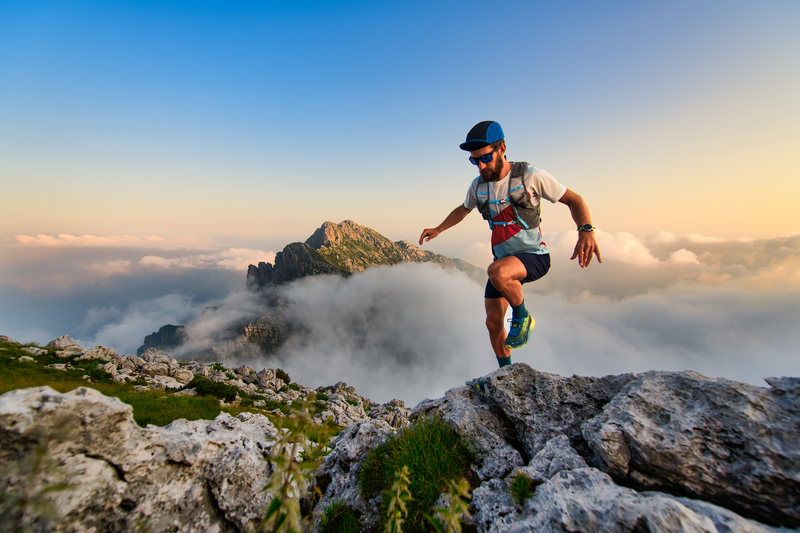
94% of researchers rate our articles as excellent or good
Learn more about the work of our research integrity team to safeguard the quality of each article we publish.
Find out more
ORIGINAL RESEARCH article
Front. Mar. Sci. , 30 June 2017
Sec. Global Change and the Future Ocean
Volume 4 - 2017 | https://doi.org/10.3389/fmars.2017.00201
This article is part of the Research Topic Seasonal to decadal prediction of marine ecosystems: opportunities, approaches and applications View all 11 articles
Decisions made by fishers and fisheries managers are informed by climate and fisheries observations that now often span more than 50 years. Multi-annual climate forecasts could further inform such decisions if they were skillful in predicting future conditions relative to the 50-year scope of past variability. We demonstrate that an existing multi-annual prediction system skillfully forecasts the probability of next year, the next 1–3 years, and the next 1–10 years being warmer or cooler than the 50-year average at the surface in coastal ecosystems. Probabilistic forecasts of upper and lower seas surface temperature (SST) terciles over the next 3 or 10 years from the GFDL CM 2.1 10-member ensemble global prediction system showed significant improvements in skill over the use of a 50-year climatology for most Large Marine Ecosystems (LMEs) in the North Atlantic, the western Pacific, and Indian oceans. Through a comparison of the forecast skill of initialized and uninitialized hindcasts, we demonstrate that this skill is largely due to the predictable signature of radiative forcing changes over the 50-year timescale rather than prediction of evolving modes of climate variability. North Atlantic LMEs stood out as the only coastal regions where initialization significantly contributed to SST prediction skill at the 1 to 10 year scale.
Living marine resources (LMRs) and the marine ecosystems within which they exist are critical to human health and coastal economies, providing services worth US$21 trillion each year (Costanza et al., 1997). LMRs are strongly influenced by climate variability (Cushing and Dickson, 1976; Sharp, 1987; Lehodey et al., 2006; Brander, 2007, 2010; Drinkwater et al., 2010; Ottersen et al., 2010), creating a challenge for marine resource managers and fishers. Temperature fluctuations, serving as proxies of important climate-driven ocean or ecosystem processes, are often associated with variation in the productivity and spatial distribution of LMRs (e.g., Ellertsen et al., 1989; Dorn, 1992; Peterman et al., 1998; Mueter et al., 2002, 2011; Beaugrand et al., 2003; Perry et al., 2005; Sullivan et al., 2005; Nye et al., 2009; Hunt et al., 2011; Kristiansen et al., 2011; Lindegren and Checkley, 2013; Pinsky et al., 2013; Pershing et al., 2015).
Century-scale temperature projections have been used to show the impact of climate change on LMRs (Stock et al., 2011). More recently, seasonal SST forecasts have started to improve management and industry decisions at shorter time-scales (Hobday et al., 2016; Tommasi et al., 2017a). Many decisions, however, would benefit from climate forecasts over multi-annual scales, in which both climate change and internal climate variability can act to provide predictability (Tommasi et al., 2017b). For instance, catch advice is dependent on a forecast of fish abundance 1–3 years into the future (Brander, 2003). To set rebuilding targets for overfished stocks, such forecasts need to be extended 10 years into the future (NRC, 2014). Currently, these stock status projections are developed using historical observations that often span 30–50 years (RAM Legacy Stock Assessment Database, www.ramlegacy.org). Fisheries managers are therefore interested in assessing if temperature over the next years to decade will be high or low relative to the past 30–50 years used to develop their management frameworks. Such forecasts can inform managers on the need to develop reference points more reflective of future conditions and climate-informed stock status projections (Tommasi et al., 2017b). Multi-annual climate predictions can also benefit long-term spatial planning decisions regarding changes to closed areas, the setting of future closures, preparation for emerging fisheries, adjustment of quotas for internationally shared fish stock, and industry capital investment decisions (Tommasi et al., 2017b). However, while the skill of seasonal SST forecasts has been assessed at an LMRs-relevant spatial scale (i.e. the coastal shelf) (Stock et al., 2015), multi-annual SST predictability in coastal ecosystems has not been quantified, limiting their use in LMRs management decisions.
How SST will evolve across inter-annual to decadal time scales is a function of both internal climate variability (e.g., El-Niño Southern Oscillation, ENSO; the Pacific Decadal Oscillation, PDO; Atlantic Multidecadal Variability, AMV) and forced climatic changes from greenhouse gases and aerosol emissions, as well as natural forcings like volcanoes and solar variations (Meehl et al., 2009, 2014; Doblas-Reyes et al., 2013). Thus, multi-annual predictive skill is dependent on initializing a climate model in the correct state of internal climate variations (i.e., an initial-value problem), imposing accurate external forcing (i.e., a boundary-value problem), and correctly simulating the evolution of the predictable climate system components arising from the initial state and external forcing. Each of these is challenging on its own. Several studies, however, have now shown that in the North Atlantic Ocean, the Southern Ocean, and, more weakly, the western North Pacific Ocean, initialization of the present climate state can significantly contribute to forecast skill over many years (van Oldenborgh et al., 2012; Doblas-Reyes et al., 2013; Yang et al., 2013; Meehl et al., 2014; Msadek et al., 2014; Corti et al., 2015). Further studies suggest that, over most of the globe, the main source of 2–10 year SST prediction skill is the externally forced signal due to greenhouse gases and aerosols (van Oldenborgh et al., 2012; Corti et al., 2015). That is, greenhouse gases, ozone and aerosol conditions today and their future evolution allow one to make meaningful predictions about the next decade relative to the range of conditions over the past half-century.
