- 1Department of Geography, University College London, London, United Kingdom
- 2Centre for Digital Public Health and Emergencies, Institute for Risk and Disaster Reduction, University College London, London, United Kingdom
- 3Centre for Biodiversity and Environment Research, Department of Genetics, Evolution and Environment, University College London, London, United Kingdom
- 4Department of Atmospheric Sciences, Institute of Astronomy, Geophysics and Atmospheric Sciences (IAG), University of São Paulo, São Paulo, Brazil
- 5Institute of Environmental Sciences, Boğaziçi University, Istanbul, Türkiye
- 6School of Engineering, European University of Lefke, Lefke, Cyprus
- 7Polytechnic School of Pernambuco, University of Pernambuco (Poli-UPE), Recife, PE, Brazil
- 8Department of Biomedical Engineering, Federal University of Pernambuco, Recife, PE, Brazil
- 9Department of Systems and Computing, Federal University of Campina Grande, Campina Grande, PB, Brazil
- 10Department of Civil, Environmental and Geomatic Engineering, University College London, London, United Kingdom
One of the barriers to performing geospatial surveillance of mosquito occupancy or infestation anywhere in the world is the paucity of primary entomologic survey data geolocated at a residential property level and matched to important risk factor information (e.g., anthropogenic, environmental, and climate) that enables the spatial risk prediction of mosquito occupancy or infestation. Such data are invaluable pieces of information for academics, policy makers, and public health program managers operating in low-resource settings in Africa, Latin America, and Southeast Asia, where mosquitoes are typically endemic. The reality is that such data remain elusive in these low-resource settings and, where available, high-quality data that include both individual and spatial characteristics to inform the geospatial description and risk patterning of infestation remain rare. There are many online sources of open-source spatial data that are reliable and can be used to address such data paucity in this context. Therefore, the aims of this article are threefold: (1) to highlight where these reliable open-source data can be acquired and how they can be used as risk factors for making spatial predictions for mosquito occupancy in general; (2) to use Brazil as a case study to demonstrate how these datasets can be combined to predict the presence of arboviruses through the use of ecological niche modeling using the maximum entropy algorithm; and (3) to discuss the benefits of using bespoke applications beyond these open-source online data sources, demonstrating for how they can be the new “gold-standard” approach for gathering primary entomologic survey data. The scope of this article was mainly limited to a Brazilian context because it builds on an existing partnership with academics and stakeholders from environmental surveillance agencies in the states of Pernambuco and Paraiba. The analysis presented in this article was also limited to a specific mosquito species, i.e., Aedes aegypti, due to its endemic status in Brazil.
1 Introduction
In an age when global viruses such as COVID-19 are an urgent public health priority for research, overshadowing vector-borne diseases, the World Health Organization (WHO) has emphasized the critical need for continued efforts to prevent the transmission of vector-borne diseases, such as malaria, dengue or Zika, which are spread by mosquitoes. While focusing on digital solutions for pandemics, the WHO has implored the global community to not relent nor allow the current pandemic to eclipse the global agenda of reducing the burden of vector-borne diseases (1).
In the context of vector-borne disease surveillance and research, residential entomologic survey data are essential for understanding the geographical and temporal variability in mosquito occupancy in residential locations. Information gathered from entomologic surveys can be used to formulate control strategies for combating mosquito populations effectively. The spatially precise and fine-scale data collected under these surveys are one of the most sought-after pieces of information by health researchers and key policy makers in the field of overlooked tropical disease epidemiology. Such data can be utilized for supporting the decision-making process when determining which high-priority areas are in need of an intervention (i.e., mosquito/larvicidal campaigns or bed nets) (2). From a Global South perspective, although many surveys have been conducted (which have been diligently documented in notable open source websites, e.g., the Malaria Atlas Project (3) and the Global Aedes Aegypti & Albopictus Compendium (4)), such residential property-level survey data with information on physical and environmental characteristics are hard to come by. Such data in most cases remain inaccessible to health researchers, policy makers, and public health program managers. This problem of data paucity is due to the lack of a systematic approach for standardizing the collation of information into a digital format that was initially recorded on paper. The timely entry of data into an electronic registry is a challenge, and this issue is especially true for many low-resource settings in countries in sub-Saharan Africa (5–7) and Latin America (8) which is a hinderance in developing a more satisfactory dashboard for infectious disease surveillance.
Mosquitoes are sensitive to changes in environment and climate that impact their movement potential and survivability. In addition, the abundance of surface water in the form of a stagnated reservoir can have a positive or adverse effect on breeding habits and populations. These serve as risk factors that can have either a positive or negative influence on mosquito abundance in an environment. For example, many cities in the northeastern region of Brazil were hit hard by the Zika virus outbreak in 2015 (9–11). Zika virus, an arboviral infection, is transmitted by the Aedes mosquito genus (i.e., via two common species known as the Aedes aegypti and Aedes albopictus), which are endemic to that region. Their increased abundance in the northeast of Brazil is typically associated with standing water, which serves as a reservoir hotspot for breeding. Apart from the presence of standing water in human dwellings, a restricted set of climatic conditions such as land-surface temperature, humidity, precipitation, and seasonality, in addition to area-level socioeconomic deprivation risk factors, interact with each other to create an environment that is tenable for the mosquito’s survival (12).
To predict the spatial and spatiotemporal distribution of illnesses such as dengue, Zika, and chikungunya, in two northeasten Brazilian cities, Recife and Campina Grande, local environmental health authorities routinely carry out surveys on a bimestrial basis. The community health workers (CHWs) from these cities are deployed to high-risk neighborhoods to visit residential properties to inspect seven different types of breeding sources to detect the presence of the Aedes mosquito and its larvae (13–17). The CHWs collect other important information that describes the property’s physical characteristics (e.g., type of building structure and presence of a garden) and waste management practices (e.g., mode of waste disposal, presence of landfill, etc.) that contribute to the proliferation of mosquitoes (18, 19). A key challenge faced by these CHWs is the use of paper-based tools to document “thousands upon thousands” of pages of entomological information collected directly from the field. The data recorded on the paper forms must then be manually input into an electronic database by the CHWs; and ultimately increases the risk of passing incomplete data to policy makers and public health managers. This problem occurs in Recife and Campina Grande and was addressed by Aldosery et al. (18), who have developed a system for handling primary data with state-of-the-art bespoke smartphone applications (18). Considering these aforementioned issues (i.e., paucity of survey data due to how they are collected at the residential premises level), the CHWs are faced with challenges of linking primary information with other broader secondary sources of data that may contain wider indicators for water sanitation and hygiene (WASH) and weather parameters which may contribute to mosquito occupancy in residential premises (4, 20, 21).
A significant number of highly reliable open-source datasets are available. These open-source data can be linked to spatially referenced survey records to augment the mosquito surveillance effort. The authors argue that these sources remain elusive to many researchers in this line of research. Therefore, the primary objectives of this research article are threefold: (1) to point out to readers where these reliable open-source data can be acquired and explain how they can be used as risk factors for making spatial predictions for mosquito occupancy in general; (2) to use Brazil as a case study to demonstrate how these datasets can be brought together to predict the presence of arboviruses through ecological niche modeling using the maximum entropy algorithm; and (3) to discuss the benefits of using bespoke technologies (smartphone applications, the Internet of Things, etc.), and to explain how these can be the new “gold-standard” approach for gathering primary entomologic survey data. The scope of this paper was limited to a Brazilian context because the research builds on an existing partnership with academics and stakeholders from environmental surveillance agencies in Recife (State of Pernambuco) and Campina Grande (State of Paraiba). We also chose the Aedes genus as the mosquito of focus due to its endemic status in Brazil and restricted the analysis specifically to the Aedes aegypti mosquito, since it is more commonly found than Aedes albopictus. Nevertheless, this article was written to build capacity for and awareness of data sources and methods, and thus it is applicable to different mosquito species and other areas in the Global South with similar circumstances.
2 Description of data sources
In this section, we highlight the various sources of data that can be obtained online. We have provided a detailed description of how to use the data for causal inference and predictive analytics for mosquito occupancy. This included shapefiles for countries as well as point and raster grids for weather and environmental data, respectively. For raster data, we particularly highlighted the most reliable and updated sources available at a high spatial resolution.
2.1 Obtaining spatial boundaries for study areas from GADM
Shapefiles can be obtained from the Global Administrative Areas Database (GADM) (https://gadm.org/index.html). The GADM is a high-resolution database that contains information on administrative areas for all countries, at all sub-divisional levels (e.g., national, state, municipal, district, and sub-district levels), and is freely accessible for research (22). For example, the shapefiles for Brazil (see https://gadm.org/download_country.html) are available at four levels (see Figure 1):
● Level 0: the country’s border (“gadm36_BRA_0.shp”)
● Level 1: boundaries for the 27 states (“gadm36_BRA_1.shp”)
● Level 2: boundaries for the 5,504 municipalities (“gadm36_BRA_2.shp”)
● Level 3: boundaries for the 10,195 districts (“gadm36_BRA_3.shp”).
