- 1Department of Biotechnology and Biomedicine, Technical University of Denmark, Kongens Lyngby, Denmark
- 2Department of Applied Mathematics and Computer Science, Technical University of Denmark, Kongens Lyngby, Denmark
- 3Instituto Clodomiro Picado, Facultad de Microbiología, Universidad de Costa Rica, San José, Costa Rica
- 4Warwick Medical School, The University of Warwick, Coventry, United Kingdom
- 5Diversity and Prototyping, Novozymes, Kongens Lyngby, Denmark
Venom-induced haemorrhage constitutes a severe pathology in snakebite envenomings, especially those inflicted by viperid species. To both explore venom activity accurately and evaluate the efficacy of viperid antivenoms for the neutralisation of haemorrhagic activity it is essential to have available a precise, quantitative tool for empirically determining venom-induced haemorrhage. Thus, we have built on our prior approach and developed a new AI-guided tool (AHA) for the quantification of venom-induced haemorrhage in mice. Using a smartphone, it takes less than a minute to take a photo, upload the image, and receive accurate information on the magnitude of a venom-induced haemorrhagic lesion in mice. This substantially decreases analysis time, reduces human error, and does not require expert haemorrhage analysis skills. Furthermore, its open access web-based graphical user interface makes it easy to use and implement in laboratories across the globe. Together, this will reduce the resources required to preclinically assess and control the quality of antivenoms, whilst also expediting the profiling of haemorrhagic activity in venoms for the wider toxinology community.
1 Introduction
Snakebite envenoming is a major public health problem, especially in the developing world (1). Indeed, it is responsible for substantial morbidity and mortality, particularly in the impoverished areas of sub-Saharan Africa, South to Southeast Asia, Papua New Guinea, and Latin America (1–4). Whilst accurate estimates are difficult to make, it is believed that between 1.8–2.7 million people worldwide are envenomed each year, resulting in 80,000 to 140,000 deaths and 400,000 survivors left with permanent sequelae, such as amputated limbs, deformities, or hypertrophic scarring (5–7).
The severity of a given envenoming is determined by several factors, such as the amount of venom injected, the anatomical location of the bite, and the physiological status of the victim (8). In addition, there is a great variability in the composition of the venoms and the predominant toxins present in different venoms, not only between genera, but also within a single species of snake (9). Consequently, the clinical manifestations (e.g., neurotoxicity, necrosis, and haemorrhage) and pathophysiological effects of envenomings can vary greatly depending on the offending snake species (10). Haemorrhage is characteristic of envenomings by many snakes, predominantly by species belonging to the family Viperidae, but also some from the family Colubridae (sensu lato); these induce local and systemic haemorrhage, further causing local tissue damage and cardiovascular disturbances (8, 11, 12). Specifically, blood vessel damage leads to extravasation, which contributes to local tissue damage and poor muscle regeneration. In addition, massive systemic haemorrhage contributes to haemodynamic disturbances and cardiovascular shock (8, 13). Consequently, the underlying mechanisms by which snake venoms induce haemorrhage, the characterisation of haemorrhagic toxins, and the clinical manifestations of envenomings have, for a long time, presented a key area of fundamental, but also translational research within the field of Toxinology (11). Venom-induced haemorrhage is mainly the consequence of the damage induced by snake venom metalloproteinases (SVMPs) on the microvasculature, due to the enzymatic degradation of key structural components in the basement membrane of capillary vessels (12). The haemorrhagic activity of venoms is further potentiated by the action of these and other venom toxins that affect haemostasis, which induce consumption coagulopathy, thrombocytopenia, and platelet hypoaggregation (1, 14).