By exploiting both sources of predictability, skillful multi-annual SST predictions are possible (Smith et al., 2007; Keenlyside et al., 2008; van Oldenborgh et al., 2012; Doblas-Reyes et al., 2013; Yang et al., 2013; Corti et al., 2015), with the North Atlantic, Indian Ocean, and western Pacific being regions of significant skill even at the longer lead times of 6–10 years (Meehl et al., 2014). Interpretation of these results must be tempered by the limited effective sample size for assessing decadal predictions (Meehl et al., 2014) but they provide reason for cautious optimism concerning the use of multi-annual to decadal predictions for marine resource applications. It remains to be assessed if the observed multi-annual prediction skill over large ocean regions results in useful multi-annual prediction skill at the coastal scales relevant to most marine resource decisions. Furthermore, it is unclear whether the forced signal, which becomes prominent across century scales, is also sufficient to produce significant skill relative to the 30–50 year reference data sets common in fisheries management. That is, will the next 1–10 years be warmer or cooler than the past 30–50 years upon which decisions are being made?
In this paper, multi-annual SST forecasts are evaluated through this fisheries lens. More specifically, we assess the ability of the forecast system to predict if conditions over the next year, 1–3 or 1–10 years will be warmer or colder than the last 50. This is also the first time that these multi-annual SST predictions are evaluated over Large Marine Ecosystems (LMEs) (Figure 1), a coastal scale relevant to managed fisheries stock. LMEs are coastal areas of 200,000 km2 or greater, whose extent is determined by similarities in ecologically relevant variables including bathymetry, hydrography, productivity, and trophic relationships (Sherman, 2014). These coastal ecosystems serve as a particularly relevant scale for LMRs decisions as, while only making up ~1/10th of the world's oceans, they provide 95% of the world's total fish catch (Stock et al., 2017). We focus on assessing the probabilistic skill of the upper and lower terciles of SST, as these events are of greatest concern to LMRs managers and industry stakeholders (e.g., Spillman et al., 2015). While forecast users are largely concerned with the overall forecast skill, to improve multi-annual prediction systems it is also important to identify sources of prediction skill. Thus, to determine the sources of multi-annual SST predictability in LMEs we verify forecasts with both initialization and external forcing via greenhouse gases and aerosols (“initialized”) and those that include just the external forcing (“uninitialized”).
Figure 1. Global map of Large Marine Ecosystems (LMEs). Image Credit: NOAA Large Marine Ecosystem Program. No permission was required from the copyright holder for the preproduction of this image.
We enlist a set of initialized and uninitialized climate forecasts to accomplish our objectives. The uninitialized forecasts isolate the contribution of the “forced” signal (i.e., that due to greenhouse gases and aerosols), while the initialized forecasts include skill arising from both the forcing and the initialization. The combination thus allows us to determine the source of prediction skill, at least between these two broad categories. Both initialized and uninitialized climate forecasts are produced with the Geophysical Fluid Dynamics (GFDL) CM 2.1 global climate model (Delworth et al., 2006). CM 2.1 has an atmospheric resolution of 2° and an ocean resolution of 1° (see Table A1 in the Supplement for a specification of how many ocean model grid points are in each LME). In initialized predictions, the model is constrained by the observed climate state using the Ensemble Coupled Data Assimilation system (ECDA, Zhang et al., 2007; Zhang and Rosati, 2010, ECDA output is available at https://www.gfdl.noaa.gov/ocean-data-assimilation-model-output/). The atmosphere assimilates the NCEP atmospheric reanalysis (Kalnay et al., 1996), while the ocean is constrained by SST observations from satellite and profiles of temperature and salinity from the World Ocean Database (Boyer et al., 2009). All simulations use observed volcanic forcing.
The chaotic nature of the climate system, uncertain and limited observations used to initialize prediction systems, imperfections in climate model formulations, and uncertainty in future radiative forcing lead to climate predictions being inherently uncertain. The first three sources of uncertainty are the most dominant for multi-annual climate forecasts. To quantify some of this uncertainty, climate prediction centers produce a set of predictions called a forecast ensemble. Each ensemble member is a prediction produced using slightly different initial conditions. These ensemble forecasts are also essential to communicate to users the likelihood of future climate outcomes through probabilistic forecasts. Here, a 10-member ensemble of retrospective predictions of 1–10-year lead time was developed by first initializing the model on January 1 of every year from 1965-2011 and then making predictions of annual SST conditions 1–10 years into the future (Yang et al., 2013; Msadek et al., 2014). For instance, a 1-year lead SST forecast initialized in 1995 corresponds to the average SST from January to December 1995, while a 10-year lead SST forecast initialized in 1995 corresponds to the average SST from January to December 2004. Hence the hindcast period varies with lead time, spanning the period of 1965–2011 for a lead 1 forecast and the period of 1974–2020 for a 10-year lead prediction. The 47 initialization years, 10 lead times, and 10 ensemble members resulted in a total of 4,700 predictions. Using these predictions, we developed SST forecasts for management timeframes of interest to LMR users: over the next year, over the next 3 years, and over the next 10 years. A 1-year lead time prediction provided the forecast of next year SST conditions. Forecasts of annual SST conditions over the next 3 or 10 years were developed by averaging predictions of 1–3-year or 1 to 10-year lead times, respectively.
The model was forced with prescribed temporally varying anthropogenic (e.g., greenhouse gases) and natural (e.g., volcanic aerosols) radiative forcing following the CMIP5 historical and RCP4.5 scenarios (Meinshausen et al., 2011). The uninitialized 10-member ensemble predictions were computed in the same manner except that the model was not initialized to observations. Its initial state is consistent with the greenhouse gas and aerosol conditions for each year, but the internal modes of climate variability (e.g., ENSO) overlying this mean climate state are random. Thus, skill of the uninitialized predictions is only dependent on the forced signal (greenhouse gases and aerosols). The predictions were retrieved from the GFDL archive, but are also available on the GFDL data portal (data1.gfdl.noaa.gov). SST predictions for each LME were extracted from the global dataset and spatially averaged over each LME.
Forecast skill was evaluated against the Hadley Centre Sea Ice and Sea Surface Temperature (HadlSST1.1) observational dataset at 1° resolution. The monthly data for 1965–2015 was downloaded from the U.K. Met Office (www.metoffice.gov.uk/hadobs/hadisst/) and annual averages were computed for each LME. Note that because of ice coverage and other issues, the Antarctic and Arctic LMEs had sparse annual observational SST data going back to the 1960s and hence were removed from the analysis.
Due to imperfections in the climate model formulation, after initialization, the model drifts to its preferred climate state. Hence, there is a systematic difference between the model and the observed climatologies. This systematic bias in the mean was removed by subtracting a lead-dependent model climatology from the prediction and computing forecast anomalies (CLIVAR, 2011). The climatology spanned the length of the hindcasts and was produced using cross-validation, that is without the year being forecasted. Note that as observations were available up to 2015, the length of hindcasts that could be verified for lead times 6–10 years ranged from 46 to 42 years, respectively, instead of the 47 years used for lead times 1–5 years.
Once the forecast anomalies were produced, the upper and lower terciles of SST for each LME were calculated. Following Becker and van den Dool (2016), for each lead time, a normal distribution was fit over all available hindcasts (minus the year being forecasted) across all ensemble members, and the standard deviation (std. dev.) was calculated. Forecast anomalies above +0.43*std. dev. were considered above normal (upper tercile) and forecast anomalies below −0.43*std. dev. were considered below normal (lower tercile). Using the standard deviation fit to the forecast data to define the forecast terciles corrects for systematic biases in forecast spread (Becker and van den Dool, 2016).
