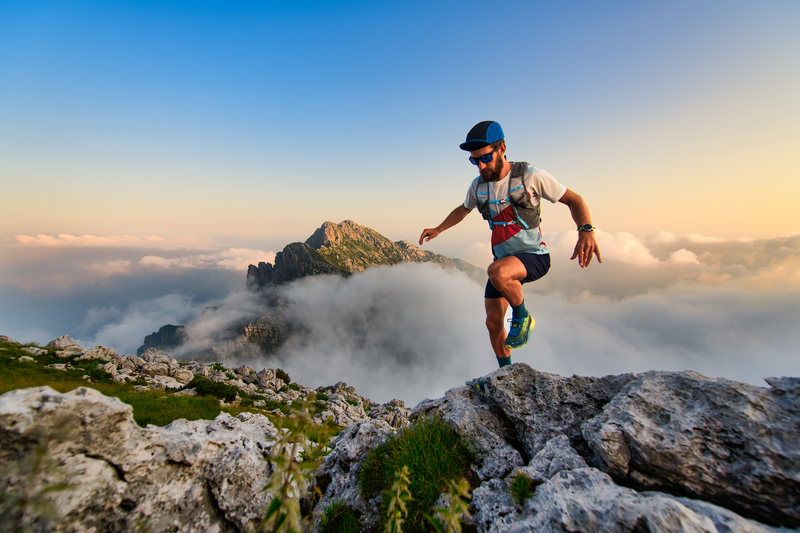
94% of researchers rate our articles as excellent or good
Learn more about the work of our research integrity team to safeguard the quality of each article we publish.
Find out more
REVIEW article
Front. Trop. Dis. , 28 July 2023
Sec. Major Tropical Diseases
Volume 4 - 2023 | https://doi.org/10.3389/fitd.2023.1151336
This article is part of the Research Topic Recent Advances In “Omics” Of Tropical Diseases View all 5 articles
The molecular biology tools available since the early 1970s have been crucial to the development of molecular epidemiology as an important branch of public health, and are used for the identification of host genetic and environmental factors associated with both communicable (CDs) and non-communicable diseases (NCDs) across human and animal populations. Molecular epidemiology has significantly contributed to the understanding of etiological agents, disease distribution, and how to track outbreaks, as well as to prevention and control measures against tropical infectious diseases. However, there have been significant limitations compromising the successful application of molecular epidemiology in low-to-middle income countries (LMICs) to address complex issues at the animal–human–environment interface. Recent advances in our capacity to generate information by means of high-throughput DNA genomic sequencing, transcriptomics, and metabolomics have allowed these tools to become accessible at ever-lower costs. Furthermore, recently emerged omics fields such as lipidomics are improving our insights into molecular epidemiology by measuring lipid phenotypes that gauge environmental and genetic factors in large epidemiological studies. In parallel, the development of bioinformatic tools has revolutionized the utility of omics, providing novel perspectives to better characterize pools of biological molecules and translate them into the structure, function, and dynamics of organisms. Unfortunately, the use of such powerful tools has not been optimal for a One Health approach to both CDs and NCDs, particularly in low-resource tropical settings. The aim of this review is to present the fundamentals of omics tools and their potential use in molecular epidemiology, and to critically discuss the impact of omics on the evolving One Health dimension applied to tropical diseases. We use Ethiopia and Brazil as model systems to illustrate existing gaps and opportunities, while also addressing global applications. Moreover, we also discuss perspectives on exploring omics based molecular epidemiology in the context of One Health as a crucial approach to preventing and mitigating the burden of CDs and NCDs at the interface of human health, animal health, and the environment. This review shows that building capacity in the tropical regions is crucial to establishing equitable global health.
Today, our planet is facing major complex global health challenges. Approximately 75% of public health emergencies associated with infectious diseases are of animal or environmental origin (1). Tropical regions such as sub-Saharan Africa, Latin America, and Southeast Asia are known to be major hotspots of emerging and re-emerging infectious zoonotic diseases of global significance (2). Furthermore, the consistent increase in major chronic non-communicable diseases (NCDs) in the tropical belt is known to be associated with environmental factors, mainly chemical hazards (3). The One Health approach addresses such complex issues at the interface of human health, animal health, plant health, and the environment (4). Recent examples of major epidemics and pandemics that necessitated One Health-oriented initiatives for prevention or mitigation include COVID-19, Ebola virus disease (EVD), Zika virus, Middle East respiratory syndrome (MERS), and many emerging and re-emerging vector-borne diseases, including dengue fever and chikungunya (5, 6).
Tropical regions, particularly those in developing countries, have experienced significant limitations in implementing these genotypic approaches to address epidemiologic problems with both communicable (CDs) and non-communicable (NCDs) diseases. We have previously reported on a detailed review of the use of molecular epidemiology to address leading bacterial and viral zoonotic diseases, and addressed the critical capacity-building needs at the interface of human health and animal health (4, 7). The problem is further accentuated with regard to the application of omics approaches. Although the use of omics approaches is rapidly expanding worldwide, with more advanced tools at ever-lower running costs, their implementation remains very limited in developing tropical regions compared with in developed regions where there is usually ample laboratory infrastructure and well-trained personnel. Moreover, although high-resolution genomic epidemiology can provide invaluable information about dissemination patterns of infectious agents, as shown in the COVID-19 pandemic (8), there is a paucity of information about the role of the environment and other potentials reservoirs of infectious agents (including zoonotic pathogens) in developing tropical regions. The successful integration of omics and non-omics data in analytical epidemiological models is a key bottleneck, and the application of more effective and relevant bioinformatics approaches to epidemiologic investigations is required (9).
Most diseases are complex in nature and result from the interaction of biotic and abiotic hazards (such as pathogens) with environmental factors or exposures at the system-level interface. Therefore, understanding these complex associations requires modeling exhaustive and appropriate data that characterize in detail such features and conditions at the molecular level. The statistical power of the strength of association between risk factors and disease states is weakened by the fact that phenotypes of different exposures are coded, not only at the genetic level but also at the macromolecular (lipid, protein, etc.) and molecular (fatty acid, amino acid, etc.) levels of detail.
In this review, we describe the conceptual fundamentals and current status of the use of various omics to address tropical and global diseases of significance to the One Health approach, including examples concerning foodborne, waterborne, vector-borne, and interrelated chronic diseases. We will highlight gaps associated with implementing omics tools in tropical developing regions using model systems from Latin America (Brazil) and eastern Africa (Ethiopia). These two tropical countries were chosen based on convenience and yet they represent developing regions with a distinct disparity in terms of economic status and availability of skilled personnel and laboratory infrastructure. We will highlight details of how genomics, metagenomics, transcriptomics, and metabolomics, such as lipidomic tools, are used to address communicable and non-communicable diseases. Finally, we recommend potential ways to develop and strengthen capacity for the application of omics tools and associated bioinformatic analyses in tropical developing regions.
The term “molecular epidemiology” emerged around the early 1970s with the use of molecular biology tools to link swine and human type A influenza virus H1N1 to the 1918 influenza pandemic (10). For the last half a century, a plethora of molecular biology techniques have been used to more accurately address epidemiologic investigations by, for example, discerning etiology, understanding risk factors, tracking sources of disease outbreaks, developing markers for diagnostic purposes, and assisting prevention and control approaches to epidemics and pandemics. Present-day molecular epidemiology is moving from the traditional reductionist approach toward omics-driven research, which is mainly spurred by the technological advances using high-throughput laboratory techniques that measure multiple biomarkers from different sources (11–14).
Molecular epidemiology must be recognized as a dynamic and rapidly evolving discipline. The molecular tools commonly applied to molecular epidemiology are usually used with the aim of investigating disease occurrence, disease distribution, or the determinants associated with disease distribution. However, sometimes the term molecular epidemiology has been misused in studies that should be more appropriately described as involving molecular taxonomy or phylogeny, as no epidemiological problem is addressed, despite the invaluable contribution of both phylogeny and taxonomy to the field of molecular epidemiology (15). Box 1 shows the definitions of terminologies pertinent to this review.
Box 1: Definitions of pertinent terminologies
Molecular epidemiology is the field of epidemiology in which information at the molecular level is obtained or explored by molecular tools to improve our capacity to make epidemiological decisions. Therefore, molecular epidemiology can provide additional information to classic epidemiological approaches (descriptive or analytic) that can be applied to a diverse health-related outcomes or events, such as investigating outbreaks, establishing surveillance systems, developing rapid diagnostic methods, detecting host genetic markers for disease susceptibility etc.
Genetic epidemiology: is the field of molecular epidemiology in which molecular biomarkers to assess genetic variations that allow predicting susceptibility and progression of disease. The term is usually applied to the study of non-communicable diseases (NCDs). Genetic epidemiology deployed the use of molecular biomarkers to assess genetic variations that allow predicting progression of various NCDs such as cancer, diabetes and cardiovascular diseases among others. Genetic epidemiology is particularly important in the control of inherited diseases and in the understanding of the multifactorial causes of diseases related to genetic aberrations among individuals or (sub-) populations.
Genomic epidemiology: is one more evolutionary step in progression of molecular epidemiology where genomic information, usually obtained by high-throughput sequencing methods and bioinformatics, such as whole genome sequencing (WGS) or metagenomics, is used to investigate the distribution and spread of infectious agents and to mitigate disease in a population. Although this term has been also used in studies involving host-related genetic background, its more frequently used to address etiologic agents.
The tools that have been commonly used over the last two-to-three decades have primarily focused on methodologies that indirectly show sequence variations of the targeted pathogenic organisms or eukaryotic gene biomarkers. Such methods are primarily based on the profiling of chromosomal or plasmid-based genetic determinants, DNA fragmentation built on targeted restriction digestion, the amplification of targeted gene(s), hybridization using targeted probes, the amplification of repetitive elements, or a combination of these approaches. Some of the most common methods used in molecular epidemiology of infectious diseases include random amplified polymorphic DNA (RAPD), restriction fragment length polymorphism (RFLP), pulsed-field gel electrophoresis (PFGE) (16, 17), amplified fragment length polymorphism (AFLP) (18), and repetitive palindromic PCR (REP-PCR) (19), among many others.
These indirect DNA fingerprinting (or also known as genotyping) approaches come with various advantages as well as limitations. They have great variability in terms of discriminatory power (measured based on Simpson’s index of diversity, i.e., the DI index) (20), repeatability (ability to give consistent results at the same conditions, usually referred to as intra-laboratory reproducibility), reproducibility (ability to give consistent results in different conditions, usually referred to as inter-laboratory reproducibility), typeability (applicability for the a specific organism or targeted genetic element), throughput (ability to test a large number of specimens in a defined period), and other optional criteria such as speed and cost. The relative importance of each criterion depends on the objectives of the epidemiologic scenario (outbreak investigation, surveillance, or research) as well as the availability of laboratory infrastructure, laboratory supplies, and skilled personnel. The incorporation of sequencing information provided by first-generation sequencing platforms (Sanger sequencing) represented an important evolutionary step in molecular epidemiology toward the development of methods such as multilocus sequence typing (MLST) (21) and spa typing, which is based on sequencing of the polymorphic X region of the protein A gene (spa) present in Staphylococcus aureus (22). A summary of the evolution of epidemiology with the application of various genotyping tools and their unique features is shown in Figure 1.
Figure 1 Schematic diagram depicting the evolution of epidemiology as a discipline from the classic descriptive/analytic epidemiology to molecular epidemiology, progressing from traditional indirect genotyping methods to the current and emerging omics applications. Block arrow (gray) shows the progression pattern of the molecular epidemiology approaches.
The development of omics in the last few decades has been of increasing importance, as they allow us to address molecular epidemiologic questions in the most direct and discriminatory ways. These approaches are rapidly expanding across the world with more advanced tools that can rapidly sequence small or large RNA- or DNA-based genomes of bacterial, viral, or parasitic organisms. Consistent with this, the whole-genome sequencing (WGS) of pathogens has become an indispensable tool in epidemiologic investigations of veterinary and livestock infectious diseases. The cost of these genomic tools is also rapidly declining, making them more affordable to use for epidemiologic research, surveillance, and outbreak investigations. However, their implementation has been very limited to developed regions where there is ample laboratory infrastructure and well-trained personnel. The need for capacity building in low- and middle-income countries (LMICs), most of which are located in tropical regions, is of paramount importance.
More recently, approaches using next-generation sequencing (NGS), also known as second-generation sequencing (SGS) or massively parallel sequencing (MPS), have enabled us to obtain high-throughput genomic information capable of determining the order of nucleotides in the whole genome or targeted regions of DNA or RNA (cDNA) with scalability and speed, providing completely new perspectives for genomic approaches. We are now experiencing the expansion of third-generation sequencing technologies, also known as long-read sequencing technologies, which represent very powerful tools for genome assembly, especially by using short- and long-read hybrid assembly strategies. Later in this review, NGS and its utilities for molecular epidemiology in a tropical diseases context is described in greater detail.
