- 1Cognitive Psychology and Didactics of Psychology, Paderborn University, Paderborn, Germany
- 2Natural Language Processing Group, Leibniz University Hannover, Hannover, Germany
The present study aims to understand how metaphors are used in explanations. According to many current theories, metaphors have a conceptual function for the understanding of abstract objects. From this theoretical assumption, we derived the hypothesis that the lower the expertise of the addressee of an explanation, the more metaphors should be used. We tested this hypothesis on a relatively natural data set of 24 published videos with close to 100,000 words overall in which experts explain abstract, mostly scientific concepts to persons of different expertise, varying from minimal (children) to profound (expert). Contrary to our expectations, the frequency of metaphors did not decrease with expertise, but actually increased. This increase could be statistically substantiated with higher differences in expertise. The study contributes to a better understanding of the use of metaphors in actual explanatory processes and how metaphor use depends on contextual factors. It thus supports the expansion of the conceptual and linguistic perspective on metaphors to include the aspect of how metaphors are used by speakers.
1 Introduction
Metaphors have been the subject of intensive research in recent decades, and there is now sufficient evidence that they fulfill a conceptual function: to help people to address unknown and especially abstract topics (Kövecses, 2002, 2021). This idea was prominently proposed by Lakoff and Johnson (1980) and further developed by them (Lakoff, 1993; Lakoff and Johnson, 1999, 2003) and other authors (extended conceptual metaphor theory: Kövecses, 2020; deliberate metaphor theory: Steen, 2008, 2023; blending theory: Fauconnier and Turner, 2002). Metaphors fulfill this conceptual function by a structural relation between the abstract target and a concrete source. If, for instance, the abstract concept of “understanding” is metaphorically equated to “grasping,” the structure of the bodily action of grasping is transferred to the abstract target domain. The metaphor's implications or entailments influence the comprehension of the target concept. Implications or entailments are inferences about the target concept that are facilitated by the metaphor's structure. Entailments differ between metaphors. For instance, the metaphors “grasping,” “seeing,” and “embracing” conceptualize understanding in partly different ways. To illustrate some of the entailments: The action of “grasping” implies a delimited object of a certain size, “seeing” entails a relationship at a distance, and “embracing” implies a social relationship. Based on this definition of metaphor, it is commonly assumed that metaphors are relevant for explanations because they allow to approach new and abstract topics (Thibodeau et al., 2017, p. 1).
In the following sections, we will briefly present the theoretical background of our study, conceptual metaphor theory (1.1) and its limits (1.2), and define our understanding of metaphors (1.3). We will also outline the ways in which metaphors in explanations have been addressed in earlier research (1.4) and then derive the hypotheses for our study (1.5).
1.1 The conceptual function of metaphors and conceptual metaphor theory
As mentioned above, the conceptual function of metaphors was first highlighted by cognitive-linguistic theories in the 1980's (e.g., Lakoff and Johnson, 1980; Kövecses, 1986). These authors reasoned that metaphors are an important and even necessary tool for understanding abstract issues, and that they do so by understanding this abstract domain (partially) in terms of another domain that is typically more concrete. Lakoff and Johnson called this a cross-domain mapping between some concrete, often experience-related and bodily source domain and an abstract target domain. This idea became the starting point of conceptual metaphor theory (CMT). In general, cognitive-linguistic approaches emphasize what may be called the conventionality and naturalness of metaphor usage. Many metaphors for abstract topics are conventional and commonplace and most will go unnoticed as metaphors in typical everyday communication.
Within CMT, there are different explanations of how the meaning transfer that is inherent in metaphors works and what its basic sources are. Lakoff and Johnson (1980, 2003) related conceptual metaphors primarily to bodily experiences and spatial relations, which take the human body and the domain of space as basic sources of metaphorical meanings. Both perspectives persist through later metaphor research but are later complemented by image schemas as sources of metaphorical mappings (e.g., Lakoff, 1987). Image schemas are directly meaningful, gestalt-like multimodal preconceptual structures, which stem from bodily experience and work by resemblance with the domain to which they are transferred. One well-studied prominent image schema is the container (Reddy, 1998).
To exemplify this, we use a sentence from the material we study. In this specific material, an expert explains his love for nanotechnology in the following way: “One of the things I love about nanoscience is it tends to break up the silos between those traditional scientific disciplines.” In this utterance, the phrasal verb “break up” and the noun “silo” are used in a non-literal, metaphorical way. Lakoff and Johnson's approach to metaphor would foreground the experience of separating something into pieces by a bodily effort (body as basic source) or the spatial relation of separated elements (spatial relations “in” and “out” as basic source). Among the image schemas involved are the container, a space with boundaries and an inside and outside that can hold content, the thing as a perceptually bounded object, and path as the trajectory along which an object (here the pieces) moves. All three have (at least) visual and haptic aspects and are assumed to be available preconceptually (e.g., Kövecses, 2021).
One focus of this early research was on identifying the basic structures that determine the meaning that is transferred by the metaphorical mapping. This is why the ideas of embodiment and image schemas, both assumed to be universal, were so prominent in this type of research. Another focus was to show experimentally that the content of conceptual metaphors indeed influences thinking (as summarized by Gibbs, 1994). Researchers also tried to understand how metaphors are actually processed. By now, there are several competing approaches of understanding metaphors (for an overview, see Holyoak and Stamenković, 2018). Less is known about the processes of metaphor production (for exceptions see, e.g., Hussey and Katz, 2009, and, on a more general level, Steen, 2008, 2017, 2023).
1.2 Limits of conceptual metaphor theory
Despite the progress made in CMT and its applications, it has become increasingly clear that many important questions had not been asked by researchers. For instance, linguists such as Langacker (1987) had suggested that grammar is a form of conceptualization, raising the question of how grammar and metaphors interact. To give an example, nouns are less frequently used metaphorically than verbs (Sullivan, 2016, p. 143), but why is this the case? Karsten et al. (2022) developed a method for analyzing verb metaphors that takes their agency (transitivity) into account, both on the lexical and the grammatical level, and thus going beyond CMT. They applied it to metaphors for academic reading (Scharlau et al., 2019) and academic writing (Scharlau et al., 2020) and were able to show that students' metaphors for both academic practices are often of only medium transitivity and therefore imply little agency, which is at odds with the practices themselves.
Additionally, there was no consensus on the level on which the structure transfer implied by metaphors works. Researchers referred to domains (such as journey or space), frames (such as processes, parts, or functions) or scenes, images schemas (such as container, object, or verticality), or mental spaces (concrete instances in communication or cognitive processing; Fauconnier and Turner, 2002). To solve this problem, Kövecses (2017) developed a four-level view, in which image schema, domain, frame, and mental space construct a hierarchy of levels that structure a conceptual metaphor. Furthermore, but in line with the cognitive focus of CMT, there was little research on metaphor and context. Different aspects of local and global context influence metaphors and metaphor usage, as, for instance Kövecses (2015) has shown. Examples are the specific discourse context and the social or cultural context.
Also, research on the actual usage of metaphor was scarce, and a comprehensive theory of metaphor that can explain all its variation, including, besides its linguistic and conceptual dimension, also the rhetorical dimension is still missing (Steen, 2023). While, as the cognitive-linguistic view emphasizes, many metaphors are used in a non-deliberate way, their metaphorical function can also be in the focus of the speaker, e.g., when she uses an unconventional or seemingly unfitting metaphor to capture attention or when, in explanations, the choice, type, or frequency of metaphors is adapted to the addressee. According to deliberate metaphor theory, there is a fundamental difference pertaining to the question whether metaphors are deliberately used as metaphors in communication or not (e.g., Steen, 2017, 2023). It is, among others, related to the distinction between novel and conventional metaphor as well as to the question how metaphor is understood, e.g., by categorization or analogy (Holyoak and Stamenković, 2018).
Finally, and quite surprisingly, there was—as Steen began to point out in 2002 (see also Steen, 2007)—no clear procedure or methodology of metaphor identification in language (and other expressions of conceptual metaphors such as gesture, images or music) and consequently no shared definition of metaphors (Holyoak and Stamenković, 2018; Steen, 2023). By now, this has changed (see, e.g., the methods proposed by Pragglejaz Group, 2007; Steen et al., 2010b; Gaskins et al., 2023), but for the early decades of metaphor research, it was an impediment.
Even though, in the following, we focus on the possible conceptual function of metaphor, we do not want to imply that the cognitive-linguistic approach to metaphor or CMT are the most appropriate approaches to metaphor. By contrast, we agree with the broader stance of deliberate metaphor theory (Steen, 2017, 2023; Steen et al., 2010b) and we will take up some of its distinctions below. Still, our focus is on the conceptual function of metaphors in explanations.
1.3 Operational definition of metaphors
In accordance with Steen (2002), we define as metaphorical any element of language that does not directly relate to what he calls the projected text world but what makes use of a cross-domain mapping. In line with conceptual-metaphor theory, the non-literal meaning needs not be deviant but can be the normal usage of a word.
Steen et al. (2010b, chapters 2.3–2.5) distinguish between direct, indirect and implicit metaphors. Direct metaphors are linguistically marked by a metaphor flag for the mapping such as the preposition “like.” Somebody saying that explaining is like throwing a light on something would use a direct metaphor. The mapping of the target domain to another domain is made explicit by the preposition. An indirect metaphor is present if linguistic marks for the mapping are missing, for example if somebody talks of “giving information.” Here, the fact that there is no literal act of handing something over to another person is not marked linguistically which is why Steen et al. call this an indirect metaphor. Steen at al. also count as a metaphor when an ellipsis or substitution (e.g., a pronoun or a deictic) refers to a metaphorical linguistic element used elsewhere in the text.
Many metaphors are nouns, verbs, adjectives, and adverbs. To give a few examples, an explanation could be described as a “scaffold” (noun), explaining could be related to “illuminating” (verb) or to “throwing bright light on something” (a combination of verb, preposition, adjective, and noun and thus four metaphors). In all these cases, a more concrete, often experience-oriented meaning is used to characterize a more abstract domain. Note that most metaphors in everyday speech are represented by single words (Gaskins et al., 2023), although this may depend on the metaphor identification procedure used.
One difficult case in the operational definition is prepositions such as “in” or “on,” as in “throwing light on.” According to Steen et al. (2010b), prepositions allow for different interpretations concerning their metaphoricity. They can be understood as metaphors in themselves. For example, “in” and “on” can be counted as metaphors from the domain of space. Prepositions can, however, also be part of a phrasal verb (as in “blend in”), a prepositional verb (as in “believe in”), or a polyword (as in “in-house”). In these cases, it is clear that they are metaphorical, but debatable whether each of these expressions should be viewed as one or two metaphors. Steen et al. do not count prepositions in phrasal verbs and polywords as single metaphors, but they do count prepositions in prepositional words.
To round off and illustrate our examination of the operational definition, here is an example from the material we study (see below) on the topic of Black Holes. It consists of three conversational turns, a question by the addressee, a child, and an answer by an expert, interrupted by a back-channeling of the child. Metaphorical expressions are underlined:
Child: So, it goes so fast that there's it's all dark?—Expert: So, it goes so fast that it goes completely dark. Any light that veers too close will fall in, will not be able to make it back out again. If a light is shining from the sun near a black hole, …—Child: Mhm.—Expert: The black hole's not touching it. Why does the light get pulled in? Why does that happen?
The underlined metaphors explain what happens to light near a black hole. Most of them are verb and preposition metaphors (“going,” “veering,” “falling in”—a phrasal verb –, “touching,” “pulling in”—another phrasal verb –), though one (“making it back out”) is a multi-word complex construction. The metaphors draw meaning from bodily actions related to movement in space. Note that the passive construction in “get pulled in” is important for the metaphorical meaning (Karsten et al., 2022). You may also have noticed that one instance of “go” is not marked as a metaphor. We will explain that exception below in the Methods section.
