- 1Dirección de Investigación, Universidad Tecnológica de Panamá, Panamá, Panama
- 2Department of Research in Virology and Biotechnology, Gorgas Memorial Institute of Health Studies, Panamá, Panama
- 3Facultad de Ingenieria de Sistemas Computacionales, Universidad Tecnológica de Panamá, Panamá, Panama
The dengue virus (DENV) is endemic in most tropical regions of Central and South America. It is known that when the number of mosquito vectors (Aedes aegypti and Aedes albopictus) for this disease becomes abundant, the number of infectious cases increases. DENV has been known to be continuously circulating in Panama since 1993, with an increasing number of cases reported in recent years after the COVID-19 pandemic, as well as other vector-borne diseases. Preventing dengue outbreaks by having an early detection system is of the utmost importance. To tackle this task, we propose an overall surveillance system framework tailored to the Panamanian situation but applicable to many countries suffering the same maladies. This manuscript presents a transdisciplinary vision that encompasses aspects of sample management, vector surveillance, sharing of weather information, and georeferencing of cases in a Geographic Information System and defining data-driven software solutions for prediction of possible outbreaks.
1 Introduction
The dengue virus (DENV) is endemic in the tropical regions of the world. Beyond the greatest scientific efforts and research, the current global dengue situation has reached unprecedented levels. More than 7 million cases worldwide have been reported to the World Health Organization (WHO) before the end of the first trimester of the year 2024 (1). Although the latest numbers have stunned the whole world, the worst is happening in the Americas, with an accumulated 4.5 million cases, that is, about 80% of the total global cases for 2023. In fact, while the four dengue serotypes (DENV-1, -2, -3, and -4) are known to be present in Asian regions and the Americas, their virulence is higher in the latter (2).
The Central and South American regions have been particularly affected by this threat. Some estimates say there is a three-fold increase compared to the same period in 2023, highlighting the acceleration of this health problem (3, 4). This situation has been noted by various authors who are pondering about it becoming a global threat (5).
Factors contributing to this increase in cases are currently unknown, but many authors point to urbanization (6), inadequate water provision (7) and waste management (8), climate change (9), changes in vector distribution and adaptation (10–12), massive population migrations (13, 14), scarce monitoring (15), and fragile health systems, mostly weakened after the COVID-19 pandemic (16).
In the specific case of Latin America, molecular and clinical information on dengue is scarce, so spatio-temporal trends are hard to determine (17). Despite the information gap in the region, there are many developments in the creation and implementation of early detection and outbreak detection systems. Some countries have developed location-based data-driven health approaches to their prediction and generation of early detection systems (18–20). Others are focusing on molecular and genomic surveillance of dengue with the creation of the Pan American Health Organization (PAHO) VIGENDA program for the Americas (21).
