- 1Division of Environmental Impact Assessment, Sálim Ali Centre for Ornithology and Natural History, Coimbatore, Tamil Nadu, India
- 2Division of Conservation Ecology, Sálim Ali Centre for Ornithology and Natural History, Coimbatore, Tamil Nadu, India
- 3Centre for Functional Biodiversity, School of Life Sciences, University of KwaZulu-Natal, Pietermaritzburg, South Africa
Introduction: Riparian habitats in the Western Ghats are vital semi-terrestrial zones playing multi-functional roles in the conservation of freshwater species. In dynamic ecosystems, we have scant knowledge on the interactive role of season and local riparian habitat conditions in driving spatiotemporal habitat use of sympatric semi-aquatic mustelids.
Methodology: We conducted seasonal monitoring of 169-196 riverine segments (250 m) in 2020 and 2021 to measure the sign encounter rates of Asian small-clawed otter (SCL) and smooth-coated otter (SCO) and recorded 29 riparian habitat variables.
Results: Our self-organizing map algorithm characterized alarge multivariate habitat data into six habitat clusters representing a gradient of riparian habitat conditions. The random forest (RF) algorithm identified forest cover, water quality, and substratum as influential factors in high quality habitat. The low-quality habitat with low sign encounter rates or no evidence of otters had anthropized stream buffers, with high proportion of agriculture, weed cover and anthropogenic disturbance as influential factors predicted by the RF algorithm. SCO distribution was restricted to the higher-order streams in close proximity to hydro-power dams. SCL had a comparatively larger spatial distribution in the lower-order streams. Our study shows that rainfall plays a significant role in enhancing the hydrological flow in non-perennial streams and also improves the water quality parameters and the riparian habitat conditions. We found highly variable encounter rates of both species across seasons and habitat quality gradients, however, overall, their mean encounter rates increased with the habitat quality gradient.
Discussion: Our findings showed that relating otter sign encounter rates with fine-scale riparian habitat quality was a useful and practical approach to monitor the sensitivity of sympatric semi-aquatic mustelids towards habitat conditions and simultaneously monitor the riparian ecosystem health, across seasons and years. The long-term persistence of sympatric Asian otters in the Bhavani-Noyyal river basin would depend on the availability of high-quality riparian habitat patches. Our findings emphasize the need to develop comprehensive riparian habitat management plans in the southern Western Ghats which involves restoration of fragmented riparian zones and maintenance of riparian habitat heterogeneity to facilitate freshwater connectivity and movements of sympatric otters.
Introduction
Riparian habitats are linear landscape features along riverine buffers, encompassing the transition zones from aquatic to terrestrial ecosystems (Graziano et al., 2022). The riparian zone plays crucial multi-functional roles through ecosystem stability, maintaining the riverine flow, and also serving as seasonal corridors for wildlife movement (Singh et al., 2021). Their linear structure and proportionately less area coverage, when compared to terrestrial forest ecosystems (Bendix, 1994; Herbert et al., 2010), makes riparian habitat specialists more vulnerable to environmental degradation (Pacini and Harper, 2007). Human activities through the spread of agriculture and invasive weeds have resulted in the degradation of riparian habitats (Nagaraja et al., 2014). Integrated approaches that link habitat quality with species habitat use are typically rare in small mammal ecological studies (Delciellos et al., 2016; Hannibal et al., 2020), yet it is much needed when assessing species-habitat relationships and for developing effective conservation strategies for protection of intact riparian patches (Regolin et al., 2021). The riparian vegetation and physiography interact with the rivers and streams making these ecosystems highly dynamic, therefore continuous monitoring requires ecologists to identify stalwart species that serve as effective indicators of riparian health (Sparrow et al., 2020), through the continuous monitoring of small mammalian species that are dependent on such fluvial ecosystems (Perinchery et al., 2011; Nawab and Hussain, 2012).
Understanding the fine-scale riparian habitat ecology of small mammalian carnivores can be useful for quantifying and predicting ecological changes (Lundy and Montgomery, 2010). Among them, the semi-aquatic mustelids are recognized as sentinels of global environmental change (Basu et al., 2007) due to their short gestation periods and high reproductive rates (Ferguson and Lariviére, 2005). Their linear home-ranges and site-fidelity along densely vegetative riparian zones (Gorman et al., 2006) shows that they are important ecological indicators of riparian habitat quality (Bedford, 2009). Otter populations residing in low quality habitats are generally exposed to environmental pollutants and contaminants that can have demographic and physiological consequences (Huang et al., 2018). Female adults are highly sensitive to riparian habitat conditions during the breeding season (Tolrà et al., 2024) as they avoid sites exposed to high human disturbance. At the population-scale, otters distributed in forested landscapes are much more sensitive to human activities (Kamjing et al., 2017) than populations in human-modified landscapes (Khoo and Lee, 2020). Thus, assessing the quality of riparian habitats through continuous monitoring is essential to understand the long-term persistence of otters (Holland et al., 2019), and its associations with habitat compositional structure and functioning of riparian ecosystems, especially when otters occupy unpredictable environmental conditions (Okes and O'Riain, 2019). Otter sign encounter rates derived from indirect evidence such as spraints, tracks or communal latrine sites are generally linked to the habitat use and resource availability (Perinchery et al., 2011; Basak et al., 2021). Sprainting activity of otters is an important marking behavior linked to the quantity and quality of local habitat features at the marked sites (Medina-Vogel et al., 2003; Prigioni et al., 2005). Otter spraint density may vary with the seasonal habitat use of individuals or groups under prevailing habitat conditions and resource availability (Hung et al., 2004). In some localities spraint density peaked during the summer months (Reuther et al., 2000) than spring and autumn (Hysaj et al., 2013; Almeida et al., 2013). Studies linking otter habitat ecology with riparian habitat quality are prominent in temperate river systems of Europe (Bedford, 2009; Prenda et al., 2001; Tolrà et al., 2024), than tropical river systems in the Global south (Cho et al., 2009; Basnet et al., 2020; Hong et al., 2021). Such linkages are crucial in riverscape monitoring programs where sympatric species of otters are distributed (Kruuk et al., 1994; Raha and Hussain, 2016) as anthropogenic threats vary from large to small rivers (Medina-Vogel et al., 2003; Marcelli and Fusillo, 2009). Thus, it is expected that otter species associated with large and wide rivers would have differential responses to the riparian habitat quality (Hwang and Larivière, 2005) than those associated with smaller or narrower streams (Prakash et al., 2012).
The Western Ghats, a UNESCO World Heritage Site is a global biodiversity hotspot that is becoming increasingly vulnerable to urbanization, over-exploitation of natural resources, pollution, and invasive species, leading to continued degradation of riparian habitats and riverine ecosystems (Iyer, 2010; Kumar et al., 2010; Molur et al., 2011). The riparian habitat heterogeneity along freshwater ecosystems in the Western Ghats supports a rich assemblage of small mammals (Molur and Singh, 2009; Kalle et al., 2013; Wordley et al., 2017; Jelil et al., 2021). Riparian forests inside the Protected Areas (PA/s) of the Western Ghats have better habitat integrity than those distributed outside the PA networks (Prakash et al., 2012). Thus, conservation of riparian forests both inside and outside PAs requires an inclusive approach in landscape and habitat management plans (Broadmeadow and Nisbet, 2004; Graziano et al., 2022). Although, the variation in the habitat structure and physiography of riparian zones varies from large to narrow streams (Merritt, 2021), evidence on their functional roles in terms of habitat quality, both inside and outside PAs can determine the habitat ecology of lesser-known semi-aquatic mustelids (Prakash et al., 2012; Almasieh and Cheraghi, 2022). The Asian small-clawed otter (Aonyx cinereus) (SCL) and smooth-coated otter (Lutrogale perspicillata) (SCO) are flagship riparian-obligates with a sympatric distribution range in the Western Ghats (Shenoy et al., 2006; Raha and Hussain, 2016; Krupa et al., 2017). Otters are vulnerable to poaching (Meena, 2002; Trivadi and Patel, 2022), illegal trafficking for pelts and oil (Gomez et al., 2016; Savage, 2022), and anthropogenic disturbance such as trapping and snaring at active holts (Moun et al., 2024). Data from WWF TRAFFIC study revealed 161 otter seizures, including 79 individuals of SCL and 31 of SCO (Gomez et al., 2016). Both species are classified as “Vulnerable” according to the International Union for Conservation of Nature (IUCN) Red List of Threatened Species (Khoo et al., 2021; Wright et al., 2021). They are listed in Appendix II of CITES and listed in “Schedule I” of the Indian Wildlife (Protection) Act, 1972 (amended to date) (The Wildlife (Protection) Amendment Act, 2022).
SCL and SCO populations along the Bhavani-Noyyal river in the southern Western Ghats are under increasing pressure from climate change, dams, and inter-basin water transfer for development (Lannerstad, 2008; Apoorva et al., 2019). This river system is part of the Cauvery river basin which is frequently exposed to fragmentation of riparian zones due to the large hydropower projects, and habitat degradation from agriculture expansion, cattle grazing, industrialization and spread of invasive species (Nagaraja et al., 2014; Palmeirim et al., 2014). Maintaining the riverscape connectivity in the Bhavani-Noyyal riverscape for movement of otter populations from upstream to downstream reaches is crucial for population persistence in the southern Western Ghats. While the importance of riparian habitat features at landscape and regional scales is well-documented (Shenoy et al., 2006; Gupta et al., 2020; Basak et al., 2021), studies demonstrating its relationship with seasonal habitat use patterns of sympatric semi-aquatic mammalian predators is rare in the Western Ghats (Raha and Hussain, 2016). Continuous monitoring of otter sign evidences along fixed riverine routes across seasons, and simultaneously recording fine-scale riparian habitat features aids in a comprehensive understanding of otter habitat preferences, seasonal movement patterns, and the effects of local environmental changes on their distribution (Mason and Macdonald, 2004).
Characterizing fine-scale habitat features for the conservation of threatened semi-aquatic mustelids is an important empirical approach to monitor the ecological conditions and understand linkages with patterns of species-specific spatiotemporal habitat use (Hobohm et al., 2021) and riparian habitat quality (Cho et al., 2009). Monitoring the spatiotemporal habitat use of otters within the characterized habitats could indicate species sensitivity to riparian habitat quality. Various methods have been applied to characterize riparian habitats using remote-sensing data, vegetation, and topography (Gonçalves et al., 2008; Rood et al., 2020; Boothroyd et al., 2021). A widely used technique is the self-organizing map (SOM) (Kohonen, 2001), a heuristic model used to visualize and explore linear and nonlinear relationships in high-dimensional datasets in multiple fields as a data reduction approach. SOM has been implemented in water quality research, e.g., classifying biological and environmental data (Kim et al., 2016; Tison et al., 2005), patterning long-term data (Kangur et al., 2013), monitoring ecosystems (Walley et al., 2000; Aguilera et al., 2001; Sanchez-Martos et al., 2002; Hong et al., 2021), and assessing animal community patterns (Konan et al., 2006; Park et al., 2014). Additionally, this approach was effective in characterizing otter habitats in Asia (Cho et al., 2009; Hong et al., 2021), analyzing anuran communities (Do et al., 2021), understanding biogeographic patterns of freshwater fish communities (Konan et al., 2006), and also examining the socioecological factors (Lyu et al., 2022).
This study applies SOM algorithms to characterize the riparian habitat of sympatric Asian otters and then utilizes a random forest approach to determine the most influential riparian environmental variables that define distinct habitat quality gradients. During the dry and wet seasons of 2020 and 2021, we monitored the spatiotemporal habitat ecology at fine-scales through sign encounter rates of two sympatric otter species, considering the water quality parameters, riverine buffers with land use, river topography, hydrological features and anthropogenic disturbance. We hypothesize that sign encounter rates of SCL and SCO would be strongly associated with habitat quality gradient and that both species would avoid streams exposed to high anthropogenic disturbance. We hypothesize that both species of otters would show varying degrees of sensitivity towards habitat quality across seasons, thereby showing spatiotemporal variation in encounter rates along the Bhavani-Noyyal riverscape.
Materials and methods
Study area
The Coimbatore Reserve Forest (CRF) lies between latitude 10°51’ and 11°27’ and longitude 76° 39’ and 77° 4’ in the southern part of the Nilgiri Biosphere Reserve (Figure 1A). CRF has seven forest ranges and 16 forest beats. Sirumugai and Mettupalayam range adjoins the neighboring districts of Nilgiri and Erode and the remaining five ranges; Karamadai, Perianayackapalayam, Coimbatore Forest range, Boluvampatti, and Madukkarai are at the boundary of Kerala and Tamil Nadu. The region receives rainfall from both southwest (May to August) and the northeast (September to November) monsoon (NEM). The minimum temperature ranges between 17.3 and 24.4°C, and maximum temperature is 29.1 to 36.6°C (Kinattinkara et al., 2022). CRF is drained by two major freshwater rivers, Bhavani and Noyyal, along with the Alaiyar river, and the Kousika river. The Bhavani river originates in the Western Ghats and continues eastwards down till the plains and joins the Cauvery at the Bhavani town. The Bhavani sub-basin is the fourth-largest sub-basin in the Cauvery river basin. Noyyal river originates from the Vellingiri hills in the Western Ghats of Coimbatore. The perennial tributaries of the Bhavani river such as Coonoor river, Kallar pallam, Halurhalla, Thattapallam, Kodungarai pallam, Thekkampatty pallam and Manthorai pallam flows through the CRF (Figure 1B). CRF covers a total of 16 sub-basins (14.2 – 210.7 km2) which are the catchment areas for Bhavani and Noyyal river alongside multiple seasonal and perennial streams that are tributaries of these rivers (Lehner et al., 2008). The elevation ranges from 450 m to 1950 m. CRF in the last century (1916-2015) showed an increasing trend in rainfall received from NEM, including an increase in the number of rainy days (Kokilavani et al., 2020; Sreelash et al., 2018). The riparian ecosystems along the Bhavani river in CRF supports an extremely rich diversity of flora (Manikandan and Balasubramanian, 2018) and fauna (Manikandan and Balasubramanian, 2016; Ramesh et al., 2020). Some of the most common riparian tree species along the fringes of Bhavani and Noyyal rivers are Terminalia arjuna, Mangifera indica, Madhuca longifolia, Diospyros peregrina, Syzygium cumini, and Pongamia pinnata. The forests along the riverine buffers are exposed to lopping, livestock grazing, firewood collection, Non-timber Forest Product collections, and some sand-quarrying, and fishing. Exotic weeds such as Lantana camara and Chromolaena odorata form the shrub-stratum. In addition Cassia tora, Amaranthus spinosus, Cyperus difformis, Datura innoxia, Sida acuta, Prosopis juliflora, Eichhornia crassipes, Pistia stratosis, Solanum nigrum and reed grass such as Typha angustifolia, were also recorded along the riverine buffers.