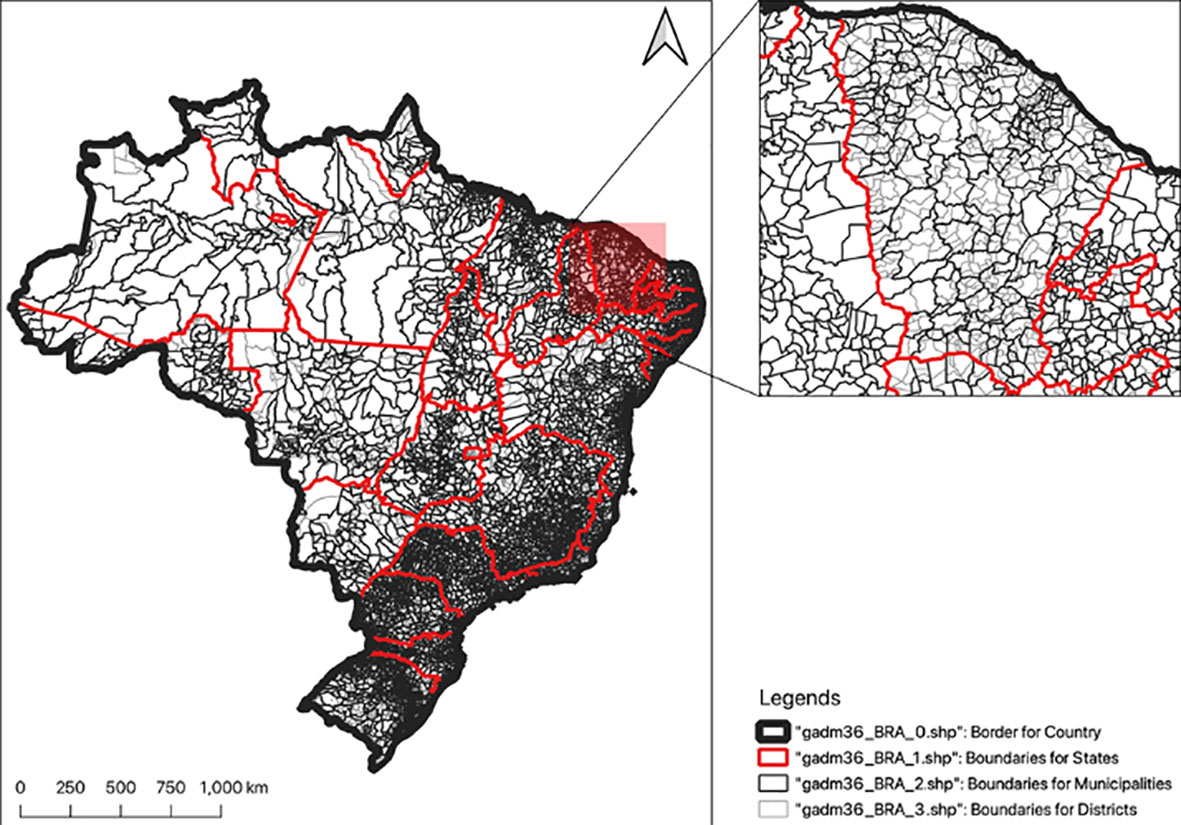
Figure 1 Shapefile (available from https://gadm.org/index.html) plotting the spatial configuration of Brazil. The administrative levels of Brazil are divided into four (0, 1, 2, and 3); however, for certain countries (especially those classified as low- or middle-income countries) the breakdown of administrative levels may differ.
2.2 Obtaining various environmental data
2.2.1 Vegetation cover from the USGS Earth Explorer
Vegetation cover is one of the prominent risk factors for mosquito-borne transmission. For some species, it provides a suitable condition for its movement potential and survivability. One important metric that is often used in the prediction of vector-borne diseases is the Normalized Difference Vegetation Index (NDVI), which is easy to derive on a raster grid (23). This metric describes whether a gridded value in a geographic space contains high or low levels of vegetation. An excellent source is the USGS Earth Explorer (https://earthexplorer.usgs.gov/), which provides users with access to several selectable aerial satellite images (e.g., via Landsat, Sentinel-2, MODIS, and radar instruments) that can be downloaded and cropped according to the spatial extent and temporal resolution of the study area of interest. The images are downloaded as bands ranging from 1 to 12. To derive the NDVI as a gridded layer, one can use the satellite data that correspond to band 4 (i.e., red) and band 5 [i.e., near infrared (NIR)]. The NDVI indices are generated as a raster image by taking the image of bands 4 (red) and 5 (NIR) using the formula (NIR - RED)/(NIR + RED). The length of the pixel (i.e., grid cell) is derived at 90.0 m, whereby each pixel contains an estimate that refers to the intensity of vegetation at a given location. A higher value shows that the presence of vegetation at a location is greater and vice versa. Readers should note that the USGS Earth Explorer has already packaged the NDVI data into several products [i.e., MOD13A1 (500 m), MOD13Q1 (250 m), MYD13A1 (500 m), and MYD13Q1 (250 m)], which are hosted on the Google Earth Engine (https://earthengine.google.com). With bespoke Python code, the NDVI data can be extracted through Google Earth Engine’s code editor (see section on data availability).
2.2.2 Obtaining land-surface elevation data from the STRM Digital Elevation Database
Elevation is often used as an important predictor for determining environmental suitability for mosquito abundance. High-altitude areas (i.e., those averaging above 1,200 m) adversely affect survival rates for most mosquitoes (24–26). The land-surface elevation layer can be obtained from the STRM 90.0 m DEM Digital Elevation Database (https://srtm.csi.cgiar.org): it is possible to select and download the tiled raster that contains land-surface elevation estimates for the study area. The user can crop (or “cookie cut”) the tile to the spatial extents of the study area of interest. The resolution for the layer is 90.0 m, where a grid cell value contains a positive (or negative) continuous measurement in meters to reflect the height of the land’s surface above (or below) sea level.
2.2.3 Obtaining aridity data from the GAI-PECD database
The Global Aridity Index & Potential Evapotranspiration Climate Database (GAI-PECD) (version 2) (see https://cgiarcsi.community/2019/01/24/global-aridity-index-and-potential-evapotranspiration-climate-database-v2/) provides high-resolution (approximately 1 km) global raster climate information for levels of environmental dryness, measured as the Aridity Index (AI) (27). Aridity is a significant environmental risk factor for determining environmental suitability for mosquito survivability (28, 29). Mosquitoes are unable to survive in harsh areas that are hyper-arid or arid and thus are completely absent in such environments; however, they can thrive in semi-arid and dry subhumid environments. The raster contains numerically derived estimates for AI (ranging from 0.0 to 0.65) describing the degree of dryness of the climate at a given location. The raster values for AI can be reclassified accordingly to four dryland subtypes: < 0.05 (hyper-arid), 0.06 to 0.20 (arid), 0.21 to 0.50 (semi-arid), and 0.51 to 0.65 (dry subhumid). Since mosquitoes cannot survive in hyper-arid and arid areas (i.e., AI 0.20), it is possible to limit the area of analysis to semi-arid and dry subhumid areas (i.e., AI > 0.20) and create a binary raster constraining the analysis to areas where they will survive (i.e., AI > 0.20).
2.3 Obtaining various anthropogenic data from WorldPop.org
An ensemble of several anthropogenic-related risk factors stored as gridded data at a high-resolution of 100 m can be accessed from Worldpop.org (https://www.worldpop.org/). WorldPop.org is an open-source spatial demographic database.
2.3.1 Built settlements
Several studies have demonstrated that the degree of urbanization in a study area is correlated with a significantly increased risk of mosquito occupancy, as urbanization inadvertently yields breeding sites within human dwellings (30, 31). The built settlements raster layer can therefore be used to model the risk of infestation. This raster layer contains binary information that defines an area as either an urban (1) or non-urban (0) location. These data can be implemented in the spatial analysis for mosquito surveillance in two ways. First, for point analysis, the data can be used to classify point features (e.g., communities, villages, and points of individual houses or residential premises) as “urban” or “non-urban” through simple overlays and pixel extraction to spatial points. Second, for area-level spatial analysis, one can calculate the fraction of surface defined to be urban or non-urban. For each country, WorldPop.org has mapped the trajectory of how built settlements have expanded over the years (32), and these raster data are available from 2010 to 2020.
2.3.2 Population density
Human population characteristics are an important feature to account for in the modeling of mosquito-borne transmission (33). The population density raster can be used to estimate counts of inhabitants at point locations. For areal analysis, these grids can be aggregated within a boundary to derive an estimate for the total number of inhabitants in an area, which is useful, as a denominator is necessary to obtain measures of disease (or infestation) frequency (e.g., prevalence or incidence rates). WorldPop.org provides a large number of raster layers that all contain discrete values that represent the estimated number of inhabitants within a given pixel and are available for many countries in the Global South from 2010 to 2020. The resource also provides raster data that are gender- and age-group-specific, which is very useful for deriving age- and sex-adjusted estimates. The details of how these layers were created are explained by Lloyd et al. (34).