1.4 Metaphors in explanations
Evidence of studies in educational (e.g., Wan and Low, 2015) and scientific contexts (e.g., Bailer-Jones and Bailer-Jones, 2002; Hofstadter, 2001; Leary, 1990) points toward the relevance of metaphors in explanations. One common research approach is to demonstrate potential limits or advantages in understanding when certain metaphors are used. For instance, Niebert and Gropengiesser (2013) showed that students' and experts' metaphorical understandings of global warming differ and that students confuse different processes and phenomena in their conceptualization. The authors also made suggestions for teaching environments that allow for these metaphorical understandings to be disentangled and clarified. Other studies have shown that many metaphors for global warming have problematic entailments (e.g., Chen, 2012; Flusberg et al., 2017 see also Ergazaki and Ampatzidis, 2012). Recently, Flusberg and Thibodeau (2023) critically evaluated different metaphors, that are used in educational or political discourse on global warming, regarding relevant dimensions (conventionality, systemicness, emotionality, and aptness). Based on these dimensions, they suggested metaphors that may help people better understand global warming and draw correct conclusions for how to counteract it and may motivate citizens to act accordingly.
Another common approach to study the influence of metaphors on understanding is called metaphor framing. Here, study participants receive descriptions of a topic with different metaphorical frames and are later asked for a decision on a specific issue within the topic. Their responses are evaluated in terms of consistency with the metaphorical frame (for a few examples, see Elmore and Luna-Lucero, 2017; Flusberg et al., 2017; Hauser and Schwarz, 2015; Scherer et al., 2015; Thibodeau et al., 2017; for summaries, see Thibodeau et al., 2019). In research on global warming, for instance, Flusberg et al. (2017) reported that the “war” metaphor for dealing with climate change led participants to perceive action as more urgent and increased their willingness to participate, compared to the “race” metaphor.
While the conceptualizing function of metaphors has been studied quite comprehensively, research on actual usage is much scarcer. When and with whom do people use what metaphors while they are explaining an abstract topic such as global warming? Does metaphor usage vary in terms of features of the context or situation, and if so, which features are these?
In the present study, we use a corpus of explanations of scientific topics addressed to persons of varying expertise to gain first insights into the context-dependent use of metaphors. Based on the aforementioned assumption of conceptual and other metaphor theories that metaphors are necessary for explaining abstract issues, it stands to reason that the more abstract the topic is for a person (e.g., due to their education), the more necessary metaphors are—or, more cautiously, that they become unnecessary when people are familiar with a topic. This may be reflected in the frequency by which metaphors are used in an explanation as the addressee's expertise increases.
1.5 Hypotheses
One very general thesis underlying CMT and much applied metaphor research is that metaphors are necessary to explain abstract concepts. If dialogue partners lack an understanding of an abstract concept, people will refer to more concrete, experience-related concepts, as has been initially argued by Lakoff and Johnson (1980; see also Gibbs, 1994; Kövecses, 1986; Lakoff and K?vecses, 1987; Lakoff, 1993). From this general understanding, we derive the hypothesis that metaphors are more relevant in dialogues in which the two partners have unequal expertise (e.g., a dialogue between an expert and a child or teen) than in those with equal expertise (a dialogue between an expert and another expert) and will thus be the more frequent, the larger the expertise difference.
We test this hypothesis on a set of videos in which an expert explains an abstract concept, mostly from the sciences, to addressees of varying expertise. We have chosen this material because it represents a comparatively standard situation of explaining. The dialogues were certainly conceived in advance and were at least partially edited. But they are spoken freely, and the unpredictable responses of the addressees make them more natural. In addition, we do not assume that metaphors were explicitly used by the participants, i.e., the metaphors should correspond to what happens in a spontaneous explanatory conversation.
We expect metaphor frequency in the dialogue parts of the experts to decrease with increased expertise of the addressee. For the addressee, we have no such hypothesis. CMT is not specific enough for conclusions about the addressee. In a good explanatory dialogue, explainers and addressees will, at least sometimes, pick up each other's metaphors. We therefore hypothesize that there will be some quantitative correlation.
2 Methods
2.1 Material
As a corpus, we use the transcripts of 24 explanation dialogue videos from the American online magazine Wired,1 an extended version of an already published dataset (Wachsmuth and Alshomary, 2022). In these videos, an expert explains the same abstract scientific issue, for instance nanotechnology or deep learning, to persons of five levels of expertise. Concerning the sample size, we used all videos that were available when we started this project (three more videos were added after that). With 98.006 words in total, the size of the corpus was just about manageable in a study with manual coding.
The 24 dialogues each have a different topic and different participants, but all include five levels (child, teen, undergraduate student, graduate, and expert), thus varying in complexity in a similar way. Wired explains the five levels as follows: “In five Levels, an expert scientist explains a high-level subject in five different layers of complexity— first to a child, then a teenager, then an undergrad majoring in the same subject, a grad student and, finally, a colleague” (Wired, 2024). According to this definition, the levels refer to a mix of age, education and closeness to the topic. Wired also speaks of levels of complexity of the explanation and underlines that the explainers should use terms that their addressee can understand. The explainers derive their expectations from this information.
The videos are comparable in that all experts had the same task of explaining an abstract concept in their field of expertise in 5 levels of complexity defined by the respective addressee. Apart from this basic similarity, the experts were free to design their explanations as they judged best. Also, the responses of the addressees were not foreseeable. The videos differ, however, in overall length and in share of words uttered by each participant. We compensated for variability in length by using the proportion of metaphors, not the absolute number, for our analyses. We regard other differences between the videos as a reasonable trade-off—reducing comparability for the sake of analyzing speech that is more natural.
Wired identifies all explainers as experts. More precisely, 17 of the experts self-identified or could be identified as university professors and three further persons as (senior or experienced) researchers. Four had other expertise as artists or entrepreneurs. Basic information on the videos is given in Table 1.
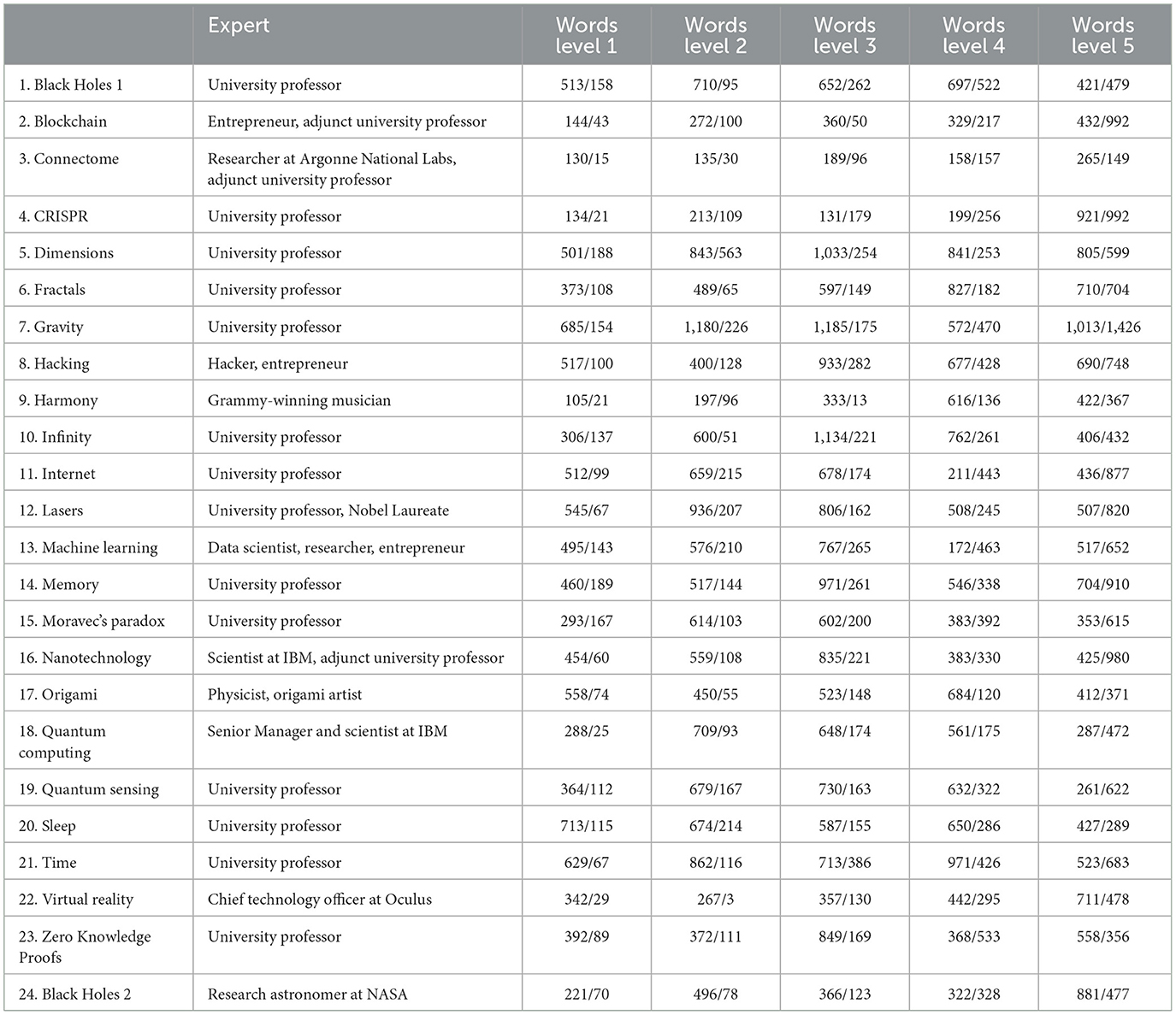
Table 1. Information on the sample: explainer expertise and word counts (explainer/addressee) for each of the videos and each of the levels.
2.2 Data coding
The transcripts were coded by two trained student coders who went through the transcripts word by word and marked metaphorical expressions. For identifying metaphors in the transcripts, we used a slightly simplified version of the metaphor identification procedure developed by Steen et al. (2010b) on the basis of the Pragglejaz Group (2007) method. The key steps identified by Pragglejaz are as follows:
1. Reading the text to establish a general understanding of its meaning,
2. Determining lexical units (words or phrases),
3. Establishing the meaning of each lexical unit in the specific context of the text,
4. Determining if the lexical unit has a more basic, concrete, or physical meaning in other contexts.
5. If there is a more basic meaning in the sense of step 4 and this is not the meaning with which the lexical unit is used in the text, it is marked as a metaphor.
Steen et al. (2010b) refine and adjust this procedure by several means. They include and differentiate direct metaphor, implicit metaphor, metaphor signals or flags, and personification metaphors. They also include more borderline cases, use word history only in rare cases to identify a word's basic meaning, and do not allow the metaphorical meaning of a word to be imported from another word type. To our knowledge, this procedure is the most clearly operationalized procedure to date for adult speech. Gaskins et al. (2023) have argued that the procedure might not be appropriate to identify metaphors in young children's speech as they might not be aware of more than one meaning or have not encountered the basic meaning identified in the procedure. This is an interesting argument and an important concern. However, the children in the Wired dialogues (for all the imprecision of the group description) are older than the children referred to by Gaskins et al. Besides, for our study designs we need a comparable procedure for all five levels.
