- 1Institutes of Humanities and Social Sciences, University of Tsukuba, Tsukuba, Japan
- 2Department of Linguistics, Seoul National University, Seoul, Republic of Korea
- 3School of International Liberal Studies, Waseda University, Tokyo, Japan
- 4Graduate School of International Culture and Communication Studies, Waseda University, Tokyo, Japan
- 5The MARCS Institute for Brain, Behaviour and Development, Western Sydney University, Sydney, NSW, Australia
One of the primary questions of second language (L2) acquisition research is how a new sound category is formed to allow for an L2 contrast that does not exist in the learner's first language (L1). Most models rely crucially on perceived (dis)similarities between L1 and L2 sounds, but a precise definition of what constitutes “similarity” has long proven elusive. The current study proposes that perceived cross-linguistic similarities are based on feature-level representations, not segmental categories. We investigate how L1 Japanese listeners learn to establish a new category for L2 American English /æ/ through a perception experiment and computational, phonological modeling. Our experimental results reveal that intermediate-level Japanese learners of English perceive /æ/ as an unusually fronted deviant of Japanese /a/. We implemented two versions of the Second Language Linguistic Perception (L2LP) model with Stochastic Optimality Theory—one mapping acoustic cues to segmental categories and another to features—and compared their simulated learning results to the experimental results. The segmental model was theoretically inadequate as it was unable explain how L1 Japanese listeners notice the deviance of /æ/ from /a/ in the first place, and was also practically implausible because the predicted overall perception patterns were too native English-like compared to real learners' perception. The featural model, however, showed that the deviance of /æ/ could be perceived due to an ill-formed combination of height and backness features, namely */low, front/. The featural model, therefore, reflected the experimental results more closely, where a new category was formed for /æ/ but not for other L2 vowels /ɛ/, /ʌ/, and /ɑ/, which although acoustically deviate from L1 /e/, /a/, and /o/, are nonetheless featurally well-formed in L1 Japanese, namely /mid, front/, /low, central/, and /mid, back/. The benefits of a feature-based approach for L2LP and other L2 models, as well as future directions for extending the approach, are discussed.
1 Introduction
Second language (L2) learners often encounter a “new” sound that does not exist in their first language (L1). Establishing a phonological representation for such new sounds is essential to L2 learning, because otherwise the lexical distinctions denoted by the phonological contrast cannot be made for successful communication. Various models have been proposed to explain how a new sound category may develop in the learner's mind, with most models focusing on the cross-linguistic perceptual relationships between L1 and L2 sounds, although the exact underlying mechanism remains to be elucidated. In this study, we propose that the process of L2 category formation can be better explained by assuming feature-level representations as the fundamental unit of perception, rather than segmental categories.1 To this end, we compare two versions of formal modeling, i.e., segment- and feature-based, of how L1 Japanese listeners form a new category for L2 American English (AmE) /æ/2 by implementing the theoretical predictions of the Second Language Linguistic Perception (L2LP) model (Escudero, 2005; van Leussen and Escudero, 2015; Escudero and Yazawa, in press) with a computational-phonological approach of Stochastic Optimality Theory (StOT; Boersma, 1998).
In the field of L2 speech perception research, two models have been particularly dominant over the last few decades (Chen and Chang, 2022): the Speech Learning Model (SLM; Flege, 1995; Flege and Bohn, 2021) and the Perceptual Assimilation Model (PAM; Best, 1995; Best and Tyler, 2007). According to SLM, learners can form a new category for an L2 sound if they discern its phonetic difference(s) from the closest L1 category and, if not, a single composite category will be used to process both L1 and L2 sounds. The likelihood of category formation therefore depends primarily on the perceived cross-linguistic phonetic dissimilarity, but other factors such as the quantity and quality of L2 input obtained in meaningful conversations are said to be also relevant. PAM agrees with SLM in that perceived cross-linguistic dissimilarity guides category formation, but with the caveat that assimilation occurs not only at the phonetic level but also at the phonological or lexical-functional level. For example, if there are many minimal pairs involving an L2 contrast that assimilates phonetically to a single L1 category, the increased communicative pressure can lead to the formation of a new phonological category to allow for distinct phonological representations of these lexical items (e.g., AmE [ʌ] and [ɑ] both being assimilated to Japanese [a], but AmE nut [nʌt] and not [nɑt] leading to Japanese /na1t/ and /na2t/, where /a1/ and /a2/ are distinct phonological categories that occupy phonetically overlapping but distinct parts of a single L1 category). While the predictions of both models have been supported by numerous studies, there is one fundamental issue that remains to be resolved: It is unclear on what basis categorical similarity should be defined. In the words of Best and Tyler (2007, p.26), “one issue [...] has not yet received adequate treatment in any model of nonnative or L2 speech perception: How listeners identify nonnative phones as equivalent to L1 phones, and the level(s) at which this occurs.” Over a decade later, Flege and Bohn (2021, p.31) restated the unresolved problem: “It remains to be determined how best to measure cross-language phonetic dissimilarity. The importance of doing so is widely accepted but a standard measurement procedure has not yet emerged.”
To illustrate this elusive goal with a concrete example, consider our case of L1 Japanese listeners learning /æ/ and adjacent vowels in L2 AmE. In cross-linguistic categorization experiments, Strange et al. (1998) found that the AmE vowel was perceived as a very poor exemplar of Japanese /a(a)/,3 receiving the lowest mean goodness-of-fit rating (two out of seven) among all AmE vowel categories, while spectrally adjacent /ɛ/, /ʌ/, and /ɑ/ received higher ratings as Japanese /e/ (four out of seven), /a/ (four out of seven), and /a(a)/4 (six out of seven), respectively. Duration-based categorization of AmE vowels as Japanese long and short vowels was observed when the stimuli were embedded in a carrier sentence, but not when they were presented in isolation. Shinohara et al. (2019) further found that the category goodness of synthetic vowel stimuli as Japanese /a/ deteriorated as the second formant (F2) frequency was increased. These studies suggest that AmE /æ/ is perceptually dissimilar from L1 Japanese /a(a)/, presumably in terms of F2 but possibly in conjunction with other cues such as the first formant (F1) frequency and duration, and is thus subject to new category formation according to SLM and PAM. L2 perception studies on Japanese listeners also showed that the AmE /æ/-/ʌ/ contrast was more discriminable than the /ɑ/-/ʌ/ contrast (Hisagi et al., 2021; Shafer et al., 2021; Shinohara et al., 2022) and that AmE /æ/ was identified with higher accuracy than /ʌ/ or /ɑ/ (Lambacher et al., 2005). These results imply that Japanese listeners perceptually distinguish AmE /æ/ from AmE /ʌ/ and /ɑ/, which themselves are assimilated to Japanese /a(a)/. However, it remains unclear why only AmE /æ/ would be perceptually distinct in the first place. Spectral distance between the L1 and L2 categories in Figure 1, which shows the production of Japanese and AmE vowels by four native speakers of each language (Nishi et al., 2008), does not seem to predict perceived category goodness very well. For example, the figure shows that AmE /ʌ/ is spectrally closer than AmE /ɑ/ to Japanese /a(a)/, but it was the latter AmE vowel that was judged to be a better fit in Strange et al. (1998). AmE /ɛ/ is also quite far from Japanese /e/ in spectral distance, but its perceived category goodness as Japanese /e/ was nonetheless as high as that of AmE /ʌ/ as Japanese /a/. One may attribute this pattern to duration given the duration-based categorization in Strange et al. (1998), but the actual duration of the target vowels (Figure 2) does not seem to provide useful clues, either. For example, since AmE /ɛ/ and /ʌ/ have almost identical duration values, the former is acoustically more distant from the L1 categories after all, despite both AmE vowels receiving equal goodness ratings. This brings us back to the question: How is L1-L2 perceptual dissimilarity determined?
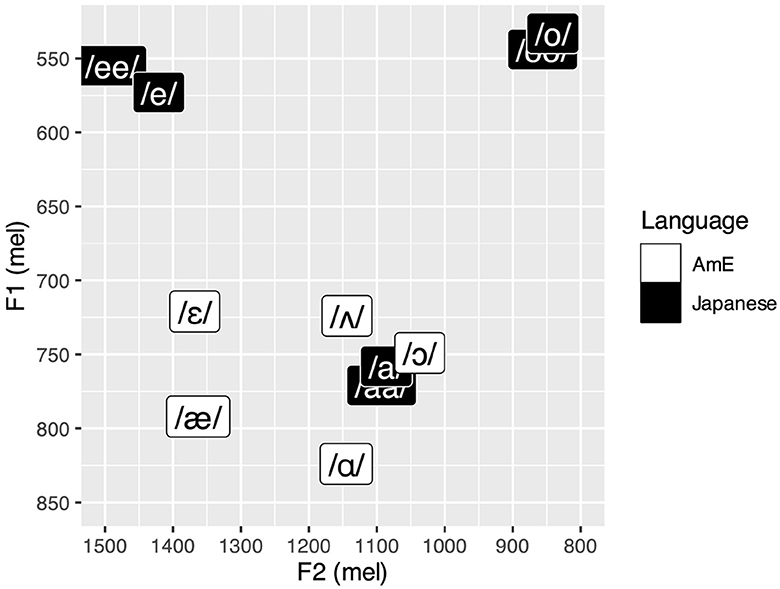
Figure 1. Mel-converted average F1 and F2 frequencies of relevant AmE and Japanese vowels. Adapted with permission from Nishi et al. (2008), licensed under Copyright 2008, Acoustical Society of America.
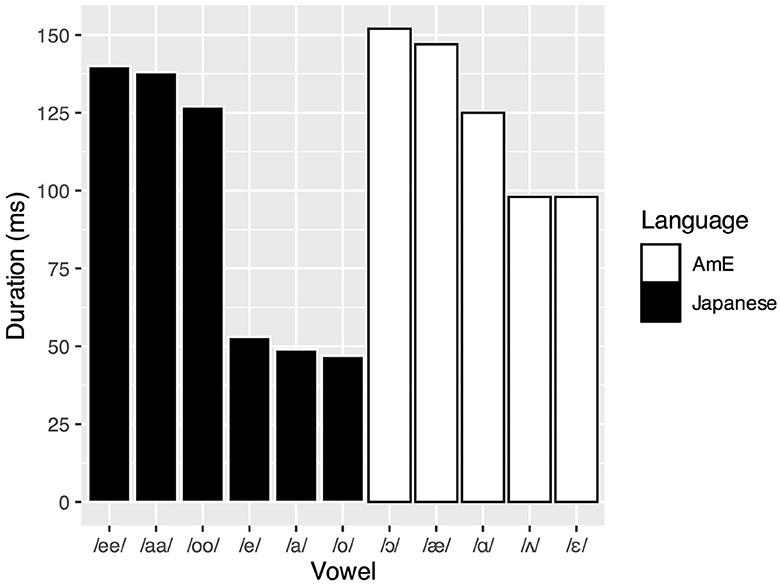
Figure 2. Average duration of relevant AmE and Japanese vowels. Adapted with permission from Nishi et al. (2008), licensed under Copyright 2008, Acoustical Society of America.
