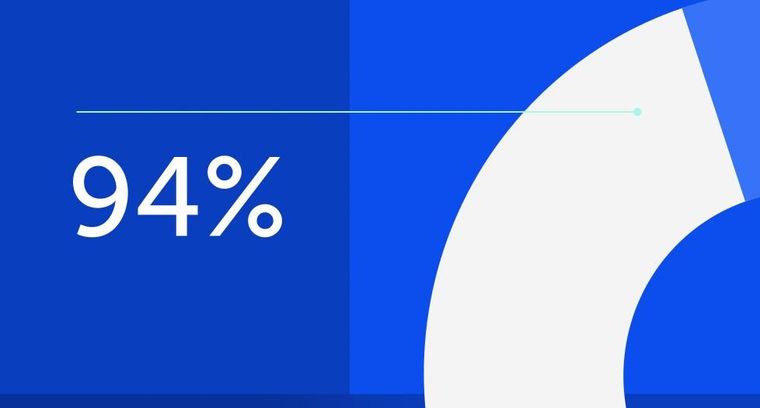
94% of researchers rate our articles as excellent or good
Learn more about the work of our research integrity team to safeguard the quality of each article we publish.
Find out more
ORIGINAL RESEARCH article
Front. Lang. Sci., 22 June 2023
Sec. Language Processing
Volume 2 - 2023 | https://doi.org/10.3389/flang.2023.1139073
This article is part of the Research TopicInsights in Language Processing: 2022View all articles
Predictive coding is considered to be an important mechanism for perceptual learning. Posterior prediction-error minimization can lead to higher rates of lasting changes in the representational hierarchy, and hence is likely to enhance the process of learning. In the field of speech processing, although considerable studies have demonstrated that a highly predictive sentence context can facilitate the perception of forthcoming word, it remains to be examined that how this type of predictability affects the perceptual learning of speech (especially degraded speech). The present study, therefore, aimed to examine whether and how the lexical predictability of spoken sentences modulates perceptual learning of speech embedded in noise, by using spoken sentences as training stimuli and strictly controlling the semantic-context constraint of these training sentences. The current study adopted a “pretest-training-posttest” procedure. Two groups of subjects participated in this perceptual learning study, with cognitive and language abilities matched across these two groups. For one group, the spoken sentences used for training all have a highly predictive semantic context; for another group, the training sentences all have a low predictive context. The results showed that both the reaction time and accuracy of the speech-in-noise intelligibility test were significantly improved in the post-training phase compared to the pre-training phase; moreover, the learning-related improvement was significantly enhanced in participants with weak-constraint sentences as training stimuli (compared to those with strong-constraint sentences as training stimuli). This enhancement effect of low lexical predictability on learning-related improvement supports a prediction-error based account of perceptual learning.
The human brain has been considered to be a prediction machine. Prediction is an important mechanism for both efficient information processing and perceptual learning (Friston, 2005; Sohoglu and Davis, 2016). Take the processing of degraded speech as an example, the word “beer” is easier to be recognized when it is embedded in a highly predictive sentence context (“he drinks the beer”) than when in a low predictive context (“he sees the beer”) (e.g., Obleser and Kotz, 2010). This immediate context not only facilitates speech perception in the moment but also can modulate perceptual learning, and therefore the listener will have improved ability to perceive the degraded speech in the future when there is no such helpful contextual information (e.g., Friston, 2005; Sohoglu and Davis, 2016). Although both the immediate context benefit and the perceptual learning effect are experience-dependent perceptual improvement, they are distinct from each other in the time courses of their improvement effects. Specifically, the facilitating effect of the immediate context occurs usually over a timescale of seconds or less, whereas perceptual learning takes place over a time-scale of minutes or longer, involving relatively long-lasting and gradual improvements in the listeners' perception abilities (e.g., Sohoglu and Davis, 2016; Bieber and Gordon-Salant, 2021). Perceptual learning can further be divided into short-term learning (online learning) and long-term learning, with the former usually involving changes within a single test session or across test sessions within one day and the latter typically referring to changes across test sessions separated by at least a day (see Bieber and Gordon-Salant, 2021 for review). This study mainly focused on the short-term perceptual learning of speech.
In the field of psycholinguistics, it has been well documented that the human brain can use a sentence's strongly constraining semantic context to predict upcoming words (e.g., “shells” in “at the seaside she picked up a lot of …”) (e.g., Dikker and Pylkkänen, 2013; Bonhage et al., 2015). This top-down lexical prediction can provide constraints to the representation of new bottom-up speech input, thereby improving speech perception (Obleser and Kotz, 2010; Grisoni et al., 2017, 2020; Li et al., 2019; Zheng et al., 2021). This facilitating effect of top-down prediction mainly profits from the current situational/semantic context in which the current perception process takes place and from the already learned knowledge retrieved from long-term memory (i.e., world knowledge and lexical knowledge). However, it remains unclear how the current context and top-down lexical predictability affects the online perceptual learning of degraded speech (i.e., speech embedded in noise). The answer to this question will provide insight into the development of learning/rehabilitation programs to optimize speech perception in suboptimal listening conditions. The present study, therefore, is mainly interested in the way by which the lexical predictability of current spoken sentences affects the online perceptual learning of degraded speech.
The predictive coding account (Rao and Ballard, 1999; Friston, 2005, 2010; Kuperberg and Jaeger, 2016) has described one important processing mechanism associated with human perception and perceptual learning (see Figure 1). According to this account, the properties of perceptual processing and learning can be explained by a generative cortical/representational hierarchy framework. On the one hand, during perception, before the actual presence of the sensory input in context, each level of the cortical/representation hierarchy (except the lowest level) is engaged in predicting the responses at the next lower level via backward connections (from higher to lower levels) and consequently provides contextual guidance to lower levels. When this top-down prediction is in accord with the actually-perceived bottom-up input, prediction error (difference between the input actually-perceived and that previously predicted) is minimized and our perception is facilitated. Besides facilitating the lower-level sensory processing of the immediate input, these backward connections coming from prior top-down prediction, following successive exposures to the same stimuli, can lead to perceptual learning through learning the connection parameters (e.g., Friston, 2005, 2010). On the other hand, if the top-down prediction is incomplete or incompatible with the actually-perceived low-level input, the prediction error (only the prediction error) will propagate through the remainder of the processing hierarchy via forward connections (from lower to higher levels), and meanwhile backward (or and lateral) connection adjustments will be initiated until this prediction error is minimized. This adjustment of the connection parameters induced by this posterior prediction-error minimization process, following repeated exposure to the same stimulus, can lead to perceptual learning too (Friston, 2005, 2010). In short, both the backward connections coming from “prior top-down prediction” and the backward (or and lateral) connection adjustments induced by “posterior prediction-error minimization” can lead to perceptual learning after the successive exposure to training stimuli. These two sources of learning are tightly related, with one source tending to be predominant over the other as a function of the specific processing situations. This predictive coding framework has been used to account for the processing and perceptual learning of language (Kuperberg and Jaeger, 2016), i.e., speech perceptual processing and learning (Sohoglu and Davis, 2016), as language processing also involves multiple levels of representations (e.g., surface sensory, phonetic/phonological, lexical/semantic, and sentence-meaning levels).