2.3.3 Night-time lighting of areas
Worldpop.org (https://www.worldpop.org/) provides resampled gridded data to show the intensity and detection of non-natural lighting on the Earth’s surface to signify the presence of anthropogenic activity, or land occupied by human settlements. Artificial lighting is an important risk factor to account for in the prediction of mosquito occupancy for two reasons: (1) as shown in a recent review, there is a growing body of literature indicating that it significantly impacts mosquito feeding behavior (i.e., mosquitoes have a preference for feeding during the night, when non-natural lighting is pronounced) (35); and (2) in the Global South, especially in sub-Saharan Africa, extensive lighting is a strong indicator of a city’s economic and structural development. Therefore, these data can be modeled as a direct risk factor; alternatively, they can be used to generate a composite for socioeconomic deprivation (36). The spatial resolution for this dataset is 100 m by 100 m.
2.4 Weather variables
Land surface temperature, humidity, and rainfall are typical weather-related risk factors that must be taken into consideration for the spatial prediction of breeding hotspots for mosquitoes, irrespective of species. The joint contribution of climatic variables plays an immense role in creating an environment that is suitable for the mosquitoes’ survivability and for its breeding and feeding habits. A group of such climatic risk factors stored as high-resolution gridded data can be accessed from several sources. Here, we describe two prominent sources: WorldClim and OpenWeatherMap API.
2.4.1 Obtaining weather-related information from WorldClim
WorldClim (https://www.worldclim.org) is a comprehensive spatial database containing high-resolution weather and climate data on a global scale (37, 38). It provides two datasets. First, it provides historical monthly weather data from 1960 to 2018, specifically for the following climate variables: minimum temperature (°C), maximum temperature (°C) (which can be recalculated to obtain either a median or mean temperature), and total precipitation (mm). It should be noted that the highest spatial resolution for these data is 2.5′ (approximately 4.5 km). Downloading the parameters will produce a highly compressed zip file containing several GeoTiff (.tif) files (i.e., the raster) for each month of the year (where January is 1 and December is 12) for a 10-year period. Second, WorldClim provides projected monthly estimates for climate data for the time periods 2021–2040, 2040–2060, 2061–2080, and 2081–2100 at four different spatial resolutions [30″ (approximately 900 m), 2.5′ (approximately 4.5 km), 5′ (approximately 9 km), and 10′ (approximately 18 km)]. The projected version provides monthly values of minimum temperature (°C), maximum temperature (°C), precipitation (mm), and 19 other bioclimatic variables, which were all derived from 23 climate models.
2.4.2 Obtaining weather-related data from the OpenWeatherMap API
Data can be downloaded from an online meteorological service called the OpenWeatherMap application program interface (API) (https://openweathermap.org/api). It provides an API with JSON endpoints to make free and unlimited calls for extracting weather values (i.e., for temperature, relative humidity (%), pressure, cloud cover, and weather description) that are current estimates; users can also extract projected estimates or “short-term” 3-hourly forecasts stretching up to 5 days in the future, which is a useful feature if the user wants to incorporate data for predicting mosquito occupancies over a short period. It should be noted that extracting data from this source is challenging. One must first register to gain access to an API key, then set up a “scheduled” extraction script to extract the current analysis and 3-hourly forecasts (for up to the next 5 days) at the selected location (i.e., using the GPS centroids of cities) via OpenWeatherMap (the information is compiled in a JSON file). This can be done via a local server (i.e., personal computer) using a crontab (https://crontab.guru/every-5-minutes) or preferably on a cloud-based online server such as the MongoDB (https://www.mongodb.com). The data extraction is performed through the following API address, with the given API key provided by the OpenWeatherMap service (the selected city’s ID number is inserted in the “ID” part of the API address): http://api.openweathermap.org/data/2.5/forecast?id=ID&APPID=KEY. It should be noted that this resource provides weather measurements only at a city level. For example, suppose we wanted to extract the weather data for Recife and Campina Grande (in Brazil) (39): we can perform this action by using the API key provided by the OpenWeatherMap API services, and then setting the API to the selected cities’ IDs by inserting the values of 3390760 (i.e., Recife, longitude –34.8811 and latitude –8.0539) and 3403642 (i.e., Campina Grande, longitude –35.8811 and latitude –7.2306) into the above link through a timed recursive loop to continuously compile the records into a local server or into a cloud-based online platform. Details of this resource were explained extensively by Musah et al. (39).
3 Materials and methods
In this section, we describe the implementation of a population-based ecological study design using spatially referenced point survey records on presence-only mosquito data, using Brazil as a case study for this demonstration. We will discuss the implementation of the maximum entropy model (MAXENT) for predicting the probability of mosquito occurrence across the whole of Brazil while accounting for other environmental attributes that impact mosquito habitats.
3.1 Data extraction from the global compendium of Aedes aegypti and Albopictus occurrence
The global compendium of the Aedes species is an open source database that is accessible via the Global Biodiversity Information Facility (GBIF) (https://www.gbif.org) (4). For this demonstration, we have restricted the analysis to the Aedes aegypti species points in Brazil. This file contains a grand total of 19,929 spatially referenced occurrence points across the world. Brazil has 5,057 survey points spanning from 1979 to 2013 that contribute to this database. The majority of the survey points for Brazil were documented in 2013 (4,410; 87%), while the remaining survey points (i.e., 594; 12%) were unevenly spread across 1979 to 2011, with 53 survey points having missing information for the year. Therefore, to determine the possible distribution of the Aedes aegypti species in Brazil, a total of 4,410 occurrence locations for the Aedes aegypti species were extracted from this database for the year 2013 only.
3.2 Study design
A country-scale ecological study design within a cross-sectional framework was used on 2013 data to retrospectively determine the following outcomes: (1) the probability of the Aedes aegypti species being present at a location in Brazil; (2) the likely areas that are environmentally suitable for Aedes aegypti; and (3) the set of restricted variables (i.e., temperature, precipitation, natural lighting, urbanization, NDVI, population density, and land surface elevation) that yields the highest contribution to mosquito occurrence prediction in a Brazilian context.
3.2.1 Gridded environmental variables
As described in section 3.2, seven predictor variables, of which two are climate related (annual temperature and precipitation in 2013), three describe the physical environment (averaged NDVI and natural lighting in 2013, and land surface elevation), and the remaining two describe the anthropogenic conditions (i.e., overall population density and urbanization, both measured for 2013), constituted the gridded data used as risk factors for mosquito occupancy. These raster grids were combined accordingly into a single multiband raster object with dimensions of 4.5 km by 4.5 km resolution to enable the following actions needed for the analysis: (1) the extraction of all environmental raster values from all seven variables onto the occurrence and absence points (see section 3.2.2); and (2) the feeding of the entire multiband raster object into the MAXENT model after it is trained for the country-scale estimation and spatial prediction for Aedes aegypti occupancy in Brazil.
3.2.2 Statistical analysis using the maximum entropy algorithm (MAXENT)
The maximum entropy algorithm is a classification algorithm that falls under the umbrella of ecological niche models, which are used to estimate the relationship between species records at sites and the environmental and spatial characteristics of those sites (40). In other words, these are distributional models that use occurrence point data in conjunction with environmental data to make a correlative model of the environmental conditions that meet an outcome’s environmental (or ecological) requirements, which, in turn, can infer zones for the relative suitability (or predictability) of an outcome. They have many applications in ecology, epidemiology, and disaster risk reduction and have been widely used for country-scale mapping for determining habitat suitability for the Aedes species in South America (41–43).
As described in section 3.1, 4,410 location data points for Aedes aegypti in 2013 in Brazil were compiled and used as presence points. Background data for twice the number of the presence points (i.e., 8,820) were generated within the extent of the study area to serve as proxy locations for pseudo-absences of Aedes aegypti. The presence and pseudo-absence points were rendered into a binary indicator that takes a Bernoulli function to model probabilities in geographic space. It should be noted that all 4,410 occurrence points were coded as 1 to signify the presence of Aedes aegypti, while the assumed background points (i.e., pseudo-absences) were coded as 0 to signify the absence of Aedes aegypti (40) (Figure 2). These points were used to extract all environmental raster values as described in section 3.2.1.
