- Department of Phoniatrics, Pedaudiology, and Communication Disorders, RWTH Aachen University, Aachen, Germany
Computer-implemented neural speech processing models can simulate patients suffering from neurogenic speech and language disorders like aphasia, dysarthria, apraxia of speech, and neurogenic stuttering. Speech production and perception tasks simulated by using quantitative neural models uncover a variety of speech symptoms if neural dysfunctions are inserted into these models. Neural model dysfunctions can be differentiated with respect to type (dysfunction of neuron cells or of neural connections), location (dysfunction appearing in a specific buffer of submodule of the model), and severity (percentage of affected neurons or neural connections in that specific submodule of buffer). It can be shown that the consideration of quantitative computer-implemented neural models of speech processing allows to refine the definition of neurogenic speech disorders by unfolding the relation between inserted neural dysfunction and resulting simulated speech behavior while the analysis of neural deficits (e.g., brain lesions) uncovered from imaging experiments with real patients does not necessarily allow to precisely determine the neurofunctional deficit and thus does not necessarily allow to give a precise neurofunctional definition of a neurogenic speech and language disorder. Furthermore, it can be shown that quantitative computer-implemented neural speech processing models are able to simulate complex communication scenarios as they appear in medical screenings, e.g., in tasks like picture naming, word comprehension, or repetition of words or of non-words (syllable sequences) used for diagnostic purposes or used in speech tasks appearing in speech therapy scenarios (treatments). Moreover, neural speech processing models which can simulate neural learning are able to simulate progress in the overall speech processing skills of a model (patient) resulting from specific treatment scenarios if these scenarios can be simulated. Thus, quantitative neural models can be used to sharpen up screening and treatment scenarios and thus increase their effectiveness by varying certain parameters of screening as well as of treatment scenarios.
1. Introduction
Neural models of speech processing comprise the modeling of speech production and speech perception/comprehension. Production models start with the specification of a verbal intention at the semantic or concept level, generate lemmata and phonological forms (cognitive-linguistic model part). These models subsequently initiate the motor execution processes including articulatory movement generation, acoustic signal generation, and sensory feedback signal generation (sensorimotor model part). Well-known neural models representing the sensorimotor part of speech production have been developed by Dell (1986) and Dell et al. (2007, 2013; spreading activation model), Roelofs (1992, 1997, 2014; WEAVER model), and Levelt et al. (1999; word production model). Well-known neural models representing the sensorimotor part have been developed by Guenther (2006, 2016; DIVA model), Guenther et al. (2006; DIVA model), and Bohland et al. (2010; GODIVA model). A biologically inspired feedback-aware speech task control approach has been introduced by Parrell et al. (2019; FACTS) and a spiking neuron model covering the linguistic and sensorimotor part has been developed by Kröger et al. (2012, 2016, 2020, 2022; ACT model). All these neural models are concrete, quantitatively implemented, and checked by computer-simulating realistic communication scenarios.
A comprehensive neurobiologically motivated but still not computer-implemented model of speech perception and comprehension is introduced by Hickok and Poeppel (2007, 2016). This model comprises modules for spectro-temporal analysis of incoming acoustic speech signals, for phonological processing and then splits in a ventral processing stream including lexical, semantic, and grammatical processing and in a dorsal stream for further auditory, somatosensory, and motor processing.
Combined production-perception models (speech processing models) are needed if the simulation of speech learning (i.e., modeling of speech acquisition) is of interest (developmental neural models; see Warlaumont and Finnegan, 2016; Kröger et al., 2022). During the babbling phase—which appears in the first year of lifetime—first sensorimotor relations establish (mainly auditory-to-motor relations) and later during the imitation phase, in which the toddler imitates speech items produced by caretakers, the mental lexicon and the grammatical repertoire of the target language is learned and stored. These speech acquisition phases are needed to be simulated in a realistic neural speech processing model. Moreover, complete neural speech processing models are needed for the simulation of speech communication scenarios, i.e., scenarios, comprising capabilities like listening to and speaking with a communication partner. It should be kept in mind that all medical screenings in case of diagnosis of speech disorders include such communication scenarios between test supervisor (communication partner) and patient (model).
It will be shown that the neural models reviewed here are able to unfold the complex associations between neural dysfunctions and symptoms of disordered speech in case of neurogenic speech and language disorders. Thus, models can help to refine the definition of speech and language disorders because an underlying neural dysfunction, which is the basis for the definition of a speech disorder, can be clearly defined in a neural model while a lesioned brain region of patients (i.e., anatomical information) which probably is located by imaging techniques in a patient (e.g., Crinion et al., 2013) does not necessarily point in a one-to-one relation on a specific neural dysfunction (i.e., functional information) nor on a specific speech and language disorder. While functional information is directly defined as inserted distortion to the model in a specific neural subnetwork, this information needs to be extracted indirectly from behavioral data by asking patients to perform specific speech and language tests in order to collect data of relevant speech errors from these screening scenarios.
In this paper we will concentrate on four types of neurogenic speech and language disorders, i.e., on aphasia, dysarthria, apraxia of speech, and neurogenic stuttering. Aphasia can be defined as a disorder resulting from neural dysfunctions arising in the cognitive-linguistic part of the speech processing network. Aphasia can affect the activation of a word at the lexical level even if motor processes are intact or can affect the comprehension of a word even if auditory perception is intact (e.g., Roelofs, 2014). Dysarthria and apraxia of speech result from neural dysfunctions in the sensorimotor part of the brain including the peripheral motor neuron system. All types of dysarthria reflect functional deficits appearing during motor execution even in case of fully functional articulatory organs (Kearney and Guenther, 2019). Apraxia of speech reflect deficits in motor planning and motor programming (Van der Merwe, 2021). Neurogenic stuttering reflects deficits in the initiation of execution of motor programs (Chang and Guenther, 2020).
Symptoms of speech and language disorders typically appear in communication situations and can be evoked in speech tasks like picture naming, narration tasks, word, non-word (logatome), or syllable repetition tasks, or in word or sentence comprehension tasks. For all types of neurogenic speech and language disorders, diagnosis procedures (also called screenings) comprise a batterie of tests and most of these tests are speech mediated tasks (i.e., the supervisor instructs the patient verbally, gives test items verbally and the patient answers verbally; a non-speech mediated task would be picture pointing like in the Token Test; here even the target words could be presented non-verbally, for example as written text). Well-known and widely used screenings in case of suspected aphasia are e.g., the Token Test (De Renzi and Vignolo, 1962; De Renzi and Faglioni, 1978), the Frenchay Aphasia Screening Test (FAST, Enderby et al., 1987), the Acute Aphasia Screening Protocol (Crary et al., 1989), the Aachen Aphasia Bedside Test (Biniek et al., 1992), and the Bedside Western Aphasia Battery (Kertesz, 2006). These screenings typically (i) assess comprehension, e.g., by pointing on objects on cards portraying a scene and/or geometric shapes, by executing simple movements based on instructions given by the test supervisor, (ii) assess expression, e.g., by describing a picture, by repeating words, or by naming of objects displayed on pictures, (iii) assess reading capabilities by reading words or short texts, and (iv) assess writing capabilities by writing words or a short text which describes a scene displayed on pictures.
Screenings for detecting dysarthria or apraxia of speech are often combined with screenings for differential diagnosis together with suspected aphasia and are sometimes subsumed as screenings for neurological communicative disorders (e.g., Araki et al., 2021) or as screenings for differential diagnosis of different types of neurogenic speech disorders (e.g., Allison et al., 2020). These screenings include verbal-linguistic sections (e.g., word and nonword repetition, object naming, word writing, dictation) and articulatory sections including non-speech tasks like oral movement analysis and tasks like diadochokinesis, i.e., repetition of syllable sequences like [badaga] or [pataka] as often as possible and as fast as possible. Apraxia of speech screenings as well include verbal-linguistic tests and articulatory tests like word and non-word repetition, sentence production, and phonological awareness tests. Here, the analysis of speech items which are uttered by patients in addition comprises phonetic transcriptions to identify prosodic and segmental errors (Ballard et al., 2016; Allison et al., 2020).
Treatments for all neurogenic speech disorders mainly comprise practice for improving speaking capabilities in case of sentence and word production. During ongoing treatment, the training concentrates on speech items with increasing length and complexity. Lexical learning strategies for the association of phonological word form and meaning aim to widen the vocabulary of patients in case of patients suffering from different forms of aphasia (Tippett et al., 2015). Practice for syllable production to learn the pronunciation of different speech sounds in typical speech-like environments and in combination with other speech sounds within a syllable is focused on in case of treatments for patients suffering from apraxia of speech (Ballard et al., 2000). In case of dysarthria in addition detailed advises are given for increasing or reducing speaking rate and speaking intelligibility or for increasing speech and non-speech motor capabilities for the neuromuscular system of several articulators including respiration and phonation (Palmer and Enderby, 2007).
2. Functional location, type, and severity of neural dysfunctions
In this paper a comprehensive sketch of a computer-implementable speech processing model is introduced (Figure 1 and Kröger et al., 2022). Figure 1 illustrates that models of speech processing can be divided in functional subnetworks or modules which specify functional locations of parts of the neural network and that each of these subnetworks or modules can be associated with specific cortical and subcortical brain regions. The model sketch presented in Figure 1 comprises a cognitive-linguistic model part for which the associations of subnetworks or modules to brain regions are outlined by Roelofs (2014) and a sensorimotor model part for which these relations are outlined by Guenther (2006), Bohland et al. (2010), and Kearney and Guenther (2019).
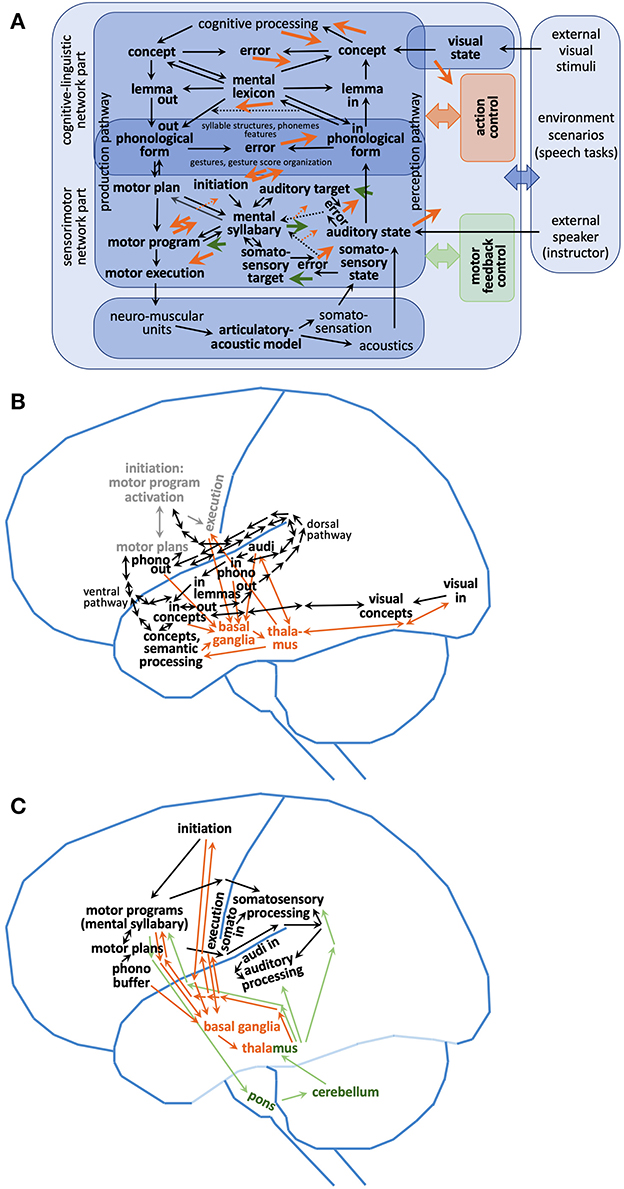
Figure 1. (A) Functional architecture of a sketch of a neural model of speech processing including (B) localization of modules of the cognitive-linguistic subnetwork and (C) localization of modules of the sensorimotor subnetwork. Light gray modules and connections within (B) represent sensorimotor components. Mental lexicon and mental syllabary are the central knowledge and skill repositories within the cognitive-linguistic and sensorimotor part of the model as indicated in (A). The mental lexicon stores concepts, lemmata and phonological forms of already learned words. The mental syllabary stores motor plans, motor programs, auditory states, and somatosensory states of already learned syllables. Mental lexicon: “in” marks input buffers; “out” marks output buffers of the mental lexicon at the phonological, lemma, and concept (i.e., semantic) level. “in”-buffers are activated in connection with auditory and somatosensory processing; see part (C) of this figure and indicates buffers at auditory and somatosensory primary cortex areas. “out” marks output buffers of the mental lexicon at all three levels (concept, lemma, and phonological form) and these buffers are activated in connection with production processes and indicates buffers at temporal as well as at frontal lobe of the neocortex. All in- and output buffers are interconnected (i.e., associated with each other by neural connections) in order to represent the whole semantic, wordform, and phonological form knowledge stored in the mental lexicon for a specific target language. Cortico-cortical loops: (i) orange arrows indicate connections between specific cortical modules (neural buffers) and the subcortical action control module (action control loop including basal ganglia and thalamus). Action control is needed for guaranteeing the correct process flow in case of any production or perception task including motor program execution. Basal ganglia and thalamus (orange) are central while cortical modules (black) are located lateral (neocortex) in (B, C). Orange dashed arrows in (A) indicate transfer of feedback information for the action control loop in case of learning (see Kröger et al., 2022). (ii) Green arrows indicate connections between specific cortical modules (neural buffers) and the subcortical motor feedback loop (motor feedback loop comprising parts of the pons, cerebellum, and thalamus). This second cortico-cortical loop is indicated by green lines and by a green box or green text in (A, C). While the action control loop controls cognitive as well as sensorimotor processes the motor feedback loop only acts on the sensorimotor components of the neural network. The bidirectional dorsal pathway (B) connects areas of the posterior superior temporal gyrus pSTG with two main areas in the frontal lobe, i.e., premotor cortex PMC and posterior inferior frontal gyrus pIFG. The bidirectional ventral pathway (B) connects areas of pIFG with two areas in the temporal gyrus, i.e., with anterior up to posterior regions of the STG/STS (i.e., a route connecting the three levels of the mental lexicon from phonological form via lemma to concept) as well as with the anterior inferior temporal gyrus, also called ventral anterior temporal lobe (Stefaniak et al., 2020). The information given in this figure is based on Friederici (2011), Ueno et al. (2011), Roelofs (2014), Stefaniak et al. (2020), and Miller and Guenther (2021). Semantic processing [(B), see Ueno et al., 2011, and cf. combinatorial network in Hickok and Poeppel, 2007] is a part of overall cognitive processing [see (A)].