The L2LP model approaches L2 category formation from a different perspective. While the model shares with SLM and PAM the view that perceptual learning is both auditory- and meaning-driven, it is unique in assuming the interplay of multiple levels of linguistic representations. Although many L2LP studies have focused on the perceptual mapping of acoustic cues onto segmental representations, some have also incorporated feature-level representations, which may be useful for modeling the perception of new L2 sounds. For example, Escudero and Boersma (2004) proposed that L1 Spanish learners' overuse of the duration cue in perceiving L2 Southern British English (SBE) /i:/-/ɪ/ contrast can be adequately modeled by assuming that the vowels are perceptually represented as “/i, long/” and “/i, short/,” respectively. That is, the learners developed a new length feature that does not exist in their L1 (because vowel length is non-phonemic in Spanish) and integrated the feature with an existing L1 segmental representation, yielding a perceptual pattern that is not seen in either Spanish or English. This type of learning scenario is called the UNFAMILIAR NEW scenario in L2LP, where L2 representations outnumber L1 representations and thus learners must establish a new category to bridge the cross-linguistic gap (hence NEW) but an important cue for the L2 contrast is not utilized in L1 phonology (hence UNFAMILIAR). The current learning scenario of our interest is also considered NEW because AmE has more vowels than Japanese, but the necessary cues for optimal perception of the target L2 vowels—F1, F2, and duration—are all FAMILIAR (because Japanese vowels contrast in height, backness, and length). We hypothesize that a feature-based modeling as in Escudero and Boersma (2004) may also be useful for modeling the FAMILIAR NEW scenario, although no previous study has formally tested this possibility yet. Another unique characteristic of L2LP is that the model's theoretical components can be computationally implemented, or simulated, to provide more concrete and testable predictions. While various computational frameworks can be used for this purpose, previous studies have generally used StOT (Escudero and Boersma, 2004; Boersma and Escudero, 2008; Yazawa et al., 2020) because it outperforms other machine learning algorithms (Escudero et al., 2007) and is compatible with the phonological theory of Optimality Theory (OT; Prince and Smolensky, 1993). The current study follows this line of work and evaluates how segment- and feature-based StOT modeling compare in explaining the process of new L2 category formation.
The incentive for feature-based modeling is not only theoretically grounded but also empirically motivated, as emerging evidence suggests the involvement of features in L1 and L2 perception. With respect to native perception, Scharinger et al. (2011) used magnetoencephalography (MEG) to map the entire Turkish vowel space onto cortical locations and found that dipole locations could be structured in terms of features (height, backness, and roundedness) rather than raw acoustic cues (F1, F2, and F3). Mesgarani et al. (2014) further used high-density direct cortical surface recordings to reveal the representation of the entire AmE sound inventory, finding response selectivity at single electrodes corresponding to features (voice, place, manner, height, and backness) rather than individual vowels and consonants. Given these results, it seems reasonable to assume that L2 sounds are also perceived through (L1) features. While most L2 perception studies have focused on segmental categories, some have explored the potential role of L1 features, with a prominent focus on phonological length (and lack thereof). Perhaps the best known study is McAllister et al. (2002), who compared the perception of L2 Swedish vowel length by L1 listeners of Estonian, AmE, and Spanish, where only Estonian has contrastive vowel length. The study found that the Estonian group outperformed the other two groups in perceptual accuracy, suggesting that the L1 length feature is positively transferred to L2 perception or, to put it another way, the lack of the length feature is negatively transferred. Pajak and Levy (2014) extended this finding by showing that native listeners of a language with vowel length contrasts showed enhanced discrimination of nonnative consonant length contrasts (i.e., geminates). This finding suggests that the L1 length feature may be shared across vowels and consonants, which appears to be accessible in L2 perception. Research on native Australian English listners (Tsukada, 2012; Tsukada et al., 2018; Yazawa et al., 2023) has also found that they can discriminate and identify Japanese vowel and consonant length contrasts fairly well without any prior knowledge or training, contrary to native AmE listeners struggling to learn the contrasts (Hirata, 2004, 2017). Taken together, previous research suggests that the presence or absence of a certain feature in the L1 (or its specific variety) predicts the ease or difficulty of L2 perception. However, to our knowledge, no prior study has provided a formal account of how existing L1 features mediate L2 category formation, which is what we aim to achieve in this study.
The remainder of this paper is organized as follows. First, in Section 2, we present a forced-choice perception experiment that investigates the use of spectral and temporal cues in the perception of the L1 Japanese and L2 AmE vowels of interest. This is intended to complement the previous studies, which did not investigate potential effects of F1 and duration cues. Section 3 then presents a formal computational modeling of new L2 category formation within the L2LP framework. Two versions of StOT-based simulations are compared, namely segmental and featural, to evaluate which better explains and replicates the experimental results. We then discuss the experimental and computational results together in Section 4, addressing the implications of feature-based modeling for L2 speech perception models (i.e., L2LP, SLM, and PAM) as well as the directions for future research. Finally, Section 5 draws the conclusion.
2 Experiment
The perception experiment reported in this section was designed to investigate how L2 AmE /æ/ and adjacent vowels are perceived in relation to L1 Japanese vowels based on three acoustic cues (F1, F2, and duration), to help model the category formation and cross-linguistic assimilation processes. Following our previous study (Yazawa et al., 2020), the experiment manipulates the ambient language context to elicit L1- and L2-specific perception modes without changing the relevant acoustic properties of the stimuli, as detailed below.
2.1 Participants
Thirty-six native Japanese listeners (22 male, 14 female) participated in the experiment. They were undergraduate or graduate students at Waseda University, Tokyo, Japan, between the ages of 18 and 35 (mean = 21.25, standard deviation = 2.97). All participants had received six years of compulsory English language education in Japanese secondary schools (from ages 13 to 18), which focused primarily on reading and grammar. They had also received some additional English instruction during college, the quality and quantity of which varied according to the courses they were enrolled in. None of the participants had spent more than a total of three months outside of Japan. TOEIC was the most common standardized test of English proficiency taken by the participants (n = 18), with a mean score of 688 (i.e., intermediate level). All participants reported normal hearing.
2.2 Stimuli
Two sets of stimuli—“Japanese” (JP) and “English” (EN)—were prepared. Both had the same phonetic form [bVs], with the spectral and temporal properties of the vowel varying in an identical manner. The JP stimuli were created from a natural token of the Japanese loanword baasu /baasu/ “birth,” as produced by a male native Japanese speaker from Tokyo, Japan. The token was phonetically realized as [ba:s] because Japanese /u/ can devoice or delete word-finally (Shaw and Kawahara, 2017; Whang and Yazawa, 2023). The EN stimuli, on the other hand, were created from a natural token of the English word bus /bʌs/, as produced by a male native AmE speaker from Minnesota, United States. For both tokens, the F1, F2, and duration of the vowel were manipulated with STRAIGHT (Kawahara, 2006) to vary in four psychoacoustically equidistant steps: F1 at 700, 750, 800, and 850 mel; F2 at 1,100, 1,200, 1,300, and 1,400 mel; and duration at 100, 114, 131, and 150 ms (i.e., natural logarithm). These steps were intended to fully cover the spectral and temporal variability of AmE /ɛ/, /æ/, /ʌ/, and /ɑ/, while also partially covering that of Japanese /ee/, /e/, /aa/, and /a/ (Figures 1, 2). The third formant (F3) was set to 1,700 mel. The fundamental frequency and intensity contours were also changed to have a mean of 120 Hz and a peak of 70 dB, respectively. The manipulations resulted in a total of 64 (4 × 4 × 4) stimuli for each of the two language sets.
2.3 Procedure
The experiment included two sessions—again “Japanese” (JP) and “English” (EN)—using the JP and EN stimuli, respectively. In order to elicit language-specific perception modes across sessions, all instructions, both oral and written, were given only in the language of the session. The two sessions were consecutive, and the session order was counterbalanced across participants to control for order effects; 18 participants (11 male, seven female) attended the EN session first, while the other 18 (11 male, seven female) attended the JP session first. In the JP session, participants were first presented with each of the 64 JP stimuli in random order and then chose one of the following four words that best matched what they had heard: beesu /beesu/ “base,” besu /besu/ “Bess,” baasu /baasu/ “birth,” and basu /basu/ “bus.” The choices are all existing loanwords in Japanese and were written in katakana orthography. Participants were instructed that they were not required to use all of the four choices. The block of 64 trials was repeated four times, with a short break in between, giving a total of 256 (64 × 4) trials for the session. The EN session followed a similar a procedure, where participants categorized the randomized 64 EN stimuli as the following four real English words (though there was no requirement to use all choices): Bess (/bɛs/), bass (/bæs/5), bus (/bʌs/), and boss (/bɑs/). The stimulus block was again repeated four times, for a total of 256 trials for the session.
Participants were tested individually in an anechoic chamber, seated in front of a MacBook Pro laptop running the experiment in the Praat ExperimentMFC format (Boersma and Weenink, 2023) and wearing Sennheiser HD 380 Pro headphones through which the stimuli were played at a comfortable volume. The entire experiment took ~30 to 40 min to complete, for which monetary compensation was provided.
2.4 Analysis
In order to quantify the participants' use of the acoustic cues, a logistic regression analysis was performed on the obtained response data per session and per response category, using the glm() function in R (R Core Team, 2023). The model structure is as follows:
where P is the probability that a given response category (e.g., JP /aa/) is chosen, and 1 − P is the probability that the other three categories (e.g., JP /ee/, /e/, or /aa/) are chosen. The odds is log-transformed to fit a sigmoidal curve to the data, which is more appropriate than the straight line of a linear regression model for analyzing speech perception data. The intercept α is the bias coefficient, which reflects how likely the particular response category is to be chosen in general. The stimulus-tuned coefficients βs represent the extent to which the F1, F2, and duration steps, coded from “1” (smallest) to “4” (largest), cause a change in the likelihood of the response category being chosen.
2.5 Results
Table 1 shows the results of the logistic regression analyses on all participants' pooled data. The coefficients βF1, βF2, and βdur can be plotted to graphically represent the estimated locations of response categories in the stimulus space (Morrison, 2007), which are shown in Figure 3.
