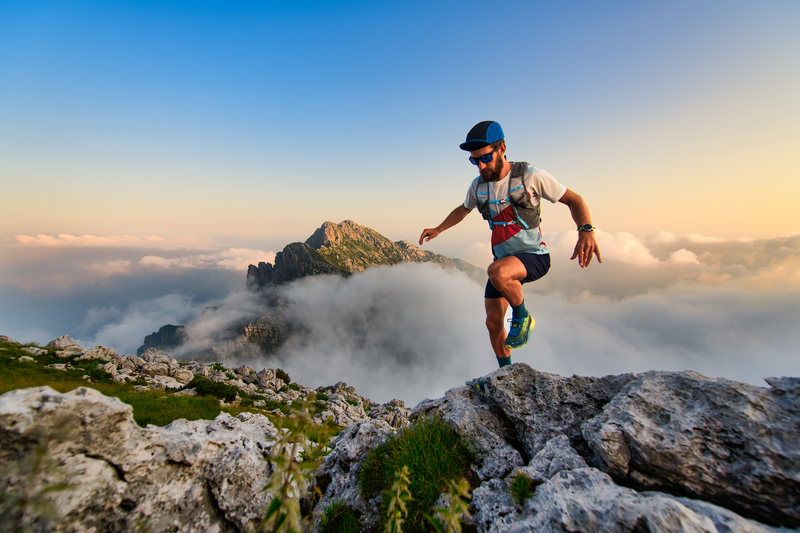
94% of researchers rate our articles as excellent or good
Learn more about the work of our research integrity team to safeguard the quality of each article we publish.
Find out more
REVIEW article
Front. Mar. Sci. , 09 May 2017
Sec. Marine Ecosystem Ecology
Volume 4 - 2017 | https://doi.org/10.3389/fmars.2017.00107
This article is part of the Research Topic Horizon Scan 2017: Emerging Issues in Marine Science View all 12 articles
Many ocean policies mandate integrated, ecosystem-based approaches to marine monitoring, driving a global need for efficient, low-cost bioindicators of marine ecological quality. Most traditional methods to assess biological quality rely on specialized expertise to provide visual identification of a limited set of specific taxonomic groups, a time-consuming process that can provide a narrow view of ecological status. In addition, microbial assemblages drive food webs but are not amenable to visual inspection and thus are largely excluded from detailed inventory. Molecular-based assessments of biodiversity and ecosystem function offer advantages over traditional methods and are increasingly being generated for a suite of taxa using a “microbes to mammals” or “barcodes to biomes” approach. Progress in these efforts coupled with continued improvements in high-throughput sequencing and bioinformatics pave the way for sequence data to be employed in formal integrated ecosystem evaluation, including food web assessments, as called for in the European Union Marine Strategy Framework Directive. DNA sequencing of bioindicators, both traditional (e.g., benthic macroinvertebrates, ichthyoplankton) and emerging (e.g., microbial assemblages, fish via eDNA), promises to improve assessment of marine biological quality by increasing the breadth, depth, and throughput of information and by reducing costs and reliance on specialized taxonomic expertise.
Marine and coastal systems provide a variety of important ecosystem services, such as food, recreation, employment, medicine, and regulation of waste, disease, and climate (Liquete et al., 2013). However, marine ecosystems and transitional waters (e.g., coastal areas, estuaries, lagoons, fjords) are increasingly stressed by multiple and often interconnected factors, such as overexploitation, chemical and nutrient pollution, pathogens, harmful algae, and hypoxia. With an estimated two-thirds of the human population living in or near coastal areas (Millennium Ecosystem Assessment, 2005), coastal population growth contributes ecosystem pressure, with virtually no marine areas unaffected by human influence (Halpern et al., 2008). In addition, sources of degradation such as elevated CO2 and temperature threaten ecosystem integrity and the capacity for marine ecosystems to remain productive (WHCEQ, 2010; NOC, 2013; Rogers, 2013; Halpern et al., 2015).
To maintain ecosystem services, many countries develop legal and policy frameworks to guide sustainable use of marine resources (Pereira et al., 2013). Examples include the United Nations Convention on the Law of the Sea, Australia's Oceans Policy, the Canada Oceans Act and Oceans Strategy, the United States Oceans Act of 2000 and National Ocean Policy Implementation Plan, the European Union Marine Strategy Framework Directive (MSFD), and the South African National Water Act. A main objective of such policies is maintenance of good ecological status (GES) in marine waters, habitats, and resources using integrated or “holistic” approaches (Borja et al., 2008, 2016; Karsenti et al., 2011; Duffy et al., 2013; Danovaro et al., 2016). For example, an Ecosystem-Based Management (EBM) approach to marine resources considers a suite of natural physical, chemical, biological, geographic, and climatic factors in context of anthropogenic activities and impacts. The goal is to protect and maintain natural ecological function while delivering ecosystem services and societal benefits (Levin et al., 2009; Elliott, 2011).
Marine monitoring and impact assessment programs are developed to respond to sustainability requirements. Such programs may evaluate marine ecological quality to inform management actions, such as establishment of harvest guidelines, habitat and species conservation plans, and setting of requirements and practices to minimize pollutants and invasive species. In many cases, long-term monitoring programs evaluate the demographic status and trends of various marine populations (Borja et al., 2010 and references therein). Holistic approaches require integrated monitoring and assessment of both abiotic and biotic parameters, including multiple species (Arkema et al., 2006; Day et al., 2008; Curtin and Prellezo, 2010; Möllmann et al., 2014) and, ideally, multiple trophic levels to capture Biodiversity and Ecosystem Function (BEF) relationships (Strong et al., 2015).
Most evaluations of marine ecological status rely on biological quality element (BQE) assessments, such as monitoring of invertebrates, fishes, or phytoplankton. Assessment of BQE populations and/or ecological relationships can be translated into straightforward classifications of ecosystem status (e.g., report cards) and provided to stakeholders, management authorities, and policy makers. However, traditional BQE monitoring poses a number of drawbacks, as outlined in Table 1.
One drawback of traditional BQE assessments is reliance on morphological taxonomy. This causes a bottleneck in sample throughput because manual sorting and visual identification is labor-intensive and slow. Identification demands a high degree of specialized taxonomic knowledge, such expertise is required for each BQE target separately, and juvenile and cryptic species can nonetheless be misidentified (Bourlat et al., 2013; Aylagas et al., 2014, 2016; Pawlowski et al., 2014; Carugati et al., 2015; Thomsen and Willerslev, 2015; Bowers et al., 2016; Bucklin et al., 2016; Danovaro et al., 2016). Estimates suggest that between 24 and 98% of marine eukaryotic species are yet to be described (Leray and Knowlton, 2016). In addition, the quantification of early life stages for stock assessment purposes, for example ichthyoplankton eggs and larvae (Harada et al., 2015) may not accurately predict life history or therefore the future adult population, which is the target of management action (Lewis et al., 2016).
The inability to inventory microbial assemblages is another drawback of typical monitoring approaches. Microbial species dominate the ocean numerically and the majority of marine primary production is microbial in origin, produced by cyanobacteria and microalgae (Duarte and Cebrian, 1996; DeLong, 2009; Amaral-Zettler et al., 2010). Microorganisms may act as sentinels of system ecological status because they respond rapidly to natural and anthropogenic environmental pressures in terms of diversity, physiology, and function (Mock and Kirkham, 2012; Nogales et al., 2011; Mock et al., 2015). They hold promise as ecosystem indicators with regard to measures of biodiversity, toxic species, pathogens, and metabolic properties that indicate ecosystem health, such as biodegradation capacity and resistance to metals and antibiotics (Ininbergs et al., 2015; Tan et al., 2015; Caruso et al., 2016). The ocean microbiome, an assemblage of bacteria, archaea, microeukaryotes, and viruses (Stulberg et al., 2016), is the heart of marine food webs. Although the need for food web assessments in marine ecosystem status is recognized (Zampoukas et al., 2014; Caruso et al., 2016), microbes are not readily amenable to visual identification (Bowers et al., 2016; Giner et al., 2016), hindering inclusion as a BQE in monitoring frameworks. Instead, marine monitoring programs are often restricted to gross measurements such as chlorophyll to capture this critical segment of the ecosystem.
Ecosystem assessments include a suite of biotic and abiotic measurements. The call for integrated monitoring recognizes that isolated BQE measures may not capture ecological status adequately (Table 1) and instead should include multiple taxonomic groups (e.g., animals, protists, bacteria) and life stages (e.g., for fish: eggs/larvae, juveniles, adults) (Aylagas et al., 2016; Thompson et al., 2016; Trivedi et al., 2016). However, harmonization is challenging (Simboura et al., 2005; Borja et al., 2014). The costs and complexity of integrated assessment can limit spatio-temporal scope and hinder the ability to realize an ecosystem approach (de Jonge et al., 2006; Vince et al., 2015).
From the perspective of cost and sample throughput, DNA sequencing offers a variety of advantages to marine monitoring and assessment programs compared to time-consuming visual inspection (Table 1). High-throughput sequencing allows faster and more accurate species identification and decreases dependence on morphological taxonomic expertise (Bourlat et al., 2013; Aylagas et al., 2014). Advantages extend to taxa traditionally monitored in marine assessment programs, such as invertebrates and fish, investigated either as individuals or assemblages (Ardura et al., 2013; Bourlat et al., 2013; Carugati et al., 2015; Harada et al., 2015; Tan et al., 2015; Zimmermann et al., 2015; Aylagas et al., 2016; Borja et al., 2016; Caruso et al., 2016; Danovaro et al., 2016; Lewis et al., 2016; Thompson et al., 2016). Information about higher trophic levels also can be gleaned from DNA extracted from filtered seawater via capture of sloughed or excreted cells, rather than direct extraction from tissue (Foote et al., 2012; Kelly et al., 2014; Kelly, 2016). Such work is buttressed by genomic-based studies which continue to advance, in part, by increased access to and application of DNA sequencing technologies (Cammen et al., 2016; Leslie and Morin, 2016). Declining costs encourage sequencing as an alternative to traditional biodiversity monitoring (Bourlat et al., 2013) and supports continued advances in conservation biology (Wallace et al., 2010; Hancock-Hanser et al., 2013).
Although information derived from DNA sequencing is yet to be formally included in current marine status assessment programs (Bourlat et al., 2013), there is widespread recognition of the importance of this approach, and an increasing number of projects (Table 2) generate sequence-based biodiversity assessments. For example, molecular approaches were found to be more time-efficient compared to visual census (Yamamoto et al., 2017) and were successfully used to identify nonindigenous species in marine waters and to retrieve taxa from benthic samples that were not identified by morphological analysis (Zaiko et al., 2015; Aylagas et al., 2016). Progress moving molecular assessments into formal monitoring is being made as evidenced by adaptation of the AZTI Marine Biotic Index (AMBI), determined by traditional manual sorting and visual identification of benthic macroinvertebrates, to an index based on genetics (gAMBI) (Aylagas et al., 2014, 2016). Success with this approach was extended to bacterial assemblages (Aylagas et al., 2017).
DNA sequencing addresses the challenges of microbial assemblage inventory. Limitations of visual identification and culture methods drove microbial ecology to become an early adopter of molecular methods (Giovannoni et al., 1990; Handelsman, 2004; Venter et al., 2004; Rusch et al., 2007). Sequencing technology and bioinformatics revolutionized the study of marine biology, providing new insights into how the “hidden majority” (Rappé and Giovannoni, 2003) mediates cycles of carbon, nutrients, oxygen, metals, and toxins and responds to ecosystem change. The field is now sufficiently mature to be considered in routine marine monitoring efforts. For example, DNA sequencing could be used to monitor marine food webs, as explicitly called out in the EU Marine Strategy Framework Directive (MSFD) (Zampoukas et al., 2014). Examples of projects (Table 2) focused on microbial assemblages include Ocean Sampling Day (Kopf et al., 2015), the TARA Oceans project (Karsenti et al., 2011; Bork et al., 2015), the Earth Microbiome Project (Gilbert et al., 2014), the Marine Biodiversity Observing Network (Duffy et al., 2013; Muller-Karger et al., 2014), and Bioplatforms Australia—Marine Microbes (NCRIS, 2016).
