are specified, their relationship to temperature can be investigated. Through this analysis, we determine if statistical relationships exist between the start day and 8-day smoothed 50 m water temperature anomalies, and if so, a time period in which these relationships are the strongest. We fit linear models relating the annual time series of temperature on a particular day (also termed the “forecast day”) to the start day time series. Models were developed for temperatures from each buoy and for the region-wide average (a total of five temperature time series) for dates between January 1 and June 30. The performance of these models was compared using their R2 statistics to identify a time window during which statistically reliable forecasts may be possible and that we would use as the forecasting period.
Paired data on NERACOOS buoy temperatures and lobster fishery start days are available for 2002 through 2016. In order to test the predictive power of the forecast, we used data from this full time series to evaluate how well the forecast may perform if future years are similar to the range of experiences in the past. Theoretical forecasts were simulated for each year using a leave-one-out approach, whereby the data from the year being predicted were excluded (i.e., a theoretical forecast for 2002 was generated using the data from 2003 to 2016). Due to the relatively small sample size and the presence of at least one “extreme” data point (2012), 95% prediction intervals were found to be too wide to be useful for communication and application. Instead, the forecast is constructed using a modified Monte Carlo (mMC) approach in order to more clearly represent the uncertainty in the forecast and the spread of the predictions based on the variance in the dataset. The mMC approach fits a series of temperature-start day linear regression models using a random sample of the available years. From the 13 years of data available to use for each forecast, the mMC simulation randomly selects 9, performs a univariate linear regression, and then uses the temperature from the forecast year to predict the start day. For each of the five temperature time series, 3,000 mMC simulations are run to ensure that repeated forecasts would be appropriately stable.
Forecast Communication
The forecast is made available to the public in the form of a graphical histogram showing the proportion of mMC simulations that falls into each of seven 1-week bins. The “normal” bin is bounded by June 30th and July 7th, and is centered on the mean start day for 2002–2011 (July 3rd), a period of years that aligns with the temperature baseline. Seven bins were required in order to keep the forecast image symmetrical while encompassing the range of observed start days. The bins are shaded according to the proportion of mMC simulations they contain, which we interpret as the likelihood of the start day being observed in that bin.
We updated the forecast weekly during the forecasting period. Results were served via a website (www.gmri.org/lobster-forecast) that delivers the forecast for the week as well as the series of forecasts produced for the season so that changes can be viewed over the forecasting period. In addition, the website provides interpretive information that (1) contextualizes temperature patterns that are shaping the forecast and (2) provides a comparison of the current forecast to comparable years in the past.
Results
Between 2002 and 2016, the spring (March, April, May) temperature range spanned ~3°C, from negative anomalies of −1.45°C in May 2004 to positive anomalies of 1.73°C in March 2012. The strength and direction of temperature anomalies in most years persisted over the spring months, with patterns in March, April, and May exhibiting tight relationships to one another. Strong negative anomalies (< −1.0°C in March, April or May) were observed in 2003 and 2004, while strong positive temperature anomalies (>1.0°C) were experienced in 2002, 2006, 2012, 2013, and 2016 (Figure 3). An analysis of temperature persistence at the buoys used for this analysis indicates that 50 m water temperature on April 1 serves as a good indicator of 50 m temperature through early to mid-October for Buoys B, E, and F and through the end of the year for Buoy I (Figure 4).
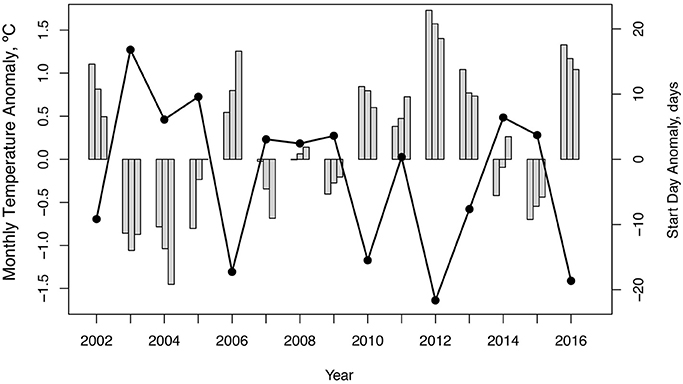
Figure 3. Spring temperatures and start days, 2002–2016. Bars show the monthly temperature anomalies for March, April, and May. The black line plots the start day anomaly from the 2002–2011 mean. The start day anomaly is generally anti-correlated with the spring temperature anomaly.