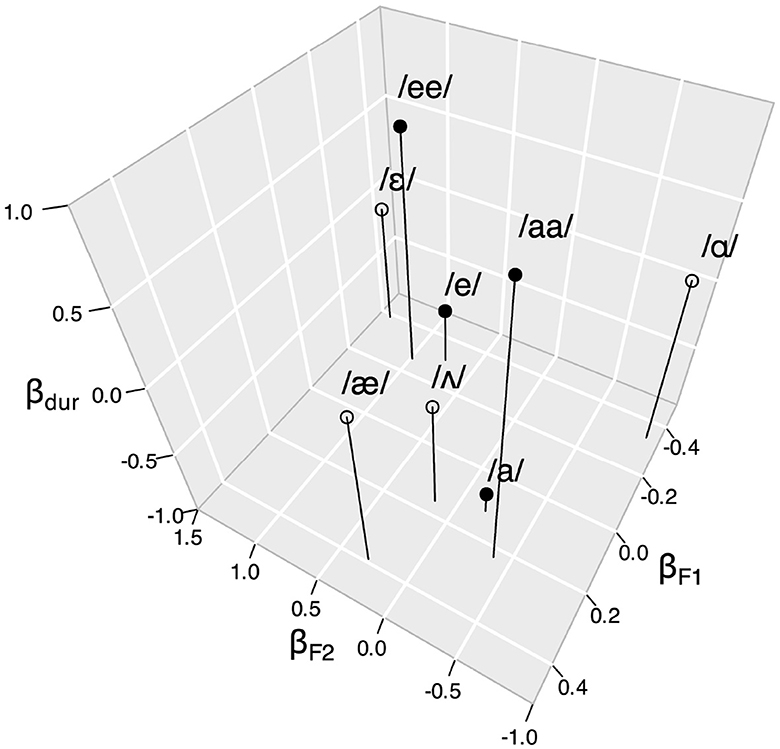
Figure 3. Plot of logistic regression coefficients in Table 1 (black = JP, white = EN).
Let us briefly examine the overall response patterns in the figure. Regarding the JP responses, the relative positions of /e/ and /a/ on the βF1-βF2 plane are as expected, since mid front /e/ should show lower βF1 and higher βF2 than low central /a/. Phonologically long /ee/ and /aa/ are proximal to their short counterparts in βF1 and βF2, but larger in βdur. This is consistent with the traditional description of Japanese long vowels as a sequence of two identical vowels at the phonological level. As for the EN responses, the relative positions of /ɛ/ and /ʌ/ are similar to those of JP /e/ and /a/, while /æ/ seems to be somewhat distant, on the βF1-βF2 plane. Far away from all other categories is /ɑ/, with very low βF1 and βF2. As for βdur, the four EN categories seem to occupy an intermediate position between JP long and short categories.
To further investigate the response patterns, linear mixed-effects (LME) models were applied to the by-participant results of the logistic regression analyses, using the lme4 (Bates et al., 2015) and lmerTest (Kuznetsova et al., 2017) packages in R. Each model is structured as follows:
The model tests whether the response categories differ on a stimulus-tuned coefficient (βF1, βF2, or βdur) at a statistically significant level, controlling for the potential variability across participants and session order. Note that both JP and EN categories are included in the model, as the coefficients can in principle be compared across sessions, since the JP and EN stimuli share the same acoustic properties.
The LME model for βF1 with JP /a/ as the reference level showed significantly smaller estimates for EN /ɛ/ (β = −1.110, s.d. = 0.265, t = −4.180, p < 0.001) and /ɑ/ (β = −0.561, s.d. = 0.265, t = −2.116, p = 0.035), suggesting that the two EN categories are higher in perceived vowel height than the reference. The model for βF2 also yielded significantly larger estimates for EN /ɛ/ (β = 5.391, s.d. = 0.372, t = 14.492, p < 0.001) and /æ/ (β = 1.248, s.d. = 0.372, t = 3.355, p < 0.001), as well as a significantly smaller estimate for EN /ɑ/ (β = −0.892, s.d. = 0.372, t = −2.397, p = 0.017), than the reference JP /a/. This suggests that EN /ɛ/ and /æ/ are perceptually represented as more fronted, and EN /ɑ/ as more back, than JP /a/. No significant difference was found between EN /ʌ/ and JP /a/ in either βF1 or βF2. As for βdur, all EN categories had significantly larger estimates than the reference JP /a/ (p < 0.05 for EN /ʌ/ and ps < 0.001 for /ɛ/, /æ/, and /ɑ/). An additional LME model with EN /ʌ/ as reference found significantly larger βdur estimates for JP /ee/ (β = 1.646, s.d. = 0.378, t = 4.359, p < 0.001) and /aa/ (β = 1.423, s.d. = 0.378, t = 3.768, p < 0.001), but no significant difference was found for the other three EN categories. The results suggest that the four EN categories are represented with an intermediate perceptual duration between the long and short JP categories, with no significant difference between the EN categories themselves.
2.6 Interpretation
The above results can be interpreted as follows. First, AmE /ɛ/ and /ʌ/ are qualitatively assimilated to Japanese /e/ and /a/, given the similar βF1 and βF2 estimates between EN /ɛ/ and JP /e/ and between EN /ʌ/ and JP /a/, respectively. If a separate category had been formed for AmE /ɛ/, which is lower in phonetic height than Japanese /e/, then βF1 for EN /ɛ/ should have been larger than that for JP /e/, but this was not the case. Also, given the non-significant differences in βF1 and βF2 between EN /ʌ/ and JP /a/, it is unlikely that AmE /ʌ/ was reliably discriminated from Japanese /a/. In contrast, AmE /æ/ was most likely perceived as a separate category. Given its significantly larger βF2 than JP /a/, the AmE vowel may be represented as “a fronted version of /a/.” While these results are consistent with previous findings, it has additionally been shown that AmE /æ/ is distinguished from Japanese /a/ by the F2 cue and not by the F1 cue.
The result for EN /ɑ/, however, was somewhat unexpected. Although AmE /ɑ/ is reported to be qualitatively assimilated to Japanese /a/ (Strange et al., 1998), the βF1 and βF2 estimates for EN /ɑ/ responses were significantly lower than for JP /a/. There are a few possible explanations for this finding. First, the learners may have associated AmE /ɑ/ with Japanese /o/ at the orthographic level, since the AmE sound is often written with “o” (e.g., boss, lot, not), as is the Japanese sound when written in the Roman alphabet (e.g., bosu /bosu/ “boss”). This possibility is particularly plausible because the participants had learned English mostly in written rather than oral form. Second, the participants may have been referring to AmE /ɔ/ rather than /ɑ/ when they chose boss as their response. The experimental design assumed that the vowel in boss is /ɑ/ because of the widespread and ongoing low back merger in many dialects of AmE (Labov et al., 2006), but some AmE speakers may still maintain the contrast and produce the word with /ɔ/, which would be perceptually assimilated to the Japanese /o/ quality (Strange et al., 1998). These two possibilities are complementary rather than mutually exclusive, and they both indicate that the very low βF1 and βF2 for the participants' boss responses can be attributed to Japanese /o/.
Finally, it is worth noting that the duration cue was not utilized very actively in the EN session. Judging from their intermediate βdur between JP long and short categories, the AmE vowel categories appear to be unspecified in terms of phonological length. This result is consistent with Strange et al. (1998)'s finding that Japanese listeners did not show duration-based categorization when AmE vowels were presented in isolation as in the current experiment.
3 Simulation
Following the above experimental results, we now present in this section a formal computational modeling of how L1 Japanese listeners may develop a new sound category for L2 AmE /æ/ (or not for other categories) within the L2LP framework. We compare two versions of simulations using StOT, one segment- and the other feature-based, as they make divergent predictions about how L1 and L2 linguistic experience shapes listeners' perception. These predictions are compared with the experimental result to evaluate which version is more plausible. We begin by outlining the general procedure of the simulations, followed by the segmental and then by featural simulations.
3.1 General procedure
With StOT, speech perception can be modeled with a set of Optimality Theoretic, negatively formulated cue constraints (Escudero, 2005, 2009; Boersma and Escudero, 2008; Boersma, 2009) that modulate the mapping of acoustic cues (e.g., [F1 = 700 mel]) onto phonological representations (i.e., segmental categories or distinctive features in our case). StOT differs from regular OT in that constraints are arranged on a continuous rather than a discrete ranking scale, and constraint rankings are allowed to shift rather than being fixed. Each constraint is assigned a ranking value representing the stringency of the constraint (e.g., 100.0). At each time of evaluation, the ranking value is temporarily perturbed by a random value called evaluation noise, drawn from a normal distribution with a mean of 0 and a specified standard deviation (e.g., 2.0). The resulting value, called selection point, is used to evaluate the candidates. For example, if a constraint C1 has a ranking value of 100.0 and the evaluation noise is 2.0, then the selection point for that constraint can be 100.4, 101.5, 99.3, etc. at each evaluation. Since the selection points change each time, the constraint rankings are not absolute as in regular OT (e.g., C1 > C2) but are probabilistic (e.g., C1 with a ranking value of 100.0 will usually outrank C2 with a ranking value of 98.0, but the latter constraint may outrank the former in some cases). This allows StOT to deal with probabilistic variation in speech perception.
The ranking values of the constraints are not determined manually, but are learned computationally from the input data through the Gradual Learning Algorithm (GLA), an error-driven algorithm for learning optimal constraint rankings in StOT (Boersma and Hayes, 2001). GLA is error-driven in that it adjusts the ranking values of relevant constraints when there is a mismatch between the output and the correct form, which the listeners are assumed to have access to via lexical knowledge and semantic context.6 Specifically, the ranking values of the constraints that lead to the incorrect winner are increased (i.e., strengthened), while those of the constraints that would lead to the correct form are decreased (i.e., weakened). The degree to which the ranking values can change is set by a small number called plasticity (e.g., 1.0), which simulates the learner's current neural or cognitive plasticity. The plasticity is set to gradually decrease over time, so that learning is fast but imprecise at an early stage (infancy and childhood) and slow but precise at a later stage (adulthood). The overall scheme allows GLA to model the effects of the lexicon and age on perceptual learning.