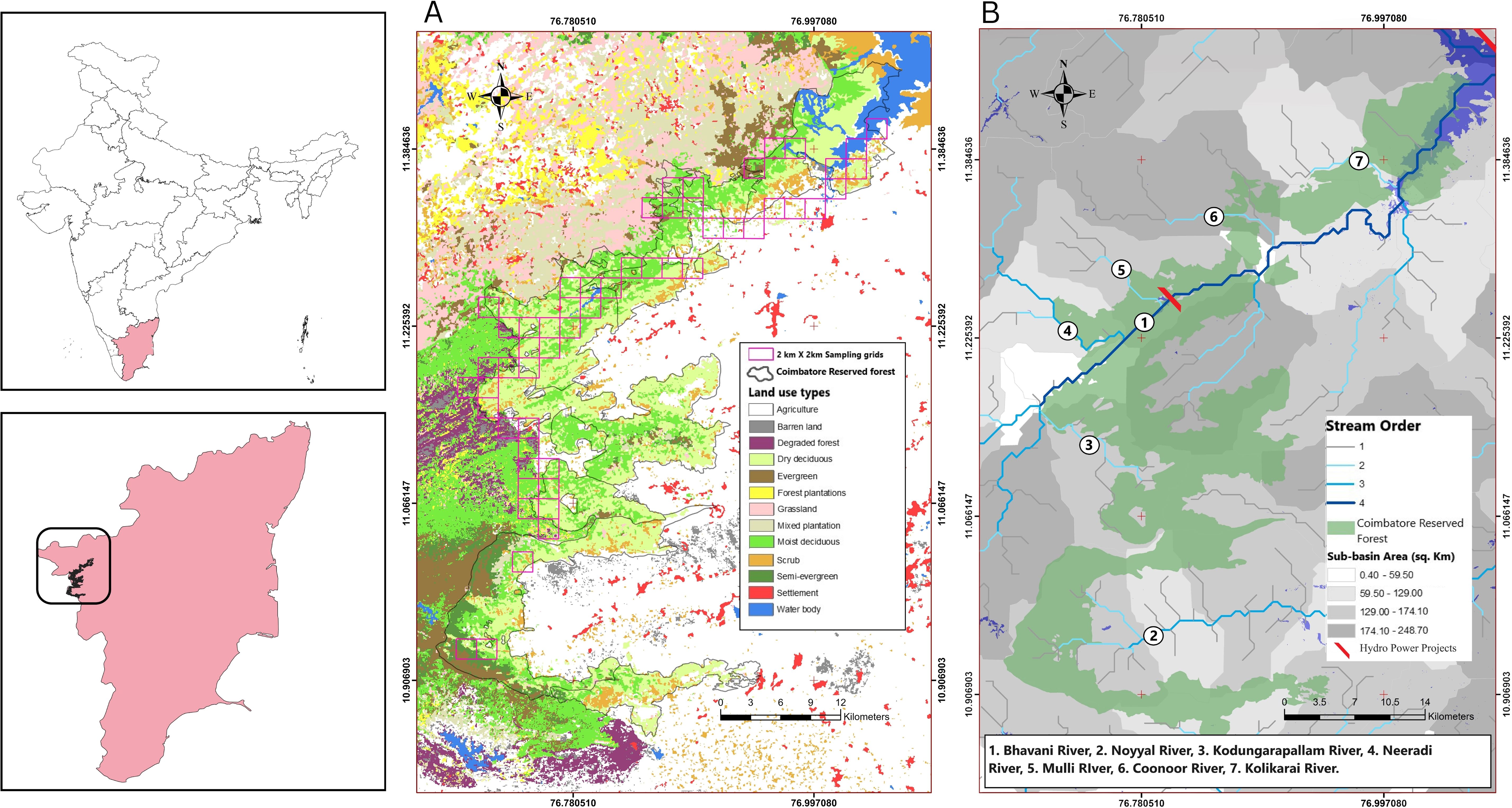
Figure 1. The study region showing the intensive sampling area for otter surveys along the Bhavani-Noyyal river basin in Coimbatore Reserve Forest, Tamil Nadu, southern Western Ghats. (A) The intensive sampling sites showing the 47 2 km x 2 km grids over the land use and vegetation types (reclassified from Roy et al., 2015). (B) The study site showing the river sub-basins from HydroBASINS spatial data (Lehner et al., 2008), stream orders (Strahler, 1952) from HydroRIVERS spatial data and the perennial and non-perennial rivers of the Bhavani-Noyyal river basin.
Field data collection on otters and riparian habitat variables
We identified perennial and seasonal water bodies such as rivers, streams, and other water channels along the Bhavani-Noyyal river sub-basins in CRF. We overlaid 2 km x 2 km grids as sampling units over the hydrology (Lehner et al., 2008) using ArcMap 10.5 (ESRI, 2016; Figure 1A). We chose this as a standard grid size for recording sign evidences of SCL and SCO as this grid size closely matches the median of the home-range estimates of radio-tagged SCO (males 2.1–6.6 km2 and females 2.1–2.7 km2) (Hussain, 1993). We conducted extensive field sampling during dry seasons (March–May 2020 hence forth called as “first dry season” and March–May 2021 hence forth called as “second dry season”) and wet seasons (August–November 2020 hence forth called as “first wet season” and August–November 2021 called as “second wet season”). A total of 47 grids were selected as intensive study sites for further monitoring in both first and second dry and wet seasons. Each grid had a 1 km riverine route, randomly selected and then divided into three to four stream segments of 250 m each (Raha and Hussain, 2016). Thus we sampled 169 stream segments in the first dry season, 196 segments in the second dry season, 177 segments in the first wet season, and 187 segments in the second wet season. We surveyed the segments in early mornings to record indirect signs (mass-latrines, spraints, claw marks, and active holts) of SCL and SCO along rocky, sandy, or vegetated banks (Kruuk et al., 1989; Basak et al., 2021). We recorded all sign evidences of otters using a handheld GARMIN 20x GPS (Garmin Ltd. 2011, Olathe, Kansas, USA) and Locus mobile mapping app (Asamm software, Krhanická, Czech Republic). For species-specific identification, tracks of the SCL were distinguished from SCO by the absence of claw marks and reduced webbing (Mohapatra et al., 2014; Raha and Hussain, 2016). We identified fresh spraints based on shape, diameter, and length (Khan et al., 2014), and their age as fresh (<2 days) or old (>2 days) based on texture, condition, moisture, and odor (Supplementary Figure 1). We considered only fresh sign evidences along 1 km routes to measure species-specific sign encounter rates (number of otter signs/km). Along the same routes, every 250 m, we recorded important fine-scale environmental data on riparian vegetation, water quality, river topography, land cover (100 m and 500 m buffers from the river edge) and anthropogenic disturbance. Details of the field and spatial measurements on 29 riparian habitat variables are shown in Supplementary Table 1.
Data analysis
We applied SOM, an unsupervised learning algorithm used in artificial neural networks also frequently referred to as the Kohonen network (Chon et al., 1996; Kohonen, 1982), to characterize the riparian habitat conditions. SOMs are valuable for exploratory data analysis and widely used for classification, patterning, and visualization (Park et al., 2003; Chon, 2011). The SOM comprises input and output layers with the input layer operating as a flow-through layer for input vectors, and the output layer consisting of a two-dimensional network of neurons arranged in a hexagonal lattice. The 29 habitat variables recorded along 169, 196, 177, and 187 stream segments corresponding to the first dry season, second dry season, first wet season, and second wet season, respectively, were subject to independent SOM learning processes for each season. We tested for multi-collinearity among 29 riparian environmental variables using the Pearson’ correlation tests that showed a lack of significant correlation (r < 0.40). During the learning process of the SOM, we log-transformed all the habitat variables and assigned to the input layer. Details on the learning process for training the data and developing the U matrix is available in the Supplementary Method and Supplementary Figures 2–5. We used the “kohonen” package for developing the SOM (Wehrens and Kruisselbrink, 2018). SOM maps were initially developed using the 5√ formula resulting in a significant number of empty neurons. Following the unsupervised learning algorithm of the SOM, neurons were classified based on their similarity. A unified distance matrix algorithm and hierarchical cluster analysis using Ward’s linkage method, based on Euclidean distance, was applied to the weights of the neurons in the SOM for further clustering of the SOM units (Jain and Dubes, 1988; Park et al., 2003). Subsequently, multi-response permutation procedures (MRPP) were conducted to assess whether significant differences existed among the habitat groups referred as “clusters”, which represent ecologically distinct habitats that capture spatial and environmental heterogeneity within the riverine system.
We then applied the random forest model using the “randomForest” package (Liaw and Wiener, 2002) to assess the importance of riparian habitat variables within each cluster of the SOM. Each stream segment with a cluster was represented as binary data (0 or 1), and the significance of each riparian habitat variable was evaluated through the random forest model, which determines the relationship between various environmental factors and the binary data. Importance was measured by the mean decrease in accuracy (MDA) and mean decrease in Gini (MDG). MDA gauges the variable importance based on accuracy, while MDG quantifies the decrease in impurity of a selected variable as decision trees branch out in the random forest model. Higher MDG values indicate more successful classification within the same category. To validate the model, 70% of the data was randomly selected and applied, while the remaining 30% was used for model accuracy verification (Efron and Tibshirani, 1993). Model accuracy was assessed using the area under the curve (AUC), ranging from 0 to 1. An AUC of 0.5 indicates performance equivalent to random sampling, while 1.0 signifies perfect classification. We tested the differences in riparian habitat parameters among clusters by applying Kruskal-Wallis tests using the “dplyr” package (Wickham and Francois, 2015), followed by pairwise Dunn’s tests with Bonferroni’s correction using the dunnTest() function in the “FSA” package (Neyman and Pearson, 1928).
Results
Clusters of characterized riparian habitats
The SOM learning processes for each season resulted in six distinct clusters (Figures 2A–D). Variables with higher MDA and MDG values were deemed as influential in explaining the cluster (Supplementary Tables 2–5). The MRPP test revealed significant compositional differences among the six clusters across all seasons (first dry season A = 0.137, P = 0.001; second dry season 2021, A = 0.2295, P = 0.001; first wet season A = 0.2722, P = 0.001; second wet season A = 0.1892, P = 0.001).
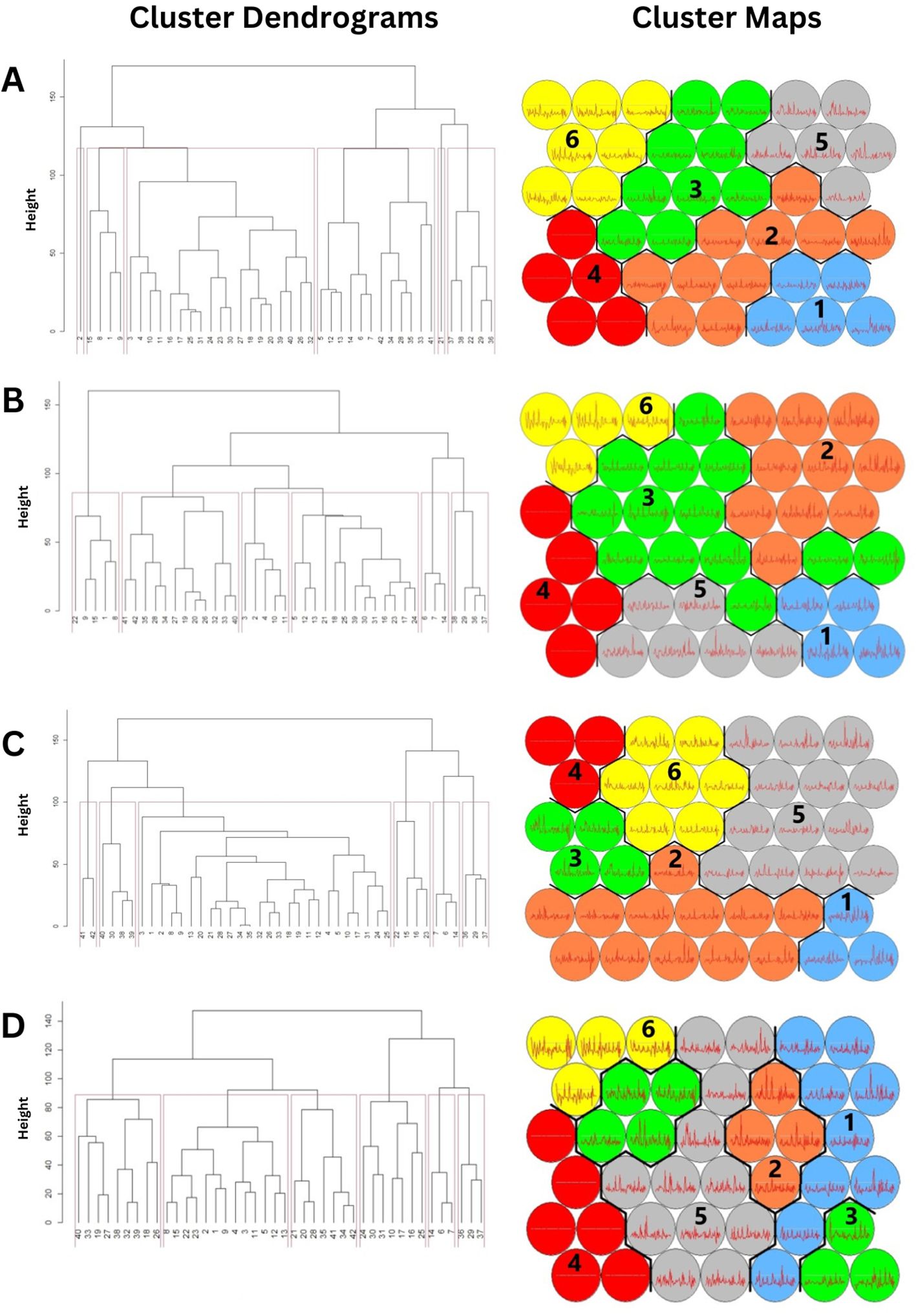
Figure 2. The cluster dendrogram and U matrix of stream segments classified into six clusters through the training process of self-organizing map (SOM). (A) First dry season. (B) Second dry season. (C) First wet season (D) Second wet season.
During the first dry season, cluster 3 comprised of the highest number of stream segments (65), followed by cluster 4 (54). Cluster 1 and 6 comprised of 14 and 26 segments, respectively, while cluster 2 and 5 comprised of the least number of segments, 6 and 5, respectively (Figure 2A). In the second dry season, cluster 3 constituted the highest number of segments (69), followed by cluster 5 (34). Clusters 1, 2, 4, and 6 had relatively less stream segments with 23, 24, 20, and 26, respectively (Figure 2B).
In the first wet season, cluster 1 comprised a high number of stream segments (91), while clusters 2, 3, 4, and 5 had relatively fewer segments with 22, 15, 20, and 19, respectively. Cluster 6 contained 10 stream segments (Figure 2C). The second wet season had cluster 1 with the maximum number of segments (59) followed by cluster 4 and 5 with 34 and 27 segments, respectively. Cluster 2, 3, and 6 had 24, 21, and 22 segments, respectively (Figure 2D).
Influential riparian habitat variables explaining the clusters and geographic distribution of the clusters
Based on the methodology of SOM described previously, 7 nodes were used for the horizontal direction and 6 nodes for the vertical direction for pattern classification of the standardized data. The U-matrix and the variable plains for the input data are shown in Supplementary Figures 2–5. All the influential habitat variables identified in random forest models showed significant differences among the six SOM clusters (Kruskal-Wallis test, P < 0.05) in all seasons (Tables 1–4). The results confirmed the significance of riparian environmental parameters and exhibited the random forest model’s high accuracy with AUC < 0.9 for each of the six clusters in both seasons.