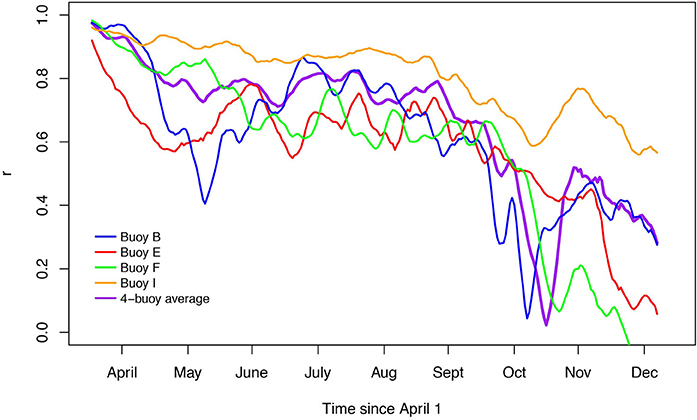
Figure 4. Persistence of correlation to April 1 temperature. Correlation of 50-m temperatures at four buoys on April 1 to temperatures on subsequent days of the year. Statistically significant correlations persist through early to mid-October at Buoys B, E, and F; through mid-November for the 4-buoy average; and through the end of the year for Buoy I.
The mean start day of the fishery between 2002 and 2011, as defined by our two-part linear procedure, was July 3. Over the entire study period, the start day varied by ~40 days, from a season that began more than 20 days earlier than the mean (negative anomaly) in 2012 to one that started 16 days later than the mean (positive anomaly) in 2003. Positive start day anomalies of more than 5 days, reflecting a late start to the season, were characteristic of 2003, 2004, 2005, and 2014. The start day defined for 2012 was the earliest in the time series, but start day anomalies in 2002, 2006, 2010, 2013, and 2016 were also more than 5 days early. A general negative association was observed between temperature anomalies and start day anomalies (Figure 3).
The strength of the relationship between 8-day smoothed 50 m temperature and start day increases from January 1 to early spring, becoming statistically significant (p < 0.05) by late January. The average coefficient of determination (R2) over all models exceeds 0.7 from March 6 through May 3, and it exceeds 0.75 from March 8 through April 20 (Figure 5). Buoy F 50 m temperatures and the 4-buoy 50 m temperature average appear to have higher capacity for predicting the start day than other buoys through March and April. The predictive capacity of Buoy B, F, and the 4-buoy average 50-m temperatures remains relatively stable through March and April. The predictive capacity of temperatures at Buoy I peaks in late March and Buoy E peaks in early April (Figure 5). Based on these results, we defined March 6 through May 3 as the forecasting period, as temperatures during this span of time provide a strong indication of the timing of the fishery 3–4 months in the future.
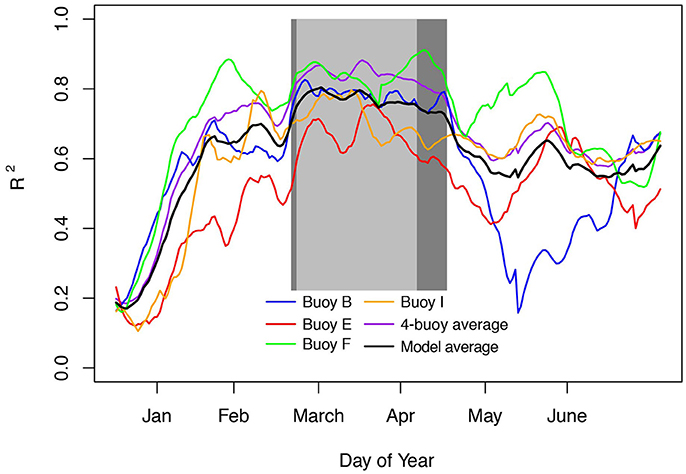
Figure 5. Temperature and start day correlations. Temperatures on January 1 through June 30 at each buoy and for the 4-buoy average were used to predict the start day of the fishery. Results show the R2-value of these regression models by day. The model average R2-values exceeded 0.75 from March 8 through April 20 (light gray rectangle) and were above 0.7 for March 6 through May 3 (dark gray rectangle).