The segmental and featural versions of the simulations use the above two computational tools, with the same parameter settings whenever possible. All constraints have an initial ranking value of 100.0, and the evaluation noise is fixed at 2.0. The plasticity is initially set to 1.0, decreasing by a factor of 0.7 per virtual year. The number of yearly input tokens was 10,000. These settings are mostly taken from previous studies, Boersma and Escudero (2008) in particular. To compare the results of the simulations with those of the experiment, we restrict the relevant auditory information provided to our virtual listeners to a range of F1 from 700 to 850 mel and a range of F2 from 1,100 to 1,400 mel, i.e., the same as the spectral stimulus space in the experiment. Duration is not included in the simulations because the target L2 AmE vowels appear to be unspecified in terms of length. Similar to the F1 and F2 steps in the experiment, the F1 and F2 ranges are divided into “bins” of equal width on the mel scale. While four bins per range would allow for a direct comparison between the experiment and the simulation, each range was assigned 16 bins for more precise modeling; as discussed in more detail in Sections 3.2 and 3.3, using 16 bins also effectively illustrates how a range of acoustic values map to certain abstract categories or features. Thus, there are 16 F1 bins with a width of 10 mel (i.e., [F1 = 700 mel], [F1 = 710 mel], ... [F1 = 850 mel]) and 16 F2 bins with a width of 20 mel ([F2 = 1,100 mel], [F2 = 11,20 mel], ... [F2 = 1,400 mel]), all of which receive a cue constraint.
The input data for training the virtual listeners are randomly generated using the parameters in Table 2. The mean formant values are taken from Nishi et al. (2008), as shown in Figure 1. The standard deviations are approximate estimates based on the formant plots in the study, as specific values are not available. Japanese /o/ is included here because it is necessary to model the perception of the AmE boss vowel. For simplicity, the three Japanese vowels /e/, /a/, and /o/ are assumed to occur at the same frequency (33.3%), as are the four AmE vowels /ɛ/, /æ/, /ʌ/, and /ɑ/ (25.0%). Although we are not entirely sure about the status of the AmE boss vowel, our virtual learners will hear both [ɑ] and [ɔ] tokens equally often (i.e., 12.5%), although there is only one target category /ɑ/ to acquire because the low back contrast is optional. In other words, the learners hear both merged and unmerged speakers but will eventually become merged listeners themselves, which we believe is a feasible scenario.
In the following two sections, we present how segmental and featural versions of virtual StOT listeners, trained with the same L1 Japanese and L2 AmE input, may develop a new category for /æ/ (and not for other AmE vowels), like the real listeners in our experiment. Each section begins with a brief illustration of cue constraints, namely cue-to-segment or cue-to-feature constraints. In line with the Full Transfer hypothesis of L2 acquisition (Schwartz and Sprouse, 1996), L2LP assumes that the initial state of L2 perception is a Full Copy of the end-state L1 grammar. Thus, we first train the perception grammar with Japanese input tokens for a total of 12 virtual years, which is copied to serve as the basis for L2 speech perception. Based on L2LP's further assumption that L2 learners have Full Access (Schwartz and Sprouse, 1996) to L1-like learning mechanisms, the copied perception grammar is then trained with AmE input in the same way, but with a decreased plasticity (1.0 × 0.712 = 0.014 at age 12, which further decreases by a factor of 0.7 per year).
3.2 Segmental simulation
3.2.1 Cue-to-segment constraints
Most previous studies aimed at formally modeling the process of L2 speech perception within L2LP (e.g., Escudero and Boersma, 2004; Boersma and Escudero, 2008; Yazawa et al., 2020) used segment-based cue constraints, such as “a value of x on the auditory continuum f should not be mapped to the phonological category y,” which we also use here. For instance, suppose that a Japanese listener hears a vowel token with [F1 = 850 mel] and [F2 = 1,400 mel] (i.e., an [æ]-like token, cf. Figure 1) and perceives it as either /e/ or /a/. Table 3 shows how this can be modeled by a total of 4 cue constraints, each of which prohibits the perception of a segmental category (e.g., */e/) based on an acoustic cue (e.g., [F1 = 850 mel]). At the top of the leftmost column is the perceptual input, i.e., the vowel token, followed in the same column by candidates for the perceptual output, i.e., what the listener perceives given the input. In this example, the constraint “[F2 = 1,400 mel] */a/” happens to outrank the constraint “[F1 = 850 mel] */e/,” making the candidate /e/ as the winner.
Note, however, that the same vowel token will not always be perceived as /e/ due to the probabilistic nature of StOT. It is possible that in some cases the constraint “[F1 = 850 mel] */e/” will outrank “[F2 = 1,400 mel] */a/,” making /a/ as the alternative winner. The probability of such an evaluation is increased by GLA if and when the listener notices that the intended from should be /a/ rather than /e/ through their lexical knowledge and the semantic context (e.g., aki “autumn” should have been perceived instead of eki “station” given the conversational context). Table 4 illustrates how such learning takes place. Here, the ranking values of the constraints that led to the perception of the incorrect winner (“✓”) are increased (“←”), while the ranking values of the constraints that would lead to the correct form (“”) are decreased (“ → ”), by the current plasticity value. This makes it more likely that the same token will be perceived as /a/ rather than /e/ in future evaluations.
3.2.2 L1 perception
Our virtual segmental learner starts with a “blank” perception grammar, which has a total of 96 cue-to-segment constraints (16 F1 bins + 16 F2 bins, multiplied by three segmental categories /e/, /a/, and /o/), all ranked at the same initial value of 100.0.7 The learner then begins to receive L1 input, namely random tokens of Japanese /e/, /a/, and /o/, which occur with equal frequency. The formant values of each vowel token is randomly determined based on the means and standard deviations in Table 2, which are then rounded to the nearest bins to be evaluated by the corresponding constraints. Whenever there is a mismatch between the perceived and intended forms, GLA updates the ranking values of the relevant cue constraints by adding or subtracting the current plasticity value.
Figure 4 shows the result of L1 learning. The grammar was tested 100 times on each combination of F1 and F2 bins. The vertical axis in the figure shows the probability of segmental categories being perceived given the F1-F2 bin combination, as calculated by logistic regression analyses as in (1) but without the duration coefficients. It can be seen that the virtual listener perceives /e/ when F1 is low and F2 is high, and /a/ when F1 is high and F2 is low, similar to the perception patterns of the real listeners in the experiment (cf. Figure 3). Note that /o/ can also be perceived when F1 and F2 are both very low.
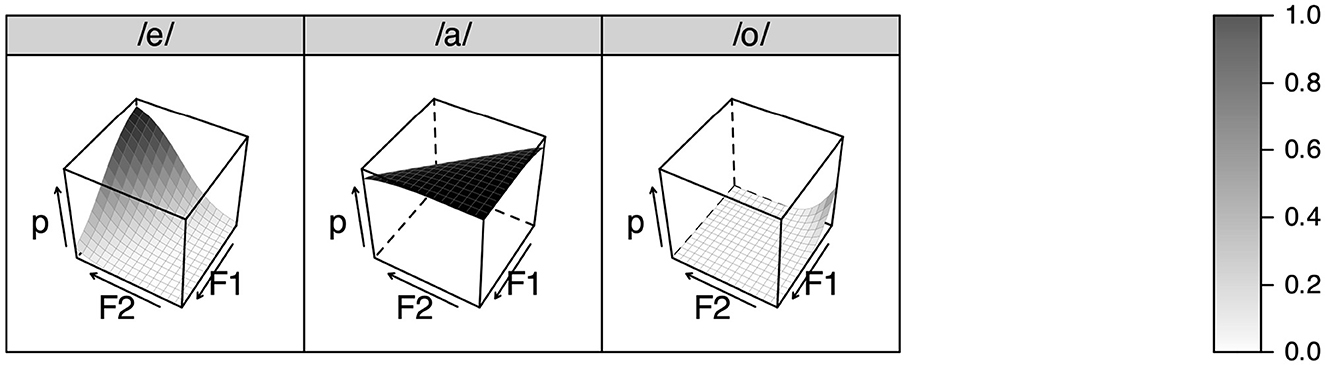
Figure 4. Simulated segmental perception after learning Japanese as L1 for 12 years, with no representation for /æ/.
3.2.3 L2 perception
The segmental learner is then exposed to L2 AmE data for the first time in life. Following L2LP's Full Copying hypothesis, the 96 cue constraints and their ranking values are copied over. Given the experimental results, the L1 vowel labels /e/, /a/, and /o/ in the copied constraints are relabeled as L2 /ɛ/, /ʌ/, and /ɑ(ɔ)/, respectively. This alone would be sufficient to explain real learners' perception of seemingly L1-assimilated vowels: /ɛ/ (= /e/) is perceived when F1 is low and and F2 is high, /ʌ/ (= /a/) when F1 is high and F2 is low, and /ɑ(ɔ)/ (= /o/) when F1 and F2 are both very low (cf. Figures 3, 4).
There is a problem, however, in that the perception of AmE /æ/ cannot be adequately modeled by mere copying. Since the grammar can only perceive three existing segmental categories, a new category for /æ/ must be manually added to the grammar. The act of adding a new category itself is not theoretically unsupported, since learners may notice a lexical distinction denoted by the vowel contrast (e.g., bass vs. bus)—perhaps due to repeated communicative errors—which motivates them to form a new phonological category. However, we encounter a puzzle here: how would the lexical distinction between bass and bus help the listeners notice the phonological contrast between /æ/ and /ʌ/ if these words sound the “same” to them? Wouldn't these words simply be represented as homophones? For example, we can see in Figure 4 that most tokens of both the bass vowel /æ/, which typically has high F1 and F2, and the bus vowel /ʌ/, which typically has low F1 and F2, are perceived as Japanese /a/. Thus, leaving open the possibility of L2 listeners manually adding a new category still begs the question of what the precise mechanism that allows the learner to do so is.
Even if we ignore this theoretical problem and add 32 new constraints (16 F1 bins + 16 F2 bins) for /æ/ (e.g., “[F1 = 1400 mel] */æ/”) to the L2 grammar, we encounter another difficulty: The simulated learning outcome does not resemble actual perceptual behavior. In fact, the model overperforms. This can be seen in Figure 5, which shows the result after learning L2 AmE for six years. Despite the decreased plasticity, the grammar has learned to correctly perceive not only /æ/ but also other vowels /ɛ/, /ʌ/, and /ɑ/, according to the acoustic distributions of the input. This is clearly different from the real learners' perception observed in the experiment, where the latter three vowels /ɛ/, /ʌ/, and /ɑ/ were perceived as Japanese /e/, /a/, and /o/, respectively. The simulated learner therefore becomes too nativelike, showing almost identical perception patterns to those of an age-matched virtual L1 AmE listener (Figure 6). This is rather unrealistic, since very few adult L2 learners, let alone those at an intermediate level, are expected to exhibit nativelike perceptual performance.