This model sketch is mainly based on two computer-implemented model approaches, i.e., on the DIVA/GODIVA approach for sensorimotor control of speech production (Guenther, 2006, 2016; Guenther et al., 2006; Bohland et al., 2010) and on the WEAVER approach for word-form encoding (Roelofs, 1992, 1997, 2014). The WEAVER model (as published by Roelofs, 2014) reflects the cognitive-linguistic part of the model sketch (Figure 1) and is based on the word production model published by Levelt et al. (1999). The DIVA/GODIVA model (as published by Miller and Guenther, 2021) reflects the sensorimotor part of the model sketch (for the differences between DIVA and GODIVA see below in this section). WEAVER as well as DIVA/GODIVA are implemented using second generation neural networks (see Appendix A). The network model developed by Kröger et al. (2016, 2020, 2022) aims for a complete representation of a speech processing network as given in Figure 1 including cognitive-linguistic and sensorimotor processing (see below) and uses a third generation neural network approach (see Appendices A–C).
The model sketch (Figure 1) comprises a mental lexicon and a mental syllabary as central knowledge and skill repositories within the cognitive-linguistic and sensorimotor part of the model (Figure 1A). The core of the mental lexicon—storing and processing cognitive speech states (concepts, lemmata, and phonological forms)—is located in the temporal lobe. Its brain locations overlap with the network part representing the auditory input states of syllables within the mental syllabary. Phonological representations as output of the mental lexicon and input for the sensorimotor part of the speech production part of the speech processing model sketch are located in the posterior part of the frontal lobe near the syllable initiation module of the speech production network.
Motor program states and somatosensory states of syllables as part of the mental syllabary are stored in the inferior parts of the frontal and parietal lobe (the transformation of phonological states into motor plans is described in detail by Bohland et al., 2010, i.e., within the GODIVA model, and this transformation is in accordance with the model concept given by Kröger et al., 2022; the processing using the mental syllabary is described in detail by DIVA model, see, e.g., Guenther et al., 2006). Speech perception is mainly located in the superior and posterior part of the temporal lobe and comprehension leads to lexical activations in the anterior part of the temporal lobe. The cortico-cortical feedback loop including basal ganglia and thalamus (action control loop) can be activated from many cortical regions and feeds neural activations back to different parts of the cortical speech processing neural network, while the cortico-cortical control loop including cerebellum via pons (motor feedback loop) is activated mainly by the production part of the sensorimotor network and feeds back its activations to this region for activating the learned auditory and somatosensory states for currently produced syllables as well as for activating motor program execution.
While imaging and lesion studies support the strong correlation between structural (anatomical) brain locations and functional aspects (functional modules) of the neural network (e.g., Batista-García-Ramó and Fernández-Verdecia, 2018; Litwińczuk et al., 2022), this does not implicate that there exists no close neighborhood or even spatial overlap of functional modules in several regions of the brain. Thus, functional deficits appearing in modules or sub-networks of the neural speech processing network cannot always be easily associated with a specific localization of dysfunctional (e.g., damaged) regions within the brain. Moreover, in the case of developmental speech and language disorders (i.e., delay of learning and storing data within the speech processing neural network; difficulties in learning), in case of speech and language disorders which result from neurodegenerative diseases, or in case of aging which may lead to (slow and limited) degeneration of the neural network, imaging studies may not indicate any specific anatomic regions or structural abnormalities which directly uncover an underlying neural deficit which probably is responsible for the occurring speech or language disorder. In all these cases specific screenings are needed in order to collect relevant behavioral data for diagnosing a speech and language disorder correctly.
It is possible to insert neural dysfunctions of any type and severity to any functional subnetwork or module (i.e., functional location) of a speech processing neural network model. Thus, a modeled neural dysfunction can be specified with respect to functional location, severity, and neural type. The functional location (i.e., a specific module or subnetwork in the model, which is affected) is correlated to a lesioned brain regions which can be identified on basis of functional imaging data, but in many cases, the identified brain areas hosting a specific sub-network, module, or buffer of the speech processing neural network are relatively broad (e.g., Golfinopoulos et al., 2010; Kearney and Guenther, 2019). The severity of a dysfunction is defined as the percentage of non-functioning neurons or of non-functioning neural connections within a module or sub-network of the neural model (e.g., Roelofs, 2014; Kröger et al., 2020). The neural type of a dysfunction separates dysfunctions of neurons (cells, specifically cell body), dysfunctions of synapses (synaptic connections), and dysfunctions of connecting pathways (axons, dendrites) within the modeled neural network (e.g., Roelofs, 2014). Moreover, some models are capable of varying concentrations of neurotransmitters like dopamine level in specific modules or subnetworks (e.g., in striatum of basal ganglia, Civier et al., 2013; Senft et al., 2016, 2018). In these models, an abnormal concentration (too low or too high) of a transmitter substance can be introduced for instantiating a further type of neural dysfunction.
As already stated above, the linguistic-cognitive part of the model sketch given in Figure 1 is mainly based on the WEAVER model and the sensorimotor part is based on the DIVA/GODIVA model.
WEAVER (Roelofs, 2014) is a second generation or node-and-link neural network (see Appendix A) consisting of seven node layers (or simply layers), separating concept level, lemma level, phonological form level, and syllable motor program level. Two input-/output-layers are labeled as lexical input and output layers and phonological forms are separated in input- and output-layers as well, while the lemma and concept level do not separate input and output forms. The syllable motor program layer in WEAVER is comparable to a motor plan level in our model sketch (Figure 1). Links are building up neural pathways for connecting different layers of the model, i.e., the layers representing concept, lemma, lexical in-/output, phoneme in-/output and motor plan level. From the functional viewpoint of neural processing these inter-layer neural connections or inter-layer links can be labeled also as neural mappings while phonemes, lemmata, and concepts are represented as neural states. Each state is represented in WEAVER by a specific node in a neural layer. Thus, this network type uses a local representation of states. The performance of the WEAVER network for simulating different types of aphasias and the temporal specification of increasing/decreasing node activation is discussed in Section 3 and in Section 5.
The DIVA/GODIVA approach differentiates 10 layers, also called neural maps in the context of this modeling approach (Guenther et al., 2006; Bohland et al., 2010; Miller and Guenther, 2021). These neural maps and their hypothetical location in the brain are discussed in Section 3 of this paper. The neural maps (i.e., initiation map, speech sound map, auditory target, state, and error map, somatosensory target, state, and error map, articulator map, and feedback control map) and the cortical mappings connecting these neural maps are displayed in Figure 1A. Here, the speech sound map is labeled motor plan map (or motor plan buffer), the feedback map is part of the mental syllabary buffer, and the articulator map is part of the motor execution buffer. These renaming of map labels in Figure 1 results from the separation of motor planning and motor programming and on quantifying motor plans and programs with respect to the concept of speech actions or articulator gestures (see Kröger et al., 2022). The functioning of the DIVA/GODIVA model and the modeling of speech disorders is discussed in Section 3 and in Section 5.
Moreover, the model sketch presented in this paper is in accordance as well with three further computational neural network models cited in this review paper (see below).
(i) The spreading activation model introduced by Dell (1986) and further developed (see Dell et al., 2007, 2013) is a three-layer second generation neural network modeling lexical processing. The three layers (phonemes, words, semantic features) are interconnected by bidirectional mappings between phoneme and word layer and between word and semantic feature layer. For each mapping, all nodes of one layer are connected with all nodes of the other layer in both directions. This allows the typical spreading of activation from one layer to another layer. The approach is mainly used for modeling aphasic speech disorders. In the later versions of the model (Dell et al., 2013) a fourth layer, i.e., an auditory input layer is added for modeling the auditory-phonetic-to-phonological conversion in a more detailed way (see Section 5).
(ii) The LICHTHEIM 2 model (Ueno et al., 2011) is based as well on a second generation neural network model and separates seven neural layers. While four of these layers are hidden layers (no specification of the type of states is needed here), whereas all other network models discussed in this paper have a specification of type of state for each layer or buffer in order to specify its layers or buffers in a functional sense as, e.g., concept, lemma, phonological form, sensory, or motor layer. The hidden layers defined in this network model are chosen with respect to neuroanatomical reasons as neural hubs within the ventral and dorsal route or speech processing. The mappings connecting all layers are bidirectional. Three of the seven layers are defined as input/output layers, i.e., an auditory input layer, a motor output layer and a semantic in-/output layer which receives semantic input information, e.g., in case of picture naming tasks and which generates semantic output information, e.g., in the case of a word comprehension task. Thus, this model can be represented by Figure 1 at least partially. It comprises an auditory input layer and a neural pathway toward the concept and semantic processing layer via temporal lobe and further to the motor plan/program layer (ventral pathway). Moreover, it comprises a neural pathway from auditory input layer to the motor plan/program layer via parietal lobe (dorsal pathway). Thus, the hidden layers of the LICHTHEIM 2 model cannot be directly associated with intermediate functional layers of our model sketch, but it can be hypothesized that the two hidden layers within the temporal lobe which are part of the ventral pathway are related to lexical processing (concept, lemma and phonological form level in Figure 1). The two further hidden layers which appear in LICHTHEIM 2—one of them within the dorsal route and located in the parietal lobe, directly connecting auditory input and the motor domain and the other located in the ventral route connecting layers of the temporal land frontal lobe and located in the opercularis-triangularis—are not easily interpretable in our model sketch.
(iii) The ACT-model in its current state (speech action model, ACT, Kröger et al., 2016, 2020, 2022) is probably most exactly represented in Figure 1 (for its neurocomputational realization see Appendix C). This model uses the spiking neural network approach developed in the NEF-SPA framework (Neural Engineering Framework, NEF, augmented by and Semantic Pointer Architecture, see Appendix B) and it is capable of representing and processing cognitive states, i.e., concept states, lemma states, and phonological form states within the perception pathway and within the production pathway of the mental lexicon (see Figure 1A) as well as sensorimotor states, i.e., motor plan states and motor program states within the further (lower) production pathway and sensorimotor and auditory states within the feedback perception pathway. Cognitive-linguistic states are hosted in cognitive-linguistic state buffers or SPA-buffers (see Figure 1A; Kröger et al., 2020; Appendix B). Higher-level motor states are hosted in the motor plan and motor program buffers (also SPA-buffers, see Kröger et al., 2022). Lower-level motor states (i.e., syllable oscillators and gesture movement trajectory estimators) are hosted in lower-level state buffers, called neuron ensembles or NEF-ensembles.
So far, the cognitive-linguistic part as well as the production-side of the sensorimotor part of the model sketch (Figure 1) are computer-implemented now by using a spiking neuron approach (Kröger et al., 2016, 2020, 2022; see also Appendix C). The feedback loop of the sensorimotor part of the model sketch has been implemented beside DIVA in a spatio-temporal activation averaging model (STAA model or second generation neural network model, Kröger et al., 2014; Kröger and Cao, 2015; Kröger and Bekolay, 2019, p. 133ff, while spiking neuron or spiking neural networks i.e., SNN's, are also called third generation neural network approaches, see Maass, 1997).
3. Anatomical locations of modules or sub-networks
Computer-implemented neural network models are simulating neural functionality. These models clearly define subnetworks which are responsible for specific functional subtasks, e.g., for selecting and activating a concept, lemma, or phonological form, or for activating a stored syllable motor plan etc. Imaging techniques allow to specify exactly those brain regions which are activated if a specific functional task is performed and thus allow to associate neural functionality and brain areas. Indefrey and Levelt (2004) and Roelofs (2014) assume that concepts which are stored in the mental lexicon are represented in anterior-ventral temporal cortex, lemmas in the mid-section of the left middle temporal gyrus, input and output lexical forms of lemmas as well as input phonemes in left posterior superior and middle temporal gyrus (Wernicke's area), while output phonemes are stored in left posterior inferior frontal gyrus (Broca's area). Syllable motor representations which are stored in the mental syllabary are represented in ventral precentral gyrus. Inter-lobe neural associations appear especially between phonological input and output forms located in part in the temporal lobe and in part in the frontal lobe (see Figure 1B). These associations are structurally realized by left arcuate fasciculus and uncinate fasciculus.
Guenther (2006), Guenther et al. (2006), Golfinopoulos et al. (2010), and Kearney and Guenther (2019) assume that the initiation map, which activates motor plans and motor programs and thus starts syllable execution as postulated in the DIVA and GODIVA models (Guenther, 2006; Bohland et al., 2010), is located in the supplementary motor area, on the medial wall of the frontal cortex. The speech sound map (a term used in DIVA and GODIVA models and represented by mental syllabary in our model sketch, Figure 1A) is assumed to be located in left ventral premotor cortex, i.e., in the ventral precentral gyrus and in the surrounding portions of posterior inferior frontal gyrus and of the anterior insula.
The articulation map (execution map in model sketch, Figure 1A) which directly activates motor neurons controlling the movements of the speech articulators is located within the ventral motor cortex (primary motor cortex). Neural buffers hosting the auditory target, state, and error maps are located within the ventral auditory cortex (temporal lobe), and those hosting the somatosensory target, state, and error maps are located in the ventral somatosensory cortex (parietal lobe). The detailed organization and functioning of the feedforward and feedback motor control system within the sensorimotor part of the model sketch using these maps is described by Guenther (2006) and Kearney and Guenther (2019) and in the context of our model sketch it is described by Kröger et al. (2020, 2022).
Two cortico-cortical feedback loops (action control loop and motor feedback loop) including basal ganglia, cerebellum, and thalamus are introduced in the model sketch (Figure 1A). The action control loop (see orange arrows, orange box, and orange text in Figures 1A, C) is responsible for all cognitive control processes needed for temporal sequencing of cognitive and sensorimotor processes in each situation, e.g., paying attention to specific incoming sensory information, deciding how to react in a specific situation, and activating motor processes for reacting. In case of a speech task like picture naming this can be the sequence of visual perception (activation of visual state), word recognition (activation of a concept from mental lexicon), and word production (phonological form activation, Kröger et al., 2016, 2020). Moreover, action control as well comprises motor planning in form of motor plan and motor program activation and motor execution (see planning and motor loop in Bohland et al., 2010 and in Miller and Guenther, 2021). This control loop starts and ends in different areas of the neocortex and includes the basal ganglia and thalamus in its center (see Figures 1B, C, solid orange lines). A second feedback loop, here called motor feedback loop (called cerebellar loop in Kearney and Guenther, 2019; green arrows and boxes in Figure 1A and green lines and green text in Figure 1C) is responsible for activating feedback control processes comparing stored and current auditory and somatosensory states and thus in part acts on syllable execution as well. This loop is activated together with current target states at mental syllabary, comprises cerebellum and thalamus in its center, and allows correction of sensory feedback states as well as of motor program states (see Figures 1A, C, green lines).
While most approaches discussed above can be represented by functionally defined box-and-arrow models, i.e., can be represented by box-and-arrow plots in which the boxes or modules define partial functions of speech processing like semantic-to-lemma or lemma-to-phonological form transformation (see, e.g., Roelofs, 2014), the LICHTHEIM 2 approach (Ueno et al., 2011) can be represented by a neuroanatomically defined box-and-arrow model. Here, the architecture of the network and thus the modules of the network are defined with respect to the neuroanatomy of the brain, but it should be kept in mind that these brain regions are mainly defined based on knowledge gathered from functional imaging experiments, so that these regions as well can be separated on the basis of neurofunctionality.