Figure 1. Predictive processing and perceptual learning in the hierarchical predictive coding architecture, which is reproduced from Friston (2005) with permission from the publisher. The number i (i.e., 1, 2, 3, 4…) indicates the specific level in the hierarchical predictive coding architecture; the larger the number, the higher the level is. The symbol φi denotes the representation unit at a specific level i, and ξi denotes the prediction error unit at that level. The higher level of representation unit (i.e., φi+1) and the corresponding backward connections (from higher to lower levels) enable the generation of prior predictions at the next lower level (i.e., level i). Prediction error reflects the difference between the predicted activity (i.e., conveyed by backward connections from level i+1 to level i) and the observed activity (i.e., the activity of the representational unit within the same level i). Only prediction error propagates through the remainder of the processing hierarchy via forward connections (from lower to higher levels) (Friston, 2005). Perceptual learning refers to “long-lasting changes to the perceptual system that improve its ability to respond to its environment and are caused by this environment” (Goldstone, 1998), which at least in part corresponds to identifying the maximum likelihood value of the connection parameters in the hierarchical predictive coding architecture (Friston, 2005).
In the past decades, there have been a considerable number of experimental evidences for perceptual learning of speech. These studies showed that listeners can use the disambiguating information that has just been presented to resolve the identity of ambiguous or degraded speech sounds and adjust perceptual boundaries in the subsequent processing of speech (Ganong, 1980; Connine et al., 1993; Newman et al., 1997; Borsky et al., 1998; Myers and Mesite, 2014). The ability of speech perception can be improved through perceptual learning among people of different ages (Peelle and Wingfield, 2005) and with different levels of hearing loss (Karawani et al., 2016).
Some studies further demonstrated that the perceptual learning of poorly recognizable speech sounds can be enhanced by high-level knowledge, such as lexical or semantic information, present in the immediate training stimuli (e.g., Norris et al., 2003; Davis et al., 2005; Eisner and McQueen, 2005; Kraljic and Samuel, 2005; Sweetow and Sabes, 2006; Miller et al., 2015; Cooper and Bradlow, 2016). For example, Norris et al. (2003) demonstrated how perceptual learning can be guided by the lexical constraint of the word used for training. In this study, listeners initially heard an ambiguous speech sound falling between/f/and/s/in the context of a single word that biased its interpretation toward either/f/or/s/. Following exposure to 20 constraining words, listeners had begun to interpret the ambiguous phoneme in a manner consistent with their previous exposure (Norris et al., 2003), which suggests that listeners had used the lexical information present in the training stimuli to guide their perceptual learning process. Subsequently, Davis et al. (2005) conducted a series of studies that explored how different types of training sentences affect perceptual learning of degraded speech. They set four types of sentences as training material: (a) Normal prose, which is normal sentences; (b) Syntactic prose, sentences in which the content words are randomly placed; (c) Jabberwocky, sentences containing real English function words but in which content words are replaced with non-words; (d) Non-word sentences. By comparison, they found that the groups trained with Normal prose and Syntactic prose identified the degraded sentences better than those trained with other non-word sentences and Jabberwocky (Davis et al., 2005), suggesting that listeners were using the lexical/semantic knowledge rather than just the low-level acoustics for perceptual learning. In sum, the studies mentioned above demonstrated that higher-level lexical/semantic representations derived from the immediate contextual information can be used to inform and guide the perceptual learning of lower-level speech features, which is in line with the assumption of the predictive coding account (Friston, 2005, 2010; Kuperberg and Jaeger, 2016).
Recently, an EEG (Electroencephalogram) study conducted by Sohoglu and Davis (2016) provided direct evidence for the predictive coding account of speech perceptual learning. This study, using magnetoencephalographic (MEG) and EEG recordings of neural responses evoked by degraded speech, showed that the recognition of spoken word was enhanced both by prior knowledge obtained from matching text and by relatively a period (around half an hour) of perceptual learning of degraded speech; meanwhile, both types of speech perception improvement are all associated with neural activity reduction in a peri-auditory region of the superior temporal gyrus (STG) (Sohoglu and Davis, 2016). This magnetoencephalographic-EEG study suggests that predictive processing is a common mechanism for the immediate perceptual effects of prior knowledge and longer-term perceptual learning of degraded speech.
Although the predictive coding account of speech perceptual learning has gotten support from the existing studies, the nature of this learning process remains to be explored for more detail. One important question is what type of training stimuli might be able to lead to better use of the prediction process to obtain a larger learning effect remains unknown. Specifically, whether and how the lexical predictability of training sentences (namely, sentences with different degrees of semantic constraint) affects the efficiency of perceptual learning is worthy of being examined, given the close relationship between speech perception learning and predictive speech processing (e.g., Friston, 2005; Sohoglu and Davis, 2016).
As for the relationship between the lexical predictability of spoken sentences used for training and their perceptual learning effect, there might be two possibilities. One possibility is that the highly predictive sentences (compared to the low predictive ones), if they are used as training stimuli, might be able to bolster the perception learning of degraded speech, just as the high-level lexical or semantic information facilitated speech perceptual learning observed in the existing studies (Norris et al., 2003; Davis et al., 2005). This reason is that, according to the “prior top-down prediction” source of perceptual learning assumed by the predictive coding account (Friston, 2005, 2010; Kuperberg and Jaeger, 2016), the strong-constraint semantic context of highly predictive sentences can be used to generate top-down prediction (e.g., lexical and even further phonetic/phonological prediction) of upcoming words of the sentence; this top-down prediction can be used to guide and optimize the backward connection parameters among the hierarchy representations, hence leading to an enhanced learning effect. The experimental studies have provided evidence for this top-down predictive processing and the facilitating effect of strong semantic constraint on speech perception (e.g., Obleser and Kotz, 2010; Grisoni et al., 2017; Wang et al., 2018; Li et al., 2019). Additionally, the neuroimaging studies also provided neural evidences for a “sharpened representation” view of predictive coding, which assumes that neural representations of low-level sensory signals are directly enhanced or “sharpened” by high-level knowledge/representation; that is, predictions passed down from the high-levels selectively enhance the representation of expected sensory signals by inhibiting inputs that are inconsistent with the predictions more strongly than consistent input (Murray et al., 2004; Blank and Davis, 2016; de Lange et al., 2018). According to this “sharpened representation,” low-level sensory neural representations of degraded speech sounds (and correspondingly backward connections) can be directly enhanced by training sentences with a highly predictive context. That is, the “prior top-down prediction” source of learning assumed by the predictive coding account, combined with the behavioral effect of lexical/semantic constraint benefit and the neural-level “sharpened representation” scheme, suggest that compared to the low predictive sentences, the highly predictive sentences for training stimuli are likely to lead to enhanced perceptual learning of the degraded speech.