3.3 Featural simulation
3.3.1 Cue-to-feature constraints
Our featural simulation is based on Boersma and Chládková (2011), who used cue-to-feature constraints such as ‘an F1 value of x should not be mapped to the feature /high/' and ‘an F2 value of y should not be mapped to the feature /back/” to model the perception of vowels in different five-vowel systems including Japanese.8 These constraints crucially differ from cue-to-segment constraints is that, in cue-to-feature constraints, the relationships between auditory continua and featural representations are non-arbitrary. That is, the auditory continuum of F1 is tied only to height features (e.g., /high/, /mid/, and /low/), and that of F2 is tied only to backness features (e.g., /front/, /central/, and /back/), unlike cue-to-segment constraints where all auditory continua in principle can be tied to any segmental category. The features are therefore “phonetically based” (i.e., they are grounded by acoustic cues) but still “phonological” (i.e., they denote phonemic contrasts and thus are distinctive) in Boersma and Chládková (2011)'s terms, which, when used in computational modeling, seem to predict real listeners' perceptual behavior better than segment-based representations (Chládková et al., 2015a). In addition to cue constraints, our featural grammar is equipped with structural constraints (Boersma et al., 2003; Boersma, 2011) that prohibit the co-occurrence of certain features, such as “/low/ and /front/ features should not co-occur.” Structural constraints are necessary to represent the well-formedness of the perceptual output, which is relevant to the process of new L2 category formation, as we show below.
Table 5 shows how a featural Japanese grammar perceives a vowel token with [F1 = 850 mel] and [F2 = 1,400 mel] (i.e., an [æ]-like token) through two height features (/mid/ and /low/) and two backness features (/front/ and /central/). The candidates are four logical combinations of these features, two of which are well-formed in the L1 (/mid, front/ = /e/ and /low, central/ = /a/) and the other two of which are ill-formed (/mid, central/ and /low, front/). Structural constraints against ill-formed perceptual output are usually learned to be ranked very high, as is the case in the table, thus excluding the perception of /mid, central/ and /low, front/. The cue constraint “[F2 = 1,400 mel] */central/” then outranks “[F1 = 850 mel] */mid/,” making /mid, front/ the winner.
Perceptual learning in the featural grammar works in the same way as in the segmental grammar, as shown in Table 6. When the listener detects a mismatch between the intended form (“”) and the perceived form (“✓”), GLA updates the grammar by increasing the ranking values of all constraints that led to the incorrect winner (“←”) and decreasing the ranking values of the constraints that would lead to the correct form (“ → ”) by the current plasticity. Note that both cue and structural constraints are learned.
3.3.2 L1 perception
Just like the segmental learner, our featural learner starts with a “blank” perception grammar, which has 80 cue constraints (16 F1-to-height constraints for each of two height features /mid/ and /low/, and 16 F2-to-backness constraints for each of 3 backness features /front/, /central/, and /back/) as well as 6 structural constraints (two height features × three backness features), all ranked at the same initial value of 100.0.9 The learner then begins to receive L1 input, namely randomly generated tokens of Japanese /e/ (/mid, front/), /a/ (/low, central/), and /o/ (/mid, back/), as per Table 2. The correspondence between features and categories (e.g., /a/ = /low, central/) is based on Boersma and Chládková (2011). Whenever there is a mismatch between the perceived and intended feature combinations, GLA updates the ranking values of the relevant cue and structural constraints by the current plasticity.
Figure 7 shows the result of L1 learning, tested in the same way as the segmental grammar. A notable difference from the segmental result (Figure 4) is that the featural grammar can perceive a feature combination that does not occur in the L1 input, despite the high-ranked structural constraints against such ill-formed output. For example, a token with high F1 and F2, which the segmental grammar perceived as /a/ most of the time or as /e/ otherwise, can sometimes be perceived as /low, front/, which has no segmental equivalent in Japanese. What this means is that the featural grammar may prefer to perceive a structurally ill-formed form such as /low, front/ over well-formed forms such as /low, central/ if there is sufficient cue evidence to support the evaluation. This essentially expresses the perceptual deviance of [æ] that segmental modeling fails to capture: The vowel is too /front/ to be /low, central/ (= /a/).
3.3.3 L2 perception
The featural learner then begins to learn L2 AmE. Since the initial L2 grammar is a copy of the L1 grammar, it has 80 cue constraints and 6 structural constraints with the copied ranking values. Following the experimental results, and to make the featural simulation compatible with the segmental one, we assume that L2 /ɛ/ is represented as /mid, front/, /ʌ/ as /low, central/, and /ɑ(ɔ)/ as /mid, back/ in the grammar. No additional constraint is needed to model /æ/ (/low, front/).
Figure 8 shows the result of learning L2 AmE for six years. It can be seen that the feature combination /low, front/ is much more likely to be perceived than it was in Figure 7 because the ranking value of the structural constraint “*/low, front/” has decreased. The weakening of the constraint occurred because in the L2 AmE environment, the features /low/ and /front/ often co-occur, and /low, front/ should be lexically distinguished from other feature combinations for successful communication. A new category therefore emerged from existing features by improving the well-formedness of the once ill-formed feature combination, without resorting to any L2-specific manipulation of the grammar as in the segmental modeling.
Another notable finding is that the simulated perception in Figure 8 differs from the simulated L1 AmE perception in Figure 9. One salient difference lies in /ʌ/, which was learned as /low, central/ by the learner grammar, whereas it is represented as /mid, back/ in the native grammar.10 The native perception is symmetrical because it reflects the production environment of AmE vowels, whereas the learner perception is asymmetrical because L2 AmE sounds are perceived through copied L1 Japanese features. The simulated learner perception actually resembles the real learners' perception, especially in the use of the F2 cue, where /ɛ/ (/mid, front/) and /æ/ (/low, front/) are perceptually more fronted, while /ɑ/ (/mid, back/) is more back, than /ʌ/ (/low, central/).
4 General discussion
This study examined how L1 Japanese learners of L2 AmE develop a new phonological representation for /æ/ by employing experimental and computational-phonological approaches. The experimental results suggested that AmE /æ/ is represented as a separate category by intermediate-level learners, distinguished from Japanese /a/ based on the F2 cue, while adjacent AmE /ɛ/, /ʌ/, and /ɑ/ are assimilated to Japanese /e/, /a/, and /o/, respectively. To explain and replicate these results with the L2LP model, segment- and feature-based versions of perceptual simulations were performed using StOT and GLA. The segmental modeling was theoretically inadequate because it failed to elucidate the mechanism for noticing the perceptual distinctness of /æ/, and was also practically implausible because the predicted overall perception patterns were too native AmE-like compared to real learners' perception. In contrast, the featural modeling explained the emergence of a new category for AmE /æ/ and the lack thereof for /ɛ/, /ʌ/, and /ɑ/ by assuming that L2 sounds are perceived through copied L1 features, i.e., */low, front/ vs. /mid, front/, /low, central/, and /mid, back/, respectively. The simulated learning outcome closely resembled real perception.
In this section, we discuss the implications of the above findings for L2LP and the other two dominant models of L2 perception, as well as directions for extending the current study in future research.
4.1 Implications for L2 perception models
4.1.1 L2LP
Our simulations have shown that, similar to the UNFAMILIAR NEW scenario in Escudero and Boersma (2004), feature-based modeling can be useful in explaining the FAMILIAR NEW scenario. While previous L2LP studies have tended to focus on the mapping of acoustic cues to segmental categories, the current study showed that representing sound categories as an integrated bundle of features can lead to more theoretically and empirically precise predictions. A salient difference observed between the segment- and feature-based simulations was in the learning outcome, which was unrealistically nativelike in the former but fairly learner-like in the latter. This is partly due to a known weakness of segmental modeling: It tends to overpredict success because GLA does not stop learning until all segmental categories are optimally perceived (unless the input data halt or the plasticity reaches zero). The featural grammar, on the other hand, remained nonnativelike because learners continued to map acoustic cues onto copied L1 features, which are organized differently from native AmE features. In order for the featural learner grammar to achieve optimal AmE perception, the L1-like feature bundles (e.g., /ʌ/ = /low, central/) must be decomposed and reorganized to fit the L2 production environment (e.g., /ʌ/ = /mid, back/), perhaps with an addition of a new height or backness feature based on the FAMILIAR cue of F1 or F2 (because, after all, AmE has more vowels than Japanese). L2LP would predict that such learning is possible but challenging, as the NEW scenario is considered as more difficult than other types of learning scenarios (SIMILAR and SUBSET scenarios). It remains to be revealed, however, whether the reorganization and addition of features based on FAMILIAR cues is less difficult than the establishment of a novel feature based on UNFAMILIAR cues. According to Chládková et al. (2022), perceptual boundary shift in a SIMILAR scenario, which involves only FAMILIAR acoustic cues, is easier than creating new perceptual mappings of an UNFAMILIAR cue in a NEW scenario. Research on Japanese listeners' perception of English /r/-/l/ contrast have also found that they rely persistently on unreliable but FAMILIAR acoustic cues such as F2 and duration, rather than the important but UNFAMILIAR cue of F3, to identify the NEW sound representation of /r/ or, in featural terms, /rhotic/ (Iverson et al., 2003; Saito and van Poeteren, 2018; Shinohara and Iverson, 2021). It is thus predicted that the FAMILIAR NEW scenario is easier than the UNFAMILIAR NEW scenario, although more modeling and empirical testing seem necessary to verify this hypothesis (cf. Yazawa, in press).
One important point about the feature-based modeling is that the relationship between acoustic cues and phonological features is considered to be language-specific and relative. For example, while the F1 cue may be mapped to three height features (/high/, /mid/, and /low) in many languages, in some languages such as Portuguese and Italian there are four target heights (/high/, /mid-high/, /mid-low/, and /low/) and in others such as Arabic and Quechua there are only two (/high/ and /low/). Also, even if two languages share the “same” set of height features, what is perceived as /high/ in one language may not be also perceived as also /high/ in another, since the actual F1 values of high vowels varies across languages or even language varieties (Chládková and Escudero, 2012). The cue-to-feature mapping patterns are also relative within a language or language variety. This is perhaps best demonstrated by Benders et al. (2012), who showed that Spanish listeners' perceptual boundary between /i/ and /e/ (i.e., /high/ and /mid/ front vowels) was shifted by the acoustic range of the stimuli and the number of available response categories. Specifically, listeners perceived a vowel token with [F1 = 410 Hz] as /e/ when the F1 value was relatively high within the stimulus range (281–410 Hz), whereas the same token was perceived as /i/ when the F1 value was relatively low within the stimulus range (410–553 Hz). The perceptual boundary also shifted when additional response categories /a/, /o/, and /u/ were made available. These results suggest that the perception of the height feature is not determined by the absolute F1 value but rather depends largely on what other features must be considered together for the task at hand, providing useful insights into why perceptual behavior seems to vary depending on the experimental design. The above discussion has an important implication for the so-called perceptual “warping” or “magnetism” of nonnative categories to native ones (Kuhl et al., 2008), which is closely related to cross-linguistic categorical assimilation. For example, it was mentioned in Section 1 that the perceived goodness of AmE /ɛ/ as Japanese /e/ was fair despite their seemingly large spectral distance. This may be because perceived vowel height and backness are defined relatively within each language rather than between two languages. That is, Japanese listeners may perceive Japanese /e/ as /mid, front/ relative to other Japanese vowels and, in the same way, AmE /ɛ/ as /mid, front/ relative to other AmE vowels, though their judgements may depend on the task at hand. Thus, a direct comparison of raw F1 values between the two languages may not be very meaningful. The proposed language-specific feature identification is compatible with L2LP's Full Copying hypothesis, which claims that L1 and L2 speech perception are handled by separate grammars.