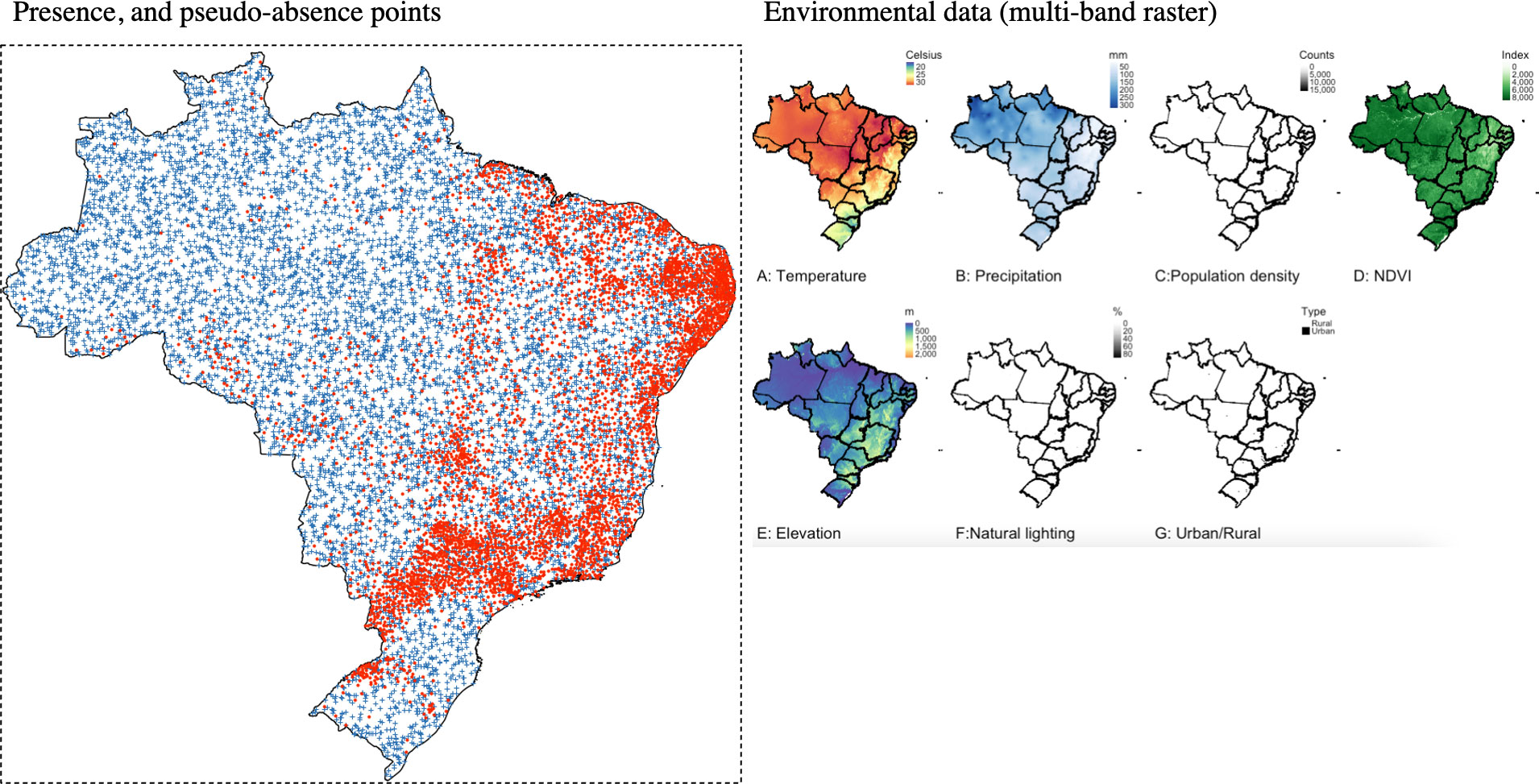
Figure 2 The right panel shows point locations with a known presence (red dots) of Aedes aegypti in 2013 in Brazil, whereas the blue crosses correspond to pseudo-absence points. The left panel shows the following Brazilian covariate data measured for 2013: (A) annual temperature; (B) annual precipitation; (C) population density; (D) NDVI; (E) land surface elevation; (F) natural lighting; and (G) urban/rural classification.
Before constructing the predictive model, we performed a fourfold cross-validation analysis by randomly withholding 25% of the presence and pseudo-absence locations as test data, and retaining the remaining 75% as training data for mapping the predictions. This meant that the model was fitted four times while withholding a separate quarter of the data – each cross-validation would churn a key indicator that was averaged to allow for overall model validation, i.e., the area under the curve (AUC) and maximum true-positive rate and true-negative rate (max TPR + TNR). AUC is an indicator of model performance where higher values indicate greater accuracy in our predictions; an AUC value of 0.5 is a common cut-off point used for assessing model performance. Hence, an AUC value of 0.5 or lower is an indication of our predictions being unreliable, while values above 0.5 and toward 1.0 indicate that our predictions are more reliable and accurate. Max TPR + TNR denotes the probability threshold at which our model maximizes the TPR and the TNR for correctly classifying a grid cell as a presence feature. It is generally accepted that this is the optimum value at which to set the threshold for the binary classification of a grid cell and the predicted probability is a reflection of the level of certainty of the classification that was mapped. We used the max TPR + TNR threshold to reclassify the region’s predicted probabilities accordingly as “suitable” and “not suitable”, whereby any value above max TPR + TNR was deemed as environmentally suitable for the Aedes species and vice versa.
All statistical analysis, including GIS mapping and MAXENT modeling, was performed in RStudio (version 2022.07.1 Build 554). All datasets along with scripts were provided for reproducible research (see section on data availability).
4 Results
4.1 Mapping the predicted probabilities and suitability regions for Brazil
After performing the fourfold cross-validation analysis, we found that the overall AUC estimate was 0.8376 (83.76%), which was obtained after averaging AUC-specific estimates for each cross-validation, i.e., 0.8435 (84.35%), 0.8403 (84.03%), 0.8333 (83.33%), and 0.8331 (83.31%). This value is greater than 0.5, thus indicating the model’s predictive reliability (Figure 3).
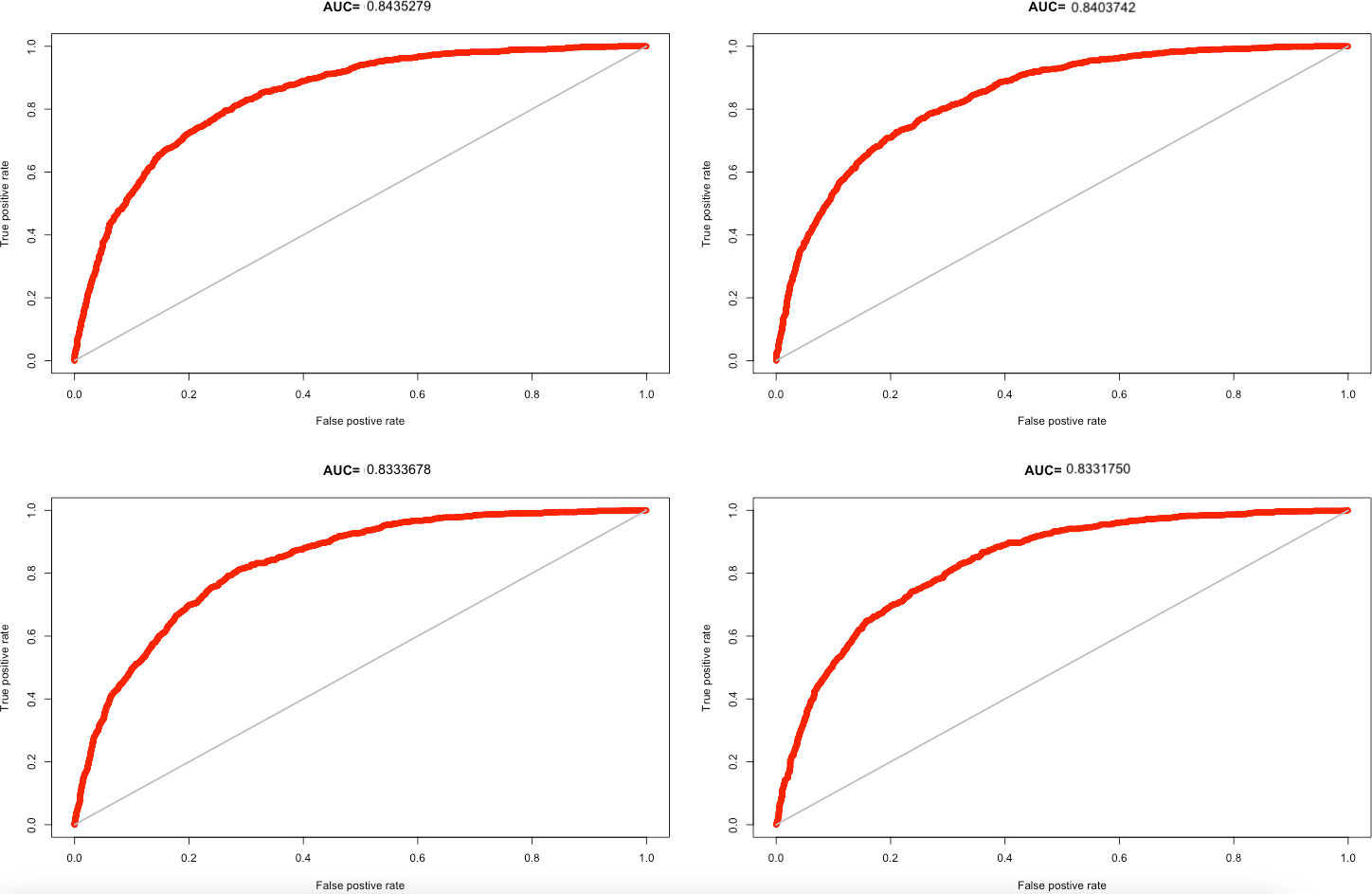
Figure 3 AUC curves from fourfold cross-validation analysis; the four estimates were averaged to 0.8376 (> 0.5) with a max TPR + TNR of 0.4953.
The optimal threshold (i.e., max TPR + TNR) at which the MAXENT model was able to correctly classify a grid cell as a presence feature for mosquito occupancy was 0.4953. Hence, we will use a predicted probability threshold of 0.4953 to reclassify areas as suitable for mosquito occupancy (Figure 4). The expected outputs are shown in Figure 4: the left panel (A) shows the predicted probability distribution of mosquito occupancy for the Aedes aegypti species throughout Brazil, retrospectively, in 2013, while the right panel (B) shows the delineated areas where they are more likely to thrive.