Panama only has an epidemiological guide for dengue detection and monitoring (22, 23), while in Mexico and Brazil (24, 25) clear strategies have been defined, and early detection systems have been developed or implemented for dengue. This Perspective article aims to analyze the state of the current system and provide an overview of what considerations it needs to take into account to make it a reality in the near future.
2 Brief history of dengue in Panama
According to Díaz et al. (26), DENV serotypes have been known to be re-circulating in Panama continuously since 1993. The cases have been growing steadily over the years, although it is known that some of its effects have been masked by COVID-19 and other circulating viruses (27). For example, the endemic cocirculation of other arboviruses such as chikungunya (28, 29) and Zika (30, 31), which are transmitted by the same vector. In addition, other clinically similar zoonotic diseases complicate the epidemiological approach and notification of cases (32), and the identification of the beginning of the dengue epidemic.
Figure 1 represents the laboratory detection of dengue in clinical cases reported through the surveillance of dengue and arboviral diseases during and between different outbreaks in Panama, most of them related to the reintroduction of DENV serotypes, from 1999 to the present (33). These samples are normally analyzed through the National Surveillance System of the Ministry of Health. From 1993 to 2012, dengue diagnosis and surveillance were performed at the Gorgas Memorial Institute for Health Studies (ICGES), the national public health institution and the national central laboratory that supports the Ministry of Health, and samples were analyzed using DENV-IgM or viral isolation according to the days of symptoms, and since 2013, viral isolation for acute samples has been replaced by the RT-PCR technique (26, 29).
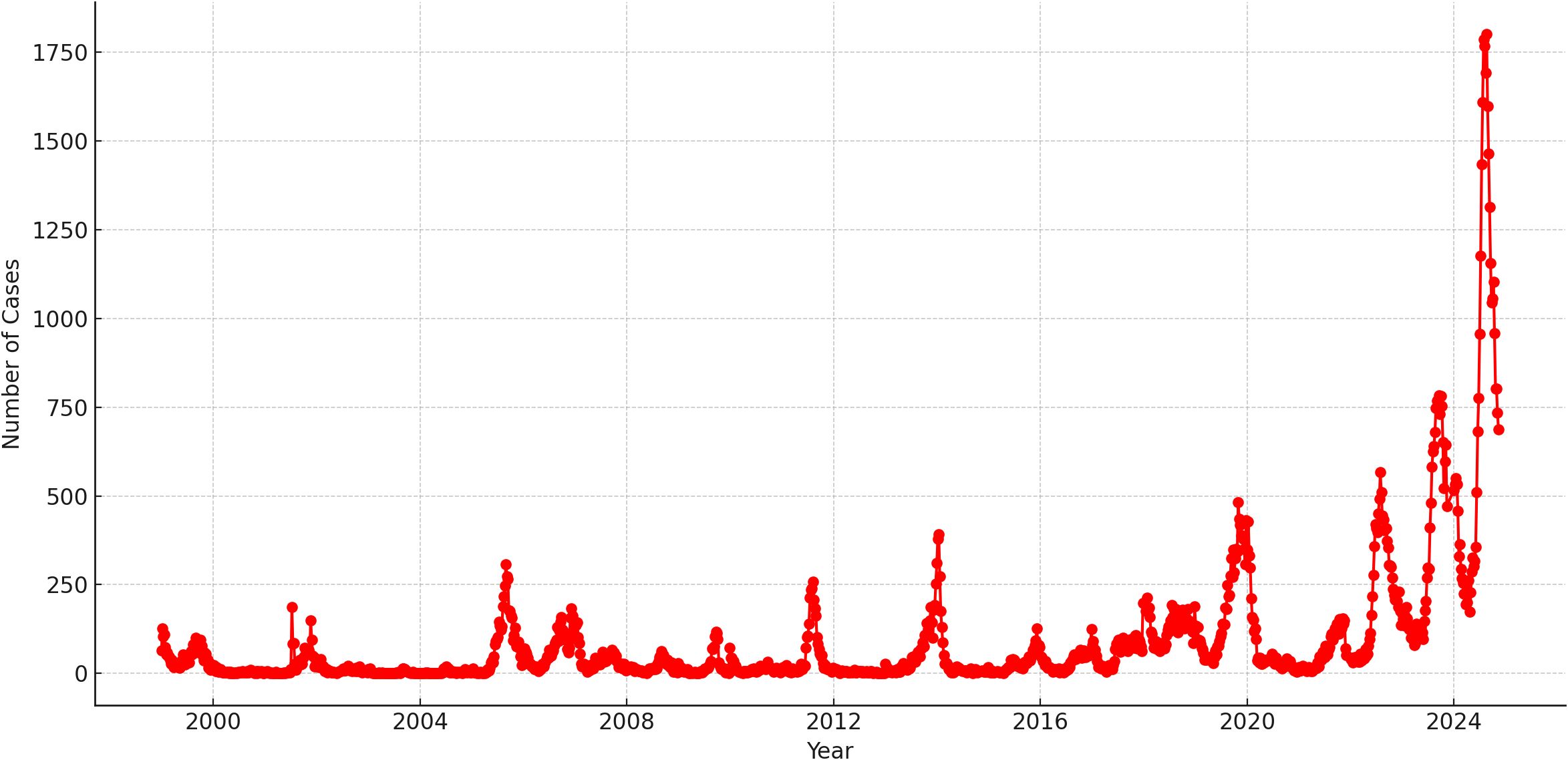
Figure 1. Dengue incidence in the Republic of Panama from 1999 to 2024 (epidemiological week #46). In this figure, the Y-axis represents the number of cases and the X-axis represents time, with each dot representing the total number of confirmed cases per epidemiological week.
More importantly, Figure 1 provides a view of the current state of dengue in the country. In 2024, the highest number of dengue cases was reported since its reintroduction into the country. This increase could be attributed to the introduction of serotype 4 (34, 35). Since this serotype had limited circulation in the country during 1999 and 2000, most of the population does not have a specific immune response to this serotype and can face increased transmission and a higher risk of severe dengue.
2.1 Current Panamanian dengue monitoring and outbreak detection system
In Panama, suspected human cases are usually first detected in primary or secondary care healthcare centers, where a physician makes a diagnosis based on clinical symptoms, and laboratory diagnostics are performed in situ (Figure 2). Confirmed cases are reported to the National Epidemiology Department (NED) of the Ministry of Health using epidemiological nexus, the NS1 positive dengue test (<9 days of onset symptoms) or DENV-IgM (between >10 and <17 days of onset symptoms). Both suspected and confirmed cases are reported to the epidemiology department of each health region and, after the mandate, later to the NED of the Ministry of Health.
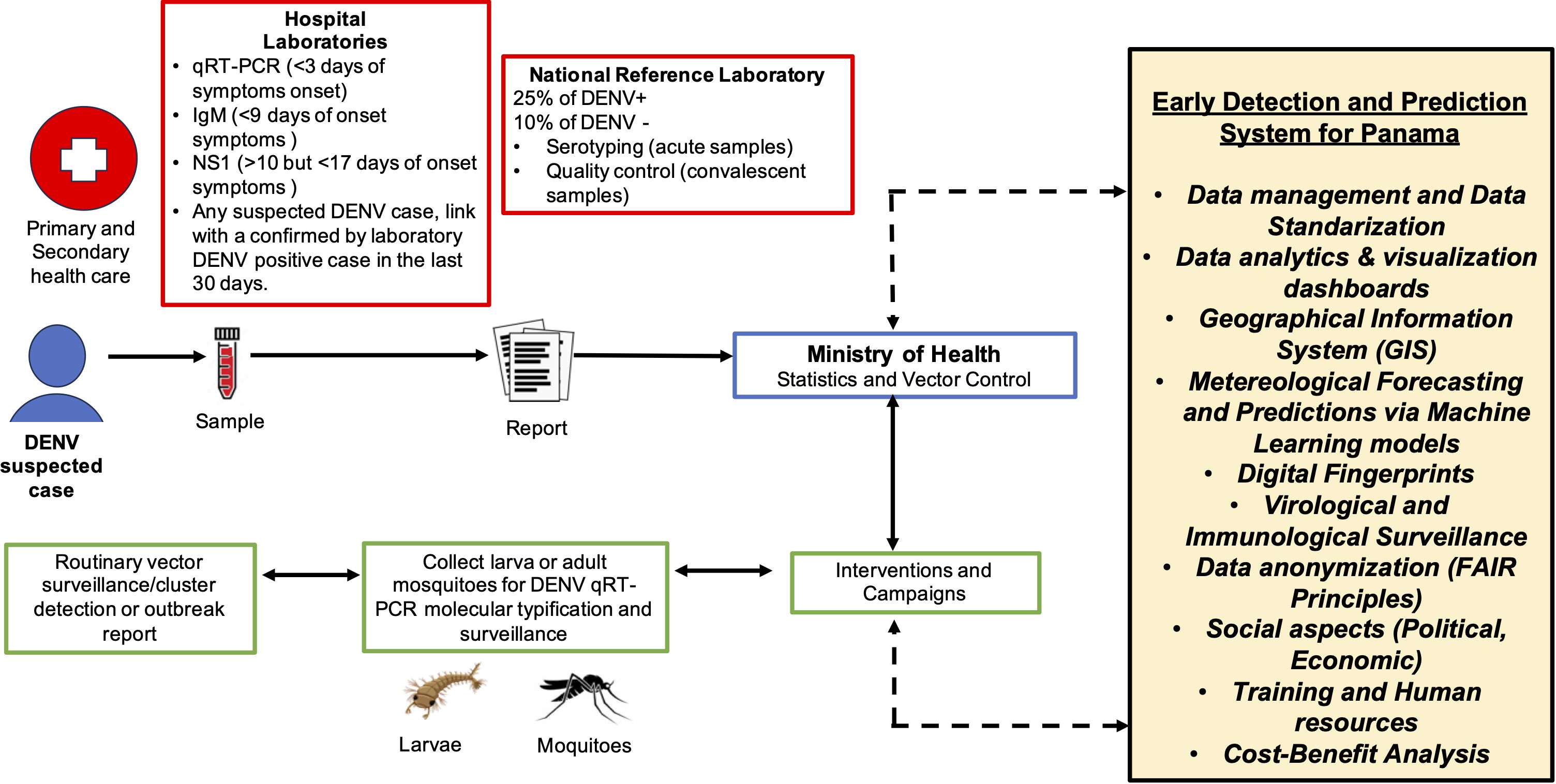
Figure 2. Proposed integrated system for the early detection and prediction of dengue outbreaks in Panama.
