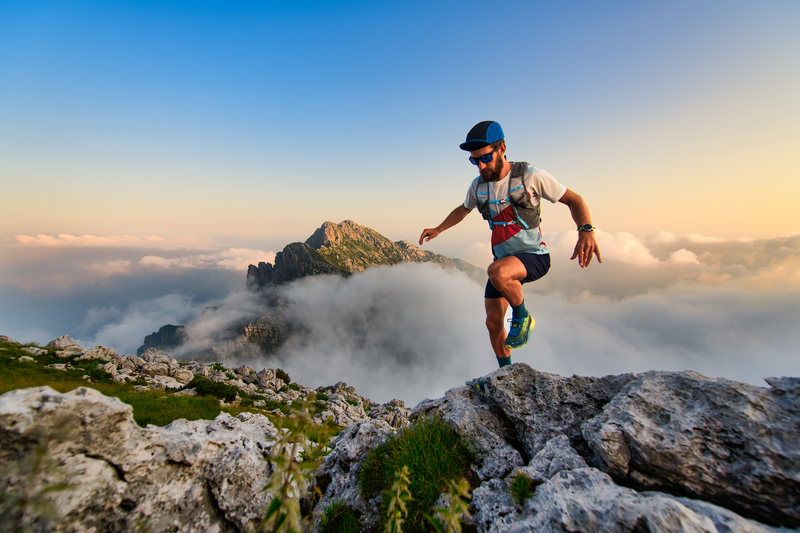
94% of researchers rate our articles as excellent or good
Learn more about the work of our research integrity team to safeguard the quality of each article we publish.
Find out more
ORIGINAL RESEARCH article
Front. Mar. Sci. , 21 September 2016
Sec. Marine Ecosystem Ecology
Volume 3 - 2016 | https://doi.org/10.3389/fmars.2016.00173
This article is part of the Research Topic Towards a synthesis of the physiology, behaviour and ecology of plankton View all 6 articles
The number of species of autotrophic communities can increase ecosystem productivity through species complementarity or through a selection effect which occurs when the biomass of the community approaches the monoculture biomass of the most productive species. Here we explore the effect of resource supply on marine primary productivity under the premise that the high local species richness of phytoplankton communities increases resource use through transient selection of productive species. Using concurrent measurements of phytoplankton community structure, nitrate fluxes into the euphotic zone, and productivity from a temperate coastal ecosystem, we find that observed productivities are best described by a population growth model in which the dominant species of the community approach their maximum growth rates. We interpret these results as evidence of species selection in communities containing a vast taxonomic repertory. The prevalence of selection effect was supported by open ocean data that show an increase in species dominance across a gradient of nutrient availability. These results highlight the way marine phytoplankton optimize resources and sustain world food stocks. We suggest that the maintenance of phytoplankton species richness is essential to sustain marine primary productivity since it guarantees the occurrence of highly productive species.
Despite half of global primary production being mediated by the activity of microscopic algae called phytoplankton, the effect of phytoplankton species richness on marine primary productivity lacks a mechanistic understanding. Theoretical models and experimental work have shown that species-rich communities are more efficient in the use of resources (i.e., biomass production per unit of resource availability) and thus, for a given rate of resource supply, these communities attain higher levels of biomass than the monocultures (Tilman et al., 1996, 1997; Loreau et al., 2001; Hooper et al., 2005; Ptacnik et al., 2008; Cardinale, 2011). This positive relationship between species richness and resource use efficiency is founded on classical competition models, in which coexisting species use different forms of resources (Tilman et al., 1997; Loreau et al., 2001). According to these models, complementarity in resource use among species increases the performance of communities above that expected from the performance of individual species grown alone (Tilman et al., 1996, 1997; Loreau et al., 2001), because the species in a mixture may utilize the resources more completely than any single species would be able to do.
The relationship between phytoplankton species richness, resource use efficiency and primary productivity remains unclear (Grover and Chrzanowski, 2004; Irigoien et al., 2004; Ptacnik et al., 2008; Cermeño et al., 2013). Arguments to explain this relationship have focused primarily on the role of interspecific competition for resources. Whereas, a suite of limiting resources control the number of species in ecosystems characterized by relatively low resource supply (Interlandi and Kilham, 2001; Grover and Chrzanowski, 2004), a few fast-growing species dominate resource use and productivity in eutrophic ecosystems (Huisman and Weissing, 1999; Irigoien et al., 2004). Alternatively, Ptacnik et al. (2008) showed that the efficiency of resource use and thus carbon fixation increases with the diversity of phytoplankton communities in freshwater and brackish habitats, supporting the view that diversity enhances productivity. The coexistence of species in marine phytoplankton communities depends to a large extent on (i) functional trade-offs among species competing for the same suite of resources (Margalef, 1978; Sommer, 1985; Litchman and Klausmeier, 2008), (ii) chaotic competitive interactions which lead to oscillations in species abundances (Huisman and Weissing, 1999), and (iii) the enormous potential of planktonic microorganisms for dispersal, which repeatedly reshuffles community structure (Finlay, 2002). These features suggest that species complementarity in marine phytoplankton influences primary productivity to a much lesser extent than in terrestrial plant communities.
Other studies have shown that species richness can increase resource use efficiency and productivity through a selection effect, which occurs when the biomass of a community approaches the monoculture biomass of the most productive species (Wardle, 1999; Loreau et al., 2001). This model states that species-rich communities have a higher chance of containing the species that achieves the highest biomass when grown alone (Loreau et al., 2001). Testing the effect of species richness on productivity involves evaluating the extent to which productivity changes as new species are added to the community. However, the number of species in natural phytoplankton communities is always remarkably high, the well-known Paradox of the Plankton (Hutchinson, 1961), which precludes testing the effect of species richness on productivity from field samples. An alternative approach is to explore the effect of resource supply on productivity under the premise that the high local species richness increases resource use through transient selection of productive species.
