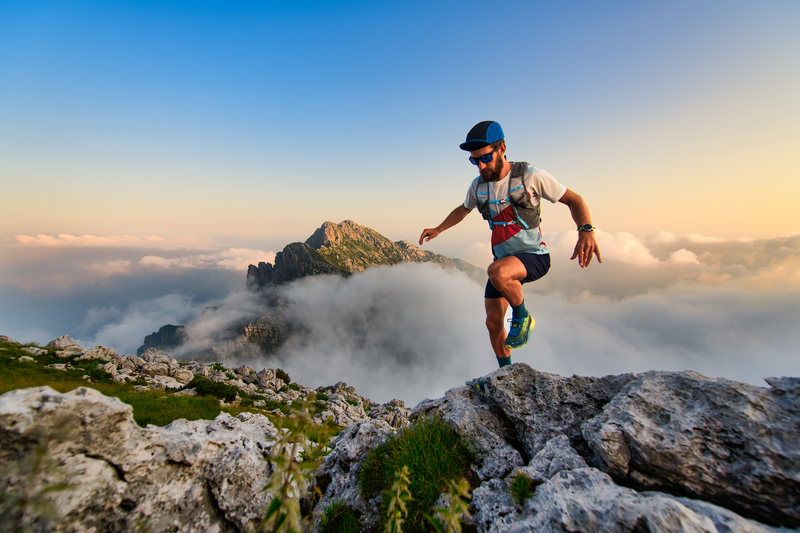
94% of researchers rate our articles as excellent or good
Learn more about the work of our research integrity team to safeguard the quality of each article we publish.
Find out more
ORIGINAL RESEARCH article
Front. Trop. Dis. , 11 May 2022
Sec. Vector Biology
Volume 3 - 2022 | https://doi.org/10.3389/fitd.2022.896289
This article is part of the Research Topic The Transition of Novel Genetic Control Strategies into Reality for Vector-Borne Tropical Disease Control and Prevention; Research Advances and next Steps View all 12 articles
The mosquito microbiota affects many aspects of mosquito biology including development and reproduction. It also strongly impacts interactions between the mosquito host and pathogens that cause important disease in humans, such as dengue and malaria. Critically, the mosquito microbiota is highly diverse and can vary in composition in response to multiple environmental variables, but these effects are not always consistent. Understanding how the environment shapes mosquito microbial diversity is a critical step in elucidating the ubiquity of key host-microbe-pathogen interactions in nature. To that end, we examined the role of time of collection, collection location and host species on mosquito microbial diversity by repeating collections at two-month intervals on a trapping grid spanning three distinct biomes. We then used 16S rRNA sequencing to compare the microbiomes of Aedes taeniorhynchus, Anopheles crucians, and Culex nigripalpus mosquitoes from those collections. We saw that mosquito diversity was strongly affected by both time and collection location. We also observed that microbial richness and diversity increased from March to May, and that An. crucians and Cx. nigripalpus had greater microbial diversity than Ae. taeniorhynchus. However, we also observed that collection location had no impact on microbial diversity except for significantly lower bacterial richness observed in mosquitoes collected from the mangrove wetlands. Our results highlight that collection time, collection location, and mosquito species each affect aspects of mosquito microbial diversity, but their importance is context dependent. We also demonstrate that these variables have differing impacts on mosquito diversity and mosquito microbial diversity. Our findings suggest that the environment likely plays an important but variable role in influencing the composition of the mosquito microbiota.
Each year, infections with mosquito-borne pathogens cause millions of human cases of diseases including malaria, dengue, and West Nile fever (1). There are more than 3500 different mosquito species, and many are implicated in transmitting pathogens that cause disease. Pathogen infection and transmission in mosquitoes is highly dependent on interactions between pathogen and mosquito host. Important factors in these interactions include pathogen replication or development cycles, and interactions between the pathogen and the host immune system, which can repress infection, or be suppressed or avoided to promote infection (2).
Another key player in host-pathogen interactions is the microbiota: the community of diverse microorganisms encompassing bacteria, fungi, viruses, and protozoa that naturally associates with mosquitoes (3–5). Mosquito immune pathways that respond to infection with arboviruses like dengue, or the Plasmodium parasites that cause malaria, can be activated by the microbiota, facilitating indirect modulation of pathogen infection in mosquitoes (6). The presence or absence of the microbiota has been linked to differential expression of antimicrobial peptides linked to the Toll and IMD immune pathways and altered dengue virus (DENV) titers in Aedes aegypti (7, 8). Similarly, antibiotic-treated Anopheles gambiae mosquitoes without their natural microbiota experienced increased susceptibility to Plasmodium falciparum infection, which was linked to stimulation of immune effectors by the microbiota (9). The presence of specific bacteria can also modulate pathogen infection. For instance, infection with Serratia marcescens or Serratia odorifera can promote susceptibility to arboviral infection (10, 11), infection with Wolbachia pipientis can reduce susceptibility to multiple mosquito-borne pathogens including DENV and Zika virus (12, 13), and infection with Chromobacterium sp. Csp_P restricts infection with both DENV and Plasmodium falciparum (14).
The microbiota plays an important role in multiple aspects of mosquito biology beyond immunity. The presence of bacteria or live eukaryotic cells is critical for normal larval development (15), with this effect linked to hypoxia and riboflavin provisioning by bacteria (16, 17). Axenic (bacteria-free) development is possible if larvae are fed certain diets or on live eukaryotic cells (18, 19). The microbiota also appears to be important to digestion and reproduction, as antibiotic treatment of Ae. aegypti reduced fecundity and reduced ability to digest blood-derived protein (20). The presence of individual bacteria can also impact host physiology. For instance, infection of An. gambiae with Asaia reduces development time (21), and infection of Ae. aegypti with Wolbachia can modulate egg development (22), longevity (23), and feeding behaviors (24). To understand how likely it is that these and similar interactions will occur in mosquitoes in nature, it is important to understand the factors that cause specific bacteria to associate with mosquitoes.
The mosquito microbiota is naturally diverse. Individual mosquitoes can be infected with dozens or even hundreds of different bacteria and there are frequently high levels of variation in microbiota composition between mosquito populations and even between individual mosquitoes (4). Mosquitoes are capable of harboring bacteria from diverse phyla, with Actinobacteria, Bacteroidetes, Firmicutes, and Proteobacteria all well represented in sequenced microbiomes (5, 25, 26). Most mosquito-associated bacteria are acquired from the environment, with larval microbiota overlapping in composition with the water from their aquatic habitats (3, 4), but microbial diversity is lower in larvae than in the environment (27). During morphogenesis, pupae appear to retain some of their larval microbiota, and bacteria can pass transstadially to adults (28). Microbial diversity is higher amongst larval mosquitoes than adults (15), and in wild mosquitoes than laboratory-reared mosquitoes. Laboratory Ae. aegypti colonies share common microbiota, regardless of their location, suggesting that, in controlled environments, their tissues promote colonization by certain bacteria (29). Critically, it is still unclear what extrinsic factors shape the composition of the microbiota, and whether the impact of these variables changes between different mosquito populations and species.
Studies have demonstrated major shifts in microbial diversity and composition due to sex, age, during diapause, and due to variation across seasons and time (30–33). The role of geography is less clear, with several studies showing that microbiota composition is influenced by collection origin (34–36), while other studies have failed to find such an effect (37, 38). In container breeding mosquitoes, container type might have a minor predictive role in shaping the microbiota (39, 40). Further studies show that collection location drives diversity, with specimens from the same location having more similar microbiota than those from other locations even if they are different mosquito species (3, 41). These findings likely highlight the fact that the microbiota is a complex system where multiple macro- and micro-environmental variables affect the abundance of bacteria in different environments where mosquitoes also occur (25, 40, 42), while intrinsic factors linked to mosquito biology dictate the ability of different bacteria to colonize mosquito tissues.