In parallel with other high-throughput measurement methods, such as liquid chromatography combined with high-resolution mass spectrometry (LC-MS/MS) and nuclear magnetic resonance (NMR) spectroscopy, we are now able to collectively detect and quantify pools of biological molecules, such as small molecule substrates, intermediates, proteins, and products of cell metabolism, providing unique chemical fingerprints associated with specific cellular processes (23). The possibility of collectively characterizing these biological molecules and analyzing how they translate into structure and biological function, supported by increasing computational biology and bioinformatics, was the milestone for omics (genomics, transcriptomics, proteomics, and metabolomics including lipidomics). These tools enable the identification of biomarkers that are often crucial for the development of diagnostic or therapeutic tools. Biomarker datasets characterizing biological features by means of genomics, transcriptomics, proteomics, lipidomics, and metabolomics are commonly known as omics data, while non-omics data are usually either phenotypic or genotypic data measuring indirect differences in genetic makeup, as described in the earlier section.
The profiling of these biomarker datasets characterizing biological phenotypes at different levels of a biological system using highly discriminatory and high-throughput biotechnologies on DNA sequence data (genomics), RNA expression levels (transcriptomics), protein translation levels (proteomics), lipid synthesis levels (lipidomics), and metabolite levels (metabolomics) is commonly called omics data (24–26). Therefore, omics is the field of biomarker discovery research using sets of advanced tools to discover multiple factors that can be applied to understanding large sets of biological molecules involved in disease or health conditions; it explores the comprehensive set of an organism’s genetic and phenotypic makeup (24).
Advanced technologies including next-generation sequencing, single- or multi-cell RNA assays, high-definition spectrometry tools, and expression assays have enabled the profiling of biological systems at the genomic, transcriptomic, proteomic, lipidomic, and metabolomic levels. In doing so, omics studies have helped to indicate early molecular signatures of low-level exposures, broadening our understanding of healthy and diseased states. At the same time, epidemiological registries gather information such as individual reports of habits and symptoms, the characterization of diseases by pathologists, and clinical electronic health records, commonly referred to as metadata. Consequently, as the application of omics tools in molecular epidemiology has increased in recent years with the improved sensitivity, higher resolution, and greater number of data garnered by omics-based assays, it has been combined with robust metadata to decipher classical epidemiologic questions.
Through these advancements in multiple biomarker discovery techniques, modern population studies have moved from “black-box epidemiology” to “systems epidemiology” (27). Nevertheless, omics-based population studies will still require the basics of epidemiological studies, such as confounding, interaction, selection bias of the population, and measurement error principles, while also exchanging the reductionist view of the traditional approach for a more complex interpretation of different exposures on biological systems using multiple biomarkers (28). Therefore, in the context of understanding the complex effects of environmental factors on biological systems, it is crucial to integrate omics data and non-omics data (metadata) in the same models of association and prediction of health or disease status. This endeavor poses several challenges regarding data generation, capture, curation, sharing, analysis, and visualization, and regarding information privacy and storage (29).
One Health is an integrated, unifying approach that aims to sustainably balance and optimize the health of humans, animals, plants, and ecosystems. It recognizes that the health of humans, domestic and wild animals, plants, and the wider environment (including ecosystems) are closely linked and interdependent. More than two-thirds of emerging and re-emerging diseases in humans are known to be of animal and environmental origin (30). Omics-based research provides in-depth information about the effect of exposure on the metabolome profile (the transcriptome, microbiome, lipidome, etc.) and how these complex changes may allow for the identification of associations between the gene expression profile and confounding environmental factors (31).
Although recent high-resolution and high-throughput omics tools are accelerating and expanding epidemiological population studies, the use of molecular tools to identify biomarkers associated to disease or health conditions started decades ago. A good example of the application of systems-level multi-omics is the Framingham Heart study established in 1948 (32), which revealed the complex dynamics between smoking, lifestyle, and metabolic disorders and susceptibility to coronary heart disease development (33, 34). In addition, genotyping studies have generated an important hypothesis on the environmental effect of benzene exposure on the development of hematological cancer (35, 36). Some of the approaches that have been used in the context of One Health include a microarray-based measurement of approximately 22,000 gene transcripts revealing a set of genes that were differentially expressed and further elucidating the biological pathways that are involved in arsenic-induced skin lesions. (37).
Although important advances have been achieved in terms of the identification of genetic markers associated with NCDs, such as diabetes mellitus (38, 39), cancer (40), and even obesity (39, 41), the vast majority of investigations have originated from developed high-income countries (42). Importantly, there is not only a scarcity of information on the genetic background of populations in LMICs, but also a lack of knowledge of the environmental risks affecting these populations. This is of paramount importance, as gene functionality can be affected by environment and nutrition (43). Moreover, the effects of environmental factors on gene functionality, driven by molecular mechanisms, such as DNA methylation and micro-RNA modifying gene expression, are heritable (44). This is particularly important for public health as an understanding of the implications of exposure factors, especially those associated with embryogenesis and gestation, can be key to preventing NCD in later life and future generations. This is the field of epigenetics (45); the mechanisms of human disease-causing mutations associated with changes in the epigenome or in the abundance and activity of proteins regulating chromatin structure are reported by Zoghbi and Beaudet (46). Epigenetic epidemiology can provide unprecedented insights into the evolutionary aspects of diseases at both the individual and population level. However, the particularities of the genetic background and biotic and abiotic exposure factors in LMICs should be considered when building rigorous causal models in these regions.
The term genomics is often used to refer to a whole-genome-sequencing (WGS) approach to understanding and managing infectious disease outbreaks, and it is inarguably one successful application of omics tools in investigating infectious diseases (47). The application of genomics in the epidemiological framework involves inferring relatedness among organisms by incorporating sets of epidemiological parameters known as metadata together with organism sequence information (48). In such a case, sequencing is usually performed in previously isolated organisms, especially cultured bacteria. For organisms containing shorter nucleic-acid contents, such as viruses, the whole genome can usually be obtained by either amplicon sequencing combinations in second-generation sequencing technologies or applying long-read sequencing third-generation technologies. On the other hand, shotgun metagenome sequencing (the sequencing of the total DNA of a sample without the previous isolation of targeted organisms) allows for the identification of the microbial community present in a sample and the characterization of these microorganisms in terms of the presence of virulence or resistance genes (49). For instance, shotgun metagenomics of blood culture bottles with periprosthetic tissue enabled the prediction of phenotypic antimicrobial resistance and virulence profiles for Staphylococcus aureus (50).
Although (meta)genomics can provide high-resolution information that is usually explored in epidemiological research, the use of genomics in routine clinical microbiology can differ significantly as the time to response is a key factor, whether it is applied to the rapid characterization of infectious agents directly from clinical samples or to the identification of pathogens to combat disease outbreaks (51). Importantly, the structural complexity and genome size of organisms have a profound impact on the practical aspects of genomics field application, including cost and speed. Although small genomes, such as viruses, facilitate the use of tools such as WGS, eukaryotic pathogens such as malaria are distributed in a wider variety of phylogenetic lineages. Their functional complexity relies on evolutionary aspects associated with pathogenicity and host adaptation (52, 53), making comparative genomics, for the identification of vaccine targets, for instance, more complicated, costly, and time-consuming (54–56).
Genomics, as is the case for all other omics fields, was spurred by technological innovation (57). The conventional methods for identifying and characterizing bacterial, viral, and parasitic pathogens from patient or environmental samples were established in the 19th century and are based on presumptive organisms in a system designed to selectively enrich specific pathogens (58). Toward the end of the 20th century, molecular and nucleic acid-based assays were used in various ways for pathogen genotyping and epidemiological traceability. NGS methods introduced a massive parallelization of DNA sequencing, exponentially increased the number of data generated, and at the same time lowered the cost of WGS (59). Hence, NGS has allowed researchers to sequence hundreds of organisms simultaneously (58, 60).
Since 2008, the National Institutes of Health (NIH) have reported a 50-fold drop in the cost per-megabase of DNA sequencing with the introduction of NGS methods. For bacterial genome sequencing, NGS methods cost approximately US $150 to US $250, compared with $94 PFGE, which was the gold standard method for genotyping foodborne bacterial infections on PulseNet (61). Further refinement of technologies has occurred through time, especially with the introduction of third-generation sequencing technologies (59). Third-generation sequencing technologies use single DNA/RNA molecules and offer long- to ultra-long reads, which are ideal for assembling repetitive mobile genetic elements and plasmids (62, 63).
On top of these technological innovations, the development of highly scalable and adaptable sequencing protocols facilitated the widespread use of WGS. A good example is the tiled amplicon sequencing approach developed for WGS of the Ebola virus in 2015 (64), Zika virus (ZIKV) in 2017, and West Nile virus (WNV) (65), and was quickly adapted for SARS-CoV-2 sequencing (the ARTIC protocol), which enabled large-scale sequencing with a considerable cost reduction ($10–50 per genome) (66). As of the compiling of this report, more than 13 million SARS-CoV-2 genomes and more than five million raw un-assembled (FASTQ) sequences have been shared openly through the Global Initiative on Sharing Avian Influenza Data (GISAID) and the National Center for Biotechnology Information (NCBI) databases, mostly using the tied amplicon sequencing protocol. This constitutes the largest single pathogen genome collection in history.
A series of molecular epidemiology methods, including PFGE, MLST, and targeted amplicon-based sequencing have been developed and used to construct phylogenetic relationships (7). NGS and the ability to perform WGS have leapfrogged the iterative improvements of these older genotyping methods (67). Whole-genome sequencing of pathogens provides a single-base-pair-level resolution, making it ideal for investigating outbreaks in a population or hospital setting involving clonal pathogens that conventional molecular epidemiologic tools were unable to discern (67). Notable examples include a S. aureus outbreak investigation in intensive care and neonatal units showing that spa typing captured a minority of the transmission events, in contrast to WGS (68). Similarly, the US Food and Drug Administration (FDA) documented multiple incidents of Salmonella (69, 70), E. coli (71), and Listeria (72) outbreaks where conventional typing tools, such as the PFGE, were unable to attribute a source. In those instances, WGS was used to investigate the relatedness of clinical isolates to food and environmental sources containing identical strains of bacterium (73). A good example of the use of genomics is the mapping of Vibrio cholerae subtypes introduced in Haiti from South Asia that caused more than 9,000 deaths in the 2010 outbreak (74).
Moreover, a contemporary population-level genetic analysis powered by WGS can comprehensively dissect a pathogen’s evolutionary history and provides insights into origins and cross-species transmission events. A recent study that looked at 10,254 S. aureus genomes including 1,896 bovine isolates showed four independent host-jump events in the last 2,500 years (Figure 2). S. aureus human-to-animal transmission facilitated evolutionary adaption in livestock, which was then followed by the dissemination of seven endemic S. aureus clones through the cattle trade, causing bovine mastitis around the world (75). Beyond high-resolution genotyping, WGS is applied as a diagnostics tool in public health and clinical laboratories to guide treatments, for example, to guide appropriate antimicrobial therapy in the case of foodborne pathogens or monitor resistance to antiviral therapy in the case of human immunodeficiency virus (HIV) infections (76). It also constitutes a second-line confirmatory test to clarify test ambiguities, differentiate between species with complex genera, such as the Mycobacterium tuberculosis complex, and verify relapse or reinfection in cases of a second episode of infection (77).
Figure 2 Time-scaled phylogenetic reconstruction of Staphylococcus aureus that originated in humans, with cross-species transmission to bovines 2,500 years ago. Branches are color coded according to the host and arrows point to the main evolutionary jumps into the bovine population. Adopted (with permission) from 75; Proceedings of the National Academy of Sciences (PNAS)].
Whole-genome sequencing and genomic epidemiology have contributed significantly to the response to major infectious disease outbreaks in tropical regions in the last two decades. Real-time monitoring of virus spread using portable sequencers was demonstrated in the 2014 Ebola virus disease (EVD) outbreak that spread through Guinea, Liberia, Sierra Leone, and Nigeria (64). The genomic analysis showed that a West African EBV variant diverged from Central African lineages around 2004 (78) and crossed from Guinea to Sierra Leone around May 2014 to cause sustained human-to-human transmission (79, 80). Furthermore, large-scale sequencing efforts involving the virus from 2013 to 2016 showed that the outbreak involved a single spillover event of the Zaire Ebola virus from an animal reservoir before it was sustained through human-to-human and cross-border transmission events (78). For EVD, direct contact and human-to-human transmission remain the typical pathways. Genomic studies have proven that outbreaks can occur through sexual transmission of the virus from persistently infected individuals. This finding was a basis for the improvement of the WHO guidance on the repeated testing of semen samples before clearing a patient (81, 82).