We used April 1 as the forecast date for display purposes in this paper since R2 values over all models were high through this date (Figure 5). We can evaluate the forecast by considering how the likelihood of predicted fishery start days compares to the “observed” start day defined by the stepwise regression analysis. Across conditions experienced in all years since 2002, the sign of the deviation of predicted start days from the center of the “normal” bin was consistent with the observed start days (Figure 6). Further, for conditions in most years, the actual start days would have been within or adjacent to the bin that was predicted to have the highest likelihood of containing the start day (Figure 6). For 10 of the 15 years, more than half of the mMC simulations predicted a start day within a 7-day window centered on the observed start day (Figure 6). The forecast model performed well for conditions in 2005, 2008, 2009, and 2012, with a greater than 33% likelihood of the predicted start days falling within a 3-day window centered on the observed start day. Conversely, the model performed poorly under 2006 conditions, with ~4% likelihood of predicting the start day within a 7-day window of the date defined as the actual start. Even for 2006 conditions though, the model would have correctly forecasted the direction of deviation from the “normal” bin by predicting an early start of the high landings period. There is no obvious bias between skill in forecasting early years as opposed to late years, and the skill in an extremely early year like 2012 is similar to the skill in years with more normal temperature conditions, such as 2008 or 2009 (Figure 6).
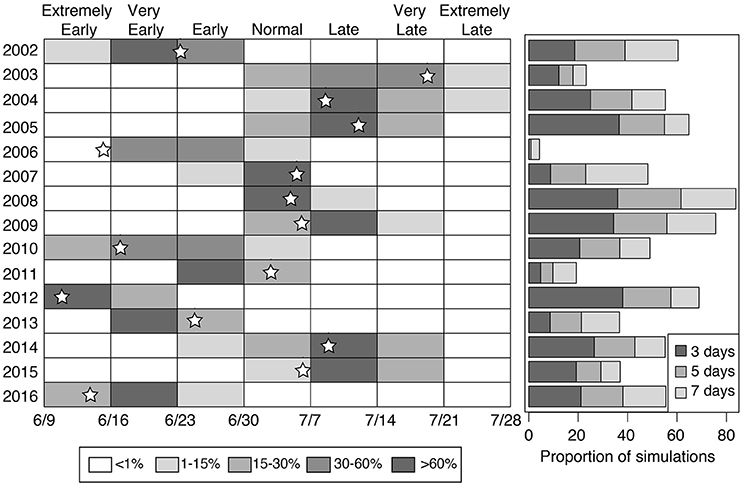
Figure 6. Past performance of forecast. An April 1 forecast is run for each year 2002–2016, using all available data except the year being tested. In the left panel, the boxes represent 7-day windows, centered on a “normal” start day bin that encompasses June 30-July 7. Bins are shaded according to the percentage of simulations that predict a start day in the week represented by the bin (legend shown below panel). The observed start day is plotted as a star. In the right panel, the stacked bar plot shows the proportion of mMC simulations predicting a start day inside a 3-, 5-, and 7-day window centered on the observed start day.
Demonstrating the forecast for 2012 conditions, the 4-buoy average model built with data from 2002 to 2016 (excluding 2012) would have given advanced warning of an extremely early start to the summer season based on conditions on April 1 (R2 = 0.86; Figure 7). The temperature-start day regression model would have predicted a start day of 166 (June 15), while the actual start occurred even a few days earlier than that, on day 163 (June 12; Figure 7). The forecast would have been for an extremely early start to the season (>75% likelihood), and it would have been stable as the spring progressed (Figure 8).
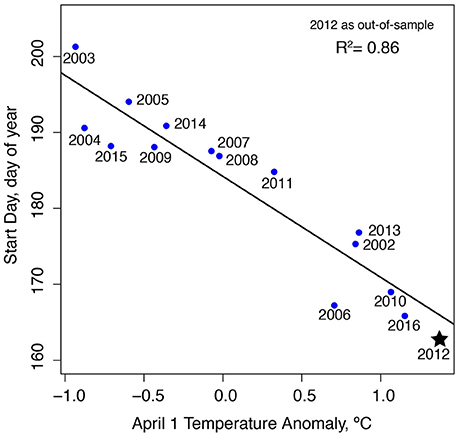
Figure 7. Simple linear regression between start day and April 1 temperature anomaly. Start days from 2002 to 2016 are plotted against the April 1 (4-buoy average) temperature anomaly from the same year. Treating 2012 as an out-of-sample test, the linear regression fits well (R2 = 0.86) and would predict a start day of 166 (June 16). The observed start day in 2012 was 163 (June 12).
