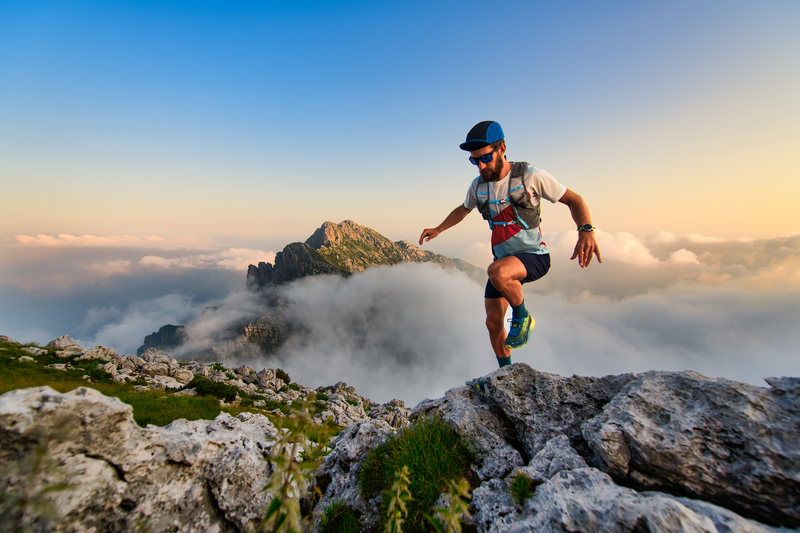
95% of researchers rate our articles as excellent or good
Learn more about the work of our research integrity team to safeguard the quality of each article we publish.
Find out more
ORIGINAL RESEARCH article
Front. Lang. Sci. , 01 February 2023
Sec. Language Processing
Volume 1 - 2022 | https://doi.org/10.3389/flang.2022.1065014
The human brain tries to process information as efficiently as possible through mechanisms like prediction. Native speakers predict linguistic information extensively, but L2 learners show variability. Interpreters use prediction while working and research shows that interpreting experience mediates L2 prediction. However, it is unclear whether advantages related to interpreting are due to higher working memory (WM) capacity, a typical characteristic of professional interpreters. To better understand the role of WM during L1 and L2 prediction, English L2 learners of Spanish with and without interpreting experience and Spanish monolinguals completed a visual-world paradigm eye-tracking task and a number-letter sequencing working memory task. The eye-tracking task measured prediction of verbal morphology (present, past) based on suprasegmental information (lexical stress: paroxytone, oxytone) and segmental information (syllabic structure: CV, CVC). Results revealed that WM mediates L1 prediction, such that higher WM facilitates prediction of morphology in monolinguals. However, higher WM hinders prediction in L2 processing for non-interpreters. Interestingly, interpreters behaved similarly to monolinguals, with higher WM facilitating L2 prediction. This study provides further understanding of the variability in L2 prediction.
Humans constantly seek patterns to predict the future. Prediction has attracted attention among psycholinguists, who debate what it really means (see Kaan and Grüter, 2021 for a discussion). We define prediction as the unconscious pre-activation of information (e.g., phonological, morphological, syntactic, or semantic) before it is available in the input. This mechanism facilitates perception by presensitizing relevant mental representations and is mostly unconscious (Bar, 2007). L1 prediction depends on task factors (e.g., frequency, speech rate, preview time, explicitness) (Huettig and Guerra, 2019) and participant factors (e.g., age, processing speed, working memory, literacy) (see Huettig, 2015, for a review). In addition, L2 prediction depends on prior prediction experience (Lozano-Argüelles et al., 2020) and language experience (L1 transfer: Dussias et al., 2013; L2 proficiency: Sagarra and Casillas, 2018). We explore additional explanations for L2 prediction variability in terms of prior prediction experience and individual cognitive differences, such as working memory (WM). Prediction is beneficial for processing efficiency, adaptation, and learning (Kaan and Grüter, 2021). Hence, understanding how different factors modulate L2 prediction provides a window into how the human mind learns and adapts to novel circumstances.
Previous research shows that prediction experience via interpreting enhances L2 morphological prediction (Lozano-Argüelles et al., 2020). However, it is unclear whether L2 prediction benefits from additional experience making predictions during interpreting or from the superior WM characteristic of interpreters (Dong and Cai, 2015). We investigated the role of WM on prediction in interpreters and non-interpreters because both prediction and interpretation involve the temporary retention of incoming information to activate long-term memory representations that will allow the rapid construction of new information. In addition, we examined the prediction of suffixes, following evidence that higher WM learners process inflectional morphology more native-like than lower WM ones, which suggests that attention to inflectional morphology may be cognitively taxing (see Sagarra, 2021 for a review). However, it is unclear whether WM only mediates L2 processing or whether it also modulates L2 prediction. The only two studies investigating the effects of WM on L2 prediction (Sagarra and Casillas, 2018; Perdomo and Kaan, 2019) do not find an association between WM and L2 prediction abilities, but they only analyzed a specific time point, rather than the progression of prediction over time. We address this limitation by analyzing the relationship between WM and eye movements over a fixed time course, using growth curve analysis. Although images are common in the visual-world paradigm, written words were selected for this experiment due to the low imageability of target words. Previous research shows that written words are suitable for research focused on the phonological representation of words (Huettig and McQueen, 2007).
Spoken word recognition involves the automatic activation of word forms based on segmental (vowels and consonants) and suprasegmental information (prosodic information such as tone or stress) (Soto-Faraco et al., 2001). Phonetic information in auditory speech helps listeners to build predictions about word endings. For example, native speakers show robust prediction strategies based on segmental and suprasegmental information (Roll et al., 2015, 2017; Sagarra and Casillas, 2018), but L2 prediction presents variability. Some studies show that L2ers can use tonal information to predict suffixes (Schremm et al., 2016), while others show the opposite (Gosselke Berthelsen et al., 2018).
Regarding suprasegmentals, studies show that Swedish and Spanish speakers use suprasegmental information to predict morphology. Swedish natives use the tone instantiated in a word's stem to predict both verbal (Söderström et al., 2012; Roll et al., 2015) and nominal (e.g., number: bilaccent1-en, “car”; bilaccent2-ar, “cars”) suffixes (Roll et al., 2010, 2013; Söderström et al., 2015). Spanish natives use lexical stress to predict verbal morphology (tense) (Sagarra and Casillas, 2018).
Contrary to the robust L1 results, L2 studies produce mixed findings (Grüter and Kaan, 2021). On the one hand, beginning German learners of Swedish do not use tone instantiated in the stem to predict number morphology (Gosselke Berthelsen et al., 2018). On the other hand, advanced L2 Swedish learners of non-tonal L1 backgrounds use tone to predict verbal morphology indicating tense (Schremm et al., 2016), despite the lack of explicit training and the absence of tone in their L1. In the same vein, advanced, but not beginning, L2 learners of Spanish use stress to predict verbal morphology when the first syllable contains a coda (CVC) (Sagarra and Casillas, 2018). Finally, beginning L2 learners improve their predictive processing (reflected in shorter reaction times and increased accuracy) after playing with a digital game aiming to strengthen the association between tones and suffixes in Swedish (Schremm et al., 2017). ERP data also suggest that short-term training increases predictive processing in low to intermediate learners of Swedish (Hed et al., 2019). However, these findings must be taken with caution due to the lack of a control group.
Concerning segmental information, Swedish speakers use both type frequency (occurrence of a pattern) and token frequency (occurrence of a particular unit) of the first two segments of a word to predict number morphology (singular/plural) (Roll et al., 2017). Similarly, Spanish natives use the syllabic structure (CV/CVC) of the first syllable to facilitate verbal morphology prediction [present/past; e.g., FIRma “(s)he signs”; firMÓ “(s)he signed”] (Sagarra and Casillas, 2018). The same patterns are found in L2 prediction based on segmental information. Advanced L2 speakers of Spanish benefit from the presence of a coda (CVC) in the first syllable of a word and use it predictively, whereas they did not predict the word ending when the coda is absent (CV) in the first syllable, regardless of proficiency (Sagarra and Casillas, 2018).
The studies presented thus far provide evidence that prosody plays an important role in how natives process language predictively and that advanced L2 speakers also use prosody predictively but to a lesser extent. However, these studies have not determined which factors modulate the prediction of morphological suffixes based on prosodic information. The present paper contributes to this growing area of research by analyzing the role of interpreting experience and WM as mediating factors in predictive processing. We explore these two factors in the following sections.
Interpreters' sustained exposure to the highly demanding task of interpreting offers a unique opportunity to investigate the adaptive mechanisms of bilingual processing. Professional interpreting requires an intensive cognitive and linguistic effort. Therefore, interpreters' cognitive adaptations go beyond the effects of L2 proficiency and exposure, showing how stringent processing conditions can drive adaptations in the bilingual mind (García, 2019). For instance, neural data indicate that extensive practice with interpreting triggers a similar brain network as that found in dense code-switchers, whose processing is characterized by the recruitment of a different neural network than the networks associated with single or dual language activation (Calabria et al., 2018). This reveals that interpreting is an extreme form of bilingual language control related to domain-general cognitive resource management (Hervais-Adelman and Babcock, 2020).
Some models of interpreting propose prediction as an optional step during simultaneous interpreting (Moser-Mercer, 1978) or as one of the strategies that allow one to better cope with a high cognitive load (Dong and Zhong, 2019). In their theory of prediction in interpreting, Amos and Pickering (2020) claim that interpreting is an ecologically unique context for investigating prediction because of the obvious advantages of anticipating information while simultaneously interpreting. According to their account, interpreters use semantic, syntactic, and phonological prediction to facilitate rapid and accurate comprehension of the speaker. Predictions are initially made through the production system in the source language, and the representation of the predicted lexical item automatically activates its translation equivalent in the target language. Such is the relevance of prediction during interpreting that most simultaneous interpreting courses include exercises to train prediction (Li, 2015). Student interpreters complete cloze tests (written and oral) where they are asked to fill in gaps based on contextual information and knowledge of collocations. Prediction also takes place during consecutive interpreting. When comparing interpreters' prediction while reading for comprehension and reading to interpret in the consecutive mode, prediction effects are stronger when the interpreters need to interpret, although enhanced prediction disappears when the cognitive load is high. These results indicate that interpreting increases prediction experience both during comprehension (in the source language) and production (in the target language) (Zhao et al., 2022).