The only specific treatment currently available for snakebite envenomings is the intravenous administration of animal-derived antivenom (8, 15). Importantly, each batch of antivenom that is produced needs to undergo rigorous quality control; this includes the assessment of the ability of antivenoms to neutralise the lethal effect of venoms in mice (16, 17). In addition, and owing to the complex pathophysiology of snakebite envenomings, other relevant effects, such as the antivenom’s neutralising potential of venom-induced haemorrhage, are also part of the preclinical assessment of antivenom efficacy (16). The most widely used method for analysing haemorrhage is the skin test originally developed in rabbits (18), and later on adapted for use in rats (19) and mice (20). In the adaptation of this method for mice, a range of different venom concentrations are injected intradermally in the abdominal region. After a predefined time interval, mice are euthanised and carefully dissected to allow the size and intensity assessment of the wound on the inner surface of the skin. Originally, this was followed solely by a rough manual measurement of the area of the haemorrhagic lesion. Since this method did not take into account the intensity of the lesion, a computationally assisted update to this method was reported in 2017, in which an image of the lesion is taken, and both the size and the intensity are measured accurately, thus providing a more systematic and quantitative evaluation of extravasation (21). The study also introduced a new unit for the assessment of the severity of a given venom-induced haemorrhagic lesion, i.e. the haemorrhagic unit (HaU) (21). Whilst this method presented an improvement in accuracy and speed of the quantification of snake venom-induced haemorrhagic activity, it still required manual identification of the lesions and specialised equipment. It thus remained subject to human error and was not optimally accessible to researchers across the globe.
Thus, we present a new and more accessible artificial intelligence (AI)-guided tool for the automatic assessment of venom-induced haemorrhage, AHA (Ai Haemorrhage Analysis; https://github.com/laprade117/AHA). We trained a machine learning algorithm to automatically identify haemorrhagic lesions, adjust for lighting biases, scale the image, extract lesion area and intensity, and calculate the HaUs. Finally, we evaluate the performance of this algorithm and discuss its utility in relation to rapid, robust, and semi-automated assessment of snake venom-induced haemorrhage.
2 Methods
2.1 Snake venom
The venom of Bothrops asper was used in this study since its haemorrhagic activity has been widely studied. Venom of B. asper (batch number 03–06 Bap P) was obtained from adult specimens captured in the Pacific region of Costa Rica and maintained in captivity at the Serpentarium of Instituto Clodomiro Picado, Universidad de Costa Rica, San José, Costa Rica. Samples of venom correspond to pools obtained from many adult specimens and were stabilised by lyophilisation and stored at −20°C. Solutions of venoms in 0.12 M NaCl, 0.04 M phosphate, pH 7.2 buffer (PBS) were prepared immediately before use.
2.2 Haemorrhagic activity
Haemorrhagic activity was assessed following the method described by Jenkins et al. (21) with some modifications (c.f. below). Briefly, groups of four mice of both sexes (18–20 g; CD-1 strain) were injected intradermally with different amounts of B. asper venom (1, 2, 4, 8, 16 μg) dissolved in 100 μL PBS. Two hours after injection, mice were sacrificed by CO2 inhalation, and their skin was dissected. Mice were first placed on a standardised A4 printout template sheet to measure the haemorrhagic lesion on the inner surface of the skin, using the method described below; then the same mice were also placed on the table without the template printout sheet and with a colour pantone (21), with pictures being taken for both approaches. All experiments involving the use of mice were approved by the Institutional Committee for the Care and Use of Laboratory Animals (CICUA) of the University of Costa Rica (approval number CICUA 82-08).
2.3 Printout sheet
To allow for a standardised analysis of the haemorrhagic lesions, as well as to facilitate the image analysis algorithms, we prepared an A4 printout sheet which the mice were placed on. This sheet outlines where to place the mice and includes different lines and boxes of defined lengths that allow for the scaling of the image (Figure 1). We also used a cut out mask to be placed on the mice to facilitate lesion identification (Figure 1). Printable versions of these two components can be found in supplementary Figures 1, 2.
