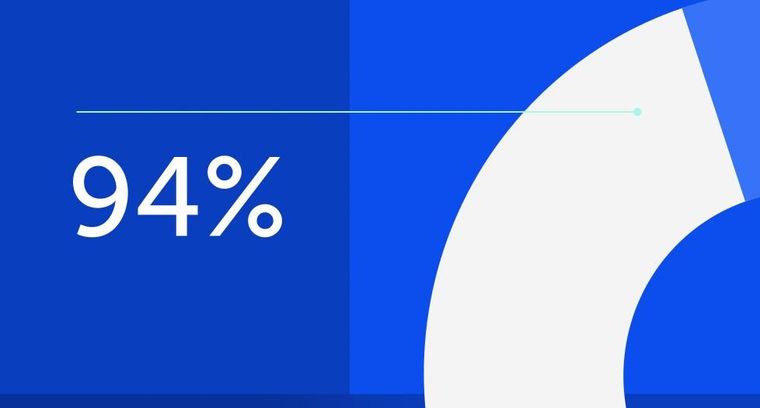
94% of researchers rate our articles as excellent or good
Learn more about the work of our research integrity team to safeguard the quality of each article we publish.
Find out more
BRIEF RESEARCH REPORT article
Front. Trop. Dis., 30 September 2024
Sec. Major Tropical Diseases
Volume 5 - 2024 | https://doi.org/10.3389/fitd.2024.1454252
This article is part of the Research TopicDigital Tools and Innovation for the Prevention and Control of Vector-borne DiseasesView all 6 articles
Artesunate-mefloquine combination therapy (AR-MQ) is a standard therapy for treating uncomplicated malaria by Plasmodium falciparum. Time-to-event (TTE) analysis is used to describe the occurrence and timing of events by yielding information about the risk of an event occurring during a specific period. Therefore, the aim of the present study is to evaluate the efficacy of AR-MQ combination therapy on the survival time of Plasmodium berghei-infected mice using TTE analysis. Here, TTE analysis was used to analyze P. berghei-infected mice receiving a single oral dose of 100 mg/kg artesunate and 55 mg/kg mefloquine or dose-matched artesunate monotherapy. Median survival was higher for AR-MQ than for monotherapy. A survival analysis to evaluate the influence of treatment on survival was performed using MonolixSuite™. The Weibull model best described the mortality time of the animals. Subsequent analysis identified that AR-MQ had a significant influence on population survival time (Te_pop), estimated at 13.66 days, population parameter for curve fitting (p_pop) at 4.39, and survival time under AR-MQ treatment (beta Te_AR-MQ) at 0.77 days. The probability of survival 7, 15, and 30 days after treatment with AR-MQ was 94.4%, 88.9%, and 14.9%, respectively. The experimental and modeling data both found that AR-MQ combination therapy yielded increased survival of infected animals.
Artemisinin-based combined therapy (ACT) is the standard treatment for children and adults with uncomplicated malaria caused by Plasmodium falciparum (1). This combination consists of an artemisinin derivative, which rapidly reduces parasite viability but has a very short plasma half-life, associated with a longer-acting drug, which should remove residual parasites and prevent parasite recrudescence. ACT can be more effective than standard monotherapy for different reasons. In terms of pharmacodynamics, it can yield additive antimalarial effects and mitigate drug-resistance issues, among other advantages (2, 3). Regarding pharmacokinetics, ACT can provide rapid and prolonged action, as it is composed of one shorter- and one longer-acting drug.
ACT was launched in 2001 and there are currently at least five different formulations recommended by WHO, although each country can choose which to authorize. One example of these therapies is a co-formulated pill of artemether and lumefantrine marketed under the trade name Coartem®, which has gained particular popularity worldwide. In comparison, artesunate-mefloquine (AR-MQ) remained relatively unknown for many decades because of a lack of clinically important knowledge about the pharmacokinetic–pharmacodynamic relationship between its components and the determinants of interpatient pharmacokinetic and pharmacodynamic variability. Nonetheless, AR-MQ is the first-line treatment for uncomplicated falciparum malaria, P. vivax malaria, and mixed infection by unknown species in many countries in Latin America (4, 5). It was only in recent years that the first modeling and experimental endeavors were made to elucidate the pharmacokinetics of this ACT and the reduced parasite viability associated with it (6–8).