Interpreters' additional practice with prediction offers a unique opportunity to explore the role of increased prediction experience on L2 processing. Indeed, interpreting enhances the use of prediction in L2 processing. Interpreters make faster predictions of verbal morphology than non-interpreter L2 speakers matched in L2 proficiency (Lozano-Argüelles et al., 2020). However, it is still unclear whether factors other than additional prediction experience could also explain mixed results in L2 prediction. We compare how WM mediates the prediction of morphology in interpreter and non-interpreter L2 learners and monolinguals to better understand how cognitive resources –measured via WM capacity–support L1 and L2 prediction, and whether interpreting experience drives processing changes in bilinguals.
WM is central to cognition. It allows many species to act beyond the here and now by maintaining information temporarily, while selectively attending to other information to support decision-making and guide actions. Numerous WM models and definitions have been proposed since Locke's first distinction between “contemplation” (currently processing) and storage in 1960 (Cowan, 2017). Some WM models conceive of WM as activated long-term memory (see Cowan et al., 2021, for a review), whereas others view WM as a separate system that interacts with long-term memory. The latter models view WM as: (1) a multi-component system comprising limited-capacity domain-specific components (verbal, visuospatial) that work together to support task performance and that are controlled by a domain-general central executive (see Baddeley et al., 2021, for a review), or a domain-general executive memory module (Logie et al., 2021; Vandierendonck, 2021); or (2) a set of knowledge in specific domains acquired through intensively practiced skills (Ericsson and Kintsch, 1995).
For some scholars, WM capacity refers to the ability to control one's attention in the face of interference or distraction (Engle, 2002, 2018). As a measure of attentional control, WM capacity has been associated with a vast array of cognitive tasks that require simultaneous processing and maintenance of information. Relevant to the current study, it remains unclear whether WM affects L2 processing of inflectional morphology or not. Some studies reveal that high WM span (operationalized as “capacity”) yields more native-like L2 verbal morphosyntactic processing at lower (e.g., Sagarra, 2013), and higher proficiency (e.g., Reichle et al., 2013). In contrast, other studies show no WM effects in higher proficiency learners (e.g., Foote, 2011; Armstrong et al., 2018). We explain this inconsistency in terms of language experience with the L2 (the advanced learners of the studies showing no WM effects were more advanced than those revealing WM effects) and the L1 (WM effects are more prominent in learners whose L1 is more different from the L2).
Interpreting is a cognitively demanding task. WM allows one to hold in memory the incoming message while processing it to produce a translation in the target language. Dong and Zhong (2019) propose an interpreting model based on language control and processing control, and WM is essential for both types of control. The cognitive complexity of interpreting gave rise to the “interpreter advantage hypothesis,” according to which the task-specific cognitive abilities developed by interpreters can be generalized to more efficient linguistic and executive skills during non-interpreting activities (García, 2014).
Extensive practice with interpreting is associated with an advantage in WM span (Padilla et al., 1995; Christoffels et al., 2006; Signorelli et al., 2011). Some studies have failed to find this interpreter advantage in WM (Chincotta and Underwood, 1998; Liu et al., 2004). However, Dong and Zhong (2019) pointed out that this could be due to methodological issues in the above-mentioned studies, such as a low sample pool, participant groups not matched in age, or not enough training or experience with interpreting. Importantly, longitudinal studies show that interpreting training and experience, instead of mere exposure to the L2, train different memory components. Simultaneous interpreting training yields enhancement in verbal short-term memory (while translation training or training in a variety of non-language subjects does not have memory benefits) (Babcock et al., 2017). Nonetheless, other studies have found that both translation and interpreting training enhance verbal WM (reading span), but not short-term memory (digit span) (Nour et al., 2020). These different results might be related to the length of training and employing different WM tasks (Nour et al., 2020).
Because prediction involves contrasting incoming input with experiences stored in memory, it is reasonable to hypothesize that WM mediates predictive processing. Indeed, (Huettig and Janse, 2016) observed that higher WM yielded stronger morphosyntactic predictions. In contrast, Otten and Van Berkum (2009) found that native speakers can predict upcoming words (both low and high WM span), but only low WM natives show an additional effort when processing in the unexpected condition. This additional effort is possibly related to an increased processing load while trying to resolve the prediction error or to the inability to suppress the original prediction. Differences between Otten and Van Berkum (2009) and Huettig and Janse (2016) could be due to using different techniques (eye-tracking and EEG respectively), but also to the sentences measuring prediction. Otten and Van Berkum (2009) included more distance between the cue and the outcome, providing more time to generate predictions. This additional time could have mitigated differences between low and high WM listeners. Kukona et al. (2016) included a reading-span task and a visuospatial memory task but found no WM effects on semantic prediction. Importantly, studies including a WM test are inconclusive. For instance, Sagarra and Casillas (2018) found WM effects on a gating task, but no WM effects on an eye-tracking task (see Perdomo and Kaan, 2019, for similar results in an eye-tracking task).
Collectively, these studies support the claim that WM is a crucial cognitive component for L1 and L2 processing, speech perception, and interpreting practice. However, the relationship between WM and prediction remains unclear. Huettig and Janse (2016) suggested that WM mediates the prediction of nouns based on morphological cues, and Perdomo and Kaan (2019) indicated that WM does not affect the prediction of syntax based on prosody. Sagarra and Casillas (2018) found that WM was irrelevant for predicting morphology based on prosody during an online task, but found a marginal effect of WM during an offline task. The difference between online and offline findings highlights the need to continue investigating the role of WM during the prediction of morphology. This issue is crucial to fully understand how cognitive resources link prosodic and morphological information to make predictions.
Prosody is key during speech comprehension, influencing different linguistic representation levels. The present study examines syllabic structure and stress in the initial syllable as a cue to predict a word's suffix. In Spanish, lexical stress and syllabic structure are linked to the number of competitors. Paroxytone words (stressed on the penultimate syllable) are associated with both present and past tenses and with a higher number of competitors than oxytone words (stressed on the last syllable), associated only with past tense; in turn, CV syllables also activate more competitors than CVC syllables. Related to prediction, several types of prosodic information have been identified as cues for prediction such as English, German, and Japanese intonation (Japanese: Nakamura et al., 2012; English: Perdomo and Kaan, 2019; German: Foltz, 2021), Swedish tones (Roll et al., 2015), and Spanish stress (Sagarra and Casillas, 2018), the focus of the present study.
Lexical stress, the prominence of a syllable in relation to other syllables in a word, distinguishes meaning both in English (PREsent vs. preSENT) and in Spanish (PApa “potato” vs. paPÁ “dad”). English is described as a stress-timed language with time intervals between stressed syllables remaining relatively stable. In contrast, Spanish is described as a syllable-timed language where both stressed and unstressed syllables are stable, displaying roughly the same duration and vowel quality patterns. Moreover, English and Spanish natives use stress differently for lexical access. While a prosodically matched prime facilitates perception and a mismatched prime hinders perception for Spanish monolinguals (Soto-Faraco et al., 2001), English monolinguals are not affected by mismatched primes (Cooper et al., 2002). This means that Spanish natives subconsciously utilize lexical stress to decrease the number of competitors during lexical access, while English natives possibly rely more strongly on other cues such as vowel reduction. Failing to detect these distinctions could explain why English L2 speakers of Spanish experience difficulties when perceiving (Face, 2005, 2006) and producing (Lord, 2007) stress.
Both Spanish and English allow open and closed syllables. Importantly, open syllables (CV), as opposed to syllables with a coda (CVC), are universally preferred (Jakobson, 1968; Hyman, 1975). Because of this bias toward CV syllables, CVC syllables are considered as marked in English and Spanish both acoustically (Hahn and Bailey, 2005) and articulatorily (Côté, 1997). Relevant to our study, the syllabic structure in the first syllable of a word is used to reduce lexical competitors (Cholin et al., 2006). This is also reflected during predictive processing, where initial segments allowing fewer endings with a higher frequency produce more robust pre-activation effects (Roll et al., 2017).
Prior research shows that L2 proficiency and L1–L2 similarity shape L2 prediction of information. However, it is unclear why even at advanced proficiency levels some L2 learners still cannot make predictions (Hopp, 2015). Lozano-Argüelles et al. (2020) showed that interpreting experience also enhanced L2 prediction, but findings were confounded because the study did not address the role of differences in cognitive resources. Research shows that higher WM capacity enhances L1 prediction of nouns based on morphological information, but it does not impact L1 and L2 prediction of syntax based on prosodic cues. Importantly, research on L1 and L2 prediction of morphology based on prosody showed inconclusive findings with null effects of WM in an online task and marginal effects on an offline task. To understand the role of WM in pre-activating morphology, we investigate the relationship between WM and prediction of verbal morphology in L2 speakers of Spanish with and without interpreting experience and Spanish native speakers. This issue is key in clarifying: (1) how cognitive resources support L1 and L2 prediction of morphology and (2) how WM and interpreting experience independently contribute to L2 prediction. We conducted a visual-world eye-tracking study, to detect whether speakers' look toward the target word upon hearing the predictive cue (lexical stress) but before hearing the suffix.