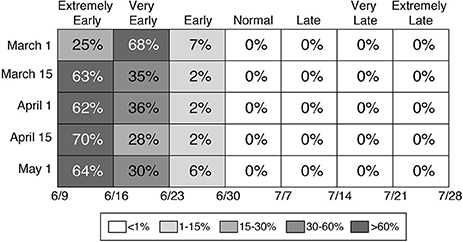
Figure 8. 2012 forecast series. A forecast is run on five dates in 2012, using data available from 2002 to 2016. The boxes, representing 7-day bins, are shaded according to the proportion of mMC simulations that predict a start day in that bin (legend shown below panel), and the proportion (rounded to the nearest percent) is displayed in each box. Based on spring temperatures in 2012, a high likelihood for an extremely early start to the high landings period would have been predicted by a forecast issued as early as March 15, and this prediction remained stable through May 1.
Discussion
As seasonal cycles that cue ecological events and human activities change in ways that move beyond typical past experiences, decision-making under variable environmental conditions will increasingly benefit from forward-looking information about resources of interest at appropriate temporal and spatial scales. We have demonstrated the technical capacity to forecast the timing of the seasonal increase in statewide landing rates in the Maine lobster fishery from buoy-based temperature observations. Using 50 m water temperatures from four NERACOOS buoys that span the coast of Maine, we are able to forecast the start day of the fishery to within 1 week of the actual start under conditions experienced in most test years. Further, we can issue this forecast 3–4 months prior to the typical shift into the high-landings period, providing important advance notice of major shifts in the timing of the fishery. The forecast performed well under conditions experienced during an extremely warm year in which the high-landings period began very early (i.e., 2012), indicating its potential reliability as climate change progresses and pushes conditions beyond those experienced in the past.
Seasonal forecasts of marine resources based on temperature have proven useful for a variety of purposes (Hobday et al., 2016). These resource-focused forecasts typically rely on an underlying forecast of temperature, but the skill of temperature forecasts is weak in coastal waters of the Northeast U.S. (Stock et al., 2015). In this forecast for the Maine lobster fishery, our predictive capacity is likely derived in part from temperature persistence. Over the Northeast U. S. large marine ecosystem, April sea surface temperatures are strongly correlated with temperatures 2 months in the future, and weaker correlation signals are maintained for 5 months (Stock et al., 2015). An analysis of temperature persistence at the buoys used for this analysis provides similar results for temperatures at 50 m depth (Figure 4).
The predictive skill of the Maine lobster fishery forecast is also likely attributable to lagged biological responses to temperature and a tight biological-social link that initiates the rise in landings rates. The annual landings cycle of the fishery is driven by the migration and molting cycle of the lobster population. The small-boat fleet (~80% of all vessels) relies on lobsters migrating into shallow nearshore waters, molting into legal size classes, and becoming more active so that they move into traps. Both seasonal migration and smaller-scale movement behaviors are cued by temperature (Cooper and Uzmann, 1971; Ennis, 1984; Crossin et al., 1998). Temperature has been shown to be an important factor in the initiation and progression of molting (Aiken, 1973; Aiken and Waddy, 1975; Kelly, 1993), a critical process that creates a tight biological-social link since 85% of landings in the fishery are of lobsters that have recently molted into a legal size class (Atlantic States Marine Fisheries Commission, 2015). It has been proposed that early stages of the molting process are triggered when a temperature of 5–6°C is reached, after which the ambient temperature determines the number of days to molting (Kelly, 1993). The fact that a temperature threshold appears to initiate the molting process provides a possible mechanism connecting April temperatures to molt timing and an uptick in fishery landings in June or July. Preliminary analyses indicate correlations between (1) the April 1 temperature anomaly and day when the 8-day smoothed 5°C temperature threshold is reached (r = −0.85 for 4-buoy average) and (2) the temperature threshold date and fishery start day (r = 0.80 for 4-buoy average). This threshold trigger for molting may also explain why the predictive skill of the forecast declines in May, rather than steadily improving as the start day draws closer (Figure 5). Further investigation is needed to fully evaluate this potential mechanism and to understand how a temperature threshold that initiates molting vs. subsequent temperatures that control the speed of that process may affect the forecast of the start day, which inherently integrates both types of temperature influences.