A subset of samples are sent to the Gorgas Memorial Institute of Health Studies (ICGES). Specifically, 25% DENV positive and 10% DENV negative samples are sent for further analysis using RT-qPCR DENV serotyping for acute samples (< 5 days after the onset of symptoms) or the quality control test of IgM antibodies for convalescent samples (6-21 days after the onset of symptoms). However, some healthcare centers do not have the personnel to take blood samples, do not have a laboratory, and lack laboratory resources or laboratory personnel, and thus, dengue laboratory diagnosis and surveillance are not done. In addition, it is difficult to respect the cold chain and to transport sera samples from limited access areas to the reference laboratory in the metropolitan city. This causes “silent” epidemiological regions that report suspected cases of dengue and other arboviral cases using epidemiological nexus or clinical diagnosis, without detection of a specific antigen, antibodies, or RT-qPCR test.
The dengue surveillance system in place in Panama integrates data generated from different components: 1) the clinical component is reported to the epidemiological department of each health center, this information is collected by region and then reported to the national epidemiological office; 2) the vector control component, where each region reports the larval index of the communities to the national vector office, which then reports to the national epidemiological office. The private sector also reports weekly the number of suspected and confirmed clinical cases directly to the national epidemiological office. Although there is a standard for immediately reporting dengue cases to epidemiology offices, most agencies report weekly or even monthly, and it has been a great challenge to have updated real-time epidemiological information to prevent outbreaks. This situation means that epidemiological action on dengue is about controlling the outbreak situation and not about preventing it.
Entomological surveillance and vector control are based on a systemic survey of houses infected with Aedes larvae (29). However, there is no automatic entomological surveillance associated with the response to the outbreak and it is not targeted to areas where human cases are highly detected. Moreover, viral molecular detection is not done as part of entomological surveillance, thus it is currently not possible to obtain viral genomic surveillance in both humans and vectors.
In addition, public health institutions are often added to the end of the sampling queue, either for human molecular sample diagnostic from healthcare institutions that do not have tests or for serotype and genomic surveillance. Finally, research projects often allow for the detailed characterization of genomic and epidemiological data of viruses retrospectively or based on outbreak responses or study-specific cohorts, and all generated data are communicated to the National Epidemiology Department.
Probably the key aspect to be noted is that in Panama there is low integration between different health systems (Ministry of Health, Social Security system, private hospitals). In addition, decisions are not taken with a multidisciplinary approach, making the situation more cumbersome. The implementation of a system for early detection and prediction of a dengue outbreak in Panama will challenge the traditional epidemiological format, which is currently based on the weekly and monthly collection of vector data of reported cases, to a permanent update of the epidemiological situation, including silent zones or zones that do not regularly send samples to reference laboratories due to the lack or limitation of resources to transport samples.
3 Characteristics of dengue early-warning systems
Baharom et al. (36) provide a systematic review of the use of the early warning system (EWS) as an outbreak prediction tool. More importantly, they selected 17 articles that describe EWS systems and came to conclusions on best practices and what they should encompass. The systematic analysis of the characteristics of the early warning system (EWS) revealed that they are based on an indicator-based surveillance (IBS) structure. An IBS predicts DENV+ outbreaks using a set of independent variables or alarm indicators. These “alarm indicators” fall into epidemiological, meteorological, entomological, socioeconomic, and population categories. Some studies also incorporate enhanced vegetation, urbanization, and education data as alarm indicators.
In general, an EWS would use the Ministry of Health data as the primary epidemiological alarm indicator when these data are available. Meteorological data are the next most used alarm indicator for studying and predicting DENV+ outbreaks (36, 37). Temperature and rainfall are the most common variables in this alarm category. An EWS model that used these two meteorological variables in combination with epidemiological data and elevation information was able to predict 75% of the Colombian outbreaks from 2007 to 2015 (38).
After epidemiological and meteorological data, entomological or mosquito data, such as House Index (HI), Aedes Index (AI), Ovitrap Index, and infection rates for male and female mosquitoes, also have potential for developing an EWS (36, 38). Combining entomological data with meteorological factors has improved the performance of EWS models in several studies (39, 40). However, transmission is known to occur even at low vector density levels (41, 42). Finally, socioeconomic alarm indicators such as population density, socioeconomic categorization indexes, and access to water and energy can play an essential role as sources of information to predict future outbreaks (36, 43). This was tested by Zhao et al. (43) when they included the GINI index, population information, and education coverage to develop a machine learning and forecasting model to predict outbreaks on different scales in Colombia.
The prediction of a DENV+ outbreak can also be studied as a data science cycle (44), as the factors needed to predict dengue cases coincide with the alarm indicators described above. However, vital data management and data processing aspects that are required for the functioning and maintenance of an EWS are: data sources, data representation, and data preparation which are in the hands of institutions and organizations generating and recollecting the information, such as the Ministry of Health in the case of case incidence. The survey by Siriyasatien et al. (44) also addresses the models used to forecast and predict an outbreak and how to evaluate their performance using evaluation metrics. For continuous response variables, i.e., incidence, mortality, total number of cases, metrics such as mean squared error (MSE), mean absolute error (MAE), or the coefficient of determination (R2) can be used (45). Finally, Siriyasatien et al. (44) present the challenges of deploying an EWS from a modeling point of view and managing massive amounts of data. One thing authors often add to an EWS and keep in line with health administrations is a focus on cost effectiveness, keeping in mind the financial costs of such a system (46, 47).
4 An integrated early-detection and prediction system for Panama
Here we describe the fundamental aspects and design of a dengue EWS that could then be presented to the Ministry of Health and all public and private institutions involved (such as ICGES, CSS, and private centers), as well as other organizations that could bring their expertise, such as the Panama association of epidemiology (SOEPIMO1) for discussion before developing a pilot study, which would allow improvement of the EWS before implementing it at the national level with constant capacity to upgrade taking into account feedback from the Ministry of Health and other users as well as its performance evaluation.