Our first research question is: does WM mediate monolinguals' ability to predict suffixes based on stress information? We expect that monolinguals will be able to utilize stress-suffix associations and that higher WM will facilitate the use of such associations. This expectation goes hand in hand with studies showing that prediction between words correlates with WM capacity in native speakers (Huettig and Janse, 2016). Our study is within words, but we still believe that we will observe WM effects. In our case, the WM effects will not be an artifact of the distance between the agreeing constituents but of frequency. We speculate that all monolinguals will activate the present and past verbs in their mental lexicon after reading them in the visual-world task and will maintain both options easily accessible. However, because of their lifelong use of Spanish and experience anticipating verb endings, natives will realize that unstressed and CV initial syllables are more frequent in Spanish than stressed and CVC initial syllables. Having higher or lower WM will affect how they react to suprasegmental frequency information. Natives with higher WM span will prioritize the most frequent option and will look at the target more or faster in paroxytone and CV conditions than oxytone and CVC conditions. In contrast, lower WM natives will react equally to all conditions, independently of stress and syllabic structure frequency. If this hypothesis is true, (a) the difference between paroxytone-CV and oxytone-CVC conditions should be smaller in listeners with less use of Spanish (both L2 learner groups), and (b) the learners with more prediction experience (interpreters) should behave closer to the monolinguals than those with less anticipating experience (non-interpreters).
The second research question is: does WM mediate advanced L2 learners' ability to predict suffixes based on stress information? We hypothesize that advanced non-interpreter L2 learners will be able to use stress-tense suffix associations in all conditions except in CV conditions and that WM will not affect their ability to utilize such associations. Advanced L2 learners still have limited knowledge, use, and prediction experience in Spanish. As a result, they will be worse than monolinguals at identifying what type of stress and syllabic structure is more frequent in Spanish. This hypothesis is in line with Sagarra and Casillas (2018). They found that advanced English learners of Spanish can use stress-tense suffix associations with stressed/unstressed and CVC–but not CV–initial syllables, and that WM does not affect their ability to use such associations. Similarly, Perdomo and Kaan (2019) reported no WM effects in L2 learners of lower proficiency levels.
Finally, the third research question is: does WM mediate interpreters' ability to predict suffixes based on stress? We predict that advanced interpreter L2 learners will be able to utilize stress-tense suffix associations in all conditions except in CV conditions, and that WM will modulate how they use such associations. We believe that they will behave more similarly to monolinguals than the non-interpreter learners. Specifically, higher WM will facilitate verbal prediction with the most frequent stress type (paroxytones). Our hypotheses are in line with prior studies showing that simultaneous interpreters do not use stress-suffix tense associations with CV conditions, predict more native-like than non-interpreter L2 learners (Lozano-Argüelles et al., 2020), and have higher WM span than non-interpreters (Dong and Cai, 2015).
The study included three groups of participants: 25 Spanish monolinguals and 57 L1 English–L2 Spanish late bilinguals, 25 non-interpreters, and 22 interpreters. We initially collected 30 non-interpreters. Because of differences in L2 proficiency, we removed the five non-interpreter participants with the lowest L2 proficiency. The monolinguals grew up in a monolingual region in Spain and, despite formal exposure to English, they reported limited proficiency and no regular exposure to English in their daily lives. The L2 learner groups grew up in an English monolingual environment, started learning Spanish after puberty in a formal setting, and most of them spent time in a Spanish-speaking country.
Participants completed the following tests in this order: a background questionnaire (5 min), a proficiency test (L2 speakers; 15 min), an eye-tracking task (15 min), a phonological short term memory task (10 min), a WM task (10 min), and a production task (15 min). All tasks were completed during one individual session of ~1 h and 15 min. The present study focuses on the WM and eye-tracking tasks.
The background questionnaire gathered information about the participant's L1 and L2 language acquisition, other languages spoken, age, L2 age of acquisition, time spent in an L2 country, schooling languages, and percentage of weekly time speaking each language. The interpreters were also asked about their professional training, years of work experience, and professional training and certifications.
Participants were between 18 and 76 years old (monolinguals: M = 30.52, SD = 10.00; non-interpreters: M = 27.96, SD = 4.69; interpreters: M = 43.23, SD = 13.12). Because of the difficulty in finding interpreters that met the requirements in the US (many are native speakers of Spanish), we decided to include older interpreters who were professionally active. Only one interpreter in the sample is above 65 years old. Both learner groups used their L2 on a regular basis. We conducted two one-sided tests of equivalence (TOST), with a Cohen's D of 0.3 to test moderate effects (Lakens, 2017), to test that both learner groups had equivalent L2 exposure (non-interpreters: M = 27.24, SD = 12.91; interpreters: M = 31.59; SD = 14.59, % of time per week; interpreters vs. non-interpreters: t(42.33) = 0.05, p = 0.521).
The non-interpreters did not have professional experience or training in either translation or interpreting techniques, whereas interpreters were formally trained through masters or professional interpreting certifications, and had worked as interpreters for at least 2 years (M = 14.16, SD = 9.23). All interpreters worked mostly in the simultaneous interpreting mode (interpreter translates while the speaker is talking) and some (n = 5) combined it with the consecutive mode (the interpreter starts the translation after the speaker finishes a speech section).
The L2 proficiency test was administered only to the L2 speaker groups and consisted of a simplified version of the Diploma de Español como Lengua Extranjera that included 56 multiple-choice questions testing grammar and vocabulary. Participants received 1 point per correct response and 0 per incorrect answer. They needed a minimum of 40 points in order to be included in the study. This test is similar to the widely cited Montrul and Slabakova (2003) and thus allows for comparability with other SLA studies.
One TOST shows that groups had equivalent proficiency in their L2 as shown in a similar range, mean and standard deviation of the scores (non-interpreters: range = 40–53, M = 46.60, SD = 3.83; interpreters: range = 40–54, M = 48.86; SD = 4.32, points in DELE test, max 56; interpreters vs. non-interpreters: t(42.36) = 1.89, p = 0.07).
Eye-movements were recorded with the EyeLink 1000 Plus desktop mount (SR Research), with a sample rate of 1k Hz, the spatial resolution 32° horizontal, 25° vertical, and an averaged calibration error of 0.25°–0.5°. The experiment was displayed to participants on a BenQ XL2420TE monitor using a resolution of 1,920 × 1,080 pixels and they received the audio through Sol Republic 1601-32 headphones.
The eye-tracking task comprised 66 sentences: 18 were practice, 16 experimental, and 32 fillers. Supplementary Appendix 4 includes the experimental sentences. All sentences were between 5 and 7 words long and all word pairs shared the initial syllable. Word frequencies were calculated using the dictionary of frequencies LEXESP (Sebastián-Gallés et al., 2000), and t-tests revealed no significant differences in frequency between the two conditions for any of the experimental or filler contrasts: tense (experimental; cambia-cambió), t = 1.865, p = 0.82; number (col-coles “cauliflower-cauliflowers”), t =0.364, p = 0.727; semantics (mar-marco “sea-frame”), t = 1.222, p = 0.268. All sentences were grammatical for both target and distractor and shared the first syllable, making the stress (or lack thereof) in the first syllable the only predictive cue. Half of the targets had a stressed first syllable (CAMbia, “he/she changes”) and half had an unstressed first syllable (camBIÓ, “he/she changed”). Moreover, about half of the experimental words contained a rhotic or nasal coda in the first syllable (CVC cambia-cambió, “(s)he changes/changed”) and the other half of the target words did not (CV bebe-bebió, “(s)he drinks/drank”). The location of the target word on the screen was counterbalanced for the left and right sides of the screen. For both filler types, half of the targets contained a long initial vowel (monosyllabic words: mes, par), and half had a short initial vowel (disyllabic: meses, parque).
Participants were randomly assigned to one of two versions. Each version included one condition of each sentence (e.g., version 1: El vecino CAMbia la clave “The neighbor changes the password”; version 2: El vecino camBIÓ la clave “The neighbor changed the password”). Practice trials remained equal in both versions of the experiment. During the eye-tracking task, participants sat in front of a screen with their heads on a chin-rest, while wearing headphones. They performed a nine-point calibration and were instructed to select as fast as possible the word they heard in the sentence by pressing the right or left shift key on the keyboard. Bottom presses before the target word were not recorded. Every trial contained a drift correction, a fixation point displayed during 250 ms, the two words (target and distractor) on the left and right sides of the screen shown for 1,000 ms as familiarization phase, and the audio containing the sentence.
To assess participants' WM, we used the Letter-Number Sequencing test adapted from the Wechsler Adult Intelligence Scale test (WAIS) (Wechsler, 1997). This test has also been widely used for non-clinical research with native and non-native speakers. In this test, participants listened to a series of numbers and letters in their L1 and were asked to remember and organize them, typing first the numbers in ascending order and then the letters in alphabetical order. We administered an oral version of the test because the oral version is more common and demanding than the visual version (Mielicki et al., 2018), and because the eye-tracking task was also oral. There were two practice trials and 20 experimental series, increasing in length from three to eight items (letters and numbers). They received 1 point per correct series (correct numbers/letters and order) and 0 per incorrect series (incorrect numbers/letters or order). Due to technical failure, WM data from one participant was missing.
The Letter-Number Sequencing test is considered a complex span test because participants must both reorder the alphanumeric characters (processing) and store them for retrieval (Conway et al., 2005; Hill et al., 2010). Shelton et al. (2009) reported that the Letter-Number Sequencing test is highly correlated with other WM tests usually employed in laboratory settings. We selected this test over other complex span tests like the Operation Span because the Letter-Number Sequencing test allows for a more direct comparison between L1 and L2 speakers due to its non-linguistic nature, avoiding potential confounds due to insufficient L2 proficiency or differences in word frequency (see Sanchez et al., 2010, for evidence that performing a WM test in an L2 yields worse results than in the L1). We chose this test over other non-linguistic tests because the Letter-number test is completely language independent. Other tests like the operation span test require participants to remember real words of a specific language.
