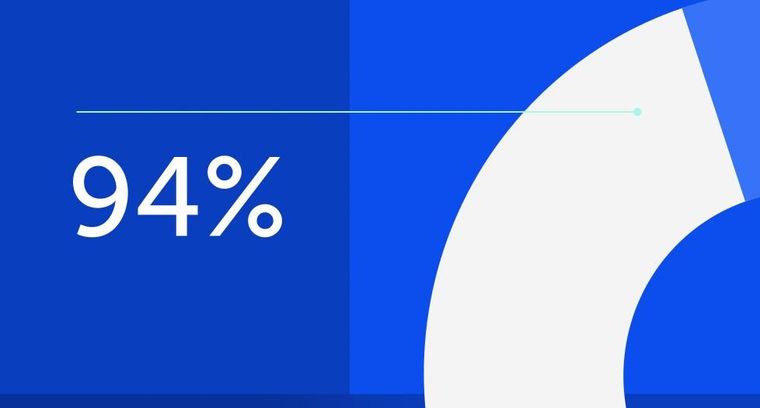
94% of researchers rate our articles as excellent or good
Learn more about the work of our research integrity team to safeguard the quality of each article we publish.
Find out more
ORIGINAL RESEARCH article
Front. Trop. Dis., 20 March 2023
Sec. Emerging Tropical Diseases
Volume 4 - 2023 | https://doi.org/10.3389/fitd.2023.1118774
Background: Dengue is the most widely distributed arboviral disease in tropical and subtropical countries. Most suspected cases are diagnosed according to the clinical criteria, and early diagnosis is difficult. Moreover, in underdeveloped countries, several factors continue to challenge the diagnosis and surveillance of dengue cases. This study aimed to design a diagnostic algorithm using rapid diagnostic tests (RDTs), ELISA tests, and clinical and hematological variables to confirm dengue cases in febrile patients in Colombia.
Methods: Altogether, 505 samples were collected. Serum samples were evaluated by RDTs (IgM and IgG antibodies and NS1 antigen), capture IgM and IgG ELISAs, and endpoint hemi-nested RT-PCR assay (qualitative). We statistically analyzed the performance of individual tests to determine the most useful ones to confirm dengue cases accurately.
Results: Individual results for IgM, IgG, and NS1 RDTs yielded lower sensitivity and specificity values than the reference standard. High sensitivity and specificity were obtained after combining IgM and NS1 ELISA results (96.3% and 96.4%) and NS1 RDT plus IgM ELISA results (90.3% and 96.2%), respectively. Adjusted odds ratios (aORs) were calculated for clinical variables and laboratory tests to differentiate dengue from other febrile illnesses (OFI). This approach showed that myalgia, abdominal tenderness, and platelet count were identified with higher sensitivity to confirm dengue cases. IgM RDT and NS1 RDT differentiated dengue cases from OFI. A positive IgM RDT or a positive NS1 RDT combined with specific signs or symptoms confirmed 81.6% of dengue cases. A combination of clinical findings and a positive NS1 RDT or positive ELISA IgM confirmed 90.6% of the cases.
Conclusion: Our findings showed that clinical diagnoses in pediatric population alone cannot confirm true dengue cases and needs to be complemented by laboratory diagnostic tests. We also demonstrate the usefulness of combining clinical criteria with RDTs, suggesting that their implementation with the IgM ELISA test improves dengue case confirmation.
Dengue is considered the most predominant human arbovirus in tropical and subtropical countries. It is caused by one of the four different dengue virus (DENV) serotypes (DENV-1 to DENV-4). It is transmitted through the bites of infected Aedes mosquitoes circulating in Asian and Latin American countries (1–5). In the initial stage of dengue infection, patients show many non-specific signs and symptoms (6–10). However, as the disease progresses, it can follow variable clinical courses, ranging from mild fever to severe symptoms, which may result in fatal outcomes (11–14).
The early diagnosis of dengue infection is difficult; most infections are unidentified or present a delayed diagnosis, leading to complications (1, 15, 16). In the last decade, dengue diagnosis has been made according to the World Health Organization (WHO) definition proposed in 2009 (17). In addition, healthcare centers and international organizations have been involved in studies to establish appropriate diagnostic protocols that integrate clinical criteria and laboratory tests (18–20). Several investigations have been conducted to design diagnostic algorithms to improve case confirmation accuracy and strengthen disease management (21–26). However, in underdeveloped countries, the inequality in access to health services, the inability to perform diagnostic tests within the same day of sample collection (due to limited equipment or trained personnel), and high costs associated with testing, remain some of the drawbacks in the surveillance system (4, 27, 28).
Colombia has a high number of dengue cases and has the second-highest prevalence rate in the Americas (29–31) after Brazil (32–34). This is due to the Aedes aegypti infestation in more than 70% of municipalities located in areas below 1,800 m above sea level (MASL) and some above 2,200 MASL (29, 35, 36). The steady circulation of all four dengue serotypes (31, 37) has led to dramatic changes in dengue endemicity, with dengue cases being reported in municipalities with no prior cases, a reduction in the mean age of patients (29, 38), and an increasing number of cases with severe dengue and fatalities (39, 40). Unfortunately, most of the reported dengue cases in Colombia are diagnosed based on clinical criteria; a third of the patients were confirmed by immunoglobulin M (IgM) enzyme-linked immunosorbent assay (ELISA) tests or reverse transcription-polymerase chain reaction (RT-PCR) (34.7%). Only severe cases or fatal outcomes are investigated by non-structural protein-1 (NS1) ELISA, RT-PCR, or viral isolation (41, 42). For these reasons, some healthcare centers perform rapid diagnostic tests (RDTs) for IgM/IgG or NS1 to improve the clinical diagnosis (43–45). Despite the widespread use of RDTs in Colombia, some reports have evaluated the performance of RDTs and suggest that a negative result in these tests does not entirely rule out dengue; therefore, clinicians should be aware of the diagnosis and confirm cases by specialized diagnostic tests (44–46).
Due to the shortcomings in implementing diagnostic tests in underdeveloped countries, recent studies in Asia and the Americas have focused on evaluating clinic variables and assigning scores depending on the frequency to establish the diagnosis (12, 21–23, 47). Although this strategy has been helpful in improving patient care, the validation of these algorithms is a complex process due to the heterogeneity of the study population and the variability in clinical findings according to each stage of the disease (48–55). Other studies have combined clinical variables with hematological or biochemical biomarkers to confirm cases, such as those developed in Colombia (56) and Vietnam (24, 57), and Brazil (48), to differentiate dengue cases from other febrile illnesses.
Most reported studies have evaluated the performance of serology and virology tests while integrating other tests as the standard reference. These studies show high sensitivity, specificity, and predictive values variability due to factors such as population, DENV serotypes, time of sample collection, and tests considered as the reference standards. However, these studies shed light on the usefulness of these approaches for case confirmation and establish their potential as effective predictors of disease severity (34, 58–67).
Colombia, as an endemic country, requires new strategies to strengthen the clinical diagnosis and laboratory confirmation of dengue cases and evaluate the sensitivity and specificity of tests used in every healthcare center independently of their complexity. These strategies may improve the early detection of cases, promote efficient control of outbreaks, and enhance the dengue surveillance system. Therefore, this study aimed to design a diagnostic algorithm using RDT and clinical and hematological variables to identify and confirm dengue cases in febrile patients from an endemic area in Colombia.
This study was revised and approved by the Ethics Committee of Hospital Universitario de La Samaritana, Bogotá (Resolution 7 and 9 of 2013 and 2014, respectively). Patients who consulted for a primary diagnosis of febrile syndrome in the Hospital de Girardot between March 2014 and July 2015 were enrolled. The Girardot municipality, located 134 km from Bogotá, has 150,000 inhabitants, and is situated at an altitude of 289 MASL with a mean temperature of 33°C and 66.4% relative humidity. Girardot reported a dengue incidence of 572.5 cases [95% confidence interval [CI] (525.3 – 619.9)] and 98,000 individuals at risk in 2010 (68) and a prevalence of dengue IgG specific antibodies higher than 90% (69).