We conducted concurrent measurements of phytoplankton community composition, nitrate fluxes (mmol N m−2 d−1) into the euphotic zone and 14C-uptake rates per unit of photosynthetic biomass (observed productivity, h−1) during a full annual cycle in a temperate coastal ecosystem. Furthermore, we calculated the expected productivity (Pexp) of a community using a population growth model and size-dependent parameterizations of physiological traits, which allowed us to define the extent of species' selection. Selection occurs when the biomass of a community approaches the monoculture biomass of the most productive species; that is when the dominant species of the community achieve their higher maximum growth rates. We computed community productivity, based on intracellular nitrate quotas assigned (i) only to dominant species (positive selection effect), (ii) only to rare species, and (iii) randomly. Then, we tested the performance of the different model estimates (Pexp) against estimates of observed (measured in simulated in situ incubations) productivity (Pobs). By comparing Pexp and Pobs we test the null hypothesis that selection effect controls community productivity. This approach presumes that the enormous species richness of these microbial communities guarantees always the occurrence of highly productive species.
Nine sampling visits were carried out in a central station at Ría de Vigo, NW-Iberian Peninsula (42 14.09°N, 8 47.18°W), where the depth is 45 m at low tide. Sampling was scheduled from February to November 2012 on a monthly basis in order to obtain data from different phases of the seasonal cycle. On each visit, we recorded vertical profiles of temperature with a Sea Bird Electronics SBE 9/11 conductivity, temperature, and depth (CTD) probe attached to a rosette. The vertical distribution of photosynthetically active irradiance (PAR, 400–700 nm) was measured with a spherical quantum sensor connected to a data logger. Incident light was retrieved from a near meteorological station that recorded PAR data throughout the study period. Seawater samples were collected from 3, 10, 20, 30, and 40 m depth in 12-L Niskin bottles. Samples for the analysis of dissolved inorganic nutrients were immediately frozen and stored at −20°C until they were analyzed in the laboratory by segmented flow analysis with Alpkem auto-analyzers (Grasshoff et al., 2007). For the determination of chlorophyll-a (Chl-a) concentration, two 250-mL replicates were filtered through 0.2-μm polycarbonate filters using low vacuum pressure (100 mm Hg). Pigments were extracted by placing the filters in 90% acetone overnight. Chl-a concentration was determined using a TD-700 fluorometer.
Microplankton species were identified in two types of samples: (i) bottle samples and (ii) net samples. For bottle samples seawater was collected from three selected depths (3, 10, and 20 m) using 12-L Niskin bottles as specified above. These three samples were dispensed into a 20-L container and gently mixed to obtain a combined sample of the water column euphotic layer (i.e., the euphotic layer extends from surface to the depth where the photosynthetic available radiation is 1% of its surface value). From this combined sample, four subsamples of 500-mL were preserved in Lugol's iodine solution (2% final concentration). Aliquots (5–50 mL) of these subsamples were settled in composite sedimentation chambers and examined with an inverted microscope (Utermöhl, 1931) until a total volume of 500–1000 mL was reached. Species identity and cell size (both based on morphological criteria) as well as the number of cells per species were determined. Unknown species were classified according to morphological descriptions, for example “Medium-sized, dark, thecate dinoflagellate.” This nomenclature was consistent throughout the study.
Net samples were collected from vertical hauls (from 20 m to surface) with a plankton net (20-μm mesh size) and preserved in Lugol's iodine solution (2% final concentration). Taking into account the volume of seawater filtered through the net and the volume collected, aliquots corresponding to water column volumes of between 500 and 9000 mL were examined as detailed above. This procedure was repeated until a total visualized volume of ~25 L was reached for each sampling day. Net samples were examined with the objective of detecting species not seen in bottle samples and thus cell counting was not carried out (cell density < 1 individual per L). A total of 290 subsamples including bottle and net samples amounting 225 L of seawater were inspected under the microscope (all sampling days included). Sampling effort was increased 1000 × with respect to traditional procedures.
The use of 20-μm mesh size hampered the detection of small-sized species not seen in bottle samples. However, because small-sized cells tend to achieve large population numbers, it is unlikely that these species were overlooked in the analysis of bottle samples. Indeed, our species-accumulation curves reached a saturation level (Cermeño et al., 2014; Rodríguez-Ramos et al., 2014). This effect did not influence our estimates of C biomass. Net samples were examined with the objective of detecting rare species, and thus their contribution to biomass can be regarded negligible.
Cell abundances were converted to carbon biomass using empirically-derived carbon to volume conversion factors (Menden-Deuer and Lessard, 2000) after determining biovolumes (Hillebrand et al., 1999).