When thinking of the design of a data-based EWS for Panama, we first need to define its goals:
1. To have significant predictive power for the next outbreaks.
2. To help local authorities in their decision-making.
The system should incorporate the necessary aspects needed to prevent and control DENV+ outbreaks which have historically occurred every few years (Figure 1), but are becoming increasingly frequent.
4.1 Standardizing data management
To support the goals stated above, data management protocols must be developed. This must begin with the standardization of data from its source and end with its distribution among stakeholders and decision-makers. A nationwide effort to standardize the acquisition, preparation, and management of epidemiological data is vital for monitoring alarm indicators for future DENV+ outbreaks in Panama and could also be applied to other arboviral outbreaks. In addition to this, we foresee the development of a standard data analytics process applied to epidemiological data, incorporating software dashboards with alarm indicators. These dashboards would integrate information from active surveillance vector indexes, their dynamics, and incidence cases. This mimics the global dengue dashboard surveillance system launched by the WHO used to integrate reports from all regions and strengthen monitoring efforts (48, 49).
4.2 Geographic information system integration
An effective EWS for Panama should also leverage a Geographic Information System (GIS) database to support spatio-temporal analysis. For example, it should provide functions to isolate points or regions of interest and perform a specific analysis (50, 51). Time-series analysis, machine learning, and compartmental models have already been used to assess the effect of climate on the incidence of DENV+ cases in the metropolitan region of Panama (52, 53). These models should be expanded to predict outbreaks on a spatio-temporal scale, incorporating factors such as population movement, urbanization, and vegetation cover to improve predictive precision (36, 44).
4.3 Alarm indicators for early detection
A robust and effective EWS needs to include three key alarm indicators. 1) Digital fingerprint, which is needed to understand what people are searching for on social networks and querying on Internet databases and search engines. More importantly, it can be positively correlated with the onset of dengue fever (54–58). 2) Virology surveillance, focused on the surveillance of a new serotype and genotype (59, 60) of Dengue or known arboviruses. 3) Immunological surveillance, to determine the previous seroprevalence of dengue or flavivirus infection in the population (61).
4.4 Ethical and legal considerations
The use of health data for predictive models requires adherence to ethical and legal standards. Patient privacy must be protected in line with WHO guidelines (62, 63). Only de-identified clinical and demographic data should be used for real-time epidemiological analysis and predictive modeling. Governance structures involving patients, clinicians, and epidemiologists should guide the development of the model and ensure compliance with national and international regulations (64, 65). Models should operate at the neighborhood level to maintain privacy while enabling targeted interventions. Transparency in the manner of how data is collected and analyzed is critical to fostering trust among stakeholders (66).
4.5 Training and communication
The ethical use of predictive models also requires extensive training for public health professionals. Decision-making processes should balance the results of the predictive model with social, economic, and political factors (18, 67). Communicating model-based scenarios and their associated risks is key to ensuring informed decisions and public trust.
4.6 Implementation and national integration
The proposed system, illustrated in Figure 2, is based on routine data collection from human and vector samples, with rapid reporting facilitated by an integrated GIS-based platform. This system can alert healthcare facilities at both the primary and secondary levels, enabling timely responses.
This initiative represents a digital transformation project for Panama. Pilot programs in specific regions could pave the way for nationwide implementation. The project will require highly trained personnel to manage epidemiological, entomological, virological, immunological, and meteorological data, as well as to develop predictive models that capture viral dynamics and transmission intensity (68, 69).
Panamanian authorities should conduct detailed cost-benefit analyses to refine this system, drawing on regional examples from Nicaragua, Colombia, and Mexico (70–73).
5 Discussion
The general capacity of countries to respond to multiple concurrent outbreaks is limited due to global resource shortages, including inequality in the availability of diagnostic kits, silent regions for national serotype and genomic surveillance, trained staff, and community awareness (74, 75). In the context of the Latin American region, the challenges include limited access to real-time epidemiological and meteorological data and a lack of information on vector behavior. This has been highlighted in studies conducted in Peru (76), Colombia (38), Mexico, and Brazil (25). These studies use predictive models to address the lack of real-time data and other important limitations such as weak intersectoral work to coordinate response and share relevant data in response to outbreaks (25). These implementations are relevant to the Panamanian situation and their highlights were considered for our proposed integrated system.
The current increase in Panamanian cases placed the country on the path to a high-risk country under the WHO framework (49). To successfully implement a comprehensive early detection and prediction system for dengue outbreaks in Panama, it is essential to address the potential limitations and challenges specific to the country.
Panama faces technical, organizational, and data infrastructure constraints, such as limited computational resources, as there is a lack of high-performance computing (HPC) centers in the country. This is supplied through external regional computational resources (77–79). Likewise, there is a known shortage of skilled workforce capacity, especially in technological fields (80, 81). More importantly, there are limitations in the ability to collect real-time incidence data, including an uneven spatial sampling of key regions and neighboring areas (26, 52, 82). These data points are essential for public health officials to identify high-risk areas over time for current and future outbreaks (26, 82).
In addition, integrating data from various sources, including public and private health systems and agencies such as the Ministry of Health and meteorological services (83), poses logistical challenges that require detailed planning and organization. Sustainability is another crucial consideration. A cost-benefit analysis should assess the feasibility of maintaining the system over time, ensuring that adequate financial and human resources are available (84). Ethical and legal considerations are also paramount, particularly in the protection of sensitive health and geospatial data. In particular, in the Panamanian case, it should also comply with Law No. 81 on Personal Data Protection, enacted on 26 March 2019, and effective since 29 March 2021 (85),
The importance of this Perspective article is that it presents a trans-disciplinary vision encompassing aspects from sample management, tracking of cases, vector surveillance, georeferencing of cases, meteorological tracking (local and satellite), and most importantly, the use of machine learning algorithms for the prediction of possible outbreaks. These transdisciplinary monitoring and surveillance programs could have immediate potential impacts and benefits for prediction, prevention, and response systems in the country (86). As an improvement in the speed and efficiency of the public health response to dengue outbreaks and with real-time information, continuous monitoring could also allow for fast adaptation of the response to any changes or to quickly detect actions and decisions that have a greater impact and should be reinforced (87). Moreover, the fact that the system can be constantly improved by taking into account faced challenges shows that it is flexible and adaptable to different situations.