Patients were invited to participate and required to provide written informed consent (consenting guardian for patients who were underage) for participation. Following the disease description by the WHO in 2009 (17), the inclusion criteria were patients with fever, malaise, headache, retro-orbital pain, myalgias, exanthema, abdominal pain, and arthralgia. Age and day of illness were not considered as inclusion criteria. Patients with an apparent infectious focus were excluded (e.g., otitis, tonsillitis, or urinary infection). The sample size was calculated based on acceptable precision for estimates of sensitivity and specificity values and the estimated prevalence of disease in an endemic area. Considering 20% loss of information in the regression models due to population characteristics and insufficient volume sample to evaluate the complete panel of diagnostic tests-due to the characteristics of the study population-, the final sample size evaluated was 505 patients.
After a thorough medical examination and completion of the clinical report form, blood samples were taken to immediately analyze hematological variables (leukocytes, erythrocytes, platelet counts, hemoglobin, and hematocrit). The sera were processed for evaluation by RDT for IgM/IgG antibodies [Dengue duo cassette (01PF10), Panbio, Alere] and NS1 RDT [Dengue early rapid (01PF20), Panbio Alere]. The remaining serum was aliquoted, frozen, and sent to the laboratory (Universidad El Bosque, Bogotá) to be stored at -80°C until further use. All samples were processed for RNA extraction (QIAmp Viral Mini Kit, Qiagen) following the manufacturer’s instructions. The isolated RNA was retrotranscribed and amplified using primers described previously (70, 71) and PCR-modified protocols (72). For the first round, SuperScript III Platinum One-step RT-PCR (Invitrogen), mD1 and D2 primers were used. The amplified product was analyzed for DENV serotypes in a multiplex format using specific primers (mD1, TS1, mTS2, TS3, and rTS4). The specificity of the assay is based on the ability of the serotype-specific primers to recognize sequences unique to each dengue virus serotype, as previously demonstrated by Lanciotti et al., 1992 and Chien et al., 2006 (70, 71).
IgM Capture ELISA [UMELISA Dengue IgM Plus (UM2016), Tecnosuma Intl, La Havana] and IgG Capture ELISA [Panbio Dengue IgG Capture ELISA (01PE10)] were used to confirm the cases. Positive results for either of these tests were further analyzed by NS1 antigen ELISA Panbio Dengue Early ELISA (01PE40). Additionally, indirect IgG ELISA [Panbio Dengue IgG Indirect ELISA (01PE30)] was performed to assess the history of dengue infection in enrolled patients. Approximately 10% of the patients provided a second serum sample during convalescence (10 to 30 days after the acute phase). These samples were processed for IgM and IgG Capture ELISA to determine seroconversion.
Dengue cases were clinically diagnosed and classified according to the 2009 WHO criteria (17). Clinical signs and symptoms identified the same day where sample was taken, was considered to stablish dengue clinical diagnosis. Additionally, hematological parameters were assessed in the following groups: dengue without warning signs (DWoWS), dengue with warning signs (DWS), and severe dengue (SD); however, the final classification was based on laboratory tests.
A confirmed dengue case was positive by IgM capture ELISA or RT-PCR or if there was an IgM seroconversion between the acute and convalescent samples – considering these tests as standard reference-. The remaining samples were categorized as having other febrile illnesses (OFI). Finally, to establish the percentage of true dengue cases, an algorithm integrating the clinical description combined with laboratory findings was derived and divided into three diagnostic categories: dengue without warning signs (DWoWS), dengue with warning signs (DWS), and severe dengue (SD) (Figure 1).
Figure 1 Design of the study dengue clinical diagnosis, case confirmation, and classification of febrile patients according to the severity of the disease. All febrile patients were evaluated, clinical and laboratory information were considered to confirm dengue cases. Non dengue cases were defined as other febrile illness. Dengue cases were classified according to disease severity DWoWS, DWS and SD.
Quantitative variables measuring central tendency (mean) and dispersion (standard deviation) were employed after checking the normality of their distribution using the Shapiro–Wilk test. If the assumption was not verified, they were described using median and interquartile ranges. Qualitative variables are described using proportions. To compare the difference between groups (i.e., according to diagnosis), a one-way ANOVA was utilized for continuous variables with normal distribution, and Kruskal–Wallis test was used for non-parametric data. For qualitative variables, differences were calculated using Pearson´s chi-square test when the expected square values were ≥5. Otherwise, Fisher’s exact test was used. Dengue diagnostic test sensitivity, specificity, and predictive values were determined. Receiver operating characteristic (ROC) curves were derived, and the area under the curve (AUC) was estimated to compare the dengue laboratory tests and the confirmation case algorithm or if there was an improvement after combining a few parameters from them.
Odds ratios (ORs) were calculated to identify the confounding clinical variables in the final diagnosis and functional and valuable variables to build a logistic regression model to measure the association between signs and symptoms and the final diagnosis outcome: DWoWS, DWS, and SD. Regression analysis was performed to calculate the adjusted odds ratios (aOR) and 95% CI; models presenting p< 0.05 were included to propose the decision trees. The model’s reliability was evaluated through re-estimation tests, the deviance test, and the Hosmer–Lemeshow goodness of fit test. Statistical significance was set at p <0.05. Statistical analyses were performed using Stata V.12.1.
Clinical and laboratory data of 505 patients were evaluated (54.7% male); the median age was 11 years (interquartile range [IR 6 – 23]), with 80.6% of patients presenting within the first seven days of the illness (Table 1). Only 21.9% had a temperature above 38°C during the day of admission (Table S1). Following the clinical criteria suggested by the WHO and the results of defined laboratory dengue tests (ELISA IgM, RT-PCR, or seroconversion), a definitive diagnosis was assigned to each patient, and 305 (76.2%) were confirmed cases of dengue with 19% DWoWS, 45.7% DWS, and 11.5% SD cases. Dengue serotype 2 (64.4%) was the most frequent in confirmed dengue cases. Patients who were negative for the selected tests were classified as having OFI (23.8%). Table S1 describes the distribution of febrile patients according to the tests considered in standard reference.
The most frequently reported symptoms in all febrile patients analyzed were myalgia (81.8%), headache (73.5%), abdominal pain (61.8%), nausea (53.8%), vomiting (53.6%), and retro-ocular pain (31%).
The main identifiable signs were abdominal pain on palpation (54.6%), hepatomegaly (14.1%), ascites (10.9%), and edema (10.3%). In addition, the leukocyte count was <4,000/mm3 in 34.9% of the patients, while platelet counts <100,000/mm3 were observed in 43.7% of the patients (Table 2).
Dengue IgM and IgG RDT were positive in 64.1% and 66.1% of the samples, respectively. However, only 30.5% of the samples were positive for dengue NS1 antigen rapid test NS1. IgM capture, IgG capture, and NS1 ELISAs were positive in 63.4%, 52.4%, and 30.5% of the patients, respectively (Figure 2).
Clinical manifestations such as myalgia (84.2% vs. 73.8%), rash (27.1% vs. 8.8%), and abdominal pain (55.7% vs. 45.9%) were significantly more frequent in dengue confirmed cases than in the OFI group (p<0.05). For example, 43.2% of patients in the OFI group had abdominal tenderness on palpation compared to 74.8% in the DWS group and 80.4% in the SD group. In addition, other symptoms such as hepatomegaly and ascites were significantly more frequent in dengue cases than in OFI patients. For instance, hepatomegaly was identified in 18.5% of DWS patients and 42.1% in the SD group vs. 3.4% in the OFI group (p<0.001). Edema was seen in 11.7% of the DWS patients and 29.8% in the SD group vs. 6.7% in the OFI group (p<0.001). In contrast, exanthema was reported in fewer patients in the entire cohort (10.1%, 5.2%, 3.6%, and 2.5% in the DWS, DWoWS, SD, and OFI groups, respectively) (Figure 3). However, the OFI group showed respiratory difficulty (5.0%) and pleural effusion (2.5%), two characteristic clinical signs commonly seen in patients with severe dengue (72.4% and 100%, respectively). In addition, platelet counts were significantly lower in the dengue confirmed cases than in the OFI group (p=0.0001).