Explicitly defining the roles of environmental variables that predict microbiota composition in mosquitoes is a key step towards understanding natural variation in mosquito microbial diversity and mosquito-microbe interactions. To that end, we sought to evaluate the role of collection time, collection location, and mosquito species in shaping mosquito microbial diversity over a small scale. Within this experimental design we were also interested in evaluating whether the factors that predict mosquito diversity also determined mosquito microbial diversity. To address these questions, we collected mosquitoes three times at two-month intervals using a six-trap grid that spanned three distinct biomes within 1km of each other. Bacterial profiles from cohorts of three mosquito species collected at different times and across the three biomes were sequenced using 2x300bp 16S rRNA Illumina MiSeq sequencing in order to assess the role of these variables in mosquito microbial diversity.
Mosquito collections for this study took place in the Oslo Riverfront Conservation Area (ORCA) and forested trails abutting the Florida Medical Entomology Laboratory (FMEL), both located in Vero Beach, Florida, United States. ORCA is a 298-acre conservation area accessible to the public, which contains approximately 1.6km of paved and unpaved walking trails and boardwalks. The FMEL campus is a 35-acre property immediately to the south of ORCA that contains 500m of unpaved walking trails accessible to researchers but not the general public. The ORCA and FMEL walking trails cross diverse biome types: estuarine wetlands dominated by mangroves to the east, coastal hammock in the center and north, and pine flatwoods in a portion of the west. For mosquitoes, these areas differ in their potential access to oviposition and larval development sites, the plant life available for nectar feeding and as resting or landing sites, and the types of animals available as potential blood meal hosts. Critically, the biomes are found within 1km of each other, making ORCA/FMEL an ideal location for assessing the role of small-scale changes in collection location on mosquito and microbial diversity.
In this study, adult mosquitoes were collected using CDC mini-light traps (John W. Hock Company) baited with cannisters containing dry ice (File S1). We intended to capture only host-seeking mosquitoes given that blood feeding can induce dysbiosis in the mosquito microbiota (15, 43). A 6-trap grid was deployed three times at approximately two-month intervals from March through July 2021 (Figure 1; map adapted from ESRI 2022. ArcGIS Desktop: Release 10.8. Redlands, CA: Environmental Systems Research Institute). On each trap night, traps were hung from the same trees. Two traps were deployed into each of three biomes (mangrove wetlands, coastal hammock, and pine flatwoods), with these paired traps placed approximately 100-200 meters apart, depending on the availability of suitable trees. On each trap night, traps were deployed between 3-6pm and collected the next morning between 8-10am. During this time, trap fans were powered by a single 9V lead-acid battery.
Figure 1 Mosquito trapping locations. Dry ice-baited CDC mini light traps were used to collect host-seeking mosquitoes within the Oslo Riverfront Conservation Area, Vero Beach, FL, USA. Pairs of traps were deployed overnight in distinct biomes: mangrove wetlands (dark blue circles), coastal hammock (light blue circles), and pine flatwoods (pink circles) at a distance of approximately 100m apart. Trapping was repeated during March, May, and July 2021. Map image in panel A adapted from ArcGis Desktop (source: Esri, DigitalGlobe, GeoEye, Earthstar Geographics, CNES/Airbus DS, USDA, USGS, AeroGRID, IGN, and the GIS User Community). Photographs in panels (B–D) taken by Eric P. Caragata.
Trap night one was conducted on March 11th, 2021, however due to unanticipated maintenance at the ORCA entry that prevented collection of the traps deployed in the pine flatwoods, collections from those areas were repeated on March 13th, 2021, the first possible date after the end of the maintenance. Trap nights two and three proceeded without issue on May 4th, 2021, and July 6th, 2021, respectively.
Upon collection, traps were returned to the laboratory and alive and intact specimens were knocked down by freezing for 1 hour at -20°C. After this time, samples were sorted on glass petri dishes chilled by ice. Mosquito samples were retained and returned to storage at -20°C while other collected insects were discarded. Mosquito samples were identified to the species level if possible, or the genus level otherwise, using morphological keys and mosquito reference texts (44–48). Samples that were in poor condition due to physical damage and could not be identified were classified as being ‘unidentifiable’.
Mosquito counts per species data (Table 1) were used to develop graphs of species abundance and species composition for each trap across biomes and across time. Total collection numbers were compared by trap night and biome using a chi-square test of independence using Graphpad Prism. Data from samples that could only be identified to the genus level and from ‘unidentifiable’ specimens were included in these two analyses but were excluded from subsequent analyses. Numbers of unique species collected were compared across habitat and time using chi-square test of independence, as above. Diversity analyses were performed using the R package vegan (v. 2.5-7). Alpha diversity (mean species diversity) was calculated using the Shannon index and the diversity() function in R. Shannon values were split by time of collection or biome. Normality was assessed using the Shapiro-Wilk test, and Shannon values were compared using Kruskal-Wallis ANOVA. Beta diversity (dissimilarity between traps) was calculated using Non-metric multidimensional scaling (NMDS) with the metaMDS() function. Permutational multivariate analysis of variance (PERMANOVA) was used to assess the impact of collection time, collection biome, time by biome interaction, and trap by trap variation on the diversity of mosquitoes collected. Species abundance data were square root-transformed, then a dissimilarity matrix was produced using the vegdist() formula, and then analyzed using the adonis2() function to build a model that incorporated those four variables.
We wanted to sequence the microbiota of three different mosquito species and observe variation in microbial diversity due to host species, biome, and time of collection. Three mosquito species were abundant across all biomes in at least two collection periods: Ae. taeniorhynchus, An. crucians, and Cx. nigripalpus. However, Ae. taeniorhynchus was only abundant in the May and July collections, and An. crucians only in the March and May collections. Accordingly, we selected 96 of the 3991 female mosquito samples for 16s rRNA sequencing (File S2) with 3-6 samples per species-time-biome combination. No Ae. taeniorhynchus from March or An. crucians from July were selected due to insufficient samples collected during those months. Culex nigripalpus samples from all collections were selected and sequenced.
Adult female mosquito samples were processed individually. Each mosquito was surface sterilized by immersion in 70% molecular grade ethanol (Fisher, BP2818500) for 2 mins, before being washed twice by immersion in sterile, DNase/RNase-free molecular grade water (Fisher, BP28191). Samples were manipulated using fine forceps that were sterilized by flame and ethanol after contact with each mosquito to limit cross-contamination. Bacterial DNA from each sample was extracted using the Quick-DNA Fungal/Bacterial Miniprep Kit (Zymo, D6005), according to manufacturer’s instructions. Sample DNA yield and absorbance ratios were determined using an ND-2000 (NanoDrop). Two no-sample (kit blank) extractions and two elution blanks were also performed and sent for microbial sequencing as negative controls.
Sample DNA was shipped to MR DNA (Shallowater, TX, USA), who performed PCR amplification for the V4 region of the 16S rRNA gene using 515F/806R primers. MR DNA also constructed libraries, performed quality assurance, conducted Illumina MiSeq to generate 2x300bp paired-end reads, as previously described (39). The MR DNA custom analysis pipeline (49) was used to generate sequencing data at different taxonomic levels and generate operational taxonomic unit (OTU) calls at a 3% divergence level. These data were returned to our research team and used in all subsequent analyses.
Data were analyzed at the class (File S3) and OTU levels (File S4). Listed taxa were verified to ensure that only bacteria were included. All taxa where the maximum abundance was less than 0.05% across all samples were removed from analysis, and these revised data sets were used to generate read counts and percentage abundance data at both taxonomic levels. OTUs that were prevalent in at least three of the four negative control samples, with an average read count of > 300 were not considered in the analyses described below. Two samples were excluded from the dataset as they returned fewer than 5000 total reads, leaving a total of 94 samples. Class level data were used to generate microbiome profiles for the individual mosquito specimens that we sequenced. For each of these specimens, we generated graphs detailing the percentage of total reads accounted for by highly abundant bacterial taxa. Highly abundant bacterial classes within the dataset were identified by ranked average read percentage across all mosquito samples. Highly abundant OTUs were identified in the same manner. Highly prevalent OTUs were identified as the proportion of samples in which an OTU was present at a percentage of total reads of greater than 0%.