Finally, the surveillance system described in this manuscript is focused on the Panamanian scenario, but it should have a broader regional impact, as Panama could be seen as a pilot, and the system could be scaled up to other tropical countries with similar meteorological, environmental, demographic, and socioeconomic conditions facing similar mosquito vector-borne disease challenges.
Data availability statement
The original contributions presented in the study are included in the article/supplementary material. Further inquiries can be directed to the corresponding author.
Author contributions
GU: Conceptualization, Writing – original draft, Writing – review & editing. YD: Conceptualization, Data curation, Methodology, Visualization, Writing – original draft, Writing – review & editing. SL-V: Conceptualization, Data curation, Methodology, Visualization, Writing – original draft, Writing – review & editing. JP: Writing – review & editing. JS-G: Conceptualization, Data curation, Funding acquisition, Methodology, Visualization, Writing – original draft, Writing – review & editing.
Funding
The author(s) declare financial support was received for the research, authorship, and/or publication of this article. The Sistema Nacional de Investigación (SNI) of the Secretaría Nacional de Ciencia Tecnología e Innnovacion (SENACYT-Panama) supports research activities by SL-V, JP, and JS-G.
Acknowledgments
The authors acknowledge administrative support provided by the Instituto Conmemorativo Gorgas para el Estudio de la Salud (ICGES) and Universidad Tecnológica de Panamá (UTP).
Conflict of interest
The authors declare that the research was conducted in the absence of any commercial or financial relationships that could be construed as a potential conflict of interest.
Publisher’s note
All claims expressed in this article are solely those of the authors and do not necessarily represent those of their affiliated organizations, or those of the publisher, the editors and the reviewers. Any product that may be evaluated in this article, or claim that may be made by its manufacturer, is not guaranteed or endorsed by the publisher.
Footnotes
References
1. World Health Organization. Disease outbreak news: Dengue – global situation. Geneva, Switzerland: World Health Organization (2024).
2. Halstead SB. Dengue in the americas and southeast asia: do they differ? Rev panamericana salud publica. (2006) 20:407–15. doi: 10.1590/s1020-49892006001100007
3. Anand A, Verma A, Khatib MN, Zahiruddin QS, Gaidhane S, Sharma RK, et al. An urgent call for action: addressing the unprecedented rise in dengue cases in the americas. Ann Med Surg. (2024) 86:3814–5. doi: 10.1097/MS9.0000000000002186
4. Rodriguez-Morales A, Montenegro-Idrogo JJ, Celis-Salinas JC, Angerami R, Villamil-Gómez W, Sarute N, et al. Unraveling the unparalleled 2024 epidemic of dengue in the americas. Rev Chil Infectología. (2024) 41:421–8. doi: 10.4067/s0716-10182024000300133
5. de Almeida MT, Merighi DGS, Visnardi AB, Gonçalves CAB, Amorim VMdF, Ferrari ASdA, et al. Latin america’s dengue outbreak poses global health threat. Viruses. (2025) 17:57. doi: 10.3390/v17010057
6. Gubler DJ. Dengue, urbanization and globalization: the unholy trinity of the 21st century. Trop Med Health. (2011) 39:S3–S11. doi: 10.2149/tmh.2011-S05
7. Valderrama A, Díaz Y, López-Vergès S. Interaction of flavivirus with their mosquito vectors and their impact on the human health in the americas. Biochem Biophys Res Commun. (2017) 492:541–7. doi: 10.1016/j.bbrc.2017.05.050
8. Overgaard HJ, Dada N, Lenhart A, Stenström TAB, Alexander N. Integrated disease management: arboviral infections and waterborne diarrhoea. Bull World Health Organ. (2021) 99:583. doi: 10.2471/BLT.20.269985
9. Trejo I, Barnard M, Spencer JA, Keithley J, Martinez KM, Crooker I, et al. Changing temperature profiles and the risk of dengue outbreaks. PloS Climate. (2023) 2:e0000115. doi: 10.1371/journal.pclm.0000115
10. Liu Z, Zhang Q, Li L, He J, Guo J, Wang Z, et al. The effect of temperature on dengue virus transmission by aedes mosquitoes. Front Cell Infection Microbiol. (2023) 13:1242173. doi: 10.3389/fcimb.2023.1242173
11. Bennett KL, McMillan WO, Loaiza JR. The impact of biological invasion and genomic local adaptation on the geographical distribution of aedes aEgypti in Panama. bioRxiv. (2019), 744607. doi: 10.1101/744607
12. Bennett KL, McMillan WO, Loaiza JR. Does local adaptation impact on the distribution of competing aedes disease vectors? Climate. (2021) 9:36. doi: 10.3390/cli9020036
13. Maljkovic Berry I, Rutvisuttinunt W, Sippy R, Beltran-Ayala E, Figueroa K, Ryan S, et al. The origins of dengue and chikungunya viruses in Ecuador following increased migration from Venezuela and Colombia. BMC Evolutionary Biol. (2020) 20:1–12. doi: 10.1186/s12862-020-1596-8
14. Naranjo L, Williams Y, Levy J, Obando R, González JA, Pachar M, et al. The endless vulnerability of migrant children in-transit across the darién gap. Am J Trop Med Hygiene. (2023) 1:515–9. doi: 10.4269/ajtmh.22-0765
15. Angelo M, Ramalho WM, Gurgel H, Belle N, Pilot E. Dengue surveillance system in Brazil: A qualitative study in the federal district. Int J Environ Res Public Health. (2020) 17:2062. doi: 10.3390/ijerph17062062
16. Rehan ST, Asghar MS, Ullah I, Mahmood H, Lee KY, Tahir MJ. Dengue outbreak and fragile healthcare system: Doctors at the verge of mental and physical stress. Brain Behav. (2022) 12:1–2. doi: 10.1002/brb3.v12.11
17. Ramos-Castañeda J, Barreto dos Santos F, Martinez-Vega R, Galvão de Araujo JM, Joint G, Sarti E. Dengue in latin america: systematic review of molecular epidemiological trends. PloS Negl Trop Dis. (2017) 11:e0005224. doi: 10.1371/journal.pntd.0005224
18. Cabrera M, Leake J, Naranjo-Torres J, Valero N, Cabrera JC, Rodríguez-Morales AJ. Dengue prediction in latin america using machine learning and the one health perspective: a literature review. Trop Med Infect Dis. (2022) 7:322. doi: 10.3390/tropicalmed7100322
19. Parra-Amaya ME, Puerta-Yepes ME, Lizarralde-Bejarano DP, Arboleda-Sánchez S. Early detection for dengue using local indicator of spatial association (lisa) analysis. Diseases. (2016) 4:16. doi: 10.3390/diseases4020016
20. Sylvestre E, Joachim C, Cecilia-Joseph E, Bouzille G, Campillo-Gimenez B, Cuggia M, et al. Data-driven methods for dengue prediction and surveillance using real-world and big data: A systematic review. PloS Negl Trop Dis. (2022) 16:e0010056. doi: 10.1371/journal.pntd.0010056
21. Pan-American Health Organization (PAHO). Redes de vigilancia genómica regional de la ops (pahogen). Washington, D.C., United States: World Health Organization (2024).