Overall, DNA sequencing is a promising approach for integrated ecosystem assessments. Sequencing data can provide information about individuals, populations, and communities, and data can be used to understand stress responses and adaptation capacity. Such information can be distilled into ecosystem indicators and potentially integrated in ecosystem models aimed to inform people dependent on marine ecosystem services.
DNA sequencing and analysis approaches vary widely, with opportunities to serve a variety of marine monitoring applications. Terminology usage shifts as methods change (e.g., the evolution of long-read Sanger to short-read Illumina sequencing) and varies among applications, particularly between microbial/macrobial and aquatic/benthic specialties. For example, the term “metagenomics” is defined simply and broadly as the analysis of genetic materials obtained directly from environmental samples, including analysis of sequenced amplicon (Xu, 2015). Alternatively, this broad definition is rejected in favor of keeping terminology for amplicon sequencing separate (e.g., “metagenetics” or “metaprofiling”) rather than as a subset of metagenomics (Esposito and Kirschberg, 2014; Escobar-Zepeda et al., 2015; Mendoza et al., 2015; Creer et al., 2016). A similar situation exists for “environmental DNA” (eDNA) which is broadly defined in some cases (Pedersen et al., 2015). However, the term eDNA is increasingly used to connote animal DNA obtained indirectly from environmental samples rather than tissues per se (Bohmann et al., 2014; Kelly et al., 2014; Kelly, 2016), and that connotation is generally used here. Additional ambiguities arise from advances in sequencing technologies. For example, both Sanger (first generation) and later platforms such as Roche 454, SOLiD, and Illumina (second generation) may be referred to as next generation sequencing (NGS) in the literature, and both second and third-generation (PacBio, Ion Torrent, Oxford Nanopore) may be referred to as massively parallel or high-throughput sequencing (HTS). Other examples of terms with ambiguous usage include: conservation genomics (Garner et al., 2016), genetic fingerprinting, tag sequencing, targeted metagenomics, metabarcoding (Mendoza et al., 2015), and community analysis. With continued modifications to technology, expanding applications, and development of bioinformatic tools, terminology is expected to remain a challenge.
In generic terms, it is anticipated that DNA sequencing as a tool in routine marine monitoring will employ high-throughput sequencing coupled with a bioinformatic analysis pipeline. Sequencing may target whole genomes or specific regions that may be amplified by PCR prior to sequencing. DNA may be obtained from an individual, an assemblage of organisms (“meta” approach), or an assemblage of partial organisms (eDNA). For the purposes here, the focus is on high throughput, short-read sequencing of amplicons from macroeukaryotic assemblages (metabarcoding) or individuals (barcoding); amplicons from assemblages of maroeukaryotes obtained indirectly from the environment rather than from whole organisms or tissues (eDNA); amplicons from prokaryotic/microeukaryotic assemblages (metagenetics); and shotgun sequencing of prokaryotic/microeukaryotic assemblages (metagenomics). A variety of references regarding extraction (Cox and Goodwin, 2013; Hazen et al., 2013), amplification, and sequencing strategies for water, sediments, and tissues are available herein, and eDNA is also reviewed extensively elsewhere (Bohmann et al., 2014; Rees et al., 2014; Pedersen et al., 2015; Thomsen and Willerslev, 2015; Shelton et al., 2016); therefore, only a brief technical overview is provided here.
Sequencing of PCR-amplified marker genes is often employed to identify organisms (Patwardhan et al., 2014). This targeted sequencing approach can provide detection of species in relatively low abundance (DeSantis et al., 2006; Sogin et al., 2006; Hamady and Knight, 2009; Quast et al., 2013) and better depth of coverage compared to shotgun whole-genome approaches (Zhou et al., 2015). For example, amplification of a nuclear 16S rRNA gene segment is typical for taxonomic classification of marine prokaryotic assemblages (Klindworth et al., 2013). Amplification of 18S rRNA regions is used for a variety of communities, including phytoplankton and other microeukaryotes (Dunthorn et al., 2012; Hugerth et al., 2014; Johnson and Martiny, 2015; del Campo et al., 2016; Giner et al., 2016), and amplification of the 23S rRNA gene is used to classify organisms such as zooplankton (Hirai et al., 2015a; Bucklin et al., 2016). Amplification of mitochondrial DNA is used to identify an assortment of organisms, with cytochrome oxidase I (COI), cytochrome b, and mitochondrial 16S as examples of popularly employed target regions (Dauble et al., 2012; Pawlowski et al., 2014; Cowart et al., 2015; Guo et al., 2015; Harada et al., 2015; Johnson and Martiny, 2015; Aylagas et al., 2016; Bucklin et al., 2016; Creer et al., 2016; Leray and Knowlton, 2016; Thompson et al., 2016; Trivedi et al., 2016). Newer approaches are being used to identify fish (Miya et al., 2015) and marine mammals (Foote et al., 2012; Ma et al., 2016) from seawater samples.
In comparison to amplifying and sequencing a specific genomic region, shotgun metagenomic methods sequence broadly across entire genomes (Thomas et al., 2012; Escobar-Zepeda et al., 2015; Sharpton, 2014; Guo et al., 2016). In general, DNA extracted from a sample is fragmented, a DNA library is prepared (Head et al., 2014) using a limited number of PCR cycles or via PCR-free methods (Mendoza et al., 2015), and the assortment of random DNA fragments is sequenced. The resulting sequences can be compared directly against genomes of known taxa or can be assembled into longer segments (contigs) which can be further annotated for gene content, function, and taxonomic assignment (Segata et al., 2012; Teeling and Glöckner, 2012; Wood and Salzberg, 2014; Bengtsson-Palme et al., 2015). This approach provides a broad view because a variety of genes are detected, including those that encode metabolic enzymes and pathways. If the goal is to characterize the functional potential of an ecosystem, shotgun sequencing is desirable because whole-genome data provides information on potential metabolic function, in addition to taxonomic information (Handelsman, 2004; Thomas et al., 2012). Furthermore, shotgun sequencing is less affected by primer bias and chimeras compared to amplicon sequencing (Guo et al., 2016). However, obtaining adequate depth of coverage can be problematic (Zhou et al., 2015), motivating some studies to utilize both amplicon and whole-genome approaches (e.g., Ocean Sampling Day, Table 2).
Regardless of the DNA sequencing approach used, bioinformatics is critical to understand the information delivered by DNA sequencing efforts. A plethora of bioinformatic tools are available, with a complete review beyond the scope of this article. Many bioinformatic tools and platforms are now tailored to gene sequences derived from environmental samples, particularly for prokaryotic assemblages (Kanehisa et al., 2008; Meyer et al., 2008; Caporaso et al., 2010; Abubucker et al., 2012; Thomas et al., 2012; Kozich et al., 2013; Hunter et al., 2014). Furthermore, de-novo assembly is increasingly being used to derive nearly complete genomes of previously unknown ecologically important organisms from metagenomes (Garza and Dutilh, 2015; Hugerth et al., 2015). A growing number of assemblers (Boisvert et al., 2012; Peng et al., 2012) and binning tools (Alneberg et al., 2014; Imelfort et al., 2014; Nurk et al., 2017) are available that assemble short sequencing reads into larger contigs and subsequently combine them into bins based on, for example, sequence similarity (Teeling and Glöckner, 2012). In addition to standard biodiversity metrics such as alpha, beta, and gamma diversity (Escobar-Zepeda et al., 2015; Zhou et al., 2015), network analysis tools aid visualization of complex ecological relationships (Mitra et al., 2010; Hurwitz et al., 2014).
Marine microbes were among the first communities to be explored by DNA sequencing (Giovannoni et al., 1990; Venter et al., 2004). Sequencing was initially applied to prokaryotic and microeukaryotic communities in seawater with techniques developed for these whole organisms because traditional culture methods were inadequate to describe microbial biodiversity in water and soil (Rappé and Giovannoni, 2003; Handelsman, 2004). The Global Ocean Sampling expedition helped establish DNA sequencing as a fundamental tool in marine ecosystem research (Rusch et al., 2007; Dupont et al., 2015). Potential benefits of DNA sequencing of microbial communities to marine assessment programs include characterization of food webs, assessing responses to disruption and stress, and detection of sensitive, rare, threatened, toxic, or invasive taxa (Hulme, 2006; Kudela et al., 2010; Mock and Kirkham, 2012; Lindeque et al., 2013; Muller-Karger et al., 2014; Pawlowski et al., 2014; Chown et al., 2015; Mock et al., 2015; Tan et al., 2015; Zaiko et al., 2015; Bowers et al., 2016; Bucklin et al., 2016).
Sequencing of environmental samples such as seawater is now applied in a macrobial sense across a range of trophic levels (“microbes to mammals”) (Trivedi et al., 2016; Valentini et al., 2016), and approaches used to inventory individuals (Ardura et al., 2013; Harada et al., 2015; Thompson et al., 2016) are being applied to assemblages (Carugati et al., 2015; Zimmermann et al., 2015; Aylagas et al., 2016). Studies demonstrate DNA metabarcoding to be a reliable method for biodiversity assessment with potential for inferring biotic indices for marine ecosystem quality assessment (Aylagas et al., 2014; Pawlowski et al., 2014; Cowart et al., 2015; Elbrecht and Leese, 2015; Visco et al., 2015; Ferrera et al., 2016). Comparable results between molecular and traditional approaches are reported in a number of studies (Hirai et al., 2015a; Lejzerowicz et al., 2015; Aylagas et al., 2016, 2017; Valentini et al., 2016), suggesting that assessment programs that require manually intensive sorting and visual inspection will be among the first to formally integrate molecular techniques. For example, larval rockfish time-series are currently used to quantify spawning stock biomass off California, USA and are integral components of rockfish stock assessment (He et al., 2015). However, the utility of the traditional approach for counting rockfish larvae based on morphology is limited because only a few species can be visually identified to species. Recent research to improve stock assessment has genetically identified rockfish larvae collected off southern California and increased from 6 to 36 the number of species with time-series (Chen, 2017). The genetically-derived data is now being considered for integration into formal stock assessments.
Newer eDNA methods allow detection of higher trophic levels, including fish and mammals used to evaluate ecological status, from DNA extracted from sediment or filtered seawater. The eDNA approach promises information about bioindicators and commercially important or protected species without the need to collect tissue or trawl through sensitive habitats (Foote et al., 2012; Bohmann et al., 2014; Thomsen and Willerslev, 2015; Evans et al., 2016; Lacoursière-Roussel et al., 2016; Shelton et al., 2016). Some studies show promising results with regard to abundance estimates (Hänfling et al., 2016; Port et al., 2016), but others show significant differences between eDNA results and traditional tows for epibenthic macroinvertebrates (Kelly et al., 2017) and zooplankton (Hirai et al., 2015b), suggesting that these methods require further research.
DNA sequencing offers insight into ecosystem stress via changes in relative abundance or completeness of enzymatic pathways associated with chemical biodegradation or resistance. For example, a number of microorganisms carry genes that confer resistance to antibiotics or heavy metals. Increased exposure to anthropogenic chemicals promotes production and dissemination of these genes. Increases in the abundance of resistance genes and of integrons, genetic elements involved in horizontal gene transfer, are documented in polluted sediments (Thureborn et al., 2013). Resistance to multiple antibiotics is more frequent at polluted sites, and resistance to antibiotics and heavy metals can co-occur (Henriques et al., 2016). A number of studies demonstrate environmental gradients showing co-occurrence of anthropogenic inputs and gene occurrence in marine environments (Tacão et al., 2012; Chen et al., 2013; Thureborn et al., 2013), providing an opportunity to use individual gene markers (e.g., blaCTX-M, merA) or a full metagenomic profile to indicate pollution in ecological status assessments.