The LICHTHEIM 2 model is based on a second generation neural network model and separates seven neural layers representing different cortical areas. The model (i) connects the auditory input layer (mid-superior temporal gyrus/sulcus, mSTG, mSTS) with the semantic layer (anterior STG/STS) by two intermediate or hidden layers within the temporal lobe via the ventral pathway, (ii) connects the semantic layer with the motor output layer (insular motor cortex) via two intermediate or hidden layers within the temporal and frontal lobe as a further part of the ventral pathway (main part of the ventral pathway), and (iii) connects the auditory input layer and motor output layer via one further intermediate or hidden layer within the parietal lobe (i.e., a hidden layer within the inferior supramarginal gyrus, inf SMG) by the dorsal pathway.
Within the functional part of our model sketch (Figure 1A) a part of the ventral route located in the temporal lobe and the further dorsal route (both routes as defined by Ueno et al., 2011) can be interpreted as the production pathway (our model sketch, Figure 1A) leading from semantic processing via lexical processing (from the concept buffer via lemma, to phonological form buffer), toward motor processing (pathway from motor via and program buffers toward motor execution). In the other (i.e., in the perceptual) direction (the perception pathway in our model sketch), the dorsal pathway (from frontal via parietal toward temporal lobe as defined by Ueno et al., 2011) can be interpreted as sensory feedback processing pathway including somatosensory and auditory state, target, and error buffers (Figure 1A), while the part of the ventral pathway located in the temporal lobe (as defined by Ueno et al., 2011) as well realizes perceptual lexical processing (i.e., comprehension).
In contrast to all other neurocomputational models and as stated above, the LICHTHEIM 2 approach does not specify the intermediate or hidden layers with respect to sensorimotor or linguistic functions (e.g., phonological, higher level auditory, or higher-level somatosensory representations) and thus can be interpreted as a basically neuroanatomical approach. Only the input- and output layers are defined concerning functions, i.e., the semantic layer for activation of word meanings, the acoustic input layer for activation of phonetic-acoustic features and the motor output layer for the activation of motor programs. Thus, the model can be interpreted as well as an early version of deep learning networks (for an overview on deep learning neural networks in speech processing see, e.g., Nassif et al., 2019; Roger et al., 2022). A further hidden layer neural network model for speech processing including the ventral visual pathway but omitting a part of the speech processing ventral pathway (connecting the anterior part of the temporal lobe directly with the inferior part of the frontal lobe) has been developed by Weems and Reggia (2006).
4. Disorders: symptoms, types of dysfunctions, lesioned brain regions
4.1. Aphasia
Aphasias are characterized by a loss of language knowledge and language skills. Production and/or comprehension of words or entire sentences can be disrupted. The cause is damage of parts of the central nervous system, e.g., after stroke or traumatic brain injury (acute-onset type aphasias), or the cause is a progressive neurodegenerative disease. The acute-onset type aphasias can be subdivided in Broca aphasia as a disturbance in speech production, Wernicke aphasia as a disturbance in speech comprehension, and global aphasia, as a mixture of both.
Other types of aphasia are conduction aphasia and transcortical aphasia. In case of conduction aphasia, both, speech production and speech comprehension are widely unaffected, but patients have difficulties to repeat unfamiliar words as well as non-words (logatomes). Transcortical aphasias can be subdivided in three types. In the case of transcortical motor aphasia, the initiation of learned words (stored in mental lexicon) is affected (e.g., picture naming is difficult), but the patient still can repeat words or syllables (direct repetition of auditory stimuli). In the case of transcortical sensory aphasia, repeating of (auditory presented) syllables, words, or phrases is also possible but without understanding the words or the meaning of the entire utterance. In the case of transcortical mixed aphasia, words can only be understood to a limited extent and can only be produced to a limited extent in a picture naming or storytelling scenario, but they can be imitated perfectly.
All these subtypes of aphasia can more easily be understood and differentiated based on a definition of the functional deficits in the neuronal network model sketch (Figure 1A), i.e., can be understood and can be differentiated based on the definition of model dysfunctions as described in Table 1. Thus, in case of Broca aphasia mainly the phonological input buffer, in case of Wernicke aphasia, mainly the phonological output buffer and in case of global aphasia, mainly the phonological in and output buffer is dysfunctional (see Section 5).
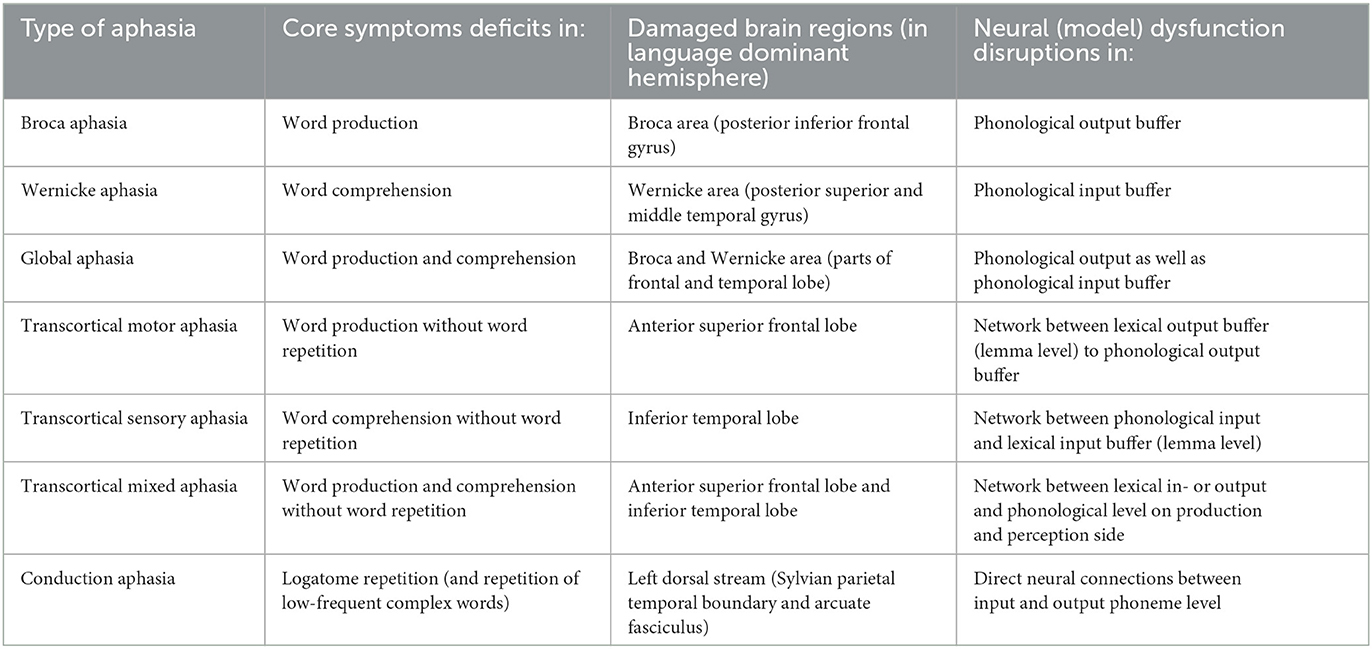
Table 1. Subtypes of aphasias, core symptoms, affected brain regions, and model dysfunctions following Roelofs (2014).
In case of the transcortical aphasias the neural connections from/toward phonological buffers are dysfunctional (i.e., dysfunctions in connecting lemma and concept level with the phonological input level of the mental lexicon in case of transcortical sensory aphasia and dysfunctions in connecting these lexical levels with phonological output level in case of transcortical motor aphasia) and in case of conduction aphasia the shortcut connection between phonological in- and output buffer is dysfunctional. Here input buffers describe the buffers on the perception pathway while output buffers describe the production pathway in Figure 1A. Modeling of these subtypes of aphasia has been demonstrated successfully by Roelofs (2014) and Kröger et al. (2020) as is described in Section 5 (modeling of symptoms). These explanations in terms of our functional model sketch (Figure 1) are also in accordance with the simulation results given by LICHTHEIM 2 model (Ueno et al., 2011).
These neural dysfunctions named above in case of several subtypes of aphasia refer to our functional model sketch (Figure 1A) but are also in accordance with the simulation results from the LICHTHEIM 2 model (Ueno et al., 2011). Here, three subtypes of conduction aphasia can be differentiated with respect to neural dysfunctions within two different cortical locations, i.e., inferior supramarginal gyrus iSMG and insular motor cortex, and with respect to the dorsal pathway connecting these two cortical areas. These locations and pathways are part of the dorsal route of speech processing and thus are independent from lexical processing which is activated via the ventral processing route (Ueno et al., 2011). Broca-Aphasia here results from neural dysfunctions within the frontal operculum and of the anterior inferior frontal lobe aIFL (Stefaniak et al., 2020) also hosting the phonological output-buffer in terms of our model sketch (Figure 1B). Wernicke-type aphasia is here analyzed as neural dysfunction appearing within the auditory input layer, but it should be kept in mind that the corresponding layers for representing and processing auditory input and its phonological interpretation are located nearby in the posterior part of the superior temporal gyrus pSTG. One further very interesting feature of the LICHTHEIM 2 model is that this model can simulate post-stroke recovery phenomena in case of different types of aphasia (Stefaniak et al., 2020 and see Section 5).
4.2. Apraxia of speech
Apraxia of speech (AOS) can be defined as a dysfunction of speech motor planning and/or speech motor programming. It is a neurogenic speech disorder which can be acquired (result of stroke, traumatic brain injury), which can have its origin in a neurodegenerative disorder (primary progressive apraxia of speech), or which can have its origin in developmental problems (childhood apraxia of speech). On the one hand, apraxia of speech does not involve the cognitive-linguistic part of the speech processing system. Thus, the patient is aware of his self-produced speech errors. On the other hand, the (peripheral) neuro-muscular system and the articulation apparatus including all speech articulators is intact as well. The affected modules are the planning and programming components in connection with parts of the mental syllabary, i.e., the central model parts of the sensorimotor part of the speech processing model in terms of our model sketch (Figure 1A, see also Van der Merwe, 2021). The core symptoms, damaged brain regions, and the model dysfunctions arising in the case of apraxia of speech are listed in Table 2.
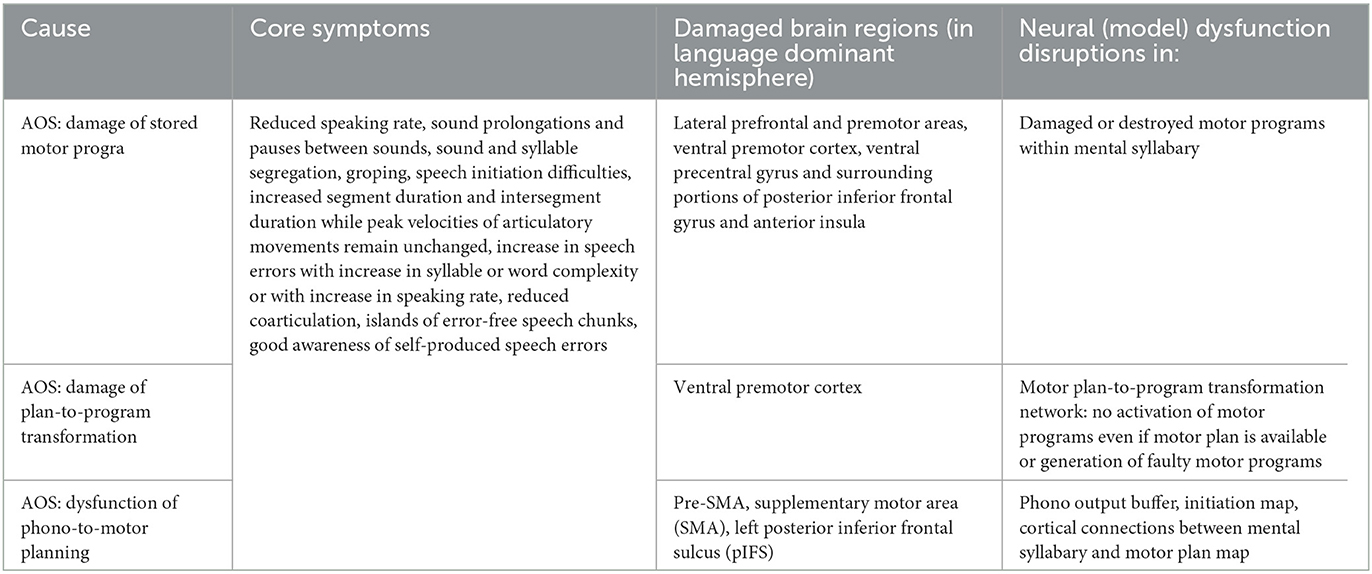
Table 2. Core symptoms, affected brain regions, and neural model dysfunctions for apraxia of speech (see Miller and Guenther, 2021; Van der Merwe, 2021).
Three main neurofunctional causes are discussed in case of AOS following Miller and Guenther (2021): (a) damage of pre-learned motor programs, stored in mentally syllabary; (b) damage in the motor plan-to-motor program transformation network (activation of a motor program if a motor plan is already activated or assembling a motor program if a motor plan is not available or only partially available); (c) dysfunction of phonological sequence-to-motor plan selection (selection of a motor plan from the continuous flow of phonological sound sequences during production process). This separation of causes is based on assumptions by considering the box-and-arrow-version of the GODIVA model (see Figures 1, 2 in Miller and Guenther, 2021, pp. 430f) but it should be noticed that these three causes do not lead to a separation of symptoms (see Table 2). Simulations of word and phrase production based on GODIVA model versions including these neural dysfunctions still need to be realized in order to simulate the symptoms listed in Table 2.
An illustration for incorrect motor plan to program transformation (i.e., motor plan realization) is the occurrence of incorrectly timed speech gestures within a syllable, i.e., the incorrect temporal coordination of gestures appearing within the motor plans of syllables (for motor plan realizations as gesture scores, see Kröger and Bekolay, 2019; Kröger et al., 2020, 2022). For example, a mistiming between a labial closing gesture and a glottal opening gesture producing the speech segment [p] may lead to the impression that the speaker produces a [p] OR a [b], i.e., the listener sometimes perceives a voiced and sometimes a voiceless segment even though the glottal opening gesture is present in both cases. The incorrect plan-to-program transformation here leads to a faulty shift of the glottal gesture toward earlier points in time which leads to the perceptual impression of a voiced version of this segment because the glottal gesture is more and more hidden behind the labial closure [see Figure 2; the transition of glottal gesture to the left side from (A) to (C)]. If the glottal gesture is produced even more early in comparison to the labial closing gesture, we even can get the effect of pre-aspiration [hb] which as well can be observed as a symptom of speakers, suffering from apraxia of speech (Figure 2D, and see Kröger, 2021). Thus, these speakers may be able to produce four variants of the segment, i.e., [hb] -> [b] -> [p] -> [ph] just by shifting the glottal opening gesture from “early” to “late” with respect to the labial closing gesture.