Another major epidemic of the last decade was the Zika virus (ZIKV) outbreak in the Americas, which emerged in Brazil, where viral whole-genome sequencing data from the vector (mosquitoes) were used to shed light on the number of country-level virus introduction events. Using ZIKV whole-genome phylogenic reconstruction, studies were able to plot the rapid expansion of the ZIKV from Brazil to Puerto Rico, Honduras, Colombia, other Caribbean islands, and the continental United States (83). Modeling involving genomic data and traveler information have provided evidence of unreported hidden outbreaks of ZIKV in Cuba, providing a framework for understanding ZIKV dynamics in the Americas (84).
Most importantly, the past 3 years have been an illustrative example of how genomic data can support conventional surveillance. More than 13 million SARS-CoV-2 sequences, compiled through GISAID, mainly from human sources, were used for real-time monitoring of the pandemic’s spread and to design mitigation strategies. In those countries with periodic sampling, the SARS-CoV-2 genome provided early insight into the emergence of viral mutations and provided a platform from which to monitor SARS-CoV-2 evolution in real time (85, 86). Large-scale genomic comparisons allowed for rapid estimation of the rate of SARS-CoV-2 mutation and evolution in the early stages of the pandemic. The initial calculation showed that the SARS-CoV-2 mutation rate was at least 10 times lower than seasonal influenza. However, with the emergence of variants of concern (VOCs), the virus acquired a higher number of non-synonymous mutations, specifically in the spike gene enabling SARS-CoV-2 to evade natural and vaccine-acquired immunity and establish re-infections in previously exposed individuals (87–90) The genomic evidence, coupled with additional experimental findings, helped to design a bivalent booster dose of the mRNA vaccines to protect patients from severe COVID-19-associated pneumonia and complications (88, 91, 92).
Another use for SARS-CoV-2 genomic data was for spotting sources of novel variants. By sequencing viral genomes in a longitudinal manner from persistently infected individuals, studies elucidated the process of variant emergence, at least in part (93, 94). In addition, the SARS-CoV-2 genomes from non-human hosts were used to track the origins of some mutations associated with animal adaptations and to identify transmission routes to humans (95, 96). Also, genomic surveillance of SARS-CoV-2 originating from environmental and wastewater sequencing provided information on upcoming waves of infection and novel variants before detection in human surveillance (97).
Metagenomics comprises NGS approaches that sequence and analyze multiple genomes of organisms simultaneously. Metagenomics combined with untargeted nucleic acid extraction allows a mixture of multiple genomes that cannot be cultured in the laboratory to be detected, enumerated, and functionally characterized. The key feature characterizing metagenomics as a unique technique is that it can be universally applied to bacterial, viral, and eukaryotic microbes all at once (98). It also circumvents the challenges of viable but non-culturable (VBNC) microbes and opens a transformative avenue for infectious disease investigations (99). Prior to metagenomics, analyzing these microbes required either isolating and amplifying a pure culture of any suspected pathogen or performing whole-genome sequencing in repeated rounds, which was highly costly, laborious, and time consuming. The utilization of nucleic acid for assessing bacterial diversity was developed based on the 16S rRNA marker gene and phylogenetic techniques pioneered in the field of environmental microbiology (100, 101). Other markers that are also specific to prokaryotic taxa, such as cpn60, rpoB, and 23S rRNA have been less frequently used (102, 103). Universal targets for eukaryotic organisms include the 18S rRNA gene or, alternatively, the nuclear ribosomal internal transcribed spacer (ITS) for specifically characterizing fungal populations (104, 105). Importantly, these targeted NGS approaches (amplicon sequencing) aiming at microbiome characterization (metataxonomics) have reduced sequencing costs compared with shot-gun metagenomics, contributing to their widespread use in microbiome studies, particularly in those focusing on gut microbial composition and dysbiosis. Although these methods provide limited information compared with metagenomics, as functional genes are not covered, the reasonable cost–benefit trade-off owing to the need for lower sequencing depth per sample has contributed to their increasing use in LIMCs. Furthermore, functional predictive bioinformatic tools based on taxonomic data are available (106) and can be exploited despite their inherent limitations.
Dysbiosis of the intestinal microbiota is closely associated with human infectious diseases. This is because the intestinal epithelial cells associated with the microbiota form the major host immune barrier. It is estimated that human somatic cells are less numerous than the microbial cells colonizing all the tissues (107). The stability of the human microbiome is influenced by several factors, including human genetics and interactions with the immune system during early development, body site, diet, antibiotic administration, and lifestyle (108). Understanding the human microbiota, how it interacts with the host, and how these microorganisms respond to infectious diseases is of critical importance for the understanding of disease epidemiology and for the development of new clinical interventions in tropical regions. For instance, unique gut microbial species have been identified in COVID-19 patients, such as Streptococcus thermophilus, Bacteroides oleiciplenus, Fusobacterium ulcerans, and Prevotella bivia (49), alongside significant changes in other taxa possibly associated with disease severity. These examples highlight the invaluable power of metagenomics in shedding light on the complex microbial interactions that clearly influence disease outcome.
Infectious diseases of viral origin, particularly RNA viruses, are shown to cause the majority of the recurrent and novel emerging zoonotic, foodborne, waterborne, and vector-borne disease epidemics globally. The timely identification of novel pathogens has a tremendous impact on the subsequent mitigation and response. Unlike bacterial (16S rRNA) and fungal (ITS) agents, viral agents lack unifying marker genes that can be used to characterize the diversity of the virome. As a result, there is a global trend toward the utilization of whole-genome metagenomic sequencing approaches as mainstream methods to identify novel viruses. A recent example is the first identification of SARS-CoV-2 from a single patient admitted to the Central Hospital of Wuhan on 26 December 2019 experiencing severe respiratory syndrome. Metagenomic sequencing of the bronchoalveolar lavage provided the first conclusive evidence of a novel RNA virus from the family Coronaviridae (109). This discovery had a clear impact: within 12 days, species-specific SARS-CoV-2 RT-PCR was developed for the global monitoring of the spread of infection and to support the public health response (110).
Nearly two-thirds of emerging infectious diseases that affect humans are zoonotic, and three-quarters of these originate in wildlife, making surveillance of wildlife for novel pathogens part of a logical strategy to prevent future emergence. The greatest paradigm shift in recent viral surveillance is the application of metagenomics techniques at prioritized One Health interfaces to identify viruses with the risk of novel emergence. Global projects, such as PREDICT and the Global Virome, utilize this approach in wildlife surveillance of potentially zoonotic viruses. Over the span of 10 years, these efforts have characterized 1,000 unique viruses, including zoonotic diseases of public health concern such as Bombali ebolavirus, Zaire ebolavirus, Marburg virus, and MERS- and SARS-like coronaviruses (111, 112). Furthermore, metagenomic approaches can detect co-infections, attribute sources, and screen environmental samples for previously unreported or divergent pathogens (113, 114). Innovative metagenomic protocols such as meta-total RNA sequencing (MeTRS) displayed improved sensitivity and linearity in detecting viral, bacterial, and fungal communities with the greatest reproducibility while requiring lower sequencing depth (Figure 3). As a result, this approach has been increasingly used to investigate the prevalence, diversity, abundance, and co-occurrence of low-abundance pathogens; for instance, this approach was applied to Campylobacter in infants to explain colonization patterns that may have occurred through multiple reservoirs or from a reservoir that several Campylobacter species coinhabit (113). In this study, our team has also shown the use of metagenomics in studying 105 stool samples collected from children infected with multiple viral genera (Figure 4). Although Enterovirus was the most common, several children were shown to be infected with three or four other viruses.
Figure 3 Campylobacter spp. prevalence, diversity, and abundance in children’s stools detected using MeTRS. Blue and red cells represent the absence or presence, respectively, of Campylobacter spp. in stool samples. Adopted from the study by Terefe et al. (113; Frontiers in Public Health). MeTRS is found to be more sensitive than PCR and detects more species of Campylobacter than reported in other studies that utilized the 16S method.
Figure 4 Metagenomic profile of the gastrointestinal tract from 105 children’s stool samples from Ethiopia. Vertical columns represent each child’s sample. The first four columns are negative controls. Enteroviruses were the most common in most children. The reads were filtered to include viruses with a large number of gene segment reads.
Analysis of the pathogen transcriptome is critical for understanding host–pathogen interactions, predicting antimicrobial resistance, predicting virulence, and tracking disease progression in humans and animals. A hybridization-based microarray approach has been used in the past for studying gene expression, but it has many limitations, such as the need to know the sequence of genes to be studied in advance, artifacts due to cross-hybridization, and inaccuracies in quantifying gene transcript levels (115, 116). Other sequencing techniques such as series analysis of gene expression (SAGE) and cap analysis gene expression (CAGE), were found to be better than a microarray approach for the quantification of genes but they are labor intensive, require large quantities of RNA, and are not useful for quantifying spliced isoforms (117). In addition, all these previous methods are expensive and very difficult to implement in tropical developing regions because of a lack of skilled personnel. Next-generation RNA sequencing (RNA-Seq) in now routinely used for studying gene expression in pathogens and the host to predict and identify regulatory pathways involved in disease pathogenesis. RNA-Seq enables a high-throughput quantitative profiling of transcriptional gene expression in an unbiased manner. Several technical approaches are currently available for RNA-Seq, with each approach having its advantages and disadvantages. Bulk RNA-Seq involves the sequencing of total RNA, which contains ribosomal RNA (rRNA), pre-mRNA, and different classes of non-coding RNA (ncRNA). This sequencing approach is useful for measuring global gene expression patterns, alternative splicing, gene fusion, new transcripts, and isoform expression (118). The most commonly used bulk RNA-Seq involves a single-end short sequencing, which is largely focused on mRNA, and involves targeting the poly(A)-tail of mRNA using poly(T) oligonucleotides rather than depleting rRNA. This approach is useful for studying differentially expressed genes (DEGs) between the biological samples. The other approach, which is primarily useful for analyzing point mutations, novel transcripts, and long non-coding RNAs, usually involves the sequencing of rRNA-depleted libraries for better in-depth coverage. Nonetheless, bulk RNA-Seq fails to capture transcriptomes of individual cells within the biological sample and their heterogeneity. Bulk RNA-seq has been used to assess drug resistance (119); the RNA interactome (120); host–parasite interactions (121); and the characterization of the pathogen stages in the vector (122) in zoonotic diseases such as leishmaniasis, which is caused by a protozoan parasite Leishmania.
Recently, the single-cell RNASeq (scRNASeq) technique has become one of the preferred tools for studying transcriptome heterogeneity and discovering rare and uncommon cells. This technique allows for a rapid analysis of gene expression profiles of up to 20,000 individual cells in a single assay, and the identification and characterization of cell populations based on their transcriptomic signatures. scRNA-seq studies using fluorescent bacterial strains have revealed host-cell heterogeneity in vitro (123–125). Furthermore, Pisu et al. (126) used a multimodal scRNASeq approach in Mycobacterium tuberculosis infection that enabled the simultaneous acquisition of the host cell transcriptome and bacterial phenotyping of the individual infected cell. They demonstrated the functional heterogeneity of infected host cells in vivo, which could not be revealed using conventional technology (126). In addition, scRNA-Seq has proved critical for investigating transcriptomic diversity in eukaryote pathogens such as Plasmodium and Leishmania. In malaria, scRNA-Seq analysis of different parasite life stages has revealed transcriptome signatures that are associated with pathogenicity (127), sexual commitment (128), asynchronous development, and sporozoite maturation (129). In addition, single-cell epigenomics and scRNA-Seq can identify the transcriptome changes that regulate the development of host Th1 and Tfh cells in malaria (130, 131). An interactive Malaria Cell Atlas developed following comprehensive functional genomics analysis by scRNA-Seq of various Plasmodium species and parasitized host cells is a major milestone for malaria research (132). Similarly, the scRNA-Seq technique has been effectively employed to identify specific Leishmania parasite populations with a discernible transcriptomic signature that are involved in the formation of parasite hybrids between different Leishmania species in vitro on stress (133). Tissue-specific dual RNA-Seq in the liver and spleen of Leishmania donovani- and Leishmania infantum-infected mice by sequencing both host and parasite transcriptomes by bulk RNA-seq has identified distinct pathways that could be involved in the pathogenesis of these two infections (134). In a recent study using in silico-cell-type deconvolution for transcriptome analysis, a unique transcriptome signature in bone marrow stem cells from L. donovani-infected mice and the blood of VL patients from India, Ethiopia, and Brazil was identified as associated with parasite persistence (135). In addition, scRNA-Seq of the cells isolated from L. major-infected mice identified cellular heterogeneity and transcriptome alterations in the cells at the site of infection (136). Nonetheless, single-cell-based RNA-seq methods have not yet been used for simultaneous analysis of both host and parasite transcripts in vivo. The analysis of host and parasite transcripts at the single-cell level would allow for the identification of novel cell type, which is not possible using conventional methods, such as flow cytometry via cell annotation by transcriptomic analysis. Such an unbiased approach can be widely applied to other infectious diseases for which reference transcriptomes are available.