Increased understanding of AR-MQ is needed to inform decisions concerning the dosage regimen and duration of treatment using this drug. One way to fill this knowledge gap is to use time-to-event (TTE) analysis (9). TTE analysis, also referred to as survival analysis, is an approach used to describe, explain, and predict the occurrence and timing of events. It is highly effective, when used appropriately, in producing critical information about the risk of an event happening over a specific period of time (10). The data used in TTE analyses describe the prognosis of diseases or drug effects, such as overall survival, time to disease progression, etc., and therefore survival data on animals that were treated or not treated with different antimalarials. To achieve this, existing parametric models can be used to perform simulations and evaluate the statistical significance of the covariates (11).
The efficacy of antimalarial compounds/drugs can be assessed through in vivo preclinical trials using rodent models, which are similar in physiology, lifecycle, and structure to human malaria parasites and can help develop understanding of their biology and pathogenesis (12, 13). P. berghei has traditionally been used to investigate the biology of liver-stage and asexual blood-stage parasites, the progression of liver disease, and also the resulting host immunity (14). With data obtained from in vivo pre-clinical trials, it is possible to build up mathematical and statistical models to describe and understand the efficacy and effect of drugs, facilitating the translation of results from pre-clinical trials into clinical trials, and also providing a theoretical basis for drug effectiveness and the chances of failure (15, 16).
To our knowledge, TTE modeling is not yet routinely used in preclinical studies. Therefore, the aim of the present study is to evaluate the efficacy of AR-MQ combination therapy on the survival time of Plasmodium berghei-infected mice using TTE analysis.
Male and female Swiss mice (6 to 8 weeks old, 18 to 22 g) were obtained from the Animal Resources Unit of Instituto Gonçalo Moniz (Fiocruz, Salvador, Brazil) and housed in cages under a 12-hour light/dark cycle with food and water ad libitum. The experimental protocol was approved by the Instituto Gonçalo Moniz ethics committee (approval no. 015/2022). Mice were infected with P. berghei (ANKA/Luc/GFP strain) (17), and infection was maintained by weekly blood passage via intraperitoneal injection of a standard inoculum of 1 x 106 parasitized erythrocytes in 200 µL 0.9% saline solution. Parasitemia was determined by May-Grunwald-Giemsa staining of smeared peripheral blood collected from tail veins.
Approximately 5 days post-infection, when infection reached > 5% parasitemia, as determined by flow cytometry to detect the GFP signal (BD LSRFortessa™ Cell Analyzer, Piscataway, NJ, USA), mice were randomly divided into three groups (n = 6/group): AR monotherapy (100 mg/kg), AR-MQ combination therapy (100 mg/kg and 55 mg/kg, respectively), and untreated (vehicle only). Drugs were administered in a single dose orally, and survival was monitored up to 30 days.
A TTE model was developed, in which the event was defined as death over time (days). Monitoring of animal death began after administration of treatment, with death overtime in the AR-MQ group being compared with death overtime in the vehicle (control) and AR groups.
The analysis was carried out using the Monolix Suite™ 2023R1 (Lixoft, France), with parametric models, adjusting the hazard function (h), and analysis of the influence of treatment. The data were analyzed with the most commonly used models: exponential, Weibull, log-logistic, uniform, Gompertz, and gamma:
Exponential
Weibull
log-logistic
Uniform
Gompertz
Gamma
The models were compared to identify the one that best captured the data on survival time and presented the lowest objective function value (OFV), a difference of at least -3.84, and acceptable Bayesian information criteria (BIC) and relative standard error (RSE, %). Their performance was also evaluated by visual predictive check (VPC), in which the Kaplan-Meier curve of the observed data is overlaid on the 90% prediction interval of the simulated data from the TTE model with 1,000 replicates.
After choosing the model, the influence of treatment on the AR and AR-MQ groups was analyzed against the untreated group (reference group). The influence of treatment was considered significant when the Wald test p-value < 0.05. At the end of the analysis, the random effects were removed from the model. With the estimated parameters, the survival function (S(t)) and the probability of the event occurring within 7, 15, and 30 days were calculated. S(t) is described by the hazard in a given time that describes the probability of an event failing to occur within that time, such that survival is:
where, t is time and S(t) is survival at time t.
The TTE model consisted of a total of 18 mice from three intervention groups. All animals were followed until the event (death) or the conclusion of the experiment on day 30, generating the Kaplan-Meier survival curve over time (days) (Figure 1) with stratification by type of treatment.