As explained above, metaphor is characterized by a transfer of meaning from a source domain onto a target concept and thus by the non-literal usage of a word in a projected text world. In the instruction, the student coders were told to mentally identify the literal usage of a word and identify potential transfer. They then indicated the words or expressions that referenced a concept that did not represent the literal situation, projected text world or situation, for both direct and indirect metaphors alike. Metaphoric expressions were nouns, verbs, adjectives, adverbs, prepositions, and idioms.
The coders were made aware of frequent types of metaphors and were given the following additional guidelines:
• One and the same expression can be metaphorical or not metaphorical depending on the context (e.g., Steen, 2023), so context is vital. Do the actual words reference an image that is not literally applicable to the situation described? When in doubt, always refer back to this question and try to identify plausible source and target domains.
• Some metaphors (substance metaphors) describe abstract concepts as if they were a tangible object with object properties. For example, “giving information” or “a sorrow shared is a sorrow halved” are expressions that treat a more abstract process as the physical handling of objects and a “hard question” is not literally hard, but difficult to answer.
• Some metaphors (personification metaphors) imply the agency or personhood of an abstract concept or an inanimate object. For example, “the test allows an early diagnosis” or “the current chooses the path of least resistance” or “the gas wants to rise up.” Here, processes are conceptualized as behavior.
• Metaphorical spatial relations, directions, and prepositions (orientational metaphors) are easy to miss, because many of them are conventional or part of compound verbs. A closer look tells us that the manager is only figuratively “above” the intern and that an affair is not located “on the side” of a marriage. Sometimes, the speakers' gestures can confirm that they imagine a preposition as a spatial concept. Here, it can be helpful to examine the original videos in addition to the transcript.
• Some metaphors are conventional and pale. It is difficult to judge if a metaphor is so conventional that it can be disregarded, and the former target concept is commonly understood to be the original meaning. As long as there is an identifiable transfer of meaning, we assume that it is a metaphor, that is, we usually opt for coding a metaphor instead of not coding one.
From the three types of metaphors identified by Steen et al. (2010b), we used direct and indirect metaphors. Implicit metaphors (i.e., ellipsis and substitution referring to a metaphorically used word) were not included because this type of use does not seem to be related to the explanatory function of metaphors. Most metaphors in the corpus were indirect, that is, used without the speaker indicating their metaphoricity. Sometimes, the speaker indicated that they were about to use a metaphor by using expressions to signal that they mean something in a non-literal way. They said, for instance, “this project is like my baby,” “I had to, in a way, sacrifice precision,” “Here is the catch, so to speak” (metaphor flags underlined). Also, the word “literally” was used to signify that something is meant figuratively, and speakers sometimes used virtual quotation mark gestures with the fingers to indicate metaphors. The student coders were advised to, when in doubt, examine the gestures in the videos.
Polywords or compounds (including idioms) were treated as defined by Steen et al. (2010b, p. 26ff.). We also followed Steen et al. in counting phrasal verbs as one metaphor because of only one identifiable transfer of meaning. Unlike Steen et al., we usually also counted prepositional verbs as one metaphor. We decided for this option because, firstly, the difference between phrasal and prepositional verbs is not entirely clear (the same verb may be counted both as phrasal and prepositional in different sources). Secondly, the choice does not make a relevant difference. The main aim of our study is the comparison across expertise levels, not the exact quantification of metaphor frequencies. We simplified step 2 of this procedure by instructing the coders to mark a verb-preposition conjunction as a metaphor if the combination had a clearly different meaning than both words individually. These cases were rare, and as we used the same rule across all dialogues. In the few cases in which verb and preposition transferred clearly differentiable meanings we still coded two metaphors. An example is “collapse under.”
When the same metaphor was used multiple times per turn, it was counted only once. We decided on this because our goal was to quantify how metaphorical one person's speech was. A person using one metaphor multiple times in the same way and a person using multiple metaphors should not be scored the same when the metaphor is continued throughout a conversational turn. If a person used the same metaphors during different turns in the conversation, however, there is new (local or emerging) context wherein that metaphor is chosen anew, and that usage is counted again. Minor changes of the same word, such as using the plural, or a different tense did not constitute the use of a new metaphor. For comparison, we also report the results including repeated usage within a turn in the Appendix.
As an exception, metaphorical expressions for the titular target concept and conventional scientific terms to it, such as Black Holes, blockchain, hacking, harmony, or machine learning, were not counted as metaphors. According to Steen et al.'s (2010b, p. 34) procedure, in cases of specialist terminology, there is often not enough contextual information for the outsider to decide about metaphoricity, but they still count these expressions as metaphors because they are metaphors for the general language user. In the context of the Wired explanations, there is sometimes no choice for the speakers: they must use these metaphorical expressions in the context of their topic. For each text and in step 1, we identified metaphors that were terminology and thus very difficult to avoid. The list of terminological metaphors is provided in Table 2.
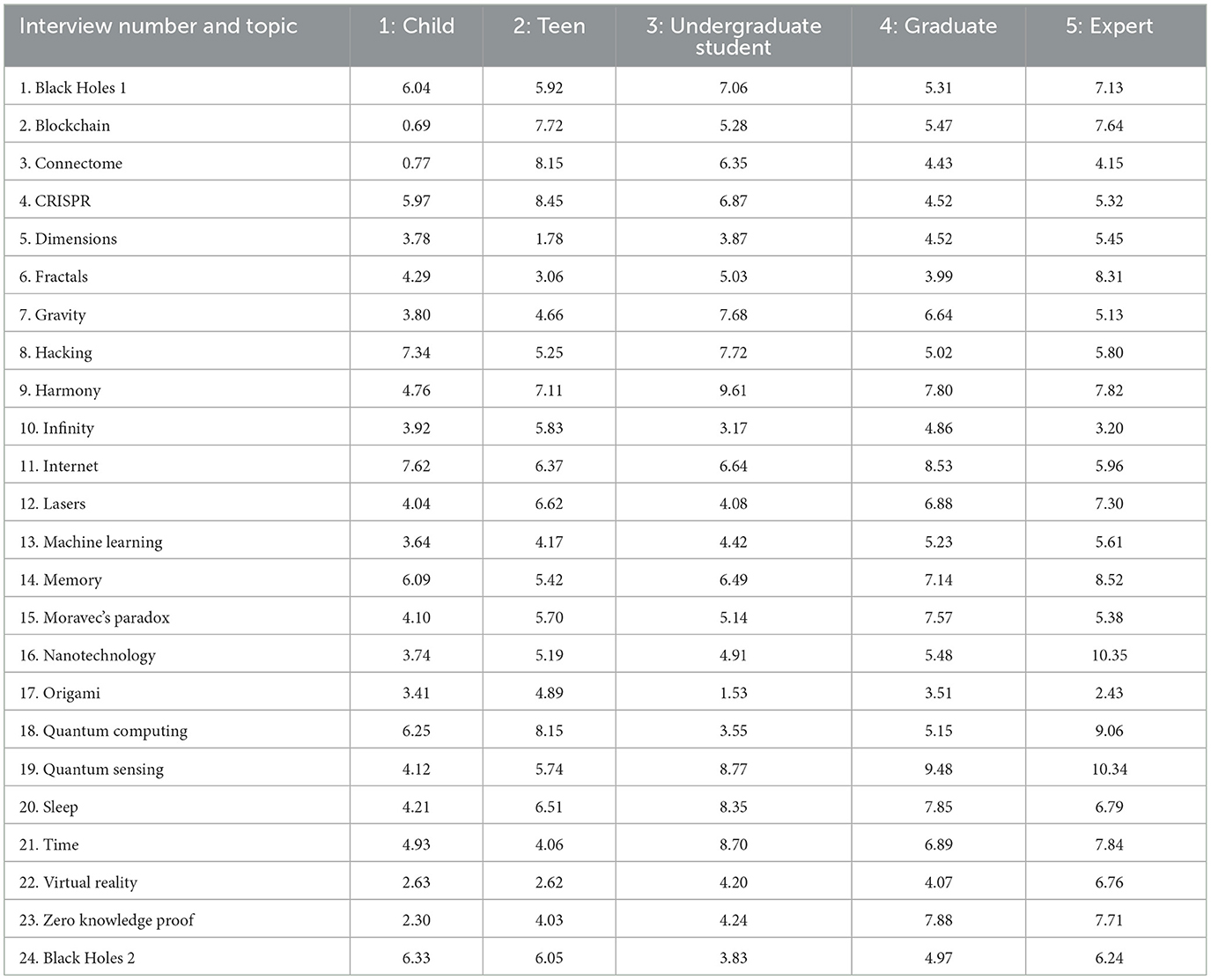
Table 2. Metaphor frequency (proportion of metaphorically used words among words overall, excluding repetitions within a turn) of the explainers in the 24 videos and across the five levels of expertise of their addressee.
Allegories posed additional difficulties. When speakers told a whole story (as Hilbert's Hotel in the video on infinity) to illustrate multiple points, it was difficult to identify which aspects were supposed to be mapped onto the target concept and should therefore considered to be metaphors. Coders were made aware that dead metaphors might be an issue as well as metaphors where it is ambiguous what is to be regarded as the “original” meaning.
3 Data
We calculated the proportion of metaphors for the explainer and the addressee, respectively. While we coded three different types of metaphors—noun, verb, and adverb/preposition metaphors as explained above—we had no specific hypotheses about them and lumped them together for statistical analysis.
3.1 Statistical procedures
We used Bayesian methods for the statistics (Kruschke, 2011). The predictor or independent variable (i.e., the expertise of the addressee) is an ordinal variable with five levels. The larger the number, the larger the expertise. The criterion is the proportion of metaphorical expressions that the explainer or the addressee uses, a value between 0 and 1. We use the proportion because the dialogues vary in length so that the same absolute number of metaphorical expressions can make up a small or large proportion of the words spoken and thereby deflate or inflate the estimated relation.
Based on these definitions, we can formulate a statistical hypothesis. There should be a decrease of the proportion of metaphors with expertise. We derive the bounds for the reasonable region of practical equivalence (ROPE, Kruschke, 2011) from Steen et al. (2010b) quantitative analysis of metaphors. They report a proportion of 6.8 % for conversation (excluding cases of doubt; p. 781). Subtracting 0.1% implicit metaphors (p. 784), which we do not count in our analysis, we reach 6.7% for conversation. We take one tenth of this general reference estimate as ROPE. It is possibly slightly too large, because Steen et al. count repeated metaphors multiple times, which we do not. But we do not know in advance how large the proportion of repeated metaphors is. Basically, if the 95% HDI of the decrease lies entirely outside the ROPE, the null model (or more precisely, the value of 0) can be rejected. We will accept the null model if the 95% HDI falls entirely within the ROPE. However, we have to be aware that, when comparing the slope estimate with the ROPE, we answer the question whether the difference between two consecutive levels is different from zero or, more precisely, how probable that is given the data. This is a narrow operationalization of our original question about a decrease in metaphoricity with expertise. In line with Bayesian thinking, we report for all four differences (one level, two levels, three levels, and four levels) whether they fall within, lie outside, or overlap with the ROPE.
We will also report the full 95% HDI, that is, the most credible parameter values. These estimates may be used in further studies.
The priors for the Bayesian linear regression were set as follows: slope ~Uniform(-2, +2). Considering the frequencies estimated by Steen et al. (2010a), we assumed that slopes per level cannot exceed ±2% and chose the uniform prior because we because we wanted to avoid setting the highest probability to zero with a normally distributed (or comparable) prior. Intercept (arbitrarily set at level 5) ~Uniform(0, 18). Again, considering the Steen et al. estimates, frequency will not exceed the 17.5% they reported for scientific texts and cannot be lower than 0%. However, we have no prior assumption about the most likely value which makes a uniform prior, despite its disadvantages, such as lack of true non-informativeness, the least problematic choice. σ (standard deviation of the data from the regression line) = HalfStudentT(sd = 2, degrees of freedom = 2). We have no quantitative expectations as to the size of the correlation between explainers' and addressees' uses of metaphors, and it is not directly related to our question. We will therefore report it with its mode and 95% HDI. The priors for the Bayesian correlation analysis were: μ (Metaphor proportion) ~ Uniform(0, 18) (see above) and cov/corr ~ LKJCholesky(η = 1). The LKJCholesky prior is uniform and restricts the values to a valid correlation and covariance matrix.