Three TOSTs show that the three groups did not differ regarding WM capacity (monolinguals: M = 9.16, SD = 1.89; non-interpreters: M = 9.00, SD = 2.15; interpreters: M = 10.27; SD = 2.98): monolinguals vs. interpreters: t(34.69) = 0.49, p = 0.69; monolinguals vs. non-interpreters: t(47.22) = 0.78, p = 0.22; interpreters vs. non-interpreters: t(37.72) = 0.639, p = 0.737. This ruled out the possibility of a group performing better than another because of superior WM.
We used the software DataViewer (SR-Research) to downsample to 50 ms bins and extract the eye-tracking data. Working memory data were extracted from ePrime (Version 2.0.10). All data cleaning and statistical analyses were performed with R [Version 4.0.3; R Core Team (2019)]. Models were fit with the package lme4 (Bates et al., 2015) and the multcomp package (Hothorn et al., 2008) served to conduct post-hoc comparisons amongst learner groups. Fixations toward the target were analyzed using the empirical-logit growth curve analysis (Mirman, 2014). The model represented the probability of looks toward the target over the time course. We selected the time window between 200 ms before the offset of the target syllable until 600 ms after. This allowed us to capture the time frame when fixations toward the target started to increase above chance. The model was centered 200 ms after the offset of the target syllable, the approximate amount of time the human mind takes to direct looks toward the target after having heard a stimulus (Salverda et al., 2014). We modeled the time course with the linear, quadratic, and cubic orthogonal polynomials and used the empirical logit (Barr, 2008) to transform binary responses (looks toward target or distractor). We included group (monolinguals, non-interpreters, interpreters), lexical stress (paroxytone, oxytone), syllabic structure (CV, CVC), and WM (0–20) as fixed effects for all time terms. We sum-coded lexical stress and syllabic structure. Parameter estimates represent effect sizes of change from CV to CVC syllables and paroxytone to oxytone stress. WM was standardized to have a mean of 0 and a standard deviation of 1. We included subject and item as random intercepts for all time terms and also by-participant random slopes for syllabic structure and lexical stress on all time terms. The baseline group predictor was the monolinguals and model parameters in the growth curve indicated differences between the learners and the monolingual group. We used nested model comparisons to assess main effects and higher-order interactions. We used pairwise comparisons to contrast non-interpreters from interpreters. Finally, only significant main effects and interactions are reported.
Polynomial effects function independently, therefore the effects on each polynomial time term are interpreted differently (Mirman, 2014). Graphs allow us to better understand the results. For example, when the interpreters' curve crosses the chance level (i.e., 50% probability) earlier than the non-interpreters' curve (i.e., the curve is displaced to the left), we can assume that interpreters start predicting earlier. When one curve crosses the intercept (in our case, the onset of the second syllable) above other curves, that group predicts target-like more often (which we refer to as “predicted more”). Finally, when one of the curves is steeper, we interpret the rate of change as more abrupt, leading to faster prediction (see, Mirman et al., 2008 for more details).
We first report significant results for the monolingual group and continue by comparing them with the learner groups. The growth curve analysis model intercept corresponds with the log odds of the baseline group (monolinguals) looking at the target averaging across all conditions (time course, stress, syllabic structure) at average working memory. The linear, quadratic and cubic time terms captured the sigmoid shape of the function (γ10 = 5.42; SE = 0.75; t = 7.26; p < 0.001; γ20 = −1.37; SE = 0.40; t = −3.46; p < 0.001; γ30 = −1.68; SE = 0.30; t = −5.64; p < 0.001). The full model summary is included in Supplementary Appendices 1–4. Figure 1 shows that, overall, monolinguals (green line) are more likely to fixate on targets under all conditions and that a paroxytonic word with CV structure (LAva) results in fewer target fixations. Crucially, at the target syllable onset–before morphological information is available–the monolinguals are already fixating on targets above chance in all conditions [CV paroxytone: 0.73 CI: (0.64, 0.80); CVC paroxytone: 0.84 CI: (0.79, 0.88); CV oxytone: 0.82 CI: (0.76, 0.87); CVC oxytone: 0.88 CI: (0.84, 0.92); see Table 1].
Figure 1. Growth curve estimates of target fixations as a function of lexical stress and syllable structure per group (M, monolinguals; NIN, non-interpreters; IN, interpreters) during the analysis window. Symbols and lines represent model estimates at mean WM, and the transparent ribbons represent ±SE. Empirical logit values on the y-axis correspond to proportions of 0.12, 0.50, 0.88, and 0.98. The thick horizontal white line represents the 50% probability of fixating on the target. The thick vertical white line indicates 200 ms after the offset of the target syllable.
Table 1. Model estimates at mean working memory for probability of target fixations ±SE at 200 ms after the target syllable offset.
There was a main effect of syllabic structure on the linear term (χ2(1) = 4.4, p =0.037) indicating that a change from CV to CVC increased the steepness of the slope (γ11 = 0.819; SE = 0.38; t = 2.18; p = 0.029). Also, there was a main effect of lexical stress on the quadratic time term (χ2(1) = 4.4, p = 0.036), showing that a change from paroxytone (stressed initial syllable) to oxytone (unstressed initial syllable) increased the steepness of the slope (γ22 = 0.57; SE = 0.24; t =2.38; p = 0.017) and decreased the bowing of the vertices (i.e., turning points; γ32 = −0.57; SE = 0.16; t = −3.59; p < 0.001). This indicates that monolinguals started to fixate on oxytone targets earlier in the time course than on paroxytone targets and that they showed a higher prediction rate at the intercept for oxytonic targets (see Figure 2. Red curves, in red, cross chance level earlier than blue curves). Regarding lexical stress and syllabic structure, the significant interaction on the cubic term (γ35 = −0.49; SE = 0.23; t = −2.17; p = 0.030) indicated sharper vertices with CV paroxytones (BEbe). This suggests that monolinguals fixated on CV paroxytones later in the time course, but did so at a faster rate.
Figure 2. Growth curve estimates of target fixations as a function of lexical stress and WM for monolinguals during the analysis window. Lines represent model estimates at −1, 0, and 1 standard deviations of WM. Empirical logit values on the y-axis correspond to proportions of 0.12, 0.50, 0.88, and 0.98. The thick horizontal white line represents the 50% probability of fixating on the target. The thick vertical white line indicates 200 ms after the offset of the target syllable.
The interaction of WM and stress on the linear (χ2(1) = 5.4, p = 0.02) and quadratic time terms (χ2(1) = 5.7, p = 0.017) showed that monolinguals had a more bowed curve in paroxytone words (γ26 =-0.86; SE = 0.25; t = −3.45; p < 0.001). That is, higher WM monolinguals pre-activatied suffixes earlier than lower WM monolinguals in paroxytone words, but WM did not affect the prediction of oxytone words (in Figure 2, oxytone curves, in red, are closer together, while paroxytone curves, in blue, indicate WM differences).
The interaction of WM and syllabic structure on the linear term (χ2(1) = 4.2, p = 0.041), indicated that higher WM monolinguals predicted earlier than lower WM monolinguals with CVC initial syllables (CAMbia/camBIÓ; γ18 = 0.23; SE = 0.11; t = 2.11; p = 0.035). This can be seen in Figure 3, the red dotted line crosses chance level (horizontal thick line) earlier in the time course than the rest of curves. This interaction points out that although WM capacity did not modulate prediction in words with CV initial syllables (BEbe/beBIÓ), all monolinguals predicted suffixes by the offset of the first syllable (all curves in Figure 3 cross chance level before the onset of the second syllable marked by the vertical thick line).
Figure 3. Growth curve estimates of target fixations as a function of syllabic structure and WM for monolinguals during the analysis window. Lines represent model estimates at −1, 0, and 1 standard deviations of WM. Empirical logit values on the y-axis correspond to proportions of 0.12, 0.50, 0.88, and 0.98. The thick horizontal white line represents the 50% probability of fixating on the target. The thick vertical white line indicates 200 ms after the offset of the target syllable.
Finally, regarding prediction at first syllable offset, monolinguals anticipated verb endings above 80% rate for all conditions, with the exception of CV paroxytones (BEbe; see Table 1). Within the remaining conditions, the one that yielded greater prediction was CVC oxytone (camBIÓ; CV paroxytone: probability = 0.726; LB = 0.638; UB = 0.799, CV oxytone: probability = 0.821; LB = 0.757; UB = 0.871, CVC paroxytone: probability = 0.844; LB = 0.792; UB = 0.885, CVC oxytone: probability = 0.882; LB = 0.838; UB = 0.916).
There was a main effect of group on the quadratic term, showing that monolinguals predicted suffixes significantly more than non-interpreters (γ23 = 1.82; SE = 0.47; t = 3.87; p < 0.001). The model also showed an interaction of syllabic structure and lexical stress for non-interpreters (γ07 = 0.89; SE = 0.28; t = 3.23; p = 0.001), indicating that adding a coda to the paroxytone condition was more beneficial for non-interpreters than for monolinguals. Moreover, there was an interaction of lexical stress × group × WM on the linear (χ2(2) = 6, p = 0.049) and quadratic time terms for non-interpreters (χ2(2) = 7.1, p = 0.028). When compared to monolinguals and averaging across syllabic structures, lower WM non-interpreters predicted earlier than higher WM non-interpreters, but only for the oxytone condition (γ010 = 0.70; SE = 0.32; t = 2.15; p = 0.031) (see Figure 4). WM did not make a difference for the paroxytone condition.
Figure 4. Growth curve estimates of target fixations as a function of lexical stress and WM for non-interpreters during the analysis window. Lines represent model estimates at −1, 0, and 1 standard deviations of WM. Empirical logit values on the y-axis correspond to proportions of 0.12, 0.50, 0.88, and 0.98. The thick horizontal white line represents the 50% probability of fixating on the target. The thick vertical white line indicates 200 ms after the offset of the target syllable.