Since 2005, the number of NGS platforms with different costs, chemistries, capacities, and applications in microbial genomics has increased. With every emerging NGS technology, it is important to evaluate its use in accordance with the laboratory’s research needs and throughput requirements before adoption. Selection of the appropriate sequencing platform requires grounded knowledge on reagent accessibility, cost, and instrument preventive and curative technical support. Several guidance documents have reviewed the infrastructural and operational need for the establishment of genomic- and bioinformatics-associated capacities (137, 138). In addition, major companies in the sequencing space offer NGS solutions varying in size, cost, and throughput according to research and clinical service needs, as in the case of high-throughput sequencing cores. However, as of today, the NGS market share of infectious diseases/microbiological research is below that of human genetic, germline testing, and cancer-related applications, making it difficult to obtain the technical support and interest from companies necessary to invest in innovative means to scale down NGS costs for routine use in infectious disease research. Often, whole-genome bacterial and viral sequencing is performed with benchtop sequencers with a few exceptions for highly repetitive bacterial genomes that may require long reads, although hybrid sequencing usually seems to be the optimal strategy for superior genome assembly in such cases. Most pathogen sequencing is performed with short-read sequencing technology for high raw read accuracy. For applications that need a greater depth of sequencing, such as the characterization of minor variants in a microbial population and metagenomic studies, high-throughput sequencers are often needed. These requirements increase the initial investment from a few thousand to millions of dollars, depending on the sequencing platform. Substantial investments are also needed for ancillary equipment associated with quality control, measurement, and library preparation. The different chemistries available for NGS also have cost implications as, for example, metagenomic sequencing requires unbiased pathogen detection, hence careful assessment and consideration is needed to avoid errors and biased amplification of organisms. This is especially important with microbiologic and clinical research where abundance can be associated with disease severity or progression (139).
In most developing countries, investment in genomics and bioinformatics capabilities was spurred more recently as a result of the COVID-19 pandemic as a reactive measure rather than a proactive investment (140). As a result, the laboratory facilities established have minimal or no computational space or expertise in the area of bioinformatics. An essential part of NGS (including genomics, metagenomics, and transcriptomics) capacity is the availability of infrastructure for data acquisition, analysis, and storage frameworks. Currently, instruments on the market produce sequencing in too large a quantity for most computer CPUs (60). Hence, laboratories in developing countries generate data that is beyond their capacity to store, manage, and analyze. In addition, the lack of harmonization in data streaming has created a choice of multiple (i.e., local, offsite, or cloud-based) platforms for data storage and the preferred approaches vary depending on cost, privacy risk, internet reliability, and ease of accessibility.
Genomic, metagenomic, and transcriptomic data analysis steps remain a challenge because the NGS raw sequence data (FASTQ) require complex bioinformatic steps to assemble fragments. Bioinformatic workflows for read-level processing, mapping, genomic assembly, polishing, variant calls, and clustering using phylogenetic approaches require individual tools that are not yet harmonized. Handling, interpreting, and making use of the massive datasets available, especially through metagenomics, requires a high level of computational skill and an up-to-date understanding of the taxonomic landscape via a well-curated database to provide context for and an interpretation of the result (67).
Another important challenge that has been observed in the context of NGS-generated information relates to data ownership and regulations for data sharing. It is undeniable that technological innovation and infectious disease control are affected by data access. In the context of microbial sequencing data, the sharing of pathogen information can improve surveillance systems and support drug development to control infectious diseases (141). An important example of the benefits of data sharing was observed during the SARS-CoV-2 pandemic, enabling a rapid response to the pandemic at both individual and population levels and contributing to the control of infection not only in high- but also middle- and low-income countries (142). However, complex barriers exist, primarily related to the conflicting interests and motivations of different sectors involved in data sharing, such as prioritizing public health, basic research, economic prosperity, and innovation. Broad discussion and coordination among sectors are urgently needed to establish rules and to ensure that data access is not a limiting factor for infectious disease control and inequality reduction.
Considering these limitations, internet and cloud technologies are providing remarkable opportunities for remote learning and data analysis, directly benefiting laboratories with limited infrastructure and a lack of specialized staff, as commonly seen in LMICs. Applications and pipelines for NGS-data analysis have been discussed elsewhere (67), including tools such as Taxonomer, EDGE, and Pathosphere. These and other low-cost or free bioinformatics platforms are shown in the Supplementary Material (S1).
The metabolome represents the entire low-molecular-weight metabolites of an organism and thus is distinctly different from genomic or transcriptomic sequences. The metabolite profiling of these entities using spectrometry techniques is termed metabolomics. As a relatively newer member of the ‘omics’ family, metabolomics seeks to define the entire complement of metabolites within a cell, tissue, or biofluid (143, 144). Metabolomics provides an analytical approach to reveal the response of a biological system to endogenous or exogenous stimuli, and, consequently, depict its steady-state physiological state (145). As the downstream product of gene transcription, proteome expression, and lipidome composition, the metabolome (including lipidomics) is tightly coupled to other omics fields such as genomics, transcriptomics, and proteomics. It represents an ideal platform for understanding the global systems biology of an organism and its relationship with environmental stimuli (146).
The development of metabolomics over the past two decades has mainly been spurred by the advancement of analytical techniques and bioinformatic tools (147). The most common instruments used in metabolomic analyses include liquid or gas chromatography coupled with high-resolution mass spectrometry (LC-MS/MS, GC-MS/MS) and nuclear magnetic resonance (NMR). The metabolomic approaches used for such purposes can generally be grouped into two classes: metabolite profiling (untargeted) and metabolite fingerprinting (targeted) strategies. The former is a hypothesis-free global investigation of metabolites in a biological system, whereas the latter represents a hypothesis-driven analysis of predefined metabolites (148–150; Castro-Puyana et al., 2013). The main objective of an untargeted metabolite-profiling approach is to establish patterns between different biological samples to potentially indicate significant differences and lead to biomarker identification. On the other hand, metabolic fingerprinting involves analysis of a specified list of metabolites as a result of certain biochemical questions about a particular pathway of an organism. Such targeted analysis characterizes phenotypes and attempts to describe the metabolic signatures or patterns left by environmental factors (151). Between these two approaches to metabolomics, the untargeted profiling of metabolites provides more potential for the discovery of novel metabolic associations of diseases, and has the potential to better characterize exposure and detect early markers of diseases, thus improving diagnostic and therapeutic methods.
The application of metabolomic techniques can provide several complementary inputs to classical molecular epidemiology approaches. The strong computational and technological application of metabolomics provides a strong correlation of metabolite data with metabolic pathways (152, 153). Moreover, the application of sensitive and robust analytical methods such as high-resolution mass spectrometry combined with chromatographic techniques (HPLC-MS/MS) or time-of-flight mass spectrometry (LC-TOF MS) significantly improve the sensitivity and specificity of small-molecule detection, and, consequently, allow the characterization and quantification of complex metabolic profiles in biological samples, which in turn results in the simultaneous measurement of hundreds of metabolites in a single sample (154, 155). In this section, we will review the application of metabolomics within the One Health concept by taking two countries, Ethiopia and Brazil, as model references and highlight the role of this technique in the clarification of issues that are relevant to the One Health topic.
The field of metabolomics has provided researchers with a new generation of tools that detect the small-molecular metabolites of organisms with improved sensitivity, ultimately improving our understanding of pathogen detection and analysis of diversity. Although this powerful analytical method has been extensively applied to molecular epidemiology and related fields, its use in the elucidation of zoonotic infections is still underexplored. This limitation is particularly prominent in the Global South, including sub-Saharan Africa and Latin America. However, there are a few studies reporting the use of metabolomics-based approaches to address zoonotic infections. For example, Lagatie et al. (156) reported on the use of metabolomics to identify potential markers of infection by the nematode Ascaris lumbricoides in populations from Ethiopia, Kenya, Belgium, and Indonesia. In another example of the application of metabolomics in One Health, serologic markers have been identified by NMR-based metabolomics in ruminants affected by caseous lymphadenitis caused by Corynebacterium pseudotuberculosis (157).
Modern metabolomic-based methods encompass the application of advanced analytical instrumentation to study the vector–host–pathogen interplay. Several metabolomic changes have been detected in response to arboviral infections—including dengue, chikungunya, malaria, and Zika virus—in serum, blood, and urine from humans, mice, and monkeys (158). Given that such markers could be identified in biofluids, such as serum and urine, metabolomic-based analysis can be used for non-invasive, population-based screening of various vector-borne diseases (159). Although there are few studies reporting the use of metabolomics in regions affected by neglected tropical and vector-borne diseases, interesting findings related to shifts in the metabolic profiles of serum samples of Zika-virus-infected newborns with or without microcephaly have been reported (160). The use of metabolomics together with other omics approaches (lipidomics, proteomics, and genomics) may provide new insights and aid future studies focused on disease comprehension and therapeutic applications for vector-borne diseases.
Food safety is a major concern for governments and regulatory agencies as a result of the significant increase in demand for animal- and plant-derived food globally in parallel with the rise of food fraud and adulteration. This is a potential field for the application of metabolomics, as there are numerous laws around the world regarding hazardous compounds such as chemical contaminants and toxins and their maximum residue limits (MRLs) (161) Current trends in food science have moved toward a multi-omics examination of metabolites using metabolomic analysis in combination with other omics technologies (e.g., genomics, transcriptomics, proteomics, and lipidomics) to elucidate the fundamental mechanisms of food spoilage and adulteration. In a study using LC-MS/MS, metabolomic methods showed the contamination of Brazil nuts with aflatoxin B1, B2, G1, and G2 (162, 163).
Owing to its robustness and high sensitivity, metabolomics has now been applied to the concept of the “exposome” (i.e., the entirety of human environmental exposures) and to the detection of the molecular imprint of intermediate markers of exposure and diseases, leading to the identification of causative agents (164). A study of Hirmi Valley liver disease (HVLD) in the Tigray region of Ethiopia by Robinson et al. (165) presented a unique application of metabolomics to human liver injury caused by plant hepatotoxins known as pyrrolizidine alkaloids. Using untargeted global 1H NMR analysis, a number of metabolites associated with liver dysfunction have been identified in urine samples of HVLD cases, together with indicators of changes to the gut microbiome in humans in response to this plant hepatotoxin.
Biological systems are continuously exposed to several environmental factors with biochemical consequences, and, subsequently, environmental metabolomics is gaining ground as one of the emerging omics fields. It can be used for examining the metabolic fingerprints of environmental stressors, and, subsequently, for the development of biomarkers of toxicant exposure, metabolic response, susceptibility, and disease diagnosis and monitoring (166, 167). The application of metabolomics in this field will bring an improved understanding of the underlying mechanisms of toxic compounds in the environment. However, little evidence exists of the application of this field in resource-limited regions globally. The use of metabolomics in tropical regions, where environmental stressors are rapidly increasing as a result of urbanization, industrialization, climate changes, and other factors, is encouraged.
NCDs are responsible for 74% of all deaths globally, of which 77% are in low- and middle-income countries (168). Current clinical practices with respect to NCDs mainly focus on a select number of biochemicals that are directly related to pathophysiology and disregard the potential interaction of several other metabolomes of a patient. Clinicians are thus prevented from making the best possible therapeutic interventions as a result of the lack of such multi-omics data that reduce the systems-level approach to diseases. Several molecular epidemiology studies have used metabolomic data to explain the metabolic pathways associated with various pathologies, subsequently developing several biomarkers for disease diagnosis and prognosis in type II diabetes, cardiovascular diseases, hypertension, cancer, and heart failure (Wang et al., 2011; 169–173).
Although no such studies were found in our model countries of Brazil and Ethiopia, a study carried out in Western Africa (i.e., the Gambia) applied metabolomics analysis to the diagnosis of pneumonia, which was shown to be successful in identifying a number of urinary and plasma metabolites with a strong correlation to the incidence and severity of childhood pneumonia infection (174).
At the macro level, similar to other omics approaches, the main challenges of applying metabolomics in tropical developing regions include the lack of skilled personnel and the lack of necessary infrastructure. When these are available, however, there are both technical and statistical challenges intrinsically associated with the processing of metabolomic data. The technical challenges include signal fluctuation, sensitivity reduction, environment alterations, analytical variances (152), which usually arise during sample handling and analytical laboratory preparation. Statistical challenges arise from the lack of proper software applications that can process the multidimensional and highly correlated metabolite variables of datasets. Moreover, there is a lack of standardized protocols concerning the type of specimens, sampling methods, storage conditions, and profiling-related variables (150, 175, 176). This is a critical factor for population-based epidemiological studies using metabolomic data from different sources.
Standardization is urgently required for the epidemiological application of metabolomics; a number of international collaborations are now working toward the development of standardized protocols guiding sample collection, processing, and data acquisition (177). Similarly, international collaborations are under way to address statistical challenges such as missing data and multiple correlated datasets, which for the most part have not yet yielded any gold standard approaches (175, 178–180).