4 Results
4.1 Coding difficulties
There were some notable dilemmas in coding. These cases could be argued to either be pale metaphors or no metaphors. Such was the case for “thing,” “go,” “way,” and “have.”
Speakers used “thing” to organize their sentences which would mean that it is not meant metaphorically even though one could argue that its basic meaning is a tangible object. The Macmillan English dictionary for advanced learners (Rundell, 2007) that Steen et al. (2010b) recommend to use when trying to identify the basic meaning of a word does not give a concrete basic meaning for “thing.” The most experience-related ones are “an object or an item” and, for the plural, “the objects that belong to a particular person or that are used for a particular purpose” (Rundell, 2007, p. 1,554). “Have” and “go” have so many uses in the English language that they, too, often serve a grammatical purpose rather than conferring meaning. This is especially the case for “have.” For “go,” the Macmillan lists “to move or travel to a place that is away from where you are now” as an experience-related meaning besides more than a dozen much less experience-related meanings, such as “to change to another condition” (Rundell, 2007, p. 641). Finally, “way” is a homonym that can mean at least manner, aspect path, means and condition (Rundell, 2007, p. 1,685). Whether it is a metaphor depends on what the coder assumes the speaker to have meant. This would be true for all homonyms, but proves especially difficult for “way,” because path metaphors are often pale and thus difficult to distinguish from literal use of the word.
For all these cases, coders could unanimously identify some cases that were metaphors and some that were not, so there was no simple solution for coding the words. The coders had to carefully consider the context for each case and accept that there could not be perfect agreement here.
Dead metaphors and metaphors where the basic meaning is ambiguous were another issue. For example, the word “cool” is in oral language most often used to mean “awesome” (the Macmillan gives “impressive because of being fashionable or attractive;” Rundell, 2007, p. 326), but its earlier meaning that is also still in use is “cold.” So, if “awesome” is the more usual meaning, it can be argued that there is no metaphor in the sentence “Lasers are really cool” while a person who insists that earlier meaning is original meaning will disagree and code “cool” as a metaphor.
4.2 Intercoder agreement and reliability
In order to prepare a proper measure of intercoder reliability, we deleted all words for which it was impossible that the coders would disagree. Including these words in the calculation would inflate the agreement by trivialities. The words we deleted were pronouns, deictics (this, that, these, those; remember that pronouns and deictics may be implicit metaphors, as defined by Steen et al. (2010b) which we, however, did not take into account in our analysis), articles, conjunctions (and, or, but, if, because), the verb be in all its forms, and some other frequent words (yes, yeah, no, would, and wouldn't).
We hereby reduced the word count from 97,527 to 69,083. In 4,539 cases, the coders did not agree on whether the word was a metaphor or not. Intercoder agreement thus is 93% and Cohen's κ can be estimated at 0.8686, a high value (Greve and Wentura, 1997, p. 11; Landis and Koch, 1977).
For the final analysis, one of the coders resolved all cases of disagreement.
4.3 Metaphor frequencies
Tables 2, 3 give the proportion of metaphorically used words per 100 words (metaphor frequency) the coders identified in the material, separately for the videos and the five levels each. Table 2 contains data for the explainer, Table 3 data for the addressee.
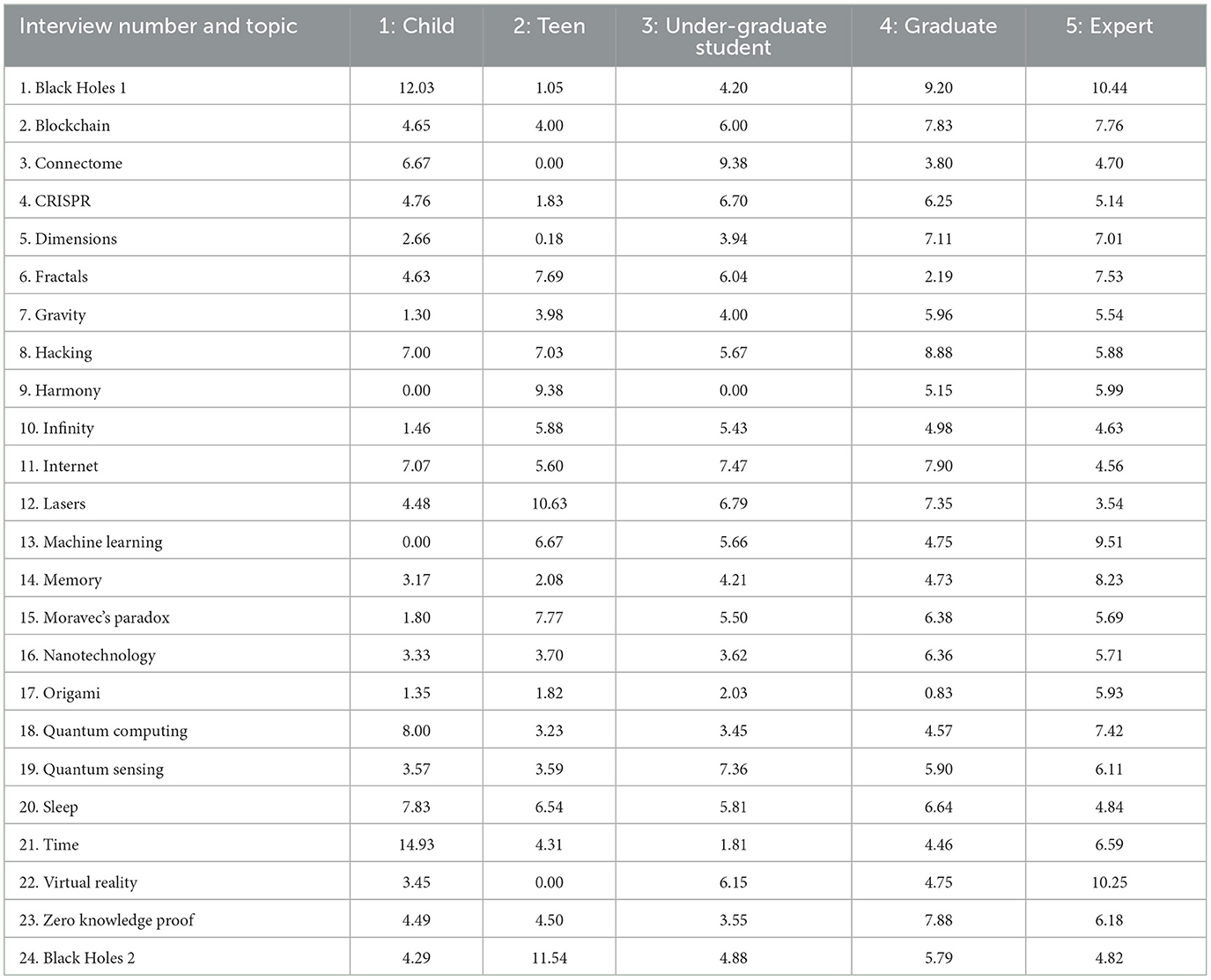
Table 3. Metaphor frequency (proportion of metaphorically used words among words overall, excluding repetitions within a turn) of the addressees in the 24 videos and across the five levels of expertise of their addressee.
The frequency varies between 0.69 and 10.35% for the explainers and between 0 and 14.93% for the addressees. The mean is 5.66% for the explainers and 5.21% for the addressees. The larger range for the addressee is mostly due to some very short videos in which the addressee spoke only a few words (see below). Explainers still used more metaphors overall than their addressees, which is apparent in Figure 1 (left compared to right).
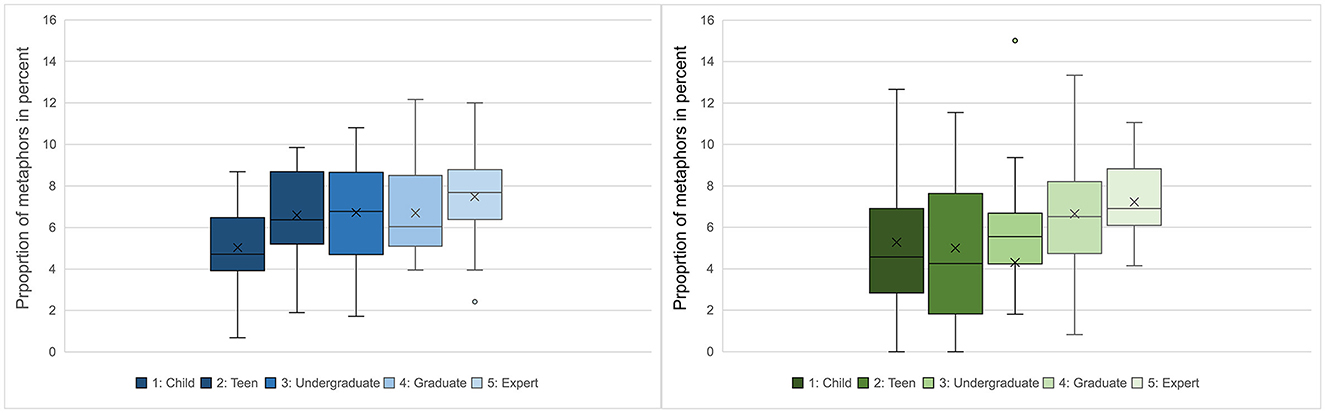
Figure 1. Proportion of metaphors (excluding repetitions within a turn) for the explainers [(left), blue] and the addressees [(right), green] across the five levels. The box contains 50% of the data points, the x indicates the mean, and the horizontal bar is the median. The whiskers are minimum and maximum, and the points indicate outliers.
Note that while the mean frequency seems to be similar to Steen et al. (2010a) estimate of 6.8% for metaphors in conversation, this has to be qualified: We counted prepositional verbs as one metaphor, whereas Steen et al. count them as two (unknown proportion), and our main estimate neither includes metaphors repeated within a turn nor what we term unavoidable terminology. Including these metaphors changed the counts by a small amount: Including repeated metaphors increases frequency by 0.83% for the explainers and 0.35% for the addressees, resulting in overall estimates of 6.48 and 5.59%. Including terminology metaphors increases frequency by 0.84% for the explainers and 0.74% for the addressees, resulting in overall estimates of 7.33 and 6.42%.
Many of the more extreme proportions for the addressee stem from dialogues in which they talked little: 0% metaphors were counted in the dialogues on harmony (level 1: 21 words of the addressee overall; level 3: 13 words), machine learning (level 1: 143 words), connectome (level 2: 16 words), and virtual reality (level 2: 3 words). The largest proportion stems from an interview on time (level 1: 67 words). For the explainer, the smallest proportion was in a level-1 dialogue on blockchain (144 words), and the two largest proportions stem from the level-5 dialogues on nanotechnology (425 words) and quantum sensing (261). None of these three cases is a particularly long or short example
Figure 1 (left) depicts the proportion of metaphors for the experts across the five levels. Figure 1 (right) does the same for the proportion of metaphors that the addressee used. Even without a statistical test, it is clear that our hypothesis is false. Numerically, metaphor frequency increases between level 1 and level 5 for the experts. Between levels 2 and 4, there are only small increases in the mean frequency. For the addresses, there seems to be an increase starting at level 3 without much difference between level 1 and 2. Variability is large.