Like the non-interpreters, the interpreters predicted suffixes at a lower rate than monolinguals (main effect of group; γ24 = 1.61; SE = 0.48; t = 3.36; p < 0.001). Then, the interaction of syllabic structure and lexical stress on the linear time term (γ28 = −0.67; SE = 0.28; t = −2.38; p = 0.017) and the cubic time term (γ39 = 0.85; SE = 0.27; t = 3.05; p = 0.002), revealed that the slope was steeper for the interpreters' group in the CVC paroxytone condition (CAMbia). In other words, interpreters predicted suffixes later but at a faster rate in CVC paroxytones (see Figure 5). Also, the interaction of lexical stress × group (IN) × WM on the intercept (γ19 = −0.57; SE = 0.26; t = −217; p = 0.030), the linear term (γ37 = 0.68; SE = 0.30; t = 2.27; p = 0.023) and the quadratic term (γ210 = 0.77; SE = 0.30; t = 2.58; p = 0.010) showed a steeper slope in the paroxytone condition. In effect, higher WM interpreters wait longer to predict information than lower WM interpreters with paroxytones, but predict at a faster rate and reach the same prediction level by the offset of the target syllable. Overall, interpreters' WM curves are closer together, indicating that WM differences between high and lower capacity groups are smaller for interpreters than for monolinguals (see Figures 2, 5).
Figure 5. Growth curve estimates of target fixations as a function of lexical stress and WM for interpreters during the analysis window. Lines represent model estimates at −1, 0, and 1 standard deviations of WM. Empirical logit values on the y-axis correspond to proportions of 0.12, 0.50, 0.88, and 0.98. The thick horizontal white line represents the 50% probability of fixating on the target. The thick vertical white line indicates 200 ms after the offset of the target syllable.
The comparison of the two L2 groups yielded an interaction of lexical stress, group, and WM on the intercept (γ19 = 0.49; SE = 0.24; t = 2.09; p = 0.037), indicating that in the oxytone condition, high WM interpreters predicted earlier, while non-interpreters with lower WM started pre-activating earlier. Furthermore, the interaction of syllabic structure, lexical stress, and group on the linear time term (γ08 = 1.57; SE = 0.28; t = 5.61; p < 0.001) and the cubic time term (γ28 = −0.85; SE = 0.28; t = −3.07; p = 0.002) showed that interpreters had a steeper slope in CVC paroxytones (CAMbia) and non-interpreters had sharper vertices in CV paroxytones (BEbe).
Thus, interpreters predicted at a faster rate than non-interpreters in CVC paroxytones (CAMbia) and non-interpreters were faster than interpreters in the CV paroxytones (BEbe). Finally, both L2 groups predicted information similarly at the offset of the target syllable (non-interpreters: CV paroxytone: probability = 0.58; LB = 0.477; UB = 0.673, CV oxytone: probability = 0.73; LB = 0.656; UB = 0.80, CVC paroxytone: probability = 0.766; LB = 0.698; UB = 0.823, CVC oxytone: probability = 0.795; LB = 0.728; UB = 0.849; intepreters: CV paroxytone: probability = 0.55; LB = 0.45; UB = 0.65, CV oxytone: probability = 0.718; LB = 0.633; UB = 0.790, CVC paroxytone: probability = 0.750; LB = 0.678; UB = 0.811, CVC oxytone: probability = 0.780; LB = 0.708; UB = 0.838).
The purpose of the present study was to tease apart the effects of interpreting experience and WM on native and non-native speakers' ability to form stress-suffix tense associations. By providing an answer to this question, we gain a further understanding of the variability found in L2 prediction. Adult advanced English learners of Spanish with and without professional interpreting experience (interpreters, non-interpreters), as well as a Spanish monolingual control group, completed an eye-tracking visual world paradigm task. Participants listened to sentences in Spanish with verbs whose initial syllables varied in stress (stressed initial: BEbe, unstressed initial: beBIÓ) and syllabic structure (CV, BEbe; CVC, CAMbia). Results show that monolinguals predicted under all conditions, but the L2 groups were unable to predict with CV-paroxytones (BEbe). Our results are in line with the Unified Competition Model (see MacWhinney, 2018, for a review), showing that, like monolinguals, advanced learners rely more on reliable cues than available cues (Li and MacWhinney, 2013). With respect to WM and stress, our results show that suffix prediction is mediated by frequency. Higher WM span yielded earlier fixations (monolinguals) and faster fixations (interpreters) at targets in paroxytones than lower WM span. This finding shows that interpreters behave more closely to monolinguals than non-interpreters. In contrast, higher WM span produced later fixations at targets in oxytones than lower WM span in non-interpreter learners. These data suggest that all groups hold both verbal interpretations in memory, and that those with higher WM prioritize the word with the most frequent type of stress to a greater extent than their lower WM peers. This explains why higher WM non-interpreter learners take longer to predict oxytones, an option they had barely considered, than lower WM non-interpreter learners. Regarding WM and syllabic structure, higher WM monolinguals predicted target suffixes earlier with CVC than CV syllabic structure, because a CVC initial syllable activates fewer lexical competitors than a CV initial syllable, even though CV is more frequent than CVC in Spanish. This is so because L2 learners with and without interpreting experience lack Spanish knowledge and are limited to pre-activating suffixes in verbs with CVC initial syllables. Taken together, our findings show that WM, frequency, and the number of lexical competitors modulate how native and non-native speakers predict word endings.
The first research question asked whether WM mediates monolinguals' ability to use stress-tense suffix associations. The hypothesis that higher WM would facilitate target suffix prediction in the more common stress condition (paroxytone) was supported. The interaction of WM and lexical stress revealed that higher WM monolinguals predicted earlier than lower WM monolinguals in paroxytones, the most common stress type in Spanish. Our results are consistent with research showing that L1 and L2 morphological processing is cognitively taxing. In effect, Hartsuiker and Barkhuysen (2006) showed that low WM natives made more agreement errors than high WM natives when the cognitive load was increased.
On the other hand, against our initial expectation, the interaction between WM and syllabic structure showed that higher WM monolinguals predicted verb endings earlier with CVC initial syllables (CAMbia/camBIÓ, the syllabic structure yielding fewer lexical competitors) than with CV initial syllables (BEbe/beBIÓ), even though CV is more frequent than CVC in Spanish. One explanation is that, although adding a coda to the initial syllable reduces the number of lexical competitors, processing an additional segment is cognitively more costly. Another option is that our experimental design has manufactured this effect. According to Soto-Faraco et al. (2001), listeners use all available cues for lexical access that allow distinction between word pairs. In our experiment, the initial syllable in every word pair presented to participants differed in terms of lexical stress (i.e., suprasegmentally). Hence, participants might have favored attention to suprasegmental cues, over segmental cues.
In parallel to the aforementioned findings, there was a main effect of lexical stress and a main effect of syllabic structure, indicating that monolinguals predicted earlier in the oxytone (beBIÓ) and CVC conditions (CAMbia) because they activate fewer options than paroxytone and CV conditions. The model also showed an interaction between lexical stress and syllabic structure, such that monolinguals predicted CV paroxytones (BEbe) later but faster than the rest of the conditions. In Spanish, the paroxytone stress-pattern is the most frequent (Morales-Font, 2014), thus related to a high number of lexical competitors. These results show that cues related to fewer lexical competitors favor prediction and that, when more lexical competitors are present, prediction is delayed. The lexical stress and syllabic structure findings mirror prior research on L1 morphological prediction with prosodic cues (Söderström et al., 2012; Roll et al., 2015; Sagarra and Casillas, 2018).
The second research question explored whether WM mediates L2 learners' ability to use stress-tense suffix associations. The hypothesis that WM would not affect fixations to target suffixes in non-interpreter L2 learners was not supported. Results showed a significant interaction of lexical stress and WM, indicating that higher WM non-interpreter learners predicted oxytone targets later than lower WM non-interpreters. One explanation is that non-interpreter learners with high WM span prioritize less frequent types of lexical stress and need extra time when the unexpected option is the target one. Another explanation is that when the possibilities are reduced through a less common stress pattern, lower WM non-interpreters can make a prediction earlier. Higher WM non-interpreters contemplate more possibilities and take longer to make a decision. Our results contradict previous research showing no WM effects on L2 prediction (Sagarra and Casillas, 2018; Perdomo and Kaan, 2019). One possible explanation is the type of analyses conducted. While previous studies used linear mixed-effects models focusing on a specific time point, the present study used growth curve analysis. Growth curve analysis, a type of linear mixed-effects model, is particularly interesting for our data because it provides information about the trajectory of prediction over time, rather than limited information at one specific time point. Interestingly, Huettig and Janse (2016) also found a WM effect using a combination of principal component analysis and multiple regressions to assess the contribution of WM to prediction performance in an eye-tracking task. Alternatively, different types of WM tasks could also explain why some studies find WM effects, while others do not. Huettig and Janse (2016) included the non-word repetition task, the backward digit span, and the Corsi block task, Perdomo and Kaan (2019) chose the forward and backward digit span, and Sagarra and Casillas (2018), similar to the present study, selected the letter-number sequencing task. Future research should take into account differences in statistical analyses and WM tasks.