Total OTU counts per sample at a minimum abundance threshold of 0.05% were compared across all samples by multiple linear regression using a model that compared the variables of collection time, mosquito species, and collection biome. Mean OTU counts were compared by mosquito species, time of collection, and biome of collection using a heat map. Alpha diversity for OTU counts was calculated via the Shannon index, as above. These data were also analyzed by multiple linear regression using a model that compared the variables of collection time, mosquito species, and collection biome. Mean Shannon index data were split by mosquito species, time of collection, and biome of collection then visualized using a heat map. NMDS analysis was performed on OTU counts using the metaMDS() function in R, as above, and the resulting output split by treatment then visualized in Microsoft Excel. PERMANOVA was performed on OTU count data using the adonis2() function, as above. This model examined the influence of time, biome, mosquito species, trap, and interactions between those variables.
Bacterial OTUs that changed in prevalence depending on the mosquito species, time of collection, or biome of collection were identified and visualized using heatmaps. OTU level abundance data were independently sorted by those three predictor variables then prevalence of infection for each OTU was calculated across mosquito samples at a threshold of greater than 0% of total reads. The variance in prevalence was calculated across treatments for each of the three predictor variables, these values were then ranked and differences in prevalence of infection were compared between the 15 OTUs with the greatest variance using chi-square tests in GraphPad prism.
Diversity analyses were performed in RStudio using the vegan package, as described above. R scripts are provided in File S5. All other analyses were performed using Graphpad Prism (V.9.3.0), as above. Figures were prepared using Graphpad Prism and Microsoft PowerPoint for Mac (v16.57).
Across the three trap nights, we collected a total of 4053 mosquitoes from 12 different mosquito species, of which 3991 were female (Table 1, File S1). Of these, 197 (4.94%) could only be identified to the genus level and a further 107 (2.68%) were unidentifiable. Collection numbers varied significantly between biomes and across time (Chi-square test: X2 = 568.9, P < 0.0001). A total of 1214 female mosquitoes (30.42%) were collected in March, 907 (22.73%) in May, and 1870 (46.86%) in July. A total of 2466 female mosquitoes (61.79%) were collected from traps in the mangrove wetlands, 658 (16.49%) from coastal hammock traps, and 867 (21.72%) from pine flatwoods traps. Species richness across time and collection locations varied from a minimum of three different species collected in pine flatwoods traps during May to a maximum of eight in the same habitat during March. An average of 5.89 mosquito species were collected in each biome across all time points. However, no significant variation in richness was observed across our collections (Chi-square test: X2 = 2.428, P = 0.6575).
We observed large-scale variation in the mosquito species we collected across time and between the different biomes (Figure 2). Four mosquito species were collected in high abundance (Figure S1): Deinocerites cancer (N = 1121, 28.09% of total mosquito collections), Culex nigripalpus (1060, 26.56%), Aedes taeniorhynchus (665, 16.66%), and Anopheles crucians (472, 11.83%). Abundance of De. cancer increased over time, with 94.65% of all specimens from that species collected in mangrove wetlands. Abundance of Cx. nigripalpus declined from March to May, and then increased from May to July. Abundance in coastal hammock was consistent across the three trapping periods, with the peak abundance observed in pine flatwoods during March (25.57%). Few Ae. taeniorhynchus were collected during March, but collections increased marginally in May and then drastically during July, with peak abundance observed in mangrove wetlands during July (44.06%). An. crucians collections peaked in March and declined steeply thereafter. Abundance was higher in pine flatwoods and coastal hammock than in mangrove wetlands.
Figure 2 Mosquito collections across time and biomes. Panels depict the relative abundance of different mosquito taxa collected during March (A), May (B), and July (C). Data are presented as a percentage of total mosquitoes collected from each trap. A total of 3991 female mosquitoes from 12 distinct species were collected, with the crabhole mosquito Deinocerites cancer being the most abundant and the Florida SLE mosquito Culex nigripalpus being the most ubiquitous. Mosquito taxa from the same genus are presented in common colors. Taxonomic status was assessed using established morphological keys, with samples being assigned to a particular species or genus, if species-level identification was not possible, or being classified as ‘unidentifiable’ if the sample condition was too poor to allow identification. CH, coastal hammock; MW, mangrove wetlands; PF, pine flatwoods; sp, species; Uncat, uncategorizable.
Analysis of Shannon index values showed no significant impact of time of collection on mosquito alpha diversity (Figure 3A. Kruskal-Wallis: H = 3.029, P = 0.231), but did show a significant impact of biome (Figure 3B. Kruskal-Wallis: H = 6.503, P = 0.031) with that effect driven by higher mosquito diversity in mangrove wetlands than in pine flatwoods (Dunn’s test: P = 0.039). In our NMDS analysis (Figure 3C; k = 2, stress = 0.1582), we observed separation of traps due to time (MDS1) and biome (MDS2). PERMANOVA confirmed that there was a significant impact of time (R2 = 0.3952, F = 16.94, P = 0.001) and biome (R2 = 0.4186, F = 17.94, P = 0.001) on mosquito diversity, with these two variables accounting for approximately 81% of the variation in the model. We also observed non-significant effects of trap-by-trap variation (R2 = 0.0275, F = 0.785, P = 0.647) and time x biome interaction (R2 = 0.0888, F = 1.902, P = 0.103).
Figure 3 Mosquito diversity analyses. Alpha diversity (mean species diversity) was assessed by collection time (A) and collection biome (B) using the Shannon index with the diversity() function in R. We observed no significant impact of time on alpha diversity (Kruskal-Wallis: P = 0.2306) but did see an effect of biome (Kruskal-Wallis: P = 0.0312), driven by higher diversity in mangrove wetlands than in pine flatwoods. We also used NMDS as a measure of beta diversity (dissimilarity between trap collection profiles) and saw good separation of traps by time and collection biome (C). PERMANOVA revealed that both time (R2 = 0.3952, P = 0.001) and biome (R2 = 0.4186, P = 0.001) were important predictors of mosquito diversity. Different shapes and colors in these panels depict individual traps across collection times and biomes. Lower-case letters (a/b) in the first two panels represent different statistical groups. CH, coastal hammock; MW, mangrove wetlands; PF, pine flatwoods.
Our 16s sequencing produced a total of 3.32 million reads. A total of 2.98 million reads were identified as being bacterial in origin, which equated to an average of 31,010 bacterial 16s rRNA reads per mosquito sample (median – 32,077 reads). Raw sequencing data were deposited in GenBank (accession ID: PRJNA818006). Reads associated with 57 different bacterial classes were detected in at least one of the 94 sequenced mosquito samples at an abundance of greater than 0.05%. Microbiome profiles of individual mosquitoes across collection time, species and biome were generated for the 12 most abundant bacterial classes (Figure 4) with these classes accounting for an average of 92.57% of all reads. By average percentage of total reads, the top five most abundant bacterial classes in the dataset were Actinobacteria (17.02%), Bacilli (14.09%), Gammaproteobacteria (13.60%), Betaproteobacteria (13.21%), and Alphaproteobacteria (12.45%). A total of 37 individual mosquito specimens had a single bacterial class representing more than 50% of all reads. These classes were: Actinobacteria (5 samples), Alphaproteobacteria (3), Bacilli (9), Betaproteobacteria (1), Epsilonproteobacteria (3), Gammaproteobacteria (4), and Spirochaetia (12). At the OTU level (Table 2) the most abundant OTU across all samples matched to Lactobacillus kunkeei. Three Spironema culicis OTUs were amongst the ten most abundant, with these corresponding to the class Spirochaetia reads. Betaproteobacterium Variovorax paradoxus was the most prevalent OTU in the dataset, being detected in 81% (76/94) of all samples. Other common members of the mosquito microbiota (5, 25) were detected at high prevalence, including Staphylococcus pasteuri (61%), Burkholderia ubonensis (51%), and Serratia marcescens (49%). Reads from five Asaia sp. OTUs were detected in the dataset. These OTUs were highly abundant amongst Ae. taeniorhynchus samples collected in July, with a peak abundance of 76.18%. Three Wolbachia OTUs were observed in the dataset, with the most prevalent of these observed in 12/94 mosquito samples. Wolbachia reads were detected at a maximum abundance of 5.37%, 0.12%, and 0.02% in An. crucians, Cx. nigripalpus, and Ae. taeniorhynchus mosquitoes, respectively, and were not indicative of widespread, stable infections.