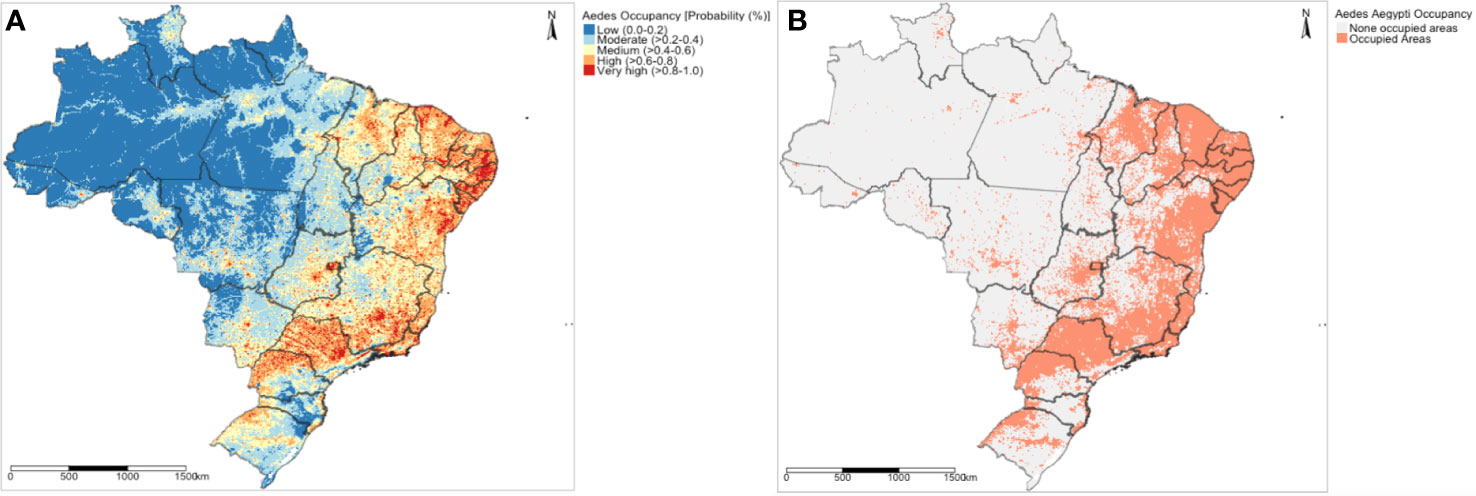
Figure 4 MAXENT modeling results showing (A) the predicted probability map of mosquito (Aedes aegypti) occupancy and (B) the suitability map based on the max TPR + TNR to illustrate where Aedes aegypti will thrive in Brazil.
The predictions were adjusted with seven different environmental covariates (Figure 3); here, we report the overall variable contributions. The population density, an anthropogenic indicator, has the highest contribution to the prediction, estimated at 75.75%, followed by natural lighting (10.29%), precipitation (6.79%), NDVI (4.18%), temperature (2.55%), land surface elevation (0.421%), and urbanization (negligible; < 0.0001%).
5 Discussion
In this article, we described a broad range of open data sources that can be harnessed for the spatial prediction of mosquito populations. We used the whole of Brazil as a motivational case study to demonstrate how these datasets can be brought together for predicting the intensity of mosquito occupancy for Aedes aegypti in a data-sparse context using the MAXENT algorithm, which showed that population density, natural lighting, and precipitation made the biggest contributions to mosquito occupancy. While this approach was rigorous and should be used when data remains elusive, the author(s) concede that there are flaws in this approach. First, the research design of this case study was retrospective, using open entomologic data that were mostly available for 2013. The predictions shown in Figure 3 are not at all representative of the current climate situation when this research article was written (i.e., 2023). However, it would be possible to use the projected climate and population-based data for 2023 (which could have been done here, but this article is simply a demonstration), which could be fed into our trained MAXENT model and would have produced the predicted probability values for a future scenario. Second, the study design itself relies upon an ecological study design within a retrospective cross-sectional framework. In this study, the data used were a combination of both point and gridded information that is at a high geographic resolution but not at an individual level, e.g., at the level of household or property. This meant that the interpretation of the predictions needed to be done with the ecological fallacy in mind. These biases limit the research’s ability to achieve both internal and external validity. To combat these biases, we argue the case for using bespoke applications for acquiring accurate entomologic data.
Our case study demonstrated the combining of open-source data to crudely map areas of mosquito habitat suitability, analytically and in an unsupervised scenario (and where data paucity is an issue). However, the authors stand by the opinion that point-level mosquito surveillance data that recorded observations at either a residential premise or property level would be the “gold standard” approach for collecting primary data at a granular resolution. This provides ample opportunity to collect more detailed information describing property characteristics that promote infestation, which was absent from our case study. In addition, it provides point-level data used in point-process models for making spatial predictions regarding infestation burden, which in turn can be integrated into an early warning system for outbreaks (44–46). We propose that the use of smartphone applications that are developed for the main purpose of collecting surveillance data to show the infestation risk and at the same time the geographic burden of such infestations is the best way to support vector control campaigns. There is now a shift toward using such applications for this purpose, especially in Central and South America, with three examples given here. First, VectorPoint is an excellent mobile surveillance application for reporting Chagas disease and infestations in Arequipa, Peru (47). The application provides a risk map based on data collected during fieldwork. Second, Chaak is a smartphone-based application system interlinked with a dashboard (for managers) dedicated to mosquito-borne disease surveillance that captures data related to the immature stages of dengue virus mosquito vectors in Mérida, Mexico (48). Third, VazaDengue is akin to VectorPoint and Chaak; however, it is only a smartphone-based system that integrates social media with citizen science to guide surveillance agents in controlling mosquitoes (49).
The authors have developed a robust surveillance system, which is cloud based, that improves the surveillance of mosquitoes by providing timely and geolocated reports regarding the presence and absence of mosquitoes in properties along with other entomological characteristics (i.e., eggs or larvae) in north-east Brazilian cities, limited specifically to Recife and Campina Grande. This was done by taking everything the CHWs use in their mosquito control campaign [i.e., surveillance reporting cards (data collection sheets) and the spatial configuration of block areas as scanned maps] and digitizing them into a format supported by the MEWAR application (a full description of its development is provided by Aldosery et al. (18) (see Figure 5). The application seeks to collect general parameters linked to infestation, ranging from spatial information to property-level characteristics such as land use, waste disposal practices, and key entomologic infestation and treatment indicators (see Table 1).
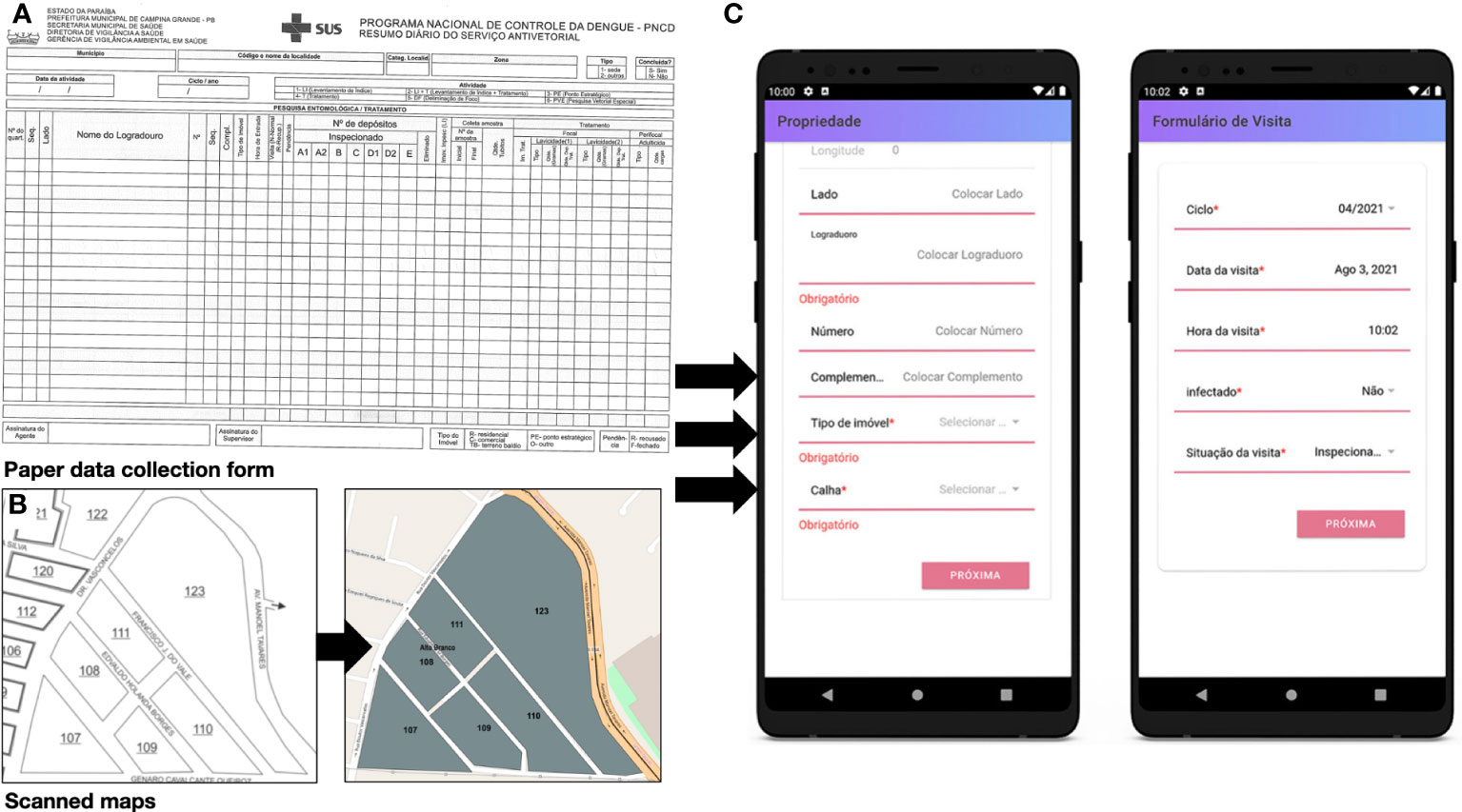
Figure 5 The conversion of surveillance data and maps (using a paper-based system for data collection) to a format that supports data collection with mobile phones. (A) Original surveillance card on which all property, spatial and entomologic data are recorded (this card is used by the control agency in Campina Grande). (B) On the left is a scanned map used by CHWs to gauge which block areas to attend and visit resident properties in, and on the right is a digitized version of the scanned map for extracting block data to be incorporated into the app. (C) Prototype version of mobile application (source image from Aldosery et al. (18).