Figure 3 Clinical features of febrile patients according to diagnosis and disease severity. Maps present clinical information from febrile patients included in the study, according to diagnosis: Dengue without warning signs (DWoWS), dengue warning signs (DWS), severe dengue (SD), Other Febrile Illness (OFI). Colors represent the percentage of patients presenting specific clinical sign, light gray represent absence of signs or symptoms in the diagnostic group.
Considering confirmed dengue cases as the reference, we compared the performance of the RDT and other tests performed in this study. Positive IgM RDT was significantly lower in DWoWS patients (60%) than in DWS (73.2%) or SD cases (77.2%) (p=0.029), and similar results were observed for IgG RDT (56.8% of dengue samples were positive and 77.1% and 80.7% were positive in the DWS and SD groups, respectively; p<0.0001). However, there was no difference in the number of NS1 RDT- positive patients between the groups (p=0.612). The percentage of IgM Capture ELISA-positive cases was significantly different between the groups (64.1%, 86.8%, and 89.1% for DWoWS, DWS, and SD, respectively; p<0.001). Similar results were observed for the IgG capture ELISA, where 39.1%, 68.1%, and 58.9% of cases were positive in the DWoWS, DWS, and SD groups, respectively (p<0.001). The NS1 ELISA test results did not show differences between the groups (p=0.630). On the other hand, RT-PCR detected significantly more cases in the DWoWS group (70.2%) than in the DWS group (55.2%) and SD group (50.0%) (p=0.018). According to the RT-PCR, the DENV-2 was the most frequently identified serotype in the DWoWS, DWS, and SD groups (69.7%, 63.0%, and 58.6%, respectively), followed by DENV-1 (12.1%, 14.2%, and 17.2% in DWoWS, DWS, and SD group, respectively). We also observed that DENV-1/DENV-2, DENV-1/DENV-3, and DENV-2/DENV-3 coinfections were slightly higher in the SD cases (13.8%) than in the DWS and dengue groups (13.4% and 9.1%, respectively) (Figure 4). Based on indirect IgG ELISA, at study entry, 85.7% of patients with febrile syndrome had IgG antibodies, and within dengue confirmed cases 83.9% DWoWS, 90.9 DWS, and 94.7 SD had IgG antibodies (p=0.069). We found that 64.5% of febrile patients (children <5 years) have had a DENV infection previously of which 77.9% developed secondary dengue infection and 22.1% primary infection.
Figure 4 Serotypes of DENV identified from dengue samples. (A) Distribution of serotypes of DENV identified according to disease severity. Co-infections are defined by the detection of two DENV serotypes in the patient's sample. (B) Description of DENV coinfections.
We analyzed different tests to determine the most accurate in confirming dengue cases. The sensitivity, specificity, predictive values, and ROC area under the curve (AUC) were estimated for IgM, IgG, NS1 RDT, IgG capture, and NS1 ELISA using confirmed dengue cases as a reference standard (positive samples for IgM Capture ELISA or RT-PCR). The individual sensitivity and specificity were 81.6% and 58.1% for capture IgM ELISA and RT-PCR, respectively (Table 3).
Individual IgM, IgG, and NS1 RDT results did not yield higher values than those obtained in the reference standard to confirm cases; similar results were observed after analyzing IgG capture and NS1 ELISAs (Table S2). However, the sensitivity (97.0%), specificity (82.1%), and ROC AUC (0.896) after combining IgM Capture and NS1 ELISA were higher than those obtained with individual tests (Table 3). However, these results were not replicated when IgM Capture ELISA was combined with IgG Capture ELISA or when NS1 ELISA was combined with IgG Capture ELISA during the analysis. To complement this information, combining the results of NS1 RDT and capture IgM ELISA showed better values (90.3%, 96.2%, and 0.932 for sensitivity, specificity, and AUC, respectively) than those obtained for each test that constituted the reference standard. Furthermore, the positive results from RDT (IgM, IgG, or NS1) combined with the RT-PCR results showed a higher sensitivity (97.4%) but low specificity (36.3%) and AUC (0.668).
On the other hand, analysis using a combination of any of the positive RDT (IgM OR IgG OR NS1) showed a higher sensitivity (90.5%) but lower specificity (37.4%) compared to ELISA tests. In addition, when we evaluated the combination of results of two RDTs, with one of them being positive (IgM OR IgG; IgM OR NS1; IgG OR NS1), the sensitivity identified was 84.1%, 80.5%, and 85.5%, respectively, and the specificities were 39.7%, 54.8%, and 52.2%, respectively. However, we observed a significant decrease in the sensitivity (22.1%) and an increase in the specificity (99.1%) by combining the positive test results of the three RDTs (Table S2). Thus, we did not find improvement in the sensitivity or specificity in confirming dengue cases upon evaluating a combination of leukocyte or platelet counts with the results of individual dengue diagnostic tests compared to the reference standard.
Using the median and interquartile ranges of leukocytes and platelets of dengue confirmed cases and OFI patients, we established a dengue-related disease cutoff of 5,100 cells/mm3 and 140,000 platelets/mm3. These data were used to perform a univariate analysis and calculate the odds ratio (OR) for symptoms, signs, and laboratory diagnostic test results. This approach showed that myalgia, abdominal tenderness, platelet count of <140,000/mm3, and the result of the RDT (alone or in combination) were identified with higher sensitivity to confirm dengue cases (Table 4).
Table 4 Univariate analysis of clinical and laboratory variables as possible predictors of dengue diagnosis.
In contrast, for this cohort, clinical signs such as fever, exanthema, leukopenia <5,100/mm3, days of disease evolution, and age were not significantly associated with dengue diagnosis. Following the univariate analysis, variables with p-values of less than 0.05 were included in the logistic regression analysis to determine the effect of these variables on the diagnosis of dengue. The adjusted OR estimates (aOR) showed that myalgia (aOR: 1.87, 95% CI: 1.04-3.38, p=0.038), abdominal tenderness (aOR: 1.89, 95% CI: 1.14-3.10, p=0.013), platelet count less than 140,000/mm3 (aOR: 2.19, 95% CI: 1.31-3.67 p=0.003), and IgM RDT positive result (aOR: 2.63, 95% CI: 1.59-4.33, p<0.001) were independent variables that enabled a differential diagnosis of dengue compared to OFI. The positive NS1 RDT results adjusted for these signs also differentiated dengue cases from OFI cases (Table 5).
Therefore, using the results of the IgM RDT, NS1 RDT, and the combination of IgM RDT or NS1 RDT with the afore mentioned clinical variables allowed the differentiation between dengue cases and OFI cases (Table 5).
The significant variables from the regression analysis were used to establish decision trees with the RDT results representing the first branch and the presence or absence of clinical signs in the secondary branches (Figure S1). For example, for the IgM RDT decision tree, compared to the confirmed cases reference standard, we detected 72.4% of the cases. In contrast, for the NS1 RDT decision tree, the percentage of detected dengue cases was 37%. Furthermore, the decision tree using the combined positive cases for NS1 RDT or IgM ELISA, we could detect 90.6% of dengue cases with LR values LR (+) = 25.88 and LR (–) = 0.09 respectively (Figures 5A, S1A; Table S5). While the decision tree using the IgM RDT OR NS1 RDT positive cases detected 81.6% of true cases (Figures 5B, S1B).