Disrupted ecosystems can also present shifts in particular taxa or overall biodiversity (Nogales et al., 2011; Mason et al., 2014; Mukherjee et al., 2017), opening the possibility to evaluate ecological status according to such metrics. Toward this goal, Aylagas et al. (2017) developed two composite indicators: (1) a sediment quality index that included organic matter content, redox potential, and metal, PAH and PCB concentrations; and (2) a bacterial index of environmental quality adapted from the AMBI. The indices were correlated, a promising result with regard to inclusion of prokaryotic assemblages into future assessment programs (Caruso et al., 2016). Furthermore, DNA sequencing approaches are used for public health applications related to human pathogens derived from fecal contamination (Tan et al., 2015; Staley and Sadowsky, 2016) and to investigate the impacts of anthropogenic stressors on the coral microbiome (Ziegler et al., 2016; Staley et al., 2017). Kelly et al. (2016) demonstrate that eDNA in seawater can be used not only for biodiversity measurement but also for assessing anthropogenic disturbance in coastal environments. In that study, sequencing of metazoan mitochondrial 16S DNA show greater diversity of sessile taxa in more urbanized sites. Higher diversity in a disturbed site also is reported for estuarine benthic eukaryotes using 18S rRNA metabarcoding (Chariton et al., 2015). In contrast, lower diversity of benthic foraminifera is reported for sites impacted by fish farming (Pawlowski et al., 2014). Such a combination of results suggests that a sole biodiversity index may be inadequate to evaluate ecosystem status and indicates that an AMBI-like approach, which weights taxa abundance by pollution tolerance, may be needed to convert taxonomic measures into indices of ecosystem function.
While DNA sequencing holds promise for marine assessment programs (Table 1), improvements are required to adapt these powerful tools to formal monitoring frameworks. One of the greatest needs, cutting across all aspects of the endeavor, is the need for standardization. Standardization is particularly critical for marine monitoring applications because long-term data set generation is a hallmark of such efforts. Standard methods and practices can be applied at every stage: study design; metadata collection and curation; sample collection and preservation; DNA extraction; inclusion of control samples; approaches to amplification, library preparation and sequencing; sequence quality control and other downstream processing; bioinformatic method documentation; and depositing of sequence results with appropriate metadata.
Standardization of metadata, a minimum common set of measurements to be recorded at the time of sample collection, is critical. Adequate metadata is required to integrate DNA sequencing information into marine ecosystem assessment programs, provide environmental context to sequence data, simplify annotation, facilitate data mining, and to allow comparisons between studies. Progress is being made to create standardized protocols (Field et al., 2008; Chain et al., 2009; Gilbert, 2015; Droege et al., 2016). Efforts that promote community collaboration and participation to produce standard practices include the MIxS standard from the Genomic Standards Consortium (GSC) (Yilmaz et al., 2011; ten Hoopen et al., 2015), the Environmental Ontology (Buttigieg et al., 2013, 2016), and data standards of the Global Genome Biodiversity Network (GGBN) (Droege et al., 2016). Despite widespread recognition of its importance and the realization that subsequent data curation is costly and time-consuming (ten Hoopen et al., 2016), compliance with metadata collection and curation standards is lacking. Gold standards with regard to data analysis, applied algorithms, and reference data sets comparable to those sought for the human medical space will improve the reliability and usability of results gained from marine sequencing studies. Recent sampling efforts such as the Ocean Sampling Day (Kopf et al., 2015), TARA Oceans project (Karsenti et al., 2011; Bork et al., 2015) and the Australian Marine Microbes Project (NCRIS, 2016) mark first steps to standardize sample preparation and sampling procedures for marine microbial ecology.
An adequate set of negative and positive control samples (e.g., mock communities, both DNA and whole-cell) are needed to track DNA preservation, extraction efficiency, contamination, and sequence errors, which can significantly affect results (Zhou et al., 2015; Allen et al., 2016; Mulcahy et al., 2016; Siegwald et al., 2017). Work initiated in the area of standardizing laboratory procedures includes much-needed development of reference materials and improved DNA extraction methods, as exemplified by establishment of the International Metagenomics and Microbiome Standards Alliance (IMMSA) (NIST, 2016). Controls used to assess artifacts and bias introduced by sample preparation, PCR, and sequencing are reviewed elsewhere (Pinto and Raskin, 2012; Elbrecht and Leese, 2015; Pedersen et al., 2015; Tan et al., 2015; Zhou et al., 2015; Aylagas et al., 2016; Danovaro et al., 2016) and should be used in conjunction with standard quality control pipelines for sequence quality, including chimeric removal (Smyth et al., 2010; Teeling and Glöckner, 2012; Zhou et al., 2014; Escobar-Zepeda et al., 2015; Jeon et al., 2015). Including mock communities in DNA sequencing efforts can assess technical issues such as incomplete DNA extraction or library preparation, PCR, and sequencing errors (Schirmer et al., 2015) and provide correction factors (Tan et al., 2015; Aylagas et al., 2016). Furthermore, simulated datasets can test the performance of both sequencing technology and analysis pipelines (Bonilla-Rosso, 2015), and bioinformatic tools can be used to mask technical variability (Leek et al., 2012).
The technical variation in sequence data is compounded by the considerable temporal and spatial biological variability in marine systems. Environmental heterogeneity is both natural (e.g., salinity gradients in transitional waters) and driven by proximity to anthropogenic influences. Study design should account for expected variability and include appropriate types and amounts of biological and technical replicates to ensure that meaningful comparisons and statistical analysis, suited to the question at hand, can be provided rather than ad-hoc sequencing based on available budget (Prosser, 2010; Knight et al., 2012; Pinto and Raskin, 2012; Thomas et al., 2012; Zhou et al., 2015). Greater sequencing coverage can aid the ability to differentiate environmental variability, with the balance of biological and technical replicates dependent on the study questions and community complexity (Teeling and Glöckner, 2012; Zhou et al., 2015).
A challenge to DNA sequencing of environmental samples is obtaining accurate taxonomic classification, particularly from short DNA sequence reads. The issue is exacerbated when reference genomes are lacking, such as for marine ecosystems and particularly for eukaryotic and viral components (Leray and Knowlton, 2016; Roux et al., 2016). Taxonomic mis-classification of reads impacts the accuracy of abundance estimates (Aylagas et al., 2016). Lack of intercalibration between morphological and molecular methods presents an additional issue, and transferability across taxa is a concern, particularly if the initial assessment used to establish the database is limited to one or two taxonomic groups (Kelly, 2016). Although comparable molecular and traditional approaches are reported (Lejzerowicz et al., 2015; Aylagas et al., 2016, 2017; Hänfling et al., 2016; Valentini et al., 2016), other studies show discrepancies (Cowart et al., 2015; Hirai et al., 2015b; Mohrbeck et al., 2015; Giner et al., 2016; Kelly et al., 2017); therefore, it has been advised to use both approaches in a complementary fashion rather than outright substitution of morphotaxonomic approaches (Borja et al., 2008; Pedersen et al., 2015; Thomsen and Willerslev, 2015). Although such an approach may deliver a holistic perspective of marine ecological status, it would not produce the desired effect of streamlining monitoring efforts, which is needed to transition research into practice (de Jonge et al., 2006).
Bioinformatic tools to aid microbial classification include similarity based approaches (Liu et al., 2011; Menzel et al., 2016), phylogenetic placement of sequences to known reference trees (Matsen et al., 2010), and using a combination of methods (Darling et al., 2014; Dröge et al., 2015). The reliance of current methods on known sequences, however, is a major drawback, as only a fraction of marine genomes (whole genomes or marker gene regions) are sequenced to date. It is worth noting that sequences obtained from metabarcoding efforts may provide value to ecosystem assessments even if reliable taxonomy cannot be assigned to them. A biodiversity index that does not require taxonomic assignment—a reference-free approach (Mendoza et al., 2015)—could in principle substitute for existing indices targeting specific species. It is noted that diversity in terms of operational taxonomic units (OTUs) vs. strict taxonomic assignment of sequences could satisfy requirements of the EU MSFD (Danovaro et al., 2016). Several bioinformatic tools are available for microbial sequences that could aid this goal (Eren et al., 2013; Callahan et al., 2016; Amir et al., 2017). For example, the Earth Microbiome Project (earthmicrobiome.org) employed Deblur (Amir et al., 2017) to deliver a set of unique sequences with single-nucleotide resolution, rather than representative sequences delivered by OTU clustering. This strategy enabled sequences to be tracked across a variety of studies and habitats and, coupled with environmental metadata, allowed global inferences regarding microbial community structure. Patterns were not dependent on taxonomic assignments, but because exact sequences were provided, taxonomy can later be assigned as reference databases improve. Such approaches can be extended from metagenetics to metagenomics and should continue to improve as longer sequence reads delivered from high-throughput platforms become more routine (Teeling and Glöckner, 2012).
Presence/absence detection appears sufficient for certain ecological quality assessment applications (Aylagas et al., 2016), although improvements in quantification would surely extend the utility of DNA sequencing for marine monitoring purposes. Some studies report adequate estimates of relative abundance (Aylagas et al., 2014, 2016; Elbrecht and Leese, 2015; Tan et al., 2015; Thomsen and Willerslev, 2015) even though read abundance does not necessarily provide a direct correlation to organism abundance because of issues that include variations in copy number, genome size, and growth condition. Bioinformatic solutions to the issue of quantification include estimation of organism abundance based on normalized read counts of clade-specific marker genes (Segata et al., 2012; Sohn et al., 2014), polymorphisms in universal markers (Luo et al., 2015), or accounting for incorrect taxonomic classification through probabilistic models (Lu et al., 2017). Differences in sequencing depth, i.e., the number of reads obtained from an environmental sample, significantly affects results (Rodriguez and Konstantinides, 2014) and can potentially render a study unable to detect low-abundance strains. In cases in which detection or abundance estimation is critical, amplicon sequencing with deep sequence coverage coupled with quantitative PCR (qPCR) may be a workable strategy.
The challenge that residual or ancient DNA poses to quantitation, particularly in benthic environments where DNA turnover is relatively slow, is reviewed extensively elsewhere (Bohmann et al., 2014; Pedersen et al., 2015; Thomsen and Willerslev, 2015). Recent studies in aquatic systems focus on DNA decay rates to better understand the potential of, and limits to, applying eDNA to marine monitoring and stock assessment (Sassoubre et al., 2016). In some cases, qPCR of eDNA is used to address the need for quantification (Laramie et al., 2015). In other cases, qPCR alone is suggested for abundance measurements of harmful algae in marine monitoring programs (Zamor et al., 2012). Overall, overcoming and/or circumventing issues that compromise abundance estimates is a research need that requires attention on all fronts—from the first steps of sample collection and DNA extraction, to routine generation of longer sequence reads, and development of better bioinformatic tools.