Performance of the retrospective forecasts was assessed by calculating forecast accuracy and the Brier Score (BrS). Accuracy measures the proportion correct of a yes/no forecast of an event (Wilks, 2011) and is defined as:
Here the event was SST being in the upper (or lower) tercile. Upper and lower tercile events were assessed separately, and the prediction for each ensemble member, initiation year, and lead time was assigned a 1 (event happened) or a 0 (event did not happen). The event was forecast to occur if more than half of the ensemble members predicted the event. Hits were the number of times that both the forecast and observations were in the upper (or lower) tercile and correct negatives were the number of times that both the forecast and observations were not in the upper (or lower) tercile. Forecast accuracy ranges between 0 and 1 (perfect skill), with, for terciles, accuracy greater than 0.56 being better than chance (Spillman and Hobday, 2014).
Forecast accuracy is a relatively easy to understand skill metric, but all the ensemble predictions are reduced to a single yes/no forecast. Hence, we also produced a probability forecast for each category as the fraction of ensemble members being in the upper (or lower) tercile for each initiation year and lead time. The quality of the probabilistic forecast was assessed using the Brier Score (BrS). The BrS is an estimate of the mean square error of the probabilistic forecast (Wilks, 2011) and is defined as:
Where t is the lead time, f is the forecasted probability of an event determined by the fraction of forecast ensemble members within the upper (lower) tercile, o is the observed probability of an event (either 0 or 1), and N is the length of the hindcast period. The BrS ranges from 0 (perfect score) to 1.
Currently, fisheries decisions assume that future environmental conditions impacting fish productivity (i.e., recruitment, mortality, growth), will be like the past. Future productivity is determined by drawing random samples from the full range of past environmental conditions. Thus, it is of interest to evaluate forecast skill relative to climatology (i.e., assume that upper and lower tercile events will always have a 0.33 probability of occurrence). Probabilistic forecast skill was hence also assessed relative to climatology using the Brier Skill Score (BSS), which compares the BrS or the forecast to that of a reference forecast (Wilks, 2011):
The BrSref was computed using the climatological frequencies (0.33 in the case of tercile events).
Finally, to assess if most of the forecast skill was derived from correctly forecasting the internal variability of the climate system (i.e., ENSO or PDO events) or from the warming trend, the skill of the initialized forecast was compared to that of an uninitialized forecast by computing a BSS where the BrSref was the probability forecast of the uninitialized forecasts, post-processed in the same manner as the initialized forecasts. The BSS ranges from 1 (perfect score) to negative infinity (no skill). Negative scores indicate no additional skill as compared to the reference forecast.
Sampling uncertainty was assessed by computing confidence intervals for each of the skill metrics described above using a bootstrap procedure (Mason, 2008; Wilks, 2011). Forecast-observation pairs were randomly resampled with replacement to generate 1,000 new forecast-observation vectors of length 47. Skill metrics were computed for each of the 1,000 vectors to obtain a sampling distribution for the skill scores. The lower and upper 95% confidence intervals were defined by the 0.025 and 0.975 quantiles.
Forecast accuracy in predicting temperatures as being in the higher or lower tercile with a 1 year lead time exceeded 0.7 in 80% of LMEs (Figures 2–4). Forecast accuracy was particularly high for LME's in the North Atlantic, with LMEs from the Norwegian to the Iberian coastal shelves in the eastern Atlantic and the Newfoundland-Labrador Shelf, the Caribbean Sea, and the North Brazil Shelf on the western side exceeding 0.8 accuracy for predictions of both upper and lower tercile events (Figures 2, 3). In the Indian Ocean, forecast accuracy exceeded 0.8 in the West-Central Australian Shelf. While accuracy was generally lower for Pacific Ocean LMEs, upper and lower tercile events in the East China Sea, South China Sea and Sulu-Celeb Sea, were predicted with over 0.8 accuracy (Figures 2, 3). By contrast, forecast accuracy in southern and eastern Pacific Ocean LMEs, namely the Indonesian Sea, the Northeast and Southeast Australian shelves, New Zealand, the Humboldt Current, the Central-American shelf, the Gulf of California, and, for the lower tercile, the California Current, was not significantly higher than random chance (Figure 4). Forecast skill was also low in the Patagonia Shelf, the Benguela Current and the Southeast US (Figures 2–4).
Figure 2. Forecast accuracy (left) and Brier Score (BrS, right) for upper SST tercile predictions for a 1 year ahead forecast and over the next 3 and 10 years. Forecast accuracy ranges between 0 (no skill) and 1 (perfect skill). The BrS ranges from 0 (perfect score) to 1 (no skill).
Figure 3. Forecast accuracy (left) and Brier Score (BrS, right) for lower SST tercile predictions for a 1 year ahead forecast and over the next 3 and 10 years. Forecast accuracy ranges between 0 and 1 (perfect skill). The BrS ranges from 0 (perfect score) to 1 (no skill).
Figure 4. Forecast Accuracy with 95% confidence intervals for each LME for upper and lower SST tercile predictions for a 1 year ahead forecast and over the next 3 and 10 years. Accuracy scores higher than the dotted line are better than chance.
When the forecast lead time was extended to 1–3 years, forecast accuracy for both upper and lower terciles generally increased (Figures 2–4). At this lead time, accuracy greater than 0.8 was extended to most LMEs in the Indian and western Pacific Ocean, and to the Scotian Shelf, Northeast US, and East Brazil Shelf in the Atlantic Ocean (Figures 2, 3). However, accuracy remained not significantly different than that of a random forecast in the Benguela Current, Southeast US and the southern and eastern Pacific Ocean (Figures 2–4).
When the forecast lead time was extended to 1–10 years, forecast accuracy for both upper and lower tercile events increased in most LMEs (Figures 2–4). Forecast accuracy for both upper and lower terciles was greater than 0.9 for all LMEs in the Indian Ocean except the Gulf of Thailand, all Atlantic LMEs except the Southeast US, the South Brazil Shelf, and the Patagonia Shelf, and in the western Pacific from the East-Central Australian shelf to the Sea of Japan (Figures 2, 3). In the eastern Pacific, the only LME showing an increase in accuracy was the equatorial Central-American Shelf (Figures 2, 3). Accuracy remained not significantly different than that of a random forecast for the Gulf of Alaska, the California Current, the Gulf of California, and the Humboldt Current (Figure 4). The observed increased prediction skill from 1 year to 1–10 year averages reflects the prominence of the forced signal in driving multi-annual prediction skill. This may seem counter-intuitive, as the 10-year time horizon minimizes the value of initialization. However, for a 1–10 years prediction this negative impact is surpassed by longer averaging periods, which smooth out unpredictable internal variability, and longer time horizons increasing the prominence of a predictable forced signal. When the averaging period was reduced to three years, as in the 1–3 years forecast, for a prediction of conditions 8–10 years into the future, forecast accuracy, while still significant over many LMEs owing to the prominence of the forced signal, decreased as compared to a 1–3 years forecast because of the reduced impact of initialization at this longer lead time (Compare Figures 2, 3–5).