Figure 5 Scheme of dengue cases confirmed by the proposed algorithms using diagnostic test, clinical and laboratory variables. (A) Algorithm NS1 RDT OR IgM ELISA (Platelets, Myalgia and Abdominal pain). The area highlighted in blue represents dengue confirmed cases (True positive) when applying the proposed algorithm identified as novel algorithm. Green circles (True negative), defined as non dengue cases by standard reference (OFI). Gray circles represent dengue cases confirmed by standard reference but negative by novel algorithm (False negative). (B) Algorithm IgM RDT OR NS1 RDT (Platelets, Myalgia and Abdominal pain), Colours description is the same as the description in section 5a. Analysis was performed using information from patients who met all clinical and laboratory variables.
Based on a robust dengue case definition, we challenged serology and clinical characteristics of dengue infection in pediatric population from endemic areas to improve case confirmation. A combined strategy yielded high sensitivity and specificity rates that could help clinicians, especially in underdeveloped countries, to gain opportunity and accuracy in confirming dengue cases in endemic areas. The evaluation of diagnostic algorithms for dengue is challenging because of the dynamics of its clinical presentation (49, 54). The disease includes significant changes hematological, biochemical, and immunological biomarkers assessed by clinicians in dengue patients (51, 73, 74), specially in patients with similar sociodemographic conditions, characteristics such as age, time of disease, degree of severity, and host genetic factors (50, 75, 76). These differences make it difficult to stablish standard diagnostic algorithms for the treatment and management of dengue due to the degree of uncertainty they generate and their effectiveness in early identification of true cases. However, the search and application of diagnostic algorithms is urgent, since they may be the major strategy to reduce the percentage of people who develop complications, and the costs associated with care and treatment of the infection.
Our results offer two reliable and efficient options that can be applied in Colombia and endemic countries with a high incidence of dengue in children, where the number of medical appointments, hospitalizations and complications related to dengue infection increase, especially in populations with unfavorable socio-economic situations and difficulties in accessing to health services for disease diagnosis and timely management. The first algorithm, which involved the simultaneous application of the NS1 RDT OR IgM ELISA combined with Platelets, Myalgias, and Abdominal pain during dengue cases management, can be used in specialized health care institutions that have the development capacity to process patients samples within the routine diagnostic panel. The second algorithm IgM RDT OR NS1 RDT combined with Platelets, Myalgias, and Abdominal pain, can be used in health care institutions in endemic areas because of the combination of low cost and accessible diagnostic tests, that complement clinical findings identified during patients assessment, which would allow early diagnosis for disease management and treatment. The application of this new algorithm could increase the number of confirmed cases, since the test has the capacity to detect those cases that IgM ELISA test can not identify or define as negative casesm and establish the diagnosis as other febrile syndrome (OFI).
The evaluation of the patients included in the study, sample collection and processing were performed in a period prior to Zika virus introduction and its spread in Colombia, which allowed the control of cross-reactivity in the tests applied. Proposed algorithms requires the evaluation of reproducibility in areas where there is evidence of dengue-zika co-circulation, in populations with similar characteristics, which could show differences in the percentage of detected cases. However, the search and application of diagnostic algorithms is urgent, since they may be the major strategy to reduce the percentage of people who develop complications, and the costs associated with care and treatment of dengue infection.
Colombia as most underdeveloped countres, laboratory diagnosis of dengue infection is based on assessment of IgM antibodies, over viral RNA detection or measure of viral antigens in patient samples. ELISA tests in diagnosis and confirmation of infectious diseases is only performed by reference laboratories or those specialiced in epidemiological surveillance.
The percentage of positive results for IgM and IgG tests observed in our study differed from those reported in other studies. Senaratne et al. found that the percentage of samples positive for IgM and IgG was 61.9% and 83.9%, respectively (77). We observed that differences could be explained by three population characteristics: (1) age of the subjects, (2) place of residence, and (3) duration of residence. We found that the percentage of children <5 years have had a DENV infection previously (64.5%), of which confirmed diagnosis of dengue infection was confirmed in 72 subjects. The analysis showed that 22.1% of this patients was experiencing a primary DENV infection, while most of these patients was experiencing secondary infections (77.9%).
We also demonstrated the usefulness of rapid diagnostic tests coupled with IgM ELISA allows the confirmation of a high percentage of dengue cases. NS1 detection in febrile patient samples has become a valuable tool in the early diagnosis of DENV infection. In this work we found that the NS1 RDT sensitivity was 37.8% and 39.3% for ELISA NS1. These values were similar to that reported by other studies in Colombia, Brazil (43, 46, 78), and Singapore (79).
However, our results differ from the findings of studies conducted in Vietnam (24, 57, 74) and Malaysia (80), where the reported sensitivity of these tests were higher than 80%. These differences in sensitivity may be related to factors such as the assay type applied (RDT OR ELISA) (43, 44, 46) and the frequency of DENV-2 infections. Previous studies have reported a loss of sensitivity of NS1 detection tests in secondary DENV-2 infections (20, 44, 57, 81). Additionally, a decrease in sensitivity of NS1 test has been observed in secondary infections due to the presence of NS1 antibodies from a previous infection, which in a secondary infection may block the antigens and inhibit its detection (43, 79, 82).
In laboratory case confirmation, the percentage of positive cases or dengue infection was statistically different between IgM RDT (64.1%) and IgM ELISA (63.4%) (p= 0.033 and p<0.0001, respectively). The obtained values for sensitivity (70.5%) and specificity (56.9%) of the IgM RDT, compared to the reference standard, were slightly lower than the values obtained by other studies which are 70-80% (60, 76). These differences may be explained by variables such as the day of illness on which samples were obtained, diagnostic test were applied and the percentage of secondary infections identified, these variables are critical to establish this type of inference.
The evaluation of diagnostic algorithms for dengue represents a challenge due to the dynamics of clinical presentation, specially in pediatric population (49, 54). Similarly, haematological, biochemical and immunological biomarkers assessed in dengue patients show significant variability (51, 73, 83). These differences have been observed even in populations with similar characteristics such as age, time of illness, degree of severity and genetic characteristics of individuals (51, 73, 75). Additionally, differences raise the challenge of including diagnostic algorithms in dengue management protocols for patients care, as their application generates a certain degree of uncertainty about their usefulness and efficacy in early case identification. However, the use of diagnostic algorithms may lead to a reduction in the percentage of individuals who develop complications and must afford the costs associated with the care of these patients.
Similar to the results reported by Tukasan et al. (84), we found differences in temperature between severe dengue and DWoWS cases (p=0.0008), as well as in DWS and DWoWS cases (p=0.0051). Analysis of signs and symptoms such as headache, myalgia, nausea and vomiting showed no significant differences between dengue cases and the OFI group (21). These results are consistent with the research findings of Malhi et al. (85) and nd Chaloemwong et al. (25). The clinical sign abdominal pain on palpation in individuals with febrile syndrome allowed classification of dengue cases according to the degree of disease severity, and statistically significant differences were found when this sign were compared between dengue cases and OFI group (p=0.005). However, this symptom was not identified as a predictor variable for inclusion in the regression models and the design of the diagnostic algorithm, so it was not included. In contrast, the patient-reported abdominal pain sign was found as a predictor variable (OR:2.36) and was considered in the development of the regression models. The results of the study are consistent with the study developed by Da Silva et al. in which found the application of the WHO clinical criteria useful to identify dengue cases with alarm signs, with a high probability of developing severe dengue (86).
In our analysis, we found that the combination leads to an increase in the predictive values (AUC 0.932) compared to the reference standard, along with a higher sensitivity (90.3%) and specificity (96.2%). These results are consistent with those of a study by Clemen et al. in Colombia (81), who observed an increase in sensitivity (between 67.2% to 79.5%) when IgM RDT and NS1 were combined with a negative clinical diagnosis (no clinical criteria for dengue case). In contrast, they found that combining a positive clinical diagnosis with RDT resulted in a decrease in sensitivity (less than 35%) and an increase in specificity (from 66.3% to 98.7% and 97.3%, respectively).