An alternative possibility is that spoken sentences with a low predictive context for training stimuli, but not those with a highly predictive context, are expected to enhance perceptual learning of degraded speech, due to the following reasons. Firstly, the predictive coding account assumes that besides the backward connections coming from “prior top-down prediction,” the backward (or and lateral) connection adjustments induced by “posterior prediction-error minimization” can affect perceptual learning. Specifically, training spoken sentences with a low predictive context (compared to those with a highly predictive context) will lead to increased prediction-error activities, given that the bottom-up words embedded in a low predictive context are less expected; these prediction errors will further pass up to higher levels of representation and also need time to be suppressed via backward and lateral connections, which provides more time or opportunity to adjust the connection parameters of the representational hierarchy, thereby bolstering perceptual learning. For example, a syntactic structure learning study supported the error-based learning theory by showing that for children and adults together, more surprising (hence less predictable) training sentences led to enhanced syntactic-structure learning when compared with predictable training sentences (Fazekas et al., 2020). Additionally, the neuroimaging studies also proved that the sensory representation of bottom-up input is not merely directly sharpened by prior predictions; instead, there is an intermediate process that encodes posterior probabilities of sensory input by considering the higher-level neural representations, with the expected parts being suppressed and only the unexpected parts (i.e., prediction error) being passed up the cortical hierarchy (e.g., in Sohoglu and Davis, 2020), which is in line with the “posterior prediction-error minimization” assumption of the predictive coding account.
Although some studies have explored the modulating effect of training material on the perceptual learning of degraded speech, these studies mainly found that the presence (vs. absence) of lexical or sub-lexical identity (e.g., Norris et al., 2003) and the presence of sentence-level information (vs. unnormal sentences) (Davis et al., 2005) lead to the enhanced training effect. The existing studies provided important evidence for the facilitating effect of high-level information (e.g., lexical or semantic information) on speech perceptual learning. However, what type of sentence (e.g., sentences with a strong-constraint or weak-constraint semantic context) might have a larger impact on the perceptual learning of degraded speech remains to be investigated.
The present study aimed to explore how the lexical predictability of spoken sentences used for training affects the effectiveness of perceptual learning of degraded speech, which would help to examine the nature of the predictive-coding account of perceptual learning in more detail.
To examine the experimental question, the present study adopted a “pre-test-training-post-test” procedure and manipulated the lexical predictability of the spoken sentences used as training stimuli. The training sentences have either a high or low level of lexical predictability, which was realized by manipulating the semantic constraint of the sentence context. Participants were divided into two groups, with cognitive and language abilities matched across these two groups: one group participated in the high-predictability training group (the training spoken sentences all having a highly predictive semantic context) and another group participated in the low-predictability training group (the training spoken sentences all having a low predictive semantic context). Both groups followed the “pre-test-training-post-test” procedure. Both the spoken sentences used in the pre-test and post-test phases and those in the training phase were embedded in noise. During the pre-test and post-test phases, participants were asked to perform a speech-in-noise intelligibility test. During the training phase, they were asked to listen to each spoken sentence three times (in an order of unclear speech-in-noise, clear speech, and unclear speech-in-noise) and judge the clarity of each presentation of the sentence; that is, participants were asked to report their subject experience of speech clarity (namely, the degree to which the spoken sentence can be recognized) on a 10-poinst scale (from 1 to 10; the larger the number, the higher the degree of clarity).
According to the “prior top-down prediction” source of perceptual learning, the training effect (speech intelligibility score: post-test-minus-pre-test) was expected to be larger in the group with low predictive sentences as training materials (compared to the group with highly predictive sentences as training materials). In contrast, according to the “posterior prediction-error minimization” source of learning, a reversed pattern of results should be observed.
This research was approved by the Ethics Committee of Institute of Psychology, Chinese Academy of Sciences. A total of 64 students participated in this experiment, which were divided into two groups: 32 participants (10 males and 22 females) joined the training with strong-constraint sentences as stimuli, and the other 32 (9 males and 23 females) participants joined that with weak-constraint sentences as stimuli.
The sample size was determined, on the one hand, based on other related studies investigating the modulating effect of lexical or semantic information on the perceptual learning of degraded speech [around twenty participants for each group that received a specific type of learning in Davis et al. (2005); twenty-one participants for the within-subject design experiment in Sohoglu and Davis (2016)], with relatively more participants recruited in the present study. In addition, according to G*power's calculations, for the modulating effect of training group (strong-constraint vs. weak-constraint) on the learning effect of the current study, at least 56 participants (28 in each group) were needed to achieve a statistical power of 0.95 based on a moderate effect size (f = 0.25) and a moderate correlation (r = 0.50) of the repeated measures.
All participants were students from universities and research institutes around the Institute of Psychology, Chinese Academy of Sciences. All participants are 18–30 years old, live and grow up in a Mandarin-speaking environment in the northern region of China, and have normal hearing. All subjects were informed of the experimental procedure and the safety of their participation before the experiment began and were paid a certain amount of money at the end of the experiment.
In order to make sure that the two groups of participants are matched on their general cognitive ability and vocabulary background, we conducted a series of experiments online. The character-based 0-back and 2-back tasks and the vocabulary test were conducted to test their working memory and long-term word knowledge. First, the 0-back and 2-back tasks are achieved by Psychopy online platform. A sequence of English letters was presented visually on the center of the screen one by one. For the 0-back task, participants were asked to judge if the current letter was the same as the two letters that were specified in advance; in the 2-back task, participants were required to judge if the current letter was the same as the one presented 2 trials ago; participants' response time and accuracy were recorded. Second, vocabulary knowledge was measured with the vocabulary subtest from the Wechsler Adult Intelligence Scale (WAIS-IV, Wechsler, 2008), during which each participant was asked to explain the lexical meaning of 40 Chinese words; the interpretation accuracy of each item was scored within the range of 0–2 according to its proximity to the standard answers; the average scores of 40 items were taken to indicate individual vocabulary knowledge (maximum = 80).
Results of independent samples t-tests showed that there is no significant difference between the two groups over both the 0-back task (response time: t (62) = 1.35, p = 0.18; accuracy: t (62) = −1.37, p = 0.17), 2-back task (response time: t (62) = 0.68, p = 0.49; accuracy: t (62) = 0.11, p = 0.91), and 2-back minus 0-back (response time: t (62) =0.17, p = 0.86; accuracy: t (62) =0.72, p = 0.47). T-test conducted over vocabulary knowledge also did not find a significant difference between the two groups (t (62) = −0.73, p = 0.47). The results of the tests above demonstrated that the two groups of participants are matched on general cognitive ability and vocabulary knowledge (see Table 1).
The present study adopted a “pre-testing_training_post-testing” procedure (post-testing_vs._pre-testing indicating learning effect) that finished within one day, with a break of 5–10 min between different phases to avoid fatigue. Meanwhile, two groups of participants were recruited, with one group being trained with strong-constraint sentences and another group with weak-constraint sentences. In addition, in order to know if the magnitude of perceptual learning is equivalent across different forms of speech signal quality, two levels of speech SNR (Signal-to-Noise Ratio) were constructed for the stimuli used in pre-testing and post-testing phrases. The spoken-sentence stimuli used in the training phase were different from those in the pre/post-testing phase in both written words and speech SNR, which helps to obtain a clearer training effect and improve the generalization of this training effect to the testing environment with different speech content or noise background.
During the pre-testing and post-testing phases, six groups of Chinese matrix sentence lists (CMS) were constructed by the research group of Xiaoqing Li (Institute of Psychology) and were used as the speech intelligibility test material. Each stimuli group includes two lists of CMSs, consequently resulting in twelve (6 groups multiplied by 2 lists) lists of CMSs in total.