Figure 1. Kaplan-Meier survival curve of TTE data (upper panel); Kaplan-Meier curve stratified by treatment: vehicle (yellow), AR monotherapy (blue), and ARMQ combination (red) (down panel).
The Weibull model was selected from the hazard function models tested (Table 1) because it was the one that provided a better description of the survival curve for the data, as seen by the BIC and OFV values, than the exponential model (ΔOFV = -33.69) and uniform model (ΔOFV = -9.71). Although the OFV values of the log-logistic, Gompertz, and gamma models were very close, there were significant differences in their BIC values.
The analysis of the influence of treatment using the BIC values and the Wald test revealed a significant difference between AR-MQ and the untreated group for Te (p-value = 1.25 x 10-8) (Table 2). The hazard function was defined as:
Table 2. Estimated parameters and influence of treatment on treatment hazard based on the final model.
where, , , , and are the individual and population parameters of the hazard function. The AR-MQ group fits the model parameter . Te is the parameter of interest; p is the curve-fitting parameter, and t is time.
The VPC of the model was obtained and stratified by treatment, with the Kaplan-Meier curve of the observed data superimposed on the 90% prediction interval calculated from the model simulations, within the confidence interval (Figure 2). The results of the VPC indicated that there was no misspecification and that the model adequately described the data and had the ability to predict the observed Kaplan Meier survival.
Figure 2. Visual predictive check (VPC) of the empirical Kaplan-Meier curve overlaid on the 90% prediction interval calculated from model simulations (upper panel); VPC stratified by treatment groups (down panel).
The estimated parameters (Table 2) demonstrate a mean population Te (survival time) of 13.66 days, with a significant difference between the AR-MQ and the untreated group, without influencing the parameter p (curve adjustment parameter).
The probability of survival S(t) of mice infected with P. berghei and at 7, 15, and 30 days was 86.9%, 78.9%, and 1.8%, after receiving AR monotherapy and 94.4%, 88.9%, and 14.9%, AR-MQ combination therapy respectively.
This study demonstrated the survival of P. berghei-infected mice treated with AR-MQ combination therapy compared to AR monotherapy and the vehicle only (control). The results demonstrate that the mean time until death for the population (Te) was 13.66 days. When analyzing the influence of treatment, Te values of 0.19 and 0.77 days were obtained for the AR monotherapy and AR-MQ groups, respectively, with a significant difference for the AR-MQ group. These results demonstrate that animals receiving AR-MQ took longer to die than animals receiving AR monotherapy. The equation was generated. The curve adjustment parameter, p, was estimated at 4.39 and showed no influence of treatment (Table 2).
The probability of survival at 7, 15, and 30 days after drug administration was calculated for the two drug-receiving groups. When AR-MQ was given (vs. AR monotherapy), the probability of survival was higher at all three times: 94.4% (vs. 86.9%) after 7 days, 88.9% (vs. 78.9%) after 15 days and 14.9% (vs. 1.8%) after 30 days.
ACT AR-MQ is considered the first-line treatment for P. falciparum in the Americas, South-East Asia Region, and Western Pacific Region (18). It is characterized by an artemisinin derivative, which rapidly reduces parasitemia during the first 3 days of treatment but has a very short plasma half-life, associated with a longer-acting drug, which should remove residual parasites and indicated for the treatment of uncomplicated malaria (2). The general recommendation is hospital admission and oral treatment for at least 24–48 h, intending to observe clinical evolution and drug tolerance (19). Otherwise, AR monotherapy is indicated for the treatment of severe malaria with intravenous administration (20) followed by an ACT for three days, and almost all patients infected with artemisinin-resistant parasites who are treated with an ACT are completely cured (18). In case there is a need to administer an ACT, such as AR-MQ, in both forms of malaria caused by P. falciparum.
The TTE model was chosen after comparing six parametric models, and their suitability was assessed using a VPC plot based on simulation and statistical fitting. The Weibull model described the data significantly better than the others. Although the Weibull, log-logistic, Gompertz, and gamma models all presented similar -2 x log-likelihood (OFV) values, the BIC was lowest for the Weibull model (Table 1). This model adequately described the animals’ survival and the influence of the different treatments, as shown by the VPC (Figure 2). The TTE model was robust enough to estimate the survival time of animals receiving different treatments and to predict the survival probabilities, and was therefore capable of providing a reliable description of the data.