4.1.2 SLM
While the current study aimed to explain the process of new category formation within the framework of L2LP, the results also have useful implications for SLM. Specifically, it can be proposed that cross-linguistic categorical dissimilarity is defined as a mismatch of existing L1 features (e.g., */low, front/), with the caveat that the actual phonetic property of a feature is language-specific and relative as discussed above. This proposal is actually compatible with one of the hypotheses (H6) of the original SLM (Flege, 1995): “The phonetic category established for L2 sounds by a bilingual may differ from a monolingual's if: [...] the bilingual's representation is based on different features, or feature weights, than a monolingual's.” In fact, many of the components of our featural modeling are compatible with the original SLM, which was full of fruitful insights into the feature-based approach. For example, it was noted in Flege (1995, p. 267) that “the features used to distinguish L1 sounds can probably not be freely recombined to produce new L2 sounds,” which is essentially what our structural constraints modeled. It was also noted on the same page that “[s]ome production difficulties may arise because features used in the L2 are not used in the L1,” later formalized in the model as the “feature” hypothesis (McAllister et al., 2002), which is closely related to the UNFAMILIAR NEW scenario of L2LP. Another point on the same page was that “[t]he phenomenon of “differential substitution” shows that we need recourse to more than just a simple listing of features used in the L1 and L2 to explain certain L2 production errors,” meaning that L1-L2 segmental substitution patterns can vary even when two L1s share the “same” feature sets, which brings us back to the aforementioned caveat about feature relativity and forward to Section 4.2 where we discuss feature hierarchy and integration. The non-absolute nature of features also relates to yet another point on the next page of Flege (1995): “features may be evaluated differently as a function of position in the syllable.”
Much of this discussion, however, did not find its way into the revised SLM (Flege and Bohn, 2021), in which the “feature” hypothesis was replaced by the “full access” hypothesis. According to the new hypothesis, L2 learners can gain unrestricted access to features not used in their L1, which aligns more closely with our segment-based modeling that overpredicted success. It is also worth noting that the term “feature” is used almost interchangeably with “cue” in the revised model, although we hope to have shown through the comparison of cue-to-segment and cue-to-feature modeling that this should not be the case. Given the compatibility of our feature-based modeling with the principles of the original SLM, perhaps separating the notions of features and cues may benefit the revised model, especially to address the perrenial issue of how L1-L2 categorical similarity should be defined. To this end, incorporating different levels of abstraction as in L2LP and PAM may be in order (cf. Section 4.1.3 below).
4.1.3 PAM
The implication of feature-based modeling for PAM is similar to that for SLM: Cross-linguistic dissimilarity can be defined as featural discrepancy. However, the implication is unique for PAM because, unlike SLM and L2LP which model speech perception as the abstraction of acoustic cues into sound representations (be them segments or features), PAM subscribes to a direct realist view that listeners directly perceive articulatory gestures of the speaker. PAM also distinguishes between phonetic and phonological levels of representations (like L2LP, in a broad sense), whereas SLM defines sound categories strictly at the phonetic or allophonic level. These differences in theoretical assumption raise a crucial question about what features really are: Are they articulatory or auditory, and phonetic or phonological? As mentioned earlier, the current study assumed what Boersma and Chládková (2011) called “phonetically based phonological features,” which can be learned from perceptual input without any articulatory knowledge because perception is considered to precede production in L1 and L2 development (Escudero, 2005, 2007; Kuhl et al., 2008). The Bidirectional Phonology and Phonetics (BiPhon) framework also proposes that these auditorily learned features are used in both perception and production (Boersma, 2011), although it remains to be seen whether articulatory features are really unnecessary to account for L1 and L2 production patterns. A related topic is whether and how the features used for segmental categorization are also relevant for higher-level phonological processes, both in perception and production. The vast body of previous OT-based research provides a useful ground for testing this, because all of the components of our feature-based StOT modeling are generally compatible with the traditional OT framework.11
4.2 Future directions
Having discussed the theoretical implications of the feature-based approach, we now address how the current modeling can be practically extended to improve its adequacy in future research. First, as for the acoustic cues, we chose not to include duration because the participants in our experiment do not seem to have used it to categorize the target L2 AmE vowels, but it remains to be modeled why L1 listeners of Japanese with phonological vowel length would show such perception patterns. This can actually be a task effect, since duration-based categorization of AmE vowels into Japanese long and short ones was only observed when AmE vowels were embedded within a carrier sentence (Strange et al., 1998), i.e., when the target vowel duration could be compared to the duration of other vowels in the carrier sentence (cf. within-language feature relativity in Section 4.1.1). Thus, the modeling may need to incorporate some kind of temporal normalization to explain the potential task dependency. Escudero and Bion (2007) modeled formant normalization and speech perception as sequential processes by first applying an external algorithm (e.g., Z-normalization) to the raw acoustic data and then feeding the normalized input to the StOT grammar, which is a promising approach for temporal normalization as well. Second, as for the perceptual output, all target features were assumed to have equal status, which is perhaps overly simplistic. Flege (1995, p. 268) noted that “[c]ertain features may enjoy an advantage over others,” and Archibald (2023) recently proposed that cross-linguistic differential substitution patterns can be explained by a contrastive hierarchy of features across languages. Greenberg and Christiansen (2019) also suggested that features are processed in a stepwise manner (e.g., voicing → manner → place) rather than all at once during online speech perception. If features are hierarchically organized and processed to be ultimately integrated into higher-level representations (Boersma et al., 2003; Escudero, 2005; Yazawa, 2020), then the perception of height and backness features may also need to be evaluated sequentially rather than simultaneously, with perhaps the height feature being processed before the backness feature (Balas, 2018). This stepwise processing can be formally modeled by assigning stratum indices (van Leussen and Escudero, 2015) besides the ranking values to the StOT constraints, and ordering the constraints first by stratum and then by ranking value (or selection point, to be precise) at each evaluation. Finally, in order to fully model the observed experimental results, orthographic influences must be included. Our simulations assumed a link between AmE /ɑ/ and Japanese /o/ representations without specifying its nature, but it seems likely that this link was established at the visual rather than the auditory level in real learners. Previous L2LP studies have already explored the orthographic influences on speech perception (Escudero and Wanrooij, 2010; Escudero et al., 2014; Escudero, 2015), but how exactly orthography fits into the model is yet to be seen. Hamann and Colombo (2017) proposed modeling orthographic and perceptual borrowing of English words into Italian by using orthographic constraints such as “assign a violation mark to the grapheme <t> that is not mapped onto the phonological form /t/” along with cue constraints, which is readily applicable to StOT-based modeling of L2 audiovisual perception. An ongoing collaboration aims at achieving this goal.
We also believe that further empirical testing is needed to complement the formal computational modeling. One limitation of the current experiment, or behavioral experiments in general, is that features cannot be directly observed in participant responses. To overcome this weakness, neural studies as in Scharinger et al. (2011) or Mesgarani et al. (2014) would be helpful. Of particular interest is how the locations and magnitudes of neural responses to auditory stimuli, which have been shown to be feature-based in native perception, are mediated by L1-L2 perceptual dissimilarity and the listeners' L2 proficiency level. Such investigations, combined with formal analyses, are necessary to provide a more comprehensive understanding of the mechanism of new L2 category formation, since theoretical models need empirical support whilst empirical data need theoretical interpretation.
5 Conclusion
This study proposed that perceived (dis)similarity between L1 and L2 sounds, which is considered crucial for the process of new L2 category formation but has long remained elusive, can be better defined by assuming feature-level representations as the fundamental unit of perception, rather than segmental categories. Through our formal modeling based on L2LP and StOT, we argued that an L2 sound (e.g., AmE /æ/) whose FAMILIAR acoustic cues (e.g., F1 and F2) map to a bundle of L1 features that is structurally ill-formed (e.g., */low, front/ in Japanese) is perceived as deviant and thus is subject to category formation, whereas an L2 sound (e.g., AmE /ɛ/, /ʌ/, and /ɑ/) whose cues map to a well-formed L1 feature bundle (e.g., /mid, front/, /low, central/, and /mid, back/ in Japanese) is prone to assimilation, regardless of the ostensible acoustic distance between L1 and L2 segmental categories. The proposed feature-based modeling was consistent with our experimental results, where real L1 Japanese listeners seem to have established a distinct representation for L2 AmE /æ/ but not for /ɛ/, /ʌ/, and /ɑ/, which the segment-based modeling failed to predict and replicate. While feature-based approaches to L2 learning are still scarce compared to the vast literature on segment-based approaches, perhaps because the intangible nature of features cannot be captured without a computational platform that is currently only available to L2LP, the benefits of adopting and extending the approach are expected to go beyond the model (e.g., SLM and PAM) and beyond the current learning scenario (i.e., other sound contrasts in different language combinations), the pursuit of which should ultimately help deepen our understanding of how L2 speech acquisition proceeds as a whole.
Data availability statement
The raw data supporting the conclusions of this article will be made available by the authors, without undue reservation.
Ethics statement
The studies involving humans were approved by Academic Research Ethical Review Committee, Waseda University. The studies were conducted in accordance with the local legislation and institutional requirements. The participants provided their written informed consent to participate in this study.
Author contributions
KY: Conceptualization, Data curation, Formal analysis, Investigation, Methodology, Software, Validation, Visualization, Writing – original draft, Writing – review & editing. JW: Conceptualization, Formal analysis, Funding acquisition, Investigation, Methodology, Software, Validation, Writing – original – draft. MK: Conceptualization, Funding acquisition, Project administration, Supervision, Writing – original draft. PE: Conceptualization, Formal analysis, Funding acquisition, Project administration, Supervision, Validation, Writing – original draft.