4.4 Statistical analysis
Figure 2 depicts the results of the Bayesian regression; Table 4 gives its parameters. The estimate for the change is +0.504% metaphors per level for the explainers. With a ROPE of ± 0.67 around 0 and the lower limit of the HDI at 0.268, this value is neither clearly inside, nor clearly outside the ROPE for adjoining levels, although 91.6% of the posterior lie within the ROPE (see Figure 3 upper left).
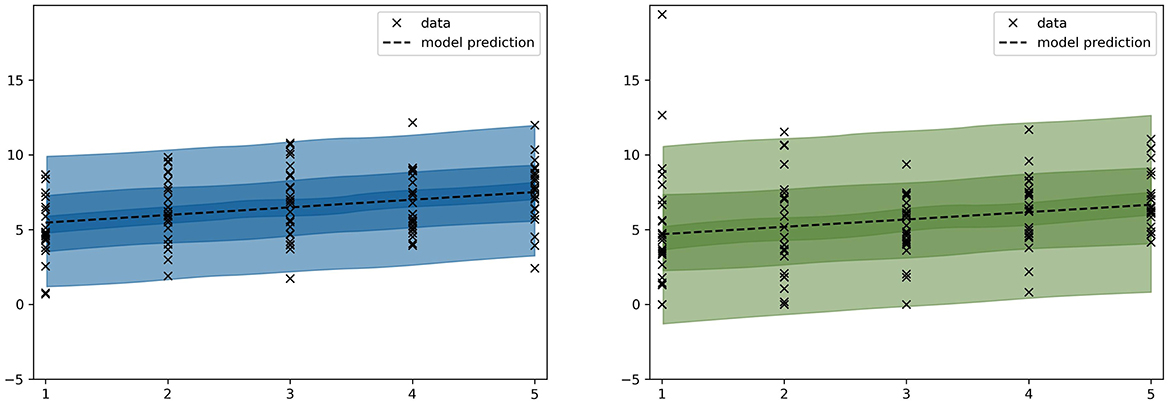
Figure 2. Results of the Bayesian regression analysis. X-axis: levels of expertise of the addressee. Y-axis: metaphor frequency in percent. The predicted regression is shown as a dashed line, the darker and lighter colored areas are the 20, 60, and 95% HDIs. [(Left); blue]: results for the explainer. [(Right); green]: results for the addressee.
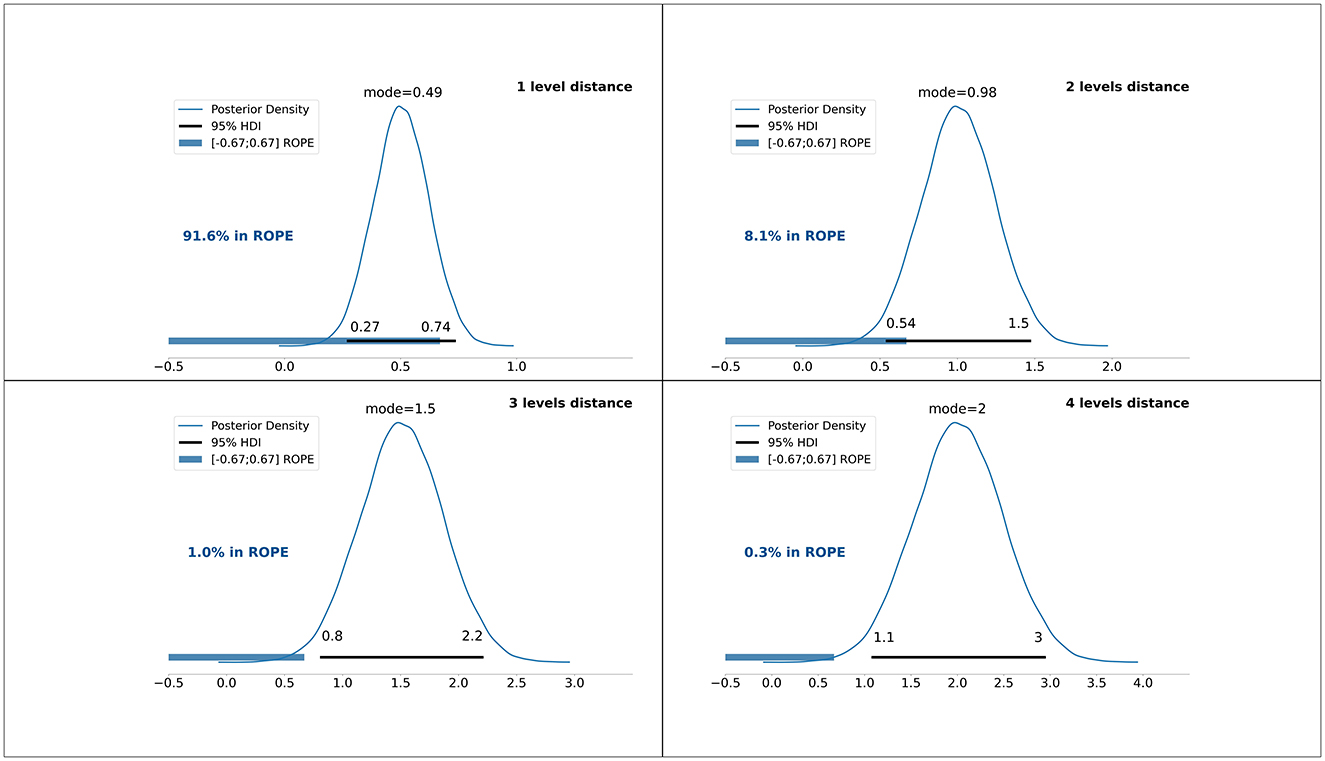
Figure 3. Posterior estimates, HDIs, and ROPES for the slopes on different level distances. X-axis: slope; Y-axis: posterior density. Data for the explainer.
The numerical increase can, however, be statistically substantiated for a distance of three levels (see Figure 3 lower left). Here, ROPE and HDI do not overlap; only 1% of the posterior estimate lie within the ROPE. For the larger difference, the overlap is even smaller (Figure 3 lower right). For the two levels distance, ROPE and HDI overlap, though with only 8.1%.
The increase per level for the addressee is estimated at 0.453%. For descriptive purposes—we did not have a hypothesis on the addressee data—, we performed the same tests as for the explainer data. Again, the slope estimate value is neither clearly inside, nor clearly outside the ROPE when comparing two adjacent levels. Slope posterior and ROPE overlap with 90.1%. The HDI is quite large indicating some power issue (compare the shaded areas in Figure 2, right part, to those of Figure 2, left part).
In the case of the addressee data, ROPE and posterior overlap for all level differences, though very little for the difference of 4 levels. This is illustrated by the posterior estimates in Figure 4.
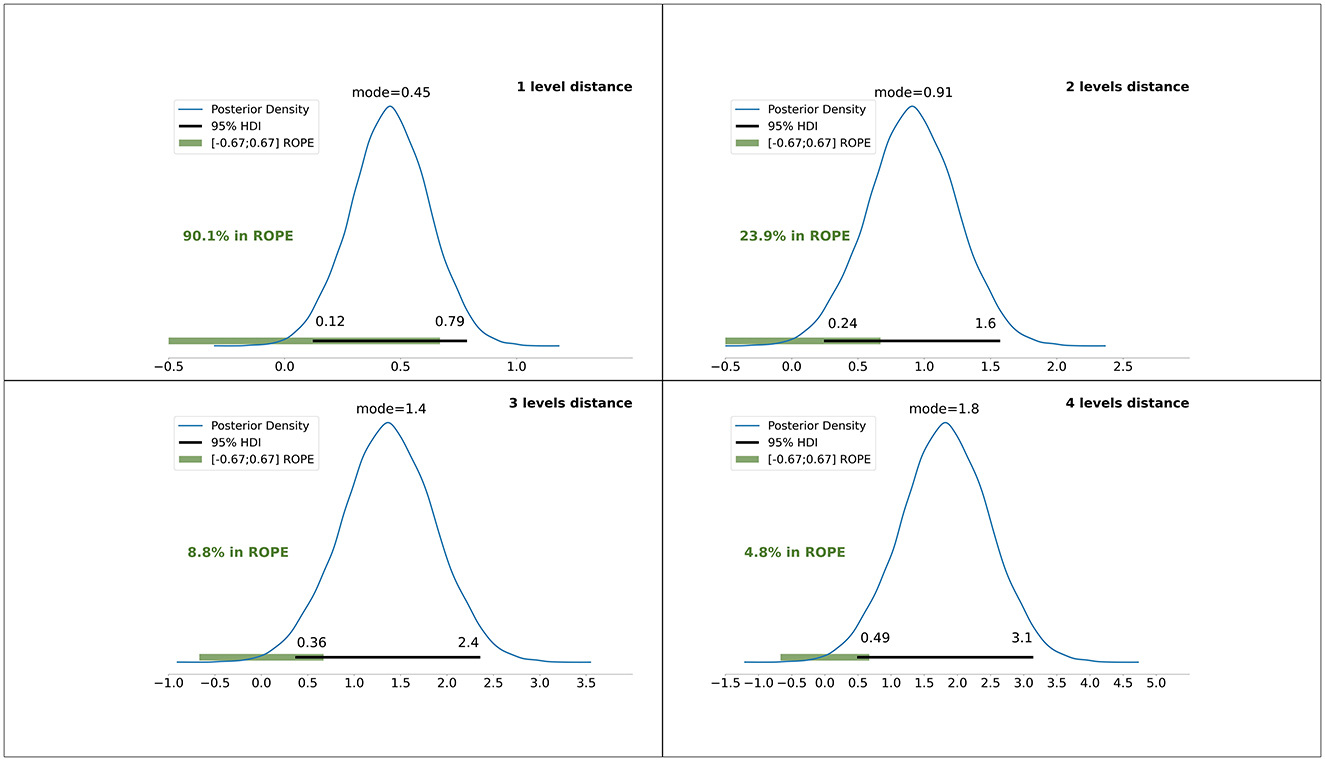
Figure 4. Posterior estimates, HDIs, and ROPES for the slopes on different level distances. X-axis: slope; Y-axis: posterior density. Data for the addressee.
Figure 5 depicts the posterior estimate for the correlation between metaphor frequency for explainers and addresses together with its HDI. The mode of the posterior is 0.23 and the HDI excludes a value of 0. These values correspond to a medium effect size.
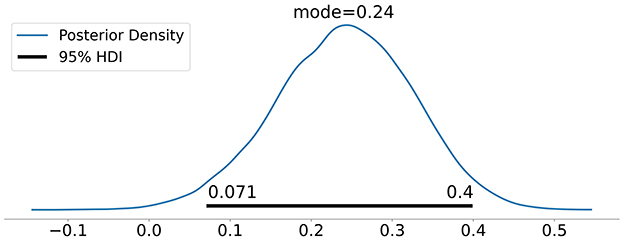
Figure 5. Posterior distribution for the correlation between metaphor frequency for explainers and addresses together with its 95% highest density interval (HDI) and mode. X-axis: correlation; Y-axis: probability density.
It is possible that our criterion to count a metaphor only once per turn—which we set because repetition of a metaphor within a turn in an explanation should not be conflated with using another, new metaphor—was not well chosen. Appendix B therefore contains an additional evaluation including repeated metaphors. The results do not differ from our present analysis.