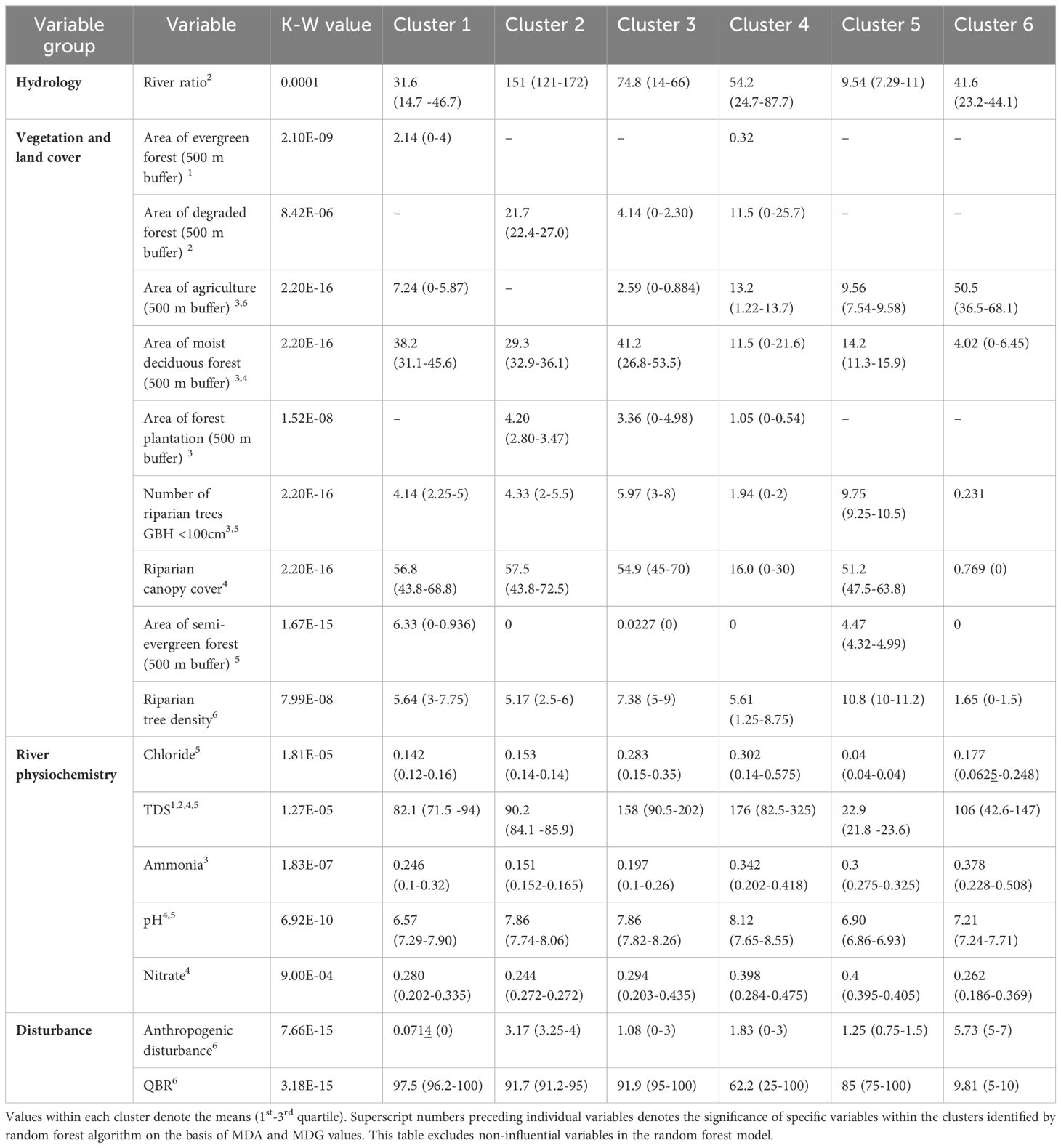
Table 1. Variation in continuous habitat factors (excluding categorical variables) across the six clusters in the first dry season delineated by SOM.
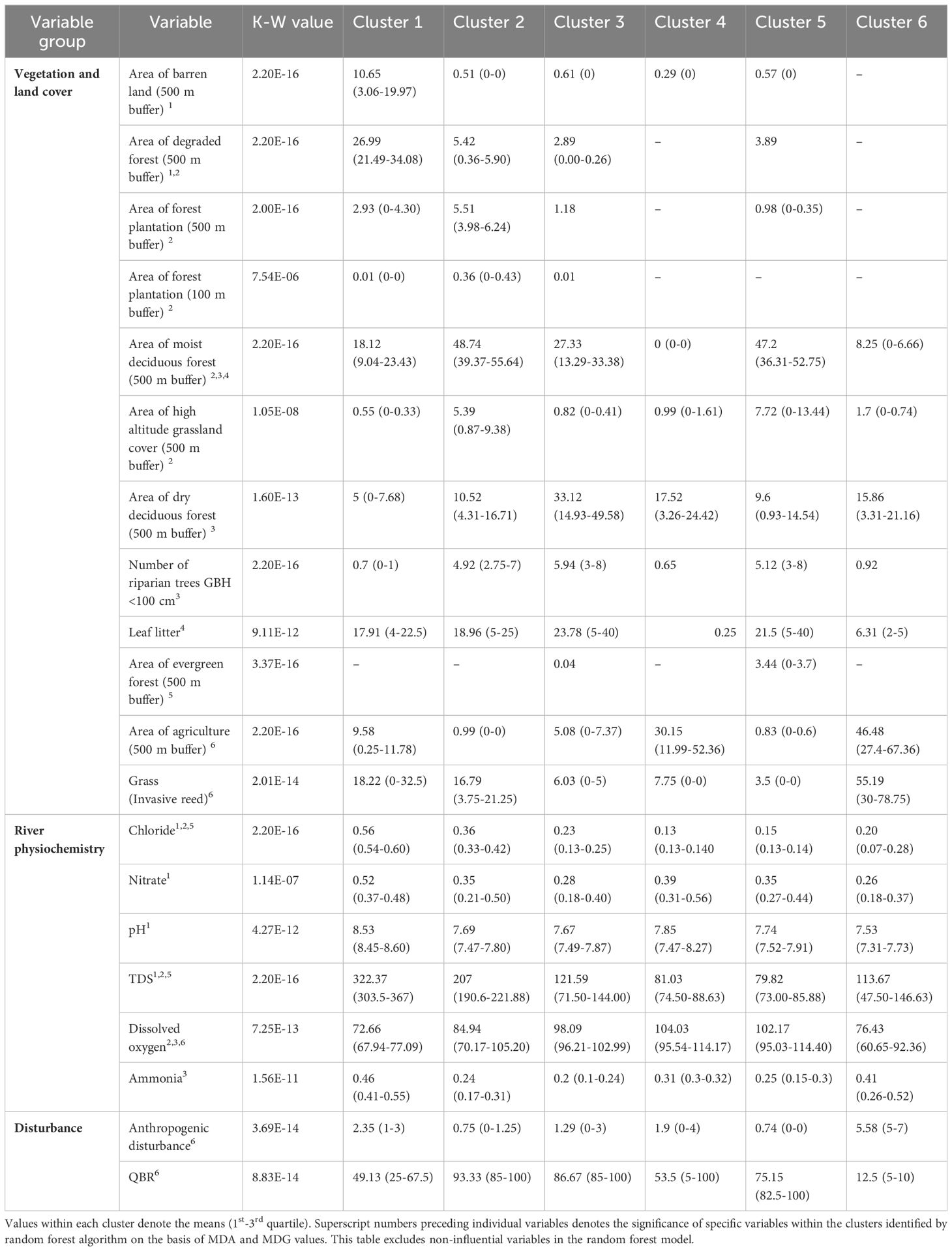
Table 2. Variation in continuous habitat factors (excluding categorical variables) across the six clusters in the second dry season delineated by SOM.
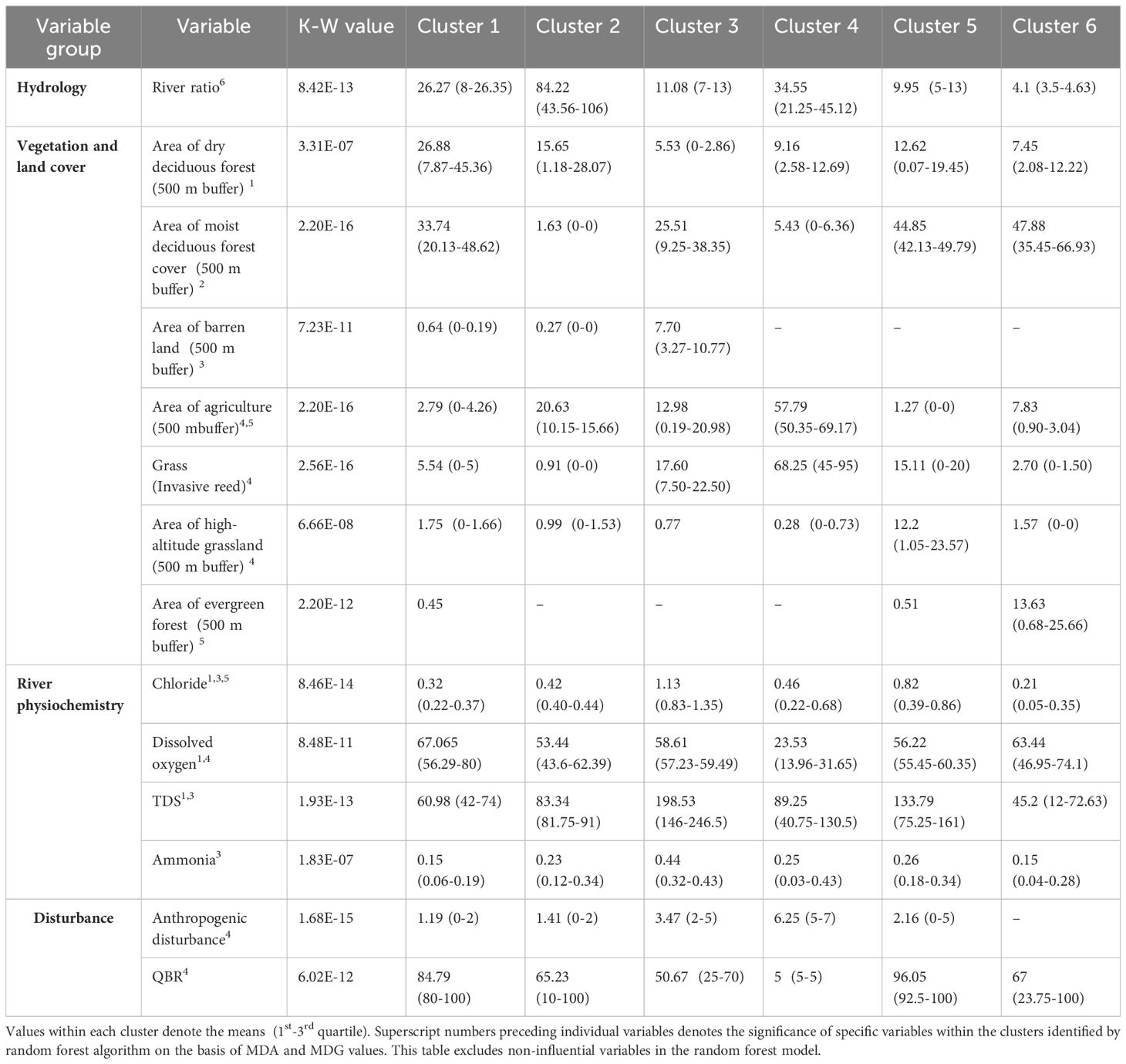
Table 3. Variation in continuous habitat factors (excluding categorical variables) across the six clusters in the first wet season delineated by SOM.
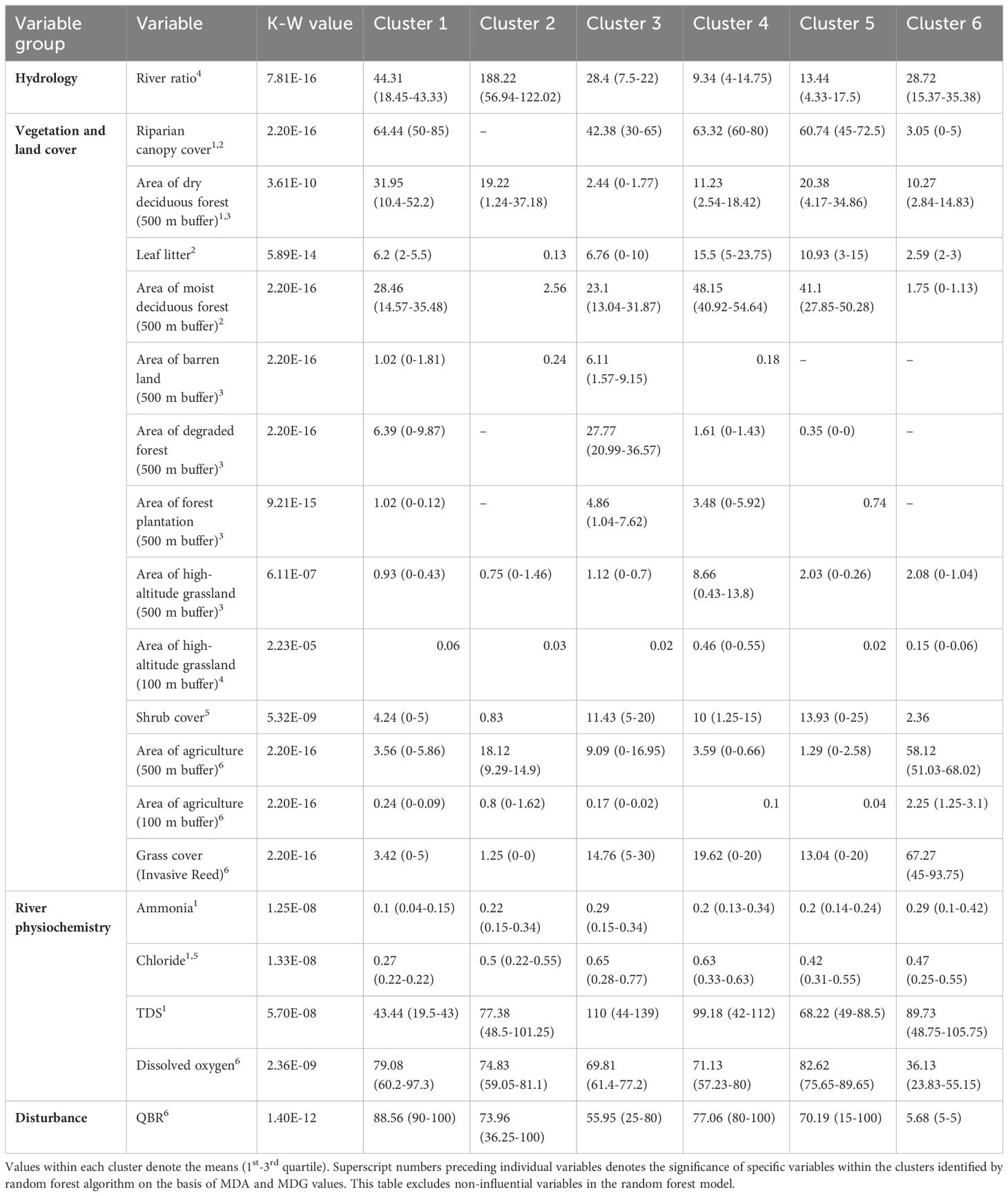
Table 4. Variation in continuous habitat factors (excluding categorical variables) across the six clusters in the second wet season delineated by SOM.