Figure 5. Forecast accuracy (top) and Brier Score (BrS, bottom) for upper and lower SST tercile predictions for a 8–10 years forecast. Forecast accuracy ranges between 0 and 1 (perfect skill). The BrS ranges from 0 (perfect score) to 1 (no skill).
Probabilistic forecast skill of both upper and lower tercile SST anomalies, as determined by the BrS, mirrored patterns in forecast accuracy. For a 1 year lead time, forecast error was highest in the Patagonia Shelf, the Southeast Australian Shelf, the Southeast US, the Benguela Current and the Gulf of California (Figures 2, 3, and Figure A1). Forecast error generally decreased with increasing forecast average, a notable exception being the eastern Pacific Ocean LMEs, the Patagonian Shelf, the South Brazil Shelf, the New Zealand Shelf, the Gulf of Thailand, and the Southeast US (Figures 2, 3, and Figure A1).
The accuracy and BrS demonstrated that forecasts of upper and lower SST terciles relative to conditions over the past 50 years were skillful for most LMEs globally and across lead times of interest to fisheries decisions (Figures 2–4). Forecasts were generally more skillful than climatology, with the BSS being positive over most LMEs for all lead times for both upper and lower tercile events (Figures 6, 7). As was the case with accuracy and BrS, BSS increased from 1, 1–3 to 1–10 years, with similar patterns of relative skill across systems. At 1 year lead times, uncertainty was sufficiently large that forecast skill was significantly better than climatology (i.e., BSS confidence interval did not cross 0) only in North Atlantic LMEs, the western equatorial Pacific Ocean LMEs, and the Western Central Australia Shelf (Figures 6, 7, and Figure A2). Skill over climatology was generally better for lower tercile events, with significantly positive BSS also extending to the Agulhas Current, Somali Coastal Current, Bay of Bengal, and all LMEs in the Australian shelf in the Indian Ocean; the California Current and Gulf of Alaska in the eastern Pacific Ocean; and to the Canary Current, the Scotian shelf, the Caribbean Sea, and the East and South Brazil shelves in the Atlantic Ocean (Figure 7 and Figure A2). As forecast skill improved with increasing forecast average for most LMEs, so did skill over climatology, except for the Southeast US, the Patagonian Shelf, the South Brazil Shelf, the New Zealand Shelf, and eastern Pacific LMEs which showed no improvement over climatology for a 10 years forecast average (Figures 6, 7, and Figure A2).
Figure 6. Brier Skill Score (BSS) for upper SST tercile predictions relative to climatology (left) and uninitialized predictions (right) for a 1 year ahead forecast and over the next 3 and 10 years. The BSS ranges from 1 (perfect score) to negative infinity (no skill). Negative scores indicate no additional skill as compared to the reference forecast.
Figure 7. Brier Skill Score (BSS) for lower SST tercile predictions relative to climatology (left) and uninitialized predictions (right) for a 1 year ahead forecast and over the next 3 and 10 years. The BSS ranges from 1 (perfect score) to negative infinity (no skill). Negative scores indicate no additional skill as compared to the reference forecast.
The BSS relative to the uninitialized forecasts was positive for most LMEs for both upper and lower tercile events for a 1 year lead time forecast, suggesting that initialization contributed to forecast skill at this lead time (Figures 6, 7). However, forecasts were uncertain and most lower 95% confidence intervals exceeded zero (Figure A3). Only predictions for the Celtic-Biscay Shelf, the Faroe Plateau, the Newfoundland-Labrador Shelf, the Gulf of Alaska, and the West-Central Australian Shelf LMEs showed significantly better skill than the uninitialized forecasts at a 1 year lead time (Figure A3). As forecast lead time progressed, skill relative to the uninitialized forecast decreased (Figures 6, 7). The BSS relative to the uninitialized forecast was near 0 or negative in many LMEs at the 10 years forecast average as much of the forecast skill was derived from the forced signal (Figures 6, 7). As for the 1 year lead time, there was largely no significant difference between initialized and uninitialized forecasts at 1–3 and 1–10 years lead times (Figure A3). Forecasts for LMEs around the Atlantic subpolar gyre, namely the Newfoundland-Labrador Shelf, Scotian Shelf, Faroes Plateau, Norwegian Sea, North Sea, Celtic-Biscay Shelf, and the Iberian Coastal, were an exception, showing significant skill over the uninitialized forecast for the 10 years forecast average for both upper and lower tercile events (Figures 6, 7 and Figure A3).
This work demonstrates that global climate forecasts have significant skill in predicting occurrence of above average warm or cold SST events at a multi-annual scale in coastal areas, with average being the mean SST conditions over the past 50 years. At a 1 year lead time, forecasts were more skillful than climatology in the North Atlantic, the Western Central Australia Shelf, and the western equatorial Pacific Ocean. Prediction skill increased with longer forecast averages. Predictions of upper and lower SST terciles over the next 3 or 10 years were significantly better than climatology over most LMEs in the North Atlantic, the western Pacific, and Indian oceans. This is consistent with studies showing multi-annual retrospective forecast skill over the Indian, western Pacific and North Atlantic oceans (van Oldenborgh et al., 2012; Doblas-Reyes et al., 2013; Karspeck et al., 2015).
Over large ocean regions, much of the predictive skill at a multi-annual scale is associated with the ability of multi-annual prediction systems to correctly predict the response of the climate system to increasing greenhouse gas emissions (van Oldenborgh et al., 2012; Doblas-Reyes et al., 2013; Yang et al., 2013; Müller et al., 2014; Corti et al., 2015; Karspeck et al., 2015). Here we show that this conclusion also applies to the regional shelf scale, with forecast skill of the uninitialized predictions being comparable to that of the initialized forecasts over most LMEs. Furthermore, as unpredictable internal variability is smoothed out by forecasting over a longer period (García-Serrano and Doblas-Reyes, 2012), the relative importance of the warming trend in driving SST increases for forecasts of SST conditions over the next 3 or 10 years and these forecasts were more skillful than 1 year lead time predictions. The importance of the radiatively forced signal in providing skill on a 1–10 years scale suggests the potential viability of a purely statistical forecasting system in those LMEs where the forced signal dominates. Such a comparison is outside of the scope of this paper. We note, however, that a statistical approach would be unable to capture changes in the rate of warming over time and the high multi-annual prediction skill of North Atlantic LMEs arising from initialization.
North Atlantic LME's stood out as the only ones where initialized SST forecasts for the next 10 years were significantly more skillful than the uninitialized predictions. Even when the forecast lead time was increased to 8–10 years, initialized forecast skill remained significantly higher than that of an uninitialized prediction for some North Atlantic LMEs (Figure A6). Other work has shown that initialization greatly improves multi-annual forecast skill in the subpolar gyre region of the North Atlantic (Keenlyside et al., 2008; Pohlmann et al., 2009; van Oldenborgh et al., 2012; Doblas-Reyes et al., 2013; Yang et al., 2013; Meehl et al., 2014; Corti et al., 2015; Karspeck et al., 2015). Multi-annual SST variability in this subpolar gyre region of the North Atlantic is influenced by ocean dynamics, particularly by decadal variability in the Atlantic meridional overturning circulation (Delworth et al., 1997; Matei et al., 2012; Robson et al., 2012a; Barrier et al., 2015). Hence, initialization of ocean conditions can substantially improve prediction skill (Matei et al., 2012; Robson et al., 2012b, 2014; Yeager et al., 2012; Yang et al., 2013; Msadek et al., 2014). Here we demonstrate that the higher skill derived from initialization can be extended to the coastal shelves influenced by the subpolar Atlantic gyre circulation.