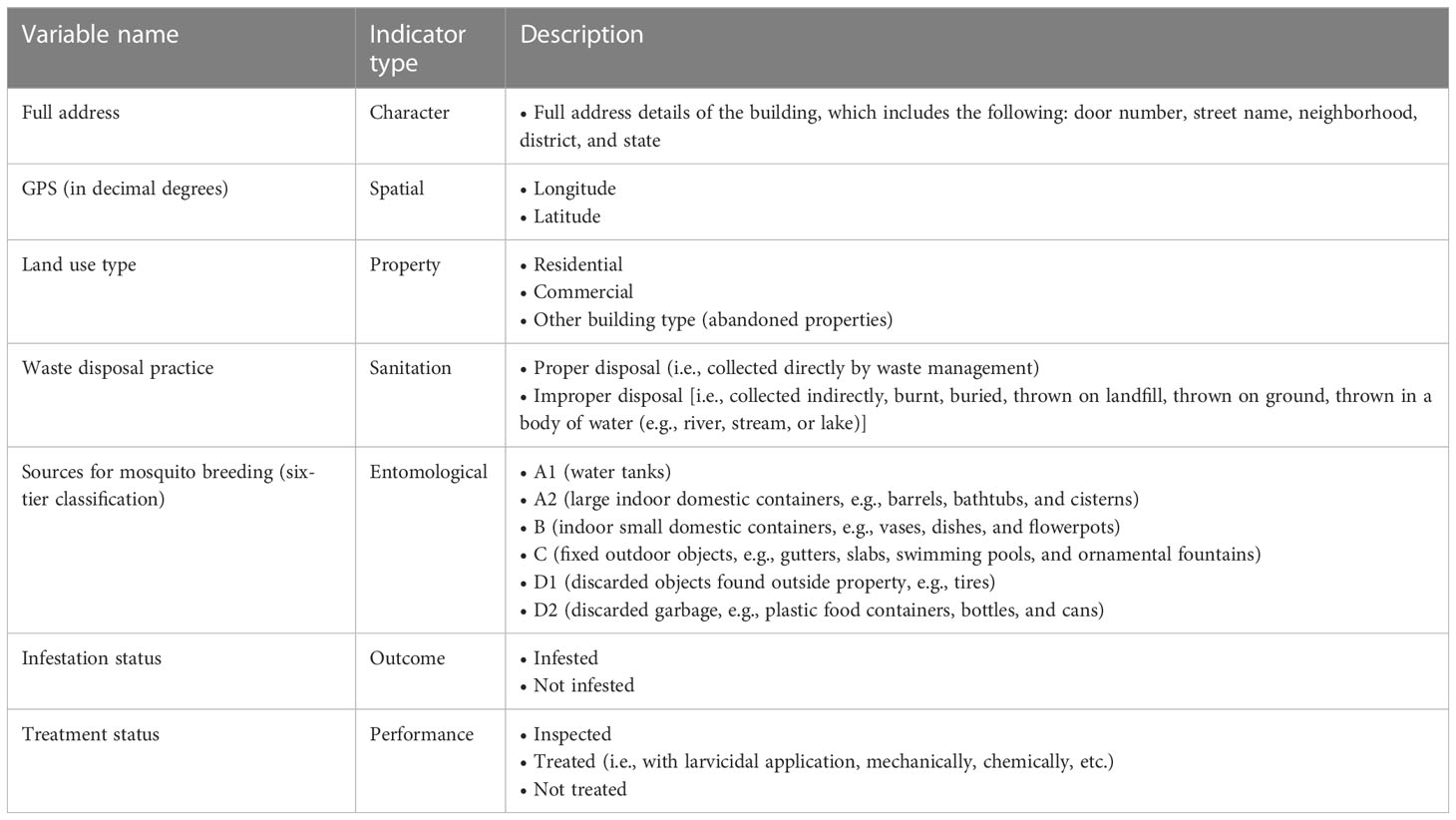
Table 1 General summary of the type of information collected through the MEWAR smartphone application mapped from Environmental Health Agents (EHA) from Recife and Campina Grande (see details in Aldosery et al. (18)).
The list of data sources that are raster based is malleable for area-level analysis—most mosquito surveillance data tend to be released at an area level; hence, these pixels can be aggregated within areal boundaries and matched to observed units to be used as risk factors. Appropriate rasters that are good candidates for aggregation include population density, climate data from WorldClim, and aridity—these can produce proxy measures for area units such as totals and other useful summary statistics (medians, averages, etc.) that can be implemented in a variety of spatial risk models at an area level [notable examples of spatial models for risk prediction include Bayesian hierarchical modeling (46, 50, 51) and machine learning (e.g., boosted regressions (52)]. Users rendering their data to this level and implementing this kind of approach should keep in mind the various biases that can occur when reporting results, and hence a pronounced bias, such as the ecological fallacy, should therefore be considered carefully. However, the use of individual-level data (i.e., residential premises) collected through primary surveys can be augmented with the open-source environmental spatial data highlighted in this article by linking their GPS coordinates by using a spatial join, overlapping the raster pixels with the surveillance points and assigning the raster values to the survey points to facilitate a much more precise and granular modeling approach. This would be invaluable, as the point-process approach for mapping is the gold standard for making spatial risk predictions for households, and the results can be further interpolated over a grid for risk coverage. There are many models that enable this kind of analysis—notable examples include Bayesian modeling frameworks such as stochastic partial differential equations using integrated nested Laplace approximations (SPDE-INLA) (53–55). These are valuable options for risk prediction and creating an early warning system for mosquito outbreaks; whereby such information can be fed into a dashboard to provide digital solutions for surveillance manager and policy makers (56, 57).
6 Conclusion
To conclude, we have identified a broad range of open-source data sources that can be harnessed as risk factors for the spatial prediction of mosquito occupancy or infestation, and we have demonstrated in a reproducible way how they can be brought together and implemented using the MAXENT algorithm within a Brazilian context. We explicitly note that this approach should be utilized within a data-sparse context. However, we also discussed the use of novel bespoke technologies, such as smartphone applications, that should be considered the better method for collecting primary entomologic data, to address the problems of data paucity and avoid potential biases that are typically found in studies using open source datasets—doing so will improve a study’s internal and external validity. This article was written to build capacity for and awareness of various data sources, demonstrating their use with reproducible methods, and thus it is applicable to different mosquito species and other areas in the Global South with similar environmental and socioeconomic conditions.
Data availability statement
The original contributions presented in the study are included in the article. All datasets, script files and instructions for reproducing the analysis shown in this original article are made freely available through our GitHub repository (https://github.com/UCLPG-MSC-SGDS/Data-Sources).
Author contributions
Conceptualization: AM and PK; data management, extraction, and analysis: AM; original draft: AM; supervision and funding acquisition: PK, WPdS, TM, LCC, OY, and TA; revision of manuscript: PK, WPdS, TM, LCC, OY, TA, IVGB, MT, SB, EB, GMMM, ACGdS, CLdL, and AA. All authors contributed to the article and approved the submitted version.