In the first dry season, cluster 1 had total dissolved solids (TDS), stream and ground substrate, stream type and evergreen forest cover as influential factors (Table 1; Supplementary Figure 6A). This cluster had 3rd order streams of Mulli and Kolikurrai, and 2nd order streams of Kallar and Kuttralam. In the first dry season, as these streams moved away from their origins, cluster 1 was replaced by cluster 5 in the Kuttralam stream, cluster 2 in the Mulli stream, and cluster 3 in the Kolikurrai stream. A similar trend was observed in subsequent seasons, with a specific cluster accounting for all lower-order streams near their points of origin and shifting as each stream progressed (Figures 3A–D). During the first wet season, cluster 6 shared these factors and geographical locations (Table 3), while cluster 5 exhibited these variables consistently across both second dry and wet seasons (Supplementary Figures 6B, D).
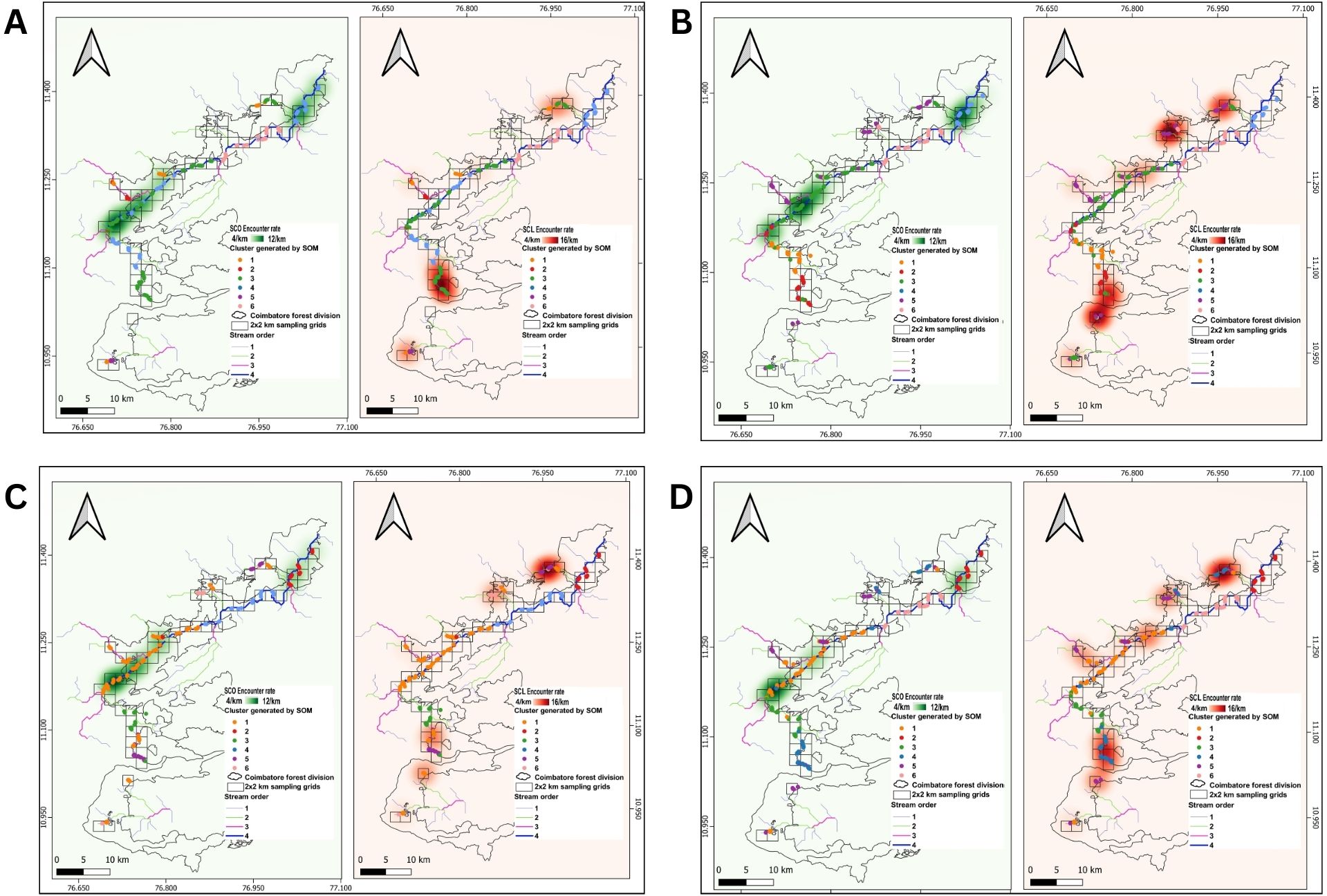
Figure 3. The geographical distribution of six clusters from the characterised stream segments with the sign encounter rates of SCO and SCL along the Bhavani-Noyyal river basin. (A) First dry season (B) Second dry season (C) First wet season (D) Second wet season.
Influential habitat factors for cluster 4 in both dry seasons and cluster 2 in both wet seasons included canopy cover, stream type, ground and stream substrate, and forest cover. These clusters represented the Bhavani river backwaters. Cluster 4 in both dry seasons and cluster 2 in both wet seasons represented the backwaters of Bhavani Sagar and Pillur Dam. Clusters 3 and 4 had sampling segments along the Bhavani river and the 2nd order Kondugarapallam river during the second wet season.
Clusters 5 during first dry and wet seasons, cluster 2 in the second dry season, and cluster 4 in the second wet season had high-altitude grasslands, moist and dry deciduous forest, semi-evergreen forest, TDS and chloride as influential variables (Supplementary Figures 6A–D). Agriculture emerged as influential in these clusters which represented the lower-order streams of Kondugarapallam, Mulli, Neeradi, Kolikurrai and Kuttralam.
Cluster 6 represented sections of the Kuttralam and Coonoor streams during the first wet season (Figures 3A–D). Cluster 1 represented all other seasonal streams, including the Kallar, Neeradi, Mulli, and Vaidehi streams. Cluster 5 primarily explained sampling sites in close proximity to the origin point during the second dry season and wet seasons, except for the Kolikurrai stream during the second wet season, where Cluster 4 accounted for these points. Cluster 1 during the second wet season and cluster 3 in the second dry season, represented the lower-order streams as they flowed from their points of origin to the confluence with the Bhavani river, the principal major river (Figures 3B, D, respectively).
Cluster 6 in both the dry seasons and second wet season, along with cluster 4 in the first wet season, had human disturbance, QBR score, riparian tree density, dissolved oxygen, agriculture, and invasive reed grass as influential factors (Tables 1–4; Supplementary Tables 2–5). These clusters represented segments along the 4th stream order of Bhavani river which were the low-quality habitats.
Cluster 3 in both dry seasons and cluster 1 in both wet seasons had proportion of agriculture, dry and moist deciduous forest cover, ground substrate, water quality and stream type as influential factors (Supplementary Figures 6A–D). The segments in these clusters cover a significant portion of the Bhavani river, from its confluence with the Siruvani and Kondugarapallam rivers in Kotathura town till the junction and then flows through human settlements and agricultural land in Nellithurai village near Mettupalayam town.
In both the dry season clusters 1, 2, whereas cluster 3 in both the wet seasons, displayed degraded forest and barren land in the riverine buffer zones as influential variables. These clusters also exhibited a notable influence of water quality parameters specifically ammonia and chloride in addition to dry deciduous forest cover. These clusters also represented lower-order streams especially a large section of Kondugarapallam river passing through the human settlements and Anaikatti town.
Seasonal otter encounter rates along the riparian habitat quality gradient
In both dry seasons, we recorded 31 SCO evidences, covering 14% of the 169 and 197 surveyed segments in the first and second dry seasons, respectively. During these same seasons, 19% of surveyed segments showed SCL presence, with 50 evidences in the first dry season and 57 in the second. In the first wet season, we found 42 SCO evidences, making 20.9% of the 177 segments positive. However, this significantly decreased in the second wet season, with 31 SCO evidences covering 11.23% of the 187 surveyed segments. In contrast, SCL presence increased from 19.77% in the first wet season to 26.74% in the second, with 74 evidences recorded in the first season and 105 in the second.
In the first dry season, SCL and SCO co-occurred in clusters 1, 3, and 4, however clusters 1 and 3 had higher SCL encounter rates (mean = 3.14/km & 2.09/km, respectively) than SCO (mean = 0.57/km & 0.86/km, respectively). In contrast, cluster 4 had minimal SCL encounter rate (mean = 0.14/km) but higher SCO encounter rate (mean = 1.11/km). Stream segments representing cluster 6 had no evidence of otters. Clusters 2 and 5 exclusively harbored SCL (mean = 0.67/km & 2/km, respectively), with few signs of SCO (Figure 4A). In the first dry season SCO mean encounter rate increased with the habitat quality gradient, however SCL was not recorded in low quality habitats and its encounter rate was higher in clusters representing moderate habitat quality than high quality clusters (Table 5).
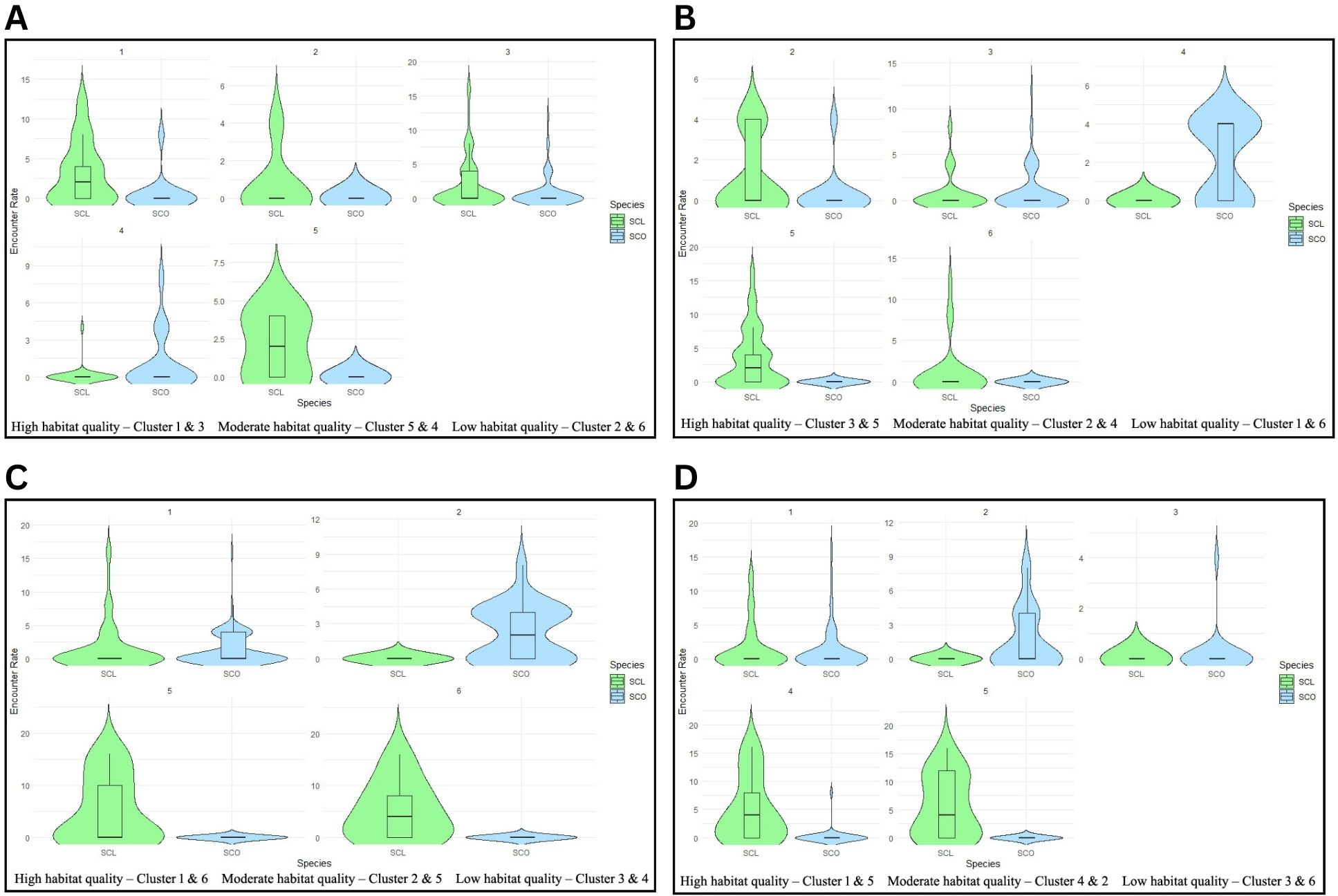
Figure 4. Violin lots showing the variation in SCO and SCL sign encounter rates across the six SOM clusters representing the habitat quality gradient. (A) First dry season. No otter evidence in cluster 6 (B) Second dry season. No otter evidence in cluster 1 (C) First wet season. No otter evidence in cluster 3 and 4. (D) Second wet season. No otter evidence in cluster 6.
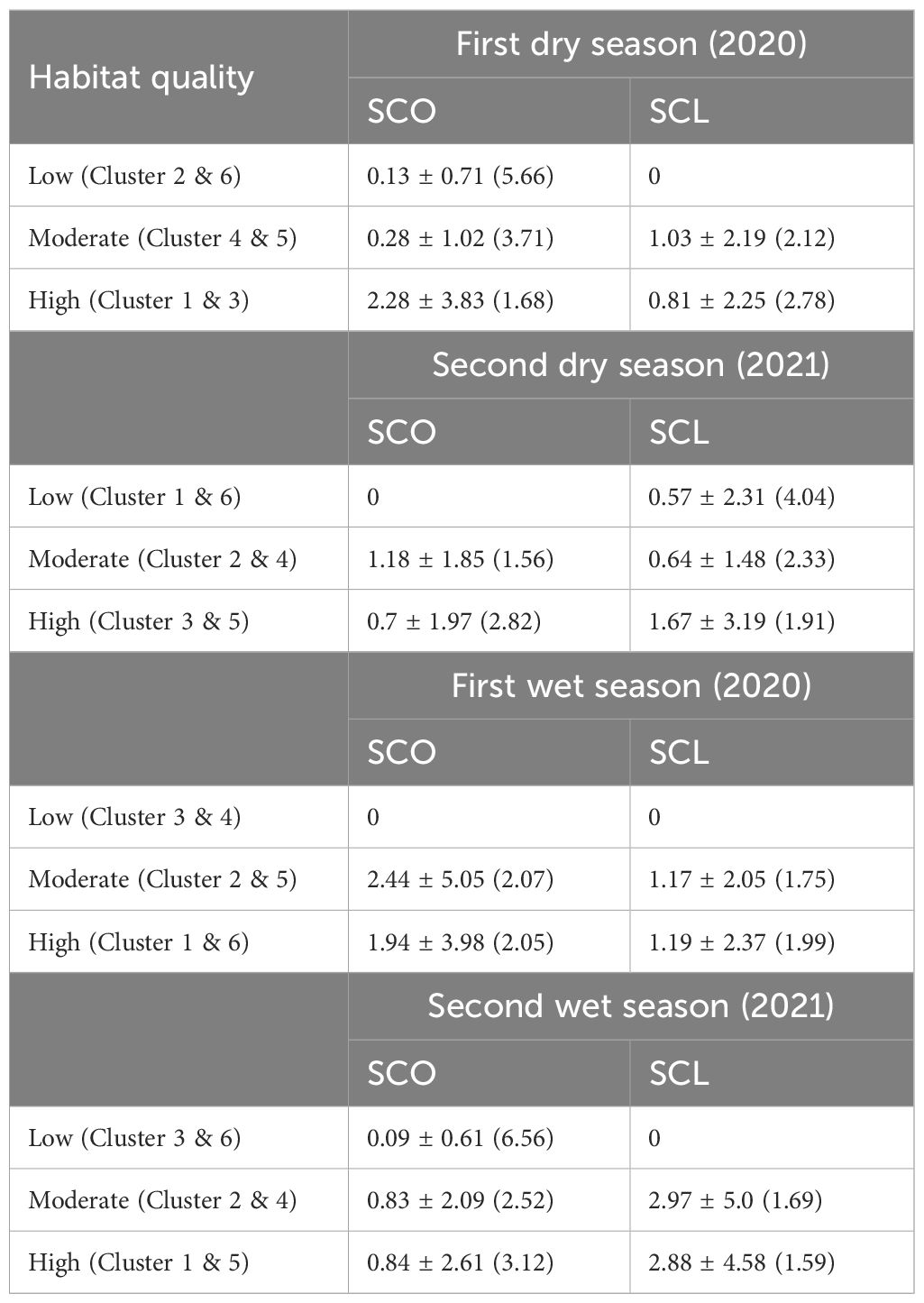
Table 5. SCO and SCL sign encounter rates as the mean number of signs per km ± SD (CV) across the habitat quality gradient in the dry and wet seasons of 2020 and 2021.