As additional information, the proportion of words in a dialogue that were spoken by the addressee increased across levels from 19% (levels 1 and 2) over 22% (level 3) and 38% (level 4) to 54% (level 5). In 17 of the level-5 explanations, the addressee uttered at least 50% of the words. Among the metaphors the explainers used, nouns and verbs were predominant (32 and 48% excluding repetitions; 50 and 21% including repetitions). The same was true for the addressees (36 and 43% excluding repetitions; 34 and 44% including repetitions).
4.5 Types of metaphors
In the following, we give some examples of metaphor use on the five levels that indicate that metaphor use may differ in a qualitative manner between the levels. While this is not the result of a systematic study, it may suffice to motivate further analysis of the openly available dataset. It seems that there is a systematic change from using mainly conventional metaphors on the first level to increasingly matter-of-course use of terminology. On level 4, usage of vivid, unexplained metaphors that are not terminology, begins and then is very apparent in the dialogue between experts (when reading the examples, remember that terminology metaphors are in italics, other metaphors underlined).
Level 1: The two metaphors in the dialogue excerpts are conventional, the examples used are literal and no metaphorical terminology is used.
Example 1: “And that's kind of what we're gonna try out today. In fact, we're gonna have you build a fractal. A tree is a really good example of a fractal, because if you look at the whole tree and then you break off a big branch of the tree” (video on fractals).
Example 2: “Yeah, it's crazy. They're really a lot of bad or malicious hackers out there who are doing just that. They're going into people's accounts and they're stealing money, but there's also another side of computer hacking where there are people who are trying to learn how those bad hackers are actually breaking into the bank accounts” (video on hacking).
Level 2: On level 2, the metaphors again are conventional and pale. In the second example, one metaphor is signaled as metaphor by “it's similar to … “Furthermore, scientific terms that are basically metaphors are used. Fewer examples and visual aids are used, some conventional metaphorical terminology is present. For comparison: The laser video expert didn't use the word “wave” on level 1; the Moravec's Paradox expert didn't use the term “reinforcement learning” for level 1.
Example 1: “Now the light bulb has to be a little bit special. It has to be a type of material that can store the energy in an excited state right? It has to stay up there really energetic and sit there for a while so that when the light comes along, it takes that energy and becomes stronger light and then the mirror sends it back and it does it again and again and again and between these two mirrors, it makes the light come out in a nice beam. In a laser, it comes out as a single color. They all come out with their waves at the same time” (video on lasers).
Example 2: “So, in reinforcement learning, the robot tries the task, and then it gets some sort of reinforcement, some sort of feedback. It's similar to how you might train a dog” (video on Moravec's Paradox).
Level 3: In example 1, the explainer frequently uses metaphorical terminology, obviously expecting that it is understandable. Even when the explainer does not fully expect the guest to know metaphorical terminology, it is introduced into the conversation (example 2). This may be characteristic of level 3.
Example 1: “They wanted to build this notion of a packet-switching network. Not a circuit switch network like a phone network where you get a dedicated path and a dedicated set of bandwidth and links from source to destination” (video on the internet).
Example 2: “Certainly, we can blow atoms up with the laser hammer and when the laser light comes in and just smacks the electrons right off the atom. And so, the question really is how do you make that? Back in the 70's and into the 80's, I know that was a long time ago for you, we had big energy lasers and we had short pulse lasers, we couldn't have big energy, short pulse lasers and actually it was my supervisor and I that figured the way around that and we got something called chirped pulse amplification. Have you heard of chirped pulse amplification by any chance?” (video on lasers; note that “getting” was not coded here as a metaphor because it is difficult verb without a clear basic meaning).
Level 4: The explainers sometimes (example 1) lean into the terminology, sometimes (example 2) use many metaphors like “dominate,” “tune,” and “headache.” These are not necessarily unconventional, but also neither subtle nor explicitly explained (like those of the lower levels).
Example 1: “So, there are three well-known famous equivalent forms of the axiom of choice. The well-ordering principle is the assumption, the axiom that any set can be well-ordered, but there are lots of subsets of real numbers that do not have a minimal element. So, that ordering is not a well ordering. So, here's the key question. Do you believe the axiom of choice¿‘ (video on infinity).
Example 2: “Because the materials are so small, they're at the nanoscale, their properties are dominated by quantum mechanics, which means that even slight changes in their size, in their orientation give profound changes in their properties. And while that's very scientifically interesting, and it allows you to tune their properties by making subtle changes, from a technology point of view, it's a bit of a headache in the sense that in technology want to optimize for a property and then repeat that over and over again” (video on nanotechnology).
Level 5: What seems to be typical at this level are vivid metaphors with no explicit explanation that are quite likely to be confusing to novices but used with confidence. Examples are “silos,” “cross pollinate,” “gnarly” code, and “playing.”
Example 1 (already partly discussed in the Introduction): “One of the things I love about nanoscience is it tends to break up the silos between those traditional scientific disciplines. My training was in chemistry, but I had to very quickly merged chemistry and physics. And now I see an area where chemistry, physics, and biology are coming together to produce new materials and new technology, and to advance the field forward. And so being in this field, you kind of have to cross pollinate between these different disciplines and kind of advance the field together” (video on nano technology).
Example 2: “The accessibility of the tooling, like, we can now do in, like, five lines of code something that would have taken 500 lines of very mathematical, messy, gnarly code even, you know, 5 years ago. And that's not an exaggeration. And there are tools that mean that pretty much anyone can pick this up and start playing with it and start to build with it” (video on machine learning).
4.6 Picking up each other's metaphors
If metaphors serve an explanatory function, they should be picked up between the two partners. Across all interviews, there were 187 instances in which one person used a metaphor that had already been used by their conversation partner. Many of these seem to be coincidental or due to the conventionality of metaphors, for instance this use of “areas” (all metaphors underlined):
Addressee: If we think about quantum sensing in particular, it really involves understanding materials, solid state materials, chemicals, you know, chemistry, biology, engineering, electrical engineering, optics, photonics, I mean so many different areas. And I think that that's one of the most exciting things about that is the degree to which it's engaging a much larger cross section of scientists. They're the ones that I think are gonna come up with the breakthroughs of saying, oh wait, I could design this molecule to do this thing.
Explainer: Yeah.
Addressee: And that I think is gonna make real breakthroughs in the next 10 years, is the fact that we're just having this much larger group of scientists.
Explainer: Right. People bring in very different perspectives into what used to be a very niche field. I remember in physics, you'd only talk to people in your subfield and now we're picking up the phone and talking to people in the different departments, completely different areas and we're forced to learn different languages (Quantum sensing, level 5)
One may note that the addressee begins their second turn with “field,” which is almost a homonym to “area,” and later uses “area.” Others are exact repetitions of the metaphoric expression. These are difficult to interpret, as can be seen in the following example with the metaphorical expression:
Explainer: We believe quantum mechanics tells us what the true laws of physics are, and so, a quantum sensor, in that sense, would reach the limits of what's attainable.
Addressee: It would be the top tier.
Explainer: It would be the top tier.
Addressee: What are you doing? Like, what are you studying? (Quantum sensing, level 3)
In both cases—conventional or pale metaphors and direct repetition—taking up the metaphor seems to have little function for the explanation: Conventional metaphors are in many cases presumably understood directly in a lexical manner (Bowdle and Gentner, 2005; Holyoak and Stamenković, 2018), and literal repetition can be interpreted as backchanneling. We identified only six instances in which a metaphor was seemingly consciously picked up by the dialogue partner and discussed or modified. An example is the following in which “big” or “giant” is picked up by the addressee using “hugely:”
Addressee: Yeah, I think that they're scary and cool. ‘Cause you can get sucked in and get lost forever and get plopped out in a random place. It's like a big, giant, black thingy.
Explainer: So, black holes, you describe them as huge. The interesting thing about black holes is they're hugely heavy, but they're actually physically really small. What really matters is the density. Do you know what density is? (Black holes, level 1)
Here, the explainer has observed a metaphor used by their addressee, takes it up and uses it in a differentiating description of the characteristics of the target concept, which supports a more complex understanding. This behavior is an example of monitoring and scaffolding or co-construction in explaining (Rohlfing et al., 2020). In two of the six instances, the explainer picked up a metaphor used by their guest, in the other four it was the other way round. Six instances in 24 dialogues with multiple turns each is very few.
5 Discussion
In the present paper, we set out to test a hypothesis that we derived from conceptual metaphor theory (CMT): The less experienced the addressee of an explanation of an abstract topic, the more frequent metaphors there should be in this explanation. We assumed this because of the very general notion in CMT that metaphors serve to account for abstract issues by a cross-domain mapping to a more concrete source.
In an analysis of 24 published video explanations, we could not support this hypothesis. If anything, metaphor frequency of the explainer increased with expertise—operationalized by five education levels: child, teen, undergraduate student, graduate, and expert. This numerical increase could be statistically supported for level differences larger than 2. The weak pattern was independent of whether metaphors repeated within a conversational turn were included in the analysis or not. We furthermore did not establish an increase for the addressee. We had no hypotheses about this part of the data. The increase is numerically present, although weaker, but it falls into the pre-defined region of practical equivalence. Finally, metaphor frequency correlated slightly between experts and addressees, indicating that the partners adapted to the other's use of metaphors, but not strongly.
The overall frequency of metaphors is plausible: Steen et al. (2010a) analyzed text samples from the British National Corpus for metaphoric density. The proportion calculated by them varied between 18.5% in academic texts and 6.8% in conversation with news and fiction in between. Of these genres, the Wired dialogues are closest to conversations, and with 7.33% for the explainers and 6.42% for the addressee (including repetitions of metaphors within a turn and terminology), the overall frequency calculated by us corresponds well to the latter value.
In the following, we will critically discuss aspects of our data and analysis that might impede the generalizability of our findings. We will also point out limitations of the study and derive questions for further research.
Although with 24 dialogues and 98,000 words overall, our dataset seemed quite large, variability between topics/explainers and between addressees was substantial. In terms of external validity, the data are certainly favorable, but even larger data sets seem necessary to provide a statistically sound statement about the influence of the addressee's expertise on metaphor frequency. Also, the dataset has specific limitations that have to be considered when interpreting the pattern that we found. Wired has a mission—to show that anything can be explained to anyone independent of their prior knowledge. There are no instances of failed and few of otherwise problematic explanations. Dialogue partners in other situations may have different expectations concerning the success of explanations which may in turn influence their metaphor use. Metaphor use may vary when explanations run less smoothly than in this specific set. The videotaped conversations for the public are a specific format and it is unknown how they overlap with more conventional explanations, e.g., at schools and universities.
Furthermore, there are at least two possible weaknesses in operationalization. Firstly, the independent variable is vague. The concept of “level” itself is metaphorical, implying categorical differences, whereas expertise is gradual. It might be more appropriate to quantify it in years of formal education, which cannot be inferred from the information Wired provides. Furthermore, the assigned levels appear plausible when considering each video by itself. But expertise levels are somewhat inconsistent across videos. If one was to compare the people who were categorized as having the same level, but were guests in different videos, they would often not be equivalent in their knowledgeability. A definition apparently open to some interpretation makes it easier to ensure that the five explanation conversations are as different from each other as possible—which is most interesting to the YouTube audience who watch all five conversations in a row. These considerations are in line with the fact that we confirmed a frequency discrepancy for larger differences in expertise; however, they also mean that we can only take the difference of three levels—which, in the present data, would be child vs. undergraduate, teen vs. graduate or undergraduate vs. expert –, from which on the difference deviates from 0, as a rough guide. Studies with a more clearly defined measure are necessary here.
Secondly, we may have chosen a non-optimal dependent measure. It seemed plausible to us to take the proportion of metaphors in spoken words as the dependent variable (excluding both repetitions within a turn and non-avoidable terminology such as “wave” or “wavelength”). Maybe metaphoricity can only be captured by a more complex (quantitative) measure. We are, as yet, not aware of any potentially more informative alternative.