Furthermore, a group effect indicated that monolinguals predicted information more than non-interpreters. These findings can have two possible explanations. First, non-interpreters could be pre-activating less because they are less able to perceive the input as stressed or unstressed due to stress realization differences in English and Spanish, or because they have less knowledge of the stress-suffix tense associations due to insufficient L2 proficiency and use. However, the results of a gating task contradict this theory. The monolinguals and the non-interpreters completed a gating task (reported in Sagarra and Casillas, 2018, due to space limitations), following the eye-tracking task. In the gating task, participants read the same two verb pairs as in the eye-tracking task, listened to a sentence with verbs segmented at the offset of the first syllable [e.g., la persona dice can “the person says sing (only first syllable)”], and guessed one of the two verbs on the screen without hearing the suffix (see Sagarra and Casillas, 2018, for more information). Both groups were equally accurate regardless of stress and syllabic structure in the gating task, even though the learners predicted less than the monolinguals in the eye-tracking task. These results demonstrate that the learners' reduced prediction abilities were not due to inability to perceive Spanish stress or insufficient knowledge of stress-suffix tense associations. Furthermore, in a follow-up study, Sagarra et al. (under review) compared monolinguals and advanced English-Spanish learners to advanced Chinese-Spanish learners and found that all groups predicted suffixes above chance before hearing them, despite stress differences in Spanish, English, and Chinese. These findings reiterate that the learners of the present study did not predict less than monolinguals due to differences in the use of lexical stress during lexical access.
Based on the data from Sagarra and Casillas's (2018) gating task and Sagarra et al.s' (under review) Chinese-Spanish learners, we propose that the non-interpreter learners' poorer prediction abilities result from having less experience with Spanish than monolinguals. Our findings are consistent with prediction accounts indicating that L2 prediction is possible but more effortful than L1 prediction (Kaan, 2014), and with L2 processing accounts suggesting that morphological information is parsed in a less automatic way than in L1 processing (Jiang, 2004). Nonetheless, it is interesting to notice that non-interpreters use cues similarly to monolinguals. In Spanish, paroxytone words are more frequent (high cue availability), whereas oxytone words are less frequent and, hence, have fewer competitors (high cue reliability). In this study, both L1 and L2 speakers favor highly reliable cues over available cues, supporting the Competition Model (MacWhinney, 2018).
The third research question investigated whether extensive interpreting experience facilitates a person's ability to use stress-tense suffix associations. We expected that interpreters would show a correlation between WM and prediction abilities and that WM would have a stronger impact on conditions with more competitors (CV and paroxytone). This was partially supported. On the one hand, high WM interpreters waited longer to make a prediction but were faster than monolinguals in the paroxytone words. This indicates that interpreters' cognitive resources, similar to monolinguals, support prediction when cognitive load is higher (i.e., paroxytone words linked to more competitors).
We contemplate different reasons why interpreters' use of WM for prediction is closer to monolinguals than to non-interpreters. First, according to Dong and Zhong's (2019) model, processing control in interpreting is achieved through divided attention via coordination and WM. They propose that interpreting training enhances coordination ability (performing multiple tasks concurrently), WM capacity, and language processing efficiency. The possibiliby of WM capacity explaining our results seems unlikely because both interpreters and non-interpreters had comparable WM scores (both groups included a range of WM scores). Nevertheless, we cannot rule out that interpreters could have better coordination or language processing efficiency skills. Second, the positive effects of interpreting experience could be akin to WM training on L2 processing. WM training, although controversial (Redick, 2019), has been recently proposed as a promising avenue to facilitate L2 acquisition (Tsai et al., 2016). This alternative explanation is consistent with Francis and Nusbaum (2009). They propose that training enhances the use of WM through increased attention to informative phonetic cues. Third, interpreters pay a high price for mistakes and are used to focus on what is relevant and ignore what is not. This is in line with Engle's WM model, which conceives WM as an individual's ability to control attention in the face of distraction.
Hence, we believe that a combination of both variables, interpreting training, and L2 use, might explain why interpreters perform closer to monolinguals in the present study. However, other factors could contribute to explaining processing differences. Interpreters might have higher proficiency than non-interpreters. The simplified version of the DELE (Spanish proficiency test) could have failed to differentiate between the L2 groups at the top range of proficiency. Also, interpreters' increased experience switching between languages could have enhanced their inhibitory control and, in turn, improved their prediction abilities. Future research should consider these variables to further understand which factors mediate L2 prediction.
WM did not affect prediction under the rest of the conditions. This finding is consistent with our monolingual data, indicating that WM in natives and interpreters only contributes to predict information when the cognitive load is increased due to the presence of more lexical competitors (paroxytone words). Also, interpreters predicted information faster in CVC paroxytones (CAMbia) than non-interpreters, indicating that additional prediction experience via interpreting enhances prediction in the L2. This is consistent with studies showing superior prediction skills in interpreter L2 learners (Lozano-Argüelles et al., 2020). We have argued that the number of competitors explains differences in prediction ability both for L1 and L2 speakers. This is in line with research indicating that dense phonological neighborhoods slow down spoken word recognition in both L1 and L2 processing (Botezatu et al., 2022). Future research should explore phonological neighborhood density as a variable explaining the differences in prediction ability during spoken word recognition.
Besides the findings detailed above, a group effect revealed that interpreters' prediction rate was lower than monolinguals' prediction rate, indicating that L2 prediction is more effortful than native prediction. Again, this finding goes in line with research showing that L2 learners can predict information, but to a lesser extent than monolinguals (Kaan, 2014; Sagarra and Casillas, 2018; Lozano-Argüelles et al., 2020). Finally, interpreters started to predict later but at a faster rate than monolinguals in CVC paroxytones (CAMbia). Interpreters learn to delay their translation of the target language as part of their training (Gile, 1995). This strategy allows them to improve comprehension of the source text, but it is more cognitively demanding because of the accumulation of information in short-term memory. We hypothesize that this strategy is transferred to L2 prediction and that interpreters are cautious before committing to a prediction under conditions with more lexical competitors (i.e., paroxytone words). Taken together, these findings are crucial for WM models, advancing our knowledge about how additional prediction experience enhances the use of WM during L2 processing.
The goal of the present study was to tease apart the effects of interpreting experience and WM on L2 prediction. Advanced adult English learners of Spanish with and without interpreting experience and Spanish monolinguals performed an eye-tracking task with Spanish verbs with initial syllables varying in stress (BEbe, beBIÓ) and syllabic structure (BEbe, CAMbia), as well as a WM task. WM facilitated prediction when the cognitive load was higher (more lexical competitors) for monolinguals and interpreters and WM affected prediction under less cognitively demanding conditions (fewer lexical competitors) in the case of non-interpreters. Differences between interpreters and non-interpreters emphasize that L2 experience (interpreting in this case) shapes the use of cognitive resources during predictive processing. These findings inform L1 and L2 prediction models by showing that morphological prediction is cognitively taxing. Interpreting experience and WM independently contribute to explaining differences in L2 prediction.
The original contributions presented in the study are publicly available. This data can be found here: https://osf.io/bmnk3/.
The studies involving human participants were reviewed and approved by IRB at Rutgers University. The patients/participants provided their written informed consent to participate in this study.
CL-A and NS contributed to conception and design of the study. CL-A collected the data for the interpreter and non-interpreter L2 learner and wrote the first draft of the manuscript. NS collected the data for the monolingual group and wrote sections of the manuscript. CL-A and JC performed the statistical analysis. All authors contributed to manuscript revision, read, and approved the submitted version.
John Jay College provided funding for open-access research.
The authors declare that the research was conducted in the absence of any commercial or financial relationships that could be construed as a potential conflict of interest.
All claims expressed in this article are solely those of the authors and do not necessarily represent those of their affiliated organizations, or those of the publisher, the editors and the reviewers. Any product that may be evaluated in this article, or claim that may be made by its manufacturer, is not guaranteed or endorsed by the publisher.
The Supplementary Material for this article can be found online at: https://www.frontiersin.org/articles/10.3389/flang.2022.1065014/full#supplementary-material
Amos, R. M., Pickering, M. J. (2020). A theory of prediction in simultaneous interpreting. Biling.: Lang. Cogn. 23, 706–715. doi: 10.1017/S1366728919000671
Armstrong, A., Bulkes, N., Tanner, D. (2018). Quantificational cues modulate the processing of English subject-verb agreement by Chinese speakers: an ERP study. Stud. Second Lang. Acquis. 40, 731–754. doi: 10.1017/S0272263118000013
Babcock, L., Capizzi, M., Arbula, S., Vallesi, A. (2017). Short-term memory improvement after simultaneous interpretation training. J. Cogn. Enhanc. 1, 254–267. doi: 10.1007/s41465-017-0011-x
Baddeley, A. D., Hitch, G. J., Allen, R. (2021). “A multicomponential model of working memory,” in Working Memory: State of the Science, eds R. H. Logie, V. Camos, and N. Cowan (Oxford, UK: Oxford University Press), 10–43. doi: 10.1093/oso/9780198842286.003.0002
Bar, M. (2007). The proactive brain: using analogies and associations to generate predictions. Trends Cogn. Sci. 11, 280–289. doi: 10.1016/j.tics.2007.05.005
Barr, D. J. (2008). Analyzing ‘visual world’ eyetracking data using multilevel logistic regression. J. Mem. Lang. 59, 457–474. doi: 10.1016/j.jml.2007.09.002
Bates, D., Mächler, M., Bolker, B., Walker, S. (2015). Fitting linear mixed-effects models using lme4. J. Statist. Softw. 67, 1–48. doi: 10.18637/jss.v067.i01
Botezatu, M. R., Kroll, J. F., Trachsel, M., Guo, T. (2022). Discourse fluency modulates spoken word recognition in monolingual and L2 speakers. Biling.: Lang. Cogn. 25, 30–443. doi: 10.1017/S1366728921001024
Calabria, M., Costa, A., Green, D. W., Abutalebi, J. (2018). Neural basis of bilingual language control. Ann. N. Y. Acad. Sci. 1426, 221–235. doi: 10.1111/nyas.13879
Chincotta, D., Underwood, G. (1998). Simultaneous interpreters and the effect of concurrent articulation on immediate memory: a bilingual digit span study. Interpreting 3, 1–20. doi: 10.1075/intp.3.1.01chi
Cholin, J., Levelt, W. J. M., Schiller, N. O. (2006). Effects of syllable frequency in speech production. Cognition 99, 205–235. doi: 10.1016/j.cognition.2005.01.009
Christoffels, I. K., de Groot, A. M. B., Kroll, J. F. (2006). Memory and language skills in simultaneous interpreters: the role of expertise and language proficiency. J. Mem. Lang. 54, 324–345. doi: 10.1016/j.jml.2005.12.004
Conway, A. R., Kane, M. J., Bunting, M. F., Hambrick, D. Z., Wilhelm, O., Engle, R. W., et al. (2005). Working memory span tasks: a methodological review and user's guide. Psychon. Bull. Rev. 12, 769–786. doi: 10.3758/BF03196772
Cooper, N., Cutler, A., Wales, R. (2002). Constraints of lexical stress on lexical access in English: evidence from native and non-native listeners. Lang. Speech 45, 207–228. doi: 10.1177/00238309020450030101
Côté, M.-H. (1997). Phonetic Salience and Consonant Cluster Simplification. MIT Working Papers, 30, 229–262.