Molecular epidemiology is a crucial discipline that allows us to address critical public health challenges. This is particularly significant considering the rising burden of communicable and non-communicable diseases worldwide, especially those at the interface of human health, animal health, and the environment, which ultimately require the One Health approach. The rise of the omics approach described in this review has brought much more powerful, discriminatory techniques that allow us to discern etiologic agents, track infectious strains, and characterize metabolites at a large scale in complex biological systems. These technological developments have enabled developed regions (mainly in the Global North) to accelerate their capability for the early detection of potential public health emergencies and the development and utilization of biomarkers for the early detection of NCDs such as cancer, among many others. In contrast to such progress, tropical developing regions (mainly in the Global South) have been far behind in terms of building and strengthening skilled personnel and laboratory infrastructure. This disparity must be addressed and narrowed so that global health can be improved equitably and the planet can be made healthy and livable for everyone.
Increasingly, omics tools have become essential for surveillance, research, and outbreak control in epidemic and pandemic settings. However, there is great variation in their implementation across the world. This disparity is not only between countries with a high GDP (commonly referred as the Global North) and a low GDP (commonly referred as the Global South), but also among countries in tropical regions, where most Global South nations are located. We recommend the following:
● A major emphasis on strengthening laboratory infrastructure capacity for omics is important.
● Considering the significant gap in human capacity development, we recommend workforce training at PhD and laboratory technician levels to establish a functional critical mass of skilled workers who can address the needs in omics.
● In order to realize the above two points, it is crucial that developing countries in tropical regions invest significant financial resources in building and strengthening omics capacity.
● To accelerate the implementation of omics, it is also important to establish more robust technology transfer and data exchange systems, encompassing data access, security, and other important areas.
● It should also be noted that omics is much broader than genomics. We recommend the implementation of a more complete package of omics, including metabolomics and transcriptomics, in order to realize effective genotyping and phenotyping profiles.
FT-S: writing—original draft, reviewing, and editing. ZM: writing—original draft, reviewing, and editing. AS: writing—original draft, reviewing, and editing. GFCS: writing—original draft, reviewing, and editing. WG: conceptualization, writing—original draft, reviewing, and editing. CO: conceptualization, writing—original draft, reviewing, and editing. All authors contributed to the article and approved the submitted version.
We thank Conselho Nacional de Desenvolvimento Científico e Tecnológico (CNPq, proc. 3136678/2020-0) for providing a Research Productivity Fellowship (Level 1-D) granted to CJBO; NIH D43: Part of the funding for this work was provided through the NIH Fogarty International Center, GID D43 TW008650; Parts of this work were also funded by the Bill & Melinda Gates Foundation OPP#1175487. Under the grant conditions of the Foundation, a Creative Commons Attribution 4.0 Generic License has already been assigned to the Author Accepted Manuscript version that might arise from this submission.
The author AS declares that they were an editorial board member of Frontiers at the time of submission. This had no impact on the peer review process and the final decision.
The remaining authors declare that the research was conducted in the absence of any commercial or financial relationships that could be construed as a potential conflict of interest.
All claims expressed in this article are solely those of the authors and do not necessarily represent those of their affiliated organizations, or those of the publisher, the editors and the reviewers. Any product that may be evaluated in this article, or claim that may be made by its manufacturer, is not guaranteed or endorsed by the publisher.
The Supplementary Material for this article can be found online at: https://www.frontiersin.org/articles/10.3389/fitd.2023.1151336/full#supplementary-material
1. Jones KE, Patel N, Levy M, Storeygard A, Balk D, Gittleman JL, et al. Global trends in emerging infectious diseases. Nature (2008) 451(7181):990–94. doi: 10.1038/nature06536
2. Allen T, Murray KA, Zambrana-Torrelio C, Morse SS, Rondinini C, Di Marco M, et al. Global hotspots and correlates of emerging zoonotic diseases. Nat Commun (2017) 8(1):1124. doi: 10.1038/s41467-017-00923-8
3. Fuller R, Rahona E, Fisher S, Caravanos J, Webb D, Kass D, et al. Pollution and non-communicable disease: time to end the neglect. Lancet Planet Health (2018) 2(3):e96–8. doi: 10.1016/S2542-5196(18)30020-2
4. Gebreyes WA, Dupouy-Camet J, Newport MJ, Oliveira CJ, Schlesinger LS, Saif YM, et al. The global one health paradigm: challenges and opportunities for tackling infectious diseases at the human, animal, and environment interface in low-resource settings. PloS Negl Trop Dis (2014) 8(11):e3257. doi: 10.1371/journal.pntd.0003257
5. Belay ED, Kile JC, Hall AJ, Barton-Behravesh C, Parsons MB, Salyer S, et al. Zoonotic disease programs for enhancing global health security. Emerg Infect Dis (2017) 23(13):S65–70. doi: 10.3201/eid2313.170544
6. Sharun K, Dhama K, Pawde AM, Gortázar C, Tiwari R, Bonilla-Aldana DK, et al. SARS-CoV-2 in animals: potential for unknown reservoir hosts and public health implications. Vet Q (2021) 41(1):181–201. doi: 10.1080/01652176.2021.1921311
7. Gebreyes WA, Jackwood D, de Oliveira CJB, Lee CW, Hoet AE, Thakur S. Molecular epidemiology of infectious zoonotic and livestock diseases. Microbiol Spectr (2020) 8(2):8–2. doi: 10.1128/microbiolspec.AME-0011-2019
8. Giovanetti M, Slavov SN, Fonseca V, Wilkinson E, Tegally H, Patané JSL, et al. Genomic epidemiology of the SARS-CoV-2 epidemic in Brazil. Nat Microbiol (2022) 7(9):1490–500. doi: 10.1038/s41564-022-01191-z
9. Lopez de Maturana E, Pineda S, Brand A, Van Steen K, Malats N. Toward the integration of omics data in epidemiological studies: still a “long and winding road”. Genet Epidemiol (2016) 40(7):558–69. doi: 10.1002/gepi.21992
10. Kilbourne ED. The molecular epidemiology of influenza. J Infect Dis (1973) 127(4):478–87. doi: 10.1093/infdis/127.4.478
11. Gerszten RE, Wang TJ. The search for new cardiovascular biomarkers. Nature (2008) 451(7181):949–52. doi: 10.1038/nature06802
12. Loscalzo J. Systems biology and personalized medicine: a network approach to human disease. Proc Am Thorac Soc (2011) 8(2):196–8. doi: 10.1513/pats.201006-041MS
13. Haring R, Wallaschofski H. Diving through the “-omics”: the case for deep phenotyping and systems epidemiology. Omics J Integr Biol (2012) 16(5):231–4. doi: 10.1089/omi.2011.0108
14. Arts IC, Weijenberg MP. New training tools for new epidemiologists. Environ Mol Mutagen (2013) 54(7):611–5. doi: 10.1002/em.21793
15. Riley LW. Laboratory Methods in Molecular Epidemiology: Bacterial Infections. Microbiol Spectr (2018) 6(6). doi: 10.1128/microbiolspec.AME-0004-2018
16. Schwartz DC, Cantor CR. Separation of yeast chromosome-sized DNAs by pulsed field gradient gel electrophoresis. Cell (1984) 37(1):67–75. doi: 10.1016/0092-8674(84)90301-5
17. Gebreyes WA, Adkins PR. The use of pulsed-field gel electrophoresis for genotyping of clostridium difficile. In: Pulse field gel electrophoresis. New York, NY: Humana Press (2015). p. 95–101.
18. Vos P, Hogers R, Bleeker M, Reijans M, Lee TVD, Hornes M, et al. AFLP: a new technique for DNA fingerprinting. Nucleic Acids Res (1995) 23(21):4407–14. doi: 10.1093/nar/23.21.4407
19. van der Zee A, Verbakel H, van Zon JC, Frenay I, van Belkum A, Peeters M, et al. Molecular genotyping of Staphylococcus aureus strains: comparison of repetitive element sequence-based PCR with various typing methods and isolation of a novel epidemicity marker. J Clin Microbiol (1999) 37(2):342–9. doi: 10.1128/JCM.37.2.342-349.1999
20. Hunter PR, Gaston MA. Numerical index of the discriminatory ability of typing systems: an application of simpson’s index of diversity. J Clin Microbiol (1988) 26(11):2465–6. doi: 10.1128/jcm.26.11.2465-2466.1988
21. Maiden MC, Bygraves JA, Feil E, Morelli G, Russell JE, Urwin R, et al. Multilocus sequence typing: a portable approach to the identification of clones within populations of pathogenic microorganisms. Proc Natl Acad Sci USA (1998) 95(6):3140–5. doi: 10.1073/pnas.95.6.3140
22. Faria NA, Carrico JA, Oliveira DC, Ramirez M, de Lencastre H. Analysis of typing methods for epidemiological surveillance of both methicillin-resistant and methicillin-susceptible Staphylococcus aureus strains. J Clin Microbiol (2008) 46(1):136–44. doi: 10.1128/JCM.01684-07
23. Daviss B. Growing pains for metabolomics: the newest’omic science is producing results–and more data than researchers know what to do with. Scientist (2005) 19(8):25–9.
24. Vlaanderen J, Moore LE, Smith MT, Lan Q, Zhang L, Skibola CF, et al. Application of OMICS technologies in occupational and environmental health research; current status and projections. Occup Environ Med (2010) 67(2):136–43. doi: 10.1136/oem.2008.042788
25. McHale CM, Zhang L, Reuben T, Smith M. Analysis of the tran-scriptome in molecular epidemiology studies. Environ Mol Mutagenesis (2013) 54:500–17. doi: 10.1002/em.21798
26. Saberi Hosnijeh F, Pechlivanis A, Keun HC, Portengen P, Bueno-de-Mesquita HB, Heederik D, et al. Serum metabolo-mic pertubations among workers exposed to 2,3,7,8-tetrachlorodi-benzo-p-dioxin (TCDD). Environ Mol Mutagenesis (2013) 54:558–65. doi: 10.1002/em.21802
27. Hu FB. Metabolic profiling of diabetes: from black-box epidemiology to systems epidemiology. Clin Chem (2011) 57(9):1224–6. doi: 10.1373/clinchem.2011.167056
28. Rundle A, Ahsan H, Vineis P. Better cancer biomarker discovery through better study design. Eur J Clin Invest (2012) 42(12):1350–9. doi: 10.1111/j.1365-2362.2012.02727.x
29. López de Maturana E, Alonso L, Alarcón P, Martín-Antoniano IA, Pineda S, Piorno L, et al. Challenges in the integration of OMICS and non-omics data. Genes (2019) 10(3):238. doi: 10.3390/genes10030238
30. WHO. Joint tripartite (FAO, OIE, WHO) and UNEP statement. Geneva, Switzerland: One Health High Level Expert Panel (OHHLEP). (2021). Available at: https://www.who.int/news/item/01-12-2021-tripartite-and-unep-support-ohhlep-s-definition-of-one-health.