Funding
The authors declare financial support was received for the research, authorship, and/or publication of this article. KY and MK's work was funded by JSPS Grant-in-Aid for Scientific Research (grant number: 21H00533). JW's work was funded by Samsung Electronics Co., Ltd. (grant number: A0342-20220008); the funder was not involved in the study design, collection, analysis, interpretation of data, the writing of this article, or the decision to submit it for publication. PE's work was funded by ARC Future Fellowship grant (grant number: FT160100514).
Acknowledgments
The authors thank Mikey Elmers for volunteering to provide his voice for the AmE stimuli.
Conflict of interest
The authors declare that the research was conducted in the absence of any commercial or financial relationships that could be construed as a potential conflict of interest.
Publisher's note
All claims expressed in this article are solely those of the authors and do not necessarily represent those of their affiliated organizations, or those of the publisher, the editors and the reviewers. Any product that may be evaluated in this article, or claim that may be made by its manufacturer, is not guaranteed or endorsed by the publisher.
Footnotes
1. ^Our view of features departs from the generally assumed universal set of binary phonological features. Specifically, we assume in this paper that features are language-specific and emergent rather than universal and innate (Boersma et al., 2003, 2022), that they are privative rather than binary (Chládková et al., 2015b), and that they are phonetically based (i.e., tied to acoustic cues) but still phonological (i.e., phonemically distinctive) in nature (Boersma and Chládková, 2011), all of which are also assumed in the Second Language Linguistic Perception model discussed below (cf. Boersma, 2009; Escudero, 2009). These specifications of features will be discussed in more detail in later sections.
2. ^AmE is considered as the target variety of English because it is widely used in the formal English language education in Japan and is most familiar to the learners (Sugimoto and Uchida, 2020).
3. ^Japanese has five vowel qualities /i/, /e/, /a/, /o/, and /u/, which form five short (1-mora) and long (2-mora) pairs. Long vowels are transcribed with double letters (e.g., /aa/) in this study because they can underlyingly be a sequence of two identical vowels. The transcription “/a(a)/” here indicates “either /a/ or /aa/” because the AmE vowel was perceived as Japanese /a/ when presented in isolation but as Japanese /aa/ when embedded in a carrier sentence.
4. ^Similar to AmE /æ/, AmE /ɑ/ was perceived as Japanese /a/ when presented in isolation but as Japanese /aa/ when embedded in a carrier sentence.
5. ^Participants were reminded that the pronunciation of bass was not /beɪs/ “low frequency sound” but /bæs/ “a type of fish” in a short practice before the EN session, where natural tokens of the four English words were used as tokens. The JP session also followed a practice with natural tokens of the four Japanese words as tokens. The Japanese and English tokens were produced by the same speakers as those in Section 2.2.
6. ^The distinction between lexical and semantic levels of representations goes beyond the scope of our simulations; see Boersma (2011) for a discussion.
7. ^The grammar is not truly “blank” because it already knows three segmental categories onto which the cues are mapped. Boersma et al. (2003) modeled how abstract categories can emerge from phonetic and lexical input, but we chose not to include such modeling in our simulation because our focus is not on how an L1 grammar is established but on how the L1 established grammar is copied and then restructured by L2 learning.
8. ^Constraints that map acoustic cues to privative features were first introduced by Boersma et al. (2003) and incorporated into L2LP by Escudero (2005). While it is possible to employ cue constraints with a binary feature such as [±long] (Hamann, 2009), we prefer privative features such as /long/ because, at least regarding the length feature, what is not “long” is not necessarily “short” but is rather unmarked (Chládková et al., 2015b). Note also that the choice of binary features in Hamann (2009) comes from a practical purpose to reduce the number of cue constraints, rather than a theoretically motivated choice.
9. ^This grammar is also not truly “blank” because it already knows two height and three backness features. Boersma et al. (2003, 2022) modeled how featural representations can emerge from phonetic and lexical input, which we again do not include in our simulation for the same reason as in footnote 7.
10. ^We used the same set of feature labels in both native and learner grammars to allow for a direct comparison between them, not because we assume a universal set of features across all languages (cf. Section 4.1.1). For example, we could relabel the /mid/ feature in the native grammar as /low-mid/ and still get the same result as Figure 9.
11. ^Traditional OT grammars can be seen as a special case of StOT grammars, with integer ranking values and zero evaluation noise.
References
Archibald, J. (2023). Differential substitution: a contrastive hierarchy account. Front. Lang. Sci. 2, 1–13. doi: 10.3389/flang.2023.1242905
Balas, A. (2018). Non-Native Vowel Perception: The Interplay of Categories and Features. Poznań: Wydawnictwo Naukowe UAM.
Bates, D., Mächler, M., Bolker, B. M., and Walker, S. C. (2015). Fitting linear mixed-effects models using lme4. J. Stat. Softw. 67, 1–48. doi: 10.18637/jss.v067.i01
Benders, T., Escudero, P., and Sjerps, M. J. (2012). The interrelation between acoustic context effects and available response categories in speech sound categorization. J. Acoust. Soc. Am. 131, 3079–3087. doi: 10.1121/1.3688512
Best, C. T. (1995). “A direct realist view of cross-language speech perception,” in Speech Perception and Linguistic Experience: Issues in Cross-Language Research, ed W. Strange (Timonium, MD: York Press), 171–204.
Best, C. T., and Tyler, M. (2007). “Nonnative and second-language speech perception: commonalities and complementarities,” in Language Experience in Second Language Speech Learning: In Honor of James Emil Flege, ed O. S. Bohn, and M. J. Munro (Amsterdam; Philadelphia, PA: John Benjamins), 13–34.
Boersma, P. (1998). Functional Phonology: Formalizing the Interactions between Articulatory and Perceptual Drives (Ph.D. thesis), University of Amsterdam. The Hague: Holland Academic Graphics.
Boersma, P. (2009). “Cue constraints and their interactions in phonological perception and production,” in Phonology in Perception, eds P. Boersma, and S. Hamann (Berlin: De Gruyter), 55–110.
Boersma, P. (2011). “A programme for bidirectional phonology and phonetics and their acquisition and evolution,” in Bidirectional Optimality Theory, eds A. Benz, and J. Mattausch (Amsterdam: John Benjamins), 33–72.
Boersma, P., and Chládková, K. (2011). “Asymmetries between speech perception and production reveal phonological structure,” in Proceedings of the 17th International Congress of Phonetic Sciences, eds W.-S. Lee, and E. Zee (Hong Kong: The University of Hong Kong), 328–331.
Boersma, P., Chládková, K., and Benders, T. (2022). Phonological features emerge substance-freely from the phonetics and the morphology. Can. J. Linguist. 67, 611–669. doi: 10.1017/cnj.2022.39
Boersma, P., and Escudero, P. (2008). “Learning to perceive a smaller L2 vowel inventory: an Optimality Theory account,” in Contrast in Phonology: Theory, Perception, Acquisition, eds P. Avery, E. Dresher, and K. Rice (Berlin: de Gruyter), 271–302.
Boersma, P., Escudero, P., and Hayes, R. (2003). “Learning abstract phonological from auditory phonetic categories: an integrated model for the acquisition of language-specific sound categories,” in Proceedings of the 15th International Congress of Phonetic Sciences, eds M. J. Solé, D. Recasens, and J. Romero (Barcelona), 1013–1016.
Boersma, P., and Hayes, B. (2001). Empirical tests of the gradual learning algorithm. Linguist. Inq. 32, 45–86. doi: 10.1162/002438901554586
Boersma, P., and Weenink, D. (2023). Praat: Doing Phonetics by Computer. Amsterdam: University of Amsterdam.
Chen, J., and Chang, H. (2022). Sketching the landscape of speech perception research (2000–2020): a bibliometric study. Front. Psychol. 13, 1–14. doi: 10.3389/fpsyg.2022.822241
Chládková, K., Boersma, P., and Benders, T. (2015a). “The perceptual basis of the feature vowel height,” in Proceedings of the 18th International Congress of Phonetic Sciences, ed The Scottish Consortium for ICPhS 2015 (Glasgow: The University of Glasgow), 711.
Chládková, K., Boersma, P., and Escudero, P. (2022). Unattended distributional training can shift phoneme boundaries. Bilingual. Lang. Cognit. 25, 827–840. doi: 10.1017/S1366728922000086
Chládková, K., and Escudero, P. (2012). Comparing vowel perception and production in Spanish and Portuguese: European versus Latin American dialects. J. Acoust. Soc. Am. 131, EL119–EL125. doi: 10.1121/1.3674991
Chládková, K., Escudero, P., and Lipski, S. C. (2015b). When “AA” is long but “A” is not short: speakers who distinguish short and long vowels in production do not necessarily encode a short long contrast in their phonological lexicon. Front. Psychol. 6, 1–8. doi: 10.3389/fpsyg.2015.00438
Escudero, P. (2005). Linguistic Perception and Second Language Acquisition: Explaining the Attainment of Optimal Phonological Categorization (PhD thesis). Utercht University. Amsterdam: LOT.
Escudero, P. (2007). “Second-language phonology: the role of perception,” in Phonology in Context, ed M. C. Pennington (London: Palgrave Macmillan), 109–134.
Escudero, P. (2009). “The linguistic perception of SIMILAR L2 sounds,” in Phonology in Perception, eds P. Boersma, and S. Hamann (Berlin: de Gruyter), 151–190.
Escudero, P. (2015). Orthography plays a limited role when learning the phonological forms of new words: the case of Spanish and English learners of novel Dutch words. Appl. Psycholinguist. 36, 7–22. doi: 10.1017/S014271641400040X
Escudero, P., and Bion, R. (2007). “Modeling vowel normalization and sound perception as sequential processes,” in Proceedings of the 16th International Congress of Phonetic Sciences, eds J. Trouvain, and W. J. Barry (Saarbrücken: Saarland University), 1413–1416.
Escudero, P., and Boersma, P. (2004). Bridging the gap between L2 speech perception research and phonological theory. Stud. Sec. Lang. Acquisit. 26, 551–585. doi: 10.1017/S0272263104040021
Escudero, P., Kastelein, J., Weiand, K., and van Son, R. J. J. H. (2007). “Formal modelling of L1 and L2 perceptual learning: computational linguistics versus machine learning,” in Proceedings of the 8th Annual Conference of the International Speech Communication Association (Antwerp: International Speech Communication Association), 1889–1892.