Grounding this forecast in a mechanistic framework is an important step in its future development. At present, the forecast model is empirical and effectively amounts to a hypothesis that temperatures in the spring trigger a chain of events that leads to inshore movement and molting of lobsters, causing the fishery to shift into its high landings summer mode. Empirical models such as this have a poor track record in fisheries (Myers, 1998) and their reliance on historical data is inherently limiting when they are applied to novel conditions. As extreme events like the 2012 heat wave are overlaid on rising mean trends, conditions will move beyond historical analogs, and forward-looking information streams that are not bounded by past conditions will be needed to provide insights that shape operational and management decisions in fisheries (Mills et al., in review). The empirical nature of the current forecast may make it less accurate in future years in which temperature patterns deviate from those encountered during the study period; this is particularly likely for years in which the temperature cycle deviates substantially from a sinusoidal shape. More importantly, the dependent variable—the timing of landings—is complex and combines influences of lobster biology with the behavior of fishermen. The latter can be strongly impacted by changes in economic conditions or regulations.
Our motivation to develop this forecast stemmed from a need expressed by leaders within the Maine lobster industry and management system following the 2012 Northwest Atlantic marine heat wave (Mills et al., 2013), and we have now demonstrated the technical capacity to issue a forecast of the seasonal timing of the lobster fishery. Since we have started providing the forecast, initial conversations with industry users indicate that they have applied the forecast information to guide decisions as diverse as scheduling seasonal maintenance and operations, setting debt payment dates, and planning for transportation needs to distribute the lobsters to processors or retail outlets. We anticipate that the forecast will enhance preparedness of the industry, supply chain, and managers for the start of the season, particularly in extremely early years in the future. This awareness should help align production capacities, marketing initiatives, and other operations to ensure a smooth flow of lobsters from the water to tables across the globe. This continuity may help optimize economic value derived from the resource during a period when lobster productivity is increasing in the state (Atlantic States Marine Fisheries Commission, 2015). In contrast to the situation in Maine, substantial temperature-related lobster population declines have decimated the fishery in more southern regions of New England USA (Atlantic States Marine Fisheries Commission, 2015). Operating the fishery and its supply chain in ways that maximize economic benefits may prove critical for buffering impacts of any future climate-related productivity declines that may occur in Maine. Given the state's high dependence on the lobster resource (i.e., 85% of the value of all fishery landings), forward-looking forecast information can improve near-term operational decisions and longer-term planning efforts to help sustain the fishery, its associated industry, and coastal communities.
While we have developed the technical capacity to issue a forecast that we anticipate will provide valuable information to users, real-world application of forecasts has proven challenging in a variety of situations (Hobday et al., 2016), and we anticipate similar challenges to uptake of the forecast information. Although it appears that we could have provided a reliable forecast of the early start to the 2012 season, sudden provision of this forecast in 2012 may not have helped the situation on the ground at that time. End-users need time to become familiar with a forecast and develop their own sense of its reliability and how to use it before they are likely to act on the information. Issuing the forecast even in years when major impacts to the landings cycle are not expected provides potential users with opportunities to relate the information to their own operations, which may be particularly valuable if another major disruption is predicted in the future. Longer-term familiarity with and confidence in the forecast may spur forward-looking planning, which will enhance resilience of individual operators and of this integrated industry to future disruptions.
Further development of the forecast will also benefit from additional end-user engagement. Hobday et al. (2016) recommended systematic engagement of stakeholders in the forecasting process to ensure the applicability and usability of the information in real-world decision-making contexts. This three-stage process begins by assessing needs of the end users, including relevant temporal and spatial scales. A forecast can then be developed to target these information needs. Finally, the implementation stage entails delivering the forecast, supporting and educating users, applying information to a decision, and gathering feedback to improve the product (Hobday et al., 2016). Initial input from users has indicated that developing the forecast at smaller spatial scales (e.g., for coastal regions or lobster management zones) would make the information more relevant and useful. In addition, benefits may be gained by targeting communication strategies and formats to specific user groups. We view the forecast that we describe herein as a pilot stage from which we can work toward a future product that is shaped by more deliberate and focused stakeholder input so that it can support a range of applications by end users.