Multi-annual prediction skill was limited for eastern Pacific coastal areas from the Gulf of Alaska to the Humboldt Current. Large scale SST variability in the eastern Pacific is strongly forced by the atmosphere (Chhak et al., 2009; Johnstone and Mantua, 2014), which has a short memory. Furthermore, ocean dynamics driving SST in this region, such as coastally trapped Kelvin waves and eastern boundary currents, act on a faster time scale than, for instance, the Atlantic overturning circulation. Hence, eastern Pacific SST predictability may be intrinsically more limited than in other regions, such as the North Atlantic, where slow ocean dynamics play a dominant role in determining SST fluctuations (Robson et al., 2014). For instance, Eastern Pacific SST variability is strongly influenced by ENSO and its teleconnections. This results in some of the highest seasonal SST prediction skill across global LMEs (Stock et al., 2015), but the low predictability for ENSO beyond the seasonal timescale (CLIVAR, 2001; Palmer et al., 2004; Jin et al., 2008; Tippett et al., 2012; Ludescher et al., 2014) reduces multi-annual prediction skill in this region (García-Serrano and Doblas-Reyes, 2012; Karspeck et al., 2015). Eastern Pacific LMEs also stood out because, unlike most LMEs, multi-annual predictability declined with increasing forecast averages. This may stem from the lower relative importance of the linear warming trend over internal variability in determining SST variability in this region (Doblas-Reyes et al., 2013, Karspeck et al., 2015). Indeed, Eastern Pacific LMEs show some of the weakest evidence of a warming trend, with SST over the two major upwelling regions, the California and Humboldt currents, decreasing over the past thirty years (Belkin, 2009).
Multi-annual predictability is also limited by model error. SST bias of CM 2.1 is most evident in upwelling regions and in the Southern Ocean (Delworth et al., 2006). LMEs in the major upwelling centers, the California, Humboldt, and Benguela currents, and those bordering the Southern Ocean, namely the Southeast Australian Shelf, New Zealand, and the Patagonian Shelf showed some of the lowest multi-annual prediction skill. Bias in Gulf Stream position, which tends to be too northerly in CM2.1 (Saba et al., 2016), may have decreased prediction skill in the Southeast US shelf LME. Moreover, SST predictability in coastal regions remains limited by the inability of current multi-annual prediction systems to represent fine-scale shelf processes, such as upwelling and coastal wave dynamics (Jacox et al., 2015), and fine-scale bottom topography (Saba et al., 2016). Higher resolution and improved representation of ocean and shelf processes may further enhance multi-annual SST predictability in LMEs. Furthermore, it should be stressed, that these results are from one model. Their robustness should be tested across multi-annual prediction systems. Operationalization of coastal scale multi-annual forecasts will require further testing with a multi-model ensemble as was done for the first real-time global multi-annual predictions in Smith et al. (2013). A multi-model ensemble may also reduce sampling uncertainty and increase predictability, as was observed for seasonal predictions of SST in LMEs (Hervieux et al., 2017).
Our work demonstrates that current multi-annual SST predictions, when assessed as anomalies relative to conditions over the past 50 years, have significant skill in many coastal ecosystems, particularly when averaged over the next three or ten years. This presents opportunities for fisheries managers, which are required to produce multi-annual forecasts of fish stock biomass. Current management decisions are based on the scope of past variability. Integration of more precise forecasts of environmental conditions into fisheries models may reduce bias in fisheries projections, provide warning of changes in fish productivity, and produce better estimates of the rebuilding potential of overfished stocks (Pershing et al., 2015; Miller et al., 2016; Tommasi et al., 2017a). Fisheries decisions would also greatly benefit from the inclusion, in addition to SST, of multi-annual predictions of other fishery-relevant variables such as bottom temperature or primary productivity (e.g. Séférian et al., 2014).
While promising, it needs to be stressed that retrospective forecast skill represents likely, not actual, future forecast skill. In particular, the hindcasts presented here included prescribed forcing from stratospheric aerosols resulting from large volcanic eruptions (such as those of El Chichón in 1982 and Pinatubo in 1991). Eruptions such as these are not presently predictable on multi-year timescales, so real-time forecasts would not be able to forecast the impact of unpredictable volcanoes. While van Oldenborgh et al. (2012), in an assessment of the relative impacts of volcanic aerosols on retrospective SST forecast skill, showed that forcing from volcanos provides only a small contribution to multi-annual SST predictability relative to the greenhouse-gas forced trend, an evaluation of real-time forecast skill (e.g., Smith et al., 2013) at an LME scale would increase condifence in the reliability of the predictive skill here presented. Development of such real-time, operational multi-annual forecast capabilities would also be required for continued use of such forecasts into fisheries decisions. Furthermore, the observed SST predictability at multi-annual scales relative to the 50-year reference stems from past and ongoing anthropogenic climate change. Thus, while there is cause for optimism regarding the utility of multi-annual predictions to the fishery sector in adapting to this warming trend, this optimism rests within the reality of strong multi-annual change.
DT and CS designed the research, DT analyzed the data, and DT, CS, MA, XY, AR, and GV wrote the paper.
The authors declare that the research was conducted in the absence of any commercial or financial relationships that could be construed as a potential conflict of interest.
Many thanks to Dr. Tom Delworth and Dr. Mitchell Bushuk for helpful comments during the internal review process. This work was funded by a National Atmospheric and Oceanic Administration (NOAA) Special Early-Stage and Experimental or Developmental grant with support from NOAA's office of Oceanic and Atmospheric Research and NOAA National Marine Fisheries Service Office of Science and Technology. Thank you to the NOAA Large Marine Ecosystem Program for providing the image for Figure 1. No permission was required from the copyright holder for the preproduction of this image. The contents in this manuscript are solely the opinions of the authors and do not constitute a statement of policy, decision, or position on behalf of the NOAA or the US Government.
The Supplementary Material for this article can be found online at: http://journal.frontiersin.org/article/10.3389/fmars.2017.00201/full#supplementary-material
Barrier, N., Deshayes, J., Treguier, A., and Cassou, C. (2015). Heat Budget in the North Atlantic Subpolar Gyre: impacts of atmospheric weather regimes on the 1995 warming event. Prog. Oceanogr. 130, 75–90. doi: 10.1016/j.pocean.2014.10.001
Beaugrand, G., Brander, K. M., Lindley, J. A., Souissi, S., and Reid, P. C. (2003). Plankton Effect on Cod Recruitment in the North Sea. Nature 426, 661–664. doi: 10.1038/nature02164
Becker, E., and van den Dool, H. (2016). Probabilistic Seasonal forecasts in the North American multimodel ensemble: a baseline skill assessment. J. Clim. 29, 3015–3026. doi: 10.1175/JCLI-D-14-00862.1
Belkin, I. M. (2009). Rapid warming of large marine ecosystems. Prog. Oceanogr. 81, 207–213. doi: 10.1016/j.pocean.2009.04.011
Boyer, T. P., Antonv, J. I., Baranova, O. K., Garcia, H. E., Johnson, D. R., Locarnini, R. A., et al. (2009). “World ocean database 2009,” in NOAA Atlas NESDIS 66, ed S. Levitus, (Washington, DC: U.S. Gov. Printing Office), 216.