In each CMS list, each sentence contains five words: the first two words form the introductory sentence frame; the last three words (namely, the critical words) have eight options each, and were used for speech intelligibility test. Each option of critical words in one sentence-position (e.g., the fifth option over the third-word position) can be randomly combined with any options of critical words in the other two sentence-positions (e.g., the sixth option over the fourth-word position and the eighth option over the fifth-word position) to form a semantically congruent and weakly-constraining sentence (see Table 2 for an example of a matrix sentence). In the present study, for each CMS list, eight versions of combinations were selected and used in the speech intelligibility test. Therefore, each CMS list includes eight sentences. In each sentence, only the last three words (critical words) were used for test vocabulary, with the number of test words (three) being within the limitation of young and aging adults' auditory working memory (Bopp and Verhaeghen, 2005; Szenkovits et al., 2012). Moreover, for the critical words in each CMS group (including two CMS lists), we calculated the proportion of each type of consonant in the total number of consonants, the proportion of each type of vowel in the total number of vowels, the proportion of each type of lexical tone in the total number of lexical tones, and eventually made the distributions computed above consistent with their distribution in modern Mandarin Chinese (Tang, 1995).
The six groups of CMSs (each group includes 2 lists of CMSs and each CMS list includes eight sentences) resulted in 96 sentences in total (see Table 2 for one list of CMSs). To validate the high semantic congruency and low context constraint of the sentences in CMS, a series of pre-tests were conducted over the written version of materials. Firstly, to examine the semantic congruency of these sentences, 16 subjects (none of whom participated in the training experiment) were recruited to perform a semantic appropriateness scoring task. The semantic congruency of the last word of each sentence in the CMSs was rated on a 7-point scale (−3 to 3), with a positive score indicating that the corresponding word is semantically congruent given its preceding sentence context (the higher the score, the larger the semantic congruency is). The result showed that all of the critical words in the six groups of CMSs have a semantic-congruency rating score larger than 0.5, indicating that all CMS sentences are semantically congruent. Meanwhile, one-way ANOVA results showed no significant differences in semantic congruency across these six groups of CMSs (F(5.90) = 1.567, p = 0.178). Secondly, to examine the context constraint of the CMS sentences, the cloze probability of the third critical word (final word of each sentence) was accessed by visually presenting the sentence frames just before the final words. Sixteen additional subjects (none of whom participated in the training experiment) were asked to complete the sentences in a meaningful way by filling in the first event that came to their mind. The result of the cloze probability pre-test showed that the cloze probability score of all sentences in the CMSs is less than 25%, which indicates that the CMS sentences have a very weakly constraining sentence context and that the critical words could not be reliably predicted from their preceding context. The one-way ANOVA also showed no significant differences in cloze probability between the six groups of CMSs (F(5.90) = 1.678, p = 0.148). The results of the above pre-tests indicate that the CMS sentences (see Table 3 for detailed test results of the matrix sentence lists.) were well-developed (with each sentence being semantically congruent while having an unpredictable context) and were suitable to be used in the speech intelligibility test.
The spoken version of the 96 CMS sentences was produced by a female speaker of Standard Mandarin Chinese, who was born and raised in Beijing, China. The recording was conducted in a soundproof laboratory with a sampling rate of 44,100 Hz. The average sentence intensity of each sentence was scaled to 70 dB. Each spoken sentence was added with its speech-shaped noise, resulting in a total of 192 experimental sentences in the test part (including 96 sentences for the SNR = 0 condition and 96 sentences for the SNR = −2 condition). Specifically, the speech-shaped noise signal was created for each sentence by using FFT and iFFT function mounted in Matlab, and was added to the corresponding spoken sentence. SNR = 0 indicates that the spoken sentence and its speech spectrum noise have matched the long-term power spectrum; SNR = −2 indicates that the long-term power spectrum of the spoken sentence is 2 dB lower than that of its speech spectrum noise.
The 192 spoken sentences embedded in noise were finally used for the pre-test and post-test phases. These sentences were divided into 4 versions of 96 sentences according to the Latin square procedure based on four experimental conditions (2 “time” [pre-test vs. post-test] multiplied by 2 “SNR condition” [0 dB vs. −2 dB]). In each version, there were 24 sentences for each of the four experimental conditions, with 48 sentences used in pre-test coming from six CMS lists and 48 sentences used in post-test coming from another (different) six CMS lists; meanwhile, in both the pre-test and post-test phases, the 24 sentences in SNR = 0 and SNR = −2 conditions also came from different CMS lists. Therefore, in each version of testing sentences, the sentence content (text content) differed across the four experimental conditions. During the pre-testing (or post-testing), the 96 spoken sentences were presented in a pseudo-random order, with the sentence coming from the same CMS list being presented at least 2 trials apart. Each participant took only one version of testing sentences during the present “pre-test_training_post-test” experiment.
The spoken sentences used in the training phase were 60 pairs of sentences selected from the stimuli used in Zheng and colleagues' study (Zheng et al., 2021). To manipulate Semantic Constraint, each pair of sentences consisted of a strongly constraining (e.g., In order to celebrate his sister's birthday, he bought a …) and a weakly constraining (e.g., In order to give his sister a surprise, he bought a…) sentence frames, leading to the following object nouns (e.g., … cake…) being either highly or low predictable. These object nouns were defined as the “critical nouns” of the present study. The critical noun in each sentence was always preceded by “a transitive verb and a classifier/adjective” (e.g., …bought a cake…). That is, each experimental sentence took the structure of “sentence frame + transitive verb + classifier/adjective + critical noun + sentence-final constituent,” with part (one or two words) of the sentence frame being different while the other words being the same across the strong- and weak-constraint conditions of each pair (see Table 4). The transitive verb immediately preceding the modifier was defined as the critical verb of the sentence.
Table 4. Illustrations of the experimental materials in the strongly vs. weakly constraining conditions.
In order to verify our manipulation of Semantic Constraint, the cloze probability of the critical noun was tested in three different pre-tests by visually presenting the sentence frames just before the critical verbs (pre-verb test), just after the critical verbs (post-verb test), and just before the critical nouns (pre-noun test) to three different groups of participants. All of the participants did not attend the training study and were asked to complete the sentences in a meaningful way by filling in the first event that came to their mind. An ANOVA with semantic constraint (strong and weak) and test type (pre-verb test, post-verb test, vs. pre-noun test) as independent factors revealed a significant main effect of semantic constraint (F(1.59) = 823.5, p < 0.001), test type (F(1.59) = 79.93, p < 0.001) and a significant interaction between semantic constraint and test type (F(2.118) = 51.75, p < 0.001). Further simple effects analysis showed that for all three test types, the predictability probability of critical nouns was significantly higher in the strong-constraint condition than in the weak-constraint condition (pre-verb test: F(1.59) = 68.41, p < 0.001; post-verb test: F(1.59) = 443.96, p < 0.001; pre-noun test: F(1.59) = 414.54, p < 0.001) (Bonferroni correction). In addition, we calculated the predictability of the critical verbs, with reference to the “pre-verb” test format. The results of the paired-samples t-test for verb predictability showed that the verb predictability was significantly higher in the strong-constraint sentences than in the weak-constraint sentences (t(59) = 4.23, p < 0.001). The above cloze probability pre-test demonstrated that our manipulation of semantic context constraint was successful (see Table 5).