22. Organización Panamericana de la Salud. Guías para el abordaje integral del dengue en Panamá (2014). Tech. rep., Organización Panamericana de la Salud (Accessed 2024-12-21).
23. Ministerio de la Salud de Panamá. Guia Nacional de Epidemiología (2018). Tech. rep., Ministerio de la Salud de Panamá (Accessed 2024-12-21).
24. Bowman LR, Tejeda GS, Coelho GE, Sulaiman LH, Gill BS, McCall PJ, et al. Alarm variables for dengue outbreaks: a multi-centre study in asia and latin america. PloS One. (2016) 11:e0157971. doi: 10.1371/journal.pone.0157971
25. Hussain-Alkhateeb L, Kroeger A, Olliaro P, Rocklöv J, Sewe MO, Tejeda G, et al. Early warning and response system (ewars) for dengue outbreaks: Recent advancements towards widespread applications in critical settings. PloS One. (2018) 13:e0196811. doi: 10.1371/journal.pone.0196811
26. Díaz Y, Chen-Germán M, Quiroz E, Carrera J-P, Cisneros J, Moreno B, et al. Molecular epidemiology of dengue in Panama: 25 years of circulation. Viruses. (2019) 11:764. doi: 10.3390/v11080764
27. Rodríguez J, d. RL, Eastwood G, Sanabria LFC. Vector control and surveillance under lockdown: Covid-19 and future pandemics. In: Planetary health approaches to understand and control vector-borne diseases. Wageningen, Netherlands: Wageningen Academic (2023). p. 206–25.
28. Díaz Y, Carrera J-P, Cerezo L, Arauz D, Guerra I, Cisneros J, et al. Chikungunya virus infection: first detection of imported and autochthonous cases in Panama. Am J Trop Med hygiene. (2015) 92:482. doi: 10.4269/ajtmh.14-0404
29. Carrera J-P, Diaz Y, Denis B, Barahona de Mosca I, Rodriguez D, Cedeno I, et al. Unusual pattern of chikungunya virus epidemic in the americas, the Panamanian experience. PloS Negl Trop Dis. (2017) 11:e0005338. doi: 10.1371/journal.pntd.0005338
30. Araúz D, De Urriola L, Jones J, Castillo M, Martínez A, Murillo E, et al. Febrile or exanthematous illness associated with zika, dengue, and chikungunya viruses, Panama. Emerging Infect Dis. (2016) 22:1515. doi: 10.3201/eid2208.160292
31. Eskildsen GA, Kramer LD, Zink SD, Dupuis AP, Wong SJ, Furuya A, et al. Integrated arbovirus surveillance improves the detection onset of zika virus in Panama. Am J Trop Med Hygiene. (2020) 102:985. doi: 10.4269/ajtmh.19-0316
32. Armién B, Muñoz C, Cedeño H, Salazar JR, Salinas TP, González P, et al. Hantavirus in Panama: twenty years of epidemiological surveillance experience. Viruses. (2023) 15:1395. doi: 10.3390/v15061395
33. PAHO. Panamá - Casos de dengue (2024). Available online at: https://www3.paho.org/data/index.php/es/temas/indicadores-dengue/dengue-subnacional/563-pan-dengue-casos-es.html (Accessed 2024-12-04).
34. Ministerio de Salud de Panamá. Boletin Informativo del MINSA. In: Boletines Semanales 2024. Dengue 2024. Semana No. 47. Panama: Ministerio de Salud, Panama (2024).
35. Chen-Germán M, Araúz D, Aguilar C, Vega M, Gonzalez C, Gondola J, et al. Detection of dengue virus serotype 4 in Panama after 23 years without circulation. Front Cell Infection Microbiol. (2024) 14:1467465. doi: 10.3389/fcimb.2024.1467465
36. Baharom M, Ahmad N, Hod R, Abdul Manaf MR. Dengue early warning system as outbreak prediction tool: a systematic review. Risk Manag Healthc Policy. (2022) 15:871–86. doi: 10.2147/RMHP.S361106
37. Baharom M, Ahmad N, Hod R, Arsad FS, Tangang F. The impact of meteorological factors on communicable disease incidence and its projection: a systematic review. Int J Environ Res Public Health. (2021) 18:11117. doi: 10.3390/ijerph182111117
38. Lee J-S, Carabali M, Lim JK, Herrera VM, Park I-Y, Villar L, et al. Early warning signal for dengue outbreaks and identification of high risk areas for dengue fever in Colombia using climate and non-climate datasets. BMC Infect Dis. (2017) 17:1–11. doi: 10.1186/s12879-017-2577-4
39. Chang F-S, Tseng Y-T, Hsu P-S, Chen C-D, Lian I-B, Chao D-Y. Re-assess vector indices threshold as an early warning tool for predicting dengue epidemic in a dengue non-endemic country. PloS Negl Trop Dis. (2015) 9:e0004043. doi: 10.1371/journal.pntd.0004043
40. Ahmad R, Suzilah I, Wan Najdah WMA, Topek O, Mustafakamal I, Lee HL. Factors determining dengue outbreak in Malaysia. PloS One. (2018) 13:e0193326. doi: 10.1371/journal.pone.0193326
41. Scott TW, Morrison AC. Aedes aEgypti density and the risk of dengue virus transmission. Ecol aspects Appl genetically modified mosquitoes. (2003) 2:187–206. Available online at: https://edepot.wur.nl/136912.