The use of TTE in the analysis of malaria survival has not been reported in the literature for pre-clinical data, but it has been reported for clinical data. In such studies, it has been used to estimate the prevalence of the disease in specific populations, such as pregnant women, and measure its impact on newborns (21), and to estimate the probability of delayed onset of malaria in association with sickle cell disease (22). TTE has also been employed in veterinary medicine, where data from clinical trials have been used to investigate the relationship between the effectiveness of therapies and patient prognosis (23). TTE models can deal with censoring and describe the occurrence of a single type of adverse effect over time. The proportional hazards model (or Cox model) is the most widely used TTE regression model, as it can be used for analyses of long-term toxicity with censoring, non-recurrent adverse effects, and time-dependent covariables, such as drug exposure (24). Regarding drug exposure, TTE can adequately describe the relationship between efficacy and safety, specifying how medication use will affect the risk of an event of interest occurring (25). For malaria, this methodology can be used to analyze data from clinical trials (26, 27), as well as the efficacy and effects of antimalarial vaccines that are being developed (28), and to analyze the survival of animals infected with malaria and evaluate the influence of different pharmacological treatments, as well as the probability of survival over a given period of time. These predictions can be used to interpret data from robust pre-clinical and clinical studies investigating the treatment of malaria.
The TTE model may be used as a surveillance tool to inform health authorities about the need for policy adjustments in malaria treatment. This quantitative approach supports evidence-based decision-making, guiding the development of new treatment guidelines or the introduction of alternative therapies as needed.
Despite this, it is essential to emphasize that TTE analysis has limitations. Among them, there is an inability to provide an estimate of effect or the related confidence interval to compare survival in different groups; it does not allow adjustment for confounding factors in etiological research or predictors in prognostic research; and it requires data categorization, not being possible to increase or decrease the relative risk of a given event associated with a one-unit increase (29).
In conclusion, the experimental and modeling data suggest that AR-MQ combination therapy produced increased survival of P. berghei-infected mice.
The raw data supporting the conclusions of this article will be made available by the authors, without undue reservation.
The animal study was approved by Instituto Gonçalo Moniz ethics committee (approval no. 015/2022). The study was conducted in accordance with the local legislation and institutional requirements.
VV: Data curation, Formal analysis, Investigation, Methodology, Software, Visualization, Writing – original draft. LC: Data curation, Investigation, Methodology, Writing – review & editing. Ad: Investigation, Methodology, Writing – review & editing. HQ: Investigation, Methodology, Writing – review & editing. DM: Funding acquisition, Resources, Supervision, Writing – review & editing. FJ: Conceptualization, Funding acquisition, Project administration, Supervision, Visualization, Writing – review & editing.
The author(s) declare financial support was received for the research, authorship, and/or publication of this article. The authors wish to thank CNPq (Brazil, grant numbers 305732/2019-6 and 440227/2022-4) and Fiocruz/Proep (Brazil, grant number IGM-002-FIO-20-2-25). This study was financed in part by the Coordenação de Aperfeiçoamento de Pessoal de Nível Superior -Brasil (CAPES) -Finance Code 001.
The authors declare that the research was conducted in the absence of any commercial or financial relationships that could be construed as a potential conflict of interest.
All claims expressed in this article are solely those of the authors and do not necessarily represent those of their affiliated organizations, or those of the publisher, the editors and the reviewers. Any product that may be evaluated in this article, or claim that may be made by its manufacturer, is not guaranteed or endorsed by the publisher.