The semantic congruency of the critical verbs, the modifiers before the critical nouns, and the critical nouns in the sentence list were also tested by recruiting another 24 participants (none of whom participated in the training experiment). Participants were visually presented with part of the sentence from the beginning of the sentence to the critical verb and were asked to rate the degree of semantic congruency of the corresponding verb given its context on a 7-point scale (−3 to 3, indicating low to high semantic congruency). The semantic congruency of the critical nouns and that of the modifiers preceding the critical nouns was evaluated in the same way as above. A paired-samples was conducted on the rating scores, and the results showed that the semantic congruency of critical nouns was significantly higher in strong semantic constraint sentences than in weak semantic constraint sentences (t(59) = 13.07, p < 0.001); the semantic congruency of critical verbs had no significant difference between strong and weak semantic constraint sentences (t(59) = 0.61, p = 0.54); the semantic congruency of modifiers preceding the noun was also not significant (t(59) = 0.22, p = 0.83) (see Table 5). The significantly higher degree of semantic congruency of critical nouns in the strong-constraint condition (compared to the weak-constraint condition) provided further evidence for the successful manipulation of contextual constraint.
The sixty pairs of training sentences were produced by a female speaker of Standard Mandarin Chinese, who was born and raised in Beijing, China. The recording was conducted in a soundproof laboratory at a sampling rate of 22,500 Hz. Speech spectrum noise was added to each of the 60 pairs of spoken sentences, with each sentence having a “SNR = −3” condition and a “SNR = −5” condition.
Finally, two versions of training sentences were constructed, with one version including only the strong-constraint sentences and another version including only the weak-constraint sentences. During the training phase of the present study, 32 participants only listened to the weak-constraint sentences, while the other 32 participants only listened to the strong-constraint sentences.
The participants were tested individually. After the participants entered the laboratory, a short introduction to the experimental procedure was given. The participants' intelligibility to speech in noise is first tested (pre-test phase), followed by the training phase (training phase), and then the post-testing (post-test phase) was implemented after the training. The experimental presentation and data collection were realized by E-prime software. The experimental speech was presented to the subjects in a soundproofed lab. Participants were instructed to sit 70 cm in front of the computer screen, listening through a FIREFACE UCX sound card and a Sennheiser HD660S headphone.
During the pre-test (or post-test) phase, before the start of each trial, a 300 ms cue with a “+” gaze point and a 500 ms blank screen were presented, followed by the experimental audio, which was followed by a vocabulary selection screen that presented the subject with a sentence frame (consisting of the first two words of the sentence) and the next three critical words in sequence (the 3rd, 4th, and 5th words of the sentence). For each of the critical words (3rd/4th/5th word), participants were presented with 8 equivalent alternative vocabulary options, and were asked to select the words they heard by pressing one of eight keys on the keyboard. Subjects were asked to press the key quickly and accurately, and to press the “space bar” to skip words if they did not hear them or were unsure of them, to avoid guessing or blind selection. Before the formal experimental process began, practice trials were given. Four practice sentences (filler materials) were set for the practice round. The accuracy and response time of the keystrokes were recorded. The whole procedure of the pre-test lasted around 10 to 15 minutes. Participants were instructed to rest for 5 to 10 minutes before the next phase.
During the training phase, participants were presented with the “unclear-clear-unclear” audio and were asked to rate the clarity of the two unclear audio sentences. Specifically, for each trial, participants first heard an unclear spoken sentence (speech in noise), and then the clear version of the same sentence was auditorily presented, which was then followed by the auditory presentation of the unclear version again. In addition, participants were required to rewrite the sentences after the first time they heard the unclear sentences to ensure that they were serious about the training process. Five practice sentences (filler materials) were set for the training part to ensure that the subjects were familiar with the experimental process. Then, the formal training process was performed, which included 60 training sentences. The participants were instructed to take a break after they finished the first 30 sentences. They started the next half when they felt ready to continue.
In order to avoid fatigue, for each participant, the post-test phase started around 5 to 10 minutes after the end of the training phase. The post-test phase was the same procedure as the pre-test phase and eventually lasted around 10 min. The whole experiment lasted around 70 min.
For the speech perception scores of the pre-test/post-test phases, analysis of accuracy response was performed over all of the corresponding data, whereas analysis of reaction time was performed over 73% of all data (with 27% of the response time data being deleted from statistical analysis due to response time longer than 5,000 ms or due to incorrect response).
For the accuracy of speech perception in the pre-test and post-test phases, analyses were conducted using R's lmerTest package (Kuznetsova et al., 2017) for a generalized linear mixed-effects model (GLME), modeled with accuracy as the dependent variable. The following model was constructed:
ACC in this model denotes accuracy of the speech intelligibility test, time denotes pre and post-test, group denotes group (strong-constraint group vs. weak-constraint group), cond denotes signal-to-noise ratio (SNR = 0 vs. SNR = −2), item denotes item factor, and subj denotes subject factor.
For the response time of speech perception in the pre-test and post-test phases, analyses were conducted using R's lmer package (Bates and Maechler, 2009) for linear mixed-effects model analyses, with the subject factor and item factor as random factors (Pinheiro and Bates, 2000; Baayen et al., 2008) for model construction. Based on the “Parsimonious Mixed Models” principle mentioned by Bates et al. (2018), in fitting each set of data, the final model that maximizes the fit was selected, and the analysis was coded using the R default treatment coding. The following model was constructed:
With model simplification, the following model was finally adopted:
RT in the model denotes speech intelligibility tests' response time, time denotes pre and post-test, group denotes group (strong constraint group vs weak constraint group), cond denotes signal-to-noise ratio (SNR = 0 vs. SNR = −2), item denotes item factor, and subj denotes subject factor.
The values of the correct sentence repetition (during the first presentation of speech-in-noise) and of the sentence clarity rating (during the first and second presentations of speech-in-noise) are shown in Table 6. To test whether the participants understood the experimental tasks, paired t-tests were adopted on the sentence rating score for the strong-constraint and weak-constraint groups separately. Results showed that there was a significant difference between the first rating scores and the second rating scores in the strong constraint group (t(31) = −15.98, p < 0.001), with scores of the second rating (post-test) significantly higher than that of the first rating (pre-test). The same is true with the weak constraint group (t(31) = −15.30, p < 0.001). The results indicate that the subjects understood the experimental task well and completed the auditory training.
For the analysis performed over the speech intelligibility accuracy during the pre-test and post-test phases, the GLME showed a significant main effect of cond (namely, signal-to-noise ratio) (F = 157.8, p < 0.001), indicating that the accuracy of speech intelligibility was significantly higher in the SNR = 0 condition than in the SNR = −2 condition. More importantly, a significant two-way interaction between time (pre-vs. post-test) and group (strong- vs. weak-constraint) (F = 4.59, p = 0.032) was observed. Further simple effects analysis showed that the accuracy of the speech intelligibility test was significantly improved in the post-test phase compared to the pre-test phase, with this improvement being significant for both the strong-constraint group (b = −0.202, SE = 0.082, z = −2.452, p = 0.014) and the weak-constraint group (b = −0.347, SE = 0.083, z = −4.167, p < 0.001) (see Table 7). In sum, the significant time × group interaction and the relative p values for the simple effect of time at each level of group (with p = 0.014 in the strong-constraint group and p < 0.001 in the weak-constraint group), taken together, suggested the training effect was significantly enhanced in the group with weak-constraint sentences as training stimuli compared to that with strong-constraint sentences as training stimuli.