Brander, K. M. (2003). What kinds of fish stock predictions do we need and what kinds of information will help us to make better predictions ? Sci. Mar. 67, 21–33. doi: 10.3989/scimar.2003.67s121
Brander, K. M. (2007). Global fish production and climate change. Proc. Natl. Acad. Sci. U.S.A. 104, 19709–19714. doi: 10.1073/pnas.0702059104
Brander, K. M. (2010). Impacts of climate change on fisheries. J. Mar. Syst. 79. 389–402. doi: 10.1016/j.jmarsys.2008.12.015
Chhak, K. C., Di Lorenzo, E., Schneider, N., and Cummins, P. F. (2009). Forcing of Low-Frequency Ocean Variability in the Northeast Pacific. J. Clim. 22, 1255–1276. doi: 10.1175/2008JCLI2639.1
Corti, S., Palmer, T., Balmaseda, M., Weisheimer, A., Drijfhout, S., Dunstone, N., et al. (2015). Impact of initial conditions versus external forcing in decadal climate predictions: a sensitivity experiment. J. Clim. 28, 4454–4470. doi: 10.1175/JCLI-D-14-00671.1
Costanza, R., d'Arge, R., de Groot, R., Farber, S., Grasso, M., Hannon, B., et al. (1997). The Value of the World's Ecosystem services and natural capital. Nature 387, 253–260. doi: 10.1038/387253a0
CLIVAR (2001). Current Status of ENSO Forecast Skill. Report to the CLIVAR Working Group on Seasonal to Inter Annual Prediction. eds B. P. Kirtman, J. Shukla, M. Balmaseda, N. Graham, C. Penland, Y. Xue, S. Zebiak, International CLIVAR Project Office, CLIVAR Publication Series No. 56. Available online at: https://eprints.soton.ac.uk/44128/1/nino3.pdf
CLIVAR (2011). “Data and Bias Correction for Decadal Climate Predictions,” in International CLIVAR Project Office, CLIVAR Publication Series No. 150. Available online at: https://eprints.soton.ac.uk/171975/1/ICPO150_Bias.pdf
Cushing, D. H., and Dickson, R. R. (1976). The biological response in the sea to climatic changes. Adv. Mar. Biol. 1, 1–122.
Delworth, T. L., Broccoli, A. J., Rosati, A., Stouffer, R. J., Balaji, V., Beesley, J. A., et al. (2006). GFDL's CM2 global coupled climate models. part i: formulation and simulation characteristics. J. Climate 19, 643–674. doi: 10.1175/JCLI3629.1
Delworth, T. L., Manabe, S., and Stouffer, R. J. (1997). Multidecadal climate variability in the Greenland Seas and surrounding regions: a coupled model simulation. Geophys. Res. Let. 24, 257–260. doi: 10.1029/96GL03927
Doblas-Reyes, F. J., Andreu-Burillo, I., Chikamoto, Y., García-Serrano, J., Guemas, V., Kimoto, M., et al. (2013). Initialized near-term regional climate change prediction. Nat. Commun. 4:1715. doi: 10.1038/ncomms2704
Dorn, M. W. (1992). Detecting environmental covariates of pacific whiting merluccius productus growth using a growth-increment regression model. Fishery Bull. 90, 260–275.
Drinkwater, K. F., Beaugrand, G., Kaeriyama, M., Kim, S., Ottersen, G., Perry, R., et al. (2010). On the processes linking climate to ecosystem changes. J. Mar. Syst. 79, 374–388. doi: 10.1016/j.jmarsys.2008.12.014
Ellertsen, B., Fossum, P., Solemdal, P., and Sundby, S. (1989). Relation between temperature and survival of eggs and 1st-feeding larvae of northeast arctic cod (Gadus Morgue L). Rapports et Procès-Verbaux Des Reunions-Conseil International Pour l'Exploration de La Mer 191, 209–219.
García-Serrano, J., and Doblas-Reyes, F. J. (2012). On the assessment of near-surface global temperature and North Atlantic Multi-decadal variability in the ENSEMBLES decadal hindcast. Clim. Dyn. 39, 2025–2040. doi: 10.1007/s00382-012-1413-1
Hobday, A. J., Spillman, C. M., Eveson, J. P., and Hartog, J. R. (2016). Seasonal forecasting for decision support in marine fisheries and aquaculture. Fish. Oceanogr. 25, 45–56. doi: 10.1111/fog.12083
Hunt, G. L., Coyle, K. O., Eisner, L. B., Farley, E. V., Heintz, R. A., Mueter, F., et al. (2011). Climate impacts on eastern bering sea foodwebs: a synthesis of new data and an assessment of the oscillating control hypothesis. ICES J. Mar. Sci. 68, 1230–1243. doi: 10.1093/icesjms/fsr036
Hervieux, G., Alexander, M. A., Stock, C. A., Jacox, M. G., Pegion, K., Becker, E., et al. (2017). More reliable coastal SST forecasts from the North American multimodel ensemble. Clim. Dyn. doi: 10.1007/s00382-017-3652-7. [Epub ahead of print].