In the second dry season, both otter species were distributed in cluster 2 (SCO mean = 0.33/km, SCL mean = 1.16/km) and 3 (SCO mean = 1.04/km, SCL mean = 0.81/km). Cluster 5 (mean = 3.41/km) and 6 (mean = 1.08/km) exclusively had SCL, whereas cluster 4 had only SCO (mean = 2.2/km). Cluster 1 had no evidence of otters (Figure 4B). In the second dry season SCL mean encounter rate increased from low to high quality clusters. In the second dry season and first wet season, SCO was absent in low quality clusters and had higher mean encounter rate in moderate quality clusters than high quality clusters (Table 5).
In the first wet season, SCL and SCO co-occurred along stream segments representing cluster 1 (SCO mean = 1.31/km & SCL 1.58/km). Cluster 2 exclusively had SCO (mean = 2.18/km), while clusters 5 and 6 exclusively had SCL with high encounter rates in both clusters (mean = 5.26/km & 5.2/km). Stream segments classified as low-quality clusters (3 and 4) had no evidence of otters (Figure 4C; Table 5).
During the second wet season, SCL and SCO co-occurred in cluster 1 (SCO mean = 1.22/km & SCL mean = 1.56/km) and 4 (SCO mean = 0.24/km & SCL mean = 5.06/km). Clusters 2 and 3 exclusively had SCO (mean = 1.67/km & 0.24/km, respectively), while cluster 5 exclusively had SCL with high encounter rate (mean = 5.78/km). Cluster 6 had no evidence of otters (Figure 4D). In the first and second wet season SCL was not recorded in low quality clusters and there was no difference in mean encounter rates between moderate and high quality clusters. In the second wet season SCO had lowest encounter rate in low quality habitats while mean encounter rates between moderate and high-quality clusters did not vary much (Table 5).
Discussion
Our study demonstrates the usefulness of machine learning algorithms to characterize otter habitats using a wide range of environmental parameters. Studies in other aquatic systems have shown that the combination of both land use data and local habitat features including physiochemical water parameters are useful indirect measures of resource availability, and provides a complete assessment of local habitat quality to monitor the drivers of spatiotemporal habitat use of otters (Prenda et al., 2001; Bedford, 2009). The SOM approach of characterization was followed by the identification of influential riparian variables explaining the habitat clusters using the random forest models. This step-wise approach allowed the examination of intricate relationships between the land use patterns at the riverine buffer and in-stream water quality parameters for comparative assessment of riparian habitat quality, inside and outside CRF. Based on our hypothesis we found that the sign evidences of SCO and SCL were absent along stream segments exposed to high anthropogenic disturbance in the Southern Western Ghats. Both species showed differential patterns of sensitivity to riparian habitat quality. There were significant changes in water quality parameters among seasons. SCL encounter rates significantly increased in both wet seasons than dry season. The co-occurrence patterns of SCL and SCO are explained by their variable encounter rates within habitat clusters.
Importance of fine-scale riparian habitat characteristics in otter ecology
Our SOM approach effectively differentiated habitat clusters at a fine-scale thereby showing the spatiotemporal variation in SCL and SCO distributions and encounter rates. Similar approach has been applied to characterize habitats for Eurasian otter Lutra lutra habitat into three clusters and otter habitat preference was monitored on a monthly basis for multiple years (Cho et al., 2009). In another study on the same species in South Korea, Hong et al. (2021) applied SOM across four years, demonstrating long-term monitoring of spraint densities and its relationships with population dispersal patterns.
In our study, SCO was restricted to the 4th order streams of the Bhavani river whereas SCL frequently used the 2nd and 3rd.order streams. Their preference for stream order is similar to those reported in earlier surveys in southern India (Perinchery et al., 2011; Prakash et al., 2012; Raha and Hussain, 2016; Moun et al., 2024). SCO preferred streams with non-turbulent flow conditions i.e. slow-flowing streams and pools with stream and ground substrate from boulder to silt and sand. We recorded significant encounter rates of SCO in the Pillur and Bhavani Sagar backwaters along the Bhavani river. These river segments had high habitat quality marked by the water quality, large sandbanks, moderate forest cover and very wide river channels with non-turbulent water flow. Earlier studies reported similar preference for undisturbed backwaters and lakes (Anoop and Hussain, 2004; Utthamapandian et al., 2023). Despite the comparatively less proportion of forest and canopy cover along the riverine buffer these clusters have characteristic pools and loose sand substrate that are preferred by SCO (Moun et al., 2024; Basak et al., 2021). The high SCO encounter rates along sand banks in backwater areas could indicate that these habitats are also important grooming sites, aiding in temperature regulation, fur maintenance, and social bonding among group members. Basak et al. (2021) and Hussain (2013) also recorded extensive use of sand banks by otters for marking and grooming.
SCL was more commonly found along rivers with cascade water flow and boulder strewn banks along the lower-order streams, particularly in the upper reaches and origin points of Kolikurrai, Mulli, Kondugarapallam, Coonoor, Kallar, and Neeradi rivers. SCL habitat preference in our study is comparable with other studies from India (Prakash et al., 2012; Palei et al., 2023) and Southeast Asia where they showed a strong preference for mountain streams, particularly the upper reaches of swiftly flowing streams (Kruuk et al., 1994; Melisch et al., 1994). Similarly, in CRF, clusters with high SCL encounter rates were associated with these streams having significant proportion of evergreen forest, high-altitude grasslands, mature woody trees in the riparian areas and low levels of TDS, chloride, and ammonia, as well as minimal agricultural activity in the riparian zones. River stretches with mature and woody trees were preferred over herb and grass cover along the riverine buffer which is consistent with findings from other studies (Mason, 1995; Prenda and Granado-Lorencio, 1996). For instance, Perinchery et al. (2011) reported that SCL extensively utilized pools over cascades and riffles, with a preference for 2nd and 3rd order streams over 1st order streams. In our study we had records of SCL in camera-traps placed at elevations < 400 m, which contradicts earlier studies that reported the species presence in high-elevation small order streams > 950 m in the Western Ghats (Perinchery et al., 2011; Raha and Hussain, 2016). Camera-traps captured multiple SCL groups, including young pups, in various streams, indicating the existence of resident breeding populations in CRF.
Effects of riparian habitat quality on spatiotemporal habitat use of otter
Habitat quality refers to the environment’s ability to provide sufficient resources and conditions necessary for the survival of individuals and the persistence of populations (Regolin et al., 2021). SOM based clusters with high riparian habitat quality were marked by minimal proportion of agriculture fields in the riverine buffer, a high proportion of forest cover, and low levels of pollutants such as TDS, ammonia, nitrate, and chloride. Clusters of moderate riparian habitat quality were characterized by banks dominated by invasive reed grass, low to medium agricultural activities, elevated levels of ammonia, nitrate, and TDS, but lower riparian tree density, and lower QBR scores, which were associated with low encounter rates. Clusters of low habitat quality were marked by high anthropogenic disturbance, a high proportion of agricultural land, extensive grazing areas, and significant invasive reed cover. Our data showed strong relationships between land-use patterns along the riverine buffer and the riparian habitat characteristics, in both structure and hydrology where the low habitat quality clusters have significant proportion of degraded forest, agriculture, barren land, grass cover, anthropogenic disturbance, and high TDS, chloride and nitrate levels. The moderate and high quality clusters had canopy cover, deciduous and evergreen forest, and low TDS and high river ratio. Most importantly we recorded holts of both species in both moderate and high habitat quality clusters and no holts were found in low habitat quality clusters in all seasons. The low quality habitat was avoided by both species where in some seasons we recorded very low encounter rates or no otter sign evidences. Earlier studies also reported that riparian habitat quality, vegetation cover, structure, and river channel alteration, were positively associated with otter encounter rates (Munné et al., 2003; Prakash et al., 2012; Theng and Sivasothi, 2016) and SCL preferred intact riparian vegetation, even in highly altered riverscapes in Southeast Asia (Pianzin et al., 2021). Although in some areas otters show plasticity in their habitat use, it is mostly attributed to the resident populations in forested habitats, otherwise otters can thrive in human-modified landscapes (Romanowski et al., 2013; Prakash et al., 2012; Dias et al., 2022). Our camera trap data during the study showed that SCO groups consisted of only an adult male, adult female, and 1-2 offsprings per breeding season, indicating probably a lower population density of the species in the CRF compared to other regions where SCO groups are known to be significantly larger (Hussain, 1996; Nawab and Hussain, 2012; Shivram et al., 2023; Moun et al., 2024).
Human-induced alterations in the water quality and riparian habitat structure are major challenges for the persistence of otter populations, emphasizing the critical need to safeguard natural habitat patches and mitigate anthropogenic disturbances in otter habitats. Deterioration in water quality can negatively impact otter distribution by reducing food resources (Prenda et al., 2001; Tüzün and Albayrak, 2005; Bedford, 2009). TDS values were significantly higher in the second dry season and drastically decreased in the second wet season. Moreover, an increase in TDS can result in elevated salinity and conductivity, leading to overall poor water quality, often stemming from human disturbances such as agriculture effluents. Chloride levels increased in the wet season than dry season. Variations in nitrate, ammonia, and chloride among clusters likely stem from sewage and agricultural runoff. Ammonia and nitrate concentrations are often higher in the dry season than in the wet season due to reduced river flow, which limits dilution of pollutants, and increased decomposition rates under higher temperatures, releasing more nitrogen compounds into the water. Additionally, agricultural runoff during the dry season can contribute to significant amounts of these effluents into rivers (Ravi et al., 2021). These factors collectively lead to higher concentrations of ammonia and nitrates during the dry season compared to the wet season. Therefore, monitoring water quality is vital for preserving the integrity of riverine ecosystems, necessitating measures to control water pollution and eutrophication to ensure the viability of otter populations across their range (de Almeida and Pereira, 2017). River ratio was a distinct influential factor in both wet seasons mainly due to the increase in river depth compared to the dry season. In the first wet season, SCO encounter rates were considerably higher in streams with moderate agriculture cover and higher river ratio. The second wet season was marked with higher rainfall with subsequent increase in river flow not only along the main Bhavani river but also along the 2nd and 3rd lower-order streams of Kondugarapallam, Mulli, Neeradi, Kolikurrai and Kuttralam. Particularly, in the second wet season, the sudden rise in the river depth along the Bhavani river would have probably been unsuitable for SCO which is explained by their lowest mean encounter rate. Unpolluted and unregulated rivers with well-preserved riparian vegetation along the banks offering various refuge sites are considered to be optimal otter habitats (Romanowski et al., 2013). The rising human populations and decreasing riparian forest cover have led to isolated populations of otters, forcing them to seek refuge in small, often fragmented riparian patches (Pagacz, 2016; Sepúlveda et al., 2007). SCO may alter their behavior and occupy suboptimal habitats when their natural habitats are disturbed (Moun et al., 2024; Romanowski et al., 2013). When we compare precipitation and rainfall data from the catchments areas of Bhavani-Noyyal rivers between the wet seasons of 2020 and 2021, we found that in 2021, there was significantly higher rainfall in both the NEM and SWM monsoons (Indian Meteorological Department, 2024). Although we found that the riparian habitat quality plays a strong role in driving spatiotemporal encounter rates of both otter species, and the increase in SCL habitat use patterns in the wet season could explain seasonally-associated niche expansion in response to the fluvial hydrological system in the non-perennial lower-order streams.
Spatiotemporal patterns of sign encounter rates in sympatric species of otters
Within a cluster we found that SCL had higher encounter rates than SCO and patterns were vice versa in some other clusters where SCO encounter rates were higher than SCL. Sympatric distributions of both species with spatio-temporal niche separation to avoid direct competition has been recorded in Western Ghats (Raha and Hussain, 2016; Krupa et al., 2017; Narasimmarajan et al., 2023). Streams nestled in non-forested landscapes outside CRF boundary that lacked evidence of both species of otters in both seasons, had low riparian habitat quality and some riverine buffers had only agriculture cover marked with high anthropogenic pressure, livestock grazing, and invasive weeds. Their sensitivity to anthropogenic pressure has been reported in previous studies (Macdonald and Mason, 1983; Prakash et al., 2012). In India SCO sign encounter rates vary from as low as 0.55/km to 7.67/km (Nawab and Hussain, 2012; Basak et al., 2021; Moun et al., 2024) while another study reported SCL sign encounter rates ranging from 2-3.7/500 m (Prakash et al., 2012) and 2-4/km (Perinchery et al., 2011). Due to the few published studies on sign encounter rates of both species, comparisons of encounter rates with existing studies becomes difficult. Nevertheless, their sympatric behavior and habitat use patterns with fine-scale habitat features are comparable.
We found interesting patterns within-season and within-habitat quality clusters where both species had variable encounter rates. The encounter rate of SCO was highest in the first wet season while SCL encounter rates increased drastically in the second wet season. In both wet seasons, we found that moderate and high habitat quality clusters were equally used by SCL while SCO used moderate habitat quality clusters more than high habitat quality clusters in the first wet season. In contrast we found significant variation in encounter rates between moderate and high habitat quality clusters in the dry season for both species. While in the wet season SCL equally used both moderate and high quality clusters. Overall, SCL appeared to show wider spatiotemporal shifts in encounter rates than SCO. These spatiotemporal patterns not only explain strategies of co-occurrence patterns in sympatric otter species but also explain the interactive roles of precipitation, rainfall and hydrology in driving dynamic changes in water quality in mountain rivers of Western Ghats. Although it is certain that the variation in riparian habitat heterogeneity drives coexistence patterns in both otter species, the habitat quality of the fluvial system is seasonally driven by local environmental changes. Prey resources for semi-aquatic mustelids are probably clumped in the dry season providing less opportunities for co-occurrence of sympatric species within-similar habitat quality gradients. The variation in otter encounter rates could also indicate spatiotemporal dispersal patterns from low to high habitat quality clusters thereby supporting the isoleg theory by Pimm and Rosenzweig (1981). Several studies have found strong relationships between otter spraint abundance and otter densities (Kofler et al., 2018), hence the low and high encounter rates measured seasonally and along the habitat quality gradient does indirectly suggests the spatiotemporal shifts in otter activity centres (Sittenthaler et al., 2020).