1. Ashley EA, Pyae Phyo A, Woodrow CJ. Malaria. Lancet. (2018) 391:1608–21. doi: 10.1016/S0140-6736(18)30324-6
2. Bukirwa H, Orton L. Artesunate plus mefloquine versus mefloquine for treating uncomplicated malaria. Cochrane Database Syst Rev. (2005) 2005(4):CD004531. doi: 10.1002/14651858.CD004531.pub2
3. Zaloumis SG, Cao P, Dini S, Davenport MP, Cromer D, Khoury DS, et al. In silico investigation of the decline in clinical efficacy of artemisinin combination therapies due to increasing artemisinin and partner drug resistance. Antimicrob Agents Chemother. (2018) 62:e01292–18. doi: 10.1128/AAC.01292-18
4. de Freitas BS, Fernandes GH, Pereira ACEdaS, Peixoto HM. Artesunate-mefloquine therapy for uncomplicated Plasmodium falciparum malaria: an updated systematic review and meta-analysis of efficacy and safety. Trans R Soc Trop Med Hyg. (2024) 118:84–94. doi: 10.1093/trstmh/trad069
5. Hernandez Maldonado J, Grundmann O. Drug-drug interactions of artemisinin-based combination therapies in malaria treatment: A narrative review of the literature. J Clin Pharmacol. (2022) 62:1197–205. doi: 10.1002/jcph.2073
6. Aogo RA, Khoury DS, Cromer D, Elliott T, Akter J, Fogg LG, et al. Quantification of host-mediated parasite clearance during blood-stage Plasmodium infection and anti-malarial drug treatment in mice. Int J Parasitol. (2018) 48:903–13. doi: 10.1016/j.ijpara.2018.05.010
7. Khoury DS, Cromer D, Elliott T, Soon MSF, Thomas BS, James KR, et al. Characterising the effect of antimalarial drugs on the maturation and clearance of murine blood-stage Plasmodium parasites in vivo. Int J Parasitol. (2017) 47:913–22. doi: 10.1016/j.ijpara.2017.05.009
8. Radohery GFR, Walz A, Gumpp C, Cherkaoui-Rbati MH, Gobeau N, Gower J, et al. Parasite viability as a measure of in vivo drug activity in preclinical and early clinical antimalarial drug assessment. Antimicrob Agents Chemother. (2022) 66:e0011422. doi: 10.1128/aac.00114-22
9. Hughes E, Wallender E, Mohamed Ali A, Jagannathan P, Savic RM. Malaria PK/PD and the role pharmacometrics can play in the global health arena: malaria treatment regimens for vulnerable populations. Clin Pharmacol Ther. (2021) 110:926–40. doi: 10.1002/cpt.2238
10. Denfeld QE, Burger D, Lee CS. Survival analysis 101: an easy start guide to analysing time-to-event data. Eur J Cardiovasc Nurs. (2023) 22:332–7. doi: 10.1093/eurjcn/zvad023
11. Lim H-S. Brief introduction to parametric time to event model. Transl Clin Pharmacol. (2021) 29:1–5. doi: 10.12793/tcp.2021.29.e7
12. Harris JV, Bohr TM, Stracener C, Landmesser ME, Torres V, Mbugua A, et al. Sequential Plasmodium chabaudi and Plasmodium berghei infections provide a novel model of severe malarial anemia. Infect Immun. (2012) 80:2997–3007. doi: 10.1128/IAI.06185-11
13. Raz A. Plasmodium berghei infection in BALB/c mice model as an animal model for malaria disease research. Methods Mol Biol. (2022) 2410:589–95. doi: 10.1007/978-1-0716-1884-4_31
14. Simwela NV, Waters AP. Current status of experimental models for the study of malaria. Parasitology. (2022) 149:1–22. doi: 10.1017/S0031182021002134
15. Atcheson E, Bauza K, Reyes-Sandoval A. A probabilistic model of pre-erythrocytic malaria vaccine combination in mice. PLoS One. (2019) 14:e0209028. doi: 10.1371/journal.pone.0209028
16. Bandeira LC, Pinto L, Carneiro CM. Pharmacometrics: the already-present future of precision pharmacology. Ther Innov Regul Sci. (2023) 57:57–69. doi: 10.1007/s43441-022-00439-4
17. Franke-Fayard B, Janse CJ, Cunha-Rodrigues M, Ramesar J, Büscher P, Que I, et al. Murine malaria parasite sequestration: CD36 is the major receptor, but cerebral pathology is unlinked to sequestration. Proc Natl Acad Sci. (2005) 102:11468–73. doi: 10.1073/pnas.0503386102
18. World malaria report 2023. Geneva: World Health Organization (2023). Licence: CC BY-NC-SA 3.0 IGO. Available at: https://www.who.int/teams/global-malaria-programme/reports/world-malaria-report-2023.