Analysis of response times revealed significant main effects for cond (signal-to-noise ratio) (F = 98.65, p < 0.001) and for time (pre vs. post-tests) (F = 62.10, p < 0.001). Specifically, the reaction time of the speech intelligibility test was significantly shorter during the post-test phase than during the pre-test phase (see Table 8), indicating the speech intelligibility improvement caused by our perceptual learning.
The present study examined how the lexical predictability of spoken sentences used for training affects the perceptual learning of degraded speech, with an aim to deepen our understanding of the internal mechanisms underlying this learning process and to find a more efficient way of training. The major results were that the training process (around 30 min of 60-sentence training) led to significant improvement of speech-in-noise perception (as indicated by increased perception accuracy and reduced response time in the post-test compared to the pre-test) in both the group with strong-constraint sentences as training stimuli and the group with weak-constraint sentences as training stimuli. More importantly, this training-related speech-in-noise perception improvement was enhanced in the weak-constraint group (as indicated by enhanced perception accuracy improvement) as compared to the strong-constraint group. These results were discussed in details below.
The present results showed that although the training-related speech-in-noise perception improvement was observed regardless of the lexical predictability of training sentences, this training improvement effect was more pronounced in the group with weak-constraint sentences as training stimuli compared to the group with strong-constraint sentences as training stimuli. In the present study, the two groups of participants were matched on their general cognitive ability (namely, working memory) and long-term vocabulary knowledge, although these two factors were found to be likely to influence predictive sentence processing (Huettig and Janse, 2016; Choi et al., 2017; Ito et al., 2018). Moreover, the speech-intelligibility-test sentences used in the pre-test and post-test phases were exactly the same across the two groups after counterbalancing. The only major difference between these two groups was the lexical predictability of the spoken sentences that were used as training stimuli. Therefore, the enhanced training effect in the weak-constraint group (compared to the strong-constraint group) was less likely to be caused by individual differences between the two groups or by stimuli differences unrelated to lexical predictability. A more rational interpretation for this training-effect enhancement is that the perceptual learning of degraded speech (e.g., speech-din-noise) can be affected by the lexical predictability of training spoken sentences, with weak-constraint and congruent sentences (compared to strong-constraint and congruent sentences) being able to bolster this perceptual learning effect and lead to greater learning-related improvement.
As mentioned in the introduction section, the existing studies have already found that perception of degraded speech can be facilitated by the perceptual learning process (e.g., Newman et al., 1997; Borsky et al., 1998; Banai and Lavner, 2019), and the learning-related speech perception improvement can be observed among people with different ages (Peelle and Wingfield, 2005) and with different levels of hearing loss (Karawani et al., 2016). The result of the present study is not only consistent with these existing studies but also extends our understanding of speech perceptual learning by showing that the low lexical-predictability of training sentences can bolster the learning-related improvement, which echoes with the enhanced syntactic learning effect by exposing participants to surprisal (vs. predictable) syntactic structure (Fazekas et al., 2020). The new findings of the present study can help to provide insight into the design of training materials and programs to improve speech perception in challenging situations.
It needs to be mentioned that, different from the enhancement of learning improvement by low lexical-predictability of training sentences in our study, Davis and colleagues' study suggested that training sentences with more high-level information led to a better perceptual learning effect. Specifically, Davis and colleagues found that participants training with normal sentences gained larger speech perceptual learning improvement compared to those training with syntactic prose (in which content words are randomly placed), (absence of content words), or nonword sentences (Davis et al., 2005). The discrepancy might be related to the different experimental manipulation and comparisons made in these two studies. That is, the comparison was made between normal sentences and unnormal sentences in Davis and colleagues' study (Davis et al., 2005), but between two types of normal sentences (namely, strong- vs. weak-constraint sentences, both including content words and syntactic information) in the present study. Compared to unnormal sentences such as Jabberwocky sentences or nonword sentences, normal sentences include more high-level information (such as lexico-semantic information) that is considered to constrain and facilitate lexical perception in a top-down manner. Although the study of Davis et al. (2005) provides evidence for the role of high-level lexico-semantic information in driving perceptual learning of distorted speech signals, it left the role of predictive processing unsettled. The present study, however, further directly manipulated the lexical predictability of normal sentences that were used as training stimuli, and hence provided new insights into the nature of the prediction-error-based theory of speech perceptual learning.
Regarding the internal mechanisms underlying perceptual learning, the predictive coding account assumes that perceptual learning involves at least the changes in the backward (or and lateral) connections of the hierarchical cortical/representational system (Rao and Ballard, 1999; Friston, 2005, 2010; Kuperberg and Jaeger, 2016). For example, the perceptual learning of degraded speech with spoken sentences as training stimuli is likely to involve hierarchical backward projections across sentence-meaning, lexical, phonetic/phonological, and auditory sensory levels of representations. The modulation/adjustment of these hierarchical connections (e.g., backward connections) during perceptual learning can not only be guided by prior top-down prediction (high-level representations predicted based on the contextual information) but also be driven by posterior prediction-error minimization (when the actually-perceived sensory input is unexpected or mismatches with the top-down predictions) (Rao and Ballard, 1999; Friston, 2005, 2010). These two sources of connection modulation are tightly related, with one source being likely to be predominant over the other as a function of the specific processing situations. According to the backward projections associated with “prior top-down prediction,” the high-level lexical/semantic representations derived from the strong-constraint sentences (compared to the weak-constraint sentences) are expected to guide and facilitate the sensory representation of upcoming sensory inputs, consequently leading to enhanced perceptual learning (see Norris et al., 2003; Davis et al., 2005). In contrast, according to the backward adjustment driven by “posterior prediction-error minimization,” the processing of the weak-constraint sentences (compared to the strong-constraint sentences) will lead to enhanced prediction error and long-lasting hierarchical projections, consequently resulting in enhanced perceptual learning. The modulation effect of the “prior top-down prediction” mechanism alone could not account for the result of the present study, which observed enhanced learning improvement in the weak-constraint condition relative to the strong-constraint condition.
Instead, the result of the present study is consistent with the learning effect pattern inferred from the “posterior prediction-error minimization” mechanism. That is, during the perceptual learning process of our present study, it might be that listeners in the weak-constraint condition (compared to those in the strong-constraint condition) are less able to use available high-level semantic context of the current sentence and knowledge retrieved from long-term memory to predict upcoming lexical information (such as the lexico-semantic and even the phonological information of upcoming words) (e.g., Kuperberg and Jaeger, 2016; Ito et al., 2017; Li et al., 2020; Zheng et al., 2021), and therefore larger prediction error (difference between the expected and actually-perceived bottom-up signals) was generated; these prediction-error activities were passed up along the representational hierarchy to the remaining higher-level representations via forward connections, and meanwhile backward (or and lateral) adjustments were initiated to suppress and minimize these prediction-error (Rao and Ballard, 1999; Friston, 2005), which provided more time for optimizing the parameters of the hierarchical connections, hence bolstering the perceptual learning of degraded speech. Therefore, listeners who were trained with low-predictive spoken sentences can get a larger perceptual learning effect when compared to those who were trained with highly-predictive sentences.