The regression models developed in our study included clinical variables and the combination of clinical variables, diagnostic tests, and hematological variables, such as leukocyte and platelet counts. These models also evaluated likelihood ratios (taking in to account variables (e.g disease prevalence), and we found that of NS1 RDT with the IgM ELISA test, together with clinical and hematological variables, showed that in endemic areas is able to confirm dengue cases with higher sensitivity and specificity, and the same algorithm in non-endemic areas strengthens the post-test odds. A Taiwanese study performed a similar analysis, which identified the usefulness of combining laboratory variables such as leukocyte and platelet counts, liver function tests, and coagulation profiles in laboratory-confirmed dengue cases. They showed low sensitivity (49.5%) with a high positive predictive value (51).
Among the limitations of our study is the low volume of serum samples obtained during the febrile phase, especially in patients under five years of age, which limited the application of the full panel of diagnostic tests, and may have resulted in a loss of individuals in the multivariate analysis. The design of the algotithms did not consider the day of illness due to most patients were between 4 and under 7 days of illness (54.1%) and lower percentage > 7 days (9.9%). However, among the main strengths of our study are the complete clinical information of the patients collected during their attendance at the institution and the number of laboratory diagnostic tests used to confirm dengue cases and could be applied in febrile patients in early or late febrile phase. In addition, the assessment of patients and the design of the algorithm applied during the study period, before the circulation of other arboviruses, minimizes possible cross-reactivity in diagnostic test results as potential confounding variables.
In conclusion, our findings show that clinical diagnosis alone is not helpful in confirming dengue cases and needs to be complemented by laboratory tests. Furthermore, the proposed algorithms demonstrate the usefulness of rapid diagnostic tests in combination with IgM ELISA to confirm a higher percentage of dengue cases. Thus, we suggest that using IgM RDT or NS1 RDT with clinical variables would allow us differentiating dengue and OFI cases. Considering that 70% of the individuals in our study involved the pediatric population (under 15 years), we suggest the evaluation and application of our algorithms in a multicenter study, in both pediatric and adult populations, to confirm their usefulness in the diagnosis and confirmation of dengue cases in endemic areas and are in risk to develop secondary infections.
The original contributions presented in the study are included in the article/Supplementary Material. Further inquiries can be directed to the corresponding author.
The studies involving human participants were reviewed and approved by Ethics Committee Hospital Universitario de La Samaritana. Written informed consent to participate in this study was provided by the participants’ legal guardian/next of kin.
CC-R: Conceptualization, patient enrolment, samples collection and processing, data management, analysis, and interpretation, manuscript writing. MV-R: Project manager, conceptualization, data interpretation, manuscript revision. EC: Molecular tests processing, data interpretation. SC-O: Samples collection, serology testing, and molecular biology processing. SP-A: Samples collection and serology testing. EB-Z: Molecular test processing. MC-P: Molecular test processing. FC-M: Data analysis and interpretation, manuscript revision. JR-H: Clinical data interpretation, classification of dengue cases according clinical criteria. SV-A: Enrolment and follow-up of patients. AP-J: Patient data interpretation, manuscript revision. JC: Principal Investigator (PI), activities of study conceptualization and funding acquisition, data interpretation and manuscript revision. All authors read and approved the final manuscript. All authors contributed to the article and approved the submitted version.
The project was funded by the Vicerrectoría de Investigaciones, Universidad El Bosque (PCI2013-457, PCI 2017-9423, PCI 2018-10297, research fellowship program) and the Colombian Department for Science, Technology, and Innovation (Minciencias) project: Red de Investigación Multidisciplinaria para la prevención y control de Enfermedades Transmitidas por Vectores Red ETV (Project 360-2011).
We are grateful to Miguel Otero Cadena, Vice-Chancellor of Research of Universidad El Bosque and members from Grupo de Virología of Universidad El Bosque. We also thank the Caucaseco Research Center (CICC), health care professionals from Hospital Universitario de La Samaritana – Unidad Funcional Girardot, for their assistance in the patient recruitment phase, follow-up, and sample collection. Finally, we are extremely grateful to the study participants, adults, children, and their parents.
The authors declare that the research was conducted in the absence of any commercial or financial relationships that could be construed as a potential conflict of interest.
All claims expressed in this article are solely those of the authors and do not necessarily represent those of their affiliated organizations, or those of the publisher, the editors and the reviewers. Any product that may be evaluated in this article, or claim that may be made by its manufacturer, is not guaranteed or endorsed by the publisher.
The Supplementary Material for this article can be found online at: https://www.frontiersin.org/articles/10.3389/fitd.2023.1118774/full#supplementary-material
DENV, Dengue virus; DWoWS, Dengue Without Warning signs; DWS, Dengue Warning Signs; SD, Severe dengue; OFI, other febrile illnesses; RDT, Rapid diagnosis test; ELISA, Enzyme-linked immunosorbent assay; AUC, area under the curve; OR, Odds ratio.
1. Guzman MG, Gubler DJ, Izquierdo A, Martinez E, Halstead SB. Dengue infection. Nat Rev Dis Primers. (2016) 2:16055. doi: 10.1038/nrdp.2016.55
2. Halstead S. Recent advances in understanding dengue. F1000Res (2019) 8. doi: 10.12688/f1000research.19197.1
3. Castro MC, Wilson ME, Bloom DE. Disease and economic burdens of dengue. Lancet Infect Diseases. (2017) 17(3):e70–e8. doi: 10.1016/S1473-3099(16)30545-X
4. Castro Rodriguez R, Carrasquilla G, Porras A, Galera-Gelvez K, Lopez Yescas JG, Rueda-Gallardo JA. The burden of dengue and the financial cost to Colombia, 2010-2012. Am J Trop Med Hyg (2016) 94(5):1065–72. doi: 10.4269/ajtmh.15-0280
5. Tapia-Conyer R, Betancourt-Cravioto M, Mendez-Galvan J. Dengue: an escalating public health problem in Latin America. Paediatr Int Child Health (2012) 32 Suppl 1:14–7. doi: 10.1179/2046904712Z.00000000046
6. Rezza G. Dengue and chikungunya: long-distance spread and outbreaks in naive areas. Pathog Glob Health (2014) 108(8):349–55. doi: 10.1179/2047773214Y.0000000163
7. Paixao ES, Teixeira MG, Rodrigues LC. Zika, chikungunya and dengue: the causes and threats of new and re-emerging arboviral diseases. BMJ Glob Health (2018) 3(Suppl 1):e000530. doi: 10.1136/bmjgh-2017-000530
8. Mardekian SK, Roberts AL. Diagnostic options and challenges for dengue and chikungunya viruses. BioMed Res Int (2015) 2015:834371. doi: 10.1155/2015/834371
9. Lee VJ, Chow A, Zheng X, Carrasco LR, Cook AR, Lye DC, et al. Simple clinical and laboratory predictors of chikungunya versus dengue infections in adults. PloS Negl Trop Dis (2012) 6(9):e1786. doi: 10.1371/journal.pntd.0001786
10. Paternina-Caicedo A, de la Hoz-Restrepo F, Diaz-Quijano F, Caicedo-Torres W, Auxiliadora Badillo-Viloria M, Bula-Anichiarico D, et al. Features of dengue and chikungunya infections of Colombian children under 24 months of age admitted to the emergency department. J Trop Pediatr (2018) 64(1):31–7. doi: 10.1093/tropej/fmx024
11. Sam SS, Omar SF, Teoh BT, Abd-Jamil J, AbuBakar S. Review of dengue hemorrhagic fever fatal cases seen among adults: a retrospective study. PloS Negl Trop Dis (2013) 7(5):e2194. doi: 10.1371/journal.pntd.0002194
12. Pongpan S, Wisitwong A, Tawichasri C, Patumanond J, Namwongprom S. Development of dengue infection severity score. ISRN Pediatr (2013) 2013:845876. doi: 10.1155/2013/845876
13. Trung DT, Trieu HT, Wills BA. Microvascular fluid exchange: Implications of the revised starling model for resuscitation of dengue shock syndrome. Front Med (Lausanne). (2020) 7:601520. doi: 10.3389/fmed.2020.601520
14. Harapan H, Michie A, Sasmono RT, Imrie A. Dengue: A minireview. Viruses (2020) 12(8):829. doi: 10.3390/v12080829
15. Muller DA, Depelsenaire AC, Young PR. Clinical and laboratory diagnosis of dengue virus infection. J Infect Dis (2017) 215(suppl_2):S89–95. doi: 10.1093/infdis/jiw649
16. Buonora SN, Passos SRL, Daumas RP, Machado MGL, Berardinelli GM, de Oliveira DNR, et al. Pitfalls in acute febrile illness diagnosis: Interobserver agreement of signs and symptoms during a dengue outbreak. J Clin Nurs (2020) 29(9-10):1590–8. doi: 10.1111/jocn.15229
17. World Health Organization. Dengue guidelines for diagnosis, treatment, prevention and control : New edition (World Health Organization). (2009). Available at: https://apps.who.int/iris/handle/10665/44188
18. World Health Organization. UNICEF/UNDP/World Bank/WHO special programme for research and training in tropical diseases. In: Handbook for clinical management of dengue, vol. 111. (2012).