Figure 4 Mosquito microbiome composition at the class level. We used 2x300bp Illumina MiSeq sequencing to profile 16s rRNA sequences in 94 Anopheles crucians (A, B), Aedes taeniorhynchus (C, D), and Culex nigripalpus (E–G) mosquito samples collected across the three trap nights and biomes, with 3-6 samples profiled for each condition. Insufficient numbers of An. crucians and Ae. taeniorhynchus were collected in July and March, respectively, so samples from those species were not sequenced for those timepoints. Each bar represents the relative abundance of all the bacteria from each sample at the class taxonomic level. Within each bar, different colors represent 12 bacterial classes, which accounted for an average of 92.57% of all reads across the dataset, and an ‘other classes’ group that represents all the remaining reads. C, coastal hammock; M, mangrove wetlands; P, pine flatwoods.
A total of 2516 OTUs were detected in the raw microbiome data. Of these, 156 were removed from the dataset due to low abundance. A further 10 OTUs were classified as contaminants due to their presence in negative control samples and were also removed from the dataset. We assessed the diversity of the mosquito microbiota using the remaining 2350 OTUs. Multiple linear regression of microbial species richness (using OTU count data at a threshold of 0.05% abundance per sample) indicated that time (F = 24.16, P < 0.0001), biome (F = 5.348, P = 0.0064), and mosquito species (F = 9.956, P = 0.0001) were all significant predictors of the number of OTUs detected. Visualization of these data via heat map revealed lower microbial richness associated with March collections, with the mangrove wetlands biome, and with Ae. taeniorhynchus (Figure 5A). Visualization of Shannon index data via a heatmap (Figure 5B) also revealed lower alpha diversity associated with March collections and with Ae. taeniorhynchus. The impact of biome was less clear for this index, although variation due to biome within species groups were observed. Similarly, multiple linear regression of Shannon index data revealed a significant impact of collection time (F = 10.859, P < 0.0001) and mosquito species (F = 23.040, P < 0.0001), but not for biome (F = 1.508, P = 0.2270).
Figure 5 The impact of mosquito species, collection time, and collection biome on mosquitomicrobial diversity. We found that all three variables impacted aspects of microbial diversity, with time and mosquito species having the strongest effects. Heatmap depicting variation in the average number of OTUs in sequenced mosquitoes depending on the mosquito species, time, and biome of collection (A). Light colors (yellow-orange) indicate a higher OTU count, while dark colors (purple-black) indicate a lower count. Time (P < 0.0001), biome (P = 0.0064), and mosquito species (P = 0.0001) were all significant predictors of the number of OTUs detected. Lower OTU counts were observed in March, for Ae. taeniorhynchus mosquitoes, and for mangrove wetlands samples. Additional heatmap and MLR analyses of alpha diversity of OTU count data (via Shannon index) for the same treatments (B) indicated a similar role for time (P < 0.0001) and species (P < 0.0001) but indicated that the role of biome was less pronounced (P = 0.2270). NMDS assessment of OTU count data beta diversity (C) indicated there were two main clusters of samples with the split being due to time of collection (March vs May/July). Samples from later timepoints that grouped with the March cluster were principally from mangrove wetlands collections. A sub-grouping of Ae. taeniorhynchus samples occurred at the base of the rightmost cluster. In panel C, the different shapes and colors depict the different treatment groups (time x species x biome). AC, Anopheles crucians; AT, Aedes taeniorhynchus; C, coastal hammock; CN, Culex nigripalpus; M, mangrove wetlands; P, pine flatwoods.
NMDS analysis of microbial beta diversity (Figure 5C; k = 2, stress = 0.2215) revealed two clusters split by time of collection. The first cluster consisted chiefly of samples from the March collection, but also included a small number of mosquitoes collected during May, predominantly those from the mangrove wetlands traps. The second cluster consisted chiefly of samples from the May and July collections. Large numbers of Ae. taeniorhynchus samples were situated at the base of this cluster, largely independent of samples from the other two species. PERMANOVA analysis of OTU data revealed that time (R2 = 0.0654, F = 3.33, P = 0.001), mosquito species (R2 = 0.0438, F = 2.31, P = 0.001), and the time by species interaction component (R2 = 0.0268, F = 1.36, P = 0.007) all had significant effects on mosquito microbial diversity. Biome did not have a significant effect (R2 = 0.0234, F = 1.19, P = 0.066). No other components in the model were significant predictors of microbial diversity and the residual component had an R2 value of 0.510.
To identify bacterial OTUs that were highly responsive to changes in host species, time of collection, and the biome of collection, we assessed OTUs by variance of prevalence for each of these three variables (Figure 6). We identified OTUs with significantly higher prevalence associated with each species of mosquito. This list included three Lactobacillus kunkeei OTUs, two Klebsiella pneumoniae OTUs and two Acinetobacter sp. OTUs. Of the 15 OTUs described in Figure 6, ten had the greatest prevalence in Ae. taeniorhynchus, four in Cx. nigripalpus, and one in An. crucians. Time-responsive OTUs all increased in prevalence between the March and May/July collections. These included three OTUs associated with Tetrasphaera sp., three with Rhodoferax sp., and two each with Acidovorax sp. and Rhodanobacter sp. We observed multiple biome-responsive OTUs with sequences similar to Acidovorax sp., Corynebacterium sp., and Rhodoferax sp. Biome-responsive OTUs typically had low prevalence in mosquitoes collected from mangrove wetlands, and higher prevalence in mosquitoes from pine flatwoods and/or coastal hammock. Using chi-square tests (File S6), significant differences in prevalence were observed between their respective treatments for 44 of the 45 comparisons depicted in Figure 6 (P < 0.05). The exception occurred for OTU140 (Propionivibrio sp.), where higher prevalence was observed in coastal hammock and pine flatwoods biomes, but the effect was not significant (chi-square; X2 = 5.96, P = 0.0509).
Figure 6 Identification of bacterial OTUs that changed in prevalence due to variation in mosquito species, time, and collection biome. Heat maps of the 15 OTUs that exhibit the highest variance in prevalence (presence in x proportion of sequenced samples) due to mosquito species (A), collection time (B), and collection biome (C). OTUs are presented as: unique OTU number | bacterial class | likely match at the species or genus level | statistical significance of comparison (chi-square test: * = P < 0.05, ** = P < 0.01, *** = P < 0.001, **** = P < 0.0001). In each comparison, multiple OTUs associated with the same bacterial species or genus were observed. OTUs of high and low prevalence were observed for each mosquito species. Three Lactobacillus kunkeei OTUs had high prevalence in Ae. taeniorhynchus and low prevalence in Cx. nigripalpus. Two Acinetobacter sp. OTUs had lower prevalence in An. crucians than in the other species. Time-responsive OTUs all experienced lower prevalence in mosquitoes collected during March. Biome-responsive OTUs all experienced lower prevalence amongst mosquitoes collected from mangrove wetlands traps. Note that the scales in the three panels differ. ACT, Actinobacteria; AC, Anopheles crucians; APB, Alphaproteobacteria; AT, Aedes taeniorhynchus; BAC, Bacilli; BPB, Betaproteobacteria; C, coastal hammock; CLO, Clostridia; CN, Culex nigripalpus; FLA, Flavobacteriia; GPB, Gammaproteobacteria; M, mangrove wetlands; P, pine flatwoods; UNK, unknown bacterial class.
We assayed the importance of time of collection, location of collection, and mosquito species in shaping the composition and diversity of the mosquito microbiota. Three mosquito species were examined: Ae. taeniorhynchus, a known carrier of EEV, VEEV, WNV, and a vector of Dirofilaria immitis, An. crucians, an historical vector of Plasmodium spp. in the Southeast United States, and Cx. nigripalpus, a vector of SLEV, EEEV, WNV, and D. immitis (48). In addition, all three species are common nuisance biters.