31. Wild CP, Scalbert A, Herceg Z. Measuring the exposome: a powerful basis for evaluating environmental exposures and cancer risk. Environ Mol Mutagen (2013) 54(7):480–99. doi: 10.1002/em.21777
32. Dawber TR, Meadors GF, Moore FE Jr. Epidemiological approaches to heart disease: the framingham study. Am J Public Health Nations Health (1951) 41(3):279–86. doi: 10.2105/AJPH.41.3.279
33. Christakis NA, Fowler JH. The collective dynamics of smoking in a large social network. N Engl J Med. (2008) 358(21):2249–58. doi: 10.1056/NEJMsa0706154
34. Wang Z, Klipfell E, Bennett BJ, Koeth R, Levison BS, DuGar B, et al. Gut flora metabolism of phosphatidylcholine promotes cardiovascular disease. Nature (2011) 472(7341):57–63. doi: 10.1038/nature09922
35. Shen M, Lan Q, Zhang L, Chanock S, Li G, Vermeulen R, et al. Polymorphisms in genes involved in DNA double-strand break repair pathway and susceptibility to benzene-induced hematotoxicity. Carcinogenesis (2006) 27(10):2083–9. doi: 10.1093/carcin/bgl061
36. Lan Q, Zhang L, Shen M, Jo WJ, Vermeulen R, Li G, et al. Large-Scale evaluation of candidate genes identifies associations between DNA repair and genomic maintenance and development of benzene hematotoxicity. Carcinogenesis (2009) 30(1):50–8. doi: 10.1093/carcin/bgn249
37. Argos M, Kibriya MG, Parvez F, Jasmine F, Rakibuz-Zaman M, Ahsan H, et al. Gene expression profiles in peripheral lymphocytes by arsenic exposure and skin lesion status in a Bangladeshi population. Cancer Epidemiol Biomarkers Prev. (2006) 15(7):1367–75. doi: 10.1158/1055-9965
38. Ali O. Genetics of type 2 diabetes. World J Diabetes (2013) 4(4):114–23. doi: 10.4239/wjd.v4.i4.114
39. Cardona A, Day FR, Perry JRB, Loh M, Chu AY, Lehne B, et al. Epigenome-wide association study of incident type 2 diabetes in a British population: EPIC-Norfolk study. Diabetes (2019) 68(12):2315–26. doi: 10.2337/db18-0290
40. Frank SA. Genetic predisposition to cancer - insights from population genetics. Nat Rev Genet (2004) 5(10):764–72. doi: 10.1038/nrg1450
41. Fawcett KA, Barroso I. The genetics of obesity: FTO leads the way. Trends Genet (2010) 26(6):266–74. doi: 10.1016/j.tig.2010.02.006
42. Muktabhant B, Schelp FP, Kraiklang R, Chupanit P, Sanchaisuriya P. Improved control of non-communicable diseases (NCDs) requires an additional advanced concept for public health - a perspective from a middle-income country. F1000Res (2019) 14(8):286. doi: 10.12688/f1000research.18423.1
43. Fradin D, Bougnères P. T2DM: why epigenetics? J Nutr Metab (2011) 2011:647514. doi: 10.1155/2011/647514
46. Zoghbi HY, Beaudet AL. Epigenetics and human disease. Cold Spring Harb Perspect Biol (2016) 1;8(2):a019497. doi: 10.1101/cshperspect.a019497
47. Tang P, Croxen MA, Hasan MR, Hsiao WW, Hoang LM. Infection control in the new age of genomic epidemiology. Am J Infect Control (2017) 45(2):170–9. doi: 10.1016/j.ajic.2016.05.015
48. Deng X, den Bakker HC, Hendriksen RS. Genomic epidemiology: whole-genome-sequencing-powered surveillance and outbreak investigation of foodborne bacterial pathogens. Annu Rev Food Sci Technol (2016) 7(1):353–74. doi: 10.1146/annurev-food-041715-033259
49. Li S, Yang S, Zhou Y, Disoma C, Dong Z, Du A, et al. Microbiome profiling using shotgun metagenomic sequencing identified unique microorganisms in COVID-19 patients with altered gut microbiota. Front Microbiol (2021) 12:712081. doi: 10.3389/fmicb.2021.712081
50. Sanabria AM, Janice J, Hjerde E, Simonsen GS, Hanssen AM. Shotgun-metagenomics based prediction of antibiotic resistance and virulence determinants in Staphylococcus aureus from periprosthetic tissue on blood culture bottles. Sci Rep (2021) 11(1):1–13. doi: 10.1038/s41598-021-00383-7
51. Motro Y, Moran-Gilad J. Next-generation sequencing applications in clinical bacteriology. Biomol Detect Quantif (2017) 14:1–6. doi: 10.1016/j.bdq.2017.10.002
52. Haldar K, Kamoun S, Hiller NL, Bhattacharje S, van Ooij C. Common infection strategies of pathogenic eukaryotes. Nat Rev Microbiol (2006) 4(12):922–31. doi: 10.1038/nrmicro1549
53. Zhang R, Zhang CT. The impact of comparative genomics on infectious disease research. Microbes infection (2006) 8(6):1613–22. doi: 10.1016/j.micinf.2005.11.019
54. Thompson J, Janse CJ, Waters AP. Comparative genomics in plasmodium: a tool for the identification of genes and functional analysis. Mol Biochem Parasitol (2001) 118(2):147–54. doi: 10.1016/S0166-6851(01)00377-2
55. Carlton JM. Genome sequencing and comparative genomics of tropical disease pathogens. Cell Microbiol (2003) 5(12):861–73. doi: 10.1046/j.1462-5822.2003.00331.x
56. Carlton J, Silva J, Hall N. The genome of model malaria parasites, and comparative genomics. Curr Issues Mol Biol (2005) 7(1):23–38. doi: 10.1046/j.1462-5822.2003.00331.x
57. Relman DA. Microbial genomics and infectious diseases. N Engl J Med (2011) 365(4):347–57. doi: 10.1056/NEJMra1003071
58. Rossen JW, Friedrich AW, Moran-Gilad J. Practical issues in implementing whole-genome-sequencing in routine diagnostic microbiology. Clin Microbiol Infect (2018) 24(4):355–60. doi: 10.1016/j.cmi.2017.11.001
59. Slatko BE, Gardner AF, Ausubel FM. Overview of next-generation sequencing technologies. Curr Protoc Mol Biol (2018) 122(1):e59. doi: 10.1002/cpmb.59
60. Stephens ZD, Lee SY, Faghri F, Campbell RH, Zhai C, Efron MJ, et al. Big data: astronomical or genomical? PloS Biol (2015) 13(7):e1002195. doi: 10.1371/journal.pbio.1002195
61. Gwinn M, MacCannell D, Armstrong GL. Next-generation sequencing of infectious pathogens. Jama (2019) 321(9):893–4. doi: 10.1001/jama.2018.21669
62. Bertrand D, Shaw J, Kalathiyappan M, Ng AHQ, Kumar MS, Li C, et al. Hybrid metagenomic assembly enables high-resolution analysis of resistance determinants and mobile elements in human microbiomes. Nat Biotechnol (2019) 37(8):937–44. doi: 10.1038/s41587-019-0191-2
63. Wang Y, Zhao Y, Bollas A, Wang Y, Au KF. Nanopore sequencing technology, bioinformatics and applications. Nat Biotechnol (2021) 39(11):1348–65. doi: 10.1038/s41587-021-01108-x
64. Quick J, Loman NJ, Duraffour S, Simpson JT, Severi E, Cowley L, et al. Real-time, portable genome sequencing for Ebola surveillance. Nature (2016) 530(7589):228–32. doi: 10.1038/nature16996
65. Quick J, Grubaugh ND, Pullan ST, Claro IM, Smith AD, Gangavarapu K, et al. Multiplex PCR method for MinION and illumina sequencing of zika and other virus genomes directly from clinical samples. Nat Protoc (2017) 12(6):1261–76. doi: 10.1038/nprot.2017.066
66. Tyson JR, James P, Stoddart D, Sparks N, Wickenhagen A, Hall G, et al. Improvements to the ARTIC multiplex PCR method for SARS-CoV-2 genome sequencing using nanopore. Preprint. BioRxiv (2020):2020.09.04.283077. doi: 10.1101/2020.09.04.283077
67. Maljkovic Berry I, Melendrez MC, Bishop-Lilly KA, Rutvisuttinunt W, Pollett S, Talundzic E, et al. Next generation sequencing and bioinformatics methodologies for infectious disease research and public health: approaches, applications, and considerations for development of laboratory capacity. J Infect Dis (2020) 221(Supplement_3):S292–307. doi: 10.1093/infdis/jiz286
68. Price JR, Golubchik T, Cole K, Wilson DJ, Crook DW, Thwaites GE, et al. Whole-genome sequencing shows that patient-to-patient transmission rarely accounts for acquisition of Staphylococcus aureus in an intensive care unit. Clin Infect Dis (2014) 58(5):609–18. doi: 10.1093/cid/cit807
69. Den Bakker HC, Allard MW, Bopp D, Brown EW, Fontana J, Iqbal Z, et al. Rapid whole-genome sequencing for surveillance of Salmonella enterica serovar enteritidis. Emerg Infect Dis (2014) 20(8):1306. doi: 10.3201/eid2008.131399
70. Centers for Disease Control and Prevention (CDC). Multistate outbreak of salmonella typhimurium infections linked to dried coconut (final update). Atlanta, Georgia, USA. (2018).
71. Crowe SJ, Bottichio L, Shade LN, Whitney BM, Corral N, Melius B, et al. Shiga toxin–producing E. coli infections associated with flour. N Engl J Med (2017) 377(21):2036–43. doi: 10.1056/NEJMoa1615910
72. Carstens CK, Salazar JK, Darkoh C. Multistate outbreaks of foodborne illness in the united states associated with fresh produce from 2010 to 2017. Front Microbiol (2019) 10:2667. doi: 10.3389/fmicb.2019.02667
73. Kato-Maeda M, Ho C, Passarelli B, Banaei N, Grinsdale J, Flores L, et al. Use of whole genome sequencing to determine the microevolution of Mycobacterium tuberculosis during an outbreak. PloS One (2013) 8(3):e58235. doi: 10.1371/journal.pone.0058235
74. Chin CS, Sorenson J, Harris JB, Robins WP, Charles RC, Jean-Charles RR, et al. The origin of the Haitian cholera outbreak strain. N Engl J Med (2011) 364(1):33–42. doi: 10.1056/NEJMoa1012928
75. Yebra G, Harling-Lee JD, Lycett S, Aarestrup FM, Larsen G, Cavaco LM, et al. Multiclonal human origin and global expansion of an endemic bacterial pathogen of livestock. Proc Natl Acad Sci USA (2022) 119(50):e2211217119. doi: 10.1073/pnas.2211217119
76. Jacob JJ, Veeraraghavan B, Vasudevan K. Metagenomic next-generation sequencing in clinical microbiology. Indian J Med Microbiol (2019) 37(2):133–40. doi: 10.4103/ijmm.IJMM_19_401
77. Burman WJ, Reves RR. Review of false-positive cultures for Mycobacterium tuberculosis and recommendations for avoiding unnecessary treatment. Clin Infect Dis (2000) 31(6):1390–5. doi: 10.1086/317504
78. Holmes EC, Dudas G, Rambaut A, Andersen KG. The evolution of Ebola virus: insights from the 2013–2016 epidemic. Nature (2016) 538(7624):193–200. doi: 10.1038/nature19790
79. Gire SK, Goba A, Andersen KG, Sealfon RS, Park DJ, Kanneh L, et al. Genomic surveillance elucidates Ebola virus origin and transmission during the 2014 outbreak. Science (2014) 345(6202):1369–72. doi: 10.1126/science.1259657
80. Gardy JL, Loman NJ. Towards a genomics-informed, real-time, global pathogen surveillance system. Nat Rev Genet (2018) 19(1):9–20. doi: 10.1038/nrg.2017.88
81. Mate SE, Kugelman JR, Nyenswah TG, Ladner JT, Wiley MR, Cordier-Lassalle T, et al. Molecular evidence of sexual transmission of Ebola virus. N Engl J Med (2015) 373(25):2448–54. doi: 10.1056/NEJMoa1509773
82. Den Boon S, Marston BJ, Nyenswah TG, Jambai A, Barry M, Keita S, et al. Ebola Virus infection associated with transmission from survivors. Emerg Infect Dis (2019) 25(2):240. doi: 10.3201/eid2502.181011
83. Metsky HC, Matranga CB, Wohl S, Schaffner SF, Freije CA, Winnicki SM, et al. Zika virus evolution and spread in the americas. Nature (2017) 546(7658):411–5. doi: 10.1038/nature22402
84. Grubaugh ND, Saraf S, Gangavarapu K, Watts A, Tan AL, Oidtman RJ, et al. Travel surveillance and genomics uncover a hidden zika outbreak during the waning epidemic. Cell (2019) 178(5):1057–71. doi: 10.1016/j.cell.2019.07.018
85. Chen C, Nadeau S, Yared M, Voinov P, Xie N, Roemer C, et al. CoV-spectrum: analysis of globally shared SARS-CoV-2 data to identify and characterize new variants. Bioinformatics (2022) 38(6):1735–7. doi: 10.1093/bioinformatics/btab856
86. Subissi L, von Gottberg A, Thukral L, Worp N, Oude Munnink BB, Rathore S, et al. An early warning system for emerging SARS-CoV-2 variants. Nat Med (2022) 28(6):1110–5. doi: 10.1038/s41591-022-01836-w
87. Harvey WT, Carabelli AM, Jackson B, Gupta RK, Thomson EC, Harrison EM, et al. SARS-CoV-2 variants, spike mutations and immune escape. Nat Rev Microbiol (2021) 19(7):409–24. doi: 10.1038/s41579-021-00573-0