Funding
This research was conducted under the project titled: Mosquito populations modeling for early warning system and rapid public health response (MEWAR). This research was funded by the Belmont Forum, which was supported in the United Kingdom by UKRI NERC under the grant NE/T013664/1, and in Turkey by TÜBİTAK under the grant 119N373. This work was supported in Brazil by FAPESP under the grants 2019/23553–1 and 2020/11567–5. We thank the Coordenação de Aperfeiçoamento de Pessoal de Nível Superior - Brasil (CAPES) - Finance Code 001 for funding the PhD research conducted by authors CL, AS, and IB in Brazil. We take the opportunity to thank the Space and Aeronautics Research Institution (National Center for Satellite Technology, King Abdulaziz City for Science and Technology, Saudi Arabia) for their support in funding the PhD research conducted by author AA in the UK.
Conflict of interest
The authors declare that the research was conducted in the absence of any commercial or financial relationships that could be construed as a potential conflict of interest.
Publisher’s note
All claims expressed in this article are solely those of the authors and do not necessarily represent those of their affiliated organizations, or those of the publisher, the editors and the reviewers. Any product that may be evaluated in this article, or claim that may be made by its manufacturer, is not guaranteed or endorsed by the publisher.
References
1. Seelig F, Bezerra H, Cameron M, Hii J, Hiscox A, Irish S, et al. The COVID-19 pandemic should not derail global vector control efforts. PloS Negl Trop Dis (2020) 14(8):e0008606. doi: 10.1371/journal.pntd.0008606
2. Benelli G. Research in mosquito control: current challenges for a brighter future. Parasitol Res (2015) 114(8):2801–5. doi: 10.1007/s00436-015-4586-9
3. Hay SI, Snow RW. The malaria atlas project: developing global maps of malaria risk. PloS Med (2006) 3(12):e473. doi: 10.1371/journal.pmed.0030473
4. Kraemer MUG, Sinka ME, Duda KA, Mylne A, Shearer FM, Brady OJ, et al. The global compendium of Aedes aegypti and Ae. albopictus occurrence. Sci Data (2015) 2(1):150035. doi: 10.1038/sdata.2015.35
5. Brooker S, Kabatereine NB, Smith JL, Mupfasoni D, Mwanje MT, Ndayishimiye O, et al. An updated atlas of human helminth infections: the example of East Africa. Int J Health Geogr (2009) 8(1):42. doi: 10.1186/1476-072X-8-42
6. Brooker S, Hotez PJ, Bundy DAP. The global atlas of helminth infection: mapping the way forward in neglected tropical disease control. PloS Negl Trop Dis (2010) 4(7):e779. doi: 10.1371/journal.pntd.0000779
7. Hay SI, George DB, Moyes CL, Brownstein JS. Big data opportunities for global infectious disease surveillance. PloS Med (2013) 10(4):e1001413. doi: 10.1371/journal.pmed.1001413
8. Maciel-de-Freitas R, Aguiar R, Bruno RV, Guimarães MC, Lourenço-de-Oliveira R, Sorgine MH, et al. Why do we need alternative tools to control mosquito-borne diseases in Latin America? Mem Inst Oswaldo Cruz (2012) 107:828–9. doi: 10.1590/S0074-02762012000600021
9. Magalhaes T, Braga C, Cordeiro MT, Oliveira ALS, Castanha PMS, Maciel APR, et al. Zika virus displacement by a chikungunya outbreak in recife, Brazil. PloS Negl Trop Dis (2017) 11(11):e0006055. doi: 10.1371/journal.pntd.0006055
10. Lowe R, Barcellos C, Brasil P, Cruz OG, Honório NA, Kuper H, et al. The zika virus epidemic in Brazil: from discovery to future implications. Int J Environ Res Public Health (2018) 15(1):96. doi: 10.3390/ijerph15010096
11. Souza AI, de Siqueira MT, Ferreira ALCG, de Freitas CU, Bezerra ACV, Ribeiro AG, et al. Geography of microcephaly in the zika era: a study of newborn distribution and socio-environmental indicators in recife, Brazil, 2015-2016. Public Health Rep (2018) 133(4):461–71. doi: 10.1177/0033354918777256
12. Tunali M, Radin AA, Başıbüyük S, Musah A, Borges IVG, Yenigun O, et al. A review exploring the overarching burden of zika virus with emphasis on epidemiological case studies from Brazil. Environ Sci pollut Res Int (2021) 28(40):55952–66. doi: 10.1007/s11356-021-15984-y
13. da Silva CC, de Lima CL, da Silva ACG, Moreno GMM, Musah A, Aldosery A, et al. Spatiotemporal forecasting for dengue, chikungunya fever and zika using machine learning and artificial expert committees based on meta-heuristics. Res BioMed Eng (2022) 38:499–537. doi: 10.1007/s42600-022-00202-6
14. de Lima CL, da Silva ACG, da Silva CC, Moreno GMM, da Silva Filho AG, Musah A, et al. Intelligent systems for dengue, chikungunya, and zika temporal and spatio-temporal forecasting: a contribution and a brief review. In: Pani SK, Dash S, dos Santos WP, Chan Bukhari SA, Flammini F, editors. Assessing COVID-19 and other pandemics and epidemics using computational modelling and data analysis. Cham: Springer International Publishing (2022). p. 299–331. doi: 10.1007/978-3-030-79753-9_17
15. Silva CCda, Lima CLde, Silva A CGda, Moreno GMM, Musah A, Aldosery A, et al. Forecasting dengue, chikungunya and zika cases in recife, Brazil: a spatio-temporal approach based on climate conditions, health notifications and machine learning. Res Soc Dev (2021) 10(12):e452101220804–e452101220804. doi: 10.33448/rsd-v10i12.20804
16. Musah A, Rubio-Solis A, Birjovanu G, dos Santos WP, Massoni T, Kostkova P. (2019). Assessing the relationship between various climatic risk factors & mosquito abundance in recife, Brazil. In: DPH'19 Proceedings of the 9th International Conference on Digital Public Health, Marseille, France. (20th to 23rd November 2019). (2019) pp. 97–101. doi: 10.1145/3357729.3357744
17. Rubio-Solis A, Musah A, Dos Santos W, Massoni T, Birjovanu G, Kostkova P. (2019). ZIKA virus: prediction of aedes mosquito larvae occurrence in recife (Brazil) using online extreme learning machine and neural networks. In: DPH'19 Proceedings of the 9th International Conference on Digital Public Health, (20th to 23rd November 2019). Marseille, France: Association for Computing Machinery (2019) pp. 101–10. doi: 10.1145/3357729.3357738
18. Aldosery A, Musah A, Birjovanu G, Moreno G, Boscor A, Dutra L, et al. MEWAR: development of a cross-platform mobile application and web dashboard system for real-time mosquito surveillance in northeast Brazil. Front Public Health (2021) 9:1623. doi: 10.3389/fpubh.2021.754072
19. Beltrán JD, Boscor A, dos Santos WP, Massoni T, Kostkova P. ZIKA: a new system to empower health workers and local communities to improve surveillance protocols by e-learning and to forecast zika virus in real time in Brazil. DH'18: Proceedings of the 2018 International Conference on Digital Health, (Lyon, France) (23rd to 26th April). (2018) pp. 90–4. doi: 10.1145/3194658.3194683
20. Leta S, Beyene TJ, De Clercq EM, Amenu K, Kraemer MUG, Revie CW. Global risk mapping for major diseases transmitted by aedes aegypti and aedes albopictus. Int J Infect Dis (2018) 67:25–35. doi: 10.1016/j.ijid.2017.11.026
21. Sallam MF, Fizer C, Pilant AN, Whung PY. Systematic review: land cover, meteorological, and socioeconomic determinants of aedes mosquito habitat for risk mapping. Int J Environ Res Public Health (2017) 14(10):1230. doi: 10.3390/ijerph14101230
22. Global administrative areas. GADM database of global administrative areas (Version 2.0) (2012). Available at: www.gadm.org.
23. Kofidou M, de Courcy Williams M, Nearchou A, Veletza S, Gemitzi A, Karakasiliotis I. Applying remotely sensed environmental information to model mosquito populations. Sustainability (2021) 13(14):7655. doi: 10.3390/su13147655
24. Attaway DF, Jacobsen KH, Falconer A, Manca G, Waters NM. Risk analysis for dengue suitability in Africa using the ArcGIS predictive analysis tools (PA tools). Acta Trop (2016) 158:248–57. doi: 10.1016/j.actatropica.2016.02.018
25. Asigau S, Hartman DA, Higashiguchi JM, Parker PG. The distribution of mosquitoes across an altitudinal gradient in the Galapagos islands. J Vector Ecol (2017) 42(2):243–53. doi: 10.1111/jvec.12264
26. Attaway DF, Jacobsen KH, Falconer A, Manca G, Rosenshein Bennett L, Waters NM. Mosquito habitat and dengue risk potential in Kenya: alternative methods to traditional risk mapping techniques. Geospatial Health (2014) 9(1):119–30. doi: 10.4081/gh.2014.10
27. Global aridity index and potential evapotranspiration (ET0) climate database v2. figshare (2019). Available at: https://figshare.com/articles/dataset/Global_Aridity_Index_and_Potential_Evapotranspiration_ET0_Climate_Database_v2/7504448/3.