Holts are essential for otter survival, influencing their access to food, preferred foraging spots, breeding success, and protection from predators (Kruuk et al., 1989). In southern India, SCO tend to avoid areas lacking vegetation and prefer holt sites with well-vegetative river banks (Anoop and Hussain, 2004). A total of four SCO holts were identified in the study area over the course of all four seasons. Two of these holts were active, while the other two were destroyed by fishermen retaliating against the damage otters caused to their fishing nets. Three holts were located in rock crevices, and one was dug by otters under the roots of Terminalia arjuna. In the case of SCL, eight holts were found, all of which were active during the sampling period. Six SCL holts were in rocky crevices, one was under fallen logs and debris brought by floods and settled on the stream side, and one was under Lantana camara thickets. All holt sites of both species were found in high quality habitat clusters suggesting the importance of rocky substratum and densely vegetative river banks, however human activity could be a potential threat to their breeding sites (Supplementary Figure 1).
Conservation importance of riparian habitats and the impact of dams and reservoirs on otters in southern Western Ghats
Our study showed that structural and compositional heterogeneity in microhabitats within riparian zones are crucial for long-term persistence of otter populations. SCL and SCO exhibit distinct habitat preferences which includes not only preference for stream orders but also preference for riverine-edge forest types where SCL used high elevation forest types and SCO used the low to moderate elevation forested buffers. Yet, overall, we found that moderate to high encounter rates were linked with the riparian habitat quality.
The spatial distribution pattern of SCO based on sign evidences, particularly clusters with high sign encounter rates had close proximity to the major reservoirs which are the Bhavani reservoir and Pillur dam. A striking observation in these patterns shows that there is no major spatiotemporal shift in SCO signs across seasons. Large hydropower projects are generally constructed along 4th order streams in the Western Ghats river basins (www.india-wris.nrsc.gov.in). Surveys involving sign detection and camera trapping in the study area indicated the absence of SCO in the Bhavani river below the Pillur dam, despite repeated surveys in all seasons. This indicates that large hydropower projects could be potential barriers to SCO movements. This led to an intriguing trend where the distribution of SCL increased in that particular area with each seasonal sampling (Figures 3A-D). While both otter species coexist in the same habitats, larger species typically dominate the resources within those habitats (Jones and Barmuta, 1998). The smaller, inferior species tend to avoid direct competition and negative interactions with the dominant species (Odden et al., 2010). However, in cases where the dominant species becomes absent from certain areas, the inferior species may occupy and utilize those unoccupied spaces (Crooks and Soulé, 1999). SCL encounter rates also increased along the 2nd order streams in the second wet season and also showed an increase in the spatial distribution of SCL evidences. This indicates that SCL distribution patterns are dynamic and probably dependent on increased stream flow in the second wet season.
Riparian vegetation not only serves as critical habitat for numerous small mammalian species but also fulfills vital ecological functions such as agricultural chemical filtration, erosion prevention, and provision of foraging zones for migratory individuals (Weinberger et al., 2019). The absence of riparian vegetation in Mettupalayam and Sirumugai areas, coupled with the lack of otter evidence in corresponding clusters, underscores the importance of maintaining riparian vegetation. It not only poses a significant threat to riverine health but also jeopardizes otter connectivity with the neigbouring river basins. The 4th stream order of the Bhavani river passing through Sirumugai and Mettupalayam serves as a critical hydrological link connecting otter populations with the Moyar river of the Moyar basin, facilitating movement and dispersal of otter territories. Riparian vegetation stands as one of the last remaining refuges for riparian-obligatory small mammals in disturbed patches, serving as corridors for dispersal and movement in the Western Ghats. The loss of riparian vegetation, coupled with increasing human activities, poses a grave threat to wildlife survival in these habitats, with suitable site availability emerging as a potential limiting factor for otter occurrence (Weinberger et al., 2019). This insight may assist in regulating or conserving these factors to enhance otter connectivity, mitigate pollution and disturbance levels, and prevent local extinctions.
In general, high quality riparian habitat patches along the banks offering various refuge sites are considered to be optimal otter habitats and this was evident from the holts recorded along the Bhavani-Noyyal river basin. While on the other hand, the streams categorized as low and moderate habitat quality could become suboptimal habitats (i.e., significantly transformed by anthropogenic pressure) within the network of high quality habitats. Therefore, identification of several such low to high quality habitats through large-scale characterization provides an essential framework to identify potential corridors for otter movement patterns within and between river basins (Cianfrani et al., 2013). Such long-term evidence-based knowledge concerning habitat requirements of sympatric South Asian otters in tropical streams of the southern Western Ghats and habitat characteristics driving their spatiotemporal variability is crucial for developing effective conservation plans for otters in the region. Our integrated approach enables framing effective conservation strategies to protect and restore critical riparian habitats, ensuring the long-term survival of otter populations in the southern Western Ghats by enhancing riparian habitat connectivity with simultaneous improvements in riverine water quality.
Data availability statement
The datasets presented in this article are not readily available because it is subject to the following licenses/restrictions: Both species of otters have the status of “Schedule I” under the Indian Wildlife Protection Act 1972, we are unable to share the actual coordinates of otter groups and their holts in the public domain. The complete raw data of habitat variables and actual coordinates can be provided to genuine users based on reasonable requests to the corresponding author. Users are requested to directly communicate with the corresponding author to access these raw data sets. Requests to access the datasets should be directed to cmlkZGhpa2FsbGVAZ21haWwuY29t.
Ethics statement
Ethical review and approval was not required for the animal study because our field surveys were based on indirect sign evidences of otters and did not require handling or capturing of otters. To conduct this study, we obtained research permits from the Tamil Nadu Forest Department under Permit No. 42/2019 in Proc. No. 376/2020/D2.
Author contributions
AM: Conceptualization, Data curation, Formal analysis, Funding acquisition, Investigation, Methodology, Software, Validation, Writing – original draft, Writing – review & editing. TR: Conceptualization, Formal analysis, Funding acquisition, Methodology, Project administration, Resources, Software, Supervision, Validation, Writing – review & editing. RK: Writing – review & editing, Conceptualization, Formal analysis, Funding acquisition, Methodology, Project administration, Resources, Software, Supervision, Validation.
Funding
The author(s) declare financial support was received for the research, authorship, and/or publication of this article. The study was supported by the DST-INSPIRE Fellowship (No. DST/INSPIRE/2019/IF190631) to AM from the Department of Science and Technology, Government of India. RK acknowledges the funding support received from the Science and Engineering Research Board (SERB), a statutory body of Department of Science and Technology (DST), Government of India, through the “Start-up Research Grant” (Grant Id: SRG/2019/000995).
Acknowledgments
We are grateful to the Director of Sálim Ali Centre for Ornithology and Natural History for his invaluable support throughout our study. We thank the Tamil Nadu Forest Department for granting research permissions with Permit No. 42/2019 in Proc. No. 376/2020/D2 to conduct field research from 2020 to 2021. Special thanks to the DFO of the Coimbatore Reserve Forest, ACF Mr. Senthil Kumar, Range officers, and anti-poaching watchers Mr. Murgesh, Mr. Vadugan, and Mr. Nagaraj for their assistance in data collection. We also appreciate the efforts of our field assistants Mr. Aravind, Mr. R Giridharan, and Mr. Keny J. Newport. This paper is a partial fulfillment of AM’s PhD at Bharathiar University.
Conflict of interest
The authors declare that the research was conducted in the absence of any commercial or financial relationships that could be construed as a potential conflict of interest.
Generative AI statement
The author(s) declare that no Generative AI was used in the creation of this manuscript.
Publisher’s note
All claims expressed in this article are solely those of the authors and do not necessarily represent those of their affiliated organizations, or those of the publisher, the editors and the reviewers. Any product that may be evaluated in this article, or claim that may be made by its manufacturer, is not guaranteed or endorsed by the publisher.
Supplementary material
The Supplementary Material for this article can be found online at: https://www.frontiersin.org/articles/10.3389/fmamm.2024.1504722/full#supplementary-material
Supplementary Figure 1 | Spraints, tracks, holts and camera-trapped images of SCO and SCL along the Bhavani-Noyyal river basin, southern Western Ghats.
Supplementary Figure 2 | SOM visualization of the distribution of riparian habitat characteristics along stream segments in the first dry season. The U-matrix represents the SOM and is used to visualize the distances between neurons and to identify the cluster structure of the map. Each component plane displays the Z transformed values of one variable along a colour gradient from lowest (red) to the highest values (white) in each map unit.
Supplementary Figure 3 | SOM visualization of the distribution of riparian habitat characteristics along stream segments in the second dry season. The U-matrix represents the SOM and is used to visualize the distances between neurons and to identify the cluster structure of the map. Each component plane displays the Z transformed values of one variable along a colour gradient from lowest (red) to the highest values (white) in each map unit.
Supplementary Figure 4 | SOM visualization of the distribution of riparian habitat characteristics along stream segments in the first wet season. The U-matrix represents the SOM and is used to visualize the distances between neurons and to identify the cluster structure of the map. Each component plane displays the Z transformed values of one variable along a colour gradient from lowest (red) to the highest values (white) in each map unit.
Supplementary Figure 5 | SOM visualization of the distribution of f riparian habitat characteristics along stream segments in the second wet season. The U-matrix represents the SOM and is used to visualize the distances between neurons and to identify the cluster structure of the map. Each component plane displays the Z transformed values of one variable along a colour gradient from lowest (red) to the highest values (white) in each map unit.
Supplementary Figure 6 | Partial dependence plots of the influential riparian habitat variables in the random forest model explaining the six habitat clusters across seasons. (A) First dry season (B) Second dry season (C) First wet season (D) Second wet season.
Supplementary Table 1 | Riparian habitat variables recorded along 250 m stream segments with their range of values or categories in the dry and wet seasons for otter habitat characterization.
Supplementary Table 2 | Variable importance in the random forest model. Mean Decrease Accuracy (MDA) and Mean Decrease Gini (MDG) of the riparian habitat variables as assigned by the random forest model among the six different clusters defined by the self-organizing map in the first dry season. MDA and MDG depends on how well the model was predicted and the overall goodness of fit, respectively.
Supplementary Table 3 | Variable importance in the random forest model. Mean Decrease Accuracy (MDA) and Mean Decrease Gini (MDG) of the riparian habitat variables as assigned by the random forest model among the six different clusters defined by the self-organizing map in the second dry season. MDA and MDG depend upon how well the model predicted and the overall goodness of fit, respectively.
Supplementary Table 4 | Variable importance in the random forest model. Mean Decrease Accuracy (MDA) and Mean Decrease Gini (MDG) of the riparian habitat variables as assigned by the random forest model among the six different clusters defined by the self-organizing map in the first wet season. MDA and MDG depend upon how well the model predicted and the overall goodness of fit, respectively.
Supplementary Table 5 | Variable importance in the random forest model. Mean Decrease Accuracy (MDA) and Mean Decrease Gini (MDG) of the riparian habitat variables as assigned by the random forest model among the six different clusters defined by the self-organizing map in the second wet season. MDA and MDG depend upon how well the model predicted and the overall goodness of fit, respectively.
Supplementary Method | Training the riparian habitat variables using SOM.
References
Aguilera P. A., Frenich A. G., Torres J. A., Castro H., Vidal J. M., Canton M. (2001). Application of the Kohonen neural network in coastal water management: methodological development for the assessment and prediction of water quality. Water. Res. 35, 4053–4062. doi: 10.1016/S0043-1354(01)00151-8
Almasieh K., Cheraghi M. (2022). Habitat suitability, core habitats and diversity hotspots for the conservation of the mustelid species in Iran. Glob. Ecol. Conserv. 36, e02120. doi: 10.1016/j.gecco.2022.e02120
Almeida D., Rodolfo N., Sayer C. D., Copp G. H. (2013). Seasonal use of ponds as foraging habitat by Eurasian otter with description of an alternative handling technique for common toad predation. Folia. Zool 62, 214–221. doi: 10.25225/fozo.v62.i3.a7.2013
Anoop K. R., Hussain S. A. (2004). Factors affecting habitat selection by smooth-coated otters (Lutra perspicillata) in Kerala, India. J. Zool 263, 417–423. doi: 10.1017/S0952836904005461
Apoorva R., Kulranjan R., Pranuti C. L., Vivek M., Srinivasan V. (2019). Contextual Water Targets Pilot Study: Noyyal-Bhavani River Basin (Bengaluru: Ashoka Trust for Research in Ecology and the Environment (ATREE), 84. Available at: https://archived.atree.org/sites/default/files/reports/CBWT_Noyyal_Bhavani_final%20report_ATREE.pdf.
Basak S., Pandav B., Johnson J. A., Hussain S. A. (2021). Resource utilisation by smooth-coated otter in the rivers of Himalayan foothills in Uttarakhand, India. Glob. Ecol. Conserv. 32, e01896. doi: 10.1016/j.gecco.2021.e01896
Basnet A., Ghimire P., Timilsina Y. P., Bist B. S. (2020). Otter research in Asia: Trends, biases and future directions. Glob. Ecol. Conserv. 24, e01391. doi: 10.1016/j.gecco.2020.e01391
Basu N., Scheuhammer A., Bursian S., Elliott J., Rouvinen-Watt K., Chan L. (2007). Mink as a sentinel species in environmental health. Environ. Res. 103, 130–144. doi: 10.1016/j.envres.2006.04.005
Bedford S. J. (2009). The effects of riparian habitat quality and biological water quality on the European Otter (Lutra lutra) in Devon. Biosci. Horiz 2, 125–133. doi: 10.1093/biohorizons/hzp015
Bendix J. (1994). Scale, direction, and pattern in riparian vegetation-environment relationships. Ann. Am. Assoc. Geogr. 84, 652–665. doi: 10.1111/j.1467-8306.1994.tb01881.x
Boothroyd R. J., Nones M., Guerrero M. (2021). Deriving planform morphology and vegetation coverage from remote sensing to support river management applications. Front. Environ. Sci. 9. doi: 10.3389/fenvs.2021.657354
Broadmeadow S., Nisbet T. R. (2004). The effects of riparian forest management on the freshwater environment: a literature review of best management practice. Hydrol. Earth Syst. Sci. 8, 286–305. doi: 10.5194/hess-8-286-2004
Cho H. S., Choi K. H., Lee S. D., Park Y. S. (2009). Characterizing habitat preference of Eurasian river otter (Lutra lutra) in streams using a self-organizing map. J. Limnol 10, 203–213. doi: 10.1007/s10201-009-0275-7
Chon T.-S. (2011). Self-organizing maps applied to ecological sciences. Ecol. Inform. 6, 50–61. doi: 10.1016/j.ecoinf.2010.11.002
Chon T.-S., Park Y. S., Moon K. H., Cha E. Y. (1996). Patternizing communities by using an artificial neural network. Ecol. Model. 90, 69–78. doi: 10.1016/0304-3800(95)00148-4
Cianfrani C., Maiorano L., Loy A., Kranz A., Lehmann A., Maggini R., et al. (2013). There and back again? Combining habitat suitability modelling and connectivity analyses to assess a potential return of the otter to Switzerland. Anim. Conserv. 16, 584–594. doi: 10.1111/acv.12033
Crooks K., Soulé M. (1999). Mesopredator release and avifaunal extinctions in a fragmented system. Nature 400, 563–566. doi: 10.1038/23028
de Almeida L. R., Pereira M. J. R. (2017). Influence of the water quality on the occurrence of the Neotropical otter (Lontra longicaudis) (Olfers 1818) in a human-altered river basin. Mar. Freshw. Res. 69, 122–127. doi: 10.1071/MF17020
Delciellos A. C., Vieira M. V., Grelle C. E., Cobra P., Cerqueira R. (2016). Habitat quality versus spatial variables as determinants of small mammal assemblages in Atlantic Forest fragments. J. Mammal 97, 253–265. doi: 10.1093/jmammal/gyv175
Dias S. J., White P. J. C., Borker A. S., Fernandes N. V. (2022). Habitat selection of smooth-coated otters (Lutrogale perspicillata) in the peri-coastal, urbanised landscape of Goa, India. Mamm. Res. 67, 299–309. doi: 10.1007/s13364-022-00639-1
Do M. S., Son S. J., Choi G., Yoo N., Koo K. S., Nam H. K. (2021). Anuran community patterns in the rice fields of the mid-western region of the Republic of Korea. Glob. Ecol. Conserv. 26, e01448. doi: 10.1016/j.gecco.2020.e01448
ESRI (2016). ArcGIS Desktop: Release 10.5. Redlands, CA, USA: Environmental Systems Research Institute.