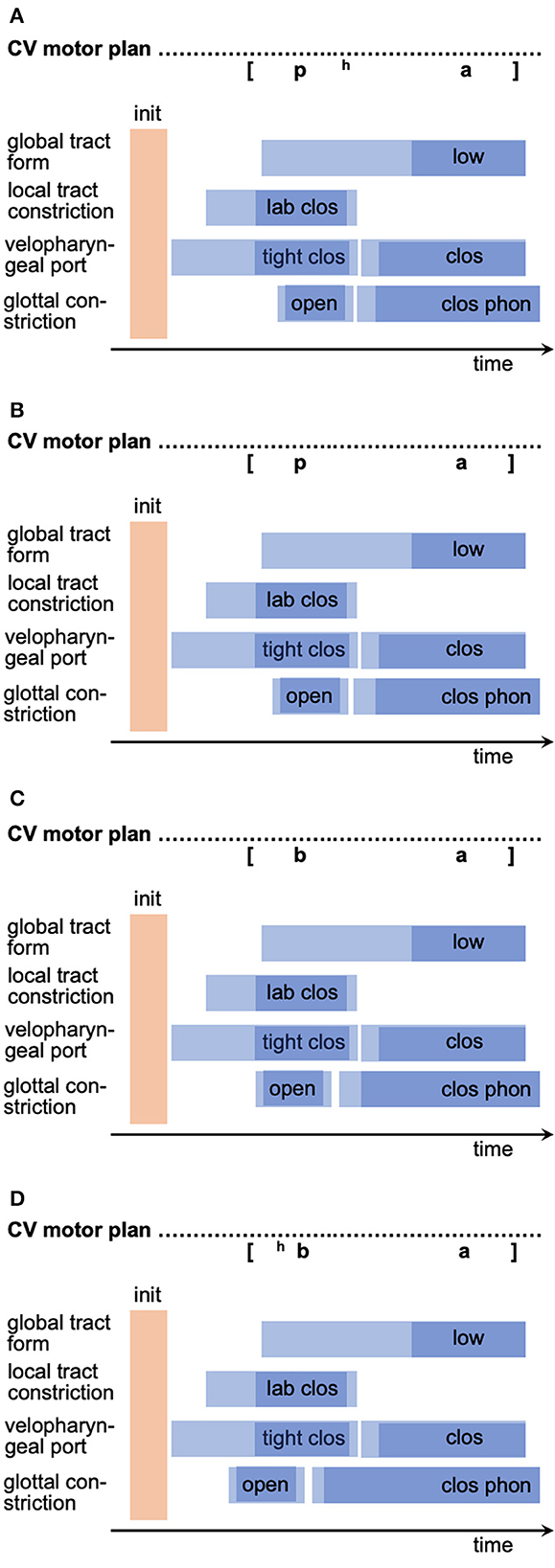
Figure 2. Four motor plans (gesture score notation, see Kröger and Bekolay, 2019; Kröger et al., 2020, 2022) of CV-syllables with variation of temporal location of glottal opening gesture relative to labial closing gesture toward earlier points in time from (A–D). Phonetic transcription of the auditory impression of the syllables is notated above each motor plan. Blue rectangles indicate the temporal duration of a gesture (vocalic tongue lowering gesture producing an/a/, labial closing gesture, velopharyngeal tight closing gestures (needed for realization of obstruents) and closing gestures (needed for realization of all other non-nasal speech sounds), glottal opening gestures (producing voiceless sound, if timed correctly) and closing gesture (producing phonation). Light blue portions indicate movement parts of a gesture; dark blue portions indicate time intervals, in which the gesture target is reached (see Kröger et al., 2022).
4.3. Dysarthria
Dysarthria is caused by neural dysfunctions appearing in the neuromuscular system as well as in both cortico-cortical feedback loops including basal ganglia or cerebellum. Several types of dysarthria can be differentiated. To understand the neurofunctional background of different types of dysarthria a detailed understanding of the organization of the whole sensorimotor part of the speech processing system including its frontend, i.e., including the neuromuscular system, which is activated during motor execution, is needed. Kearney and Guenther (2019) give an overview concerning the influence of the cortico-cortical feedback loop via the basal ganglia (BG) and thalamus (action control loop) and the feedback loop via the cerebellum and thalamus (motor feedback loop) on these neurogenic speech disorders.
The neurogenic speech disorder associated with damage in the cerebellum is ataxic dysarthria. Here, the damage of parts of the cerebellum which can be caused, e.g., by traumata or by vascular diseases, leads to disturbances in the interaction of feedforward motor signals and feedback sensory signals caused by the motor feedback loop (Kearney and Guenther, 2019; green arrows in Figure 1A). Thus, dysfunction within the motor feedback loop lead to deficits in generating precisely timed control commands and thus to deficits in the direct online control of articulation (ibid., and see Table 3).
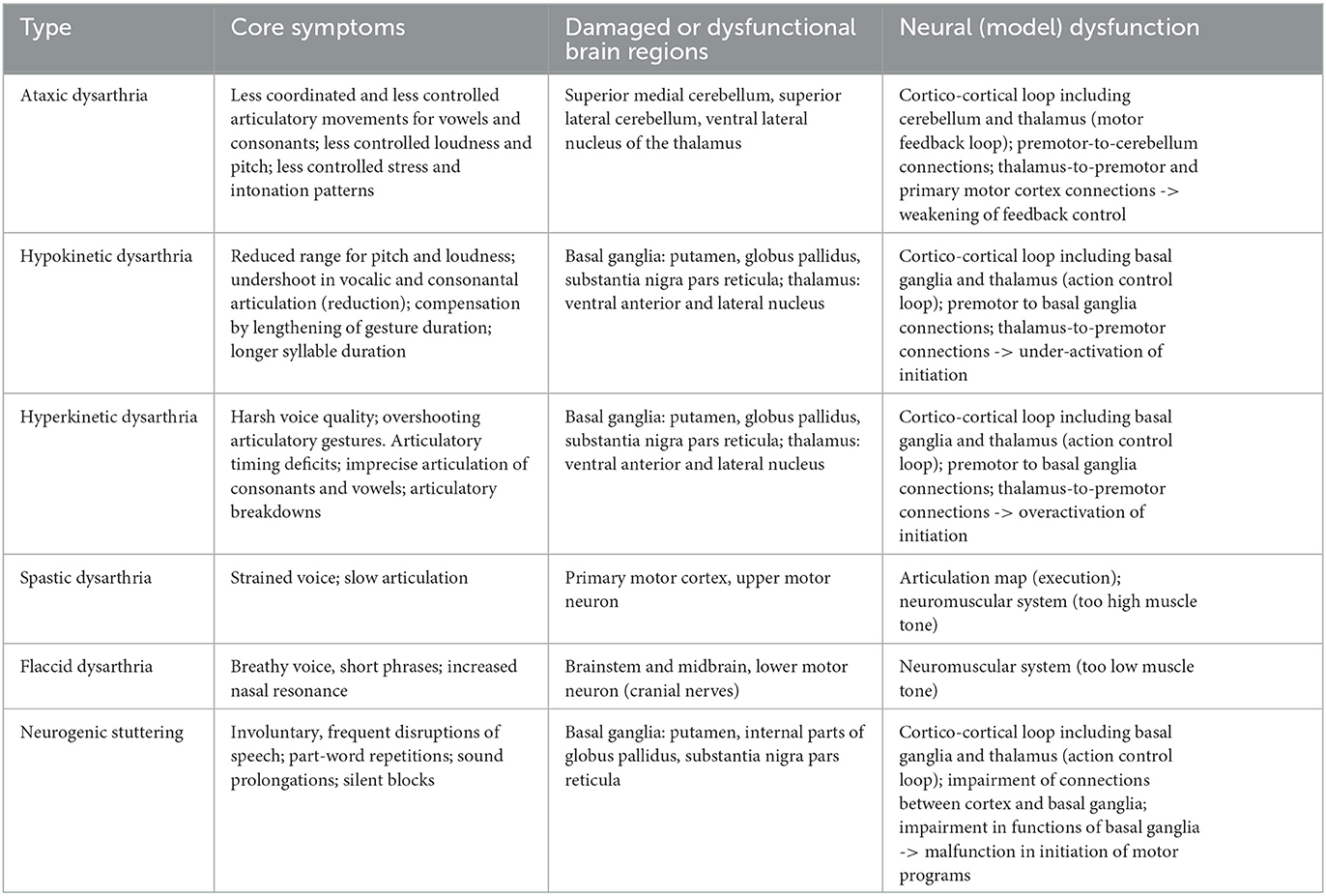
Table 3. Core symptoms, affected brain regions, and neural model dysfunctions for different subtypes of dysarthria and for neurogenic stuttering (see Golfinopoulos et al., 2010; Kearney and Guenther, 2019; Chang and Guenther, 2020; Miller and Guenther, 2021).
The dysfunctions occurring in the action control loop (basal ganglia and thalamus) lead to hypokinetic or hyperkinetic dysarthria. These dysfunctions can be caused by neurodegenerative diseases such as Parkinson's disease (reduction in the functionality of the striatum as part of the basal ganglia due to the reduction of dopamine) or Huntington's disease (damage of brain cells because of gene mutation, neural cell damage in striatum and later in cortical neural cells). This results in a malfunction of the whole action control loop which in case of speech production leads to under- or overactivation of states within the initiation map, motor program map and motor execution map (see orange arrows within the sensorimotor part of the model sketch displayed in Figure 1A; the motor execution map is called articulation map in DIVA/GODIVA model) and thus to under- or overactivation of (mainly syllabic) motor plans and motor programs which subsequently influences the correctness of the appearance of all speech gestures within each syllable and which as well leads to an incorrect timing of whole syllables.
Underactivation of the neural states mentioned above occurs in hypokinetic dysarthria and leads to symptoms like reduction in articulatory movements and decrease in pitch and loudness range (see Table 3). Moreover, underactivation of neural states within initiation map leads to weakening of motor plan activation and thus leads to longer syllable durations and to slowing down articulatory movements. Overactivation of states within the initiation map leads to neural overactivation at the motor plan and motor program level and may be the source for neural malfunction occurring in hyperkinetic dysarthria. Overactivation of motor plans and programs and thus of articulatory gestures leads to harsh vowel quality and overshooting articulatory gestures. Overshoot destroys the timing of gestures as defined in the motor plan and may lead to imprecise articulation of consonants and distorted vowels, and—if gesture activation does not stop—to irregular articulatory breakdowns.
Spastic dysarthria is caused by an impairment of the upper motor neurons located in the primary motor cortex while flaccid dysarthria is caused by an impairment of the lower motor neurons located in the midbrain and in the brain stem. Thus, both subtypes of dysarthria can be labeled as impairments of the neuromuscular system. Patient suffering from flaccid dysarthria show symptoms like breathy voice resulting from insufficient glottal closing gestures, short phrases resulting from too short activation of all gestures within an utterance and increased nasal resonance resulting from imperfect closure of the velopharyngeal port and imprecise articulation (Kröger, 2022). These symptoms can easily be attributed to a reduced muscle tension (too low muscle tonus) and reduced duration of all muscle activations. Spastic dysarthria leads to symptoms like strained voice and slow articulation (ibid.). This behavior typically results from a too high muscle tonus and too long activation of muscle actions. In this case it is difficult to complete speech gestures in normal time intervals as pre-specified by the motor programs of syllables. Detailed simulations of these symptoms need the implementation of more detailed neuromuscular systems as part of articulatory models within the entire neural speech processing model (see Section 7).
4.4. Neurogenic stuttering
Stuttering can appear after stroke, or as a comorbidity of neurological diseases. But in most cases stuttering is a developmental disorder typically emerging at 2–5 years of age in about 3%−8% of preschool-aged children (Chang and Guenther, 2020). Developmental stuttering resoles without treatment within 2 years in 75% of cases (ibid.). Core symptoms of stuttering are involuntary, frequent disruptions during ongoing speech such as part-word repetitions, sound prolongations, and silent blocks, which interrupt fluent speech (Chang and Guenther, 2020). Because many functional causes are discussed in case of stuttering, we will concentrate here on dysfunctions of the neural system and thus we label the disorder discussed here neurogenic stuttering.
Civier et al. (2013) and Chang and Guenther (2020) claim that the functional deficit underlying neurogenic stuttering is due to a malfunction within the cortico-cortical feedback loop including basal ganglia and thalamus (action control loop). One of the responsibilities of this cortico-cortical loop is to initiate the execution of speech motor programs. If this initiation process is not working in a proper way this may cause interruptions by blocking the production and execution of a syllable or by blocking the production and execution of a whole utterance directly at its beginning. Civier et al. (2013) simulated the production of syllable sequences using the GODIVA model by impairing (i) the projections between cortical regions and input regions of the basal ganglia, i.e., the cortical input projections toward the basal ganglia, labeled as white matter abnormalities (ibid.) and by impairing (ii) the striatum as part of the basal ganglia by reducing the dopamine level within that part of the model. The performed simulations show typical dysfluency symptoms like part-word or syllable repetitions, sound prolongations, and silent blocks, which all interrupt fluent speech. Similar results were reported by reduction of the dopamine level in the striatum of basal ganglia using a spiking neuron model (Senft et al., 2016, 2018).
5. Simulation of symptoms
The main questions which will be answered in this chapter for each existing computer-implemented neural model is: Which speech and language disorder is intended to be simulated with this dysfunctional model? Which dysfunctions (type and location) are inserted in that model to simulate these speech and language disorders? Which simulation scenarios (screening tasks) were simulated using that dysfunctional model in order to simulate all typical symptoms of the targeted speech and language disorder?
Five main groups of computer-implemented neural models will be discussed here, i.e., WEAVER, Dell's spreading activation model, LICHTHEIM 2, DIVA/GODIVA and ACT. The WEAVER model of Roelofs (2014) as well as the spreading activation model of Dell et al. (2013) are second generation network models (see Appendix A) comprising semantic, lemma, and phoneme layers (comparable to neural maps in DIVA/GODIVA and to buffers in ACT) of nodes (comparable cells in DIVA/GODIVA and to neuron ensembles in ACT) connected by bidirectional inter-layer links or inter-layer neural pathways (representing neural connections). While the terms layers, nodes, links are defining second generation neural network approaches (using spatio-temporal activation averaging, see Kröger and Bekolay, 2019, p. 133ff and see Appendix A) the terms buffers, cells and synaptic connections are used in adaptive neural network approaches (DIVA: Guenther, 2006, 2016; Guenther et al., 2006, GODIVA: Bohland et al., 2010) and in third generation neural network approaches also called spiking neuron models (Kröger et al., 2016, 2020, 2022; Senft et al., 2016, 2018; Stille et al., 2020; also labeled as ACT model, see Kröger et al., 2012; and see Appendices A, B).
The WEAVER model (Roelofs, 2014) can simulate cognitive-linguistic production and perception/comprehension processes and is able to generate typical speech symptoms appearing in different forms of aphasia, i.e., Broca's, Wernicke's, conduction, transcortical motor, transcortical sensory, and mixed transcortical aphasia. These symptoms comprise production or comprehension of a wrong word, a complete failure of word production, or in case of nonwords (meaningless syllables or syllable sequences) the production of no or of a wrong or reduced sequence of speech sounds. These symptoms typically appear in question-answering scenarios and are tried to be evoked in medical screenings (questions by test supervisor; answers by patient) comprising picture naming, word repetition, word comprehension, or logatome (i.e., nonword) repetition tasks (see introduction for the definition of these tasks and see Table 4). The LICHTHEIM 2 approach (Ueno et al., 2011) can simulate different types of conduction aphasia, Broca- and Wernicke aphasia by simulating the same types of tests, i.e., naming, word comprehension and word and logatome repetition. The brain lesions inserted in this model are not primarily functional but defined from cortical locations but can be interpreted in a functional way as is explained already in Section 3.