Escudero, P., Simon, E., and Mulak, K. E. (2014). Learning words in a new language: orthography doesn't always help. Biling. Lang. Cogn. 17, 384–395. doi: 10.1017/S1366728913000436
Escudero, P., and Wanrooij, K. (2010). The effect of L1 orthography on non-native vowel perception. Lang. Speech 53, 343–365. doi: 10.1177/0023830910371447
Escudero P. Yazawa K. (in press). “The second language linguistic perception model (L2LP),” in The Cambridge Handbook of Bilingual Phonetics and Phonology, ed M. Amengual (Cambridge: Cambridge University Press).
Flege, J. E. (1995). “Second language speech learning: theory, findings, and problems,” in Speech Perception and Linguistic Experience: Issues in Cross-Language Research, ed W. Strange (Timonium, MD: York Press), 233–277.
Flege, J. E., and Bohn, O.-S. (2021). “The revised speech learning model (SLM-r),” in Second Language Speech Learning: Theoretical and Empirical Progress, ed R. Wayland (Cambridge: Cambridge University Press), 3–83.
Greenberg, S., and Christiansen, T. U. (2019). The perceptual flow of phonetic information. Attent. Percept. Psychophys. 81, 884–896. doi: 10.3758/s13414-019-01666-y
Hamann, S. (2009). “Variation in the perception of an L2 contrast: a combined phonetic and phonological account,” in Variation and Gradience in Phonetics and Phonology, eds F. Kügler, C. Féry, and R. van de Vijver (Berlin: De Gruyter), 71–98.
Hamann, S., and Colombo, I. E. (2017). A formal account of the interaction of orthography and perception. Nat. Lang. Linguist. Theory 35, 683–714. doi: 10.1007/s11049-017-9362-3
Hirata, Y. (2004). Training native English speakers to perceive Japanese length contrasts in word versus sentence contexts. J. Acoust. Soc. Am. 116, 2384–2394. doi: 10.1121/1.1783351
Hirata, Y. (2017). “Second language learners' production of geminate consonants in Japanese,” in The Phonetics and Phonology of Geminate Consonants, ed H. Kubozono (Oxford: Oxford University Press), 163–184.
Hisagi, M., Higby, E., Zandona, M., Kent, J., Castillo, D., Davidovich, I., et al. (2021). “Perceptual discrimination measure of non-native phoneme perception in early and late Spanish-English & Japanese-English bilinguals,” in Proceedings of Meetings on Acoustics, Vol. 42 (The Acoustical Society of America), 1–13.
Iverson, P., Kuhl, P., Akahane-Yamada, R., Diesch, E., Tohkura, Y., Kettermann, A., et al. (2003). A perceptual interference account of acquisition difficulties for non-native phonemes. Cognition 87, B47–B57. doi: 10.1016/S0010-0277(02)00198-1
Kawahara, H. (2006). STRAIGHT, exploitation of the other aspect of VOCODER: Perceptually isomorphic decomposition of speech sounds. Acoust. Sci. Technol. 27, 349–353. doi: 10.1250/ast.27.349
Kuhl, P. K., Conboy, B. T., Coffey-Corina, S., Padden, D., Rivera-Gaxiola, M., and Nelson, T. (2008). Phonetic learning as a pathway to language: new data and native language magnet theory expanded (NLM-e). Philos. Transact. R. Soc. B Biol. Sci. 363, 979–1000. doi: 10.1098/rstb.2007.2154
Kuznetsova, A., Brockhoff, P. B., and Christensen, R. H. B. (2017). lmerTest package: tests in linear mixed effects models. J. Stat. Softw. 82, 1–26. doi: 10.18637/jss.v082.i13
Labov, W., Ash, S., and Boberg, C. (2006). The Atlas of North American English: Phonetics, Phonology and Sound Change. Berlin: De Gruyter.
Lambacher, S. G., Martens, W. L., Kakehi, K., Marasinghe, C. A., and Molholt, G. (2005). The effects of identification training on the identification and production of American English vowels by native speakers of Japanese. Appl. Psycholinguist. 26, 227–249. doi: 10.1017/S0142716405050150
McAllister, R., Flege, J. E., and Piske, T. (2002). The influence of L1 on the acquisition of Swedish quantity by native speakers of Spanish, English and Estonian. J. Phon. 30, 229–258. doi: 10.1006/jpho.2002.0174
Mesgarani, N., Cheung, C., Johnson, K., and Chang, E. F. (2014). Phonetic feature encoding in human superior temporal gyrus. Science 343, 1006–1010. doi: 10.1126/science.1245994
Morrison, G. S. (2007). “Logistic regression modelling for first-and second-language perception data,” in Segmental and Prosodic Issues in Romance Phonology, eds M. J. Solé, P. Prieto, J. Mascaró, and M. J. Sol? (Amsterdam: John Benjamins), 219–236.
Nishi, K., Strange, W., Akahane-Yamada, R., Kubo, R., and Trent-Brown, S. A. (2008). Acoustic and perceptual similarity of Japanese and American English vowels. J. Acoust. Soc. Am. 124, 576–588. doi: 10.1121/1.2931949
Pajak, B., and Levy, R. (2014). The role of abstraction in non-native speech perception. J. Phon. 46, 147–160. doi: 10.1016/j.wocn.2014.07.001
Prince, A., and Smolensky, P. (1993). Optimality Theory: Constraint Interaction in Generative Grammar. Rutgers University Center for Cognitive Science Technical Report 2. New Jersey.
R Core Team (2023). R: A Language and Environment for Statistical Computing. R Foundation for Statistical Computing. Vienna.
Saito, K., and van Poeteren, K. (2018). The perception-production link revisited: the case of Japanese learners' English /ɹ/ performance. Int. J. Appl. Linguist. 28, 3–17. doi: 10.1111/ijal.12175
Scharinger, M., Idsardi, W. J., and Poe, S. (2011). A comprehensive three-dimensional cortical map of vowel space. J. Cogn. Neurosci. 23, 3972–3982. doi: 10.1162/jocn_a_00056
Schwartz, B. D., and Sprouse, R. A. (1996). L2 cognitive states and the full transfer/full access model. Sec. Lang. Res. 12, 40–72. doi: 10.1177/026765839601200103
Shafer, V. L., Kresh, S., Ito, K., Hisagi, M., Vidal, N., Higby, E., et al. (2021). The neural timecourse of American English vowel discrimination by Japanese, Russian and Spanish second-language learners of English. Biling. Lang. Cogn. 24, 642–655. doi: 10.1017/S1366728921000201
Shaw, J. A., and Kawahara, S. (2017). The lingual articulation of devoiced /u/ in Tokyo Japanese. J. Phon. 66, 100–119. doi: 10.1016/j.wocn.2017.09.007
Shinohara, Y., Han, C., and Hestvik, A. (2019). “Effects of perceptual assimilation: the perception of English /æ/, /ʌ/, and /ɑ/ by Japanese speakers,” in Proceedings of the 19th International Congress of Phonetic Sciences, eds S. Calhoun, P. Escudero, M. Tabain, and P. Warren (Melbourne, VIC. Australasian Speech Science and Technology Association Inc.), 2344–2348.
Shinohara, Y., Han, C., and Hestvik, A. (2022). Discriminability and prototypicality of nonnative vowels. Stud. Second Lang. Acquis. 44, 1260–1278. doi: 10.1017/S0272263121000978
Shinohara, Y., and Iverson, P. (2021). The effect of age on English /r/-/l/ perceptual training outcomes for Japanese speakers. J. Phon. 89, 1–24. doi: 10.1016/j.wocn.2021.101108
Strange, W., Akahane-Yamada, R., Kubo, R., Trent-Brown, S. A., Nishi, K., and Jenkins, J. J. (1998). Perceptual assimilation of American English vowels by Japanese listeners. J. Phon. 26, 311–344. doi: 10.1006/jpho.1998.0078
Sugimoto, J., and Uchida, Y. (2020). English phonetics and teacher training: designing a phonetics course for Japanese preservice teachers. J. Phonet. Soc. Jpn 24, 22–35. doi: 10.24467/onseikenkyu.24.0_22
Tsukada, K. (2012). Comparison of native versus nonnative perception of vowel length contrasts in Arabic and Japanese. Appl. Psycholinguist. 33, 501–516. doi: 10.1017/S0142716411000452
Tsukada, K., Cox, F., Hajek, J., and Hirata, Y. (2018). Non-native Japanese learners' perception of consonant length in Japanese and Italian. Sec. Lang. Res. 34, 179–200. doi: 10.1177/0267658317719494
van Leussen, J.-W., and Escudero, P. (2015). Learning to perceive and recognize a second language: The L2LP model revised. Front. Psychol. 6, 1–12. doi: 10.3389/fpsyg.2015.01000
Whang, J., and Yazawa, K. (2023). Modeling a phonotactic approach to segment recovery: the case of Japanese high vowels. Stud. Phonet. Phonol. Morphol. 29, 271–295. doi: 10.17959/sppm.2023.29.2.271
Yazawa, K. (2020). Testing Second Language Linguistic Perception : A Case Study of Japanese, American English, and Australian English Vowels (Ph.D. thesis). Waseda University, Tokyo.
Yazawa K. (in press). NEW sounds can be easier to learn than SIMILAR sounds, but only when acoustic cues are FAMILIAR. Tsukuba Eng. Stud. 42.
Yazawa, K., Whang, J., and Escudero, P. (2023). Australian English listeners' perception of Japanese vowel length reveals underlying phonological knowledge. Front. Psychol. 14, 1–14. doi: 10.3389/fpsyg.2023.1122471
Keywords: Second Language Linguistic Perception (L2LP) model, Stochastic Optimality Theory, Gradual Learning Algorithm, category formation, features, computational modeling, Japanese, American English
Citation: Yazawa K, Whang J, Kondo M and Escudero P (2023) Feature-driven new sound category formation: computational implementation with the L2LP model and beyond. Front. Lang. Sci. 2:1303511. doi: 10.3389/flang.2023.1303511
Received: 28 September 2023; Accepted: 22 November 2023;
Published: 20 December 2023.
Edited by:
Baris Kabak, Julius Maximilian University of Würzburg, GermanyReviewed by:
Mathias Scharinger, University of Marburg, GermanySilke Hamann, University of Amsterdam, Netherlands
Copyright © 2023 Yazawa, Whang, Kondo and Escudero. This is an open-access article distributed under the terms of the Creative Commons Attribution License (CC BY). The use, distribution or reproduction in other forums is permitted, provided the original author(s) and the copyright owner(s) are credited and that the original publication in this journal is cited, in accordance with accepted academic practice. No use, distribution or reproduction is permitted which does not comply with these terms.
*Correspondence: Kakeru Yazawa, eWF6YXdhLmtha2VydS5nYiYjeDAwMDQwO3UudHN1a3ViYS5hYy5qcA==; James Whang, amFtZXN3JiN4MDAwNDA7c251LmFjLmty