19. Ministerio de la Protección Social, Instituto Nacional de Salud, Organización Panamericana de la Salud. Guía para la atención clínica integral del paciente con dengue. (2010). p. 46.
20. Mora-Cardenas E, Aloise C, Faoro V, Knap Gasper N, Korva M, Caracciolo I, et al. Comparative specificity and sensitivity of NS1-based serological assays for the detection of flavivirus immune response. PloS Negl Trop Dis (2020) 14(1):e0008039. doi: 10.1371/journal.pntd.0008039
21. Tanner L, Schreiber M, Low JG, Ong A, Tolfvenstam T, Lai YL, et al. Decision tree algorithms predict the diagnosis and outcome of dengue fever in the early phase of illness. PloS Negl Trop Dis (2008) 2(3):e196. doi: 10.1371/journal.pntd.0000196
22. Lee VJ, Lye DC, Sun Y, Leo YS. Decision tree algorithm in deciding hospitalization for adult patients with dengue haemorrhagic fever in Singapore. Trop Med Int Health (2009) 14(9):1154–9. doi: 10.1111/j.1365-3156.2009.02337.x
23. Lee IK, Liu JW, Chen YH, Chen YC, Tsai CY, Huang SY, et al. Development of a simple clinical risk score for early prediction of severe dengue in adult patients. PloS One (2016) 11(5):e0154772. doi: 10.1371/journal.pone.0154772
24. Nguyen MT, Ho TN, Nguyen VV, Nguyen TH, Ha MT, Ta VT, et al. An evidence-based algorithm for early prognosis of severe dengue in the outpatient setting. Clin Infect Dis (2017) 64(5):656–63. doi: 10.1093/cid/ciw863
25. Chaloemwong J, Tantiworawit A, Rattanathammethee T, Hantrakool S, Chai-Adisaksopha C, Rattarittamrong E, et al. Useful clinical features and hematological parameters for the diagnosis of dengue infection in patients with acute febrile illness: a retrospective study. BMC Hematol (2018) 18:20. doi: 10.1186/s12878-018-0116-1
26. Prodjosoewojo S, Riswari SF, Djauhari H, Kosasih H, van Pelt LJ, Alisjahbana B, et al. A novel diagnostic algorithm equipped on an automated hematology analyzer to differentiate between common causes of febrile illness in southeast Asia. PloS Negl Trop Dis (2019) 13(3):e0007183. doi: 10.1371/journal.pntd.0007183
27. Stahl H-C, Butenschoen VM, Tran HT, Gozzer E, Skewes R, Mahendradhata Y, et al. Cost of dengue outbreaks: literature review and country case studies. BMC Public Health (2013) 13(1048):11. doi: 10.1186/1471-2458-13-1048
28. Castro Rodriguez R, Galera-Gelvez K, Lopez Yescas JG, Rueda-Gallardo JA. Costs of dengue to the health system and individuals in Colombia from 2010 to 2012. Am J Trop Med Hyg (2015) 92(4):709–14. doi: 10.4269/ajtmh.14-0386
29. Padilla J, Rojas D, Sáenz-Gómez R. Dengue in Colombia: Epidemiology of Hyperendemic Reemergence. Guías de impresión (Bogotá, Colombia) (2012).
30. Villar LA, Rojas DP, Besada-Lombana S, Sarti E. Epidemiological trends of dengue disease in Colombia (2000-2011): a systematic review. PloS Negl Trop Dis (2015) 9(3):e0003499. doi: 10.1371/journal.pntd.0003499
31. Gutierrez-Barbosa H, Medina-Moreno S, Zapata JC, Chua JV. Dengue infections in Colombia: Epidemiological trends of a hyperendemic country. Trop Med Infect Dis (2020) 5(4):156. doi: 10.3390/tropicalmed5040156
32. Bavia L, Melanda FN, de Arruda TB, Mosimann ALP, Silveira GF, Aoki MN, et al. Epidemiological study on dengue in southern Brazil under the perspective of climate and poverty. Sci Rep (2020) 10(1):2127. doi: 10.1038/s41598-020-58542-1
33. Andrioli DC, Busato MA, Lutinski JA. Spatial and temporal distribution of dengue in Brazil, 1990 - 2017. PloS One (2020) 15(2):e0228346. doi: 10.1371/journal.pone.0228346
34. Luna E, Figueiredo G, Levi J, Campos S, Felix A, Souza N, et al. Data on dengue incidence in south-eastern Brazil, 2014-2018. Data Brief. (2020) 29:105266. doi: 10.1016/j.dib.2020.105266
35. Perez-Castro R, Castellanos JE, Olano VA, Matiz MI, Jaramillo JF, Vargas SL, et al. Detection of all four dengue serotypes in aedes aegypti female mosquitoes collected in a rural area in Colombia. Mem Inst Oswaldo Cruz. (2016) 111(4):233–40. doi: 10.1590/0074-02760150363
36. Velandia-Romero ML, Olano VA, Coronel-Ruiz C, Cabezas L, Calderon MA, Castellanos JE, et al. Detección del virus del dengue en larvas y pupas de aedes aegypti recolectadas en áreas rurales del municipio de anapoima. Biomédica (2017) 2017:193–200. doi: 10.7705/biomedica.v37i0.3584
37. Castellanos JE, Coronel-Ruiz C, Parra-Alvarez S, Castilla MG, Calvo EP, Arévalo-Herrera M, et al. Description of high rates of unapparent and simultaneous multiple dengue virus infection in a Colombian jungle settlement. Trop Biomed (2016) 33:375–82.
38. Carabali M, Lim JK, Velez DC, Trujillo A, Egurrola J, Lee KS, et al. Dengue virus serological prevalence and seroconversion rates in children and adults in medellin, Colombia: implications for vaccine introduction. Int J Infect Dis (2017) 58:27–36. doi: 10.1016/j.ijid.2017.02.016
39. Salgado DM, Vega MR, Panqueva CA, Garzón M, Rodríguez-Godoy JA. Multi-organic dysfunction syndrome caused by dengue 3 in children of Neiva Huila, Colombia. Rev Fac Med (2008) 56(2):147–55.
40. Salgado D, Panqueba C, Castro D, Vega M, Rodriguez J. Myocarditis in children affected by dengue hemorrhagic fever in a teaching hospital in Colombia. Rev salud pública (2009) 11(4):591–600.
41. Instituto Nacional de Salud. Subdirección de prevención, vigilancia y control en salud pública. In: Informe final dengue, Colombia, 2014. Instituto Nacional de Salud (2014).