DNA sequences can be obtained cheaply and quickly; a decade ago a standard sequencing run returned a few thousand DNA base pairs. Now for the same cost, sequencers routinely generate hundreds of billions of base pairs in a single run. Despite the enormous opportunities posed by this technological revolution, the vast amount of data generated requires new solutions for data handling, storage, processing, documentation, visualization, and dissemination (Desai et al., 2012; Muir et al., 2016). This, in turn, drives a critical need (and current gap) for bioinformatic infrastructure and expertise. Adequate investment must be paid to obtain and maintain the tailored IT infrastructure needed to handle the increasing data volume and sophistication of processing. New developments such as cloud computing provide cost efficient solutions for decentralized storage and analysis of Big Data (Stein, 2010) while facilitating research collaborations. However, a common problem for sequencing projects is a dearth of experienced and skilled bioinformaticians. This is partly due to the rapid expansion of the field; the need has expanded faster than the ability to train scientists (Hughey and Karplus, 2003). In addition, the skill sets needed are diverse and project-dependent. The result is that many bioinformatic tools are executed by researchers without sufficient training and knowledge of the underlying algorithms. Choosing the correct pipeline is important (Siegwald et al., 2017), and competence in bioinformatics is required to choose the appropriate analysis software from the vast number of available tools. Despite the critical importance of bioinformatics expertise to sequencing studies, bioinformaticians can lack appropriate career and development opportunities (Chang, 2015). There is a need for institutions and funding bodies to address this gap and to create attractive career paths and salary models for current and future bioinformaticians. Meanwhile, education and training opportunities are being created to close the bioinformatic workforce gap (Edwards et al., 2013; Atwood et al., 2015), creating a fundamental difference between the expertise gap in bioinformatics and the one for traditional taxonomic identity (Table 1).
Although there is progress to be made, environmental sample DNA can now be routinely analyzed by high-throughput sequencing methods. Furthermore, there is growing ability to apply other ‘omic approaches to environmental samples, such as metatranscriptomic, proteomic, and epigenetic analyses. Sequencing approaches empower mechanistic understanding of ecosystem dynamics and integration into marine monitoring and assessment programs will allow “environmental intelligence” (who is there, what they do, and how they are impacted by changing conditions) to be gathered with scope and detail never before available. In addition to improving routine assessment methods that rely on sorting & visual inspection (e.g., benthic invertebrates, ichthyoplankton), sequencing can provide a detailed inventory of the microbial portion of the food web. Inclusion of this fundamental component should add value to marine monitoring programs, allowing holistic ecological assessment from both a taxonomic and functional perspective. Simultaneously, eDNA approaches offer the ability to inventory higher organisms from seawater samples. Moreover, molecular approaches are amenable to integration into automated in-situ or remotely operated platforms (Yamahara et al., 2015; Bowers et al., 2016), further opening the possibility to increase temporal or spatial sample coverage while realizing economies compared to ship or satellite sensing. Environmental managers seek to take advantage of DNA sequencing for environmental assessment and management given potential savings in labor costs, faster sample throughput, and the relative ease of integration across trophic levels. As research science makes progress in developing techniques, standardizing practices, and demonstrating efficacy for monitoring missions, increased integration of DNA sequencing into formal monitoring programs is expected.
KDG was responsible for overview of DNA sequencing as a tool applied to marine ecological status monitoring. TK and LRT contributed to bioinformatics portions of the review. BD, IC, and JCM contributed to comparisons between traditional and metagenomic biodiversity assessments and application to ecological indexes. ART contributed to eDNA and fisheries perspectives.
The authors declare that the research was conducted in the absence of any commercial or financial relationships that could be construed as a potential conflict of interest.
The reviewer KV and handling Editor declared their shared affiliation, and the handling Editor states that the process nevertheless met the standards of a fair and objective review.
The authors would like to thank to the Fundação para a Ciência e Tecnologia (FCT) for funding the research in the Marine and Environmental Sciences Centre (MARE) through the project UID/MAR/04292/2013. BD was supported by FCT through a postdoctoral grant (SFRH/BPD/115162/2016). Funding for LRT was provided by NOAA's Atlantic Oceanographic and Meteorological Laboratory (AOML) and the Mississippi State University/NOAA Northern Gulf Institute.
Abubucker, S., Segata, N., Goll, J., Schubert, A. M., Izard, J., Cantarel, B. L., et al. (2012). Metabolic reconstruction for metagenomic data and its application to the human microbiome. PLoS Comput. Biol. 8:e1002358. doi: 10.1371/journal.pcbi.1002358
Allen, H. K., Bayles, D. O., Looft, T., Trachsel, J., Bass, B. E., Alt, D. P., et al. (2016). Pipeline for amplifying and analyzing amplicons of the V1-V3 region of the 16S rRNA gene. BMC Res. Notes 9:380. doi: 10.1186/s13104-016-2172-6
Alneberg, J., Bjarnason, B. S., de Bruijn, I., Schirmer, M., Quick, J., Ijaz, U. Z., et al. (2014). Binning metagenomic contigs by coverage and composition. Nat. Methods 11, 1144–1146. doi: 10.1038/nmeth.3103
Amaral-Zettler, L., Artigas, L. F., Baross, J., Bharathi, L., Boetius, A., Chandramohan, D., et al. (2010). “A global census of marine microbes,” in Life in the World's Oceans: Diversity, Distribution and Abundance, ed A. D. McIntyre (Hoboken, NJ: Wiley-Blackwell), 221–245.
Amir, A., McDonald, D., Navas-Molina, J. A., Kopylova, E., Morton, J. T., Xu, Z. Z., et al. (2017). Deblur rapidly resolves single-nucleotide community sequence patterns. mSystems 2:e00191–16. doi: 10.1128/mSystems.00191-16
Ardura, A., Planes, S., and Garcia-Vazquez, E. (2013). Applications of DNA barcoding to fish landings: authentication and diversity assessment. ZooKeys 365, 49–65. doi: 10.3897/zookeys.365.6409
Arkema, K. K., Abramson, S. C., and Dewsbury, B. M. (2006). Marine ecosystem-based management: from characterization to implementation. Front. Ecol. Environ. 4, 525–532. doi: 10.1890/1540-9295(2006)4[525:MEMFCT]2.0.CO;2
Atwood, T. K., Bongcam-Rudloff, E., Brazas, M. E., Corpas, M., Gaudet, P., Lewitter, F., et al. (2015). GOBLET: The Global Organisation for Bioinformatics Learning, Education and Training. PLoS Comput Biol. 11:e1004143. doi: 10.1371/journal.pcbi.1004143
Aylagas, E., Borja, A., Irigoien, X., and Rodriguez-Ezpeleta, N. (2016). Benchmarking DNA metabarcoding for biodiversity-based monitoring and assessment. Front. Mar. Sci. 3:96. doi: 10.3389/fmars.2016.00096
Aylagas, E., Borja, A., and Rodríguez-Ezpeleta, N. (2014). Environmental status assessment using DNA metabarcoding: towards a genetics based Marine Biotic Index (gAMBI). PLoS ONE 6:e90529. doi: 10.1371/journal.pone.0090529
Aylagas, E., Borja, A., Tangherlini, M., Dell'Anno, A., Corinaldesi, C., Mich, C. T., et al. (2017). A bacterial community-based index to assess the ecological status of estuarine and coastal environments. Mar. Pollut. Bull. 114, 679–688. doi: 10.1016/j.marpolbul.2016.10.050
Bengtsson-Palme, J., Hartmann, M., Eriksson, K. M., Pal, C., Thorell, K., Larsson, D. G. J., et al. (2015). METAXA2: improved identification and taxonomic classification of small and large subunit rRNA in metagenomic data. Molec. Ecol. Resour. 15, 1403–1414. doi: 10.1111/1755-0998.12399
Bohmann, K. A., Evans, M. T. P., Gilbert, G., Carvalho, S., Creer, M., Knapp, M., et al. (2014). Environmental DNA for wildlife biology and biodiversity monitoring. Trends Ecol. Evol. 29:485. doi: 10.1016/j.tree.2014.05.012
Boisvert, S., Raymond, F., Godzaridis, E., Laviolette, F., and Corbeil, J. R. (2012). Meta: scalable de novo metagenome assembly and profiling. Genome Biol. 13:R122. doi: 10.1186/gb-2012-13-12-r122
Bonilla-Rosso, G. (2015). “Lessons learned from simulated metagenomic datasets,” in Encyclopedia of Metagenomics: Genes, Genomes and Metagenomes: Basics, Methods, Databases and Tools, ed K. E. Nelson (New York, NY: Springer), 353–359.
Borja, A., Bricker, S. B., Dauer, D. M., Demetriades, N. T., Ferreira, J. G., Forbes, A. T., et al. (2008). Overview of integrative tools and methods in assessing ecological integrity in estuarine and coastal systems worldwide. Mar. Pollut. Bull. 56, 1519–1537. doi: 10.1016/j.marpolbul.2008.07.005
Borja, A., Elliott, M., Andersen, J. H., Berg, T., Carstensen, J., Halpern, B. S., et al. (2016). Overview of integrative assessment of marine systems: the ecosystem approach in practice. Front. Mar. Sci. 3:20. doi: 10.3389/fmars.2016.00020
Borja, A., Elliott, M., Carstensen, J., Heiskanen, A.-S., and van de Bund, W. (2010). Marine management – towards an integrated implementation of the european marine strategy framework and the water framework directives. Mar. Poll. Bull. 60, 2175–2186. doi: 10.1016/j.marpolbul.2010.09.026
Borja, A., Prins, T. C., Simboura, N., Andersen, J. H., Berg, T., Marques, J. C., et al. (2014). Tales from a thousand and one ways to integrate marine ecosystem components when assessing the environmental status. Front. Mar. Sci. 1:72. doi: 10.3389/fmars.2014.00072
Bork, P., de Vargas, C., Gorsky, G., Karsenti, E., and Wincker, P., and Tara Oceans (2015). Tara Oceans studies plankton at planetary scale. Introduction. Science 348:873. doi: 10.1126/science.aac5605
Bourlat, S. J., Borja, A., Gilbert, J., Taylor, M. I., Davies, N., Weisberg, S. B., et al. (2013). Genomics in marine monitoring: new opportunities for assessing marine health status. Mar. Pollut. Bull. 74, 19–31. doi: 10.1016/j.marpolbul.2013.05.042
Bowers, H. A., Marin, R., Birch, J. M., Scholin, C. A., and Doucette, G. J. (2016). Recovery and identification of Pseudo-nitzschia (Bacillariophyceae) frustules from natural samples acquired using the environmental sample processor. J. Phycol. 52, 135–140. doi: 10.1111/jpy.12369
Bucklin, A., Lindeque, P. K., Rodriguez-Ezpeleta, N., Albaina, A., and Lehtiniemi, M. (2016). Metabarcoding of marine zooplankton: prospects, progress and pitfalls. J. Plankton Res. 38, 393–400. doi: 10.1093/plankt/fbw023
Buttigieg, P. L., Morrison, N., Smith, B., Mungall, C. J., and Lewis, S. E. (2013). The environment ontology: contextualising biological and biomedical entities. J. Biomed. Semantics. 4:43. doi: 10.1186/2041-1480-4-43
Buttigieg, P. L., Pafilis, E., Lewis, S. E., Schildhauer, M. P., Walls, R. L., and Mungall, C. J. (2016). The environment ontology in 2016: bridging domains with increased scope, semantic density, and interoperation. J. Biomed. Semantics. 7:57. doi: 10.1186/s13326-016-0097-6
Callahan, B. J., McMurdie, P. J., Rosen, M. J., Han, A. W., Johnson, A. J. A., and Holmes, S. P. (2016). DADA2: high-resolution sample inference from Illumina amplicon data. Nat. Methods. 13, 581–583. doi: 10.1038/nmeth.3869
Cammen, K. M., Andrews, K. R., Carroll, E. L., Foote, A. D., Humble, E., Khudyakov, J. I., et al. (2016). Genomic methods take the plunge: recent advances in high-throughput sequencing of marine mammals. J. Heredity 107, 481–495. doi: 10.1093/jhered/esw044
Caporaso, J. G., Kuczynski, J., Stombaugh, J., Bittinger, K., Bushman, F. D., Costello, E. K., et al. (2010). QIIME allows analysis of high-throughput community sequencing data. Nat. Methods 7, 335–336. doi: 10.1038/nmeth.f.303
Carugati, L., Corinaldesi, C., Dell'Anno, A., and Danovaro, R. (2015). Metagenetic tools for the census of marine meiofaunal biodiversity. Mar. Genomics 24, 11–20. doi: 10.1016/j.margen.2015.04.010
Caruso, G., La Ferla, R., Azzaro, M., Zoppini, A., Marino, G., Petochi, T., et al. (2016). Microbial assemblages for environmental quality assessment: knowledge, gaps and usefulness in the European Marine Strategy Framework Directive. Crit. Rev. Microbiol. 42, 883–904. doi: 10.3109/1040841X.2015.1087380
Chain, P. S. G., Grafham, D. V., Fulton, R. S., FitzGerald, M. G., Hostetler, J., Muzny, D., et al. (2009). Genome project standards in a new era of sequencing. Science 326, 236–237. doi: 10.1126/science.1180614
Chang, J. (2015). Core services: reward bioinformaticians, Nature 520, 151–152. doi: 10.1038/520151a
Chariton, A. A., Stephenson, S., Morgan, M. J., Steven, A. D. L., Colloff, M. J., Court, L., et al. (2015). Metabarcoding of benthic eukaryote communities predicts the ecological condition of estuaries. Envrion. Pollut. 203, 165–174. doi: 10.1016/j.envpol.2015.03.047
Chen, D. C. (2017). Larval Rockfish Diversity Abundance Dynamics in Association with a Marine Protected Area in the Southern California Bight. MS Thesis, University of San Diego.