The present study still has some limitations that need to be addressed in future studies. It only demonstrated the facilitating effect of low predictive sentences (compared to highly predictive sentences) on the perceptual learning of degraded speech at the behavioral level. The precise neural mechanisms underlining these perceptual learning effects remain to be examined with the help of neuroimaging techniques. Moreover, further studies are needed to explore how to make full use of the “prior top-down prediction” and “posterior prediction-error minimization” sources of perceptual learning to design training stimuli to reach more efficient learning. In addition, the long-term consolidation of this speech perceptual learning effect and the corresponding neural mechanisms also need to be examined in the future.
The present study examined how the lexical predictability of spoken sentences affects perceptual learning of degraded speech, by using spoken sentences (embedded in noise) as training materials and strictly controlling the lexical predictability of these training sentences. The results showed that the accuracy of the speech-in-noise intelligibility test was significantly improved in the post-training phase relative to the pre-training phase, and this learning-related improvement was significantly enhanced in listeners with weak-constraint sentences as training stimuli (compared to those with strong-constraint sentences as training stimuli). This enhancement effect of low lexical predictability on learning-related improvement supports a prediction-error-based account of perceptual learning.
The raw data supporting the conclusions of this article will be made available by the authors, without undue reservation.
The studies involving human participants were reviewed and approved by Ethics Committee of Institute of Psychology, Chinese Academy of Sciences. The patients/participants provided their written informed consent to participate in this study.
YL: methodology, investigation, formal analysis, visualization, and writing—original draft. CF and CL: methodology and writing—review and editing. XL: conceptualization, methodology, writing—review and editing, resources, and funding acquisition. All authors contributed to the article and approved the submitted version.
This work was supported by Grants from the National Natural Science Foundation of China (32171057).
The authors declare that the research was conducted in the absence of any commercial or financial relationships that could be construed as a potential conflict of interest.
All claims expressed in this article are solely those of the authors and do not necessarily represent those of their affiliated organizations, or those of the publisher, the editors and the reviewers. Any product that may be evaluated in this article, or claim that may be made by its manufacturer, is not guaranteed or endorsed by the publisher.
Baayen, R. H., Davidson, D. J., and Bates, D. M. (2008). Mixed-effects modeling with crossed random effects for subjects and items. J. Mem. Lang. 59, 390–412. doi: 10.1016/j.jml.12005
Banai, K., and Lavner, Y. (2019). Effects of stimulus repetition and training schedule on the perceptual learning of time-compressed speech and its transfer. Atten. Percept. Psychophys. 81, 2944–2955. doi: 10.3758/s13414-019-01714-7
Bates, D., Kliegl, R., Vasishth, S., and Baayen, R. H. (2018). Parsimonious mixed models. arXiv:1506.04967 [stat.ME]. doi: 10.48550/arXiv.1506.04967
Bates, D., and Maechler, M. (2009). lme4: Linear Mixed-Effects Models Using S4 Classes. R Package Version 0.999375-32. [Computer software]. Available online at: http://cran.r-project.org/web/packages/lme4/index.html
Bieber, R. E., and Gordon-Salant, S. (2021). Improving older adults' understanding of challenging speech: auditory training, rapid adaptation and perceptual learning. Hear Res. 402, 108054. doi: 10.1016/j.heares.2020.108054
Blank, H., and Davis, M. H. (2016). Prediction errors but not sharpened signals simulate multivoxel fMRI patterns during speech perception. PLoS Biol. 14, e1002577. doi: 10.1371/journal.pbio.1002577
Bonhage, C. E., Mueller, J. L., Friederici, A. D., and Fiebach, C. J. (2015). (2015). Combined eye tracking and fMRI reveals neural basis of linguistic predictions during sentence comprehension. Cortex 68, 33–47. doi: 10.1016/j.cortex.04011
Bopp, K. L., and Verhaeghen, P. (2005). Aging and verbal memory span: a meta-analysis. J. Gerontol. B. Psychol. Sci. Soc. Sci. 60, 223–33. doi: 10.1093/GERONB/60.5. P223
Borsky, S., Tuller, B., and Shapiro, L. P. (1998). “How to milk a coat:” the effects of semantic and acoustic information on phoneme categorization. J. Acoust. Soc. Am. 103, 2670–2676. doi: 10.1121/1.422787
Choi, W., Lowder, M. W., Ferreira, F., Swaab, T. Y., and Henderson, J. M. (2017). Effects of word predictability and preview lexicality on eye movements during reading: a comparison between young and older adults. Psychol. Aging 32, 232. doi: 10.1037/pag0000160
Connine, C. M., Titone, D., and Wang, J. (1993). Auditory word recognition: extrinsic and intrinsic effects of word frequency. J. Exp. Psychol. Learn. Mem. Cogn. 19, 81–94. doi: 10.1037/0278-7393.19.1.81
Cooper, A., and Bradlow, A. R. (2016). Linguistically guided adaptation to foreign-accented speech. J. Acoust. Soc. Am. 140, EL378–EL384. doi: 10.1121/1.4966585
Davis, M. H., Johnsrude, I. S., Hervais-Adelman, A., Taylor, K., and Mcgettigan, C. (2005). Lexical information drives perceptual learning of distorted speech: evidence from the comprehension of noise-vocoded sentences. J. Exp. Psychol. Gen. 134, 222–241. doi: 10.1037/0096-3445.134.2.222
de Lange, F. P., Heilbron, M., and Kok, P. (2018). How do expectations shape perception? Trends. Cogn. Sci. 22, 764–779. doi: 10.1016/j.tics.06002
Dikker, S., and Pylkkänen, L. (2013). Predicting language: MEG evidence for lexical preactivation. Brain. Lang. 127, 55–64. doi: 10.1016/j.bandl.08004
Eisner, F., and McQueen, J. M. (2005). The specificity of perceptual learning in speech processing. Percept. Psychophys. 67, 224–238. doi: 10.3758/BF03206487
Fazekas, J., Jessop, A., Pine, J., and Rowland, C. (2020). Do children learn from their prediction mistakes? A registered report evaluating error-based theories of language acquisition. R. Soc. Open. Sci. 7, 180877. doi: 10.1098/rsos.180877
Friston, K. (2005). A theory of cortical responses. Philos. Trans. R. Soc. B, Biol. Sci. 360, 815–836. doi: 10.1098/rstb.2005.1622
Friston, K. (2010). The free-energy principle: a unified brain theory? Nat. Rev. Neurosci. 11, 127–138. doi: 10.1038/nrn2787
Ganong, W. F. (1980). Phonetic categorization in auditory word perception. J. Exp. Psychol. Hum. Percept. Perform. 61, 110–125. doi: 10.1037//0096-61110