Ferguson S., Lariviére S. (2005). “Is mustelid life history different?,” in Martens and Fishers (Martes) in Human-Altered Environments. Eds. Harrison D. J., Fuller A. K., Proulx G. (Springer, Boston, MA), 3–20.
Gomez L., Leupen B. T. C., Theng M., Fernandez K., Savage M. (2016). Illegal Otter Trade: An analysis of seizures in selected Asian countries, (1980–2015) (Petaling Jaya, Selangor, Malaysia: TRAFFIC). Available at: https://www.traffic.org/publications/reports/illegal-otter-trade/.
Gonçalves M. L., Netto M. L. A., Costa J. A. F., Zullo Junior J. (2008). An unsupervised method of classifying remotely sensed images using Kohonen self-organizing maps and agglomerative hierarchical clustering methods. Int. J. Remote Sens 29, 3171–3207. doi: 10.1080/01431160701442146
Gorman T. A., Erb J. D., McMillan B. R., Martin D. J. (2006). Space use and sociality of river otters (Lontra canadensis) in Minnesota. J. Mammal 87, 740–747. doi: 10.1644/05-MAMM-A-337R1.1
Graziano M. P., Deguire A. K., Surasinghe T. D. (2022). Riparian buffers as a critical landscape feature: insights for riverscape conservation and policy renovations. Diversity 14, 172. doi: 10.3390/d14030172
Gupta N., Tiwari V., Everard M., Savage M., Hussain S. A., Chadwick M. A., et al. (2020). Assessing the distribution pattern of otters in four rivers of the Indian Himalayan biodiversity hotspot. Aquat. Conserv. 30, 601–610. doi: 10.1002/aqc.3284
Hannibal W., Cunha N. L. D., Figueiredo V. V., Teresa F. B., Ferreira V. L. (2020). Traits reveal how habitat-quality gradients structure small mammal communities in a fragmented tropical landscape. Austral. Ecol. 45, 79–88. doi: 10.1111/aec.12831
Herbert M. E., McIntyre P. B., Doran P. J., Allan J. D., Abell R. (2010). Terrestrial reserve networks do not adequately represent aquatic ecosystems. Conserv. Biol. 24, 1002–1011. doi: 10.1111/j.1523-1739.2010.01460.x
Hobohm C., Moro-Richter M., Beierkuhnlein C. (2021). “Distribution and habitat affinity of endemic and threatened species: global and European assessment,” in Perspectives for Biodiversity and Ecosystems. Ed. Hobohm C. (Springer, Cham), 233–277.
Holland A. M., Schauber E. M., Nielsen C. K., Hellgren E. C. (2019). River otter and mink occupancy dynamics in riparian systems. J. Wildl. Manage 83, 1552–1564. doi: 10.1002/jwmg.21745
Hong S., Chon T. S., Joo G. J. (2021). Spatial distribution patterns of Eurasian Otter (Lutra Lutra) in association with environmental factors unravelled by machine learning and diffusion kernel method. J. Environ. Inform 37, 130–141. doi: 10.3808/jei.202000443
Huang A. C., Nelson C., Elliott J. E., Guertin D. A., Ritland C., Drouillard K., et al. (2018). River otters (Lontra canadensis) "trapped" in a coastal environment contaminated with persistent organic pollutants: demographic and physiological consequences. Environ. pollut. 238, 306–316. doi: 10.1016/j.envpol.2018.03.035
Hung C. M., Li S. H., Lee L. L. (2004). Faecal DNA typing to determine the abundance and spatial organisation of otters (Lutra lutra) along two stream systems in Kinmen. Anim. Conserv. 7, 301–311. doi: 10.1017/S1367943004001453
Hussain S. A. (1993). Aspects of the Ecology of Smooth-Coated Otter (Lutra perspicillata) in National Chambal Sanctuary (Aligarh: Aligarh Muslim University).
Hussain S. A. (1996). Group size, group structure and breeding in smooth-coated otter Lutra perspicillata Geoffroy (Carnivora, Mustelidae) in National Chambal Sanctuary, India. Mammalia 60, 289–297. doi: 10.1515/mamm.1996.60.2.289
Hussain S. A. (2013). Activity pattern, behavioural activity and interspecific interaction of smooth-coated otter (Lutrogale perspicillata) in National Chambal Sanctuary, India. IUCN Otter Spec. Group Bull. 30, 5–17. Available at: https://www.iucnosgbull.org/Volume30/Hussain_2013.html.
Hwang Y. T., Larivière S. (2005). Lutrogale perspicillata. Mamm. Species 786, 1–4. doi: 10.1644/786.1
Hysaj E., Bego F., Prigioni C., Balestrieri A. (2013). Distribution and marking intensity of the Eurasian otter, Lutra lutra, on the River Drinos (southern Albania). Folia. Zool 62, 115–120. doi: 10.25225/fozo.v62.i2.a5.2013
Indian Meteorological Department (2024). Monsoon Reports. Available online at: https://mausam.imd.gov.in/chennai/monsoon.php (Accessed 21 June 2024).
Iyer R. R. (2010). Governance of water: the legal questions. South. Asian. Surv. 17, 147–157. doi: 10.1177/097152311001700111
Jain A. K., Dubes R. C. (1988). Algorithms for Clustering Data (Upper Saddle River, NJ: Prentice-Hall, Inc).
Jelil S. N., Gaykar A., Girkar N., Ben C., Hayward M. W., Krishnamurthy R. (2021). Mammal persistence along riparian forests in western India within a hydropower reservoir 55 years post construction. Front. Ecol. Evol. 9. doi: 10.3389/fevo.2021.643285
Jones M. E., Barmuta L. A. (1998). Diet overlap and relative abundance of sympatric dasyurid carnivores: a hypothesis of competition. J. Anim. Ecol. 67, 410–421. doi: 10.1046/j.1365-2656.1998.00203.x
Kalle R., Ramesh T., Qureshi Q., Sankar K. (2013). Predicting the distribution pattern of small carnivores in response to environmental factors in the Western Ghats. PloS One 8, e79295. doi: 10.1371/journal.pone.0079295
Kamjing A., Ngoprasert D., Steinmetz R., Chutipong W., Savini T., Gale G. A. (2017). Determinants of smooth-coated otter occupancy in a rapidly urbanizing coastal landscape in Southeast Asia. Mamm. Biol. 87, 168–175. doi: 10.1016/j.mambio.2017.08.006
Kangur K., Kangur P., Ginter K., Orru K., Haldna M., Möls T., et al. (2013). Long-term effects of extreme weather events and eutrophication on the fish community of shallow Lake Peipsi (Estonia/Russia). J. Limnol 72, 30. doi: 10.4081/jlimnol.2013.e30
Khan M. S., Dimri N. K., Nawab A., Ilyas O., Gautam P. (2014). Habitat use pattern and conservation status of smooth-coated otters Lutrogale perspicillata in the Upper Ganges Basin, India. Anim. Biodivers. Conserv. 37, 69–76. Available at: http://abc.museucienciesjournals.cat/?lang=en.
Khoo M., Basak S., Sivasothi N., de Silva P. K., Lubis R. I. (2021). “Lutrogale perspicillata,” in The IUCN Red List of Threatened Species 2021. e.T12427A164579961. doi: 10.2305/IUCN.UK.2021-3.RLTS.T12427A164579961.en (Accessed July 05, 2024).
Khoo M. D., Lee H. (2020). The urban smooth-coated otters Lutrogale perspicillata of Singapore: a review of the reasons for success. Int. Zoo Yearb 54, 60–71. doi: 10.1111/izy.12262
Kim J. Y., Bhatta K., Rastogi G., Muduli P. R., Do Y., Kim D. K., et al. (2016). Application of multivariate analysis to determine spatial and temporal changes in water quality after new channel construction in the Chilika Lagoon. Ecol. Eng. 90, 314–319. doi: 10.1016/j.ecoleng.2016.01.053
Kinattinkara S., Arumugam T., Kuppusamy S., Krishnan M. (2022). Land use/land cover changes of Noyyal watershed in Coimbatore district, India, mapped using remote sensing techniques. Environ. Sci. pollut. Res. 29, 77878–77891. doi: 10.1007/s11356-022-18707-z
Kofler H., Lampa S., Ludwig T. (2018).Fischotterverbreitung und Populationsgrößen in Niederösterreich 2018. In: Endbericht im Auftrag der Abt e i lung Naturschutz des Amtes der Niederösterreichischen Landesregierung, Final Project Report. Available online at: http://www.noe.gv.at/noe/Naturschutz/Fischotter_NOe_2018_fin.pdf (Accessed 24 Nov 2019).
Kohonen T. (1982). Self-organized formation of topologically correct feature maps. Biol. Cybern 43, 59–69. doi: 10.1007/BF00337288
Kokilavani S., Pangayarselvi R., Ramanathan S. P., Dheebakaran G. A., Sathyamoorthy N. K., Maragatham N., et al. (2020). SARIMA modelling and forecasting of monthly rainfall patterns for Coimbatore, Tamil Nadu, India. Curr. J. Appl. Sci. Technol. 39, 69–76. doi: 10.9734/cjast/2020/v39i830594
Konan F. K., Leprieur F., Ouattara A., Brosse S., Grenouillet G., Gourène G., et al. (2006). Spatio-temporal patterns of fish assemblages in coastal West African rivers: a self-organizing map approach. Aquat. Living Resour 19, 361–370. doi: 10.1051/alr:2007006
Krupa H., Borker A., Gopal A. (2017). Photographic record of sympatry between Asian small-clawed otter and smooth-coated otter in the Northern Western Ghats, India. IUCN Otter Spec. Group Bull. 34, 51–57. Available at: https://www.iucnosgbull.org/Volume34/Krupa_et_al_2017.html.
Kruuk H., Kanchanasaka B., O'sullivan S., Wanghongsa S. (1994). Niche separation in three sympatric otters Lutra perspicillata, L. lutra and Aonyx cinerea in Huai Kha Khaeng, Thailand. Biol. Conserv. 69, 115–120. doi: 10.1016/0006-3207(94)90334-4
Kruuk H., Moorhouse A., Conroy J. W. H., Durbin L., Frears S. (1989). An estimate of numbers and habitat preferences of otters Lutra lutra in Shetland, UK. Biol. Conserv. 49, 241–254. doi: 10.1016/0006-3207(89)90046-3
Kumar A., Bisht B. S., Joshi V. D., Singh A. K., Talwar A. (2010). Physical, chemical and bacteriological study of water from rivers of Uttarakhand. J. Hum. Ecol. 32, 169–173. doi: 10.1080/09709274.2010.11906336
Lannerstad M. (2008). Planned and unplanned water use in a closed South Indian Basin. Int. J. Water Resour. Dev. 24, 289–304. doi: 10.1080/07900620701723612
Lehner B., Verdin K., Jarvis A. (2008). New global hydrography derived from spaceborne elevation data. Eos Trans. Am. Geophysical Union 89, 93–94. doi: 10.1029/2008EO100001
Liaw A., Wiener M. (2002). Classification and regression by randomForest. R News 2, 18–22. Available at: http://CRAN.R-project.org/doc/Rnews/.
Lundy M. G., Montgomery W. I. (2010). A multi-scale analysis of the habitat associations of European otter and American mink and the implications for farm scale conservation schemes. Biodivers. Conserv. 19, 3849–3859. doi: 10.1007/s10531-010-9934-6
Lyu R., Clarke K. C., Tian X., Zhao W., Pang J., Zhang J. (2022). Land use zoning management to coordinate the supply–demand imbalance of ecosystem services: A case study in the city belt along the yellow river in ningxia, China. Front. Environ. Sci. 10. doi: 10.3389/fenvs.2022.911190
Macdonald S. M., Mason C. F. (1983). Some factors influencing the distribution of otters (Lutra lutra). Mammal Rev. 13, 1–10. doi: 10.1111/j.1365-2907.1983.tb00259.x
Manikandan P., Balasubramanian P. (2016). Bird diversity of riparian forest in the Nilgiri Biosphere Reserve, India. Indian For 142, 979–988. doi: 10.36808/if/2016/v142i10/85722
Manikandan P., Balasubramanian P. (2018). Diversity and composition of woody vegetation in a riparian forest of Western Ghats, Southern India. Int. J. Ecol. Environ. Sci. 44, 1–9.
Marcelli M., Fusillo R. (2009). Assessing range re-expansion and recolonization of human-impacted landscapes by threatened species: a case study of the otter (Lutra lutra) in Italy. Biodivers. Conserv. 18, 2941–2959. doi: 10.1007/s10531-009-9618-2
Mason C. F. (1995). Habitat quality, water quality and otter distribution. Hystrix It. J. Mamm 7, 195–207. doi: 10.4404/hystrix-7.1-2-4070
Mason C. F., Macdonald S. M. (2004). Growth in otter (Lutra lutra) populations in the UK as shown by long-term monitoring. Ambio 33, 148–152. doi: 10.1579/0044-7447-33.3.148
Medina-Vogel G., Kaufman V. S., Monsalve R., Gomez V. (2003). The influence of riparian vegetation, woody debris, stream morphology and human activity on the use of rivers by southern river otters in Lontra provocax in Chile. Oryx 37, 422–430. doi: 10.1017/S0030605303000784
Meena V. (2002). Otter poaching in Palni Hills. Zoos' Print J, 17, 696–698. Available at: https://zoosprint.org/index.php/zpj/article/view/5977.
Melisch R., Asmoro P., Kusumawardhami L. (1994). Major steps taken towards otter conservation in Indonesia. IUCN Otter Spec. Group Bull. 10, 21–24. Available at: https://www.iucnosgbull.org/Volume10/Melisch_et_al_1994.html.
Merritt D. M. (2021). “Riparian zones,” in Encyclopedia of Inland Waters, 2nd Edn. Ed. Tockner K. (Elsevier, Amsterdam), 276–289.
Mohapatra P. P., Palei H. S., Hussain S. A. (2014). Occurrence of Asian small-clawed otter Aonyx cinereus (Illiger 1815) in Eastern India. Curr. Sci. 107, 367–370. Available at: https://www.currentscience.ac.in/Volumes/107/03/0367.pdf.
Molur S., Singh M. (2009). Non-volant small mammals of the Western Ghats of Coorg District, southern India. J. Threat. Taxa 1, 589–608. doi: 10.11609/JoTT.o2330.589-608
Molur S., Smith K. G., Daniel B. A., Darwall W. R. T. (2011). The Status and Distribution of Freshwater Biodiversity in the Western Ghats, India (Cambridge, UK and Gland, Switzerland: IUCN, and Coimbatore, India: Zoo Outreach Organisation). Available at: https://portals.iucn.org/library/sites/library/files/documents/RL-540-001.pdf.