The supply of nitrate into the euphotic zone due to vertical diffusion was calculated following Fick's law as the product of the nitrate gradient between 10 and 40 m, obtained by linearly fitting nitrate concentrations in this layer, and vertical diffusivity (Kz) averaged for the same depth interval (Osborn, 1980). Vertical diffusivity (Kz, m2 s−1) was estimated as (Osborn, 1980),
where e is a mixing efficiency (e = 0.2 herein) (Oakey, 1982), ε the dissipation rate of the turbulent kinetic energy and N2 the Brunt-Väisälä frequency measured by a free-falling microstructure turbulence profiler (MSS) (Prandke and Stips, 1998) down to a maximum depth of 45 m (Figure 1). The MSS profiler was equipped with a suite of high-resolution microstructure sensors, including two airfoil shear probes (type PNS06), one fast-response bead thermistor (Thermometrics FP07), high-precision conductivity-temperature-depth (CTD) sensors, and also a sensor to measure the horizontal acceleration of the profiler. The profiler was carefully balanced to have negative buoyancy in the water column and a sinking velocity of 0.3–0.7 m s−1. ε was computed in 512 data point segments, with 50% overlap, from the microstructure shear variance under the assumption of isotropic turbulence. The shear variance was computed by integrating the shear power spectrum. The lower integration limit was determined considering the size of the bins, and set to 2 cycles per meter (cpm). The upper cut-off wave number for the integration of the shear spectrum was set as the Kolmogoroff number, which was determined by an iterative procedure. The maximum uppercut-off was not allowed to exceed 30 cpm to avoid the noisy part of the spectrum. Assuming a universal form of the shear spectrum, ε was corrected for the loss of variance below and above the used integration limits, using the polynomial functions (Prandke et al., 2000). Peaks due to particle collisions were removed by comparing the dissipation rates computed simultaneously from the two shear sensors, which were finally averaged.
Figure 1. Vertical distribution of temperature, vertical diffusivity or mixing (Kz, note the logarithmic scale), and chlorophyll-a concentration estimated from the microturbulence profiler deployed from February to November 2012 at the central station at the Ría de Vigo. The 10 microturbulence profiles deployed at each sampling are plotted. The black/magenta line represents the euphotic layer depth computed as the depth where the photosynthetic available radiation (PAR) is 1% of its surface value. Top labels indicate the concentration of inorganic nitrogen (nitrate plus ammonium) in the surface waters (mmol N m−3), the nitrate flux into the euphotic zone (mmol N m−2 d−1), and the solar radiation dose (Watts m−2). Bottom labels indicate sampling dates.
The Brunt-Väisälä frequency (N2) was derived from the CTD profiles according to the equation:
where g is the acceleration due to gravity (9.8 m s−2), ρw is the density of seawater (~1025 kg m−3), and is the vertical potential density gradient. The dissipation rate of the turbulent kinetic energy (ε) and the Brunt-Väisälä frequency were averaged over depth intervals of 1 m length.
Advection in this system is dominated by the influence of coastal upwelling induced by northerly winds during most of spring and summer (Fraga, 1981). The transport of nitrate through advection was calculated by using a simple box model, where the system is considered as a single box divided into two layers, the deeper one influenced by upwelled water and the surface layer dominated by the outgoing flow. Assuming that the bottom layer volume is constant over time compared to the incoming bottom flux (QB), vertical advection would be comparable to QB (in m3 s−1),computed as the product of the upwelling index (IW, m3 s−1 km−1) and the length of the mouth of the Ría of Vigo (ca. 10 km). IW was calculated according to Wooster et al. (1976) as,
where ρair is the density of air, 1.22 kg·m−3 at 15°C; C is an empirical drag coefficient (dimensionless), 1.3·10−3; f is the Coriolis parameter, 9.946·10−5 s−1 at 43° latitude; ρwis the density of seawater; W is wind speed (in units of m·s−1); and WN is the north component of wind speed. Wind data were taken at the Cabo Silleiro meteorological station. Vertical velocities (VZ) were computed dividing QB by the surface area of Ría de Vigo (ca. 174 km2). Finally, the transport of nitrate into the euphotic zone though vertical advection was computed as the product of VZ and the concentration of nitrate sampled at 40 m (Figure 1).
Averaged daily PAR radiation in the euphotic layer was computed by combining daily PAR surface radiation data and the vertical attenuation coefficients. The solar radiation dose (SRD, in Watts m−2) was computed as (Vallina and Simó, 2007),
where I0, k, and MLD are surface irradiance, light attenuation coefficient (Kirk, 1994) and mixed layer depth, respectively (Figure 1). k was measured each sampling using the LiCOR quantum sensor (photometer) attached to the CTD.
Carbon fixation rates were determined by running simulated “in situ” incubations with the radioisotope 14C. Four 75-ml acid-washed polystyrene bottles (three light and one dark bottle) were filled with seawater from surface, 10, and 20 m depth. Each bottle was inoculated with ~370 kBq (10 μCi) of NaH14CO3 and then incubated for 2 h starting at noon. An incubator equipped with a set of blue and neutral density plastic filters was used. This incubator reproduced the irradiance conditions at the original depths where the samples had been collected. A system of re-circulating water was used to maintain incubation temperature within 1.5°C of the original temperature at each sampling depth.
At the end of the incubations, samples were filtered through 0.2 μm polycarbonate filters under low-vacuum pressure (< 100 mm Hg). Inorganic carbon on the filters was removed by exposing them to concentrated HCl fumes overnight. After removal of inorganic 14C, filters were placed into scintillation vials to which 4 mL of scintillation cocktail were added. The radioactivity on each sample was determined on a 1409-012Wallac scintillation counter which used an internal standard for quenching correction. Dark bottle disintegrations per minute (DPMs) were subtracted from the light bottle DPMs in order to calculate particulate organic carbon production rates (mgC m−3h−1).
The contribution of small phytoplankton to total primary production is in the range 5–30% (no winter–winter) in this coastal ecosystem (Cermeño et al., 2006). We subtracted 5 and 30% to estimates of primary production during no winter and winter, respectively, to eliminate the contribution of the smallest phytoplankton groups (< 2 μm equivalent spherical diameter, ESD), which were not considered in our estimates of photosynthetic carbon biomass. Finally, primary productivity (h−1) was calculated dividing the mean primary production (mgC m−3h−1) across the water column by estimates of photosynthetic carbon biomass (mgC m−3).