In the present analysis, we treated all metaphors as being equal. This idea originates from CMT and its focus on everyday, non-deliberate, unobtrusive metaphor use. However, metaphors were qualitatively different. Indeed, many metaphors were inconspicuous and conventional. Some, however, were salient and vivid, as for instance that “stars are born together in two-star systems and […] die” (Black Holes I, level 2), or, on galaxies, “gravitationally, they're all friends” (Black Holes I, level 4) or “you kind of have to cross pollinate between these different disciplines” (Nanotechnology, level 5). In the Results section, we hypothesized a progression from conventional, non-terminological metaphors over terminological metaphors to vivid and unconventional metaphors across levels. A more qualitative measure of metaphor use might capture this feature of salience or richness and results may differ. Also, some metaphors might highlight an aspect of the target concept that others had not previously considered. Overall, the coders had the impression that the metaphors in the expert-to-expert conversations were often more unusual, vivid, and salient. At this stage of the analysis, this is an assumption, but it could be tested in a follow-up analysis. The latter point refers to an important issue that we have omitted in this study, the use of metaphors as metaphors—what Steen (2008) calls the rhetorical aspect in his three-dimensional model. CMT does not differentiate between metaphors used as metaphors and indirect metaphors for which the metaphoricity is not indicated by the speaker.
There are further limitations with respect to the material. The material has been revised (as can be seen by instances of cuts in videos) and it is quite likely that it has also been somewhat scripted. We have no information on what has been edited. As already mentioned, the videos have a message and Wired, a media company that focuses on education, has an aim. Whatever the exact editing, actual explanations in everyday situations—at school, at the doctor's or pharmacy, or in conversations between friends—could therefore have characteristics that do not appear in this material. On the other hand, it can be argued that the use of metaphors that we have examined here appears to be predominantly non-intentional, is therefore probably not in the focus of the speakers' or editors' control and might thus have escaped editing.
To sum up, it seems that metaphors are less frequently used when expert explainers address people with little expertise. This raises the question of what explanations there are for the increase of metaphor frequency with expertise. For children and teens, visual aids may contribute to the low number of metaphors. Almost all explainers exhibited a preference for visual aids when explaining to children and teens. For instance, when the expert showed them an experiment using a string (video on fractals) or a robot (video on Moravec's paradox), most utterances were literal and referencing the concrete objects and activities that were supposed to foster understanding of the abstract concept. This is a possible reason as to why there were relatively fewer metaphors in the transcripts at the lower levels. If the experts had not prepared materials that could be used to showcase the target phenomena in a very literal way, they perhaps would have been forced to use more linguistic metaphors. According to CMT, gestures, visualizations, actions, and other phenomena can be metaphorical, too (e.g., Kövecses, 2021; Lakoff and Johnson, 1980). Let us, however, note that two reasons at least speak against the possibility that including these metaphors would change the relation to a negative one. Firstly, aids were mostly used for children and teens, whereas the reduction in metaphors also holds for undergraduates and graduates. Secondly, the number of action- or visualization-related metaphors was not high enough to compensate for the difference between levels.
Secondly, the level-5 videos were somewhat different from the others. These are the only dialogues in which the addressee speaks as many words as the explainer—on average even more (56.45%). There is still explaining going on (at least that is the intention of Wired), but it is more among equals. Looking at the data, it might be that the three middle levels are not very different from each other. What is most apparent is that metaphor frequency is lower on level 1 and higher on level 5. While this may be related to the poor differentiability of expertise in the middle levels, another explanation might be that data are instead due to two genres or discourse types, one being a dialogue of experts and another one being an explanation to a clear non-expert. This possible explanation is, however, challenged by the fact that the proportion of metaphors at level 5 is far removed from the 17.5% that Steen et al. (2010a) report for scientific texts, even if we subtract implicit metaphors, which Steen et al. counted but we did not (0.2%), and repetitions (in our data set 0.8% for the explainer and 0.57% for the addressee) and unavoidable metaphors (in our data set 0.84% for the explainer and 0.74% for the addressee).
6 Further research
Where can we go on from the present stage of analysis? For CMT, with its assumed close connection between the understanding or explanation of abstract topics and the use of metaphors, it seems highly relevant to us to determine under what conditions—if any—a relationship between explaining and (quantitative) metaphor use exists. In order to clarify whether there is a relationship between the expertise of the addressee and the frequency of metaphors, it would be necessary, as already mentioned above, to operationalize the expertise more clearly. If levels of expertise are separated more clearly than in the current material with its relatively broad and less well-defined categories, a potential correlation—which is indicated by the slight increase from the first to the last level in particular—or its alternative, a grouping into two discourse types, may become clearer.
We therefore suggest replicating the study with the independent variable (expertise of the addressee) defined more precisely. This could be done with clearly operationalized groups and respective information to the explainers. Further experimental studies are needed to identify processes involved in metaphor use. An interesting experimental manipulation would be to deliberately enforce or prevent usage of metaphors. Most explainers increased their use of metaphors across levels, so they apparently can employ more or fewer metaphors. Such studies would be most convincing if either a measure of explanation quality or a measure of the addressee's understanding was employed.
Considering the quantitative data of Steen et al. (2010a,b), one possible explanation of our findings is that more expertise can make the conversation more academic, almost like shift from one text genre (pedagogical or social conversation, which is particularly pure at level 1 and becomes more mixed at levels 2 to 3 or 4) to another (profound academic discourse, which occurs mainly at level 5 and sometimes at level 4). It is also likely that more metaphors become necessary when talking amongst experts, because the specific conversation topics are more complex, sophisticated and abstract, even if the overall topic remains the same. We therefore also suggest following up on the question whether the difference in metaphor frequency might be due to the influence of two genres. This could be realized by studying partial experts (graduates) in two explicitly defined settings, an educational one (such as a seminar) and a scientific one (such as a scientific presentation) which make one or the other genre appropriate.
The correlation analysis showed that there was a slight quantitative resemblance in metaphor frequency between the two dialogue partners. However, this is at most indirect evidence for our assumption that in a good explanation, the partners would tend to pick up each other's metaphors. Again, experimental studies would help to answer this question, for instance by instructing explainers or addressees to explicitly pick up metaphors in an experimental condition compared to giving no such instruction.
For all such follow-ups, it is vital to test the possible relation in different contextual conditions, especially ones that are less edited or scripted, if such material can be made available. An interesting case would be educational dialogues with school children and university students of different grades or levels on the same topic. Another option, though only for the question about the influence of expertise, is an analysis of textbooks for the same groups. It will be important to make sure that the same topic is explained in such comparative studies. There were substantial differences in metaphor frequency between the Wired dialogues. We cannot say to what extent these are due to speaker characteristics or the topics, but it seems advisable to minimize such sources of variability.
It seems advisable to take a much closer look at potential contextual influences and variables. As Cameron (2003, p. 2) put it, “context works at many levels of detail.” She distinguishes between physical, social, interactional, linguistic, and conceptual context (p. 4). Pertaining to the physical setting of the present material, it would be interesting to study explanatory dialogues when visual aids—remember that explainers had some propensity for using visual aids on level 1—are not possible. Regarding the social context—roles, relationships, and group memberships—it is interesting how explainers of medium expertise (e.g., undergraduate students) would explain the same topic to addressees with less or more expertise. One limit of the Wired dataset is that the explainer always is a renowned expert. As an interactional context variable, one could study whether or how metaphor use changes as the dialogue progresses. An interesting aspect of the micro context would be speaker moves in explanations. For instance, Fisher et al. (2024), distinguished between moves on the content, the rhetorical, and the discourse management level. Similarly, Wachsmuth and Alshomary (2022) suggested several moves, i.e., dialogue acts specific for explanations (e.g., testing understanding or prior knowledge, providing an explanation, requesting an explanation, signaling (non-) understanding and the like. Metaphor use could be compared with regard to the different moves or following signals of understanding and non-understanding (Türk et al., 2024). Finally, on the conceptual level, strong or vivid metaphors could be compared to more conventional or pale ones. We already mentioned that it seems that unusual and vivid metaphors appear mostly in dialogues between experts, but why exactly is this the case, and what would happen if they were used in other situations or by an explainer of little expertise?
7 Conclusions and implications
Metaphor use in explanations seems to follow patterns such as some adaptation between speakers and an increase with level of expertise of the addressee. An interesting question for future research is how these patterns arise. It is specifically interesting what the reasons, considerations or practices are that drive explainers to use more metaphors when talking to addressees of high expertise. It is certainly also interesting to investigate the influence of other contextual factors on metaphor use and to compare different factors regarding their influence. A long-term goal of such studies is to support actual explanations in educational contexts and in the public sphere through the appropriate use of metaphors.
After corroborating the identified patterns, research such as the present study could result in recommendations for educational settings. To derive these, however, some important links are still missing. We found that metaphor frequency varies with the expertise of the addressee but we can only speculate on why this is the case. Potential causes are the expectations of the explainer (Wired advised them to think of the levels as increasing complexity) and mutual reinforcements between the two speakers (remember the frequency correlation between the explainer and the addressee). Another important influence that would have to be addressed are signals of understanding or non-understanding by the addressee. As yet, we cannot even be sure whether metaphor use increased understanding because Wired provides no information on the addressee's understanding. Before deriving recommendations, these gaps will have to be closed by further research.
It would be presumptuous to claim that the present study calls CMT into question—even if this were limited to the assumption (derived by us from CMT) that metaphors should be all the more necessary in verbal explanations of abstract concepts, the less expertise the addressee has. Our analysis nevertheless highlights the long way from this basic assumption to concrete linguistic explanatory processes. It thus underlines something that Steen (e.g., 2008, 2017) has been advocating for a long time: the investigation of the actual use of metaphors. He claims the need for a third dimension—communication—to be added to the linguistic and conceptual dimension of metaphor studies. In other words, we want to advance empirical research into CMT. In this we follow Steen, who recently pointed out that empirical investigation is significantly less advanced than the theoretical elaboration of CMT (2023, p. 3).
Data availability statement
Publicly available datasets were analyzed in this study. This data can be found at: the coded transcripts will upon publication be made available at the OSF (https://osf.io/94a2j/).
Ethics statement
Ethical approval was not required for the study involving humans in accordance with the local legislation and institutional requirements. Written informed consent to participate in this study was not required from the participants or the participants' legal guardians/next of kin in accordance with the national legislation and the institutional requirements.
Author contributions
IS: Conceptualization, Data curation, Funding acquisition, Investigation, Methodology, Project administration, Visualization, Writing – original draft, Writing – review & editing. MK: Conceptualization, Data curation, Investigation, Methodology, Validation, Visualization, Writing – original draft, Writing – review & editing. MS: Writing – original draft, Writing – review & editing, Conceptualization. HW: Conceptualization, Funding acquisition, Supervision, Writing – original draft, Writing – review & editing.
Funding
The author(s) declare financial support was received for the research, authorship, and/or publication of this article. This work was supported by Deutsche Forschungsgemeinschaft (DFG, German Research Foundation) under grant TRR 318/1 2021-438445824: Constructing Explainability.
Acknowledgments
We thank Dolph Hielscher and Philip Porwol for help with coding the material and Kai Biermeier for the Bayesian analysis.
Conflict of interest
The authors declare that the research was conducted in the absence of any commercial or financial relationships that could be construed as a potential conflict of interest.
Publisher's note
All claims expressed in this article are solely those of the authors and do not necessarily represent those of their affiliated organizations, or those of the publisher, the editors and the reviewers. Any product that may be evaluated in this article, or claim that may be made by its manufacturer, is not guaranteed or endorsed by the publisher.