Cowan, N. (2017). The many faces of working memory and short-term storage. Psychon. Bull. Rev. 24, 1158–1170. doi: 10.3758/s13423-016-1191-6
Cowan, N., Morey, C., Naveh-Benjamin, M. (2021). “An embedded-processes approach to working memory: how is it distinct from other approaches, and to what ends?” in Working Memory: State of the Science, eds R. H. Logie, V. Camos, and N. Cowan (Oxford: Oxford University Press), 44–84. doi: 10.1093/oso/9780198842286.003.0003
Dong, Y., Cai, R. (2015). “4. Working memory and interpreting: a commentary on theoretical models,” in Working Memory in Second Language Acquisition and Processing, eds Z. Wen, M. Borges Mota, and A. McNeill (Bristol: Multilingual Matters), 63–82. doi: 10.21832/9781783093595-008
Dong, Y., Zhong, F. (2019). “The intense bilingual experience of interpreting and its neurocognitive consequences,” in The Handbook of the Neuroscience of Multilingualism, ed J. Schwieter (Chichester: Wiley-Blackwell), 685–700. doi: 10.1002/9781119387725.ch33
Dussias, P. E., Valdés Kroff, J. R., Guzzardo Tamargo, R. E., Gerfen, C. (2013). When Gender and Looking Go Hand in Hand. Stud. Second Lang. Acquis. 35, 353–387. doi: 10.1017/S0272263112000915
Engle, R. W. (2002). Working memory capacity as executive function. Curr. Dir. Psychol. Sci. 11, 19–23. doi: 10.1111/1467-8721.00160
Engle, R. W. (2018). Working memory and executive attention: a revisit. Perspectives on Psychol. Sci. 13, 190–193. doi: 10.1177/1745691617720478
Ericsson, K. A., Kintsch, W. (1995). Long-term working memory. Psychol. Rev. 102, 211–245. doi: 10.1037/0033-295X.102.2.211
Face, T. L. (2005). Syllable weight and the perception of Spanish stress placement by second language learners. J. Lang. Learn. 3, 90–103.
Face, T. L. (2006). Cognitive factors in the perception of Spanish stress placement: implications for a model of speech perception. Linguistics 44, 1237–1267. doi: 10.1515/LING.2006.040
Foltz, A. (2021). Using prosody to predict upcoming referents in the L1 and the L2: the role of recent exposure. Stud. Second Lang. Acquis. 43, 753–780. doi: 10.1017/S0272263120000509
Foote, R. (2011). Integrated knowledge of agreement in early and late english-spanish bilinguals. Appl. Psycholinguist. 32, 187. doi: 10.1017/S0142716410000342
Francis, A., Nusbaum, H. (2009). Effects of intelligibility on working memory demand for speech perception. Atten. Percept. Psychophys. 71, 1360–1374. doi: 10.3758/APP.71.6.1360
García, A. M. (2014). The interpreter advantage hypothesis: preliminary data patterns and empirically motivated questions. J. Am. Transl. Interpret. Stud. Assoc. 9, 219–238. doi: 10.1075/tis.9.2.04gar
García, A. M. (2019). The Neurocognition of Translation and Interpreting. Amsterdam/Philadelphia, PA: John Benjamins Publishing, 268. doi: 10.1075/btl.147
Gile, D. (1995). Basic Concepts and Models for Interpreter and Translator Training. Amsterdam/Philadelphia, PA: John Benjamins Publishing. doi: 10.1075/btl.8(1st)
Gosselke Berthelsen, S., Horne, M., Brännström, K. J., Shtyrov, Y., Roll, M. (2018). Neural processing of morphosyntactic tonal cues in second-language learners. J. Neurolinguistics 45, 60–78. doi: 10.1016/j.jneuroling.2017.09.001
Grüter, T., Kaan, E. (2021). Prediction in Second Language Processing and Learning. Amsterdam: John Benjamin Publishing Company.
Hahn, U., Bailey, T. M. (2005). What makes words sound similar? Cognition 97, 227–267. doi: 10.1016/j.cognition.2004.09.006
Hartsuiker, R. J., Barkhuysen, P. N. (2006). Language production and working memory: the case of subject-verb agreement. Lang. Cogn. Process. 21, 181–204. doi: 10.1080/01690960400002117
Hed, A., Schremm, A., Horne, M., Roll, M. (2019). Neural correlates of second language acquisition of tone-grammar associations. Ment. Lex. 14, 98–123. doi: 10.1075/ml.17018.hed
Hervais-Adelman, A., Babcock, L. (2020). The neurobiology of simultaneous interpreting: where extreme language control and cognitive control intersect. Biling.: Lang. Cogn. 23, 740–751. doi: 10.1017/S1366728919000324
Hill, B. D., Elliott, E. M., Shelton, J. T., Pella, R. D., O'Jile, J. R., Gouvier, W. D.(2010) Can we improve the clinical assessment of working memory? An evaluation of the Wechsler Adult Intelligence Scale-Third Edition using a working memory criterion construct. J. Clin. Exper. Neuropsychol. 32, 315–323. doi: 10.1080/13803390903032529
Hopp, H. (2015). Individual differences in the second language processing of object–subject ambiguities. Appl. Psycholinguist. 36, 129–173. doi: 10.1017/S0142716413000180
Hothorn, T., Bretz, F., Westfall, P. (2008). Simultaneous inference in general parametric models. Biom. J. 50, 346–363. doi: 10.1002/bimj.200810425
Huettig, F. (2015). Four central questions about prediction in language processing. Brain Res. 1626, 118–135. doi: 10.1016/j.brainres.2015.02.014
Huettig, F., Guerra, E. (2019). Effects of speech rate, preview time of visual context, and participant instructions reveal strong limits on prediction in language processing. Brain Res. 1706, 196–208. doi: 10.1016/j.brainres.2018.11.013
Huettig, F., Janse, E. (2016). Individual differences in working memory and processing speed predict anticipatory spoken language processing in the visual world. Lang. Cogn. Neurosci. 31, 80–93. doi: 10.1080/23273798.2015.1047459
Huettig, F., McQueen, J. M. (2007). The tug of war between phonological, semantic and shape information in language-mediated visual search. J. Mem. Lang. 57, 460–482. doi: 10.1016/j.jml.2007.02.001
Jakobson, R. (1968). Child Language: Aphasia and Phonological Universals. Berlin: Walter de Gruyter. doi: 10.1515/9783111353562
Jiang, N. (2004). Morphological insensitivity in second language processing. Appl. Psycholinguist. 25, 603–634. doi: 10.1017/S0142716404001298
Kaan, E. (2014). Predictive sentence processing in L2 and L1: what is different? Linguist. Approaches Biling. 4, 257–282. doi: 10.1075/lab.4.2.05kaa
Kaan, E., Grüter, T. (2021). Prediction in Second Language Processing and Learning. Amsterdam: John Benjamins Publishing Company, 26–46. doi: 10.1075/bpa.12
Kukona, A., Braze, D., Johns, C. L., Mencl, E., Van Dyke, J. A., Magnuson, J. S., et al. (2016). The real-time prediction and inhibition of linguistic outcomes: effects of language and literacy skill. Acta Psychol. 171, 72–84. doi: 10.1016/j.actpsy.2016.09.009
Lakens, D. (2017). Equivalence tests. Soc. Psychol. Personal. Sci. 8, 355–362. doi: 10.1177/1948550617697177
Li, P., MacWhinney, B. (2013). “Competition model,” in The Encyclopedia of Applied Linguistics, ed C. A. Chapelle. doi: 10.1002/9781405198431.wbeal0168
Li, X. (2015). Putting interpreting strategies in their place Justifications for teaching strategies in interpreter training *. Babel 2, 170–192. doi: 10.1075/babel.61.2.02li
Liu, M., Schallert, D. L., Carroll, P. J. (2004). Working memory and expertise in simultaneous interpreting. Interpreting 6, 19–42. doi: 10.1075/intp.6.1.04liu
Logie, R. H., Belletier, C., Doherty, J. D. (2021). “Integrating theories of working memory,” in Working Memory: State of the Science, eds R. H. Logie, V. Camos, and N. Cowan (Oxford: Oxford University Press), 389–429. doi: 10.1093/oso/9780198842286.003.0014
Lord, G. (2007). The role of lexicon in learning second language stress patterns. Appl. Lang. Learn. 17, 1–14.