We calculated monoculture productivities by using a suite of allometric models (Table 1), which assign size-dependent physiological traits such as maximum growth rates and nutrient subsistence quotas to component species. Then, expected productivity (Pexp) was computed as the weighted (by the relative biomass of species in the community) average of the monoculture productivities for the component species.
Following Droop's cell quota model, the species-specific growth rate (μ) can be described as a function of the cellular quota of the limiting nutrient (Q) and the minimum nutrient quota (Qmin), below which cells cannot grow (Droop, 1973; Grover, 1991),
where μmax is the theoretical maximum growth rate when Q >> Qmin, SRD is the solar radiation dose average over the upper mixed layer, and KSRD is the half saturation constant for light acquisition (Cermeño et al., 2005a). The last term represents the temperature correction factor where Q10 is 1.88, T is the growth temperature in situ and T0 is 18°C. The model parameters μmax and Qmin for each species were obtained from allometric equations that relate maximum growth rate and nutrient quotas to cell size (Table 1) (Edwards et al., 2012; Marañón et al., 2013). Primary production in this coastal ecosystem is strongly influenced by nutrient supply from the adjacent shelf via surface water mass intrusions or upwelling of nutrient-rich deep waters. This oceanic component of nutrient supply is primarily characterized by nitrogen limiting conditions for phytoplankton growth. The half saturation constants for light acquisition were obtained from previously published photosynthesis-irradiance curves (50–100 μmol m−2s−1 during summer and winter, respectively) (Cermeño et al., 2005a). The nutrient uptake rate is maximum when Q is close to Qmin, but decreases as cells fill up with nutrients and Q approaches its maximum potential (Gotham and Rhee, 1981). However, by confining acquired nutrients to a storage vacuole, individual cells enhance the nutrient gradient across the plasmalemma, and hence avoid nutrient saturation states (Raven, 1997). Intracellular storage capacity introduces a time lag between the exhaustion of the external nutrient concentration and the actual nutrient limitation of growth. The light limitation term was parameterized according to a Michaelis–Menten function.
We estimated the intracellular nutrient quota (Q) of individual cells dividing the amount of nitrate accumulated from diffusive fluxes and advective transport over the course of 24 h (μmol L−1) by the abundance of individuals (cells L−1). We selected 24 h time span as it falls, on average, within the doubling time of phytoplankton cells in this coastal ecosystem (Cermeño et al., 2005b). Nitrate fluxes were transformed to cumulative nitrate concentration by dividing over the water column depth interval (10–40 m) across which diffusive fluxes were computed (see above). This model assumes that external nitrate supplies are all incorporated into the cells as internal stores, thereby optimizing the use of incoming nitrate fluxes. Then, we calculated Q/Qmin ratios from allometric equations (Edwards et al., 2012; Marañón et al., 2013) relating Qmin to species' cell size, and compared them with estimates of the Q/Qmin ratio obtained under laboratory controlled conditions (Marañón et al., 2013). The intracellular nitrate quotas assigned by the model were consistent with those measured in laboratory microcosms. Finally, we performed Monte Carlo simulations (1000 trials) by randomly selecting size-dependent physiological traits values from the entire range of values, including their statistical variances, in existing literature compilations (Edwards et al., 2012; Marañón et al., 2013). This method provides the entire range of potential solutions.
We conducted a concurrent analysis of primary production rates, biomass and species dominance (a measure of evenness) using a comprehensive ocean dataset. For each individual community we calculated the Berger-Parker dominance index (d), which measures the numerical importance of the most abundant species, d = Nmax/N, where Nmax is the number of individuals in the most abundant species, and N is the total number of individuals in the sample. Because population abundances span several orders of magnitude depending on species cell size, we computed the Berger-Parker dominance index in terms of population C biomass.
The rate of nitrate supply to the euphotic zone (diffusive plus advective) was in the range 0–42 mmol N m−2 d−1 (Figure 1). We differentiated three oceanographic situations based on density, nutrient and chlorophyll profiles throughout the water column namely, winter mixing, spring bloom, and summer upwelling. The February, October, and November samplings were characterized by winter mixing conditions. March and May were characterized by hydrographic conditions typical of spring bloom. The other samplings were considered punctual situations of the upwelling-stratification succession, which dominates during summer in this coastal ecosystem. Phytoplankton carbon biomass and primary production rate (14C-uptake rate in simulated in situ incubations) were in the range 22–460 mg C m−3 and 0.15–53 mg C m−3h−1, respectively, and were congruent with the rates of nitrate supply and the solar radiation dose. Increased sampling effort (1000 ×) with respect to traditional protocols revealed that these phytoplankton communities are twice as diverse as previously estimated (Cermeño et al., 2014). The resulting estimates of species richness were not correlated with primary productivity (calculated as 14C-uptake rate per unit of photosynthetic C biomass, h−1) (Figure 2). On average, >80% of community biomass was accounted for by < 15% of the species present in these communities (Figure 2). This is probably the reason why our analysis finds no relationship between total species richness and productivity.
Figure 2. Relationship between phytoplankton species richness and primary productivity. The number of dominant species (>80% community biomass) and the total number of species are plotted (gray and black dots, respectively). The error bars are the standard deviation. The volume of sampled examined under the microscope was increased three orders of magnitude with respect to traditional procedures.