Supplementary material
The Supplementary Material for this article can be found online at: https://www.frontiersin.org/articles/10.3389/flang.2024.1474924/full#supplementary-material
Footnotes
References
Bailer-Jones, D. M., and Bailer-Jones, C. A. (2002). “Modeling data: analogies in neural networks, simulated annealing and genetic algorithms,” in Model-Based Reasoning: Science, Technology, Values, eds. L. Magnami and N. J. Nersessian (Berlin: Springer), 147–165.
Bowdle, B. F., and Gentner, D. (2005). The career of metaphor. Psychol. Rev. 112, 193–216. doi: 10.1037/0033-295X.112.1.193
Chen, X. (2012). “The greenhouse metaphor and the greenhouse effect: a case study of a flawed analogous model,” in Philosophy and Cognitive Science, eds. L. Magnani and P. Li (Berlin: Springer), 105–114.
Elmore, K. C., and Luna-Lucero, M. (2017). Light bulbs or seeds? how metaphors for ideas influence judgments about genius. Soc. Psychol. Personal. Sci. 8, 200–208. doi: 10.1177/1948550616667611
Ergazaki, M., and Ampatzidis, G. (2012). Students' reasoning about the future of disturbed or protected ecosystems & the idea of the “balance of nature”. Res. Sci. Educ. 42, 511–530. doi: 10.1007/s11165-011-9208-7
Fauconnier, G., and Turner, M. (2002). The Way We Think: Conceptual Blending and the Mind's Hidden Complexities. New York, NY: Basic Books.
Fisher, J. B., Weise, M., Wilkens, J., Nitschke, C., and Rohlfing, K. J. (2024). ADEX Corpus A01. Open Science Foundation. doi: 10.17605/OSF.IO/K5FWY
Flusberg, S. J., Matlock, T., and Thibodeau, P. H. (2017). Metaphors for the war (or race) against climate change. Environ. Commun. 11, 769–783. doi: 10.1080/17524032.2017.1289111
Flusberg, S. J., and Thibodeau, P. H. (2023). Why is mother earth on life support? metaphors in environmental discourse. Top. Cogn. Sci. 15, 522–545. doi: 10.1111/tops.12651
Gaskins, D. K., Falcone, M., and Rundblad, G. (2023). A usage-based approach to metaphor identification and analysis in child speech. Lang. Cogn. 16, 32–56. doi: 10.1017/langcog.2023.17
Greve, W., and Wentura, D. (1997). Wissenschaftliche Beobachtung: Eine Einführung, 2nd Edn. Weinheim: Beltz/Psychologie Verlags Union.
Hauser, D. J., and Schwarz, N. (2015). The war on prevention: Bellicose cancer metaphors hurt (some) prevention intentions. Personal. Soc. Psychol. Bullet. 41, 66–77. doi: 10.1177/0146167214557006
Hofstadter, D. R. (2001). “Epilogue: analogy as the core of cognition,” in The Analogical Mind: Perspectives From Cognitive Science, eds. D. Gentner, K. J. Holyoak, and B. N. Kokinov (Cambridge, MA: MIT Press), 499–538.
Holyoak, K. J., and Stamenković, D. (2018). Metaphor comprehension: a critical review of theories and evidence. Psychol. Bullet. 144, 641–671. doi: 10.1037/bul0000145
Hussey, K. A., and Katz, A. N. (2009). Perception of the use of metaphor by an interlocutor in discourse. Metaphor Symbol 24, 203–236. doi: 10.1080/10926480903310237
Karsten, A., Schwede, J., Körber, M., and Scharlau, I. (2022). “Transitivitätscharakteristika als Werkzeug in der systematischen qualitativen Metaphernanalyse,” in Die Praxis der systematischen Metaphernanalyse, eds. R. Schmitt, J. Schröder, L. Pfaller, and A.-K. Hoklas (Baltimore, MD: Springer VS), 201–215.
Kövecses, Z. (1986). Metaphors of Anger, Pride, and Love: A Lexical Approach to the Structure of Concepts. Amsterdam: John Benjamins.
Kövecses, Z. (2015). Where Metaphors Come From: Reconsidering Context in Metaphor. Oxford: Oxford University Press.
Kövecses, Z. (2021). “Standard and extended conceptual metaphor theory,” in The Routledge Handbook of Cognitive Linguistics, eds. W. Xu and J. R. Taylor (London: Routledge), 191–203.
Kruschke, J. K. (2011). Bayesian assessment of null values via parameter estimation and model comparison. Perspect. Psychol. Sci. 6, 299–312. doi: 10.1177/1745691611406925
Lakoff, G. (1987). Women, Fire and Dangerous Things: What Categories Reveal About the Mind. Chicago, IL: University of Chicago Press.
Lakoff, G. (1993). “The contemporary theory of metaphor,” in Metaphor and Thought, ed. A. Ortony (Cambridge: Cambridge University Press), 202–251.
Lakoff, G., and Johnson, M. (1999). Philosophy in the Flesh: The Embodied Mind and Its Challenge to Western Thought. New York, NY: Basic Books.
Lakoff, G., and Johnson, M. (2003). Metaphors We Live By: With a New Afterword, 2nd Edn. Chicago, IL: University of Chicago Press.
Lakoff, G., and K?vecses, Z. (1987). “The cognitive model of anger inherent in American English,” in Cultural Models in Language and Thought, eds. D. Holland and N. Quinn (Cambridge: Cambridge University Press), 195–221.
Landis, J. R., and Koch, G. G. (1977). The measurement of observer agreement for categorical data. Biometrics 33, 159–174. doi: 10.2307/2529310
Langacker, R. W. (1987). Foundation of Cognitive Grammar: Theoretical Prerequisites, Vol. 1. Stanford, CA: Stanford University Press.
Niebert, K., and Gropengiesser, H. (2013). Understanding and communicating climate change in metaphors. Environ. Educ. Res. 19, 282–302. doi: 10.1080/13504622.2012.690855
Pragglejaz Group (2007). MIP: a method for identifying metaphorically used words in discourse. Metaphor Symbol 22, 1–39. doi: 10.1080/10926480709336752
Reddy, M. J. (1998). “The conduit metaphor: a case of frame conflict in our language about language,” in Metaphor and Thought, 2nd edn, ed. A. Ortony (Cambridge: Cambridge University Press), 164–201.
Rohlfing, K. J., Cimiano, P., Scharlau, I., Matzner, T., Buhl, H. M., Buschmeier, H., et al. (2020). Explanation as a social practice: towards a conceptual framework to foster social design of AI systems. IEEE Trans. Cogn. Dev. Syst. 13, 717–728. doi: 10.1109/TCDS.2020.3044366
Rundell, M. (ed.)., (2007). The Macmillan English Dictionary for Advanced Learners, 2nd Edn. Macmillan Education.
Scharlau, I., Karsten, A., and Rohlfing, K. J. (2020). Building, emptying out, or dreaming? Action structures and space in undergraduates' metaphors of academic writing. J. Writ. Res. 12, 493–529. doi: 10.17239/jowr-2021.12.03.01
Scharlau, I., Körber, M., and Karsten, A. (2019). Plunging into a world? A novel approach to undergraduates' metaphors of reading. Frontl. Learn. Res. 9, 25–57. doi: 10.14786/flr.v7i4.559
Scherer, A. M., Scherer, L. D., and Fagerlin, A. (2015). Getting ahead of illness: using metaphors to influence medical decision making. Med. Decision Mak. 35, 37–45. doi: 10.1177/0272989X14522547
Steen, G. (2002). Towards a procedure for metaphor identification. Lang. Literat. 11, 17–33. doi: 10.1177/096394700201100103
Steen, G. (2008). The paradox of metaphor: why we need a three-dimensional model of metaphor. Metaphor Symbol 23, 213–241. doi: 10.1080/10926480802426753
Steen, G. (2017). Deliberate metaphor theory: basic assumptions, main tenets, urgent issues. Intercult. Pragmat. 14, 1–24. doi: 10.1515/ip-2017-0001
Steen, G. J. (2007). Finding Metaphor in Grammar and Usage: A Methodological Analysis of Theory and Research. Amsterdam: John Benjamins.
Steen, G. J. (2023). Thinking by metaphor, fast and slow: deliberate metaphor theory offers a new model for metaphor and its comprehension. Front. Psychol. 14:1242888. doi: 10.3389/fpsyg.2023.1242888
Steen, G. J., Dorst, A. G., Herrmann, J. B., Kaal, A. A., and Krennmayr, T. (2010a). Metaphor in usage. Cogn. Linguist. 21, 765–796. doi: 10.1515/cogl.2010.024
Steen, G. J., Dorst, A. G., Herrmann, J. B., Kaal, A. A., Krennmayr, T., and Pasma, T. (2010b). A Method for Linguistic Metaphor Identification: From MIP to MIPVU. Amsterdam: John Benjamins.
Sullivan, K. (2016). Integrating constructional semantics and conceptual metaphor. Construct. Fram. 8, 141–165. doi: 10.1075/cf.8.2.02sul
Thibodeau, P. H., Crow, L., and Flusberg, S. J. (2017). The metaphor police: a case study of the of metaphor in explanation. Psychon. Bullet. Rev. 24, 1375–1386. doi: 10.3758/s13423-016-1192-5
Thibodeau, P. H., Matlock, T., and Flusberg, S. J. (2019). The role of metaphor in communication and thought. Lang. Linguist. Compass 13:e12327. doi: 10.1111/lnc3.12327
Türk, O., Lazarov, S., Wang, Y., Buschmeier, H., Grimminger, A., and Wagner, P. (2024). “Predictability of understanding in explanatory interactions based on multimodal cues,” in Proceedings of the 26th ACM International Conference on Multimodal Interaction (ICMI '24), November 04-08. 2024, San Jose, Costa Rica. New York, NY: ACM.
Wachsmuth, H., and Alshomary, M. (2022). ““Mama always had a way of explaining things so I could understand”: a dialogue corpus for learning to construct explanations,” in Proceedings of the 29th International Conference on Computational Linguistics (Gyeongju), 344–354.
Wan, W., and Low, G. (2015). Elicited Metaphor Analysis in Educational Discourse. Amsterdam: John Benjamins.
Wired (2024). 5 Levels. Available at: https://www.wired.com/video/series/5-levels (accessed November 10, 2024).
Keywords: metaphor, conceptual metaphor, conceptual metaphor theory, metaphor usage, explaining, explanation
Citation: Scharlau I, Körber M, Sengupta M and Wachsmuth H (2024) When to use a metaphor: metaphors in dialogical explanations with addressees of different expertise. Front. Lang. Sci. 3:1474924. doi: 10.3389/flang.2024.1474924
Received: 02 August 2024; Accepted: 25 October 2024;
Published: 18 December 2024.
Edited by:
Liliana Tolchinsky, University of Barcelona, SpainReviewed by:
Dorota Katarzyna Gaskins, King's College London, United KingdomXia Zhao, Jiangsu University of Science and Technology, China
Copyright © 2024 Scharlau, Körber, Sengupta and Wachsmuth. This is an open-access article distributed under the terms of the Creative Commons Attribution License (CC BY). The use, distribution or reproduction in other forums is permitted, provided the original author(s) and the copyright owner(s) are credited and that the original publication in this journal is cited, in accordance with accepted academic practice. No use, distribution or reproduction is permitted which does not comply with these terms.
*Correspondence: Ingrid Scharlau, aW5ncmlkLnNjaGFybGF1QHVuaS1wYWRlcmJvcm4uZGU=