The impacts of climate change are being felt by fisheries in many marine ecosystems. While climate-related forecast information tends to focus on gradual environmental trends over 50- to 100-year time horizons, fishing industry participants face decisions on much more immediate time scales. These decisions may be affected not just by the magnitude of environmental conditions but also by their timing, as evidenced by the experience of the Maine lobster fishery during the 2012 ocean heat wave (Mills et al., 2013). Providing forward-looking information relevant to the scales at which decisions are made is a critical step, and seasonal forecasts offer one avenue toward achieving this alignment. As shifts in the timing of warming and cooling occur in marine ecosystems (Burrows et al., 2011; Thomas et al., 2017), the phenology of life events in organisms, operations and outcomes of fisheries targeting those species, and effectiveness of fishery management efforts may all be affected (Mundy and Evenson, 2011; Peer and Miller, 2014). Seasonal forecast information directed toward the phenology of important events can provide a longer planning horizon than weather forecasts and bring climate information to bear on fisheries at a time scale that is meaningful for operational and management decisions made throughout the fishing industry.
Author Contributions
AP and KM conceived of the forecast. CH led the design of the model with guidance from AP and KM. CH implemented the models. All authors wrote the paper.
Conflict of Interest Statement
The authors declare that the research was conducted in the absence of any commercial or financial relationships that could be construed as a potential conflict of interest.
Acknowledgments
This forecast was initiated with support from NSF Coastal SEES (OCE 1325484) and was developed with funds from NASA EPSCoR through Maine Space Grant Consortium (EP-15-03). The Maine Department of Marine Resources (ME DMR) provided landings data. Conversations with Carl Wilson (Maine Department of Marine Resources), Patrice McCarron (Maine Lobstermen's Association), and Curt Brown shaped early phases of the forecast development.
References
Aiken, D. E. (1973). Proecdysis, setal development, and molt prediction in the American lobster (Homarus americanus). J. Fish. Res. Board Can. 30, 1337–1344. doi: 10.1139/f73-214
Aiken, D. E., and Waddy, S. L. (1975). Temperature increase can cause hyperecdysonism in American lobsters (Homarus americanus) injected with ecdysteron. J. Fish. Res. Board Can. 32, 1843–1845. doi: 10.1139/f75-218
Alexander, K. E., Leavenworth, W. B., Willis, T. V., Hall, C., Mattocks, S., Bittner, S. M., et al. (2017). Tambora and the mackerel year: phenology and fisheries during an extreme climate event. Sci. Adv. 3:e1601635. doi: 10.1126/sciadv.1601635
Alexander, M. A., Scott, J. D., Friedland, K., Mills, K. E., Nye, J. A., Pershing, A. J., et al. (in press). Projected sea surface temperatures over the 21st century: Changes in the mean, variability extremes. Elementa.
Atlantic States Marine Fisheries Commission (2015). American Lobster Benchmark Stock Assessment and Peer Review Report. Atlantic State Marine Fisheries Commission, Stock Assessment Report, Washington, DC.
Burrows, M. T., Schoeman, D. S., Buckley, L. B., Moore, P., Poloczanska, E. S., Brander, K. M., et al. (2011). The pace of shifting climate in marine and terrestrial ecosystems. Science 334, 652–655. doi: 10.1126/science.1210288
Cooper, R. A., and Uzmann, J. R. (1971). Migrations and growth of deep-sea lobsters, Homarus americanus. Science 171, 288–290. doi: 10.1126/science.171.3968.288
Crossin, G. T., Abdulazziz Al-Ayoub, S., Jury, S. H., Howell, W. H., and Watson, W. H. (1998). Behavioral thermoregulation in the American lobster Homarus americanus. J. Exp. Biol. 201, 365–374.