We first calculated Pexp by allowing dominant species in the communities (>80% of carbon biomass) to increase their intracellular nitrate stores. In doing so, we assume that the most dominant species in the communities were those with the highest instantaneous growth rates. In the model, intracellular nitrate quotas are adjusted by the rate of nitrate supply into the euphotic zone, which is computed from estimates of diffusive and advective fluxes based on novel measurements of microstructure turbulence in the water column (Figure 1, see Section Materials and Methods). The resulting quotas were consistent with those measured in laboratory cultures under steady-state and pulsed nitrate supplies (not shown). The estimates of Pexp closely approached Pobs (regression slope: 0.72, 95% confidence interval: 0.57, 0.85) (Student's t-test comparison of slopes p > 0.01) (Figure 3A). We failed to replicate these results by increasing the nitrate stores of non-dominant species only (Figure 3B).
Figure 3. Relationship between observed productivity and expected productivity based on internal nitrates stores assigned (A) to dominant species, (B) to non-dominant species, and (C) randomly. Monte Carlo simulations consider thousands of possible combinations of allometric parameters, including their statistical variances, relating physiological trait values to species' cell size (gray dots). The mean values for each sampling day are also plotted (red dots). Color intensity is proportional to the magnitude of resource supply. Triangles and circles denote samples collected during winter mixing and summer upwelling, respectively. Variability in observed productivity is the standard deviation of the measurements. The dashed line is the 1:1 relationship.
Enhanced nitrate supplies via upwelling, eddy diffusivity, river runoff or atmospheric deposition can increase productivity through selection of species with particular traits but also through changes in the physiological status of the entire community. We call the latter the physiological effect. To quantify this effect, we randomly assigned trait values and nitrate quotas to component species on each simulation (Prandom), thereby preventing the emergence of superior competitors. The analysis shows that this effect explains a relatively small fraction of Pobs (< 20%) during spring bloom and summer upwelling (Figure 3C).
Our estimates of intracellular nitrate quota could be biased by the uncertainty associated with the measurements of nitrate fluxes into the euphotic zone and the extent to which nitrate was allocated among individuals (see Section Materials and Methods). We performed a sensitivity analysis to investigate the effect of varying nitrate stores on the Pexp/Pobs ratio (expressed in percentage). Additionally, we subtracted the contribution percentage accounted for by the physiological effect described above (Prandom/Pobs). The resultant is the percentage contribution to primary productivity due to selection effect (Figure 4). Selection increased with the bulk of nitrate stores considered in the simulations. We calculate that >40% of productivity enhancement during spring and summer was related to selection effect (Figure 4), that is to selection of species with specific physiological traits.
Figure 4. Sensitivity analysis showing the effect of varying ecosystem productivity and internal nitrate stores on (Pexp/Pobs)− (Prandom/Pobs), a measure of selection effect. Contour lines represent the enhancement of productivity (%) associated with selection effect. Data used to compose the figure (small dots) and data derived from field measurements (triangles) are also shown.
Using a comprehensive ocean dataset (Marañón et al., 2014), we found that primary production rates co-varied closely with biomass (Figure 5A). From oligotrophic regions to coastal waters, mean biomass increased by a factor of 20–30, whereas primary production rate increased by a factor of >100 (Figure 5A). The exponent of the fitted line was significantly higher than 1, taking a value of 1.46 (95% confidence interval: 1.35, 1.58) (Student's t-test p < 0.01). This result implies that as microalgal biomass increases, primary production rates increase at a faster rate. Our analysis also shows that this pattern is associated with an increase in community dominance (Figure 5B). Thus, to the extent that this biogeographic pattern arises from selection of productive species, this analysis extends the implications of our results to broad regions of the ocean.
Figure 5. (A) Relationship between primary production rate and phytoplankton C biomass across disparate regions of the ocean. Each data point represents the biomass and primary production rate of a phytoplankton community from data compilations (color dots) and this study (black dots). The error bars are the standard deviation. Lines represent the situation in which primary production rates increase proportional to biomass (dashed), the best fit to the entire dataset (solid) and the best fit to data from this study (dotted). (B) Changes in species' dominance (Berger-Parker dominance index) plotted against community biomass. The pattern suggests that the strength of selection increases with increasing resource availability.
Our analysis provides a new insight into the effect of phytoplankton species richness on marine primary productivity beyond the classical two-dimensional species richness-productivity approach. We find that species selection rather than complementarity controls primary productivity in these marine microbial plankton communities. Differences in the way phytoplankton species use different forms of nitrogen or phosphorus (Alexander et al., 2015), morphological adaptations to different water flow regimes (Cardinale, 2011) and specialization to particular ranges of the underwater light spectrum (Stomp et al., 2004; Behl et al., 2011) have been recognized as potential strategies through which resource specialization and niche partitioning increase community-level productivity. Phytoplankton communities could also benefit from facilitative interactions in subtropical ocean regions, where nitrogen fixers represent a sizeable fraction of the autotrophic plankton community (Karl et al., 2002), significantly contributing to nitrogen budgets in these environments. These evidences support the view that phytoplankton species richness can increase primary productivity through positive interactions among species (i.e., complementarity, facilitation). However, widespread evidence that phytoplankton communities exhibit markedly uneven species-abundance distributions, together with the observation that Pobs were best described by a model in which the dominant species in the community approached their maximum productivities (Figure 3B) suggests that selection effect dominates productivity in marine phytoplankton.
Positive selection occurs when the biomass of the community approaches the monoculture biomass of the most productive species. To first order, the productivity or growth rate of phytoplankton species depends on (i) biophysical constraints, which impose a species-specific maximum growth rate (Chisholm, 1992; Marañón et al., 2013), and (ii) resource availability, which determines the extent to which the growth rate of a species approaches its theoretical maximum. According to this, positive selection operates through changes in community structure and the ability of species to hoard nutrients. A closer inspection of our dataset indicates that the most dominant species of the communities are either of intermediate size and/or vacuolated (e.g., Asterionellopsis spp., Ceratulina pelagica, Chaetoceros spp.) (Table S1). Conversely, prey-predator interactions such as killing-the-winner or hydrodynamical processes such as downwelling events, which deprive non-motile species from access to light, can promote the selection of slow-growing species (negative selection). The outcome of negative selection is a reduction in resource use per unit time and productivity.