88. Lucas C, Vogels CB, Yildirim I, Rothman JE, Lu P, Monteiro V, et al. Impact of circulating SARS-CoV-2 variants on mRNA vaccine-induced immunity. Nature (2021) 600(7889):523–9. doi: 10.1038/s41586-021-04085-y
89. Murano K, Guo Y, Siomi H. The emergence of SARS-CoV-2 variants threatens to decrease the efficacy of neutralizing antibodies and vaccines. Biochem Soc Trans (2021) 49(6):2879–90. doi: 10.1042/BST20210859
90. Wibmer CK, Ayres F, Hermanus T, Madzivhandila M, Kgagudi P, Oosthuysen B, et al. SARS-CoV-2 501Y. V2 escapes neutralization by south African COVID-19 donor plasma. Nat Med (2021) 27(4):622–5. doi: 10.1038/s41591-021-01285-x
91. Chalkias S, Harper C, Vrbicky K, Walsh SR, Essink B, Brosz A, et al. A bivalent omicron-containing booster vaccine against covid-19. N Engl J Med (2022) 387(14):1279–91. doi: 10.1056/NEJMoa2208343
92. Sun C, Xie C, Bu GL, Zhong LY, Zeng MS. Molecular characteristics, immune evasion, and impact of SARS-CoV-2 variants. Curr Signal Transduction Ther (2022) 7(1):1–25. doi: 10.1038/s41392-022-01039-2
93. Landis JT, Moorad R, Pluta LJ, Caro-Vegas C, McNamara RP, Eason AB, et al. Intra-Host Evolution Provides for the Continuous Emergence of SARS-CoV-2 Variants. mBio (2023) 14(2):e0344822. doi: 10.1128/mbio.03448-22
94. Chaguza C, Hahn AM, Petrone ME, Zhou S, Ferguson D, Breban MI, et al. Genomic Surveillance Initiative; Schulz W, Swanstrom RI, Roberts SC, Grubaugh ND. Accelerated SARS-CoV-2 intrahost evolution leading to distinct genotypes during chronic infection. Cell Rep Med (2023) 4(2):100943. doi: 10.1016/j.xcrm.2023.100943
95. Hammer AS, Quaade ML, Rasmussen TB, Fonager J, Rasmussen M, Mundbjerg K, et al. SARS-CoV-2 transmission between mink (Neovison vison) and humans, Denmark. Emerg Infect Dis (2021) 27(2):547. doi: 10.3201/eid2702.203794
96. Hoffmann M, Zhang L, Krüger N, Graichen L, Kleine-Weber H, Hofmann-Winkler H, et al. SARS-CoV-2 mutations acquired in mink reduce antibody-mediated neutralization. Cell Rep (2021) 35(3):109017. doi: 10.1016/j.celrep.2021.109017
97. Wu F, Xiao A, Zhang J, Moniz K, Endo N, Armas F, et al. Wastewater surveillance of SARS-CoV-2 across 40 US states from February to June 2020. Water Res (2021) 202:117400. doi: 10.1016/j.watres.2021.117400
98. Sharpton TJ. An introduction to the analysis of shotgun metagenomic data. Front Plant Sci (2014) 5:209. doi: 10.3389/fpls.2014.00209
99. Laudadio I, Fulci V, Stronati L, Carissimi C. Next-generation metagenomics: methodological challenges and opportunities. Omics J Integr Biol (2019) 23(7):327–33. doi: 10.1089/omi.2019.0073
100. Stackebrandt E, Ludwig W, Weizenegger M, Dorn S, McGill TJ, Fox GE, et al. Comparative 16S rRNA oligonucleotide analyses and murein types of round-spore-forming bacilli and non-spore-forming relatives. Microbiology (1987) 133(9):2523–9. doi: 10.1099/00221287-133-9-2523
101. Woese CR. Bacterial evolution. Microbiol Rev (1987) 51(2):221–71. doi: 10.1128/mr.51.2.221-271.1987
102. Case RJ, Boucher Y, Dahllöf I, Holmström C, Doolittle WF, Kjelleberg S. Use of 16S rRNA and rpoB genes as molecular markers for microbial ecology studies. Appl Environ Microbiol (2007) 73(1):278–88. doi: 10.1128/AEM.01177-06
103. Shvartsman E, Richmond ME, Schellenberg JJ, Lamont A, Perciani C, Russell JN, et al. Comparative analysis of DNA extraction and PCR product purification methods for cervicovaginal microbiome analysis using cpn 60 microbial profiling. PloS One (2022) 17(1):e0262355. doi: 10.1371/journal.pone.0262355
104. Huffnagle GB, Noverr MC. The emerging world of the fungal microbiome. Trends Microbiol (2013) 21(7):334–41. doi: 10.1016/j.tim.2013.04.002
105. Kataoka T, Yamaguchi H, Sato M, Watanabe T, Taniuchi Y, Kuwata A, et al. Seasonal and geographical distribution of near-surface small photosynthetic eukaryotes in the western north pacific determined by pyrosequencing of 18S rDNA. FEMS Microbiol Ecol (2017) 93(2):fiw229. doi: 10.1093/femsec/fiw229
106. Douglas GM, Maffei VJ, Zaneveld JR, Yurgel SN, Brown JR, Taylor CM, et al. PICRUSt2 for prediction of metagenome functions. Nat Biotechnol (2020) 38(6):685–8. doi: 10.1038/s41587-020-0548-6
107. Sender R, Fuchs S, Milo R. Are we really vastly outnumbered? revisiting the ratio of bacterial to host cells in humans. Cell (2016) 164(3):337–40. doi: 10.1016/j.cell.2016.01.013
108. Gilbert JA, Blaser MJ, Caporaso JG, Jansson JK, Lynch SV, Knight R. Current understanding of the human microbiome. Nat Med (2018) 24(4):392–400. doi: 10.1038/nm.4517
109. Wu F, Zhao S, Yu B, Chen YM, Wang W, Song ZG, et al. A new coronavirus associated with human respiratory disease in China. Nature (2020) 579(7798):265–9. doi: 10.1038/s41586-020-2008-3
110. Corman V, Bleicker T, Brünink S, Drosten C, Zambon M. Diagnostic detection of 2019-nCoV by real-time RT-PCR. World Health Organ (2020) 17:1–13.
111. Goldstein T, Anthony SJ, Gbakima A, Bird B, Bangura J, Tremeau-Bravard A, et al. The discovery of a new ebolavirus, bombali virus, adds further support for bats as hosts of ebolaviruses. Int J Infect Dis (2019) 79:4–5. doi: 10.1016/j.ijid.2018.11.030
112. Tian J, Sun J, Li D, Wang N, Wang L, Zhang C, et al. Emerging viruses: cross-species transmission of coronaviruses, filoviruses, henipaviruses and rotaviruses from bats. Cell Rep (2022), 110969. doi: 10.1016/j.celrep.2022.110969
113. Terefe Y, Deblais L, Ghanem M, Helmy YA, Mummed B, Chen D, et al. Co-Occurrence of campylobacter species in children from eastern Ethiopia, and their association with environmental enteric dysfunction, diarrhea, and host microbiome. Front Public Health (2020) 8:99. doi: 10.3389/fpubh.2020.00099
114. Havelaar AH, Brhane M, Ahmed IA, Kedir J, Chen D, Deblais L, et al. Unravelling the reservoirs for colonization of infants with campylobacter spp. in rural Ethiopia: protocol for a longitudinal study during a global pandemic and political tensions. BMJ Open (2022) 12(10):061311. doi: 10.1136/bmjopen-2022-061311
115. Casneuf T, Van de Peer Y, Huber W. In situ analysis of cross-hybridisation on microarrays and the inference of expression correlation. BMC Bioinform (2007) 8(1):461. doi: 10.1186/1471-2105-8-461
116. Shendure J. The beginning of the end for microarrays? Nat Methods (2008) 5:585–7. doi: 10.1038/nmeth0708-585
117. Kukurba KR, Montgomery SB. RNA Sequencing and analysis. Cold Spring Harbor Protoc (2015) 2015:951–69. doi: 10.1101/pdb.top084970
118. Hegenbarth J-C, Lezzoche G, De Windt LJ, Stoll M. Perspectives on bulk-tissue RNA sequencing and single-cell RNA sequencing for cardiac transcriptomics. Front Mol Biosci (2022) 2. doi: 10.3389/fmmed.2022.839338
119. Perea-Martínez A, García-Hernández R, Manzano JI, Gamarro F. Transcriptomic analysis in human macrophages infected with therapeutic failure clinical isolates of leishmania infantum. ACS Infect Dis (2022) 8(4):800–10. doi: 10.1021/acsinfecdis.1c00513
120. Kalesh K, Wei W, Mantilla BS, Roumeliotis TI, Choudhary J, Denny PW. Transcriptome-wide identification of coding and noncoding RNA-binding proteins defines the comprehensive RNA interactome of leishmania mexicana. Microbiol Spectr (2022) 10(1):e02422–21. doi: 10.1128/spectrum.02422-21
121. Dillon LA, Suresh R, Okrah K, Corrada Bravo H, Mosser DM, El-Sayed NM. Simultaneous transcriptional profiling of leishmania major and its murine macrophage host cell reveals insights into host-pathogen interactions. BMC Genomics (2015) 16(1):1–15. doi: 10.1186/s12864-015-2237-2
122. Inbar E, Hughitt VK, Dillon LA, Ghosh K, El-Sayed NM, Sacks DL. The transcriptome of leishmania major developmental stages in their natural sand fly vector. MBio (2017) 8(2):e00029–17. doi: 10.1128/mBio.00029-17
123. Avraham R, Haseley N, Brown D, Penaranda C, Jijon HB, Trombetta JJ, et al. Pathogen cell-to-cell variability drives heterogeneity in host immune responses. Cell (2015) 162(6):1309–21. doi: 10.1016/j.cell.2015.08.027
124. Saliba A-E, Li L, Westermann AJ, Appenzeller S, Stapels DAC, Schulte LN, et al. Single-cell RNA-seq ties macrophage polarization to growth rate of intracellular Salmonella. Nat Microbiol (2016) 2:16206. doi: 10.1038/nmicrobiol.2016.206
125. Avital G, Avraham R, Fan A, Hashimshony T, Hung DT, Yanai I. scDual-seq: mapping the gene regulatory program of Salmonella infection by host and pathogen single-cell RNA-sequencing. Genome Biol (2017) 18(1):1–8. doi: 10.1186/s13059-017-1340-x
126. Pisu D, Huang L, Narang V, Theriault M, Lê-Bury G, Lee B, et al. Single cell analysis of M. tuberculosis phenotype and macrophage lineages in the infected lung. J Exp Med (2021) 218(9):e20210615. doi: 10.1084/jem.20210615
127. Reid AJ, Talman AM, Bennett HM, Gomes AR, Sanders MJ, Illingworth CJR, et al. Single-cell RNA-seq reveals hidden transcriptional variation in malaria parasites. Elife (2018) 27(7):e33105. doi: 10.7554/eLife.33105
128. Poran A, Nötzel C, Aly O, Mencia-Trinchant N, Harris CT, Guzman ML, et al. Single-cell RNA sequencing reveals a signature of sexual commitment in malaria parasites. Nature (2017) 551(7678):95–9. doi: 10.1038/nature24280
129. Bogale HN, Pascini TV, Kanatani S, Sá JM, Wellems TE, Sinnis P, et al. Transcriptional heterogeneity and tightly regulated changes in gene expression during plasmodium berghei sporozoite development. Proc Natl Acad Sci U S A (2021) 118(10):e2023438118. doi: 10.1073/pnas.2023438118
130. Lönnberg T, Svensson V, James KR, Fernandez-Ruiz D, Sebina I, Montandon R, et al. Single-cell RNA-seq and computational analysis using temporal mixture modeling resolves TH1/TFH fate bifurcation in malaria. Sci Immunol (2017) 2(9):eaal2192. doi: 10.1126/sciimmunol.aal2192
131. Soon MS, Lee HJ, Engel JA, Straube J, Thomas BS, Pernold CP, et al. Transcriptome dynamics of CD4+ T cells during malaria maps gradual transit from effector to memory. Nat Immunol (2020) 21(12):1597–610. doi: 10.1038/s41590-020-0800-8
132. Howick VM, Russell AJ, Andrews T, Heaton H, Reid AJ, Natarajan K, et al. The malaria cell atlas: single parasite transcriptomes across the complete plasmodium life cycle. Science (2019) 365(6455):eaaw2619. doi: 10.1126/science.aaw2619
133. Louradour I, Ferreira TR, Duge E, Karunaweera N, Paun A, Sacks D. Stress conditions promote leishmania hybridization in vitro marked by expression of the ancestral gamete fusogen HAP2 as revealed by single-cell RNA-seq. Elife (2022) 11:e73488. doi: 10.7554/eLife.73488
134. Forrester S, Goundry A, Dias BT, Leal-Calvo T, Moraes MO, Kaye PM, et al. Tissue specific dual RNA-seq defines host–parasite interplay in murine visceral leishmaniasis caused by leishmania donovani and leishmania infantum. Microbiol Spectr (2022) 10(2):e00679–22. doi: 10.1128/spectrum.00679-22
135. Dirkx L, Hendrickx S, Merlot M, Bulté D, Starick M, Elst J, et al. Long-term hematopoietic stem cells as a parasite niche during treatment failure in visceral leishmaniasis. Commun Biol (2022) 5(1):626. doi: 10.1038/s42003-022-03591-7
136. Venugopal G, Bird JT, Washam CL, Roys H, Bowlin A, Byrum SD, et al. In vivo transcriptional analysis of mice infected with leishmania major unveils cellular heterogeneity and altered transcriptomic profiling at single-cell resolution. PloS Negl Trop Dis (2022) 16(7):e0010518. doi: 10.1371/journal.pntd.0010518
137. WHO. The use of next-generation sequencing technologies for the detection of mutations associated with drug resistance in mycobacterium tuberculosis complex: technical guide (No. WHO/CDS/TB/2018.19). Geneva, Switzerland: World Health Organization (2018).