Our findings indicate that collection time was the most important variable of the three we assessed. Between the March and May/July collections we observed a significant increase in microbial richness (OTU count), an increase in alpha diversity, and a shift in the composition of the microbiota. We also saw that OTUs in the Actinobacteria and Proteobacteria phyla accounted for the majority of OTUs with the greatest variation in prevalence due to time, suggesting that bacteria in these groups could be those most likely to have variable associations with mosquitoes over time. It is known that shifts in time are an important variable in microbiome assembly. For instance, the microbiota of Ae. aegypti in Puerto Rico varied significantly due to collection time (39). It is possible that the time-dependent changes in microbial diversity we observed were driven by seasonal changes, as other studies have demonstrated that such changes can influence microbial diversity. For instance, microbial diversity in An. gambiae and An. coluzzii from Ghana was higher in the dry season than the wet season (30). Another study saw repeatable, seasonal shifts in abundance and/or prevalence of multiple bacteria taxa and observed that these correlated with shifts in patterns of WNV infection (50). Our experimental design did not allow for rigorous assessment of the effect of changes in seasons, and while we saw increased mean monthly peak temperatures as time progressed during our trapping (March - 26°C, May - 30°C, July - 32°C), we did not see notable differences in rainfall in the month prior to collection (March – 152.4mm, May – 96.8mm, July – 118.1mm; Data from Vero Beach Municipal Weather Station, accessed via Weather Underground). Nominally, March and May fall within the dry season in central Florida, while July falls at the beginning of the wet season.
Mosquito species was another important variable in our dataset. Previous studies indicate that there are natural, species-specific differences in microbial diversity. For instance, higher diversity amongst Ae. aegypti and Ae. albopictus compared to Cx. quinquefasciatus (51), and high variability in alpha diversity between mosquitoes from the genera Aedes (including subgenus Ochlerotatus), Coquilletidia, and Culex (50). Similar to those findings, we observed that Ae. taeniorhynchus mosquitoes had lower microbial richness, alpha diversity, and differed in Beta diversity than the other two species we examined. If microorganisms fill physical and functional niches within the mosquito digestive tract (52), it is possible that there are fewer niches available in species such as Ae. taeniorhynchus, perhaps through differential immune response or nutrient availability. While microbial diversity in Ae. taeniorhynchus was low, we did observe multiple OTUs with higher prevalence in that species than the other two. This includes Lactobacillus kunkeei, a fructophilic bacterium that produces large quantities of lactic and acetic acid from glucose (53), and Acinetobacter nectaris, which is commonly found in plant nectar and pollinators (54, 55), which could be indicative of nectar feeding. Conversely, there was a higher prevalence of Shigella sonnei, an opportunistic pathogen often found in contaminated water that causes Shigellosis (56) and Brachymonas sp., a genus of denitrifying bacteria (57), in An. crucians and Cx. nigripalpus than Ae. taeniorhynchus. Our analyses showed little overall difference in microbial diversity between An. crucians and Cx. nigripalpus, although there were subtle differences in species richness and alpha diversity when comparing time by biome combinations for these species. Several previous studies have highlighted that host species can be less important than collection location when predicting microbial diversity (3, 41). In this context, our data highlight the fact that mosquito species-driven effects can act on multiple elements of microbial diversity, but that the importance of species in microbiota assembly is likely context dependent.
The effect of biome on the mosquito microbiota was less clear than for the other two test variables. We saw a clear impact of biome of collection on microbial richness, with mosquitoes from the mangrove wetlands having significantly fewer OTUs. We also saw several OTUs that had high prevalence in either pine flatwoods mosquitoes or coastal hammock and pine flatwoods mosquitoes. These findings indicated that biome of collection did have some influence on mosquito microbial diversity. However, we saw no significant impact of biome on alpha or beta diversity, with no clear clustering of samples by biome in the NMDS analysis, suggesting that the overall impact was minor. These findings are in line with previous studies that examined the effect of location on mosquito microbial diversity where a significant role was outlined in some studies (34–36, 42) but not in others (37, 38). In the case of our study, a distance of less than 1 km is a very small-scale difference in location, and this might have contributed to the effect of biome/collection location being minor. In some studies, larger-scale distances in collection origin have revealed distinct effects on microbial diversity. For instance, Ae. aegypti from Guadeloupe and French Guiana formed largely independent clusters in principle coordinate analysis (40). However, similar effects have been found over small distances, as with An. gambiae in Burkina Faso, where specimens from three villages separated by approximately 10 km also had distinct microbiomes (35). In contrast, collections from other studies ranged over 50 km in Kenya (38) and several hundred kilometers in Panama (37) and saw no clear impact of collection location. Given these disparities, it is possible that certain mosquito habitats are more important to microbial diversity than others, and that in some mosquito populations, other variables make more significant contributions to microbial diversity than location. It is also important to note that within our experimental design, a mosquito’s location of development was not controlled, only its location of collection, so it was entirely possible that we collected and sequenced mosquitoes that had moved between biomes. The dispersal ranges of An. crucians and Cx. nigripalpus are estimated at approximately 1.6 km (58, 59), while Ae. taeniorhynchus is more mobile with an estimated dispersal range of 2 – 20 miles (60). These observations suggest that all three species could be expected to move freely across our short trap grid.
Similar to the findings of other studies (61–63) our data indicated that mosquito abundance was strongly influenced by both time of collection and collection location. Mosquito beta diversity was also affected by both variables, but alpha diversity was affected by location but not time, and species richness did not vary significantly due to time of collection. These findings differed greatly from our observations of mosquito microbial diversity where time, but not collection location impacted alpha and beta diversity, and where species richness was significantly impacted by both variables. In our PERMANOVA models, the time by species interaction term was a significant predictor of microbial diversity but not mosquito diversity. We also observed contrasting mosquito and microbial diversity in the mangrove wetlands (high mosquito diversity but low microbial diversity), and during March (no clear impact on mosquito diversity but lower microbial richness and diversity). Collectively, these observations suggest that factors that predict mosquito diversity are not necessarily good predictors of mosquito microbial diversity. Additionally, the variables of time, collection location, and time x collection interaction within our PERMANOVA models of mosquito and microbial diversity differed in the amount of variation they explained. In the mosquito model, these terms had a combined R2 of 0.9026, while in the microbial model, the equivalent R2 was 0.1156. This suggests that changes in mosquito diversity might be well explained by a simple model consisting of those macroenvironmental variables, but such a model would be insufficient to explain the majority of the variation seen in the microbiota of wild mosquitoes. If such an effect were broadly applicable, it would likely imply that there are other variables playing major driving roles in mosquito microbiota assembly. Given that host-microbiota-environment interactions appear be a complex system, it is possible that these variables differ in importance in a context dependent manner. This list of variables could include a range of macro- and micro-environmental parameters, particularly those linked to the larval aquatic environment, which were not accounted for in our experimental design, or they might be factors intrinsic to mosquito biology that affect permissiveness to different microorganisms, such as immune processes or microbe-microbe competition. Additionally, our findings highlight that there is a need to consider including additional environmental variables, including climate measurements like rainfall and local temperatures, in models describing mosquito microbial diversity. We believe it is worth investigating these factors in greater depth in a broad range of mosquito populations.
Within our experimental design, mosquito age, blood feeding status, development location, and genetic relatedness to other collected mosquitoes were all uncontrolled factors, with several of these being uncontrollable due to the fact that we collected and sequenced wild mosquitoes. All of these factors could feasibly influence the likelihood of exposure to a particular bacterium, and also the composition of the microbiome. Given our use of dry ice-baited traps, we expect that the majority of our collection were young female mosquitoes seeking their first blood meal, however, it is feasible that we may have collected and sequenced older mosquitoes that had previously fed and laid eggs. Developmental variation could have been controlled through the use of emergence traps; however, this could have caused increased genetic relatedness between samples from the same site and trapping in this manner could be difficult to implement as the three species we examined typically develop in natural breeding sites, which can be difficult to locate. Still, it is possible that some of these factors contributed to variation in microbial diversity unaccounted for by our model. Our experimental design was also imbalanced by time-dependent shifts in abundance of Ae. taeniorhynchus and An. crucians, which meant we only had microbiome data from three mosquito species available during one of the three collection periods. We chose to proceed with this design rather than sequencing a greater number of samples from two species over two collection months as we felt that the former design would ultimately prove more informative. If we had been able to sequence all three species across all three collection periods it is possible that we might have seen different effects of species by time and species by biome interactions on microbial diversity. Likewise, this imbalance could have led to overestimation of the importance of species and species by time effects in our data.