A hypothetical decrease in species richness would reduce the likelihood of communities to harbor highly productive species. In theory, this would be unimportant in continuous (chemostat-like) nutrient supply ecosystems, in which communities attain a stationary biomass level that maintains indefinitely resource limitation. However, planktonic ecosystems rarely reach the steady-state, giving rise to changes in selection pressures. Dispersal, habitat connectivity and species interactions facilitate the maintenance of phytoplankton species richness, which guarantees the occurrence of productive, opportunist species in these communities. As a consequence, the processes that maintain phytoplankton species richness contribute to sustain marine primary productivity.
Our analysis is based on concurrent measurements of nitrate fluxes into the euphotic zone and productivity. The internal stores growth model used to estimate expected productivities assumes that there is a time lag between nitrate acquisition and use. We recognize that our estimates of Pexp should have been calculated according to the nitrate fluxes prior to the samplings. However, Ekman transport, calculated from instrumental records, indicates that the hydrodynamical conditions prior to the sampling were relatively similar to those observed during the sampling days (not shown).
Our results point to fundamental differences in the effect of species richness on the productivity of terrestrial and marine planktonic ecosystems. Terrestrial plants, but also seaweeds and biofilm communities, most commonly maximize resource use through specialization and niche partitioning within spatially-structured habitats (Tilman et al., 1996; Bracken and Stachowicz, 2006; Cardinale, 2011). In these ecosystems, species richness increases productivity either primary production per unit of photosynthetic biomass (as defined in this study), resource use efficiency (e.g., Cardinale, 2011) or peak standing crop (e.g., Tilman et al., 1996) through complementarity in resource use among species, which specialize in a particular suite of resources. Conversely, the performance of marine phytoplankton communities depends on transient selection of productive species. This ecological strategy represents a way of temporal complementarity in marine planktonic ecosystems in which physical perturbations (of the same order than the generation time of phytoplankton individuals) repeatedly reset habitat structure, and hence available resources must be consumed as quickly as possible (Steele, 1985). If resource specialization were a common rule in marine phytoplankton communities, environmental variability and the taxonomic randomness of seed inoculums could jeopardize resource use. We conclude that speed triumphs over specificity in marine phytoplankton.
In summary, the enormous diversity of phytoplankton communities and its effect on marine primary productivity can be viewed as a recurrent process of seeding and selection. The fact that selection controls productivity implies that, transiently, specific monocultures would be more productive than mixed species' assemblages (Filstrup et al., 2014). Transient selection of species is the most parsimonious strategy to optimize resource use in unstable, highly dynamic plankton ecosystems. Phytoplankton blooms, often dominated by a single species, are the most obvious manifestation of selection effect. This way of resource use sustains world's wild fish stocks and the oceans' biologically-driven carbon sequestration (Falkowski, 2012).
Overall design of the study: PC. Detailed design and implementation of the study: PC, FF, EM, BM, SV. Interpretation and discussion: All authors. Writing and correcting the paper: All authors.
This research was supported by grants CTM2011-25035, CTM2012-30680, and CGL2013-41256-P from the Spanish Government (SG). PC and SV are supported by Ramon y Cajal contracts from the SG.
The authors declare that the research was conducted in the absence of any commercial or financial relationships that could be construed as a potential conflict of interest.
We thank C. Pedrós-Alió and the reviewers for comments on the manuscript.
The Supplementary Material for this article can be found online at: http://journal.frontiersin.org/article/10.3389/fmars.2016.00173
Alexander, H., Jenkins, B. D., Rynearson, T. A., and Dyhrman, S. T. (2015). Metatranscriptome analyses indicate resource partitioning between diatoms in the field. Proc. Natl. Acad. Sci. U.S.A. 112, E2182–E2190. doi: 10.1073/pnas.1421993112
Behl, S., Donval, A., and Stibor, H. (2011). The relative importance of species diversity and functional group diversity on carbon uptake in phytoplankton communities. Limnol. Oceanogr. 56, 683–694. doi: 10.4319/lo.2011.56.2.0683
Bracken, M. E. S., and Stachowicz, J. J. (2006). Seaweed diversity enhances nitrogen uptake via complementary use of nitrate and ammonium. Ecology 87, 2397–2403. doi: 10.1890/0012-9658(2006)87[2397:SDENUV]2.0.CO;2
Cardinale, B. J. (2011). Biodiversity improves water quality through niche partitioning. Nature 472, 86–89. doi: 10.1038/nature09904
Cermeño, P., Estévez-Blanco, P., Marañón, E., and Fernández, E. (2005a). Maximum photosynthetic efficiency of size-fractionated phytoplankton assessed by 14C uptake and fast repetition rate fluorometry. Limnol. Oceanogr. 50, 1438–1446. doi: 10.4319/lo.2005.50.5.1438
Cermeño, P., Marañón, E., Pérez, V., Serret, P., Fernández, E., and Castro, C. G. (2006). Phytoplankton size structure and primary production in a highly dynamic coastal ecosystem (Ría de Vigo, NW-Spain): Seasonal and short-time scale variability. Estuar. Coast. Shelf Sci. 67, 251–266. doi: 10.1016/j.ecss.2005.11.027
Cermeño, P., Marañón, E., Rodríguez, J., and Fernández, E. (2005b). Large-sized phytoplankton sustain higher carbon-specific photosynthesis than smaller cells in a coastal eutrophic ecosystem. Mar. Ecol. Prog. Ser. 297, 51–60. doi: 10.3354/meps297051
Cermeño, P., Rodríguez-Ramos, T., Dornelas, M., Figueiras, F. G., Marañón, E., Teixeira, I. G., et al. (2013). Species richness in marine phytoplankton communities is not correlated to ecosystem productivity. Mar. Ecol. Prog. Ser. 488, 1–9. doi: 10.3354/meps10443
Cermeño, P., Teixeira, I. G., Branco, M., Figueiras, F. G., and Marañón, E. (2014). Sampling the limits of species richness in marine phytoplankton communities. J. Plankton Res. 36, 1135–1139. doi: 10.1093/plankt/fbu033
Chisholm, S. W. (1992). “Phytoplankton size,” in Primary productivity and Biogeochemical Cycles in the Sea, eds P. G. Falkowski and A. D. Woodhead (New York, NY: Plenum Press), 213–217.