Ennis, G. P. (1984). Small-scale seasonal movements of the American lobster Homarus americanus. T. Am. Fish. Soc. 113, 336–338. doi: 10.1577/1548-8659(1984)113<336:SSMOTA>2.0.CO;2
Hamlet, A. F., Huppert, D., and Lettenmaier, D. P. (2002). Economic value of long-lead streamflow forecasts for Columbia river hydropower. J. Water Resour. Plan. Manage. 128, 91–101. doi: 10.1061/(ASCE)0733-9496(2002)128:2(91)
Hansen, J. W., Mason, S. J., Sun, L., and Tall, A. (2011). Review of seasonal climate forecasting for agriculture in sub-Saharan Africa. Expl. Agric. 47, 205–240, doi: 10.1017/S0014479710000876
Hill, K. T., Crone, P. R., Demer, D. A., Zwolinski, J., Dorval, E., and Macewicz, B. J. (2014). Assessment of the Pacific Sardine Resource in 2014 for U.S.A. Management in 2014-2015. NOAA 521. Silver Spring, MD: Technical Memorandum NOAA-TM-NMFS-SWFSC-531. Available online at: https://swfsc.noaa.gov/publications/TM/SWFSC/NOAA-TM-NMFS-SWFSC-531.pdf
Hobday, A. J., Spillman, C. M., Paige Eveson, J., and Hartog, J. R. (2016). Seasonal forecasting for decision support in marine fisheries and aquaculture. Fish. Oceanogr. 25(Suppl. 1), 45–56. doi: 10.1111/fog.12083
Jacobson, L. D., and McClatchie, S. (2013). Comment on temperature-dependent stock-recruit modeling for Pacific sardine (Sardinops sagax) in Jacobson and MacGall (1995), McClatchie et al. (2010), and Lindegren and Checkley (2013). Can. J. Fish. Aq. Sci. 70, 1566–1569. doi: 10.1139/cjfas-2013-0128
Kelly, K. (1993). Temperature-Molt Relationships in Lobsters Near Boothbay Harbor, Maine 1989-92. Boothbay Harbor, ME: Maine Department of Marine Resources.
Kwon, H.-H., Brown, C., Xu, K., and Lall, U. (2009). Seasonal and annual maximum streamflow forecasting using climate information: application to the three gorges dam in the Yangtze River basin, China. Hydrolog. Sci. J. 54, 582–595. doi: 10.1623/hysj.54.3.582
Lazo, J. K., Morss, R. E., and Demuth, J. L. (2009). 300 billion served: sources, perceptions, uses, and values of weather forecasts. Bull. Am. Meteorol. Soc. 90, 785–798. doi: 10.1175/2008BAMS2604.1
Mills, K. E., Pershing, A. J., Brown, C., Chen, Y., Chiang, F., Holland, D., et al. (2013). Fisheries management in a changing climate: lessons from the 2012 ocean heat wave in the Northwest Atlantic. Oceanography 26, 191–195. doi: 10.5670/oceanog.2013.27
Mora, C., Frazier, A. G., Longman, R. J., Dacks, R. S., Walton, M. M., Tong, E. J., et al. (2013). The projected timing of climate departures from recent variability. Nature 502, 183–187. doi: 10.1038/nature12540
Mundy, P. R., and Evenson, D. F. (2011). Environmental controls of phenology of high-latitude Chinook salmon populations of the Yukon River, North America, with application to fishery management. ICES J. Mar. Sci. 68, 1155–1164. doi: 10.1093/icesjms/fsr080
Myers, R. A. (1998). When do environment-recruitment correlations work? Rev. Fish Biol. Fish. 8, 285–305. doi: 10.1023/A:1008828730759
National Marine Fisheries Service (2016). Commercial Fisheries Statistics. Available online at: https://www.st.nmfs.noaa.gov/commercial-fisheries (Accessed on December 27, 2016).