Chen, B., Yang, Y., Liang, X., yu, K., Zhang, T., and Li, X. (2013). Metagenomic profiles of antibiotic resistance genes (ARGs) between human impacted estuary and deep ocean sediments. Environ. Sci. Technol. 47, 12753−12760. doi: 10.1021/es403818e
Chown, S. L., Hodgins, K. A., Griffin, P. C., Oakeshott, J. G., Byrne, M., and Hoffmann, A. A. (2015). Biological invasions, climate change and genomics. Evol. Appl. 8, 23–46. doi: 10.1111/eva.12234
Cowart, D. A., Pinheiro, M., Mouchel, O., Maguer, M., Grall, J., Miné, J., et al. (2015). Metabarcoding is powerful yet still blind: a comparative analysis of morphological and molecular surveys of seagrass communities. PLoS ONE 10:e0117562. doi: 10.1371/journal.pone.0117562
Cox, A. M., and Goodwin, K. D. (2013). Analysis of sample preparation methods for quantitative detection of DNA by molecular assays and marine biosensors. Mar. Pollut. Bull. 73, 47–56. doi: 10.1016/j.marpolbul.2013.06.006
Creer, S., Deiner, K., Frey, S., Porazinska, D., Taberlet, P., Thomas, W. K., et al. (2016). The ecologist's field guide to sequence-based identification of biodiversity. Methods Ecol. Evol. 7, 1008–1018. doi: 10.1111/2041-210X.12574
Curtin, R., and Prellezo, R. (2010). Understanding marine ecosystem based management: a literature review. Mar. Policy 34, 821–830. doi: 10.1016/j.marpol.2010.01.003
Danovaro, R., Carugati, L., Berzano, M., Cahill, A. E., Carvalho, S., Chenuil, A., et al. (2016). Implementing and innovating marine monitoring approaches for assessing marine environmental status. Front. Mar. Sci. 3:213. doi: 10.3389/fmars.2016.00213
Darling, A. E., Jospin, G., Lowe, E., Matsen, A. F., Bik, H. M., and Eisen, J. A. (2014). PhyloSift: phylogenetic analysis of genomes and metagenomes. PeerJ. 2:e243. doi: 10.7717/peerj.243
Dauble, A. D., Heppell, S. A., and Johansson, M. L. (2012). Settlement patterns of young-of-the-year rockfish among six Oregon estuaries experiencing different levels of human development. Mar. Ecol. Prog. Ser. 448, 143–154. doi: 10.3354/meps09504
Day, V., Paxinos, R., Emmett, J., Wright, A., and Goecker, M. (2008). The marine planning framework for South Australia: a new ecosystem-based zoning policy for marine management. Mar. Policy 32, 535–543. doi: 10.1016/j.marpol.2007.10.009
de Jonge, V. N., Elliott, M., and Brauer, V. S. (2006). Marine monitoring: its shortcomings and mismatch with the EU Water Framework Directive's objectives. Mar. Pollut. Bull. 53, 5–19. doi: 10.1016/j.marpolbul.2005.11.026
del Campo, J., Guillou, L., Hehenberger, E., Logares, R., Löpez-García, P., and Massana, R. (2016). Ecological and evolutionary significance of novel protist lineages. Eur. J. Protistol. 55(Pt A), 4–11. doi: 10.1016/j.ejop.2016.02.002
DeLong, E. F. (2009). The microbial ocean from genomes to biomes. Nature 459, 200–206. doi: 10.1038/nature08059
Desai, N., Antonopoulos, D., Gilbert, J. A., Glass, E. M., and Meyer, F. (2012). From genomics to metagenomics. Curr. Opin. Biotech. 23, 72–76. doi: 10.1016/j.copbio.2011.12.017
DeSantis, T. Z., Hugenholtz, P., Larsen, N., Rojas, M., Brodie, E. L., Keller, K., et al. (2006). Greengenes, a chimera-checked 16S rRNA gene database and workbench compatible with ARB. Appl. Environ. Microbiol. 72, 5069–5072. doi: 10.1128/AEM.03006-05
Droege, G., Barker, K., Seberg, O., Coddington, J., Benson, E., Berendsohn, W. G., et al. (2016). The Global Genome Biodiversity Network (GGBN) data standard specification. Database 2016:baw125. doi: 10.1093/database/baw125
Dröge, J., Gregor, I., and McHardy, A. C. (2015). Taxator-tk: precise taxonomic assignment of metagenomes by fast approximation of evolutionary neighborhoods. Bioinformatics 31, 817–824. doi: 10.1093/bioinformatics/btu745
Duarte, C. M., and Cebrian, J. (1996). The fate of marine autotrophic production. Limnol. Oceanogr. 41, 1758–1766. doi: 10.4319/lo.1996.41.8.1758
Duffy, J. E., Amaral-Zettler, L. A., Fautin, D. G., Paulay, G., Rynearson, T. A., Sosik, H. M., et al. (2013). Envisioning a marine biodiversity observation network. Bioscience 63, 350–361. doi: 10.1525/bio.2013.63.5.8
Dunthorn, M., Klier, J., Bunge, J., and Stoeck, T. (2012). Comparing the hyper-variable V4 and V9 regions of the small subunit rDNA for assessment of ciliate environmental diversity. J. Eukaryot. Microbiol. 59, 185–187. doi: 10.1111/j.1550-7408.2011.00602.x
Dupont, C. L., McCrow, J. P., Valas, R., Moustafa, A., Walworth, N., Goodenough, U., et al. (2015). Genomes and gene expression across light and productivity gradients in eastern subtropical Pacific microbial communities. ISME J. 9, 1076–1092. doi: 10.1038/ismej.2014.198
Edwards, R. A., Haggerty, J. M., Cassman, N., Busch, J. C., Aguinaldo, K., Chinta, S., et al. (2013). Microbes, metagenomes and marine mammals: enabling the next generation of scientist to enter the genomic era. BMC Genomics 14:600. doi: 10.1186/1471-2164-14-600
Elbrecht, V., and Leese, F. (2015). Can DNA-based ecosystem assessments quantify species abundance? Testing primer bias and biomass-sequence relationships with an innovative metabarcoding protocol. PLoS ONE 10:e0130324. doi: 10.1371/journal.pone.0130324
Elliott, M. (2011). Marine science and management means tackling exogenic unmanaged pressures and endogenic managed pressures —a numbered guide. Mar. Pollut. Bull. 62, 651–655. doi: 10.1016/j.marpolbul.2010.11.033
Eren, A. M., Maignien, L., Sul, W. J., Murphy, L. G., and Grim, S. L. (2013). Oligotyping: differentiating between closely related microbial taxa using 16S rRNA gene data. Methods Ecol. Evol. 4, 1111–1119. doi: 10.1111/2041-210X.12114
Escobar-Zepeda, A., Vera-Ponce de León, A., and Sanchez-Flores, A. (2015). The road to metagenomics: from microbiology to DNA sequencing technologies and bioinformatics. Front. Genetics 6:348. doi: 10.3389/fgene.2015.00348
Esposito, A., and Kirschberg, M. (2014). How many 16S-based studies should be included in a metagenomic conference? It may be a matter of etymology. FEMS Microbiol. Lett. 351, 145–146. doi: 10.1111/1574-6968.12375
Evans, N. T., Olds, B. P., Renshaw, M. A., Turner, C. R., Li, Y., Jerde, C. L., et al. (2016). Quantification of mesocosm fish and amphibian species diversity via environmental DNA metabarcoding. Mol. Ecol. Resour. 16, 29–41. doi: 10.1111/1755-0998.12433
Ferrera, I., Giner, C. R., Reñé, A., Camp, J., Massana, R., Gasol, J. M., et al. (2016). Evaluation of alternative high-throughput sequencing methodologies for the monitoring of marine picoplanktonic biodiversity based on rRNA gene amplicons. Front. Mar. Sci. 3:147. doi: 10.3389/fmars.2016.00147
Field, D., Garrity, G., Gray, T., Morrison, N., Selengut, J., Sterk, P., et al. (2008). The minimum information about a genome sequence (MIGS) specification. Nat. Biotechnol. 26, 541–547. doi: 10.1038/nbt1360
Foote, A. D., Thomsen, P. F., Sveegaard, S., Wahlberg, M., Kielgast, J., Kyhn, L. A., et al. (2012). Investigating the potential use of environmental DNA (eDNA) for genetic monitoring of marine mammals. PLoS ONE 7:e41781. doi: 10.1371/journal.pone.0041781
Garner, B. A., Hand, B. K., Amish, S. J., Bernatchez, L., Foster, J. T., Miller, K. M., et al. (2016). Genomics in conservation: case studies and bridging the gap between data and application. Trends Ecol. Evol. 31, 81–83. doi: 10.1016/j.tree.2015.10.009
Garza, D. R., and Dutilh, B. E. (2015). From cultured to uncultured genome sequences: metagenomics and modeling microbial ecosystems. Cell. Mol. Life Sci. 72, 4287–4308. doi: 10.1007/s00018-015-2004-1
Gilbert, J. (2015). “Metagenomics, metadata, and meta-analysis,” in Encyclopedia of Metagenomics: Genes, Genomes and Metagenomes: Basics, Methods, Databases and Tools, ed K. E. Nelson (New York, NY: Springer), 439–441.