Jacox, M. G., Fiechter, J., Moore, A. M., and Edwards, C. A. (2015). ENSO and the california current coastal upwelling response. J. Geophys. Res. 120, 1691–1702. doi: 10.1002/2014jc010650
Jin, E. K., Kinter, J. L. III., Wang, B., Park, C-K., Kang, I-S., Kirtman, B. P., et al. (2008). Current status of ENSO prediction skill in coupled ocean–atmosphere models. Clim. Dyn. 31, 647–664. doi: 10.1007/s00382-008-0397-3
Johnstone, J. A., and Mantua, N. J. (2014). Atmospheric controls on Northeast Pacific Temperature Variability and Change, 1900–2012. Proc. Natl. Acad. Sci. U.S.A. 111, 14360–14365. doi: 10.1073/pnas.1318371111
Kalnay, E., Kanamitsu, M., Kistler, R., Collins, W., Deaven, D., Gandin, L., et al. (1996). The NCEP/NCAR 40-Year Reanalysis Project. Bull. Am. Meteorol. Soc. 77, 437–471. doi: 10.1175/1520-0477(1996)077<0437:TNYRP>2.0.CO;2
Karspeck, A., Yeager, S., Danabasoglu, G., and Teng, H. (2015). An Evaluation of experimental decadal predictions using CCSM4. Clim. Dyn. 44, 907–923. doi: 10.1007/s00382-014-2212-7
Keenlyside, N. S., Latif, M., Jungclaus, J., Kornblueh, L., and Roeckner, E. (2008). Advancing decadal-scale climate prediction in the North Atlantic sector. Nature 453, 84–88. doi: 10.1038/nature06921
Kristiansen, T., Drinkwater, K. F., Lough, R. G., and Sundby, S. (2011). Recruitment variability in North Atlantic Cod and match-mismatch dynamics. PLoS ONE 6:e17456. doi: 10.1371/journal.pone.0017456
Lehodey, P., Alheit, J., Barange, M., Baumgartner, T., Beaugrand, G., Drinkwater, K., et al. (2006). Climate variability, fish, and fisheries. J. Clim. 19, 5009–5030. doi: 10.1175/JCLI3898.1
Lindegren, M., and Checkley, D. M. (2013). Temperature dependence of pacific sardine (Sardinops Sagax) recruitment in the California current ecosystem revisited and revised. Can. J. Fish. Aquat. Sci. 70, 245–252. doi: 10.1139/cjfas-2012-0211
Ludescher, J., Gozolchiani, A., Bogachev, M. I., Bunde, A., Havlin, S., Ernst, W. G., et al. (2014). Very early warning of next El Niño. Proc. Natl. Acad. Sci. U.S.A. 111, 2064–2066. doi: 10.1073/pnas.1323058111
Mason, S. J. (2008). Understanding forecast verification statistics. Meteorol. Appl. 15, 31–40. doi: 10.1002/met.51
Matei, D., Pohlmann, H., Jungclaus, J., Müller, W., Haak, H., and Marotzke, J. (2012). Two tales of initializing decadal climate prediction experiments with the ECHAM5/MPI-OM model. J. Clim. 25, 8502–8523. doi: 10.1175/JCLI-D-11-00633.1
Meehl, G. A., Goddard, L., Boer, G., Burgman, R., Branstator, G., Cassou, C., et al. (2014). Decadal climate prediction an update from the trenches. Bull. Am. Meteorol. Soc. 95, 243–267. doi: 10.1175/BAMS-D-12-00241.1
Meehl, G. A., Goddard, L., Murphy, J., Stouffer, R., J., Boer, G., Danabasoglu, G., et al. (2009). Decadal prediction: can it be skillful? Bull. Am. Meteorol. Soc. 90, 1467–1485. doi: 10.1175/2009BAMS2778.1
Meinshausen, M., Smith, S. J., Calvin, K., Daniel, J. S., Kainuma, M. L. T., Lamarqueet, J.-F., et al. (2011). The RCP greenhouse gas concentrations and their extensions from 1765 to 2300. Clim. Change 109, 213–241. doi: 10.1007/s10584-011-0156-z
Miller, T. J., Hare, J. A., and Alade, L. A. (2016). A state-space approach to incorporating environmental effects on recruitment in an age-structured assessment model with an application to Southern New England yellowtail flounder. Can. J. Fish. Aquat. Sci. 73, 1261–1270. doi: 10.1139/cjfas-2015-0339
Msadek, R., Delworth, T., L., Rosati, A., Anderson, W., Vecchi, G., Chang, Y., S., et al. (2014). Predicting a decadal shift in North Atlantic Climate variability using the GFDL forecast system. J. Clim. 27, 6472–6496. doi: 10.1175/JCLI-D-13-00476.1
Mueter, F. J., Bond, N. A., Ianelli, J. N., and Hollowed, A. B. (2011). Expected declines in recruitment of walleye pollock (Theragra Chalcogramma) in the Eastern bering sea under future climate change. ICES J. Mar. Sci. 68, 1284–1296. doi: 10.1093/icesjms/fsr022
Mueter, F. J., Peterman, R. M., and Pyper, B. J. (2002). Opposite Effects of ocean temperature on survival rates of 120 stocks of Pacific Salmon (Oncorhynchus Spp.) in Northern and Southern Areas. Can. J. Fish. Aquat. Sci. 59, 456–463. doi: 10.1139/f02-020
Müller, W. A., Pohlmann, H., Sienz, F., and Smith, D. (2014). Decadal Climate predictions for the period 1901-2010 with a coupled climate model. Geophys. Res. Lett. 41, 2100–2107. doi: 10.1002/2014GL059259
NRC (2014). Evaluating the Effectiveness of Fish Stock Rebuilding Plans in the United States. Washington, DC: The National Academies Press.
Nye, J. A., Link, J. S., Hare, J. A., and Overholtz, W. J. (2009). Changing spatial distribution of fish stocks in relation to climate and population size on the northeast united states continental shelf. Mar. Ecol. Prog. Ser. 393, 111–129. doi: 10.3354/meps08220
Ottersen, G., Kim, S., Huse, G., Polovina, J. J., and Stenseth, N. C. (2010). Major pathways by which climate may force marine fish populations. J. Mar. Syst. 79, 343–360. doi: 10.1016/j.jmarsys.2008.12.013
Palmer, T. N., Doblas-Reyes, F. J., Hagedorn, R., Alessandri, A., Gualdi, S., Andersen, U., et al. (2004). Development of a European Multimodal Ensemble System for Seasonal-to-Interannual Prediction (DEMETER). Bull. Am. Meteorol. Soc. 85, 853–872. doi: 10.1175/BAMS-85-6-853
Perry, A. L., Low, P. J., Ellis, J. R., and Reynolds, J. D. (2005). Climate change and distribution shifts in marine fishes. Science 308, 1912–1915. doi: 10.1126/science.1111322
Pershing, A. J., Alexander, M. A., Hernandez, C. M., Kerr, L. A., Le Bris, A., Mills, K. E., et al. (2015). Slow adaptation in the face of rapid warming leads to collapse of the gulf of maine cod fishery. Science 350, 809–812. doi: 10.1126/science.aac9819
Peterman, R. M., Pyper, B. J., Lapointe, M. F., Adkison, M. D., and Walters, C. J. (1998). Patterns of covariation in survival rates of British Columbian and Alaskan Sockeye Salmon (Oncorhynchus Nerka) Stocks. Can. J. Fish. Aquat. Sci. 55, 2503–2517. doi: 10.1139/f98-179
Pinsky, M. L., Worm, B., Fogarty, M. J., Sarmiento, J. L., and Levin, S. A. (2013). Marine taxa track local climate velocities. Science 341, 1239–1242. doi: 10.1126/science.1239352
Pohlmann, H., Jungclaus, J. H., Köhl, A., Stammer, D., and Marotzke, J. (2009). Initializing decadal climate predictions with the GECCO oceanic synthesis: effects on the North Atlantic. J. Clim. 22, 3926–3938. doi: 10.1175/2009JCLI2535.1
Robson, J. I., Sutton, R. T., Lohmann, K., Smith, D., and Palmer, M., D. (2012a). Causes of the rapid warming of the North Atlantic Ocean in the Mid-1990s. J. Clim. 25, 4116–4134. doi: 10.1175/JCLI-D-11-00443.1