138. Davedow T, Carleton H, Kubota K, Palm D, Schroeder M, Gerner-Smidt P, et al. PulseNet international survey on the implementation of whole genome sequencing in low and middle-income countries for foodborne disease surveillance. Foodborne Pathog Dis (2022) 19(5):332–40. doi: 10.1089/fpd.2021.0110
139. Graf EH, Simmon KE, Tardif KD, Hymas W, Flygare S, Eilbeck K, et al. Unbiased detection of respiratory viruses by use of RNA sequencing-based metagenomics: a systematic comparison to a commercial PCR panel. J Clin Microbiol (2016) 54(4):1000–7. doi: 10.1128/JCM.03060-15
140. Inzaule SC, Tessema SK, Kebede Y, Ouma AEO, Nkengasong JN. Genomic-informed pathogen surveillance in Africa: opportunities and challenges. Lancet Infect Dis (2021) 21(9):e281–9. doi: 10.1016/S1473-3099(20)30939-7
141. Ribeiro CDS, van Roode MY, Haringhuizen GB, Koopmans MP, Claassen E, van de Burgwal LH. How ownership rights over microorganisms affect infectious disease control and innovation: a root-cause analysis of barriers to data sharing as experienced by key stakeholders. PloS One (2018) 13(5):e0195885. doi: 10.1371/journal.pone.0195885
142. Rios RS, Zheng KI, Zheng MH. Data sharing during COVID-19 pandemic: what to take away. Expert Rev Gastroenterol Hepatol (2020) 14(12):1125–30. doi: 10.1080/17474124.2020.1815533
143. Harrigan GG, Goodacre R. Metabolic profiling: its role in biomarker discovery and gene function analysis: its role in biomarker discovery and gene function analysis. Springer Science & Business Media (2003).
144. Griffiths-Jones S, Saini HK, Van Dongen S, Enright AJ. miRBase: tools for microRNA genomics. Nucleic Acids Res (2007) 36(suppl_1):D154–8. doi: 10.1093/nar/gkm952
145. Nicholson JK, Lindon JC, Holmes E. ‘Metabonomics’: understanding the metabolic responses of living systems to pathophysiological stimuli via multivariate statistical analysis of biological NMR spectroscopic data. Xenobiotica (1999) 29(11):1181–9. doi: 10.1080/004982599238047
146. Fiehn O. Metabolomics–the link between genotypes and phenotypes. Funct Genomics (2002), 155–71. doi: 10.1007/978-94-010-0448-0_11
147. Kirpich A, Ainsworth EA, Wedow JM, Newman JR, Michailidis G, McIntyre LM. Variable selection in omics data: a practical evaluation of small sample sizes. PloS One (2018) 13(6):e0197910. doi: 10.1371/journal.pone.0197910
148. Liesenfeld DB, Habermann N, Owen RW, Scalbert A, Ulrich CM. Review of mass spectrometry–based metabolomics in cancer research metabolomics in cancer research. Cancer Epidemiol Biomarkers Prev (2013) 22(12):2182–201. doi: 10.1158/1055-9965.EPI-13-0584
149. Su Y, Wang J, Shi M, Niu X, Yu X, Gao L, et al. Metabolomic and network analysis of astaxanthin-producing haematococcus pluvialis under various stress conditions. Bioresour Technol (2014) 170:522–9. doi: 10.1016/j.biortech.2014.08.018
150. Tzoulaki I, Ebbels TM, Valdes A, Elliott P, Ioannidis JP. Design and analysis of metabolomics studies in epidemiologic research: a primer on-omic technologies. Am J Epidemiol (2014) 180(2):129–39. doi: 10.1093/aje/kwu143
151. Liang Q, Wang C, Li B, Zhang AH. Metabolic fingerprinting to understand therapeutic effects and mechanisms of silybin on acute liver damage in rat. Pharmacogn Mag (2015) 11(43):586. doi: 10.4103/0973-1296.160469
152. Dunn WB, Broadhurst DI, Atherton HJ, Goodacre R, Griffin JL. Systems level studies of mammalian metabolomes: the roles of mass spectrometry and nuclear magnetic resonance spectroscopy. Chem Soc Rev (2011) 40(1):387–426. doi: 10.1039/B906712B
153. Wishart DS. Advances in metabolite identification. Bioanalysis (2011) 3(15):1769–82. doi: 10.4155/bio.11.155
154. Psychogios N, Hau DD, Peng J, Guo AC, Mandal R, Bouatra S, et al. The human serum metabolome. PloS One (2011) 6(2):e16957. doi: 10.1371/journal.pone.0016957
155. Bouatra S, Aziat F, Mandal R, Guo AC, Wilson MR, Knox C, et al. The human urine metabolome. PloS One (2013) 8(9):e73076. doi: 10.1371/journal.pone.0073076
156. Lagatie O, Verheyen A, Van Asten S, Odiere MR, Djuardi Y, Levecke B, et al. 2-methyl-pentanoyl-carnitine (2-MPC): a urine biomarker for patent ascaris lumbricoides infection. Sci Rep (2020) 10(1):1–13. doi: 10.1038/s41598-020-72804-y
157. De Moraes Pontes JG, De Santana FB, Portela RW, Azevedo V, Poppi RJ. Biomarkers of the caseous lymphadenitis in sheep by NMR-based metabolomics. Metabolomics (Los Angel) (2017) 7(190):2153–0769. doi: 10.4172/2153-0769.1000190
158. Byers NM, Fleshman AC, Perera R, Molins CR. Metabolomic insights into human arboviral infections: dengue, chikungunya, and zika viruses. Viruses (2019) 11(3):225. doi: 10.3390/v11030225
159. Abdelrazig S, Ortori CA, Davey G, Deressa W, Mulleta D, Barrett DA, et al. A metabolomic analytical approach permits identification of urinary biomarkers for plasmodium falciparum infection: a case–control study. Malar J (2017) 16(1):1–8. doi: 10.1186/s12936-017-1875-z
160. Nunes EDC, Filippis AMD, Pereira TDE, Faria NRDC, Salgado Á, Santos CS, et al. Untargeted metabolomics insights into newborns with congenital zika infection. Pathogens (2021) 10(4):468. doi: 10.3390/pathogens10040468
161. Medina S, Pereira JA, Silva P, Perestrelo R, Câmara JS. Food fingerprints–a valuable tool to monitor food authenticity and safety. Food Chem (2019) 278:144–62. doi: 10.1016/j.foodchem.2018.11.046
162. Xavier JJM, Scussel VM. Development of an LC-MS/MS method for the determination of aflatoxins B1, B2, G1, and G2 in Brazil nut. Int J Environ Anal Chem (2008) 88(6):425–33. doi: 10.1080/03067310701836816
163. Kang MJ, Suh JH. Metabolomics as a tool to evaluate nut quality and safety. Trends Food Sci Technol (2022). doi: 10.1016/j.tifs.2022.11.002
164. Ellis JK, Athersuch TJ, Thomas LD, Teichert F, Pérez-Trujillo M, Svendsen C, et al. Metabolic profiling detects early effects of environmental and lifestyle exposure to cadmium in a human population. BMC Med (2012) 10(1):1–10. doi: 10.1186/1741-7015-10-61
165. Robinson O, Toledano MB, Sands C, Beckonert O, J. Want E, Goldin R, et al. Global metabolic changes induced by plant-derived pyrrolizidine alkaloids following a human poisoning outbreak and in a mouse model. Toxicol Res (2016) 5(6):1594–603. doi: 10.1039/C6TX00221H
166. Lin CY, Viant MR, Tjeerdema RS. Metabolomics: methodologies and applications in the environmental sciences. J Pestic Sci (2006) 31(3):245–51. doi: 10.1584/jpestics.31.245
167. Bundy JG, Davey MP, Viant MR. Environmental metabolomics: a critical review and future perspectives. Metabolomics (2009) 5(1):3–21c. doi: 10.1007/s11306-008-0152-0
168. Saha A, Alleyne G. Recognizing noncommunicable diseases as a global health security threat. Bull World Health Organ (2018) 96(11):792–3. doi: 10.2471/blt.17.205732
169. Holmes E, Loo RL, Stamler J, Bictash M, Yap IK, Chan Q, et al. Human metabolic phenotype diversity and its association with diet and blood pressure. Nature (2008) 453(7193):396–400. doi: 10.1038/nature06882
170. Wang-Sattler R, Yu Z, Herder C, Messias AC, Floegel A, He Y, et al. Novel biomarkers for pre-diabetes identified by metabolomics. Mol Syst Biol (2012) 8(1):615. doi: 10.1038/msb.2012.43
171. Zheng Y, Yu B, Alexander D, Manolio TA, Aguilar D, Coresh J, et al. Associations between metabolomic compounds and incident heart failure among African americans: the ARIC study. Am J Epidemiol (2013) 178(4):534–42. doi: 10.1093/aje/kwt004
172. Würtz P, Havulinna AS, Soininen P, Tynkkynen T, Prieto-Merino D, Tillin T, et al. Metabolite profiling and cardiovascular event risk: a prospective study of 3 population-based cohorts. Circulation (2015) 131(9):774–85. doi: 10.1161/CIRCULATIONAHA.114.013116
173. Huang J, Mondul AM, Weinstein SJ, Koutros S, Derkach A, Karoly E, et al. Serum metabolomic profiling of prostate cancer risk in the prostate, lung, colorectal, and ovarian cancer screening trial. Br J Cancer (2016) 115(9):1087–95. doi: 10.1038/bjc.2016.305
174. Laiakis EC, Morris GA, Fornace AJ Jr., Howie SR. Metabolomic analysis in severe childhood pneumonia in the Gambia, West Africa: findings from a pilot study. PloS One (2010) 5(9):12655. doi: 10.1371/journal.pone.0012655
175. Saccenti E, Hoefsloot HC, Smilde AK, Westerhuis JA, Hendriks MM. Reflections on univariate and multivariate analysis of metabolomics data. Metabolomics (2014) 10(3):361–74. doi: 10.1007/s11306-013-0598-6
176. Kirwan JA, Brennan L, Broadhurst D, Fiehn O, Cascante M, Dunn WB, et al. Preanalytical processing and biobanking procedures of biological samples for metabolomics research: a white paper, community perspective (for “Precision medicine and pharmacometabolomics task group”–the metabolomics society initiative). Clin Chem (2018) 64(8):1158–82. doi: 10.1373/clinchem.2018.287045
177. Dudzik D, Barbas-Bernardos C, García A, Barbas C. Quality assurance procedures for mass spectrometry untargeted metabolomics. a review. J Pharm Biomed Anal (2018) 147:149–73. doi: 10.1016/j.jpba.2017.07.044
178. Hrydziuszko O, Viant MR. Missing values in mass spectrometry-based metabolomics: an undervalued step in the data processing pipeline. Metabolomics (2012) 8(1):161–74. doi: 10.1007/s11306-011-0366-4
179. Ren S, Hinzman AA, Kang EL, Szczesniak RD, Lu LJ. Computational and statistical analysis of metabolomics data. Metabolomics (2015) 11(6):1492–513. doi: 10.1007/s11306-015-0823-6
Keywords: communicable diseases, non-communicable diseases, omics, metabolomics, genomics, transcriptomics, lipidomics
Citation: Tigistu-Sahle F, Mekuria ZH, Satoskar AR, Sales GFC, Gebreyes WA and Oliveira CJB (2023) Challenges and opportunities of molecular epidemiology: using omics to address complex One Health issues in tropical settings. Front. Trop. Dis 4:1151336. doi: 10.3389/fitd.2023.1151336
Received: 27 January 2023; Accepted: 22 May 2023;
Published: 28 July 2023.
Edited by:
Marcelo A. Soares, National Cancer Institute (INCA), BrazilReviewed by:
Matthew M. Hernandez, Icahn School of Medicine at Mount Sinai, United StatesCopyright © 2023 Tigistu-Sahle, Mekuria, Satoskar, Sales, Gebreyes and Oliveira. This is an open-access article distributed under the terms of the Creative Commons Attribution License (CC BY). The use, distribution or reproduction in other forums is permitted, provided the original author(s) and the copyright owner(s) are credited and that the original publication in this journal is cited, in accordance with accepted academic practice. No use, distribution or reproduction is permitted which does not comply with these terms.
*Correspondence: Feven Tigistu-Sahle, c2FobGUuMkBvc3UuZWR1
Disclaimer: All claims expressed in this article are solely those of the authors and do not necessarily represent those of their affiliated organizations, or those of the publisher, the editors and the reviewers. Any product that may be evaluated in this article or claim that may be made by its manufacturer is not guaranteed or endorsed by the publisher.
Research integrity at Frontiers
Learn more about the work of our research integrity team to safeguard the quality of each article we publish.