Droop, M. R. (1973). Some thoughts on nutrient limitation in algae. J. Phycol. 9, 264–272. doi: 10.1111/j.1529-8817.1973.tb04092.x
Edwards, K. F., Thomas, M. K., Klausmeier, C. A., and Litchman, E. (2012). Allometric scaling and taxonomic variation in nutrient utilization traits and maximum growth rate of phytoplankton. Limnol. Oceanogr. 57, 554–566. doi: 10.4319/lo.2012.57.2.0554
Falkowski, P. (2012). Ocean science: the power of plankton. Nature 483, S17–S20. doi: 10.1038/483S17a
Filstrup, C. T., Hillebrand, H., Heathcote, A. J., Harpole, W. S., and Downing, J. A. (2014). Cyanobacteria dominance influences resource use efficiency and community turnover in phytoplankton and zooplankton communities. Ecol. Lett. 17, 464–474. doi: 10.1111/ele.12246
Finlay, B. J. (2002). Global dispersal of free-living microbial eukaryote species. Science 296, 1061–1063. doi: 10.1126/science.1070710
Fraga, F. (1981). “Upwelling off the Galician coast, northwest Spain,” in Coastal Upwelling. Coastal and Estuarine Science 1, ed F. A. Richards (Washington, DC: American Geophysical Union), 176–182.
Gotham, I. J., and Rhee, G. Y. (1981). Comparative kinetic studies of nitrate-limited uptake in phytoplankton in continuous culture. J. Phycol. 17, 309–314. doi: 10.1111/j.0022-3646.1981.00309.x
Grasshoff, K., Kremling, K., and Ehrhardt, M. (2007). “Determination of nutrients,” in Methods of Seawater Analysis, eds H. P. Hansen and F. Koroleff (Wiley-VHC Verlag GmbH), 159–228.
Grover, J. P. (1991). Resource competition in a variable environment: phytoplankton growing according to the variable-internal-stores model. Am. Nat. 138, 811. doi: 10.1086/285254
Grover, J. P., and Chrzanowski, T. H. (2004). Limiting resources, disturbance, and diversity in phytoplankton communities. Ecol. Monogr. 74, 533–551. doi: 10.1890/03-4073
Hillebrand, H., Dürselen, C.-D., Kirschtel, D., Pollingher, U., and Zohary, T. (1999). Biovolume calculation for pelagic and benthic microalgae. J. Phycol. 35, 403–424. doi: 10.1046/j.1529-8817.1999.3520403.x
Hooper, D. U., Chapin, F. S., Ewel, J. J., Hector, A., Inchausti, P., Lavorel, S., et al. (2005). Effects of biodiversity on ecosystem functioning: a consensus of current knowledge. Ecol. Monogr. 75, 3–35. doi: 10.1890/04-0922
Huisman, J., and Weissing, F. J. (1999). Biodiversity of plankton by species oscillations and chaos. Nature 402, 407–410. doi: 10.1038/46540
Hutchinson, G. E. (1961). The Paradox of the Plankton. Am. Natural. 95, 137–145. doi: 10.1086/282171
Interlandi, S. J., and Kilham, S. S. (2001). Limiting resources and the regulation of diversity in phytoplankton communities. Ecology 82, 1270–1282. doi: 10.1890/0012-9658(2001)082[1270:LRATRO]2.0.CO;2
Irigoien, X., Huisman, J., and Harris, R. P. (2004). Global biodiversity patterns of marine phytoplankton and zooplankton. Nature 429, 863–867. doi: 10.1038/nature02593
Karl, D., Michaels, A., Bergman, B., Capone, D., Carpenter, E., Letelier, R., et al. (2002). Dinitrogen fixation in the world's oceans. Biogeochemistry 57–58, 47–98. doi: 10.1023/A:1015798105851
Kirk, J. T. O. (1994). Light and Photosynthesis in Aquatic Ecosystems. New York, NY: Cambridge University Press.
Litchman, E., and Klausmeier, C. A. (2008). Trait-based community ecology of phytoplankton. Annu. Rev. Ecol. Evol. Syst. 39, 615–639. doi: 10.1146/annurev.ecolsys.39.110707.173549
Loreau, M., Naeem, S., Inchausti, P., Bengtsson, J., Grime, J. P., Hector, A., et al. (2001). Biodiversity and ecosystem functioning: current knowledge and future challenges. Science 294, 804–808. doi: 10.1126/science.1064088
Marañón, E., Cermeño, P., Huete-Ortega, M., López-Sandoval, D. C., Mouriño-Carballido, B., and Rodríguez-Ramos, T. (2014). Resource supply overrides temperature as a controlling factor of marine phytoplankton growth. PLoS ONE 9:e99312. doi: 10.1371/journal.pone.0099312
Marañón, E., Cermeño, P., López-Sandoval, D. C., Rodríguez-Ramos, T., Sobrino, C., Huete-Ortega, M., et al. (2013). Unimodal size scaling of phytoplankton growth and the size dependence of nutrient uptake and use. Ecol. Lett. 16, 371–379. doi: 10.1111/ele.12052
Margalef, R. (1978). Life-forms of phytoplankton as survival alternatives in an unstable environment. Oceanol. Acta 1, 493–509.