Robson, J. I., Sutton, R. T., and Smith, D. M. (2012b). Initialized decadal predictions of the rapid warming of the North Atlantic Ocean in the Mid 1990s. Geophys. Res. Lett. 39:L19713. doi: 10.1029/2012gl053370
Robson, J. I., Sutton, R. T., and Smith, D. (2014). Decadal Predictions of the cooling and freshening of the North Atlantic in the 1960s and the role of Ocean Circulation. Clim. Dyn. 42, 2353–2365. doi: 10.1007/s00382-014-2115-7
Saba, V. S., Griffies, S. M., Anderson, W. G., Winton, M., Alexander, M. A., Delworth, T. L., et al. (2016). Enhanced warming of the Northwest Atlantic Ocean under climate change. J. Geophys. Res. 121, 118–132. doi: 10.1002/2015jc011346
Séférian, R., Bopp, L., Gehlen, M., Swingedouw, D., Mignot, J., Guilyardi, E., et al. (2014). Multiyear predictability of tropical marine productivity. Proc. Natl. Acad. Sci. U.S.A. 111, 11646–11651. doi: 10.1073/pnas.1315855111
Sharp, G. D. (1987). Climate and fisheries: cause and effect or managing the long and short of It All. South Afr. J. Mar. Sci. 5, 811–838. doi: 10.2989/025776187784522414
Sherman, K. (2014). Adaptive management institutions at the regional level: the case of large marine ecosystems. Ocean Coast. Manag. 90, 38–49. doi: 10.1016/j.ocecoaman.2013.06.008
Smith, D. M., Cusack, S., Colman, A. W., Folland, C. K., Harris, G. R., and Murphy, J. M. (2007). Improved surface temperature prediction for the coming decade from a global climate model. Science 317, 796–799. doi: 10.1126/science.1139540
Smith, D. M., Scaife, A. A., Boer, G. J., Caian, M., Doblas-Reyes, F. J., Guemas, V., et al. (2013). Real-time multi-model decadal climate predictions. Clim. Dyn. 41, 2875–2888. doi: 10.1007/s00382-012-1600-0
Spillman, C. M., Hartog, J. R., Hobday, A. J., and Hudson, D. (2015). Predicting environmental drivers for prawn aquaculture production to aid improved farm management. Aquaculture 447, 56–65. doi: 10.1016/j.aquaculture.2015.02.008
Spillman, C. M., and Hobday, A. J. (2014). Dynamical seasonal ocean forecasts to aid salmon farm management in a climate hotspot. Clim. Risk Manag. 1, 25–38. doi: 10.1016/j.crm.2013.12.001
Stock, C. A., Alexander, M. A., Bond, N. A., Brander, K. M., Cheung, W. W. L., Curchitser, E. N., et al. (2011). On the use of IPCC-class models to assess the impact of climate on living marine resources. Prog. Oceanogr. 88, 1–27. doi: 10.1016/j.pocean.2010.09.001
Stock, C. A., John, J. G., Rykaczewski, R. R., Asch, R. G., Cheung, W. W. L., et al. (2017). Reconciling fisheries catch and ocean productivity. Proc. Natl. Acad. Sci. U.S.A. 114, E1441–E1449. doi: 10.1073/pnas.1610238114
Stock, C. A., Pegion, K., Vecchi, G. A., Alexander, M. A., Tommasi, D., Bond, N. A., et al. (2015). Seasonal sea surface temperature anomaly prediction for coastal ecosystems. Prog. Oceanogr. 137, 219–236. doi: 10.1016/j.pocean.2015.06.007
Sullivan, M. C., Cowen, R. K., and Steves, B. P. (2005). Evidence for Atmosphere-ocean forcing of yellowtail flounder (Limanda Ferruginea) recruitment in the middle atlantic bight. Fish. Oceanogr. 14, 386–399. doi: 10.1111/j.1365-2419.2005.00343.x
Tippett, M. K., Barnston, A. G., and Li, S. (2012). Performance of recent multimodel ENSO forecasts. J. Appl. Meteorol. Climatol. 51, 637–654. doi: 10.1175/JAMC-D-11-093.1
Tommasi, D., Stock, C. A., Hobday, A. J., Methot, R., Kaplan, I. C., Eveson, J. P., et al. (2017a). Managing living marine resources in a dynamic environment: the role of seasonal to decadal climate forecasts. Prog. Oceanogr. 152, 15–49. doi: 10.1016/j.pocean.2016.12.011
Tommasi, D., Stock, C. A., Pegion, K., Vecchi, G. A., Methot, R. D., Checkley, D. M., et al. (2017b). Improved management of small pelagic fisheries through seasonal climate prediction. Ecol. Appl. 27, 378–388. doi: 10.1002/eap.1458
van Oldenborgh, G. J., Doblas-Reyes, F. J., Wouters, B., and Hazeleger, W. (2012). Decadal prediction skill in a multi-model ensemble. Clim. Dyn. 38, 1263–1280. doi: 10.1007/s00382-012-1313-4
Yang, X., Rosati, A., Zhang, S., Delworth, T. L., Gudgel, R. G., Zhang, R., Vecchi, G., et al. (2013). A predictable AMO-like Pattern in the GFDL fully coupled ensemble initialization and decadal forecasting system. J. Clim. 26, 650–661. doi: 10.1175/JCLI-D-12-00231.1
Yeager, S., Karspeck, A., Danabasoglu, G., Tribbia, J., and Teng, H. (2012). A decadal prediction case study: late twentieth-century north atlantic ocean heat content. J. Clim. 25, 5173–5189. doi: 10.1175/JCLI-D-11-00595.1
Zhang, S., Harrison, M. J., Rosati, A., Wittenberg, A., Zhang, S., and Wittenberg, A. (2007). System design and evaluation of coupled ensemble data assimilation for global oceanic climate studies. Monthly Weather Rev. 135, 3541–3564. doi: 10.1175/MWR3466.1
Keywords: decadal predictions, multi-annual predictions, climate forecasts, large marine ecosystems, fisheries, probabilistic forecasts, sea-surface temperature predictions
Citation: Tommasi D, Stock CA, Alexander MA, Yang X, Rosati A and Vecchi GA (2017) Multi-Annual Climate Predictions for Fisheries: An Assessment of Skill of Sea Surface Temperature Forecasts for Large Marine Ecosystems. Front. Mar. Sci. 4:201. doi: 10.3389/fmars.2017.00201
Received: 01 April 2017; Accepted: 12 June 2017;
Published: 30 June 2017.
Edited by:
Corinna Schrum, University of Bergen, NorwayReviewed by:
Heather Cannaby, National Oceanography Centre Liverpool, United KingdomCopyright © 2017 Tommasi, Stock, Alexander, Yang, Rosati and Vecchi. This is an open-access article distributed under the terms of the Creative Commons Attribution License (CC BY). The use, distribution or reproduction in other forums is permitted, provided the original author(s) or licensor are credited and that the original publication in this journal is cited, in accordance with accepted academic practice. No use, distribution or reproduction is permitted which does not comply with these terms.
*Correspondence: Desiree Tommasi, ZGVzaXJlZS50b21tYXNpQG5vYWEuZ292
Disclaimer: All claims expressed in this article are solely those of the authors and do not necessarily represent those of their affiliated organizations, or those of the publisher, the editors and the reviewers. Any product that may be evaluated in this article or claim that may be made by its manufacturer is not guaranteed or endorsed by the publisher.
Research integrity at Frontiers
Learn more about the work of our research integrity team to safeguard the quality of each article we publish.