Moun A., Kumar P., Priya M., Ramesh T., Kalle R. (2024). Multi-scale habitat influences sprainting and group size of a freshwater-obligate smooth-coated otter (Lutrogale perspicillata) in Tungabhadra Otter Conservation Reserve, India. Ecol. Process 13, 12. doi: 10.1186/s13717-024-00492-x
Munné A., Prat N., Solà C., Bonada N., Rieradevall M. (2003). A simple field method for assessing the ecological quality of riparian habitat in rivers and streams: QBR index. Aquat. Conserv. 13, 147–163. doi: 10.1002/aqc.529
Nagaraja B. C., Sunil C., Somashekar R. K. (2014). “Protection of riparian habitats to conserve keystone species with reference to Terminalia arjuna - a case study from South India,” in Biodiversity - The Dynamic Balance of the Planet. Ed. Grillo O. (IntechOpen, London). doi: 10.5772/58355
Narasimmarajan K., Hayward M. W., Palanivel S., Mathai M. T. (2023). Population status and temporal activity pattern of two vulnerable otter species from camera-trapping in the Southern Western Ghats biodiversity hotspots. IUCN Otter Spec. Group Bull. 40, 172–185. Available at: https://iucnosgbull.org/Volume40/Narasimmarajan_et_al_2003.html.
Nawab A., Hussain S. A. (2012). Factors affecting the occurrence of smooth-coated otter in aquatic systems of the Upper Gangetic Plains, India. Aquat. Conserv. 22, 616–625. doi: 10.1002/aqc.2253
Neyman J., Pearson E. S. (1928). On the use and interpretation of certain test criteria for purposes of statistical inference: Part I. Biometrika 20, 175–240. doi: 10.1093/biomet/20A.1-2.175
Odden M., Wegge P., Fredriksen T. (2010). Do tigers displace leopards? If so, why? Ecol. Res. 25, 875–881. doi: 10.1007/s11284-010-0723-1
Okes N. C., O'Riain M. J. (2019). Can opportunistic citizen sightings assist in the monitoring of an elusive, crepuscular mammal in an urban environment? Urban. Ecosyst. 22, 483–492. doi: 10.1007/s11252-019-0829-3
Pacini N., Harper D. M. (2007). “Aquatic, semi-aquatic and riparian vertebrates,” in Tropical Stream Ecology. Ed. Dudgeon D. (Elsevier, Amsterdam), 147–197. doi: 10.1016/B978-012088449-0.50008-X
Pagacz S. (2016). The effect of a major drainage divide on the gene flow of a semiaquatic carnivore, the Eurasian otter. J. Mammal 97, 1164–1176. doi: 10.1093/jmammal/gyw066
Palei H. S., Mohapatra P. P., Hussain S. A. (2023). Habitat selection and diet of the Asian small-clawed otter in Karlapat Wildlife Sanctuary, Odisha, India. Écoscience 30, 17–26. doi: 10.1080/11956860.2023.2165020
Palmeirim A. F., Peres C. A., Rosas F. C. W. (2014). Giant otter population responses to habitat expansion and degradation induced by a mega hydroelectric dam. Biol. Conserv. 174, 30–38. doi: 10.1016/j.biocon.2014.03.015
Park Y. S., Céréghino R., Compin A., Lek S. (2003). Applications of artificial neural networks for patterning and predicting aquatic insect species richness in running waters. Ecol. Model. 160, 265–280. doi: 10.1016/S0304-3800(02)00258-2
Park S. H., Hosoishi S., Ogata K., Kuboki Y. (2014). Clustering of ant communities and indicator species analysis using self-organizing maps. CR Biol. 337, 545–552. doi: 10.1016/j.crvi.2014.07.003
Perinchery A., Jathanna D., Kumar A. (2011). Factors determining occupancy and habitat use by Asian small-clawed otters in the Western Ghats, India. J. Mammal 92, 796–802. doi: 10.1644/10-MAMM-A-323.1
Pianzin A., Wong A., Bernard H. (2021). Riparian reserves serve as a critical refuge for Asian otters (Aonyx cinereus and Lutrogale perspicillata) in oil palm dominated landscapes of Sabah, Malaysian Borneo. IUCN Otter Spec. Group Bull. 38, 133–154. Available at: https://www.iucnosgbull.org/Volume38/Pianzin_et_al_2021.html.
Pimm S. L., Rosenzweig M. L. (1981). Competitors and habitat use. Oikos 37, 1–6. doi: 10.2307/3544067
Prakash N., Mudappa D., Raman T. R. S., Kumar A. (2012). Conservation of the Asian small-clawed otter (Aonyx cinereus) in human-modified landscapes, Western Ghats, India. Trop. Conserv. Sci. 5, 67–78. doi: 10.1177/194008291200500107
Prenda J., Granado-Lorencio C. (1996). The relative influence of riparian habitat structure and fish availability on otter Lutra lutra L. sprainting activity in a small Mediterranean catchment. Biol. Conserv. 76, 9–15. doi: 10.1016/0006-3207(95)00080-1
Prenda J., López-Nieves P., Bravo R. (2001). Conservation of otter (Lutra lutra) in a Mediterranean area: the importance of habitat quality and temporal variation in water availability. Aquat. Conserv. 11, 343–355. doi: 10.1002/aqc.454
Prigioni C., Remonti L., Balestrieri A., Sgrosso S., Priore G., Misin C., et al. (2005). Distribution and sprainting activity of the otter (Lutra lutra) in the Pollino National Park (southern Italy). Ethol. Ecol. Evol. 17, 171–180. doi: 10.1080/08927014.2005.9522606
Raha A., Hussain S. A. (2016). Factors affecting habitat selection by three sympatric otter species in the southern Western Ghats, India. Acta Ecol. Sin. 36, 45–49. doi: 10.1016/j.chnaes.2015.12.002
Ramesh T., Kalle R., Milda D., Gayathri V., Thanikodi M., Ashish K., et al. (2020). Patterns of livestock predation risk by large carnivores in India's Eastern and Western Ghats. Glob. Ecol. Conserv. 24, e01366. doi: 10.1016/j.gecco.2020.e01366
Ravi N. K., Srivastava A., Ram K., Jha P. K. (2021). Nutrient chemistry and eutrophication risk assessment of the Ghaghara river, India. Water Supply 21, 3486–3502. doi: 10.2166/ws.2021.110
Regolin A. L., Oliveira-Santos L. G., Ribeiro M. C., Bailey L. L. (2021). Habitat quality, not habitat amount, drives mammalian habitat use in the Brazilian Pantanal. Landsc. Ecol. 36, 2519–2533. doi: 10.1007/s10980-021-01280-0
Reuther C., Dolch D., Green R., Jahrl J., Jefferies D. J., Krekemeyer A., et al. (2000). Surveying and monitoring distribution and population trends of the Eurasian otter (Lutra lutra). Guidelines and Evaluation of the Standard Method for Surveys as recommended by the European Section of the IUCN/SSC Otter Specialist Group. - Habitat No. 12, Aktion Fischotterschutz e.V., Hankensbüttel, 148.
Romanowski J., Brzeziński M., Żmihorski M. (2013). Habitat correlates of the Eurasian otter Lutra lutra recolonizing Central Poland. Acta Theriol 58, 149–155. doi: 10.1007/s13364-012-0107-8
Rood S. B., Scott M. L., Dixon M., González E., Marks C. O., Shafroth P. B., et al. (2020). Ecological interfaces between land and flowing water: themes and trends in riparian research and management. Wetlands 40, 1801–1811. doi: 10.1007/s13157-020-01392-4
Roy P. S., Behera M. D., Murthy M. S.R., Roy A., Singh S., Kushwaha S. P.S., et al. (2015). New vegetation type map of India prepared using satellite remote sensing: Comparison with global vegetation maps and utilities. Int. J. Appl. Earth Obs. Geoinf. 39, 142–159. doi: 10.1016/j.jag.2015.03.003
Sanchez-Martos F., Aguilera P. A., Garrido-Frenich A., Torres J. A., Pulido-Bosch A. (2002). Assessment of groundwater quality by means of self-organizing maps: application in a semiarid area. Environ. Manage 30, 0716–0726. doi: 10.1007/s00267-002-2746-z
Savage M. (2022). Otters in Northeast India: a review of the sparse available information. IUCN Otter Spec. Group Bull. 39, 81–89. Available at: https://www.iucnosgbull.org/Volume39/Savage_2022.html:~:text=All%20three%20otter%20species%20have,23%20sign%20of%20Eurasian%20Otters.
Sepúlveda M. A., Bartheld J. L., Monsalve R., Gómez V., Medina-Vogel G. (2007). Habitat use and spatial behaviour of the endangered Southern river otter (Lontra provocax) in riparian habitats of Chile: conservation implications. Biol. Conserv. 140, 329–338. doi: 10.1016/j.biocon.2007.08.026
Shenoy K., Varma S., Devi Prasad K. V. (2006). Factors determining habitat choice of the smooth-coated otter, Lutra perspicillata in a South Indian river system. Curr. Sci. 91, 637–643. Available at: https://www.jstor.org/stable/24094370.
Shivram A., Sivasothi N., Hsu C. D., Hodges K. E. (2023). Population distribution and causes of mortality of smooth-coated otters, Lutrogale perspicillata, in Singapore. J. Mammal 104, 496–508. doi: 10.1093/jmammal/gyad007
Singh R., Tiwari A., Singh G. (2021). Managing riparian zones for river health improvement: an integrated approach. Landsc. Ecol. Eng. 17, 3–19. doi: 10.1007/s11355-020-00436-5
Sittenthaler M., Schöll E. M., Leeb C., Haring E., Parz-Gollner R., Hackländer K. (2020). Marking behaviour and census of Eurasian Otters (Lutra lutra) in riverine habitats: what can scat abundances and non-invasive genetic sampling tell us about Otter numbers? Mamm. Res. 65, 191–202. doi: 10.1007/s13364-020-00486-y
Sparrow B. D., Edwards W., Munroe S. E. M., Wardle G. M., Guerin G. R., Bastin J. F., et al. (2020). Effective ecosystem monitoring requires a multi-scaled approach. Biol. Rev. 95, 1706–1719. doi: 10.1111/brv.12636
Sreelash K., Sharma R. K., Gayathri J. A., Upendra B., Maya K., Padmalal D. (2018). Impact of rainfall variability on river hydrology: a case study of Southern Western Ghats, India. J. Geol. Soc India 92, 548–554. doi: 10.1007/s12594-018-1065-9
Strahler A. N. (1952). Hypsometric (area-attitude) analysis of erosional topography. Geol. Soc Am. Bull. 63, 1117–1142. doi: 10.1130/0016-7606(1952)63[1117:HAAOET]2.0.CO;2
The Wildlife (Protection) Amendment Act. (2022). Ministry of Environment and Forests (New Delhi: Government of India). Available at: https://pib.gov.in/PressReleasePage.aspx?PRID=1923681.
Theng M., Sivasothi N. (2016). The smooth-coated otter Lutrogale perspicillata (Mammalia: Mustelidae) in Singapore: establishment and expansion in natural and semi-urban environments. IUCN Otter Spec. Group Bull. 33, 37–49. Available at: https://www.iucnosgbull.org/Volume33/Theng_Sivasothil_2016.html.
Tison J., Park Y. S., Coste M., Wasson J. G., Ector L., Rimet F., et al. (2005). Typology of diatom communities and the influence of hydro-ecoregions: a study on the French hydrosystem scale. Water Res. 39, 3177–3188. doi: 10.1016/j.watres.2005.05.029
Tolrà A., Ruiz-Olmo J., Riera J. L. (2024). Human disturbance and habitat structure drive Eurasian otter habitat selection in heavily anthropized river basins. Biodivers. Conserv. 33, 1683–1710. doi: 10.1007/s10531-024-02891-0
Trivadi K., Patel A. (2022). Smooth-coated otter distribution and report on illegal otter trafficking in Valsad, India. IUCN Otter Spec. Group Bull. 39, 196–201. Available at: https://iucnosgbull.org/Volume39/Trivadi_Patel_2022.html:~:text=Hereby%2C%20we%20present%20distribution%20of,from%20illegal%20trafficking%20in%20Valsad.
Tüzün İ., Albayrak İ. (2005). The effect of disturbances to habitat quality on otter (Lutra lutra) activity in the river Kızılırmak (Turkey): a case study. Turk. J. Zool 29, 327–335. Available at: https://journals.tubitak.gov.tr/zoology/vol29/iss4/7.
Utthamapandian U., Sutaria D., Francis P., Arulmohan R., Alex Kirubakaran A., Louwin Anand D., et al. (2023). Occurrence of smooth-coated otters (Lutrogale perspicillata) in Mudasal Odai coastal backwaters, Tamil Nadu, India. IUCN Otter Spec. Group Bull. 40, 16–25. Available at: https://www.iucnosgbull.org/Volume40/Utthamapandian_et_al_2023.html.
Walley W. J., Martin R. W., O'Connor M. A. (2000). “"Self-organising maps for the classification and diagnosis of river quality from biological and environmental data,",” in Environmental Software Systems: Environmental Information and Decision Support. Eds. Denzer R., Swayne D. A., Purvis M., Schimak G. (Springer, Boston, MA), 27–41. doi: 10.1007/978-0-387-35503-0_4
Wehrens R., Kruisselbrink J. (2018). Flexible self-organizing maps in kohonen 3.0. J. Stat. Softw 87, 1–18. doi: 10.18637/jss.v087.i07
Weinberger I. C., Muff S., Kranz A., Bontadina F. (2019). Riparian vegetation provides crucial shelter for resting otters in a human-dominated landscape. Mamm. Biol. 98, 179–187. doi: 10.1016/j.mambio.2019.09.001
Wickham H., Francois R. (2015). dplyr: A Grammar of Data Manipulation. R package version 0.4.2. Available at: http://CRAN.R-project.org/package=dplyr (Accessed June 18, 2024).
Wordley C. F., Sankaran M., Mudappa D., Altringham J. D. (2017). Bats in the Ghats: agricultural intensification reduces functional diversity and increases trait filtering in a biodiversity hotspot in India. Biol. Conserv. 210, 48–55. doi: 10.1016/j.biocon.2017.03.026
Keywords: freshwater conservation, habitat characterization, random forest, self-organizing map, sign encounter rate, semi-aquatic mammal, Mustelidae, seasonal monitoring
Citation: Moun A, Ramesh T and Kalle R (2024) Riparian habitat quality and seasonality drives spatiotemporal habitat ecology of sympatric Asian otters in southern Western Ghats. Front. Mamm. Sci. 3:1504722. doi: 10.3389/fmamm.2024.1504722
Received: 07 October 2024; Accepted: 20 November 2024;
Published: 06 December 2024.
Edited by:
Kyle Shanebeck, University of California, Santa Barbara, United StatesReviewed by:
John Cm Sha, National Parks Board, SingaporeIgnasi Torre, Granollers Museum of Natural Sciences, Spain
Copyright © 2024 Moun, Ramesh and Kalle. This is an open-access article distributed under the terms of the Creative Commons Attribution License (CC BY). The use, distribution or reproduction in other forums is permitted, provided the original author(s) and the copyright owner(s) are credited and that the original publication in this journal is cited, in accordance with accepted academic practice. No use, distribution or reproduction is permitted which does not comply with these terms.
*Correspondence: Riddhika Kalle, cmlkZGhpa2FsbGVAZ21haWwuY29t