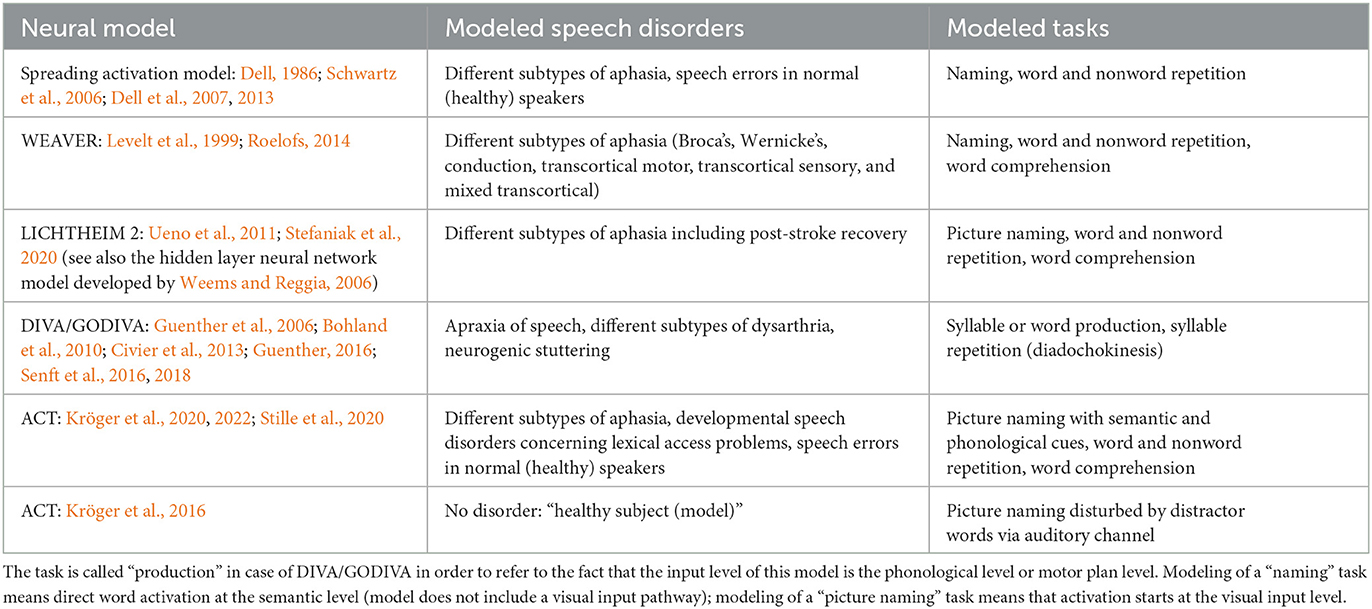
Table 4. Speech disorders and listing of tasks for the generation of associated speech errors already simulated by using different computer-implemented quantitative neural models of speech processing.
To evoke these symptoms by simulation, two neural types of dysfunctions can be chosen in WEAVER (ibid.). (i) Reduction in strength of neural activation appearing in the nodes because of a specific percentage of inactive or dead neurons or (ii) reduction in strength of neural activation forwarded in synaptic connections between neurons which results from a specific percentage of inactive or dead synaptic connections or links. These types of model dysfunctions can be inserted in neuron buffers at concept, lemma, or phonological form levels on the perception or production pathway or can be inserted in the neural connections between these buffers within the production or perception pathway (see Figure 1). The severity of a dysfunction is modeled in WEAVER by (i) the parameter decay rate affecting the nodes (i.e., the model layers), and by (ii) the parameter connection weight affecting the links (i.e., the connections between layers). Thus, the stronger the decay rate or the lower the connection weight the higher the number of damaged nodes or links and the higher the rate of symptoms produced in simulated speech tasks.
A comprehensive description of typical simulations for generating symptoms in different forms of aphasia using the WEAVER model is given by Roelofs (2014). The neural dysfunctions corresponding to different forms of aphasia are inserted at the phonological, the lemma, and the concept in- and output layers in form of increase in decay rate which models an increasing number of dysfunctional neurons within these layers. Furthermore, neural dysfunctions are inserted in form of decrease in connection weight for modeling an increasing number of dysfunctional neural connections between layers (see chapter 2 of this paper and see ibid., p. 37f). Three types of tests were simulated. (i) Word production is simulated by introducing neural activation at the phonological input layer representing a phonological word form of a specific target word and by evaluating whether the correct activation appears at the syllable nodes below the phonological output layer (i.e., motor plan nodes in Figure 1). (ii) Word comprehension is simulated in the same way concerning target word activation but here, the activation at the concept level layer is evaluated. (iii) Logatome repetition is simulated in the same way as word production but here instead of target words target syllables are activated for which no corresponding lemma and concept exists (i.e., phonologically well-formed syllables without word meaning in the target language). If logatomes are not part of the model vocabulary, it is possible to simulate logatome repletion by using words or syllables of the target language if the neural connection between phonological form level and lemma level is interrupted in the model at the perception side in order to avoid a coactivation of word forms and concepts.
Simulation results of these types of tests are interpreted as an error if no neural activation arises at the concept level (comprehension test) or at the motor plan level (word production and logatome repetition test) or if the occurring neural activation pattern represents a wrong word. The results from this interpretation of simulated errors indicate for all types of aphasia that the error rate increases with increasing neural damage of layers or neural connections at least for one of the three tests. Thus, a strong error rate appears for the word production and repetition test in case of neural damage within the phonological output layer (Broca aphasia), a strong increase in error rate appears for word comprehension and repetition in case of neural damage within the phonological input layer (Wernicke aphasia), a strong increase in error rate appears for logatome repetition in case of neural damage within the neural pathway between input and output phonological layer (conduction aphasia), a strong increase in error rate appears for word production only in case of neural damage within the neural pathway between lemma and output phonological layer (transcortical motor aphasia), a strong increase in error rate appears for word comprehension only in case of neural damage within the neural pathway between input phonological and lemma layer (transcortical sensory aphasia), and a strong increase in error rate appears for word production and comprehension in case of neural damage within the neural pathway between lemma buffers and concept layers within input or perception and within production or output pathway (mixed aphasia; see ibid, Figure 2 on p. 38).
Dell (1986) introduces a weight-decay approach implemented as dysfunction-inserting approach in his spreading activation model which represents a network-wide reduction in connection weight (weight parameter affecting links and thus the connections between layers) combined with a decrease or increase in activation-decay rate (decay parameter affecting activation of nodes within a layer). In later versions of the spreading activation model the weight parameter is split into separate semantic (s-weight) and phonological (p-weight) weights representing different functional locations of dysfunctions (between semantic and word layer and between word and phoneme layer), while the activation decay in nodes cannot be changed. The continuity thesis (Schwartz et al., 2006, p. 232) implies that an increase/decrease, i.e., the strength of each of these parameters changes the model performance from normal to random (i.e., toward incorrect or abnormal behavior) while the quotient of strength of these parameters, i.e., the degree of dominance of one of these parameters, characterizes the type of disorder. An auditory input layer and a further weight parameter (nl-weight) is introduced by Dell et al. (2013) which describes dysfunctions between the auditory input layer (added in this new model variant) and phoneme layer and which in addition allows the modeling of auditory input disorders. The tasks simulated by spreading activation models were (picture) naming by inserting primary or input neural activation at the semantic layer, and word and nonword repetition by inserting neural activation at the auditory layer of the model (see Table 4). Furthermore, Dell et al. (2013) introduces a concept which allows an association of cortical locations of brain lesions and model dysfunctions by associating model and patient behavioral data. The simulation results are comparable with those generated by the WEAVER model (Roelofs, 2014).
In the LICHTHEIM 2 model (Ueno et al., 2011) brain lesions are defined from a neuroanatomical viewpoint as white matter damage and gray matter damage. Gray matter damage is modeled here as an insertion of white noise to the activation pattern of nodes within a specific network layer (damage within a specific layer) while white matter damage is modeled as a partial removal of neural links of a neural pathway interconnecting two neighboring neural layers of the network model (damage of a specific neural pathway). Both types of damage are applied in combination with increasing severity leading to an increasing number of speech errors appearing in the simulated naming, word comprehension, and word and logatome repetition tasks.
Because the LICHTHEIM 2 model is capable of modeling lexical relearning by applying a set of training stimuli in form of auditory and related motor activation patterns to the auditory and motor layer and by adjusting synaptic connections of the hidden layers along the lexical route within the temporal lobe, this model is capable of simulating post-stroke neural recovery via the ventral route if the dorsal route is damaged (see Stefaniak et al., 2020, p. 47ff). This relearning (readjustment of synaptic link weights) applied to the model can be interpreted as a post-stroke learning or recovery.
The adaptive neural production models DIVA and GODIVA developed by Guenther (2006, 2016), Guenther et al. (2006), and Bohland et al. (2010) simulate neural processes of speech learning (early phases of speech acquisition, i.e., babbling and imitation) and neural processes of feedback-controlled speech production (sensorimotor part of the speech production model; feedforward and feedback control). These models comprise motor planning (i.e., selection of executable chunks at the phonological level), motor program selection, and motor program execution. The DIVA/GODIVA model components (modules or subnetworks) are associated with specific cortical as well as subcortical brain regions (Kearney and Guenther, 2019, and see above, chapter “anatomical locations”) and several modules or subnetworks can be identified in the DIVA/GODIVA models which cause symptoms of dysarthria or apraxia of speech (Kearney and Guenther, 2019, pp. 11ff; Miller and Guenther, 2021, pp. 432ff, and see above, chapter “disorders”). A concrete simulation study using the GODIVA model has been performed for simulation neurogenic stuttering (Civier et al., 2013, Table 3). Model parameters characterizing neural dysfunctions were (i) number of defective cells within distinct cortical or subcortical modules, (ii) number of defective neural connection weights between cells of distinct modules of the model, and (iii) change in dopamine level within striatal component of the modeled basal ganglia module of the model. Typical symptoms which can be simulated using this model by performing a word production task are (i) slower initiation of execution of a motor program (ibid., p. 272), leading to prolongation of preceding syllables as well as to silent blocks.
The spiking neuron model developed by Kröger et al. (2016, 2020, 2022), also called ACT model (Kröger et al., 2012) is capable of simulating speech errors as produced by normal speaking subjects (picture naming task without and with auditory distractor signals, Kröger et al., 2016, see also Table 4), is capable of simulating speech errors produced by speakers suffering from different forms of aphasia (word comprehension tasks and word and nonword production tasks, Kröger et al., 2020, p. 13f) and by subjects suffering from developmental (neurogenic) speech deficits concerning lexical access (picture naming tasks with auditorily presented phonological or semantic cues, Kröger et al., 2020, p. 14f), and is capable of simulating symptoms of neurogenic stuttering which result from changes in dopamine level within the basal ganglia (syllable repetition tasks, Senft et al., 2016).
In case of simulating different forms of aphasia the same types of simulations are used here for ACT as described above for the WEAVER model, i.e., simulations of word production, word comprehension and of logatome repetition. The same buffers and neural pathways are disturbed in the ACT model as already described above for the WEAVER model. But in the ACT model we are able to directly deactivate a specific number of neurons in the phonological form, lemma, and concept buffers. And in case of the neural pathways we are apbel to directly deactivate of a specific number of neurons within the associative memories because in ACT we have a direct modeling of neurons within buffers and within associative memories, while in WEAVER the neuron modeling is more indirect by using nodes and links (for the definition of nodes, links, neurons, neuron buffers and associative memories see Kröger and Bekolay, 2019, p.133ff).
Changes in dopamine level were also induced in the GODIVA model (Civier et al., 2013) which also resulted in typical symptoms of neurogenic stuttering, i.e., silent blocks and prolongation of syllables. In the ACT model used by Senft et al. (2016, 2018) changes of dopamine level (here reduction of dopamine level) led to symptoms of stuttering like omission of syllables, like errors in syllable ordering, as well as to repetitions of the same syllable. The task used here was repetition of a pre-learned sequence of syllables like [ba-da-ga] (this kind of task is also called diadochokinesis).
Simulations of a word repetition task performed with the normal model (healthy subject in the ACT model, Kröger et al., 2016) leads to speech errors (wrong word is produced or no word is produced) if the production process is distracted by placing perceptual events like distractor words during the production process of a target word within a picture naming task. Distractor words are most effective if they are semantically and/or phonologically similar with the target word, presented by the picture. An interesting result of this simulation study is that even the normal picture naming task executed in the ACT model without inserting any neural dysfunction produces a low rate of speech errors as it is the case for normal speakers in normal conversation or reading scenarios. This error rate increases dramatically in case of word repetition tasks including distractor words.
Simulation of picture naming in case of inserting model dysfunctions concerning neurons (rate of ablated neurons within a model buffer) and concerning neural connections (rate of ablated neurons in an association memory, see Kröger et al., 2020) for simulating subtypes of aphasia leads to reduction of correct word productions in picture naming tasks (Table 3). Errors appearing here are the production of wrong or of no words. Same holds for the non-word repetition task. The repetition of the correct syllable sequence goes down, and errors appear like production of a wrong syllable sequence or no syllable production at all. Comparable results appear for the word comprehension task. The comprehension rate becomes low and beside correct word meanings more and more wrong meanings become activated at the semantic level of the neural model or no item is activated at that level if the severity of the neural dysfunction (rate of ablated neurons) is increased in the model (Kröger et al., 2022).
Stille et al. (2020) was able to show that phonological or semantic cues can help to increase the rate of correct word productions in a picture naming task if a target word is not produced in a first trail (the model is not able to activate the correct word at the semantic or phonological level) and if cues are given by the environment (by a communication partner) by using the ACT model. Here, neural dysfunctions were inserted in the neural model at the semantic level of the production pathway in order to model lexical access problems.
In general, the simulations performed using the ACT approach (Kröger et al., 2020; Stille et al., 2020) suggest that the “punctual” model dysfunctions implemented in case of modeling different types of speech disorders lead to a variety of different speech symptoms like (i) phonological distortions if a wrong but phonologically similar syllable or word is activated, (ii) to a drop out of a word production or word comprehension if the activation of an item at the phonological, lemma, or concept level is too low, and (iii) wrong word production or wrong word comprehension if further (non-similar) items are co-activated at the phonological, lemma, or concept level.
6. Neural models for medical research: modeling of screening scenarios
Beside a detailed neurobiologically inspired architecture, a neural model of speech processing needs to be able to simulate different communication scenarios as they typically appear in medical screenings (the modeled speech tasks are already mentioned in chapter “Simulation of symptoms” in this paper). Thus, the neural model needs to be able to react on an auditory or visual input (stimuli) and the model should include initiation processes for activating the production process at the cognitive-linguistic level (semantic, lemma, and phonological level) by these stimuli and to further activate motor plans, motor programs, and motor execution as well as to activate the perception and comprehension process. In addition, neural models should include halt or correction procedures during an ongoing production process (see generation of an error signal by comparing intended and produced sensorimotor signals via sensorimotor feedback loop; Kröger et al., 2016). These model features mentioned above are available in the DIVA/GODIVA model (Guenther et al., 2006; Bohland et al., 2010), in the ACT model (Kröger et al., 2012, 2016, 2020, 2022), and in part in the WEAVER model of Roelofs (1992, 1997, 2014) as well as in the spreading activation model of Dell (1986) and Dell et al. (2007, 2013).