42. Instituto Nacional de Salud. Subdirección de prevención, vigilancia y control en salud pública. In: Informe del evento dengue, Colombia, hasta el décimo tercer período epidemiológico, 2015, vol. 29. (2015).
43. Osorio L, Ramirez M, Bonelo A, Villar LA, Parra BA. Comparison of the diagnostic accuracy of commercial NS1-based diagnostic tests for early dengue infection. Virol J (2010) 7(361):1–10. doi: 10.1186/1743-422X-7-361
44. Osorio L, Uribe M, Ardila GI, Orejuela Y, Velasco M, Bonelo A, et al. The use of rapid dengue diagnostic tests in a routine clinical setting in a dengue-endemic area of Colombia. Mem Inst Oswaldo Cruz. (2015) 110(4):510–6. doi: 10.1590/0074-02760140359
45. Tello-Cajiao ME, Osorio L. Impact of dengue rapid diagnostic tests on the prescription of antibiotics and anti-inflammatory drugs by physicians in an endemic area in Colombia. Am J Trop Med Hyg (2019) 101(3):696–704. doi: 10.4269/ajtmh.19-0222
46. Piedrahita LD, Agudelo IY, Trujillo AI, Ramirez RE, Osorio JE, Restrepo BN. Evaluation of commercially available assays for diagnosis of acute dengue in schoolchildren during an epidemic period in medellin, Colombia. Am J Trop Med Hyg (2016) 95(2):315–21. doi: 10.4269/ajtmh.15-0492
47. Low GKK, Kagize J, Faull KJ, Azahar A. Diagnostic accuracy and predictive value in differentiating the severity of dengue infection. Trop Med Int Health (2019) 24(10):1169–97. doi: 10.1111/tmi.13294
48. Daumas RP, Passos SR, Oliveira RV, Nogueira RM, Georg I, Marzochi KF, et al. Clinical and laboratory features that discriminate dengue from other febrile illnesses a diagnostic accuracy study in Rio de Janeiro, Brazil. BMC Infect Dis (2013) 13:77. doi: 10.1186/1471-2334-13-77
49. Lovera D, Araya S, Mesquita MJ, Avalos C, Ledesma S, Arbo A. Prospective applicability study of the new dengue classification system for clinical management in children. Pediatr Infect Dis J (2014) 33(9):933–5. doi: 10.1097/INF.0000000000000340
50. Phakhounthong K, Chaovalit P, Jittamala P, Blacksell SD, Carter MJ, Turner P, et al. Predicting the severity of dengue fever in children on admission based on clinical features and laboratory indicators: application of classification tree analysis. BMC Pediatr (2018) 18(1):109. doi: 10.1186/s12887-018-1078-y
51. Ho TS, Wang SM, Lin YS, Liu CC. Clinical and laboratory predictive markers for acute dengue infection. J Biomed Sci (2013) 20(1):75. doi: 10.1186/1423-0127-20-75
52. Jaenisch T, Tam DT, Kieu NT, Van Ngoc T, Nam NT, Van Kinh N, et al. Clinical evaluation of dengue and identification of risk factors for severe disease: protocol for a multicentre study in 8 countries. BMC Infect Dis (2016) 16:120. doi: 10.1186/s12879-016-1440-3
53. Diaz-Quijano FA, Figueiredo GM, Waldman EA, Figueiredo WM, Cardoso MRA, Campos SRC, et al. Comparison of clinical tools for dengue diagnosis in a pediatric population-based cohort. Trans R Soc Trop Med Hyg (2019) 113(4):212–20. doi: 10.1093/trstmh/try135
54. Khosavanna RR, Kareko BW, Brady AC, Booty BL, Nix CD, Lyski ZL, et al. Clinical symptoms of dengue infection among patients from a non-endemic area and potential for a predictive model: A multiple logistic regression analysis and decision tree. Am J Trop Med Hyg (2021) 104(1):121–9. doi: 10.4269/ajtmh.20-0192
55. Caicedo-Borrero DM, Tovar JR, Mendez A, Parra B, Bonelo A, Celis J, et al. Development and performance of dengue diagnostic clinical algorithms in Colombia. Am J Trop Med Hyg (2020) 102(6):1226–36. doi: 10.4269/ajtmh.19-0722
56. Caicedo M, Méndez A, Tovar J, Osorio L. Desarrollo de algoritmos clínicos para el diagnóstico del dengue en Colombia. Biomédica (2019) 39:170–85. doi: 10.7705/biomedica.v39i1.3990
57. Tuan NM, Nhan HT, Chau NV, Hung NT, Tuan HM, Tram TV, et al. Sensitivity and specificity of a novel classifier for the early diagnosis of dengue. PloS Negl Trop Dis (2015) 9(4):e0003638. doi: 10.1371/journal.pntd.0003638
58. Guzman MG, Jaenisch T, Gaczkowski R, Ty Hang VT, Sekaran SD, Kroeger A, et al. Multi-country evaluation of the sensitivity and specificity of two commercially-available NS1 ELISA assays for dengue diagnosis. PloS Negl Trop Dis (2010) 4(8):e811. doi: 10.1371/journal.pntd.0000811
59. Fry SR, Meyer M, Semple MG, Simmons CP, Sekaran SD, Huang JX, et al. The diagnostic sensitivity of dengue rapid test assays is significantly enhanced by using a combined antigen and antibody testing approach. PloS Negl Trop Dis (2011) 5(6):e1199. doi: 10.1371/journal.pntd.0001199
60. Tontulawat P PP, Thongmee C, Theamboonlers A, Kamolvarin N, Poovorawan Y. Evaluation of rapid immunochromatographic NS1 test, anti-dengue IgM test, semi-nested PCR and IgM ELISA for detection of dengue virus. Southeast Asian J Trop Med Public Health (2011) 42(3):570–8.
61. Ahmad MH, Ibrahim MI, Mohamed Z, Ismail N, Abdullah MA, Shueb RH, et al. The sensitivity, specificity and accuracy of warning signs in predicting severe dengue, the severe dengue prevalence and its associated factors. Int J Environ Res Public Health (2018) 15(9):2018. doi: 10.3390/ijerph15092018
62. Mata VE, Passos SRL, Hokerberg YHM, Berardinelli GM, Dos Santos MAB, Fukuoka LVB, et al. Precisao e confiabilidade de um teste imuno-cromatografico rapido NS1 para diagnostico DENV-1 no ponto de atendimento e no laboratorio. BMC Infect Dis (2017) 17(1):594.