19. Pousibet-Puerto Joaquín, Salas-Coronas Joaquín, Sánchez-Crespo A, Molina-Arrebola MA, Soriano-Pérez MJ, Giménez-López MJosé, et al. Impact of using artemisinin-based combination therapy (ACT) in the treatment of uncomplicated malaria from plasmodium falciparum in a non-endemic zone. Malaria J. (2016) 15:339. doi: 10.1186/s12936-016-1408-1
20. Botta A, Comelli A, Vellere I, Chechi F, Bianchi L, Giulia G, et al. Artesunate Monotherapy versus Artesunate plus Quinine Combination Therapy for Treatment of Imported Severe Malaria: A TropNet Retrospective Cohort Study. Infection. (2022) 50:949–58. doi: 10.1007/s15010-022-01771-5
21. Bal M, Ghosal J, Das A, Sandeepta S, Pati S, Dutta A, et al. Impact of sub-patent malaria during pregnancy on birth-weight in Odisha, India: time-to-event analysis of prospective longitudinal follow-up of a survey. J Epidemiol Glob Health. (2023) 13:23–31. doi: 10.1007/s44197-022-00082-0
22. Crompton PD, Traore B, Kayentao K, Doumbo S, Ongoiba A, Diakite SAS, et al. Sickle cell trait is associated with a delayed onset of malaria: implications for time-to-event analysis in clinical studies of malaria. J Infect Dis. (2008) 198:1265–75. doi: 10.1086/592224
23. Oyama MA, Shaw PA, Ellenberg SS. Considerations for analysis of time-to-event outcomes subject to competing risks in veterinary clinical studies. J Vet Cardiol. (2018) 20:143–53. doi: 10.1016/j.jvc.2018.03.001
24. Coz E, Fauvernier M, Maucort-Boulch D. An overview of regression models for adverse events analysis. Drug Saf. (2024) 47:205–16. doi: 10.1007/s40264-023-01380-7
25. Danieli C, Abrahamowicz M. Competing risks modeling of cumulative effects of time-varying drug exposures. Stat Methods Med Res. (2019) 28:248–62. doi: 10.1177/0962280217720947
26. de Oliveira AM, Chavez J, de Leon GP, Durand S, Arrospide N, Roberts J, et al. Efficacy and effectiveness of mefloquine and artesunate combination therapy for uncomplicated plasmodium falciparum malaria in the Peruvian amazon. Am J Trop Med Hygiene. (2011) 85:573–78. doi: 10.4269/ajtmh.2011.11-0250
27. Santelli AC, Ribeiro I, Daher André, Boulos M, Marchesini PB, La Corte dos Santos R, et al. Effect of artesunate-mefloquine fixed-dose combination in malaria transmission in amazon basin communities. Malaria J. (2012) 11:286. doi: 10.1186/1475-2875-11-286
28. Cheung YB, Ma X, Lam KF, Milligan eP. Estimation of the primary, secondary and composite effects of malaria vaccines using data on multiple clinical malaria episodes. Vaccine. (2020) 38:4964–69. doi: 10.1016/j.vaccine.2020.05.086
Keywords: survival analysis, malaria, Plasmodium berghei, Plasmodium falciparum, antimalarial drug combination
Citation: Vieira Santos V, Campos Pereira L, dos Santos Miranda ALL, Quadros HC, Magalhães Moreira DR and Johansson Azeredo F (2024) Efficacy of artesunate-mefloquine combination therapy on survival in Plasmodium berghei-infected mice: a time-to-event analysis. Front. Trop. Dis 5:1454252. doi: 10.3389/fitd.2024.1454252
Received: 24 June 2024; Accepted: 10 September 2024;
Published: 30 September 2024.
Edited by:
Vanderson de Souza Sampaio, Fundação de Medicina Tropical Doutor Heitor Vieira Dourado (FMT-HVD), BrazilReviewed by:
Claudia Rios-Velásquez, Instituto Leônidas & Maria Deane (ILMD/Fiocruz Amazônia), BrazilCopyright © 2024 Vieira Santos, Campos Pereira, dos Santos Miranda, Quadros, Magalhães Moreira and Johansson Azeredo. This is an open-access article distributed under the terms of the Creative Commons Attribution License (CC BY). The use, distribution or reproduction in other forums is permitted, provided the original author(s) and the copyright owner(s) are credited and that the original publication in this journal is cited, in accordance with accepted academic practice. No use, distribution or reproduction is permitted which does not comply with these terms.
*Correspondence: Francine Johansson Azeredo, ZnJhbmNpbmVqQHVmbC5lZHU=
Disclaimer: All claims expressed in this article are solely those of the authors and do not necessarily represent those of their affiliated organizations, or those of the publisher, the editors and the reviewers. Any product that may be evaluated in this article or claim that may be made by its manufacturer is not guaranteed or endorsed by the publisher.
Research integrity at Frontiers
Learn more about the work of our research integrity team to safeguard the quality of each article we publish.