Our results highlight that each of our three test variables of collection time, collection location, and mosquito species affected microbial richness, diversity, prevalence, and/or abundance. However, their importance was relative and context dependent. Collection time was clearly the most influential variable in our experimental design and appears likely to be a major factor in shaping host-microbe interactions in wild mosquitoes. It remains to be seen whether time-induced shifts in microbial diversity are consistent from year to year in mosquito populations around the world, and clarifying this remains an important future direction for microbiome research. Likewise, the implications of such shifts on host-microbe interactions, particularly those associated with mosquito immunity and pathogen infection are also unclear. However, from our data it is apparent that microbiome studies involving field collections should attempt to collect samples over as brief a window as possible. We also show that small-scale changes in collection location can impact microbial richness and the prevalence of certain OTUs, but may not have a major impact on other elements of mosquito microbial diversity. In the context of results from other studies, this finding indicates that there are likely key factors associated with certain habitats, but not others, that influence microbial diversity in mosquitoes. Identifying these factors and determining whether they consistently modulate microbial diversity over time is a pivotal step in our understanding of mosquito microbiota assembly. Finally, we demonstrate that locations with high mosquito diversity do not necessarily produce mosquitoes with high microbial diversity. It could be interesting to determine whether there is a similar effect in mosquito larvae and their aquatic habitats as this observation could potentially be linked to the larval habitat preferences of different mosquito species.
Collectively, our findings indicate that it is important to attempt to control ecological variables when surveying mosquito microbiomes in nature, as this could help to avoid major biases in the resulting data. Controlling these variables could also provide a useful platform for answering fundamental questions about the biology of mosquito-microbe interactions in nature, including whether there are networks of microbes that co-associate with mosquitoes under certain conditions, whether microbiomes associated with particular ecosystems or changes in time have different impacts on mosquito biology at the functional level, or if different microbiomes impact pathogen infection and transmission in adult mosquitoes. It is clear that environmental variables play an important role in shaping mosquito microbial diversity, but our data also indicate that it is possible that microbial diversity is a complex system shaped by multiple variables of minor effect that vary in their importance between different mosquito populations, over time, and across geographic space.
The datasets presented in this study can be found in online repositories. The names of the repository/repositories and accession number(s) can be found below: NCBI GenBank; PRJNA818006.
DP-R, MR, BG, and EC contributed to conception and design of the study. DP-R, MR, KP, and EC performed mosquito collections. DP-R, MR, KP, and BG identified mosquito specimens. MR performed DNA extractions. EC performed the statistical analysis and wrote the first draft of the manuscript. All authors contributed to the manuscript revision, read, and approved the submitted version.
This project was funded through a grant to EC from the Oslo Riverfront Conservation Area Endowment, and through the United States Department of Agriculture (USDA) National Institute of Food and Agriculture, Hatch project 1026692, also to EC. KP was supported by an NSF S-STEM summer undergraduate research stipend through award 1643780.
The authors declare that the research was conducted in the absence of any commercial or financial relationships that could be construed as a potential conflict of interest.
All claims expressed in this article are solely those of the authors and do not necessarily represent those of their affiliated organizations, or those of the publisher, the editors and the reviewers. Any product that may be evaluated in this article, or claim that may be made by its manufacturer, is not guaranteed or endorsed by the publisher.
We would like to thank Dr. Estelle Martin, Ashley Malcolm, and Sarah Shapiro for assistance with mosquito collections, Dr. Lary Reeves, Dr. Dongmin Kim, Kristen Sloyer, and Anthony Cruz for assistance with mosquito identification, and Dr. Lindsay Campbell for assistance with arcgis. We would also like to extend a special thank you to Dr. Scot Dowd from MR DNA for timely delivery of the sequencing data.
The Supplementary Material for this article can be found online at: https://www.frontiersin.org/articles/10.3389/fitd.2022.896289/full#supplementary-material
2. Simões ML, Caragata EP, Dimopoulos G. Diverse Host and Restriction Factors Regulate Mosquito-Pathogen Interactions. Trends Parasitol (2018) 34(7):603–16. doi: 10.1016/j.pt.2018.04.011
3. Coon KL, Brown MR, Strand MR. Mosquitoes Host Communities of Bacteria That are Essential for Development But Vary Greatly Between Local Habitats. Mol Ecol (2016) 25(22):5806–26. doi: 10.1111/mec.13877
4. Gimonneau G, Tchioffo MT, Abate L, Boissiere A, Awono-Ambene PH, Nsango SE, et al. Composition of Anopheles Coluzzii and Anopheles Gambiae Microbiota From Larval to Adult Stages. Infect Genet Evol (2014) 28:715–24. doi: 10.1016/j.meegid.2014.09.029
5. Gendrin M, Christophides G. The Anopheles Mosquito Microbiota and Their Impact on Pathogen Transmission. In: Manguin S, editor. Anopheles Mosquitoes New Insights Into Malaria Vectors. 1. London: IntechOpen. (2013).
6. Caragata EP, Short SM. Vector Microbiota and Immunity: Modulating Arthropod Susceptibility to Vertebrate Pathogens. Curr Opin Insect Sci (2022) 50:100875. doi: 10.1016/j.cois.2022.100875
7. Ramirez JL, Souza-Neto J, Torres Cosme R, Rovira J, Ortiz A, Pascale JM, et al. Reciprocal Tripartite Interactions Between the Aedes Aegypti Midgut Microbiota, Innate Immune System and Dengue Virus Influences Vector Competence. PloS Negl Trop Dis (2012) 6(3):e1561. doi: 10.1371/journal.pntd.0001561
8. Xi Z, Ramirez JL, Dimopoulos G. The Aedes Aegypti Toll Pathway Controls Dengue Virus Infection. PloS Pathog (2008) 4(7):e1000098. doi: 10.1371/journal.ppat.1000098
9. Dong Y, Manfredini F, Dimopoulos G. Implication of the Mosquito Midgut Microbiota in the Defense Against Malaria Parasites. PloS Pathog (2009) 5(5):e1000423. doi: 10.1371/journal.ppat.1000423
10. Apte-Deshpande AD, Paingankar MS, Gokhale MD, Deobagkar DN. Serratia Odorifera Mediated Enhancement in Susceptibility of Aedes Aegypti for Chikungunya Virus. Indian J Med Res (2014) 139(5):762–8.