Menden-Deuer, S., and Lessard, E. J. (2000). Carbon to volume relationships for dinoflagellates, diatoms, and other protist plankton. Limnol. Oceanogr. 45, 569–579. doi: 10.4319/lo.2000.45.3.0569
Oakey, N. S. (1982). Determination of the rate of dissipation of turbulent energy from simultaneous temperature and velocity shear microstructure measurements. J. Phys. Oceanogr. 12, 256–271.
Osborn, T. R. (1980). Estimates of the local rate of vertical diffusion from dissipation measurements. J. Phys. Oceanogr. 10, 83–89.
Prandke, H., Holtsch, K., and Stips, A. (2000). MITEC Technology Development: The Microstructure/Turbulence Measuring System MSS. Ispra: Space Applications Institute.
Prandke, H., and Stips, A. (1998). Test measurements with an operational microstructure-turbulence profiler: detection limit of dissipation rates. Aquat. Sci. 60, 191–209. doi: 10.1007/s000270050036
Ptacnik, R., Solimini, A. G., Andersen, T., Tamminen, T., Brettum, P., Lepistö, L., et al. (2008). Diversity predicts stability and resource use efficiency in natural phytoplankton communities. Proc. Natl. Acad. Sci. U.S.A. 105, 5134–5138. doi: 10.1073/pnas.0708328105
Raven, J. A. (1997). “The vacuole: a cost-benefit analysis,” in Advances in Botanical Research, eds D. Sanders, R. A. Leigh, and J. A. Callow (Oxford, UK: Academic Press), 59–86.
Rodríguez-Ramos, T., Dornelas, M., Marañón, E., and Cermeño, P. (2014). Conventional sampling methods severely underestimate phytoplankton species richness. J. Plankton Res. 36, 334–343. doi: 10.1093/plankt/fbt115
Sommer, U. (1985). Comparison between steady state and non-steady state competition: experiments with natural phytoplankton. Limnol. Oceanogr. 30, 335–346. doi: 10.4319/lo.1985.30.2.0335
Steele, J. H. (1985). A comparison of terrestrial and marine ecological systems. Nature 313, 355–358. doi: 10.1038/313355a0
Stomp, M., Huisman, J., de Jongh, F., Veraart, A. J., Gerla, D., Rijkeboer, M., et al. (2004). Adaptive divergence in pigment composition promotes phytoplankton biodiversity. Nature 432, 104–107. doi: 10.1038/nature03044
Tilman, D., Lehman, C. L., and Thomson, K. T. (1997). Plant diversity and ecosystem productivity: theoretical considerations. Proc. Natl. Acad. Sci. U.S.A. 94, 1857–1861. doi: 10.1073/pnas.94.5.1857
Tilman, D., Wedin, D., and Knops, J. (1996). Productivity and sustainability influenced by biodiversity in grassland ecosystems. Nature 379, 718–720. doi: 10.1038/379718a0
Utermöhl, V. H. (1931). Neue Wege in der quantitativen Erfassung des Planktons (Mit besondere Beriicksichtigung des Ultraplanktons). Verh. Int. Verein. Theor. Angew. Limnol. 5, 567–595.
Vallina, S. M., and Simó, R. (2007). Strong relationship between DMS and the solar radiation dose over the global surface ocean. Science 315, 506–508. doi: 10.1126/science.1133680
Wardle, D. A. (1999). Is “sampling effect” a problem for experiments investigating biodiversity-ecosystem function relationships? Oikos 87, 403–407.
Keywords: marine phytoplankton, species richness, primary productivity, resource use, selection effect
Citation: Cermeño P, Chouciño P, Fernández-Castro B, Figueiras FG, Marañón E, Marrasé C, Mouriño-Carballido B, Pérez-Lorenzo M, Rodríguez-Ramos T, Teixeira IG and Vallina SM (2016) Marine Primary Productivity Is Driven by a Selection Effect. Front. Mar. Sci. 3:173. doi: 10.3389/fmars.2016.00173
Received: 25 April 2016; Accepted: 30 August 2016;
Published: 21 September 2016.
Edited by:
Alberto Basset, University of Salento, ItalyReviewed by:
Angel Pérez-Ruzafa, University of Murcia, SpainCopyright © 2016 Cermeño, Chouciño, Fernández-Castro, Figueiras, Marañón, Marrasé, Mouriño-Carballido, Pérez-Lorenzo, Rodríguez-Ramos, Teixeira and Vallina. This is an open-access article distributed under the terms of the Creative Commons Attribution License (CC BY). The use, distribution or reproduction in other forums is permitted, provided the original author(s) or licensor are credited and that the original publication in this journal is cited, in accordance with accepted academic practice. No use, distribution or reproduction is permitted which does not comply with these terms.
*Correspondence: Pedro Cermeño, cGVkcm9jZXJtZW5vQGljbS5jc2ljLmVz
Disclaimer: All claims expressed in this article are solely those of the authors and do not necessarily represent those of their affiliated organizations, or those of the publisher, the editors and the reviewers. Any product that may be evaluated in this article or claim that may be made by its manufacturer is not guaranteed or endorsed by the publisher.
Research integrity at Frontiers
Learn more about the work of our research integrity team to safeguard the quality of each article we publish.