42. Bowman LR, Runge-Ranzinger S, McCall P. Assessing the relationship between vector indices and dengue transmission: a systematic review of the evidence. PloS Negl Trop Dis. (2014) 8:e2848. doi: 10.1371/journal.pntd.0002848
43. Zhao N, Charland K, Carabali M, Nsoesie EO, Maheu-Giroux M, Rees E, et al. Machine learning and dengue forecasting: Comparing random forests and artificial neural networks for predicting dengue burden at national and sub-national scales in Colombia. PloS Negl Trop Dis. (2020) 14:e0008056. doi: 10.1371/journal.pntd.0008056
44. Siriyasatien P, Chadsuthi S, Jampachaisri K, Kesorn K. Dengue epidemics prediction: A survey of the state-of-the-art based on data science processes. IEEE Access. (2018) 6:53757–95. doi: 10.1109/ACCESS.2018.2871241
45. Handelman GS, Kok HK, Chandra RV, Razavi AH, Huang S, Brooks M, et al. Peering into the black box of artificial intelligence: evaluation metrics of machine learning methods. Am J Roentgenology. (2019) 212:38–43. doi: 10.2214/AJR.18.20224
46. Ismail S, Fildes R, Ahmad R, Ali WNWM, Omar T. The practicality of Malaysia dengue outbreak forecasting model as an early warning system. Infect Dis Model. (2022) 7:510–25. doi: 10.1016/j.idm.2022.07.008
47. Hussain-Alkhateeb L, Rivera Ramirez T, Kroeger A, Gozzer E, Runge-Ranzinger S. Early warning systems (ewss) for chikungunya, dengue, malaria, yellow fever, and zika outbreaks: What is the evidence? a scoping review. PloS Negl Trop Dis. (2021) 15:e0009686. doi: 10.1371/journal.pntd.0009686
48. W.H.O. Global dengue surveillance. (2024). Tech. rep., World Health Organization (Accessed 2024-12-04).
49. W.H.O. Dengue - Global situation. (2024). Tech. rep., World Health Organization (Accessed 2024-12-04).
50. Calistri P, Conte A, Natale F, Possenti L, Savini L, Danzetta ML, et al. Systems for prevention and control of epidemic emergencies. Veterinaria Italiana. (2013) 49:255–61. doi: 10.12834/VetIt.1206.06
51. Pfeiffer DU. Spatial analysis in epidemiology. Oxford, United Kingdom: Oxford University Press (2008).
52. Navarro Valencia V, Díaz Y, Pascale JM, Boni MF, Sanchez-Galan JE. Assessing the effect of climate variables on the incidence of dengue cases in the metropolitan region of Panama city. Int J Environ Res Public Health. (2021) 18:12108. doi: 10.3390/ijerph182212108
53. Valencia VAN, Díaz Y, Pascale JM, Boni MF, Sanchez-Galan JE. Using compartmental models and particle swarm optimization to assess dengue basic reproduction number r0 for the republic of Panama in the 1999-2022 period. Heliyon. (2023) 9:1–18. doi: 10.1016/j.heliyon.2023.e15424
54. Sivaprasad A, Beevi NS, Manojkumar T. Dengue and early warning systems: a review based on social network analysis. Proc Comput Sci. (2020) 171:253–62. doi: 10.1016/j.procs.2020.04.027
55. Wahyuni S, Sediyono E, Sembiring I. Dengue prediction model: A systematic review using social network analysis. In: Journal of Physics: Conference Series, vol. 1844. Bristol, United Kingdom: IOP Publishing (2021). p. 012012.
56. Saire JEC. Building intelligent indicators to detect dengue epidemics in Brazil using social networks. In: 2019 IEEE Colombian Conference on Applications in Computational Intelligence (ColCACI). Barranquilla, Colombia: IEEE (2019). p. 1–5.
57. Liu D, Guo S, Zou M, Chen C, Deng F, Xie Z, et al. A dengue fever predicting model based on baidu search index data and climate data in south China. PloS One. (2019) 14:e0226841. doi: 10.1371/journal.pone.0226841
58. Li Z, Liu T, Zhu G, Lin H, Zhang Y, He J, et al. Dengue baidu search index data can improve the prediction of local dengue epidemic: A case study in guangzhou, China. PloS Negl Trop Dis. (2017) 11:e0005354. doi: 10.1371/journal.pntd.0005354
59. Beatty ME, Stone A, Fitzsimons DW, Hanna JN, Lam SK, Vong S, et al. Best practices in dengue surveillance: a report from the asia-pacific and americas dengue prevention boards. PloS Negl Trop Dis. (2010) 4:e890. doi: 10.1371/journal.pntd.0000890
60. Raafat N, Blacksell SD, Maude RJ. A review of dengue diagnostics and implications for surveillance and control. Trans R Soc Trop Med Hygiene. (2019) 113:653–60. doi: 10.1093/trstmh/trz068
61. Pollett S, Melendrez M, Berry IM, Duchêne S, Salje H, Cummings D, et al. Understanding dengue virus evolution to support epidemic surveillance and counter-measure development. Infection Genet Evol. (2018) 62:279–95. doi: 10.1016/j.meegid.2018.04.032
62. Haynes CL, Cook GA, Jones MA. Legal and ethical considerations in processing patientidentifiable data without patient consent: lessons learnt from developing a disease register. J Med Ethics. (2007) 33:302–7. doi: 10.1136/jme.2006.016907
63. Gliklich RE, Dreyer NA, Leavy MB. “Registries for evaluating patient outcomes: A user’s guide. AHRQ Methods for Effective Health Care. 3rd edition”. Rockville (MD): Agency for Healthcare Research and Quality (US); Report No.: 13(14)-EHC111. (2014). Available online at: https://www.ncbi.nlm.nih.gov/books/NBK208616/
64. Cohen IG, Amarasingham R, Shah A, Xie B, Lo B. The legal and ethical concerns that arise from using complex predictive analytics in health care. Health affairs. (2014) 33:1139–47. doi: 10.1377/hlthaff.2014.0048
65. Michelson KN, Klugman CM, Kho AN, Gerke S. Ethical considerations related to using machine learning-based prediction of mortality in the pediatric intensive care unit. J Pediatr. (2022) 247:125–8. doi: 10.1016/j.jpeds.2021.12.069
66. Olawade DB, Wada OJ, David-Olawade AC, Kunonga E, Abaire O, Ling J. Using artificial intelligence to improve public health: a narrative review. Front Public Health. (2023) 11:1196397. doi: 10.3389/fpubh.2023.1196397