11. Wu P, Sun P, Nie K, Zhu Y, Shi M, Xiao C, et al. A Gut Commensal Bacterium Promotes Mosquito Permissiveness to Arboviruses. Cell Host Microbe (2019) 25(1):101–12.e5. doi: 10.1016/j.chom.2018.11.004
12. Dutra HL, Rocha MN, Dias FB, Mansur SB, Caragata EP, Moreira LA. Wolbachia Blocks Currently Circulating Zika Virus Isolates in Brazilian Aedes Aegypti Mosquitoes. Cell Host Microbe (2016) 19(6):771–4. doi: 10.1016/j.chom.2016.04.021
13. Moreira LA, Iturbe-Ormaetxe I, Jeffery JA, Lu G, Pyke AT, Hedges LM, et al. A Wolbachia Symbiont in Aedes Aegypti Limits Infection With Dengue, Chikungunya, and Plasmodium. Cell (2009) 139(7):1268–78. doi: 10.1016/j.cell.2009.11.042
14. Ramirez JL, Short SM, Bahia AC, Saraiva RG, Dong Y, Kang S, et al. Chromobacterium Csp_P Reduces Malaria and Dengue Infection in Vector Mosquitoes and Has Entomopathogenic and In Vitro Anti-Pathogen Activities. PloS Pathog (2014) 10(10):e1004398. doi: 10.1371/journal.ppat.1004398
15. Coon KL, Vogel KJ, Brown MR, Strand MR. Mosquitoes Rely on Their Gut Microbiota for Development. Mol Ecol (2014) 23(11):2727–39. doi: 10.1111/mec.12771
16. Coon KL, Valzania L, McKinney DA, Vogel KJ, Brown MR, Strand MR. Bacteria-Mediated Hypoxia Functions as a Signal for Mosquito Development. Proc Natl Acad Sci USA (2017) 114(27):E5362–E9. doi: 10.1073/pnas.1702983114
17. Wang Y, Eum JH, Harrison RE, Valzania L, Yang X, Johnson JA, et al. Riboflavin Instability Is a Key Factor Underlying the Requirement of a Gut Microbiota for Mosquito Development. Proc Natl Acad Sci USA (2021) 118(15):e2101080118. doi: 10.1073/pnas.2101080118
18. Correa MA, Matusovsky B, Brackney DE, Steven B. Generation of Axenic Aedes Aegypti Demonstrate Live Bacteria Are Not Required for Mosquito Development. Nat Commun (2018) 9(1):4464. doi: 10.1038/s41467-018-07014-2
19. Valzania L, Martinson VG, Harrison RE, Boyd BM, Coon KL, Brown MR, et al. Both Living Bacteria and Eukaryotes in the Mosquito Gut Promote Growth of Larvae. PloS Negl Trop Dis (2018) 12(7):e0006638. doi: 10.1371/journal.pntd.0006638
20. Gaio A, Gusmao DS, Santos AV, Berbert-Molina MA, Pimenta PF, Lemos FJ. Contribution of Midgut Bacteria to Blood Digestion and Egg Production in Aedes Aegypti (Diptera: Culicidae) (L.). Parasit Vectors (2011) 4:105. doi: 10.1186/1756-3305-4-105
21. Mitraka E, Stathopoulos S, Siden-Kiamos I, Christophides GK, Louis C. Asaia Accelerates Larval Development of Anopheles Gambiae. Pathog Glob Health (2013) 107(6):305–11. doi: 10.1179/2047773213Y.0000000106
22. Pimenta de Oliveira S, Dantas de Oliveira C, Viana Sant'Anna MR, Carneiro Dutra HL, Caragata EP, Moreira LA. Wolbachia Infection in Aedes Aegypti Mosquitoes Alters Blood Meal Excretion and Delays Oviposition Without Affecting Trypsin Activity. Insect Biochem Mol Biol (2017) 87:65–74. doi: 10.1016/j.ibmb.2017.06.010
23. Walker T, Johnson PH, Moreira LA, Iturbe-Ormaetxe I, Frentiu FD, McMeniman CJ, et al. The Wmel Wolbachia Strain Blocks Dengue and Invades Caged Aedes Aegypti Populations. Nature (2011) 476(7361):450–3. doi: 10.1038/nature10355
24. Turley AP, Moreira LA, O'Neill SL, McGraw EA. Wolbachia Infection Reduces Blood-Feeding Success in the Dengue Fever Mosquito, Aedes Aegypti. PloS Negl Trop Dis (2009) 3(9):e516. doi: 10.1371/journal.pntd.0000516
25. David MR, Santos LM, Vicente AC, Maciel-de-Freitas R. Effects of Environment, Dietary Regime and Ageing on the Dengue Vector Microbiota: Evidence of a Core Microbiota Throughout Aedes Aegypti Lifespan. Mem Inst Oswaldo Cruz (2016) 111(9):577–87. doi: 10.1590/0074-02760160238
26. Wang Y, Gilbreath TM 3rd, Kukutla P, Yan G, Xu J. Dynamic Gut Microbiome Across Life History of the Malaria Mosquito Anopheles Gambiae in Kenya. PloS One (2011) 6(9):e24767. doi: 10.1371/journal.pone.0024767
27. Scolari F, Sandionigi A, Carlassara M, Bruno A, Casiraghi M, Bonizzoni M. Exploring Changes in the Microbiota of Aedes Albopictus: Comparison Among Breeding Site Water, Larvae, and Adults. Front Microbiol (2021) 12:624170. doi: 10.3389/fmicb.2021.624170
28. Galeano-Castañeda Y, Bascunan P, Serre D, Correa MM. Trans-Stadial Fate of the Gut Bacterial Microbiota in Anopheles Albimanus. Acta Trop (2020) 201:105204. doi: 10.1016/j.actatropica.2019.105204
29. Dickson LB, Ghozlane A, Volant S, Bouchier C, Ma L, Vega-Rua A, et al. Diverse Laboratory Colonies of Aedes Aegypti Harbor the Same Adult Midgut Bacterial Microbiome. Parasit Vectors (2018) 11(1):207. doi: 10.1186/s13071-018-2780-1
30. Akorli J, Gendrin M, Pels NA, Yeboah-Manu D, Christophides GK, Wilson MD. Seasonality and Locality Affect the Diversity of Anopheles Gambiae and Anopheles Coluzzii Midgut Microbiota From Ghana. PloS One (2016) 11(6):e0157529. doi: 10.1371/journal.pone.0157529
31. Didion EM, Doyle M, Benoit JB. Bacterial Communities of Lab and Field Northern House Mosquitoes (Diptera: Culicidae) Throughout Diapause. J Med Entomol (2022) 59(2):648–58. doi: 10.1093/jme/tjab184
32. Duguma D, Hall MW, Smartt CT, Neufeld JD. Temporal Variations of Microbiota Associated With the Immature Stages of Two Florida Culex Mosquito Vectors. Microb Ecol (2017) 74(4):979–89. doi: 10.1007/s00248-017-0988-9
33. Minard G, Tran FH, Tran Van V, Fournier C, Potier P, Roiz D, et al. Shared Larval Rearing Environment, Sex, Female Size and Genetic Diversity Shape Ae. Albopictus Bacterial Microbiota. PloS One (2018) 13(4):e0194521. doi: 10.1371/journal.pone.0194521
34. Boissière A, Tchioffo MT, Bachar D, Abate L, Marie A, Nsango SE, et al. Midgut Microbiota of the Malaria Mosquito Vector Anopheles Gambiae and Interactions With Plasmodium Falciparum Infection. PloS Pathog (2012) 8(5):e1002742. doi: 10.1371/journal.ppat.1002742
35. Buck M, Nilsson LK, Brunius C, Dabire RK, Hopkins R, Terenius O. Bacterial Associations Reveal Spatial Population Dynamics in Anopheles Gambiae Mosquitoes. Sci Rep (2016) 6:22806. doi: 10.1038/srep22806
36. Muturi EJ, Kim CH, Bara J, Bach EM, Siddappaji MH. Culex Pipiens and Culex Restuans Mosquitoes Harbor Distinct Microbiota Dominated by Few Bacterial Taxa. Parasit Vectors (2016) 9:18. doi: 10.1186/s13071-016-1299-6
37. Bennett KL, Gomez-Martinez C, Chin Y, Saltonstall K, McMillan WO, Rovira JR, et al. Dynamics and Diversity of Bacteria Associated With the Disease Vectors Aedes Aegypti and Aedes Albopictus. Sci Rep (2019) 9(1):12160. doi: 10.1038/s41598-019-48414-8
38. Osei-Poku J, Mbogo CM, Palmer WJ, Jiggins FM. Deep Sequencing Reveals Extensive Variation in the Gut Microbiota of Wild Mosquitoes From Kenya. Mol Ecol (2012) 21(20):5138–50. doi: 10.1111/j.1365-294X.2012.05759.x
39. Caragata EP, Otero LM, Tikhe CV, Barrera R, Dimopoulos G. Microbial Diversity of Adult Aedes Aegypti and Water Collected From Different Mosquito Aquatic Habitats in Puerto Rico. Microb Ecol (2021) 83(1):182–201.