The simulation of communication scenarios like those appearing in medical screenings between test supervisor and patient requires (i) an always active perceptive input channel even during ongoing production processes, (ii) the modeling of temporal aspects for forwarding neural activation patterns along the perception and production pathways, e.g., the modeling of concept to lemma to phonological form conversion, (iii) the modeling of cortical and sensorimotor action control (modeling of cortico-cortical feedback loop including basal ganglia and thalamus) for initiating specific cognitive and/or motor action is dominant at specific points in time, and (iv) the modeling of motor feedback (modeling of cortico-cortical feedback loop including cerebellum and thalamus) in order to simulate online control for all motor actions.
Especially the action control component is important in order to model different communication scenarios (i.e., different tasks as part of a medical screening) because different scenarios need the activation of different processing paths within the neural model. In the case of picture naming an external visual stimulus (e.g., a picture displaying a specific object) is initially processed by the visual object recognition module leading to a neural activation at the entry of the cognitive-linguistic module for representing that visually activated item at the semantic level of the perception pathway (Figure 1A). Because the patient (the model) is instructed to name the object in this task, this semantic activation is directly forwarded toward the production pathway (by skipping further cognitive processing) which results in a cascade of neural transformations from semantic state via lemma state to phonological form state and further from motor plan via motor program activation toward execution of the motor program by activating the neuromuscular system. Subsequently, online feedback procedures activate the auditory and somatosensory state of the produced speech item (sensory feedback state) which can be compared with the auditory and somatosensory expectations (learned and stored target states), activated earlier within the production process, which may lead to an online repair of a not well-articulated syllable or to a halt of articulation in case of a severe articulation error. In case of normal speech, in most cases the sensory expectations (target states stored in mental syllabary) match with the feedback signal produced during articulation (currently produced sensory feedback states) and no error signal is generated and thus the neural processing for generating an articulatory correction or a halt signal, which is realized by the action control loop (Kröger et al., 2016, 2020), needs not to be activated.
Word repetition tasks start at the auditory input level, i.e., activation of an auditory (input) state by a signal (stimulus) produced by the communication partner (external speaker, Figure 1A; test supervisor in a medical screening scenario). The resulting auditory state activated at the auditory input buffer activates an auditory state of mental syllabary (syllable level) or can be analyzed in smaller chunks leading to an activation of speech sound candidates (e.g., Guenther et al., 2006). In both cases this leads to an activation of phonological (input) states and subsequently leads to an activation of lemma candidates and to the activation of a semantic state within the perception/comprehension pathway (Figure 1A). The activated semantic state (concept buffer, see Figure 1A) directly activates the production pathway in the case of the word repetition task and subsequently a word candidate is selected within the production pathway and subsequently processed and its motor program is executed in the same way as described above for the picture naming task (e.g., Kröger et al., 2020).
In the case of non-word repetition (logatome repetition), i.e., repetition of a syllable sequence with no meaning in the target language (mother tongue or learned language), the neural activation at the phonological level does not lead to an activation of a lemma and of a concept stored in the mental lexicon. In this case the shortcut between phonological input and output phonological level is activated (dashed black line in Figure 1A; and see Kröger et al., 2020) leads to a direct activation of the phonological form within the production pathway of the model (phonological output buffer, Figure 1A) leading to further activations of motor plans, motor programs and subsequently to motor execution for the activated logatome or syllable sequence.
Word comprehension tasks activate the same neural pathway as already mentioned in case of the word repetition task. But here the processing already ends at the level of concept activation (activation of a meaning, e.g., Kröger et al., 2020). In case of a medical screening task for word comprehension, the target word is presented acoustically by the test supervisor and the patient is asked to point on one specific picture as part of a list of pictures to allow the test supervisor to see the word candidate, selected by the patient. This pointing procedure which is part of the scenario is not included in most models because neural activations can be directly accessed at all levels within the model (here at the concept level) which allows a direct monitoring of concept selection within the task by the patient (model) even without an explicit motor reaction.
In case of all these tasks (all these communication scenarios) described above the patient is already prepared or primed for giving a specific motor reaction, i.e., speaking by using the speech articulation apparatus or gesturing by using the arm-hand apparatus, if an input stimulus is seen or heard. Thus, in case of medical screenings the action control loop is already prepared for activating a specific sequence of cognitive and motor actions which is the consequence of a priming procedure, i.e., a consequence of preparing and instructing the patient or model for executing a specific task. Thus, even if a neural model does not include an action control loop (not including a model of basal ganglia and thalamus), the neural model can be shaped in a way for executing a specific task, i.e., by activating a specific input buffer (e.g., auditory input buffer or visual input buffer) which always leads to a chain of co-activations of further buffers in order to perform the neural processing required for executing a specific task. Thus, tasks can be performed also in case of the spreading activation model of Dell (1986) and Dell et al. (2007, 2013) and in case of the WEAVER model of Roelofs (1992, 1997, 2014) without an explicit modeling of action control.
A relatively complex medical screening task based on a complex communication scenario has been simulated by Stille et al. (2020) using the ACT model. Here, as part of a picture naming task—designed for quantifying lexical access mechanisms—semantic and phonological cues were provided auditorily (by the test supervisor) only in those cases, where a word is not directly produced by the patient (by the model) in a time interval of a few seconds. In these cases, a second and a third word production trial is started by providing acoustic phonological or semantic cues in parallel to the still available visual information. Thus, in case of this task, the action control loop allows word production directly by visual input (a picture representing the target word, for example a pic of a ball) an later the action control loop allows word production based on visual input but added by auditory input (test instructor gives a phonological cue like “the word starts with a [b]” or a semantic cue “the object can be thrown or kicked”). It has been shown by Stille et al. (2020) that in case of modeling a mental lexicon as it is acquired by children suffering from lexical access problems, lexical dysfunctions can be divided in “within level” and “between level dysfunctions,” i.e., in neural dysfunctions appearing within the neural buffers storing and ordering concept, lemma, or phonological form information with respect to semantic, grammatical, or phonological information and in neural dysfunctions appearing in the neural pathways between neural buffers for forwarding information from concept to lemma, or from lemma to phonological form buffers, i.e., in buffers representing the association of concepts to their lemmata as well as of lemmata to their phonological forms. Based on the simulations, Stille et al. (2020) found performance differences for lexical selection and activation processes if neural dysfunctions are located at the semantic and/or if neural dysfunction are located at the phonological level of the mental lexicon.
7. Discussion
Neural modeling of speech processing allows to unfold the relations between location, type, and severity of a neural dysfunction inserted into a model and type and frequency of arising speech symptoms if simulations of specific communication scenarios (speech tasks) are performed using this model. In such a research endeavor the location of a dysfunction within a neural model is defined in a functional and not directly in an anatomically way. While a functional subnetwork or module of a neural model can be associated with an anatomic location in a direct way (see Roelofs, 2014 in case of the WAVER model; see Guenther, 2006; Guenther et al., 2006; Kearney and Guenther, 2019 in case of the DIVA/GODIVA models and Ueno et al., 2011 in case of LICHTHEIM 2 model) it in not always simple to identify a specific neural dysfunction on the basis of disruptions or damage appearing in a specific anatomical location within the central nervous system of a patient. Thus, it is not easy to associate brain lesions, disruptions, or abnormalities appearing in a specific anatomic location of the central nervous system (probably identified by neuroimaging methods applied to patients) and functional deficits in speech and language processing. For example, a brain lesion arising in the mid part of the temporal lobe may result in dysfunctions of different lexical submodules or buffers, for example lemma and concept buffer at the production as well as on the perception pathway. Or in case of a neurodegenerative abnormality of the basal ganglia we need to know in detail how this defect affects the cortico-striatal association network to differentiate dysfunctions (functional deficits) with respect to connections of the action control loop with the sensorimotor part or with the linguistic-cognitive part of the speech processing network. Thus, insertions of defined neural dysfunctions in computer-implemented quantitative neural models and its behavioral results generated (i.e., simulated) in speech tasks, allow to refine the definition of a speech or language disorder with respect to its etiology. It should be kept in mind that the LICHTHEIM 2 model here plays an intermediate role, because this model is not defined primarily in the neurofunctional domain (only the input and output layers are defined in a functional way) but refers to the neuroanatomical domain because the model separates different intermediate (or hidden) neural layers by specifying their neuroanatomical location but without specifying these hidden layers directly in a functional sense (e.g., for representing phonological, lemma, or concept forms).
Moreover, it should be kept in mind that a model could generate plausible simulation results even if the underlying neural mechanisms implemented in that model are not (strictly) similar with those appearing in humans. But in order to hold a high similarity of natural and simulated neural processes all neural models mentioned in this paper include basic as well as advanced neurofunctional knowledge gained from natural data as it is available from contemporary literature.
Furthermore, it needs to be mentioned that models are helpful to define functionality and to associate specific functionality appearing in specific brain regions with specific submodules of neural models, but it should be kept in mind that the hypothetical association of brain regions with submodules of neural models does not automatically strengthen the neurobiological reality of a model.
Nevertheless, the potential application of neural models in medical research could be manifold. (i) Neural models of speech processing allow to simulate medial screenings (tasks which are used for the diagnosis of speech and language disorders) by simulating the corresponding communication scenarios between patient and test supervisor. If location, type, and severity of an inserted dysfunction is varied in the model the simulation results could uncover the sensitivity of a screening task with respect to a specific neural dysfunction. Thus, simulations of screening tasks allow to estimate the effectiveness of that screening task to uncover a specific speech and language disorder. (ii) Because speech screening tasks usually are shaped or configured manually based on the experience of leading experts in the field of diagnosis and therapy of speech and language disorders, this information concerning effectiveness or sensitivity of a specific screening task could help to optimize screenings by varying all available task scenario parameters like type, number, or complexity of test items, number or repetition of trials, etc. (iii) Because of potential learning and familiarity effects and because of ethical reasons, a screening task can be undertaken only one time with a patient, while model simulations can be repeated as often as necessary by using the same computer-implemented model. Thus, a high number of patients is needed, all suffering from the same type and same severity of a speech or language disorder, to generate meaningful results for the optimization of a speech screening. Such a research endeavor is difficult to realize because of the need of a high number of well diagnosed patients for conducting such a research study, while neural models are capable to fulfill these demands more easily. A model is capable to repeat a screening task as often as needed and the location, type, and severity of the (inserted) neural dysfunction is clearly defined. This could result in a high reliability for the results concerning the association of location, type, and severity of a neural dysfunction and type and number of relevant speech symptoms. Moreover, no ethical conflicts appear in case of using computer models. (iv) All aspects discussed above are also applicable for the development of therapy scenarios. Because learning effects can be simulated in neural models as well, it is possible to quantify these learning effect and thus to quantify the efficiency of a therapy scenario for different types and severity levels of a specific speech and language disorder. Moreover, it is possible to vary all parameters of a therapy scenario (e.g., length of intervention, number and type of speech items trained in the therapy scenario, different designs concerning the increase in complexity of test items during a therapy, etc.) in order to find an optimally shaped treatment procedure (therapy scenario). But it needs to be stated here that all these ideas for simulation experiments have not been realized thus far. This disadvantage is mainly due to the current state of the art in neural modeling. Most models are currently used mainly for simulating typical behavioral effects like the production of striking symptoms, in order to exemplify the quality of modeling already reached in these days, but further model development and further simulations need to be done in order to simulate more complex screening or therapy scenarios in order to be able to deliver statistically significant results which allow to modify these scenarios toward more efficiency.
The detailedness of neural models of speech production is already on a relative high level in case of the cognitive-linguistic model part (Roelofs, 2014; Kröger et al., 2020) as well as in case of the sensorimotor model part (Guenther, 2006; Guenther et al., 2006; Bohland et al., 2010; Kröger et al., 2022). This allows a detailed modeling of speech and language disorders like aphasia with respect to all lexical aspects (production and comprehension deficits) as well as for apraxia of speech (production deficits with respect to planning and programming of syllables and syllable sequences). While the modeling of vocal tract geometries and vocal fold dynamics including all aerodynamic and acoustic relations is already on a high level as well (for a review see Kröger, 2022), there are still deficits in modeling the neuromuscular system and especially there is no overall consent concerning a control concept for neural activation at the level of the neuromuscular system directly controlling speech articulators (ibid.). This limits our current modeling endeavors for example concerning the simulation of articulatory consequences of dysarthric speech disorders especially in case of flaccid dysarthria and in case of spastic dysarthria (abnormal muscle tone). But due to the existence of already very detailed models of the basal ganglia and of the cortico-cortical control loop including basal ganglia and thalamus (Kröger et al., 2022), the modeling of other types of dysarthria is already possible but has not exemplified yet. In case of neurogenic stuttering the detailed implementation of this cortico-cortical action control loop already gave plausible simulation results for symptoms appearing in stuttering (see Civier et al., 2013).
Unfortunately, the neurobiologically based quantitative modeling of speech perception and speech comprehension is not as developed as it is the case for speech production. Specifically, there exist no comprehensive models which include the important concept of brain waves (gamma and theta waves, see Hickok and Poeppel, 2007; Ghitza, 2011; Ghitza et al., 2013) which is needed in order to model speech perception and speech comprehension realistically in a neurobiologically grounded way.
Because the microscopic functional level of natural neural networks—i.e., the cellular level of neurons and their functioning within subnetworks as well as within the whole network of speech processing—is not or not easily accessible by imaging as well as by functional electro-analytical methods up to now (e.g., Batista-García-Ramó and Fernández-Verdecia, 2018), neurobiologically inspired and computer-implemented quantitative neural models are currently an important and advantageous research tool in order to get a detailed and quantitative impression of the neurobiological functioning in speech processing (production and perception).
Finally, it needs to be stated that even if it seems to be attractive to develop computational models which probably are able to mimic the functionality of the neural system of speech production and speech perception including comprehension or of other human capabilities it should be kept in mind that all these models up to now are only of limited benefits form the viewpoint of answering fundamental research questions. And it needs to be stated that the idea of understanding speech and language disorders from a functional point of view is not new (e.g., Lichtheim, 1885). Much of the information given in chapter 4 of this paper concerning the functional specification of different types of speech and language disorders may also be deducible from box-and-arrow models (e.g., Datteri and Laudisa, 2014). But the chance to derive quantitative results like those concerning severity levels of a disorder or concerning the sensitivity level of a screening in order to detect a specific disorder or how effective a therapy method may be in strengthening a specific speaking behavior of a (model-) patient can be seized by simulating specific tasks and opens a path in the direction of increasing the efficiency of diagnosis and therapy tools. This increase in efficiency of diagnosis and therapy tools can be reached hardly by other methods as is already mentioned above in this paper (because of the need of a huge number of patients to participate in a research endeavor and because these patients then have to pass an number of screenings of therapy modules without a clear therapeutic benefit for them. Moreover, all patients recruited for this kind of studies need to be excellently diagnosed and should suffer from specific and isolated type of disorder and furthermore the exact degrees of severity of the disorder needs to be known as well, in order to get meaningful results).
Author contributions
The author confirms being the sole contributor of this work and has approved it for publication.