Lozano-Argüelles, C., Sagarra, N., Casillas, J. V. (2020). Slowly but surely: interpreting facilitates L2 morphological anticipation based on suprasegmental and segmental information. Biling.: Lang. Cogn. 23, 752–762. doi: 10.1017/S1366728919000634
MacWhinney, B. (2018). “A unified model of first and second language learning,” in Sources of Variation in First Language Acquisition: Languages, Contexts, and Learners, eds M. Hickmann, E. Veneziano, and H. Jisa (Amsterdam: John Benjamins), 287–312. doi: 10.1075/tilar.22.15mac
Mielicki, M. K., Koppel, R. H., Valencia, G., Wiley, J. (2018). Measuring working memory capacity with the letter-number sequencing task: advantages of visual administration.University of Illinois at Chicago. Journal Contribution. 32, 805–814. doi: 10.1002/acp.3468
Mirman, D., Dixon, J. A., Magnuson, J. S. (2008). Statistical and computational models of the visual world paradigm: Growth curves and individual differences. J. Mem. Lang. 59, 475–494.
Montrul, S., Slabakova, R. (2003). Competence similarities between native and near-native speakers: an investigation of the preterite-imperfect contrast in Spanish. Stud. Second Lang. Acquis. 25, 351–398. doi: 10.1017/S0272263103000159
Morales-Font, A. (2014). “El acento [The accent],” in Fonología a Generativa Contemporánea de la Lengua Española [Contemporary generative phonology of the Spanish language], eds R. A. Núñez., S. Colina., and T. G. Bradley, 2nd ed. (Washington, DC: Georgetown University Press), 235–265.
Moser-Mercer, B. (1978). “Simultaneous interpretation: a hypothetical model and its practical application,” in Language Interpretation and Communication, eds D. Gerver, and H. W. Sinaiko (New York, NY: Plenum Press), 353–368. doi: 10.1007/978-1-4615-9077-4_31
Nakamura, C., Arai, M., Mazuka, R. (2012). Immediate use of prosody and context in predicting a syntactic structure. Cognition 125, 317–323. doi: 10.1016/j.cognition.2012.07.016
Nour, S., Struys, E., Stengers, H. (2020). Adaptive control in interpreters: assessing the impact of training and experience on working memory. Biling.: Lang. Cogn. 23, 772–779. doi: 10.1017/S1366728920000127
Otten, M., Van Berkum, J. J. A. (2009). Does working memory capacity affect the ability to predict upcoming words in discourse? Brain Res. 1291, 92–101. doi: 10.1016/j.brainres.2009.07.042
Padilla, P., Bajo, M. T., Cañas, J. J., Padilla, F. (1995). Cognitive processes of memory in simultaneous interpretation. Top. Interpret. Res. 3, 61–71.
Perdomo, M., Kaan, E. (2019). Prosodic cues in second-language speech processing: a visual world eye-tracking study. Second Lang. Res. 37. doi: 10.1177/0267658319879196
R Core Team (2019). R: A Language and Environment for Statistical Computing. Vienna, Austria: R Foundation for Statistical Computing. Available online at: https://www.R-project.org/ (accessed September 2022).
Redick, T. S. (2019). The hype cycle of working memory training. Curr. Direct Psychol. Sci. 28, 423–429. doi: 10.1177/0963721419848668
Reichle, R. V., Tremblay, A., Coughlin, C. E., Voss, E., Tai, S., Li, Z., et al. (2013). “Working-memory capacity effects in the processing of non-adjacent subject-verb agreement: an event-related brain potentials study,” in Selected Proceedings of the 2011 Second Language Research Forum. Somerville, MA: Cascadilla Proceedings Project.
Roll, M., Horne, M., Lindgren, M. (2010). Word accents and morphology-ERPs of Swedish word processing. Brain Res. 1330, 114–123. doi: 10.1016/j.brainres.2010.03.020
Roll, M., Söderström, P., Frid, J., Mannfolk, P., Horne, M. (2017). Forehearing words: pre-activation of word endings at word onset. Neurosci. Lett. 658, 57–61. doi: 10.1016/j.neulet.2017.08.030
Roll, M., Söderström, P., Horne, M. (2013). Word-stem tones cue suffixes in the brain. Brain Res. 1520, 116–120. doi: 10.1016/j.brainres.2013.05.013
Roll, M., Söderström, P., Mannfolk, P., Shtyrov, Y., Johansson, M., Westen, D. V., et al. (2015). Word tones cueing morphosyntactic structure: neuroanatomical substrates and activation time-course assessed by EEG and fMRI. Brain Lang. 150, 14–21. doi: 10.1016/j.bandl.2015.07.009
Sagarra, N. (2013). “Working memory in second language acquisition,” in The Encyclopedia of Applied Linguistics, eds C. A. Chapelle (Oxford, UK: Wiley-Blackwell), 6207–6215. doi: 10.1002/9781405198431.wbeal1286
Sagarra, N. (2021). “When more is better: higher L1-L2 similarity, L2 proficiency, and working memory facilitate L2 morphosyntactic processing,” in Research on Second Language Processing and Processing Instruction, eds M. Leeser, G. Keating, and W. Wong (Studies in Bilingualism, Vol. 62). (Amsterdam: John Benjamins), 125–150. doi: 10.1075/sibil.62.04sag
Sagarra, N., Casillas, J. V. (2018). Suprasegmental information cues morphological anticipation during L1/L2 lexical access. J. Second Lang. Stud. 1, 31–59. doi: 10.1075/jsls.17026.sag
Salverda, A. P., Kleinschmidt, D., Tanenhaus, M. K. (2014). Immediate effects of anticipatory coarticulation in spoken-word recognition. J. Mem. Lang. 71, 145–163. doi: 10.1016/j.jml.2013.11.002
Sanchez, C. A., Wiley, J., Miura, T. K., Colflesh, G. J., Ricks, T. R., Jensen, M. S., et al. (2010). Assessing working memory capacity in a non-native language. Learn. Individ. Differ. 20, 488–493. doi: 10.1016/j.lindif.2010.04.001
Schremm, A., Hed, A., Horne, M., Roll, M. (2017). Training predictive L2 processing with a digital game: prototype promotes acquisition of anticipatory use of tone-suffix associations. Comput. Educ. 114, 206–221. doi: 10.1016/j.compedu.2017.07.006
Schremm, A., Söderström, P., Horne, M., Roll, M. (2016). Implicit acquisition of tone-suffix connections in L2 learners of Swedish. Ment. Lex. 11, 55–75. doi: 10.1075/ml.11.1.03sch
Sebastián-Gallés, N., Martí, M. A., Carreiras, M., Cuetos, F. (2000). LEXESP: Una base de datos informatizada del español. Barcelona: Universitat de Barcelona.
Shelton, J. T., Elliott, E. M., Hill, B. D., Calamia, M. R., Gouvier, W. D. (2009). A comparison of laboratory and clinical working memory tests and their prediction of fluid intelligence. Intelligence 37, 283–293. doi: 10.1016/j.intell.2008.11.005
Signorelli, T. M., Haarmann, H. J., Obler, L. K. (2011). Working memory in simultaneous interpreters: effects of task and age. Int. J. Biling. 16, 198–212. doi: 10.1177/1367006911403200
Söderström, P., Horne, M., Roll, M. (2015). “Using tonal cues to predict inflections,” in Proceedings of Fonetik 2015, Lund University, Sweden Using. Lund, Sweden.
Söderström, P., Roll, M., Horne, M. (2012). Processing morphologically conditioned word accents. Ment. Lex. 7, 77–89. doi: 10.1075/ml.7.1.04soe
Soto-Faraco, S., Sebastián-Gallés, N., Cutler, A. (2001). Segmental and suprasegmental mismatch in lexical access. J. Mem. Lang. 45, 412–432. doi: 10.1006/jmla.2000.2783
Tsai, N., Au, J., Jaeggi, S. M. (2016). “Working memory, language processing, and implications of malleability for second language acquisition,” in Cognitive Individual Differences in Second Language Processing and Acquisition, eds G. Granena, Y. Yilmaz, and D. O. Jackon (Amsterdam: John Benjamins Publishing Company), 69–88. doi: 10.1075/bpa.3
Vandierendonck, A. (2021). “Multicomponent working memory system with distributed executive control,” in Working Memory: State of the Science, eds R. H. Logie, V. Camos, and N. Cowan (Oxford, UK: Oxford University Press), 150–174. doi: 10.1093/oso/9780198842286.003.0006
Wechsler, D. (1997). WAIS-III: Wechsler Adult Intelligence Scale. San Antonio, TX: Psychological Corporation. doi: 10.1037/t49755-000
Keywords: working memory, prosody, stress, syllabic structure, interpreting, L2 processing, prediction
Citation: Lozano-Argüelles C, Sagarra N and Casillas JV (2023) Interpreting experience and working memory effects on L1 and L2 morphological prediction. Front. Lang. Sci. 1:1065014. doi: 10.3389/flang.2022.1065014
Received: 09 October 2022; Accepted: 16 December 2022;
Published: 01 February 2023.
Edited by:
Ruiming Wang, South China Normal University, ChinaCopyright © 2023 Lozano-Argüelles, Sagarra and Casillas. This is an open-access article distributed under the terms of the Creative Commons Attribution License (CC BY). The use, distribution or reproduction in other forums is permitted, provided the original author(s) and the copyright owner(s) are credited and that the original publication in this journal is cited, in accordance with accepted academic practice. No use, distribution or reproduction is permitted which does not comply with these terms.
*Correspondence: Cristina Lozano-Argüelles, Y2xvemFub2FyZ3VlbGxlc0BqamF5LmN1bnkuZWR1
Disclaimer: All claims expressed in this article are solely those of the authors and do not necessarily represent those of their affiliated organizations, or those of the publisher, the editors and the reviewers. Any product that may be evaluated in this article or claim that may be made by its manufacturer is not guaranteed or endorsed by the publisher.
Research integrity at Frontiers
Learn more about the work of our research integrity team to safeguard the quality of each article we publish.