Goldstone, R. L. (1998). Perceptual learning. Annu. Rev. Psychol. 49, 585612. doi: 10.1146/annurev.psych.49.1.585
Grisoni, L., Mille, M. C., and Pulvermülle, F. (2017). Neural correlates of semantic prediction and resolution in sentence processing. J. Neurosci. 37, 4848–4858. doi: 10.1523/jneurosci.2800-16.2017
Grisoni, L., Tomasello, R., and Pulvermüller, F. (2020). Correlated brain indexes of semantic prediction and prediction error: brain localization and category specificity. Cereb. Cortex. 31, 1553–1568. doi: 10.1093/cercor/bhaa308
Huettig, F., and Janse, E. (2016). Individual differences in working memory and processing speed predict anticipatory spoken language processing in the visual world. Lang. Cogn. Neurosci. 31, 80–93. doi: 10.1007/s10519-009-9315-7
Ito, A., Martin, A. E., and Nieuwland, M. S. (2017). Why the A/AN prediction effect may be hard to replicate: a rebuttal to Delong, Urbach, and Kutas (2017). Lang. Cogn. Neurosci. 32, 974–983. doi: 10.1080/23273798.2017.1323112
Ito, A., Pickering, M. J., and Corley, M. (2018). Investigating the time-course of phonological prediction in native and non-native speakers of English: a visual world eye-tracking study. J. Mem. Lang. 98, 1–11. doi: 10.1016/J.JML.09002
Karawani, H., Bitan, T., Attias, J., and Banai, K. (2016). Auditory perceptual learning in adults with and without age-related hearing loss. Front. Psychol. 6, 2066. doi: 10.3389/fpsyg.2015.02066
Kraljic, T., and Samuel, A. G. (2005). Perceptual learning for speech: is there a return to normal? Cogn. Psychol. 51, 141–178. doi: 10.1016/j.cogpsych.05001
Kuperberg, G. R., and Jaeger, T. F. (2016). What do we mean by prediction in language comprehension? Lang. Cogn. Neurosci. 31, 1–28. doi: 10.1080/23273798.2015.1102299
Kuznetsova, A., Brockhoff, P. B., and Christensen, R. H. (2017). lmerTest package: tests in linear mixed effects models. J. Stat. Softw. 82, 13. doi: 10.18637./jss.v082.i13
Li, X., Ren, G., Zheng, Y., and Chen, Y. (2020). How does dialectal experience modulate anticipatory speech processing? J. Mem. Lang. 115, 104169. doi: 10.16/j.jml.2020.104169
Li, X., Shao, X., Xia, J., and Xu, X. (2019). The cognitive and neural oscillatory mechanisms underlying the facilitating effect of rhythm regularity on speech comprehension. J. Neurolinguistics, 49, 155–167. doi: 10.1016/j.jneuroling.05004
Miller, J. D., Watson, C. S., Dubno, J. R., and Leek, M. R. (2015). Evaluation of speechperception training for hearing aid users: a multisite study in progress. Semin. Hear. 36, 273–283. doi: 10.1055/s-0035-1564453
Murray, S. O., Schrater, P., and Kersten, D. (2004). (2004). Perceptual grouping and the interactions between visual cortical Areas. Neural. Netw. 17, 695–705. doi: 10.1016/j.neunet.03010
Myers, E. B., and Mesite, L. M. (2014). Neural systems underlying perceptual adjustment to non-standard speech tokens. J. Mem. Lang. 76, 80–93. doi: 10.1016/j.jml
Newman, R. S., Sawusch, J. R., and Luce, P. A. (1997). Lexical neighborhood effects in phonetic processing. J. Exp. Psychol. Hum. Percept. Perform. 23, 873–889. doi: 10.1037/0096-233.87
Norris, D., McQueen, J. M., and Cutler, A. (2003). Perceptual learning in speech. Cognit. Psychol. 47, 204–238. doi: 10.1016/S0010-0285(03)00006-9
Obleser, J., and Kotz, S. A. (2010). Expectancy constraints in degraded speech modulate the language comprehension network. Cereb. Cortex. 20, 633–640. doi: 10.1093/cercor/bhp128
Peelle, J. E., and Wingfield, A. (2005). Dissociations in perceptual learning revealed by adult age differences in adaptation to time-compressed speech. J. Exp. Psychol. Hum. Percept. Perform. 31, 1315. doi: 10.1037/0096-316.1315
Pinheiro, J. C., and Bates, D. M. (2000). Mixed-Effects models in S and S-PLUS. New York, NY: Springer. doi: 10.1007/978-1-4419-0318-1
Rao, R. P., and Ballard, D. H. (1999). Predictive coding in the visual cortex: a functional interpretation of some extra-classical receptive-field effects. Nature Neurosci. 2, 79–87. doi: 10.1038/4580
Sohoglu, E., and Davis, M. H. (2016). Perceptual learning of degraded speech by minimizing prediction error. Proc. Natl. Acad. Sci. doi: 10.1073./pnas.1523266113
Sohoglu, E., and Davis, M. H. (2020). Rapid computations of spectrotemporal prediction error support perception of degraded speech. Elife 9, e58077. doi: 10.7554/eLife.58077
Sweetow, R., and Sabes, J. (2006). The need for and development of an adaptive listening and communication enhancement (LACE) program. J. Am. Acad. Audiol. 17, 538–558. doi: 10.3766/jaaa.17.8.2
Szenkovits, G., Peelle, J. E., Norris, D., and Davis, M. H. (2012). Individual differences in premotor and motor recruitment during speech perception. Neuropsychologia 50, 1380–1392. doi: 10.1016/j.neuropsychologia.02023
Tang, Y. (1995). The counting analysis of Mandarin speech[??]. J. Chengde. Teachers. College. Nationalities. 1, 66–76. doi: 10.16729/j.cnki.jhnun.01017
Wang, L., Kuperberg, G., and Jensen, O. (2018). Specific lexico-semantic predictions are associated with unique spatial and temporal patterns of neural activity. Elife, 7, e39061. doi: 10.7554/eLife.39061
Keywords: speech intelligibility, noised speech, lexical predictability, perceptual learning, predictive coding account
Citation: Li Y, Fan C, Liu C and Li X (2023) The modulating effect of lexical predictability on perceptual learning of degraded speech. Front. Lang. Sci. 2:1139073. doi: 10.3389/flang.2023.1139073
Received: 06 January 2023; Accepted: 26 May 2023;
Published: 22 June 2023.
Edited by:
Anna M. Woollams, The University of Manchester, United KingdomReviewed by:
Bernd J. Kröger, RWTH Aachen University, GermanyCopyright © 2023 Li, Fan, Liu and Li. This is an open-access article distributed under the terms of the Creative Commons Attribution License (CC BY). The use, distribution or reproduction in other forums is permitted, provided the original author(s) and the copyright owner(s) are credited and that the original publication in this journal is cited, in accordance with accepted academic practice. No use, distribution or reproduction is permitted which does not comply with these terms.
*Correspondence: Xiaoqing Li, bGl4cUBwc3ljaC5hYy5jbg==
Disclaimer: All claims expressed in this article are solely those of the authors and do not necessarily represent those of their affiliated organizations, or those of the publisher, the editors and the reviewers. Any product that may be evaluated in this article or claim that may be made by its manufacturer is not guaranteed or endorsed by the publisher.
Research integrity at Frontiers
Learn more about the work of our research integrity team to safeguard the quality of each article we publish.