Conflict of interest
The author declares that the research was conducted in the absence of any commercial or financial relationships that could be construed as a potential conflict of interest.
Publisher's note
All claims expressed in this article are solely those of the authors and do not necessarily represent those of their affiliated organizations, or those of the publisher, the editors and the reviewers. Any product that may be evaluated in this article, or claim that may be made by its manufacturer, is not guaranteed or endorsed by the publisher.
References
Allison, K. M., Cordella, C., Iuzzini-Seigel, J., and Green, J. R. (2020). Differential diagnosis of apraxia of speech in children and adults: a scoping review. J. Speech Lang. Hear. Res. 63, 2952–2994. doi: 10.1044/2020_JSLHR-20-00061
Araki, K., Hirano, Y., Kozono, M., Fujitani, J., and Shimizu, E. (2021). The screening test for aphasia and dysarthria (STAD) for patients with neurological communicative disorders: a large-scale, multicenter validation study in Japan. Folia Phoniatr. Logop. 74, 195–208. doi: 10.1159/000519381
Ballard, K. J., Azizi, L., Duffy, J. R., McNeil, M. R., Halaki, M., O'Dwyer, N., et al. (2016). A predictive model for diagnosing stroke-related apraxia of speech. Neuropsychologia 81, 129–139. doi: 10.1016/j.neuropsychologia.2015.12.010
Ballard, K. J., Granier, J. P., and Robin, D. A. (2000). Understanding the nature of apraxia of speech: theory, analysis, and treatment. Aphasiology 14, 969–995. doi: 10.1080/02687030050156575
Batista-García-Ramó, K., and Fernández-Verdecia, C. I. (2018). What we know about the brain structure-function relationship. Behav. Sci. 8, 39. doi: 10.3390/bs8040039
Biniek, R., Huber, W., Glindemann, R., Willmes, K., and Klumm, H. (1992). [The aachen aphasia bedside test–criteria for validity of psychologic tests] Der Aachener Aphasie-Bedside-Test–Testpsychologische Gutekriterien. Nervenarzt 63, 473–479.
Bohland, J. W., Bullock, D., and Guenther, F. H. (2010). Neural representations and mechanisms for the performance of simple speech sequences. J. Cogn. Neurosci. 22, 1504–1529. doi: 10.1162/jocn.2009.21306
Chang, S. E., and Guenther, F. H. (2020). Involvement of the cortico-basal ganglia-thalamocortical loop in developmental stuttering. Front. Psychol. 10, 3088. doi: 10.3389/fpsyg.2019.03088
Civier, O., Bullock, D., Max, L., and Guenther, F. H. (2013). Computational modeling of stuttering caused by impairments in a basal ganglia thalamo-cortical circuit involved in syllable selection and initiation. Brain Lang. 126, 263–278. doi: 10.1016/j.bandl.2013.05.016
Crary, M. A., Haak, N. J., and Malinsky, A. E. (1989). Preliminary psychometric evaluation of an acute aphasia screening protocol. Aphasiology 3, 611–618. doi: 10.1080/02687038908249027
Crinion, J., Holland, A. L., Copland, D. A., Thompson, C. K., and Hillis, A. E. (2013). Neuroimaging in aphasia treatment research: quantifying brain lesions after stroke. Neuroimage 73, 208–214. doi: 10.1016/j.neuroimage.2012.07.044
Datteri, E., and Laudisa, F. (2014). Box-and-arrow explanations need not be more abstract than neuroscientific mechanism descriptions. Front. Psychol. 5, 464. doi: 10.3389/fpsyg.2014.00464
De Renzi, E., and Faglioni, P. (1978). Normative data and screening power of a shortened version of the token test. Cortex 14, 41–49. doi: 10.1016/S0010-9452(78)80006-9
De Renzi, E., and Vignolo, L. A. (1962). The token test: a sensitive test to detect receptive disturbances in aphasics. Brain 85, 665–678. doi: 10.1093/brain/85.4.665
Dell, G. S. (1986). A spreading activation theory of retrieval in language production. Psychol. Rev. 93, 283–321. doi: 10.1037/0033-295X.93.3.283
Dell, G. S., Martin, N., and Schwartz, M. F. (2007). A case-series test of the interactive two-step model of lexical access: predicting word repetition from picture naming. J. Mem. Lang. 56, 490–520. doi: 10.1016/j.jml.2006.05.007
Dell, G. S., Schwartz, M. F., Nozari, N., Faseyitan, O., and Coslett, H. B. (2013). Voxel-based lesion-parameter mapping: identifying the neural correlates of a computational model of word production, Cognition 128, 380–396. doi: 10.1016/j.cognition.2013.05.007
Eliasmith, C. (2013). How to Build a Brain: A Neural Architecture for Biological Cognition. New York, NY: Oxford University Press. doi: 10.1093/acprof:oso/9780199794546.001.0001
Eliasmith, C., and Anderson, C. H. (2003). Neural Engineering: Computation, Representation, and Dynamics in Neurobiological Systems. Cambridge, MA: MIT Press.
Eliasmith, C., Stewart, T. C., Choo, X., Bekolay, T., DeWolf, T., Tang, Y., et al. (2012). A large-scale model of the functioning brain. Science 338, 1202–1205. doi: 10.1126/science.1225266
Enderby, P., Wood, V., and Wade, D. (1987). Frenchay Aphasia Screening Test: (FAST). Cornwall, UK: Test Manual Whurr Publishers.
Friederici, A. D. (2011). The brain basis of language processing: from structure to function. Physiol. Rev. 91, 1357–1392. doi: 10.1152/physrev.00006.2011
Ghitza, O. (2011). Linking speech perception and neurophysiology: speech decoding guided by cascaded oscillators locked to the input rhythm. Front. Psychol. 2, 130. doi: 10.3389/fpsyg.2011.00130
Ghitza, O., Giraud, A. L., and Poeppel, D. (2013). Neuronal oscillations and speech perception: critical-band temporal envelopes are the essence. Front. Hum. Neurosci. 6, 340. doi: 10.3389/fnhum.2012.00340
Golfinopoulos, E., Tourville, J. A., and Guenther, F. H. (2010). The integration of large-scale neural network modeling and functional brain imaging in speech motor control. Neuroimage 52, 862–874. doi: 10.1016/j.neuroimage.2009.10.023
Guenther, F. H. (2006). Cortical interactions underlying the production of speech sounds. J. Commun. Disord. 39, 350–365. doi: 10.1016/j.jcomdis.2006.06.013
Guenther, F. H. (2016). Neural Control of Speech. Cambridge, MA: MIT Press. doi: 10.7551/mitpress/10471.001.0001
Guenther, F. H., Ghosh, S. S., and Tourville, J. A. (2006). Neural modeling and imaging of the cortical interactions underlying syllable production. Brain Lang. 96, 280–301. doi: 10.1016/j.bandl.2005.06.001
Hickok, G., and Poeppel, D. (2007). The cortical organization of speech processing. Nat. Rev. Neurosci. 8, 393402. doi: 10.1038/nrn2113
Hickok, G., and Poeppel, D. (2016). “Neural basis of speech perception,” in Neurobiology of Language, eds G. Hickok and S. L. Small (Cambridge, MA: Academic Press), 299–310. doi: 10.1016/B978-0-12-407794-2.00025-0
Indefrey, P., and Levelt, W. J. M. (2004). The spatial and temporal signatures of word production components. Cognition 92, 101–144. doi: 10.1016/j.cognition.2002.06.001
Kearney, E., and Guenther, F. H. (2019). Articulating: the neural mechanisms of speech production. Lang. Cogn. Neurosci. 34, 1214–1229. doi: 10.1080/23273798.2019.1589541
Kertesz, A. (2006). Western Aphasia Battery Revised. San Antonio, TX: Harcourt Assessment. doi: 10.1037/t15168-000
Kröger, B. J. (2021). “Modeling dysfunctions in the coordination of voice and supraglottal articulation in neurogenic speech disorders,” in Models and Analysis of Vocal Emissions for Biomedical Applications, ed. C. Manfredi (Firenze: Firenze University Press), 79–82.
Kröger, B. J. (2022). Computer-implemented articulatory models for speech production: a review. Front. Robot. AI 9, 796739. doi: 10.3389/frobt.2022.796739
Kröger, B. J., Bafna, T., and Cao, M. (2019). Emergence of an action repository as part of a biologically inspired model of speech processing: the role of somatosensory information in learning phonetic-phonological sound features. Front. Psychol. 10, 1462. doi: 10.3389/fpsyg.2019.01462
Kröger, B. J., and Bekolay, T. (2019). Neural Modeling of Speech Processing and Speech Learning. An Introduction. Berlin: Springer International Publishing. ISBN 978-3-030-15852-1. doi: 10.1007/978-3-030-15853-8
Kröger, B. J., Bekolay, T., and Cao, M. (2022). On the emergence of phonological knowledge and on motor planning and motor programming in a developmental model of speech production. Front. Hum. Neurosci. 16, 844529. doi: 10.3389/fnhum.2022.844529
Kröger, B. J., and Cao, M. (2015). The emergence of phonetic-phonological features in a biologically inspired model of speech processing. J. Phon. 53, 88–100. doi: 10.1016/j.wocn.2015.09.006
Kröger, B. J., Crawford, E., Bekolay, T., and Eliasmith, C. (2016). Modeling interactions between speech production and perception: speech error detection at semantic and phonological levels and the inner speech loop. Front. Comput. Neurosci. 10, 51. doi: 10.3389/fncom.2016.00051
Kröger, B. J., Kannampuzha, J., Eckers, C., Heim, S., Kaufmann, E., Neuschaefer-Rube, C., et al. (2012). “The neurophonetic model of speech processing ACT: structure, knowledge acquisition, and function modes,” in Cognitive Behavioural Systems, LNCS 7403, eds A. Esposito, A. M. Esposito, A. Vinciarelli, R. Hoffmann, and V. C. Müller (Berlin: Springer), 398–404. doi: 10.1007/978-3-642-34584-5_35
Kröger, B. J., Kannampuzha, J., and Kaufmann, E. (2014). Associative learning and self-organization as basic principles for simulating speech acquisition, speech production, and speech perception. EPJ Nonlinear Biomed. Phys. 2, 2. doi: 10.1140/epjnbp15
Kröger, B. J., Stille, C., Blouw, P., Bekolay, T., and Stewart, T. C. (2020). Hierarchical sequencing and feedforward and feedback control mechanisms in speech production: a preliminary approach for modeling normal and disordered speech. Front. Comput. Neurosci. 14, 99. doi: 10.3389/fncom.2020.573554
Levelt, W. J. M., Roelofs, A., and Meyer, A. S. (1999). A theory of lexical access in speech production. Behav. Brain Sci. 22, 1–75. doi: 10.1017/S0140525X99001776
Litwińczuk, M. C., Muhlert, N., Cloutman, L., Trujillo-Barreto, N., and Woollams, A. (2022). Combination of structural and functional connectivity explains unique variation in specific domains of cognitive function. Neuroimage 262, 119531. doi: 10.1016/j.neuroimage.2022.119531
Maass, W. (1997). Networks of spiking neurons: the third generation of neural network models. Neural Netw. 10, 1659–1671. doi: 10.1016/S0893-6080(97)00011-7
Miller, H. E., and Guenther, F. H. (2021). Modelling speech motor programming and apraxia of speech in the DIVA/GODIVA neurocomputational framework. Aphasiology 35, 424–441. doi: 10.1080/02687038.2020.1765307
Nassif, A. B., Shahin, I., Attili, I. M., and Shaalan, K. (2019). Speech recognition using deep neural networks: a systematic review. IEEE Access 7, 19143–19165. doi: 10.1109/ACCESS.2019.2896880
Palmer, R., and Enderby, P. (2007). Methods of speech therapy treatment for stable dysarthria: a review. Adv. Speech Lang. Pathol. 9, 140–153. doi: 10.1080/14417040600970606
Parrell, B., Ramanarayanan, V., Nagarajan, S., and Houde, J. (2019). The FACTS model of speech motor control: fusing state estimation and task-based control. PLoS Comput. Biol. 15, e1007321. doi: 10.1371/journal.pcbi.1007321
Ponulak, F., and Kasinski, A. (2011). Introduction to spiking neural networks: information processing, learning and applications. Acta Neurobiol. Exp. 71, 409–433. Available online at: https://www.ncbi.nlm.nih.gov/nlmcatalog?term=%22Acta+Neurobiol+Exp+%28Wars%29%22%5BTitle+Abbreviation%5D
Rockland, K. S., and Ichinohe, N. (2004). Some thoughts on cortical minicolumns. Exp. Brain Res. 158, 265–277. doi: 10.1007/s00221-004-2024-9
Roelofs, A. (1992). A spreading-activation theory of lemma retrieval in speaking. Cognition 42, 107–142. doi: 10.1016/0010-0277(92)90041-F
Roelofs, A. (1997). The WEAVER model of word-form encoding in speech production. Cognition 64, 249–284. doi: 10.1016/S0010-0277(97)00027-9
Roelofs, A. (2014). A dorsal-pathway account of aphasic language production: the WEAVER++/ARC model. Cortex 59, 33–48. doi: 10.1016/j.cortex.2014.07.001
Roger, V., Farinas, J., and Pinquier, J. (2022). Deep neural networks for automatic speech processing: a survey from large corpora to limited data. J. Audio Speech Music Proc. 2022, 19. doi: 10.1186/s13636-022-00251-w
Schwartz, M. F., Dell, G. S., Martin, N., Gahl, S., and Sobel, P. (2006). A case-series test of the interactive two-step model of lexical access: evidence from picture naming. J. Mem. Lang. 54, 228–264. doi: 10.1016/j.jml.2005.10.001
Senft, V., Stewart, T. C., Bekolay, T., Eliasmith, C., and Kröger, B. J. (2016). Reduction of dopamine in basal ganglia and its effects on syllable sequencing in speech: a computer simulation study. Basal Ganglia 6, 7–17. doi: 10.1016/j.baga.2015.10.003
Senft, V., Stewart, T. C., Bekolay, T., Eliasmith, C., and Kröger, B. J. (2018). Inhibiting basal ganglia regions reduces syllable sequencing errors in parkinson's disease: a computer simulation study. Front. Comput. Neurosci. 12, 41. doi: 10.3389/fncom.2018.00041
Stefaniak, J. D., Halai, A. D., and Lambon Ralph, M. A. (2020). The neural and neurocomputational bases of recovery from post-stroke aphasia. Nat. Rev. Neurol. 16, 43–55. doi: 10.1038/s41582-019-0282-1
Stewart, T. C., and Eliasmith, C. (2014). Large-scale synthesis of functional spiking neural circuits. Proc. IEEE 102, 881–898. doi: 10.1109/JPROC.2014.2306061
Stille, C., Bekolay, T., Blouw, P., and Kröger, B. J. (2020). Modeling the mental lexicon as part of long-term and working memory and simulating lexical access in a naming task including semantic and phonological cues. Front. Psychol. 11, 1594. doi: 10.3389/fpsyg.2020.01594
Tippett, D. C., Hillis, A. E., and Tsapkini, K. (2015). Treatment of primary progressive aphasia. Curr. Treat. Options Neurol. 17, 362. doi: 10.1007/s11940-015-0362-5
Ueno, T., Saito, S., Rogers, T. T., and Lambon Ralph, M. A. (2011). Lichtheim 2: synthesizing aphasia and the neural basis of language in a neurocomputational model of the dual dorsal-ventral language pathways. Neuron 72, 385–396. doi: 10.1016/j.neuron.2011.09.013
Van der Merwe, A. (2021). New perspectives on speech motor planning and programming in the context of the four- level model and its implications for understanding the pathophysio-logy underlying apraxia of speech and other motor speech disorders. Aphasiology 35, 397–423. doi: 10.1080/02687038.2020.1765306
Warlaumont, A. S., and Finnegan, M. K. (2016). Learning to produce syllabic speech sounds via reward-modulated neural plasticity. PLoS ONE 11, e0145096. doi: 10.1371/journal.pone.0145096
Weems, S. A., and Reggia, J. A. (2006). Simulating single word processing in the classic aphasia syndromes based on the Wernicke–Lichtheim–Geschwind theory. Brain Lang. 98, 291–309. doi: 10.1016/j.bandl.2006.06.001
Yamazaki, K., Vo-Ho, V. K., Bulsara, D., and Le, N. (2022). Spiking neural networks and their applications: a review. Brain Sci. 12, 863. doi: 10.3390/brainsci12070863
Appendix
Appendix A: second and third generation artificial neural networks
Second generation neural networks (node-and-link networks) are composed of nodes (ensembles of neurons) and links or edges (connections between nodes). Nodes can be interpreted from the neurobiological perspective as bundles of neighboring neurons, i.e., as a set of neurons within a small brain region. Nodes are functionally characterized by its activation level. The activation level is calculated from the input activation stemming from all preceding nodes which are connected to this node via neural links. Thus, a node does not directly represent a neuron in a narrow neurobiological sense but summarizes the neural activity of a set of neurons (i.e., spatial averaging) over a time interval (temporal averaging; not less than 10 ms in most of these networks; see the spatial temporal averaging approach, STAA approach, Kröger and Bekolay, 2019, p. 133 ff).