Gilbert, J. A., Jansson, J. K., and Knight, R. (2014). The Earth Microbiome Project: successes and aspirations. BMC Biol. 12:69. doi: 10.1186/s12915-014-0069-1
Giner, C. R., Forn, I., Romac, S., Logares, R., de Vargas, C., and Massana, R. (2016). Environmental sequencing provides reasonable estimates of the relative abundance of specific picoeukaryotes. Appl. Environ. Microbiol. 82, 4757–4766. doi: 10.1128/AEM.00560-16
Giovannoni, S. J., Britschgi, T. B., Moyer, C. L., and Field, K. G. (1990). Genetic diversity in sargasso sea bacterioplankton. Nature 345, 60–63. doi: 10.1038/345060a0
Guo, J., Cole, J. R., Zhang, Q., Brown, C. T., and Tiedje, J. M. (2016). Microbial community analysis with ribosomal gene fragments from shotgun metagenomes. Appl. Environ. Microbiol. 82, 157–166. doi: 10.1128/AEM.02772-15
Guo, L., Sui, Z., Zhang, S., Ren, Y., and Liu, Y. (2015). Comparison of potential diatom ‘barcode’ genes (the 18S rRNA gene and ITS, COI, rbcL) and their effectiveness in discriminating and determining species taxonomy in the Bacillariophyta. Int. J. Syst. Evol. Microbiol. 65(Pt 4), 1369–1380. doi: 10.1099/ijs.0.000076
Halpern, B. S., Frazier, M., Potapenko, J., Casey, K. S., Koenig, K., Longo, C., et al. (2015). Spatial and temporal changes in cumulative human impacts on the world's ocean. Nature Comm. 6:7615. doi: 10.1038/ncomms8615
Halpern, B. S., Walbridge, S., Selkoe, K. A., Kappel, C. V., Micheli, F., Agrosa, C., et al. (2008). A global map of human impact on marine ecosystems. Science 319, 948. doi: 10.1126/science.1149345
Hamady, M., and Knight, R. (2009). Microbial community profiling for human microbiome projects: tools, techniques, and challenges. Genome Res. 19, 1141–1152. doi: 10.1101/gr.085464.108
Hancock-Hanser, B. L., Frey, A., Leslie, M. S., Dutton, P. H., Archer, F. I., and Morin, P. A. (2013). Targeted multiplex next-generation sequencing: advances in techniques of mitochondrial and nuclear DNA sequencing for population genomics. Mol. Ecol. Resour. 13, 254–268. doi: 10.1111/1755-0998.12059
Handelsman, J. (2004). Metagenomics: application of genomics to uncultured microorganisms. Microbiol. Mol. Biol. Rev. 68, 669–685. doi: 10.1128/MMBR.68.4.669-685.2004
Hänfling, B., Lawson Handley, L., Read, D. S., Hahn, C., Li, J., Nichols, P., et al. (2016). Environmental DNA metabarcoding of lake fish communities reflects long-term data from established survey methods. Mol. Ecol. 25, 3101–3119. doi: 10.1111/mec.13660
Harada, A. E., Lindgren, E. A., Hermsmeier, M. C., Rogowski, P. A., Terrill, E., and Burton, R. S. (2015). Monitoring spawning activity in a southern California marine protected area using molecular identification of fish eggs. PLoS ONE 10:e0134647. doi: 10.1371/journal.pone.0134647
Hazen, T. C., Rocha, A. M., and Techtmann, S. M. (2013). Advances in monitoring environmental microbes. Curr. Opin. Biotech. 24, 526–533. doi: 10.1016/j.copbio.2012.10.020
He, X., Field, J. C., Pearson, D. E., Lefebvre, L., and Lindley, S. (2015). Status of Bocaccio, Sebastes Paucispinis, in the Conception, Monterey and Eureka INPFC Areas for 2015. Portland, OR: Pacific Fisheries Management Council.
Head, S. R., Komori, H. K., LaMere, S. A., Whisenant, T., Van Nieuwerburgh, F., Salomon, D. R., et al. (2014). Library construction for next-generation sequencing: overviews and challenges. Biotechniques 56, 61–64. doi: 10.2144/000114133
Henriques, I., Tacao, M., Leite, L., Fidalgo, C., Araujo, S., Oliveira, C., et al. (2016). Co-selection of antibiotic and metal(loid) resistance in gram-negative epiphytic bacteria from contaminated salt marshes. Mar. Pollut. Bull. 109, 427–434. doi: 10.1016/j.marpolbul.2016.05.031
Hirai, J., Kuriyama, M., Ichikawa, T., Hidaka, K., and Tsuda, A. (2015a). A metagenetic approach for revealing community structure of marine planktonic copepods. Mol. Ecol. Resour. 15, 68–80. doi: 10.1111/1755-0998.12294
Hirai, J., Yasuike, M., Fujiwara, A., Nakamura, Y., Hamaoka, S., Katakura, S., et al. (2015b). Effects of plankton net characteristics on metagenetic community analysis of metazoan zooplankton in a coastal marine ecosystem. J. Exp. Mar. Biol. Ecol. 469, 36–43. doi: 10.1016/j.jembe.2015.04.011
Hugerth, L. W., Larsson, J., Alneberg, J., Lindh, M. V., Legrand, C., Pinhassi, J., et al. (2015). Metagenome-assembled genomes uncover a global brackish microbiome. Genome Biol. 16:279. doi: 10.1186/s13059-015-0834-7
Hugerth, L. W., Muller, E. E. L., Hu, Y. O. O., Lebrun, L. A. M., Roume, H., Lundin, D., et al. (2014). Systematic design of 18S rRNA gene primers for determining eukaryotic diversity in microbial consortia. PLoS ONE 9:e95567. doi: 10.1371/journal.pone.0095567
Hughey, R., and Karplus, K. (2003). Bioinformatics: a new field in Engineering Education. J. End. Educ. 92, 101–104. doi: 10.1002/j.2168-9830.2003.tb00745.x
Hulme, P. E. (2006). Beyond control: wider implications for the management of biological invasions. J. Appl. Ecol. 43, 835–847. doi: 10.1111/j.1365-2664.2006.01227.x
Hunter, S., Corbett, M., Denise, H., Fraser, M., Gonzalez-Beltran, A., Hunter, C., et al. (2014). EBI metagenomics–a new resource for the analysis and archiving of metagenomic data. Nucleic Acids Res. 42, D600–D606. doi: 10.1093/nar/gkt961
Hurwitz, B. L., Westveld, A. H., Brum, J. R., and Sullivan, M. B. (2014). Modeling ecological drivers in marine viral communities using comparative metagenomics and network analyses. Proc. Natl. Acad. Sci. U.S.A. 111, 10714–10719. doi: 10.1073/pnas.1319778111
Imelfort, M., Parks, D., Woodcroft, B. J., Dennis, P., Hugenholtz, P., and Tyson, G. W. (2014). GroopM: an automated tool for the recovery of population genomes from related metagenomes. Peer J. 2:e603. doi: 10.7717/peerj.603
Ininbergs, K., Bergman, B., Larsson, J., and Ekman, M. (2015). Microbial metagenomics in the Baltic Sea: recent advancements and prospects for environmental monitoring. Ambio 44(Suppl. 3), 439–450. doi: 10.1007/s13280-015-0663-7
Jeon, Y. S., Park, S. C., Lim, J., Chun, J., and Kim, B. S. (2015). Improved pipeline for reducing erroneous identification by 16S rRNA sequences using the Illumina MiSeq platform. J. Microbiol. 53, 60–69. doi: 10.1007/s12275-015-4601-y
Johnson, Z. I., and Martiny, A. C. (2015). Techniques for quantifying phytoplankton biodiversity. Ann. Rev. Mar. Sci. 7, 299–324. doi: 10.1146/annurev-marine-010814-015902
Kanehisa, M., Araki, M., Goto, S., Hattori, M., Hirakawa, M., Itoh, M., et al. (2008). KEGG for linking genomes to life and the environment. Nucleic Acids Res. 36, D480–D484. doi: 10.1093/nar/gkm882
Karsenti, E., Acinas, S. G., Bork, P., Bowler, C., De Vargas, C., Raes, J., et al. (2011). A holistic approach to marine eco-systems biology. PLoS Biol. 9:e1001177. doi: 10.1371/journal.pbio.1001177
Kelly, R. P. (2016). Making environmental DNA count. Molec. Ecol. Res. 16, 10–12. doi: 10.1111/1755-0998.12455
Kelly, R. P., Closek, C. J., O'Donnell, J. L., Kralj, J. E., Shelton, A. O., and Samhouri, J. F. (2017). Genetic and manual survey methods yield different and complementary views of an ecosystem. Front. Mar. Sci. 3:283. doi: 10.3389/fmars.2016.00283
Kelly, R. P., O'Donnell, J. L., Lowell, N. C., Shelton, A. O., Samhouri, J. F., Hennessey, S. M., et al. (2016). Genetic signatures of ecological diversity along an urbanization gradient. Peer J. 4:e2444. doi: 10.7717/peerj.2444
Kelly, R. P., Port, J. A., Yamahara, K. M., Martone, R. G., Lowell, N., Thomsen, P. F., et al. (2014). Harnessing DNA to improve environmental management. Science 344:1455. doi: 10.1126/science.1251156
Klindworth, A., Pruesse, E., Schweer, T., Peplies, J., Quast, C., Horn, M., et al. (2013). Evaluation of general 16S ribosomal RNA gene PCR primers for classical and next-generation sequencing-based diversity studies. Nucl. Acids Res. 41:e1. doi: 10.1093/nar/gks808
Knight, R., Jansson, J., Field, D., Fierer, N., Desai, N., Fuhrman, J. A., et al. (2012). Unlocking the potential of metagenomics through replicated experimental design. Nat. Biotechnol. 30, 513–520. doi: 10.1038/nbt.2235
Kopf, A., Bicak, M., Kottmann, R., Schnetzer, J., Kostadinov, I., Lehmann, K., et al. (2015). The ocean sampling day consortium. GigaSci. 4:27. doi: 10.1186/s13742-015-0066-5
Kozich, J. J., Westcott, S. L., Baxter, N. T., Highlander, S. K., and Schloss, P. D. (2013). Development of a dual-index sequencing strategy and curation pipeline for analyzing amplicon sequence data on the MiSeq Illumina sequencing platform. Appl. Environ. Microbiol. 79, 5112–5120. doi: 10.1128/AEM.01043-13
Kudela, R. M., Howard, M. D. A., Jenkins, B. D., Miller, P. E., and Smith, G. J. (2010). Using the molecular toolbox to compare harmful algal blooms in upwelling systems. Prog. Oceanogr. 85, 108–121. doi: 10.1016/j.pocean.2010.02.007
Lacoursière-Roussel, A., Côté, G., Leclerc, V., and Bernatchez, L. (2016). Quantifying relative fish abundance with eDNA: a promising tool for fisheries management. J. Appl. Ecol. 53, 1148–1157. doi: 10.1111/1365-2664.12598
Laramie, M. B., Pilliod, D. S., and Goldberg, C. S. (2015). Characterizing the distribution of an endangered salmonid using environmental DNA analysis. Biol. Conserv. 183, 29–37. doi: 10.1016/j.biocon.2014.11.025
Leek, J. T., Johnson, W. E., Parker, H. S., Jaffe, A. E., and Storey, J. D. (2012). The sva package for removing batch effects and other unwanted variation in high-throughput experiments. Bioinfor 28, 882–883. doi: 10.1093/bioinformatics/bts034
Lejzerowicz, F., Esling, P., Pillet, L., Wilding, T. A., Black, K. D., and Pawlowski, J. (2015). High-throughput sequencing and morphology perform equally well for benthic monitoring of marine ecosystems. Sci. Rep. 5:13932. doi: 10.1038/srep13932
Leray, M., and Knowlton, N. (2016). Censusing marine eukaryotic diversity in the twenty-first century. Philos. Trans. R Soc. Lond. B Biol. Sci. 371:20150331. doi: 10.1098/rstb.2015.0331
Leslie, M. S., and Morin, P. A. (2016). Using genome-wide SNPs to detect structure in high-diversity and low-divergence populations of severely impacted eastern tropical pacific spinner (Stenella longirostris) and pantropical spotted dolphins (S. attenuata). Front. Mar. Sci. 3:253. doi: 10.3389/fmars.2016.00253
Levin, P. S., Fogarty, M. J., Murawski, S. A., and Fluharty, D. (2009). Integrated ecosystem assessments: developing the scientific basis for ecosystem-based management of the ocean. PLoS Biol. 7:e1000014. doi: 10.1371/journal.pbio.1000014
Lewis, L. A., Richardson, D. E., Zakharov, E. V., and Hanner, R. (2016). Integrating DNA barcoding of fish eggs into ichthyoplankton monitoring programs. Fishery Bull. 114, 153–165. doi: 10.7755/FB.114.2.3
Lindeque, P. K., Parry, H. E., Harmer, R. A., Somerfield, P. J., and Atkinson, A. (2013). Next generation sequencing reveals the hidden diversity of zooplankton assemblages. PLoS ONE 8:e81327. doi: 10.1371/journal.pone.0081327
Liquete, C., Piroddi, C., Drakou, E. G., Gurney, L., Katsanevakis, S., Charef, A., et al. (2013). Current status and future prospects for the assessment of marine and coastal ecosystem services: a systematic review. PLoS ONE 8:e67737. doi: 10.1371/journal.pone.0067737
Liu, B., Gibbons, T., Ghodsi, M., Treangen, T., and Pop, M. (2011). Accurate and fast estimation of taxonomic profiles from metagenomic shotgun sequences. BMC Genomics 12(Suppl. 2):S4. doi: 10.1186/1471-2164-12-S2-S4
Lu, J., Breitwieser, P. F. P., Thielen, P., and Salzberg, S. L. (2017). Bracken: estimating species abundance in metagenomics data. PeerJ. Comput. Sci. 3:e104. doi: 10.7717/peerj-cs.104
Luo, C., Knight, R., Siljander, H., Knip, M., Ramnik, J. X., and Gevers, D. (2015). ConStrains identifies microbial strains in metagenomic datasets. Nat. Biotechnol. 33, 1045–1052. doi: 10.1038/nbt.3319
Ma, H., Stewart, K., Lougheed, S., Zheng, J., Wang, Y., and Zhao, J. (2016). Characterization, optimization, and validation of environmental DNA (eDNA) markers to detect an endangered aquatic mammal. Conserv. Genetics Res. 8, 561–568. doi: 10.1007/s12686-016-0597-9
Mason, O. U., Scott, N. M., Gonzalez, A., Robbins-Pianka, A., Bælum, J., Kimbrel, J., et al. (2014). Metagenomics reveals sediment microbial community response to Deepwater Horizon oil spill. ISME J. 8, 1464–1475. doi: 10.1038/ismej.2013.254
Matsen, A. F., Kodner, R. B., and Armbrust, E. V. (2010). Pplacer: linear time maximum-likelihood and Bayesian phylogenetic placement of sequences onto a fixed reference tree. BMC Bioinform. 11:538. doi: 10.1186/1471-2105-11-538
Mendoza, M. L. Z., Sicheritz-Pontén, T., and Gilbert, M. T. (2015). Environmental genes and genomes: understanding the differences and challenges in the approaches and software for their analyses. Brief. Bioinform. 16, 745–758. doi: 10.1093/bib/bbv001
Menzel, P., Ng, K. L., and Krogh, A. (2016). Fast and sensitive taxonomic classification for metagenomics with Kaiju. Nature 7:1125. doi: 10.1038/ncomms11257
Meyer, F., Paarmann, D., D'Souza, M., Olson, R., Glass, E. M., Kubal, M., et al. (2008). The metagenomics RAST server – a public resource for the automatic phylogenetic and functional analysis of metagenomes. BMC Bioinform. 9:386. doi: 10.1186/1471-2105-9-386
Millennium Ecosystem Assessment (2005). Ecosystems and Human Well-Being: Wetlands and Water Synthesis. Washington, DC: World Resources Institute.