63. Hunsperger EA, Munoz-Jordan J, Beltran M, Colon C, Carrion J, Vazquez J, et al. Performance of dengue diagnostic tests in a single-specimen diagnostic algorithm. J Infect Dis (2016) 214(6):836–44. doi: 10.1093/infdis/jiw103
64. Nascimento EJM, George JK, Velasco M, Bonaparte MI, Zheng L, DiazGranados CA, et al. Development of an anti-dengue NS1 IgG ELISA to evaluate exposure to dengue virus. J Virol Methods (2018) 257:48–57. doi: 10.1016/j.jviromet.2018.03.007
65. Liu LT, Chen CH, Tsai CY, Lin PC, Hsu MC, Huang BY, et al. Evaluation of rapid diagnostic tests to detect dengue virus infections in Taiwan. PloS One (2020) 15(9):e0239710. doi: 10.1371/journal.pone.0239710
66. Matusali G, Colavita F, Carletti F, Lalle E, Bordi L, Vairo F, et al. Performance of rapid tests in the management of dengue fever imported cases in Lazio, Italy 2014-2019. Int J Infect Dis (2020) 99:193–8. doi: 10.1016/j.ijid.2020.07.008
67. Blessmann J, Winkelmann Y, Keoviengkhone L, Sopraseuth V, Kann S, Hansen J, et al. Assessment of diagnostic and analytic performance of the SD bioline dengue duo test for dengue virus (DENV) infections in an endemic area (Savannakhet province, lao people's democratic republic). PloS One (2020) 15(3):e0230337. doi: 10.1371/journal.pone.0230337
68. Garcia-Betancourt T, Higuera-Mendieta DR, Gonzalez-Uribe C, Cortes S, Quintero J. Understanding water storage practices of urban residents of an endemic dengue area in Colombia: Perceptions, rationale and socio-demographic characteristics. PloS One (2015) 10(6):e0129054. doi: 10.1371/journal.pone.0129054
69. Velandia-Romero ML, Coronel-Ruiz C, Castro-Bonilla L, Camacho-Ortega S, Calderon-Pelaez MA, Castellanos A, et al. Prevalence of dengue antibodies in healthy children and adults in different Colombian endemic areas. Int J Infect Dis (2020) 91:9–16. doi: 10.1016/j.ijid.2019.10.045
70. Lanciotti RS, Calisher CH, Gubler DJ, Chang GJ, Vorndam AV. Rapid detection and typing of dengue viruses from clinical samples by using reverse transcriptase-polymerase chain reaction. J. Clin Microbiol (1992) 30(3):545–51. doi: 10.1128/jcm.30.3.545-551.1992
71. Chien LJ, Liao TL, Shu PY, Huang JH, Gubler DJ, Chang GJ. Development of real-time reverse transcriptase PCR assays to detect and serotype dengue viruses. J Clin Microbiol (2006) 44(4):1295–304. doi: 10.1128/JCM.44.4.1295-1304.2006
72. Calvo EP, Sanchez-Quete F, Duran S, Sandoval I, Castellanos JE. Easy and inexpensive molecular detection of dengue, chikungunya and zika viruses in febrile patients. Acta Trop (2016) 163:32–7. doi: 10.1016/j.actatropica.2016.07.021
73. Dhanoa A, Hassan SS, Ngim CF, Lau CF, Chan TS, Adnan NA, et al. Impact of dengue virus (DENV) co-infection on clinical manifestations, disease severity and laboratory parameters. BMC Infect Dis (2016) 16(1):406. doi: 10.1186/s12879-016-1731-8
74. Ngwe Tun MM, Nguyen TTT, Ando T, Dumre SP, Soe AM, Buerano CC, et al. Clinical, virological, and cytokine profiles of children infected with dengue virus during the outbreak in southern Vietnam in 2017. Am J Trop Med Hyg (2020) 102(6):1217–25. doi: 10.4269/ajtmh.19-0607
75. Baitha U, Halkur Shankar S, Kodan P, Singla P, Ahuja J, Agarwal S, et al. Leucocytosis and early organ involvement as risk factors of mortality in adults with dengue fever. Drug Discovery Ther (2021) 14(6):313–8.
76. Carter MJ, Emary KR, Moore CE, Parry CM, Sona S, Putchhat H, et al. Rapid diagnostic tests for dengue virus infection in febrile Cambodian children: diagnostic accuracy and incorporation into diagnostic algorithms. PloS Negl Trop Dis (2015) 9(2):e0003424. doi: 10.1371/journal.pntd.0003424
77. Senaratne UTN, Murugananthan K, Sirisena P, Carr JM, Noordeen F. Dengue virus co-infections with multiple serotypes do not result in a different clinical outcome compared to mono-infections. Epidemiol Infect (2020) 148:e119. doi: 10.1017/S0950268820000229
78. Prado PS, Almeida Junior JTD, Abreu LT, Silva CG, Souza LDC, Gomes MC, et al. Validation and reliability of the rapid diagnostic test 'SD bioeasy dengue duo' for dengue diagnosis in Brazil: a phase III study. Mem Inst Oswaldo Cruz. (2018) 113(8):e170433. doi: 10.1590/0074-02760170433
79. Pok Kwoon-Yong LY-L, Joshua S, Lee-Ching Ng. Evaluation of nonstructural 1 antigen assays for the diagnosis and surveillance of dengue in Singapore. Vector Borne Zoonotic Dis (2010) 10(10):1009–16. doi: 10.1089/vbz.2008.0176
80. Mat Jusoh TNA, Shueb RH. Performance evaluation of commercial dengue diagnostic tests for early detection of dengue in clinical samples. J Trop Med (2017) 2017:4687182.
81. Clemen G, Angel J, Montes C, Tovar JR, Osorio L. Contribución de la prueba rápida NS1 e IgM al diagnóstico de dengue en Colombia en el periodo pre-zika. Infectio (2019) 23(3):259–65. doi: 10.22354/in.v23i3.790
82. Zhang H, Li W, Wang J, Peng H, Che X, Chen X, et al. NS1-based tests with diagnostic utility for confirming dengue infection: a meta-analysis. Int J Infect Dis (2014) 26:57–66. doi: 10.1016/j.ijid.2014.02.002
83. Ngwe Tun MM, Thant KZ, Inoue S, Kurosawa Y, Lwin YY, Lin S, et al. Serological characterization of dengue virus infections observed among dengue hemorrhagic fever/dengue shock syndrome cases in upper Myanmar. J Med Virol (2013) 85(7):1258–66. doi: 10.1002/jmv.23577
84. Tukasan C, Furlan NB, Estofolete CF, Nogueira ML, da Silva NS. Evaluation of the importance of fever with respect to dengue prognosis according to the 2009 WHO classification: a retrospective study. BMC Infect Dis (2017) 17(1):6. doi: 10.1186/s12879-016-2128-4
85. Mallhi TH, Khan AH, Adnan AS, Sarriff A, Khan YH, Jummaat F. Clinico-laboratory spectrum of dengue viral infection and risk factors associated with dengue hemorrhagic fever: a retrospective study. BMC Infect Dis (2015) 15:399. doi: 10.1186/s12879-015-1141-3
Keywords: dengue, diagnosis, algorithm, case confirmation, diagnostic tests
Citation: Coronel-Ruiz C, Velandia-Romero ML, Calvo E, Camacho-Ortega S, Parra-Alvarez S, Beltrán EO, Calderón-Pelaez MA, Porras-Ramírez A, Cortés-Muñoz F, Rojas-Hernandez JP, Velasco-Alvarez S, Pinzón-Junca A and Castellanos JE (2023) Improving dengue diagnosis and case confirmation in children by combining rapid diagnostic tests, clinical, and laboratory variables. Front. Trop. Dis 4:1118774. doi: 10.3389/fitd.2023.1118774
Received: 08 December 2022; Accepted: 01 March 2023;
Published: 20 March 2023.
Edited by:
Alfonso J. Rodriguez-Morales, Fundacion Universitaria Autónoma de las Américas, ColombiaReviewed by:
Ruth Martinez, Universidad de Santander, ColombiaCopyright © 2023 Coronel-Ruiz, Velandia-Romero, Calvo, Camacho-Ortega, Parra-Alvarez, Beltrán, Calderón-Pelaez, Porras-Ramírez, Cortés-Muñoz, Rojas-Hernandez, Velasco-Alvarez, Pinzón-Junca and Castellanos. This is an open-access article distributed under the terms of the Creative Commons Attribution License (CC BY). The use, distribution or reproduction in other forums is permitted, provided the original author(s) and the copyright owner(s) are credited and that the original publication in this journal is cited, in accordance with accepted academic practice. No use, distribution or reproduction is permitted which does not comply with these terms.
*Correspondence: Jaime E. Castellanos, Y2FzdGVsbGFub3NqYWltZUB1bmJvc3F1ZS5lZHUuY28=
Disclaimer: All claims expressed in this article are solely those of the authors and do not necessarily represent those of their affiliated organizations, or those of the publisher, the editors and the reviewers. Any product that may be evaluated in this article or claim that may be made by its manufacturer is not guaranteed or endorsed by the publisher.
Research integrity at Frontiers
Learn more about the work of our research integrity team to safeguard the quality of each article we publish.