An important characteristic of node-and-link networks is that nodes are organized in layers representing states like, e.g., phonological forms, lemmata, concepts, motor plans, auditory, or somatosensory states etc. If these layers and nodes of a second generation network need to be interpreted in a neuroanatomical context, a layer would represent a small portion of the cortical brain surface and a node would represent probably a cortical column. In this context it must be emphasized that these artificial neural network layers should not be confused with the neuroanatomically defined five cortical layers I, II, III, IV, and V ordered in parallel to the neocortical surface (see, e.g., Rockland and Ichinohe, 2004). The layers of an artificial neural networks if there is a need to interpret them in neurobiologically usually represent different small areas within different cortical regions, e.g., areas for hosting and processing auditory forms, phonological forms, lemmata, and concepts in the temporal lobe, or areas for hosting motor plan and motor program forms in the frontal lobe. Moreover, a node within that specific artificial neural network layer can probably be assumed to represent all neurons appearing in a cortical column within the specific cortical area specified by that layer. This holds in the same way for the buffers (replacing layers) and spiking neurons (replacing nodes) as defined in the third generation spiking neural networks (see below). In these second or third generation network models the nodes of a layer (or spiking neurons in a buffer) are connected with the nodes (spiking neurons) of another layer (buffer). Thus, links (neural connections) between different layers or buffers can be interpreted as neural connections between cortical columns representing different cortical areas.
A further characteristic of second generation neural networks or node-and-link networks is their lack of explicit temporal processing. If the network has already be trained and if the network is running in performance mode (e.g., speech production or speech perception mode), the input layer of the network model normally is activated by a stimulus and a resulting neural activation pattern appears at the output layer in one simulation step (called “performance trial”) without any further temporal specification. In case of speech processing the input and output neural activation patterns (applied to the input layer and appearing at the output layer) thus need to encode the entire syllable-, word-, or phrase-sized auditory or motor pattern, so that the temporal organization of motor or auditory patterns are coded intrinsically in these neural activation patterns (see e.g., Kröger and Cao, 2015).
In the case of learning or training of these second generation neural networks (training mode, e.g., for supervised learning) a set of input/output training stimuli is applied to the network several times, i.e., in several training epochs. Here, in a comparable way to the performance mode, one training step alone does not need any temporal specification (like one performance trial). But because during a training procedure the link weights of the neural network are altered in each training step and because the network during training slowly but increasingly performs better and better with an increasing number of training steps and training epochs, this leads to a notion of an increase in learning (increase in knowledge) over time.
Third generation neural networks (spiking neuron networks, SNNs, e.g., Ponulak and Kasinski, 2011; Yamazaki et al., 2022) aim for a neurobiologically plausible modeling of neurons and neural connections. Here, spiking neuron models are used for modeling the neurobiology of a neuron including synaptic connections coming from preceding neurons [i.e., modeling the increase of voltage of the cell membrane potential resulting from incoming presynaptic spikes, modeling the generation of a postsynaptic pulse (or spike) if the firing threshold of the membrane potential is reached, modeling the post-spike refractory period for the membrane potential, modeling temporal delay stemming from synaptic input connections, etc.]. Thus, the temporal features of signal processing are modeled in a more neurobiologically inspired way in third generation neural networks and all temporal features need not to be set externally for this type of networks in comparison to second generation neural networks (e.g., the setting of activation decay rates for nodes, see Dell et al., 2013; Roelofs, 2014). In third generation network models temporal parameters are directly controlled by the synapse model (temporal delay for transforming an incoming presynaptic spike in a specific postsynaptic current for increasing/decreasing the membrane potential depending on the type of synaptic connection: exhibitory or inhibitory) and by the kernel or cell model of the neuron (setting the duration of the post-spike latency period and of the time constant for rate of increase/decrease of membrane potential in case of a presynaptic spike entering an exhibitory/inhibitory synaptic connection).
In third generation or spiking neural networks time is modeled directly within its basic unit, i.e., within the spiking neuron model including its synapses. Simulations can be performed here as a function of time. Even incoming static signals are applied to an input buffer of the model during a defined time interval leading to defined spike trains for further processing with the neural network. Thus, in the NEF-SPA framework (see Appendix B) the forwarding of a neural state from buffer to buffer with or without further processing by intermediately connected associative memories leads to a specific delay between input and output signal as a result of a neurobiologically inspired synaptic processing (here for leaky integrate-and-fire neurons, LIF neurons, see Eliasmith, 2013). This delay in processing is about 50 ms from phonological form to concept activation (perception pathway) or vice versa from concept to phonological form activation (production pathway) in our ACT model (see e.g., Kröger et al., 2016, 2020). Moreover, this implicit modeling of time allows a straight-forward modeling of action selection as is needed, e.g., for task execution even in case of simple speech tasks (see e.g., Kröger et al., 2020, 2022).
In order to include temporal aspects in second generation network models a temporal model can be added to second generation neural models as done for WEAVER and for DIVA/GODIVA. Here, an activation time interval is defined (e.g., 10 ms) and neural activation is recalculated for a sequence of these time intervals. Here, neural activation of each node within each layer decreases to a certain degree per time interval and the activation of each node per time interval results from the activation level of the preceding time step which is altered only slightly by each new incoming inhibitory or excitatory activation from presynaptic nodes in a current time step. This as well allows a modeling of action selection processes as introduced by Bohland et al. (2010), e.g., for modeling the chunking of a phonological input chain with respect to motor program selection.
Appendix B: The NEF-SPA framework of third generation spiking neural networks
The NEF-SPA framework (Neural Engineering Framework, NEF, augmented by and Semantic Pointer Architecture, see Eliasmith and Anderson, 2003; Eliasmith, 2013; Stewart and Eliasmith, 2014, Appendix B) allows the development of large-scale brain models including peripheral modules (i.e., for sensory input and motor output processing, see Eliasmith et al., 2012) by using a third generation neural network approach. This framework delivers basic elements for hosting neural states (neuron ensembles and neuron buffers) and for forwarding and/or processing neural information by defining the synaptic weights of all neural connections associating two neuron ensembles or neuron buffers. The default neuron model is the leaky integrate-and-fire neuron model, i.e., a spiking neuron model capable of modeling synaptic processing (excitatory as well as inhibitory synaptic connections) and capable of modeling all temporal features concerning the increase or decrease of the membrane potential resulting from incoming presynaptic spikes as well as capable of triggering postsynaptic spikes. Cognitive and higher level sensory and motor states are hosted in this network type by higher-level state buffers, also called SPA-buffers (e.g., lexical states, see Section 5 of this paper). Lower-level motor states (i.e., syllable oscillators and gesture movement trajectory estimators; see Section 5 of this paper) as well as lower-level auditory states are hosted in this network type by lower-level state buffers, called neuron ensembles or NEF-ensembles.
The Semantic Pointer Architecture SPA which is based on the NEF represents cognitive and higher-level sensory and motor states in form of vectors in a D-dimensional vector space. Different vector spaces need to be defined for different types of items, e.g., for words, lemmata, phonological forms, motor plans, motor programs as well as for higher level auditory, somatosensory, and phonetic forms representing syllables. Semantic, grammatical, or phonological similarities as well as motor plan or motor program similarities or similarities of higher-level auditory, somatosensory, and phonetic forms can be modeled and stored as sets of S-pointers in Semantic Pointer Networks (Kröger et al., 2016). Typical examples for similarities are (i) at the semantic level: e.g., “boy” and “girl” are similar items with respect to the subordinate item “humans”, “dog,” and “cat” are similar items with respect to the subordinate item “animals”; (ii) at the lemma level: e.g., “dog” and “cat” are nouns, “to bark” and “to meow” are verbs; (ii) at other levels: e.g., the syllables/dog/and/dodge/are phonological as well as phonetically, auditorily and motorically similar, because both words start with same consonant followed by the same vowel, etc.
A semantic pointer or S-pointer is a mathematical construct pointing on a specific item and on its neural state (e.g., “dog”, “‘cat”). Different sets of S-pointers appear in different D-dimensional vector spaces and thus define different item categories (e.g., concept, lemma, phonological form, etc.). Each S-pointer defines its own neural activation pattern which represents one item as neural state in a state buffer. State buffers are implemented in the NEF-SPA framework as a set of D neuron ensembles (NEF-ensembles) where each neuron ensemble is a set of N neurons (typically: N = 20 ··· 100) representing a “value” while the SPA-buffer can represent a whole D-dimensional S-pointer (typically: D = 500 in case of representing a full vocabulary of a language, Kröger et al., 2016). This concept of the SPA based on the NEF allows a straight-forward implementation and a direct combination of cognitive-linguistic modules (mainly using SPA-buffers) with (lower-level) sensorimotor modules (mainly using NEF-ensembles) for building up large-scale spiking neural networks, e.g., for speech processing (Kröger et al., 2020, 2022).
Concerning the use of associative memories within the NEF-SPA framework (see below, Appendix C) it should be mentioned that a direct connection of two buffers just leads to a (simple) forwarding of neural information without further information processing. Synaptic weights here model a (new) coding of each S-pointer in each buffer, but the underlying meaning of an activated neural state (of an item) in simply connected buffers remains the same, e.g., a phonological item stays as the same phonological item in the next buffer, even if the neural activation pattern (i.e., the coding and decoding of neural activity in each buffer) is different from buffer to buffer. In order to transform a state from one vocabulary to another vocabulary (e.g., from phonological forms to lemmata and so on) an associative memory need to be interposed between both buffers. This makes the modeling of neural pathways a little more complex but allows the modeling of neural dysfunctions in buffers as well as in neural pathway by using the same process, i.e., by ablating neurons. Thus, ablating neurons in buffers is used for modeling dysfunctions within buffers while ablating neurons within associative memories is used for modeling dysfunctions within the neural pathways between buffers (see, e.g., Stille et al., 2020).
The neurobiologically-inspired motivation for the definition of all basic elements used within the NEF-SPA framework (e.g., buffers, memories, simple neural connection pathways, S-pointer networks, binding and unbinding buffers, etc.) is motivated by the theoretical background delivered for the NEF-SPA framework (see Eliasmith, 2013; Stewart and Eliasmith, 2014). It has been proved that only few basic NEF and SPA elements allow the development of large-scale brain models capable of modeling a wide range of human behavior (cognitive as well as sensorimotor aspects of behavior, see Eliasmith et al., 2012; Eliasmith, 2013; Stewart and Eliasmith, 2014).
Appendix C: The ACT model
A third generation SNN (see Appendix A), developed in the NEF-SPA context (see Appendix B), is used for modeling the cognitive-linguistic model part as well as for modeling the production side of the sensorimotor model, i.e., the implementation of motor plan, motor program, and motor execution buffer in our ACT model (see Figure 1A and see Kröger et al., 2016, 2020, 2022; the name ACT is based on an early modeling of the sensorimotor part of the model: speech action model, see Kröger et al., 2012). The neural pathways and mappings connecting the lexical and sensorimotor state buffers are realized using intermediate associative memories. Associative memories as further elements which can be incorporated in neural pathways connecting SPA-buffers are needed in order to map states from one item category onto states of another item category (e.g., concepts onto lemmata or lemmata onto phonological forms and vice versa; see below). The semantic pointer networks for concepts, lemmata, and phonological forms are represented and stored as part of the mental lexicon (Figure 1A) and the semantic pointer networks for motor plans, motor programs, auditory and somatosensory states of syllables are represented and stored as part of the mental syllabary (Kröger et al., 2012). Not implemented thus far in terms of this NEF-SPA third generation neural network is the feedback loop within the sensorimotor part of our model sketch (i.e., somatosensory and auditory state, target, and error buffers for auditory and somatosensory processing, see also Figures 1A, C). But the sensorimotor part exemplified in our model sketch is already implemented as second generation neural network (node-and-link network) and is used for simulating early states of speech acquisition like the babbling phase and the imitation phase of newborns and toddlers (see Kröger et al., 2014, 2019; Kröger and Cao, 2015). A shortcoming of the second generation network approach is that lower-level auditory, somatosensory, as well as motor states of syllables, words or phrases cannot be represented in a temporal flexible way. Here, we need to define a fixed time window (e.g., of about 500 ms) for all types of states representing syllables, words, or phrases.
Keywords: neural model of speech processing, speech production, speech perception, speech disorder, neural dysfunction, brain lesion, communication scenarios, medical screening
Citation: Kröger BJ (2023) Modeling speech processing in case of neurogenic speech and language disorders: neural dysfunctions, brain lesions, and speech behavior. Front. Lang. Sci. 2:1100774. doi: 10.3389/flang.2023.1100774
Received: 17 November 2022; Accepted: 13 September 2023;
Published: 09 October 2023.
Edited by:
Qing Cai, East China Normal University, ChinaReviewed by:
Elena Barbieri, Northwestern University, United StatesYa-Ning Chang, National Chung Cheng University, Taiwan
Copyright © 2023 Kröger. This is an open-access article distributed under the terms of the Creative Commons Attribution License (CC BY). The use, distribution or reproduction in other forums is permitted, provided the original author(s) and the copyright owner(s) are credited and that the original publication in this journal is cited, in accordance with accepted academic practice. No use, distribution or reproduction is permitted which does not comply with these terms.
*Correspondence: Bernd J. Kröger, bernd.kroeger@rwth-aachen.de