Mitra, S., Gilbert, J. A., Field, D., and Huson, D. H. (2010). Comparison of multiple metagenomes using phylogenetic networks based on ecological indices. ISME J. 4, 1236–1242. doi: 10.1038/ismej.2010.51
Miya, M., Sato, Y., Fukunaga, T., Sado, T., Pulsen, Y., Sato, K., et al. (2015). MiFish, a set of universal PCR primers for metabarcoding environmental DNA from fishes: detection of more than 230 subtropical marine species. R. Soc. Open Sci. 2:150088. doi: 10.1098/rsos.150088
Mock, T., Daines, S. J., Geider, R., Collins, S., Metodiev, M., Millar, A. J., et al. (2015). Bridging the gap between omics and earth system science to better understand how environmental change impacts marine microbes. Glob. Change Biol. 22, 61–75. doi: 10.1111/gcb.12983
Mock, T., and Kirkham, A. (2012). What can we learn from genomics approaches in marine ecology? From sequences to eco-systems biology! Mar. Ecol. 33, 131–148. doi: 10.1111/j.1439-0485.2011.00479.x
Mohrbeck, I., Raupach, M. J., Martìnez Arbizu, P., Knebelsberger, T., and Laakmann, S. (2015). High-throughput sequencing-the key to rapid biodiversity assessment of marine metazoa? PLoS ONE 10:e0140342. doi: 10.1371/journal.pone.0140342
Möllmann, C., Lindegren, M., Blenckner, T., Bergström, L., Casini, M., Diekmann, R., et al. (2014). Implementing ecosystem-based fisheries management: from single-species to integrated ecosystem assessment and advice for Baltic Sea fish stocks. ICES J. Mar. Sci. 71, 1187–1197. doi: 10.1093/icesjms/fst123
Muir, P., Li, S., Lou, S., Wang, D., Spakowics, D. J., Salichos, L., et al. (2016). The real cost of sequencing: scaling computation to keep pace with data integration. Genome Biol. 17:53. doi: 10.1186/s13059-016-0917-0
Mukherjee, A., Chettri, B., Langpoklakpam, J. S., Basak, P., Prasad, A., Mukherjee, A. K., et al. (2017). Bioinformatic approaches including predictive metagenomic profiling reveal characteristics of bacterial response to petroleum hydrocarbon contamination in diverse environments. Sci. Rep. 7, 1108. doi: 10.1038/s41598-017-01126-3
Mulcahy, D. G., Macdonald, K. S., Brady, S. G., Meyer, C., Barker, K. B., and Coddington, J. (2016). Greater than X kb: a quantitative assessment of preservation conditions on genomic DNA quality, and a proposed standard for genome-quality DNA. PeerJ. 4:e2528. doi: 10.7717/peerj.2528
Muller-Karger, F. E., Kavanaugh, M. T., Montes, E., Balch, W. M., Breitbart, M., Chavez, F. P., et al. (2014). A framework for a marine biodiversity observing network within changing continental shelf seascapes. Oceanography 27, 18–23. doi: 10.5670/oceanog.2014.56
NCRIS (2016). Bioplatforms Australia 2016 Annual Report. Available online at: http://www.bioplatforms.com/wp-content/uploads/BPA_2016_Annual_Report_v12_WEB.pdf (Accessed on: 2-2017).
NOC (2013). National Ocean Policy Implementation Plan. National Ocean Council. Available online at: http://www.whitehouse.gov/sites/default/files/national_ocean_policy_implementation_plan.pdf
Nogales, B., Lanfranconi, M. P., Piña-Villalonga, J. M., and Bosch, R. (2011). Anthropogenic perturbations in marine microbial communities. FEMS Microbiol. Rev. 35, 275–298. doi: 10.1111/j.1574-6976.2010.00248.x
Nurk, S., Meleshko, D., Korobeynikov, A., and Pevzner, P., A. (2017). metaSPAdes: a new versatile de novo metagenomics assembler. Genome Res. doi: 10.1101/gr.213959.116. [Epub ahead of print].
Patwardhan, A., Ray, S., and Roy, A. (2014). Molecular markers in phylogenetic studies - a review. J. Phylogen. Evol. Biol. 2:1000131. doi: 10.4172/2329-9002.1000131
Pawlowski, J., Lejzerowicz, F., and Esling, P. (2014). Next-generation environmental diversity surveys of foraminifera: preparing the future. Biol. Bull. 227, 93–106. doi: 10.1086/BBLv227n2p93
Pedersen, M. W., Overballe-Petersen, S., Ermini, L., Sarkissian, C. D., Haile, J., Hellstrom, M., et al. (2015). Ancient and modern environmental DNA. Philos. Trans. R. Soc. Lond. B. Biol. Sci. 370:20130383. doi: 10.1098/rstb.2013.0383
Peng, Y., Leung, H. C., Yiu, S. M., and Chin, F. Y. (2012). IDBA-UD: a de novo assembler for single-cell and metagenomic sequencing data with highly uneven depth. Bioinformatics 28, 1420–1428. doi: 10.1093/bioinformatics/bts174
Pereira, H. M., Ferrier, S., Walters, M., Geller, G. N., Jongman, R. H., and Scholes, R. J. (2013). Ecology. Essential biodiversity variables. Science 339, 277–278. doi: 10.1126/science.1229931
Pinto, A. J., and Raskin, L. (2012). PCR biases distort bacterial and archaeal community structure in pyrosequencing datasets. PLoS ONE 7:e43093. doi: 10.1371/journal.pone.0043093
Port, J. A., O'Donnell, J. L., Romero-Maraccini, O. C., Leary, P. R., Litvin, S. Y., Nickols, K. J., et al. (2016). Assessing vertebrate biodiversity in a kelp forest ecosystem using environmental DNA. Mol. Ecol. 25, 527–541. doi: 10.1111/mec.13481
Prosser, J. I. (2010). Replicate or lie. Environ. Microbiol. 12, 1806–1810. doi: 10.1111/j.1462-2920.2010.02201.x
Quast, C., Pruesse, E., Yilmaz, P., Gerken, J., Schweer, T., Yarza, P., et al. (2013). The SILVA ribosomal RNA gene database project: improved data processing and web-based tools. Nucleic Acids Res. 41, D590–D596. doi: 10.1093/nar/gks1219
Rappé, B. A., and Giovannoni, S. J. (2003). The uncultured microbial majority. Ann. Rev. Microbiol. 57 369–394. doi: 10.1146/annurev.micro.57.030502.090759
Rees, H. C., Maddison, B. C., Middleditch, D. J., Patmore, J. R. M., and Gough, K. C. (2014). The detection of aquatic animal species using environmental DNA - a review of eDNA as a survey tool in ecology. J. Appl. Ecol. 51, 1450–1459 doi: 10.1111/1365-2664.12306
Rodriguez-R., L. M., and Konstantinides, L. (2014). Estimating coverage in metagenomic data sets and why it matters. ISME J. 8, 2349–2351. doi: 10.1038/ismej.2014.76
Rogers, A. D. (ed.). (2013). The Global State of the ocean; interactions between stresses, impacts and some potential solutions. Synthesis papers from the International Programme on the State of the Ocean 2011 and 2012 Workshops. Mar Pollut Bull. 74, 491–552. Available online at: http://www.sciencedirect.com/science/journal/0025326X/74
Roux, S., Brum, J. R., Dutilh, B. E., Sunagawa, S., Duhaime, M. B., Loy, A., et al. (2016). Ecogenomics and potential biogeochemical impacts of globally abundant ocean viruses. Nature 537, 689–693. doi: 10.1038/nature19366
Rusch, D. B., Halpern, A. L., Sutton, G., Heidelberg, K. B., Williamson, S., Yooseph, S., et al. (2007). The Sorcerer II global ocean sampling expedition: northwest Atlantic through eastern tropical Pacific. PLoS Biol. 5:e77. doi: 10.1371/journal.pbio.0050077
Sassoubre, L. M., Yamahara, K. M., Gardner, L. D., Block, B. A., and Boehm, A. B. (2016). Quantification of environmental DNA (eDNA) shedding and decay rates for three marine fish. Env. Sci. Technol. 50, 10456–10464. doi: 10.1021/acs.est.6b03114
Schirmer, M., Ijaz, U. Z., D'Amore, R., Hall, N., Sloan, W. T., Quince, C., et al. (2015). Insight into biases and sequencing errors for amplicon sequencing with the Illumina MiSeq platform. Nucleic Acids Res. 43:e37. doi: 10.1093/nar/gku1341
Segata, N., Waldron, L., Ballarini, A., Narasimhan, V., Jousson, O., and Huttenhower, C. (2012). Metagenomic microbial community profiling using unique clade-specific marker genes. Nat. Methods 9, 811–814. doi: 10.1038/nmeth.2066
Sharpton, T. J. (2014). An introduction to the analysis of shotgun metagenomic data. Front. Plant Sci. 2014:209. doi: 10.3389/fpls.2014.00209
Shelton, A. O., O'Donnell, J. L., Samhouri, J. F., Lowell, N., Williams, G. D., and Kelly, R. P. (2016). A framework for inferring biological communities from environmental DNA. Ecol. Appl. 26, 1645–1659. doi: 10.1890/15-1733.1
Siegwald, L., Touzet, H., Lemoine, Y., Hot, D., Audebert, C., and Caboche, S. (2017). Assessment of common and emerging bioinformatics pipelines for targeted metagenomics. PLoS ONE 12:e0169563. doi: 10.1371/journal.pone.0169563
Simboura, N., Panayotidis, P., and Papathanassiou, E. (2005). A synthesis of the biological quality elements for the implementation of the European Water Framework Directive in the Mediterranean ecoregion: the case of Saronikos Gulf. Ecol. Indic. 5, 252–266. doi: 10.1016/j.ecolind.2005.03.006
Smyth, R. P., Schlub, T. E., Grimm, A., Venturi, V., Chopra, A., Mallal, S., et al. (2010). Reducing chimera formation during PCR amplification to ensure accurate genotyping. Gene. 469, 45–51. doi: 10.1016/j.gene.2010.08.009
Sogin, M. L., Morrison, H. G., Huber, J. A., Welch, D. M., Huse, S. M., Neal, P. R., et al. (2006). Microbial diversity in the deep sea and the underexplored “rare biosphere.” Proc. Natl. Acad. Sci. U.S.A. 103, 12115–12120. doi: 10.1073/pnas.0605127103
Sohn, M. B., An, L., Pookhao, N., and Li, Q. (2014). Accurate genome relative abundance estimation for closely related species in a metagenomic sample. BMC Bioinformat. 15:242. doi: 10.1186/1471-2105-15-242
Staley, C., Kaser, T., Gidley, M. L., Enochs, I. C., Jones, P. R., Goodwin, K. D., et al. (2017). A next-generation sequencing approach to characterize the impacts of land-based sources of pollution on the microbiota of southeast florida coral reefs. Appl. Environ. Microbiol. doi: 10.1128/AEM.03378-16. [Epub ahead of print].