67. Lee LM, Ortiz SE, Pavela G, Jennings B. Public health code of ethics: Deliberative decision-making and reflective practice. Am J Public Health. (2020) 110:489–91. doi: 10.2105/AJPH.2020.305568
68. Taylor-Salmon E, Hill V, Paul LM, Koch RT, Breban MI, Chaguza C, et al. Travel surveillance uncovers dengue virus dynamics and introductions in the caribbean. Nat Commun. (2024) 15:3508. doi: 10.1038/s41467-024-47774-8
69. Cattarino L, Rodriguez-Barraquer I, Imai N, Cummings DA, Ferguson NM. Mapping global variation in dengue transmission intensity. Sci Trans Med. (2020) 12:eaax4144. doi: 10.1126/scitranslmed.aax4144
70. Constenla D, Armien B, Arredondo J, Carabali M, Carrasquilla G, Castro R, et al. Costing dengue fever cases and outbreaks: recommendations from a costing dengue working group in the americas. Value Health Regional Issues. (2015) 8:80–91. doi: 10.1016/j.vhri.2015.06.001
71. Wettstein ZS, Fleming M, Chang AY, Copenhaver DJ, Wateska AR, Bartsch SM, et al. Total economic cost and burden of dengue in Nicaragua: 1996–2010. Am J Trop Med hygiene. (2012) 87:616. doi: 10.4269/ajtmh.2012.12-0146
72. Rodriguez RC, Galera-Gelvez K, Yescas JGL, Rueda-Gallardo JA. Costs of dengue to the health system and individuals in Colombia from 2010 to 2012. Am J Trop Med hygiene. (2015) 92:709. doi: 10.4269/ajtmh.14-0386
73. Zubieta-Zavala A, Salinas-Escudero G, Ramírez-Chávez A, García-Valladares L, López-Cervantes M, López Yescas JG, et al. Calculation of the average cost per case of dengue fever in Mexico using a micro-costing approach. PloS Negl Trop Dis. (2016) 10:e0004897. doi: 10.1371/journal.pntd.0004897
74. W.H.O. Early detection, assessment and response to acute public health events: implementation of early warning and response with a focus on event-based surveillance: interim version. Tech. rep., Geneva, Switzerland: World Health Organization (2014).
75. Runge-Ranzinger S, Kroeger A, Olliaro P, McCall PJ, Sanchez´ Tejeda G, Lloyd LS, et al. Dengue contingency planning: from research to policy and practice. PloS Negl Trop Dis. (2016) 10:e0004916. doi: 10.1371/journal.pntd.0004916
76. Buczak AL, Koshute PT, Babin SM, Feighner BH, Lewis SH. A data-driven epidemiological prediction method for dengue outbreaks using local and remote sensing data. BMC Med Inf decision making. (2012) 12:1–20. doi: 10.1186/1472-6947-12-124
77. Gitler I, Gomes ATA, Nesmachnow S. The latin american supercomputing ecosystem for science. Commun ACM. (2020) 63:66–71. doi: 10.1145/3419977
78. Barroso-Flores J. Accelerating economic development in latin america through overcoming access challenges to supercomputing infrastructure. Nat Comput Sci. (2024) 4:644–5. doi: 10.1038/s43588-024-00686-5
79. Sistema de Computacion Avanzada para America Latina y el Caribe (SCALAC). HighPerformance Computing Robust Systems Report in Latin America and Caribbean” Ver.2.3. (2024). Tech. rep., Sistema de Computacion Avanzada para America Latina y el Caribe (SCALAC (Accessed 22-12-2024).
80. Boumadan M, Torres M, Valdés RG, Castro FF. Technology and the digital divide in Panama. In: From Digital Divide to Digital Inclusion: Challenges, Perspectives and Trends in the Development of Digital Competences. London, United Kingdom: Springer (2024). p. 395–409.
81. U.S. Department of State. 2020 Investment Climate Statements: Panama. Tech. rep., Washington, D.C., United States: U.S. Department of State (2020).
82. Whiteman A, Desjardins MR, Eskildsen GA, Loaiza JR. Detecting space-time clusters of dengue fever in Panama after adjusting for vector surveillance data. PloS Negl Trop Dis. (2019) 13:e0007266. doi: 10.1371/journal.pntd.0007266
83. Wimberly M. Meteorological data for public health surveillance. Open Access Government. (2024) 42:1–4. doi: 10.56367/OAG-042-10923
84. Tipton WLM. Who will make sense of all the data? Assessing the impacts of technology on the weather, water, and climate workforce. Tech. rep., Boston, MA, United States: The American Meteorological Society (2021). p. E.
85. Ramiro MA. Data protection in latin america. In: Research Handbook on Privacy and Data Protection Law. Cheltenham, United Kingdom: Edward Elgar Publishing (2022). p. 140–59.
86. Zaki R, Roffeei SN, Hii YL, Yahya A, Appannan M, Said MA, et al. Public perception and attitude towards dengue prevention activity and response to dengue early warning in Malaysia. PloS One. (2019) 14:e0212497. doi: 10.1371/journal.pone.0212497
Keywords: dengue virus, arbovirus, epidemiological surveillance, outbreak, one health, mosquito vectors, early detection
Citation: Ureña GE, Diaz Y, Pascale JM, López-Vergès S and Sanchez-Galan JE (2025) A framework for the early detection and prediction of dengue outbreaks in the Republic of Panama. Front. Trop. Dis. 5:1465856. doi: 10.3389/fitd.2024.1465856
Received: 17 July 2024; Accepted: 30 December 2024;
Published: 27 January 2025.
Edited by:
Jorge Abelardo Falcón-Lezama, Universidad Juárez Autónoma de Tabasco, MexicoReviewed by:
Maria Jesus Rios-Blancas, National Institute of Public Health, MexicoCopyright © 2025 Ureña, Diaz, Pascale, López-Vergès and Sanchez-Galan. This is an open-access article distributed under the terms of the Creative Commons Attribution License (CC BY). The use, distribution or reproduction in other forums is permitted, provided the original author(s) and the copyright owner(s) are credited and that the original publication in this journal is cited, in accordance with accepted academic practice. No use, distribution or reproduction is permitted which does not comply with these terms.
*Correspondence: Javier E. Sanchez-Galan, amF2aWVyLnNhbmNoZXpnYWxhbkB1dHAuYWMucGE=
†These authors have contributed equally to this work and share first authorship