Peer, A. C., and Miller, T. J. (2014). Climate change, migration phenology, and fisheries management interact with unanticipated consequences. N. Am. J. Fish. Manage. 34, 94–110. doi: 10.1080/02755947.2013.847877
Pershing, A. J., Alexander, M. A., Hernandez, C. M., Kerr, L. A., Le Bris, A., Mills, K. E., et al. (2015). Slow adaptation in the face of rapid warming leads to collapse of the Gulf of Maine cod fishery. Science 350, 809–812. doi: 10.1126/science.aac9819
Pettigrew, N. R., Fikes, C. P., and Beard, M. K. (2011). Advances in the ocean observing system in the Gulf of Maine: technical capabilities and scientific results. Mar. Technol. Soc. J. 45, 85–97. doi: 10.4031/MTSJ.45.1.11
Poloczanska, E. S., Brown, C. J., Sydeman, W. J., Kiessling, W., Schoeman, D. S., Moore, P. J., et al. (2013). Global imprint of climate change on marine life. Nat. Clim. Change 3, 919–925, doi: 10.1038/nclimate1958
Ramírez-Rodrigues, M. A., Alderman, P. D., Stefanova, L., Cossani, C. M., Flores, D., and Asseng, S. (2016). The value of seasonal forecasts for irrigated, supplementary irrigated, and rainfed wheat cropping systems in northwest Mexico. Agric. Syst. 147, 76–86. doi: 10.1016/j.agsy.2016.05.005
Shepard, C. C., Agostini, V. N., Gilmer, B., Allen, T., Stone, J., Brooks, W., et al. (2012). Assessing future risk: quantifying the effects of sea level rise on storm surge risk for the southern shores of Long Island, New York. Nat. Hazards 60, 727–745. doi: 10.1007/s11069-011-0046-8
Stock, C. A., Pegion, K., Vecchi, G. A., Alexander, M. A., Tommasi, D., Bond, N. A., et al. (2015). Seasonal sea surface temperature anomaly prediction for coastal ecosystems. Prog. Oceanogr. 137, 219–236. doi: 10.1016/j.pocean.2015.06.007
Sweet, S. K., Griffin, K. L., Steltzer, H., Gough, L., and Boelman, N. T. (2015). Greater deciduous shrub abundance extends tundra peak season and increases modeled net CO2 uptake. Glob. Change Biol. 21, 2394–2409, doi: 10.1111/gcb.12852
Szuwalski, C. S., Vert-pre, K. A., Punt, A. E., Branch, T. A., and Hilborn, R. (2014). Examining common assumptions about recruitment: a meta-analysis of recruitment dynamics for worldwide marine fisheries. Fish Fish. 16, 633–648, doi: 10.1111/faf.12083
Thomas, A. C., Pershing, A. J., Friedland, K. D., Nye, J. A., Mills, K. E., Alexander, M. A., et al. (2017). Seasonal trends and phenology shifts in sea surface temperature on the North American northeastern continental shelf. Elementa Sci. Anthropocene 5:48. doi: 10.1525/elementa.240
Tommasi, D., Stock, C. A., Pegion, K., Vecchi, G. A., Methot, R. D., Alexander, M. A., et al. (2017). Improved management of small pelagic fisheries through seasonal climate prediction. Ecol. Appl. 27, 378–388. doi: 10.1002/eap.1458
Vert-pre, K. A., Amoroso, R. O., Jensen, O. P., and Hilborn, R. (2013). Frequency and intensity of productivity regime shifts in marine fish stocks. Proc. Natl. Acad. Sci. U.S.A. 110, 1779–1784. doi: 10.1073/pnas.1214879110
Keywords: seasonal forecast, temperature, fishery landings, lobster fishery, climate variability
Citation: Mills KE, Pershing AJ and Hernández CM (2017) Forecasting the Seasonal Timing of Maine's Lobster Fishery. Front. Mar. Sci. 4:337. doi: 10.3389/fmars.2017.00337
Received: 27 February 2017; Accepted: 12 October 2017;
Published: 02 November 2017.
Edited by:
Gretchen E. Hofmann, University of California, Santa Barbara, United StatesReviewed by:
Donald F. Boesch, University of Maryland Center For Environmental Sciences, United StatesArtur Piotr Palacz, International Ocean Carbon Coordination Project (IOCCP), Poland
Copyright © 2017 Mills, Pershing and Hernández. This is an open-access article distributed under the terms of the Creative Commons Attribution License (CC BY). The use, distribution or reproduction in other forums is permitted, provided the original author(s) or licensor are credited and that the original publication in this journal is cited, in accordance with accepted academic practice. No use, distribution or reproduction is permitted which does not comply with these terms.
*Correspondence: Katherine E. Mills, a21pbGxzQGdtcmkub3Jn