40. Hery L, Guidez A, Durand AA, Delannay C, Normandeau-Guimond J, Reynaud Y, et al. Natural Variation in Physicochemical Profiles and Bacterial Communities Associated With Aedes Aegypti Breeding Sites and Larvae on Guadeloupe and French Guiana. Microb Ecol (2021) 81(1):93–109. doi: 10.1007/s00248-020-01544-3
41. Muturi EJ, Lagos-Kutz D, Dunlap C, Ramirez JL, Rooney AP, Hartman GL, et al. Mosquito Microbiota Cluster by Host Sampling Location. Parasit Vectors (2018) 11(1):468. doi: 10.1186/s13071-018-3036-9
42. Bogale HN, Cannon MV, Keita K, Camara D, Barry Y, Keita M, et al. Relative Contributions of Various Endogenous and Exogenous Factors to the Mosquito Microbiota. Parasit Vectors (2020) 13(1):619. doi: 10.1186/s13071-020-04491-7
43. Muturi EJ, Dunlap C, Ramirez JL, Rooney AP, Kim CH. Host Blood-Meal Source has a Strong Impact on Gut Microbiota of Aedes Aegypti. FEMS Microbiol Ecol (2019) 95(1). doi: 10.1093/femsec/fiy213
44. Burkett-Cadena N. Mosquitoes of the Southeastern United States. 1 ed. Tuscaloosa, Alabama: The University of Alabama Press (2013).
45. Darsie RFJ, Morris CD. Keys to the Adult Females and Fourth Instar Larvae of the Mosquitoes of Florida (Diptera, Culicidae). DeLeon Springs, Florida: The Florida Mosquito Control Association (2003).
46. Darsie RFJ, Ward RA. Identification and Geographical Distribution of the Mosquitoes of North America, North of Mexico. Gainesville, Florida: University Press of Florida/State University System (2005).
47. Harrison BA, Byrd BD, Sither CB, Whit PB. The Mosquitoes of the Mid-Atlantic Region: An Identification Guide. 1 ed. Madison Heights, Michigan: Publishing XPress (2016).
48. Wilkerson RC, Linton Y-M, Strickman D. Mosquitoes of the World. 1 ed. Baltimore, Maryland: Johns Hopkins University Press (2021).
49. Glassing A, Dowd SE, Galandiuk S, Davis B, Jorden JR, Chiodini RJ. Changes in 16s RNA Gene Microbial Community Profiling by Concentration of Prokaryotic DNA. J Microbiol Methods (2015) 119:239–42. doi: 10.1016/j.mimet.2015.11.001
50. Novakova E, Woodhams DC, Rodriguez-Ruano SM, Brucker RM, Leff JW, Maharaj A, et al. Mosquito Microbiome Dynamics, A Background for Prevalence and Seasonality of West Nile Virus. Front Microbiol (2017) 8:526. doi: 10.3389/fmicb.2017.00526
51. Hegde S, Khanipov K, Albayrak L, Golovko G, Pimenova M, Saldana MA, et al. Microbiome Interaction Networks and Community Structure From Laboratory-Reared and Field-Collected Aedes Aegypti, Aedes Albopictus, and Culex Quinquefasciatus Mosquito Vectors. Front Microbiol (2018) 9:2160. doi: 10.3389/fmicb.2018.02160
52. Singh S, Singh A, Baweja V, Roy A, Chakraborty A, Singh IK. Molecular Rationale of Insect-Microbes Symbiosis-From Insect Behaviour to Mechanism. Microorganisms (2021) 9(12):2422. doi: 10.3390/microorganisms9122422
53. Endo A, Irisawa T, Futagawa-Endo Y, Takano K, du Toit M, Okada S, et al. Characterization and Emended Description of Lactobacillus Kunkeei as a Fructophilic Lactic Acid Bacterium. Int J Syst Evol Microbiol (2012) 623):500–4. doi: 10.1099/ijs.0.031054-0
54. Alvarez-Pérez S, Lievens B, Jacquemyn H, Herrera CM. Acinetobacter Nectaris Sp. Nov. And Acinetobacter Boissieri Sp. Nov., Isolated From Floral Nectar of Wild Mediterranean Insect-Pollinated Plants. Int J Syst Evol Microbiol (2013) 634):1532–9. doi: 10.1099/ijs.0.043489-0
55. Kim PS, Shin NR, Kim JY, Yun JH, Hyun DW, Bae JW. Acinetobacter Apis Sp. Nov., Isolated From the Intestinal Tract of a Honey Bee, Apis Mellifera. J Microbiol (2014) 52(8):639–45. doi: 10.1007/s12275-014-4078-0
56. Leclerc H, Schwartzbrod L, Dei-Cas E. Microbial Agents Associated With Waterborne Diseases. Crit Rev Microbiol (2002) 28(4):371–409. doi: 10.1080/1040-840291046768
57. Leta S, Assefa F, Dalhammar G. Enhancing Biological Nitrogen Removal From Tannery Effluent by Using the Efficient Brachymonas Denitrificans in Pilot Plant Operations. World J Microb Biot (2005) 21(4):545–52. doi: 10.1007/s11274-004-3272-3
58. Edman JD, Bidlingmayer WL. Flight Capacity of Blood-Engorged Mosquitoes. Mosquito News (1969) 29(3):386–92.
59. Metz CW. Anopheles Crucians: Habits of Larvæ and Adults. Public Health Reports (1896-1970). Public Health Reports (1918) 33(49):2156–69. doi: 10.2307/4574958
60. Provost MW. The Dispersal of Aedes Taeniorhynchus. 1. Preliminary Studies. Mosquito News (1952) 12(3):174–90.
61. Alencar J, Mello CF, Barbosa LS, Gil-Santana HR, Maia Dde A, Marcondes CB, et al. Diversity of Yellow Fever Mosquito Vectors in the Atlantic Forest of Rio De Janeiro, Brazil. Rev Soc Bras Med Trop (2016) 49(3):351–6. doi: 10.1590/0037-8682-0438-2015
62. Juliāo GR, Abad-Franch F, Lourenco-De-Oliveira R, Luz SL. Measuring Mosquito Diversity Patterns in an Amazonian Terra Firme Rain Forest. J Med Entomol (2010) 47(2):121–8. doi: 10.1603/ME09060
63. Wilk da Silva R, Mucci LF, Ceretti-Junior W, Duarte A, Marrelli MT, Medeiros-Sousa AR. Influence of Landscape Composition and Configuration on the Richness and Abundance of Potential Sylvatic Yellow Fever Vectors in a Remnant of Atlantic Forest in the City of Sao Paulo, Brazil. Acta Trop (2020) 204:105385. doi: 10.1016/j.actatropica.2020.105385
Keywords: mosquito, vector, microbiota, 16s rRNA profiling, environmental variation, Aedes taeniorhynchus, Anopheles crucians, Culex nigripalpus
Citation: Pérez-Ramos DW, Ramos MM, Payne KC, Giordano BV and Caragata EP (2022) Collection Time, Location, and Mosquito Species Have Distinct Impacts on the Mosquito Microbiota. Front. Trop. Dis 3:896289. doi: 10.3389/fitd.2022.896289
Received: 14 March 2022; Accepted: 01 April 2022;
Published: 11 May 2022.
Edited by:
Adam E. Vorsino, United States Fish and Wildlife Service, United StatesReviewed by:
Priscilla Seabourn, Association of Public Health Laboratories, United StatesCopyright © 2022 Pérez-Ramos, Ramos, Payne, Giordano and Caragata. This is an open-access article distributed under the terms of the Creative Commons Attribution License (CC BY). The use, distribution or reproduction in other forums is permitted, provided the original author(s) and the copyright owner(s) are credited and that the original publication in this journal is cited, in accordance with accepted academic practice. No use, distribution or reproduction is permitted which does not comply with these terms.
*Correspondence: Eric P. Caragata, ZS5jYXJhZ2F0YUB1ZmwuZWR1
†These authors have contributed equally to this work and share first authorship
Disclaimer: All claims expressed in this article are solely those of the authors and do not necessarily represent those of their affiliated organizations, or those of the publisher, the editors and the reviewers. Any product that may be evaluated in this article or claim that may be made by its manufacturer is not guaranteed or endorsed by the publisher.
Research integrity at Frontiers
Learn more about the work of our research integrity team to safeguard the quality of each article we publish.