Staley, C., and Sadowsky, M. J. (2016). Application of metagenomics to assess microbial communities in water and other environmental matrices. J. Mar. Biol. Assoc. UK. 96, 121–129. doi: 10.1017/S0025315415001496
Stein, L. D. (2010). The case for cloud computing in genome informatics. Genome Biol. 11:207. doi: 10.1186/gb-2010-11-5-207
Strong, J. A., Andonegi, E., Bizsel, K. C., Danovaro, R., Elliott, M., Franco, A., et al. (2015). Marine biodiversity and ecosystem function relationships: the potential for practical monitoring applications. Estuar. Coast. Shelf Sci. 161, 46–64. doi: 10.1016/j.ecss.2015.04.008
Stulberg, E., Fravel, D., Proctor, L. M., Murray, D. M., LoTempio, J., Chrisey, L., et al. (2016). An assessment of US microbiome research. Nature Microbiol. 1:15015. doi: 10.1038/nmicrobiol.2015.15
Tacão, M., Correia, A., and Henriques, I. (2012). Resistance to broad-spectrum antibiotics in aquatic systems: anthropogenic activities modulate the dissemination of blaCTX-M-like genes. Appl. Environ. Microbiol. 78, 4134–4140. doi: 10.1128/AEM.00359-12
Tan, B., Ng, C., Nshimyimana, J., Loh, L. L., Gin, K., and Thompson, J. (2015). Next-generation sequencing (NGS) for assessment of microbial water quality: current progress, challenges, and future opportunities. Front. Microbiol. 6:1027. doi: 10.3389/fmicb.2015.01027
Teeling, H., and Glöckner, F. O. (2012). Current opportunities and challenges in microbial metagenome analysis—a bioinformatic perspective. Brief. Bioinformatics 13, 728–742. doi: 10.1093/bib/bbs039
ten Hoopen, P., Amid, C., Buttigieg, P. L., Pafilis, E., Bravakos, P., Cerdeño-Tárraga, A. M., et al. (2016). Value, but high costs in post-deposition data curation. Database (Oxford). 2016:bav126. doi: 10.1093/database/bav126
ten Hoopen, P., Pesant, S., Kottmann, R., Kopf, A., Bicak, M., Claus, S., et al. (2015). Marine microbial biodiversity, bioinformatics and biotechnology (M2B3) data reporting and service standards. Stand. Genomic Sci. 10:20. doi: 10.1186/s40793-015-0001-5
Thomas, T., Gilbert, J., and Meyer, F. (2012). Metagenomics - a guide from sampling to data analysis. Microb. Inform. Exp. 2:3. doi: 10.1186/2042-5783-2-3
Thompson, A. R., Hyde, J. R., Watson, W., Chen, D. C., and Guo, L. W. (2016). Rockfish assemblage structure and spawning locations in southern California identified through larval sampling. Mar. Ecol. Prog. Ser. 547, 177–192. doi: 10.3354/meps11633
Thomsen, P. F., and Willerslev, E. (2015). Environmental DNA - An emerging tool in conservation for monitoring past and present biodiversity. Biol. Cons. 183, 4–18 doi: 10.1016/j.biocon.2014.11.019
Thureborn, P., Lundin, D., Plathan, J., Poole, A. M., Sjõberg, B. M., and Sjöing, S. A. (2013). metagenomics transect into the deepest point of the Baltic Sea reveals clear stratification of microbial functional capacities. PLoS ONE 8:e74983. doi: 10.1371/journal.pone.0074983
Trivedi, S., Aloufi, A. A., Ansari, A. A., and Ghosh, S. K. (2016). Role of DNA barcoding in marine biodiversity assessment and conservation: an update. Saudi J. Biol. Sci. 23, 161–171. doi: 10.1016/j.sjbs.2015.01.001
Valentini, A., Taberlet, P., Miaud, C., Civade, R., Herder, J., Thomsen, P. F., et al. (2016). Next-generation monitoring of aquatic biodiversity using environmental DNA metabarcoding. Mol. Ecol. 25, 929–942. doi: 10.1111/mec.13428
Venter, J. C., et al (2004). Environmental genome shotgun sequencing of the Sargasso Sea. Science 304, 66–74. doi: 10.1126/science.1093857
Vince, J., Smith, A. D. M., Sainsbury, K., Cresswell, I. D., Smith, D. C., and Haward, M. (2015). Australia's Oceans Policy: past, present and future. Mar. Policy 57, 1–8. doi: 10.1016/j.marpol.2015.02.014
Visco, J. A., Apothéloz-Perret-Gentil, L., Cordonier, A., Esling, P., Pillet, L., and Pawlowski, J. (2015). Environmental monitoring: inferring the diatom index from next-generation sequencing data. Environ. Sci. Technol. 49, 7597–7605. doi: 10.1021/es506158m
Wallace, B. P., DiMatteo, A. D., Hurley, B. J., Finkbeiner, E. M., Bolten, A. B., Chaloupka, M. Y., et al. (2010). Regional management units for marine turtles: A novel framework for prioritizing conservation and research across multiple scales. PLoS ONE 5:e15465. doi: 10.1371/journal.pone.0015465
WHCEQ (2010). Final Recommendations of the Interagency Ocean Policy Task Force July 19, 2010. The White House Council of Environmental Quality. Available online at: https://obamawhitehouse.archives.gov/files/documents/OPTF_FinalRecs.pdf
Wood, D. E., and Salzberg, S. L. (2014). Kraken: ultrafast metagenomic sequence classification using exact alignments. Genome Biol. 15:R46. doi: 10.1186/gb-2014-15-3-r46
Xu, J. (2015). “Microbial ecology in the age of metagenomics: an Introduction,” in Encyclopedia of Metagenomics: Genes, Genomes and Metagenomes: Basics, Methods, Databases and Tools, ed K. E. Nelson (New York, NY: Springer), 475–479.
Yamahara, K. M., Demir-Hilton, E., Preston, C. M., Marin, R., Pargett, D., Roman, B., et al. (2015). Simultaneous monitoring of faecal indicators and harmful algae using an in-situ autonomous sensor. Lett. Appl. Microbiol. 61, 130–138. doi: 10.1111/lam.12432
Yamamoto, S., Masuda, R., Sato, Y., Sado, T., Araki, H., Kondoh, M., et al. (2017). Environmental DNA metabarcoding reveals local fish communities in a species-rich coastal sea. Sci. Rep. 7:40368. doi: 10.1038/srep40368
Yilmaz, P., et al. (2011). Minimum information about a marker gene sequence (MIMARKS) and minimum information about any (x) sequence (MIxS) specifications. Nat. Biotechnol. 29:415. doi: 10.1038/nbt.1823
Zaiko, A., Samuiloviene, A., Ardura, A., and Garcia-Vazquez, E. (2015). Metabarcoding approach for nonindigenous species surveillance in marine coastal waters. Mar. Pollut. Bull. 100, 53–59. doi: 10.1016/j.marpolbul.2015.09.030
Zamor, R. M., Glenn, K. L., and Hambright, K. D. (2012). Incorporating molecular tools into routine HAB monitoring programs: using qPCR to track invasive Prymnesium. Harmful Algae 15, 1–7. doi: 10.1016/j.hal.2011.10.028
Zampoukas, N., Palialexis, A., Duffek, A., Graveland, J., Giorgi, G., Hagebro, C., et al. (2014). “Technical guidance on monitoring for the marine strategy framework directive,” in Joint Research Centre of the European Commission. EUR 26499 (Brussels: European Commission Joint Research Centre Institute for Environment and Sustainability).
Zhou, J., He, Z., Yang, Y., Deng, Y., Tringe, S. G., and Alvarez-Cohen, L. (2015). High-throughput metagenomic technologies for complex microbial community analysis: open and closed formats. MBio 6, e02288–e02214. doi: 10.1128/mBio.02288-14
Zhou, Q., Su, X., and Ning, K. (2014). Assessment of quality control approaches for metagenomic data analysis. Sci. Rep. 2014:6957. doi: 10.1038/srep06957
Ziegler, M., Roik, A., Porter, A., Zubier, K., Mudarris, M. S., Ormond, R., et al. (2016). Coral microbial community dynamics in response to anthropogenic impacts near a major city in the central Red Sea. Mar. Pollut. Bull. 105, 629–640. doi: 10.1016/j.marpolbul.2015.12.045
Keywords: metagenetics, metagenomics, metabarcoding, eDNA, marine biological quality element (BQE), good ecological status (GES), biodiversity and ecosystem function (BEF)
Citation: Goodwin KD, Thompson LR, Duarte B, Kahlke T, Thompson AR, Marques JC and Caçador I (2017) DNA Sequencing as a Tool to Monitor Marine Ecological Status. Front. Mar. Sci. 4:107. doi: 10.3389/fmars.2017.00107
Received: 02 November 2016; Accepted: 31 March 2017;
Published: 09 May 2017.
Edited by:
Christos Dimitrios Arvanitidis, Hellenic Centre for Marine Research, GreeceReviewed by:
Franck Lejzerowicz, Université de Genève, SwitzerlandCopyright © 2017 Goodwin, Thompson, Duarte, Kahlke, Thompson, Marques and Caçador. This is an open-access article distributed under the terms of the Creative Commons Attribution License (CC BY). The use, distribution or reproduction in other forums is permitted, provided the original author(s) or licensor are credited and that the original publication in this journal is cited, in accordance with accepted academic practice. No use, distribution or reproduction is permitted which does not comply with these terms.
*Correspondence: Kelly D. Goodwin, a2VsbHkuZ29vZHdpbkBub2FhLmdvdg==
Disclaimer: All claims expressed in this article are solely those of the authors and do not necessarily represent those of their affiliated organizations, or those of the publisher, the editors and the reviewers. Any product that may be evaluated in this article or claim that may be made by its manufacturer is not guaranteed or endorsed by the publisher.
Research integrity at Frontiers
Learn more about the work of our research integrity team to safeguard the quality of each article we publish.