28. Paz S. Effects of climate change on vector-borne diseases: an updated focus on West Nile virus in humans. Emerg Top Life Sci (2019) 3(2):143–52. doi: 10.1042/ETLS20180124
29. Sinka ME, Golding N, Massey NC, Wiebe A, Huang Z, Hay SI, et al. Modelling the relative abundance of the primary African vectors of malaria before and after the implementation of indoor, insecticide-based vector control. Malar J (2016) 15(1):142. doi: 10.1186/s12936-016-1187-8
30. Kolimenakis A, Heinz S, Wilson ML, Winkler V, Yakob L, Michaelakis A, et al. The role of urbanisation in the spread of aedes mosquitoes and the diseases they transmit–a systematic review. PloS Negl Trop Dis (2021) 15(9):e0009631. doi: 10.1371/journal.pntd.0009631
31. Čabanová V, Miterpáková M, Valentová D, Blažejová H, Rudolf I, Stloukal E, et al. Urbanization impact on mosquito community and the transmission potential of filarial infection in central Europe. Parasit Vectors (2018) 11(1):261. doi: 10.1186/s13071-018-2845-1
32. Nieves JJ, Sorichetta A, Linard C, Bondarenko M, Steele JE, Stevens FR, et al. Annually modelling built-settlements between remotely-sensed observations using relative changes in subnational populations and lights at night. Comput Environ Urban Syst (2020) 80:101444. doi: 10.1016/j.compenvurbsys.2019.101444
33. Shaw WR, Catteruccia F. Vector biology meets disease control: using basic research to fight vector-borne diseases. Nat Microbiol (2019) 4(1):20–34. doi: 10.1038/s41564-018-0214-7
34. Lloyd CT, Sorichetta A, Tatem AJ. High resolution global gridded data for use in population studies. Sci Data (2017) 4(1):170001. doi: 10.1038/sdata.2017.1
35. Barghini A, de Medeiros BAS. Artificial lighting as a vector attractant and cause of disease diffusion. Environ Health Perspect (2010) 118(11):1503–6. doi: 10.1289/ehp.1002115
36. O’Hanlon SJ, Slater HC, Cheke RA, Boatin BA, Coffeng LE, Pion SDS, et al. Model-based geostatistical mapping of the prevalence of onchocerca volvulus in West Africa. PloS Negl Trop Dis (2016) 10(1):e0004328. doi: 10.1371/journal.pntd.0004328
37. Fick SE, Hijmans RJ. WorldClim 2: new 1-km spatial resolution climate surfaces for global land areas. Int J Climatol (2017) 37(12):4302–15. doi: 10.1002/joc.5086
38. Harris I, Jones PD, Osborn T j., Lister D h. Updated high-resolution grids of monthly climatic observations – the CRU TS3.10 dataset. Int J Climatol (2014) 34(3):623–42. doi: 10.1002/joc.3711
39. Musah A, Dutra LMM, Aldosery A, Browning E, Ambrizzi T, Borges IVG, et al. An evaluation of the OpenWeatherMap API versus INMET using weather data from two Brazilian cities: recife and campina grande. Data (2022) 7(8):106. doi: 10.3390/data7080106
40. Elith J, Phillips SJ, Hastie T, Dudík M, Chee YE, Yates CJ. A statistical explanation of MaxEnt for ecologists. Divers Distrib (2011) 17(1):43–57. doi: 10.1111/j.1472-4642.2010.00725.x
41. Estallo EL, Sangermano F, Grech M, Ludueña-Almeida F, Frías-Cespedes M, Ainete M, et al. Modelling the distribution of the vector aedes aegypti in a central Argentine city. Med Vet Entomol (2018) 32(4):451–61. doi: 10.1111/mve.12323
42. Arboleda S, Jaramillo- ON, Peterson AT. Spatial and temporal dynamics of aedes aegypti larval sites in bello, Colombia. J Vector Ecol (2012) 37(1):37–48. doi: 10.1111/j.1948-7134.2012.00198.x
43. Portilla Cabrera CV, Selvaraj JJ. Geographic shifts in the bioclimatic suitability for aedes aegypti under climate change scenarios in Colombia. Heliyon (2020) 6(1):e03101. doi: 10.1016/j.heliyon.2019.e03101
44. Racloz V, Ramsey R, Tong S, Hu W. Surveillance of dengue fever virus: a review of epidemiological models and early warning systems. PloS Negl Trop Dis (2012) 6(5):e1648. doi: 10.1371/journal.pntd.0001648
45. Semenza JC. Prototype early warning systems for vector-borne diseases in Europe. Int J Environ Res Public Health (2015) 12(6):6333–51. doi: 10.3390/ijerph120606333
46. Lowe R, Bailey TC, Stephenson DB, Jupp TE, Graham RJ, Barcellos C, et al. The development of an early warning system for climate-sensitive disease risk with a focus on dengue epidemics in southeast Brazil. Stat Med (2013) 32(5):864–83. doi: 10.1002/sim.5549
47. Gutfraind A, Peterson JK, Rose EB, Arevalo-Nieto C, Sheen J, Condori-Luna GF, et al. Integrating evidence, models and maps to enhance chagas disease vector surveillance. PloS Negl Trop Dis (2018) 12(11):e0006883. doi: 10.1371/journal.pntd.0006883
48. Lozano–Fuentes S, Wedyan F, Hernandez–Garcia E, Sadhu D, Ghosh S, Bieman JM, et al. Cell phone-based system (Chaak) for surveillance of immatures of dengue virus mosquito vectors. J Med Entomol (2013) 50(4):879–89. doi: 10.1603/ME13008
49. Sousa L, de Mello R, Cedrim D, Garcia A, Missier P, Uchôa A, et al. VazaDengue: an information system for preventing and combating mosquito-borne diseases with social networks. Inf Syst (2018) 75:26–42. doi: 10.1016/j.is.2018.02.003
50. Lowe R, Bailey TC, Stephenson DB, Graham RJ, Coelho CAS, Sá Carvalho M, et al. Spatio-temporal modelling of climate-sensitive disease risk: towards an early warning system for dengue in Brazil. Comput Geosci (2011) 37(3):371–81. doi: 10.1016/j.cageo.2010.01.008
51. Lowe R, Coelho CA, Barcellos C, Carvalho MS, Catão RDC, Coelho GE, et al. Evaluating probabilistic dengue risk forecasts from a prototype early warning system for Brazil. eLife (2016) 5:e11285. doi: 10.7554/eLife.11285
52. Ashby J, Moreno-Madriñán MJ, Yiannoutsos CT, Stanforth A. Niche modeling of dengue fever using remotely sensed environmental factors and boosted regression trees. Remote Sens (2017) 9(4):328. doi: 10.3390/rs9040328
53. Moraga P, Dean C, Inoue J, Morawiecki P, Noureen SR, Wang F. Bayesian Spatial modelling of geostatistical data using INLA and SPDE methods: a case study predicting malaria risk in Mozambique. Spat Spatio-Temporal Epidemiol (2021) 39:100440. doi: 10.1016/j.sste.2021.100440
54. Alegana VA, Macharia PM, Muchiri S, Mumo E, Oyugi E, Kamau A, et al. Plasmodium falciparum parasite prevalence in East Africa: updating data for malaria stratification. PloS Glob Public Health (2021) 1(12):e0000014. doi: 10.1371/journal.pgph.0000014
55. Juan P, Díaz-Avalos C, Mejía-Domínguez NR, Mateu J. Hierarchical spatial modeling of the presence of chagas disease insect vectors in Argentina. A Comp approach Stoch Environ Res Risk Assess (2017) 31(2):461–79. doi: 10.1007/s00477-016-1340-5
56. Kostkova P. Disease surveillance data sharing for public health: the next ethical frontiers. Life Sci Soc Policy (2018) 14:16. doi: 10.1186/s40504-018-0078-x
Keywords: maximum entropy (MAXENT), GIS, mosquito occupancy, environmental suitability, Aedes aegypti, Brazil
Citation: Musah A, Browning E, Aldosery A, Valerio Graciano Borges I, Ambrizzi T, Tunali M, Başibüyük S, Yenigün O, Moreno GMM, de Lima CL, da Silva ACG, dos Santos WP, Massoni T, Campos LC and Kostkova P (2023) Coalescing disparate data sources for the geospatial prediction of mosquito abundance, using Brazil as a motivating case study. Front. Trop. Dis 4:1039735. doi: 10.3389/fitd.2023.1039735
Received: 08 September 2022; Accepted: 14 April 2023;
Published: 26 May 2023.
Edited by:
Moses Okpeku, University of KwaZulu-Natal, South AfricaReviewed by:
Sk Ajim Ali, Aligarh Muslim University, IndiaRodrigo Morchón García, University of Salamanca, Spain
Chandana Unnithan, Lifeguard Digital Health, Inc., Canada
Copyright © 2023 Musah, Browning, Aldosery, Valerio Graciano Borges, Ambrizzi, Tunali, Başibüyük, Yenigün, Moreno, de Lima, da Silva, dos Santos, Massoni, Campos and Kostkova. This is an open-access article distributed under the terms of the Creative Commons Attribution License (CC BY). The use, distribution or reproduction in other forums is permitted, provided the original author(s) and the copyright owner(s) are credited and that the original publication in this journal is cited, in accordance with accepted academic practice. No use, distribution or reproduction is permitted which does not comply with these terms.
*Correspondence: Anwar Musah, a.musah@ucl.ac.uk