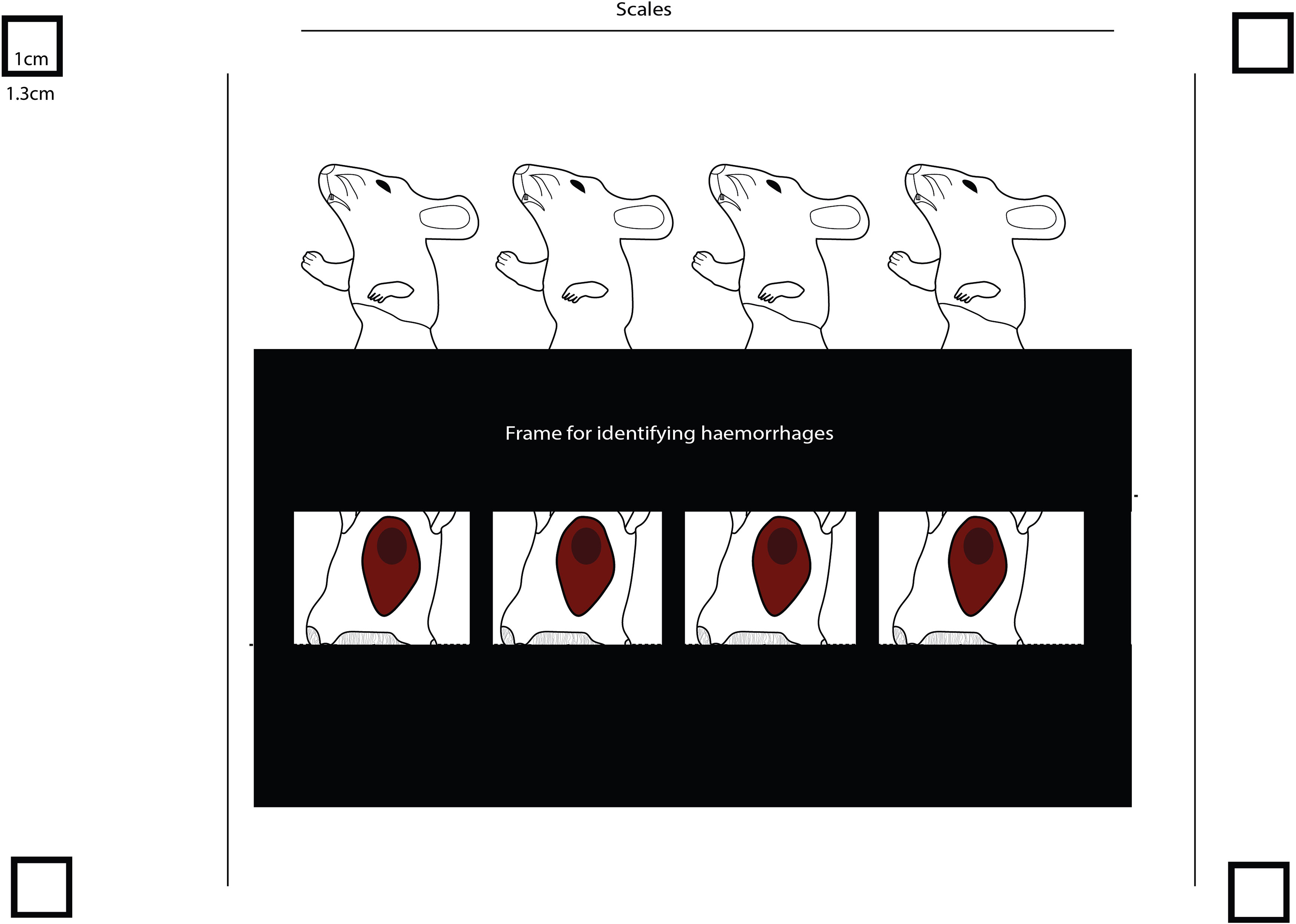
Figure 1 Explanation of the printable template upon which the mice should be placed. The multiple scales across the page allow for the automatic scaling by the tool and take into account pictures obtained from different angles. The black frame acts as a cut out mask to facilitate the automatic identification of haemorrhagic lesions.
2.4 Description of machine learning guided approach of quantifying haemorrhagic activity
We trained a machine learning algorithm to automatically identify haemorrhagic lesions, adjust for lighting biases, scale the image, extract haemorrhagic lesion area and intensity, and calculate the HaUs. This was then implemented in an accessible fashion via a graphical user interface (GUI) as the AHA tool (Figure 2).
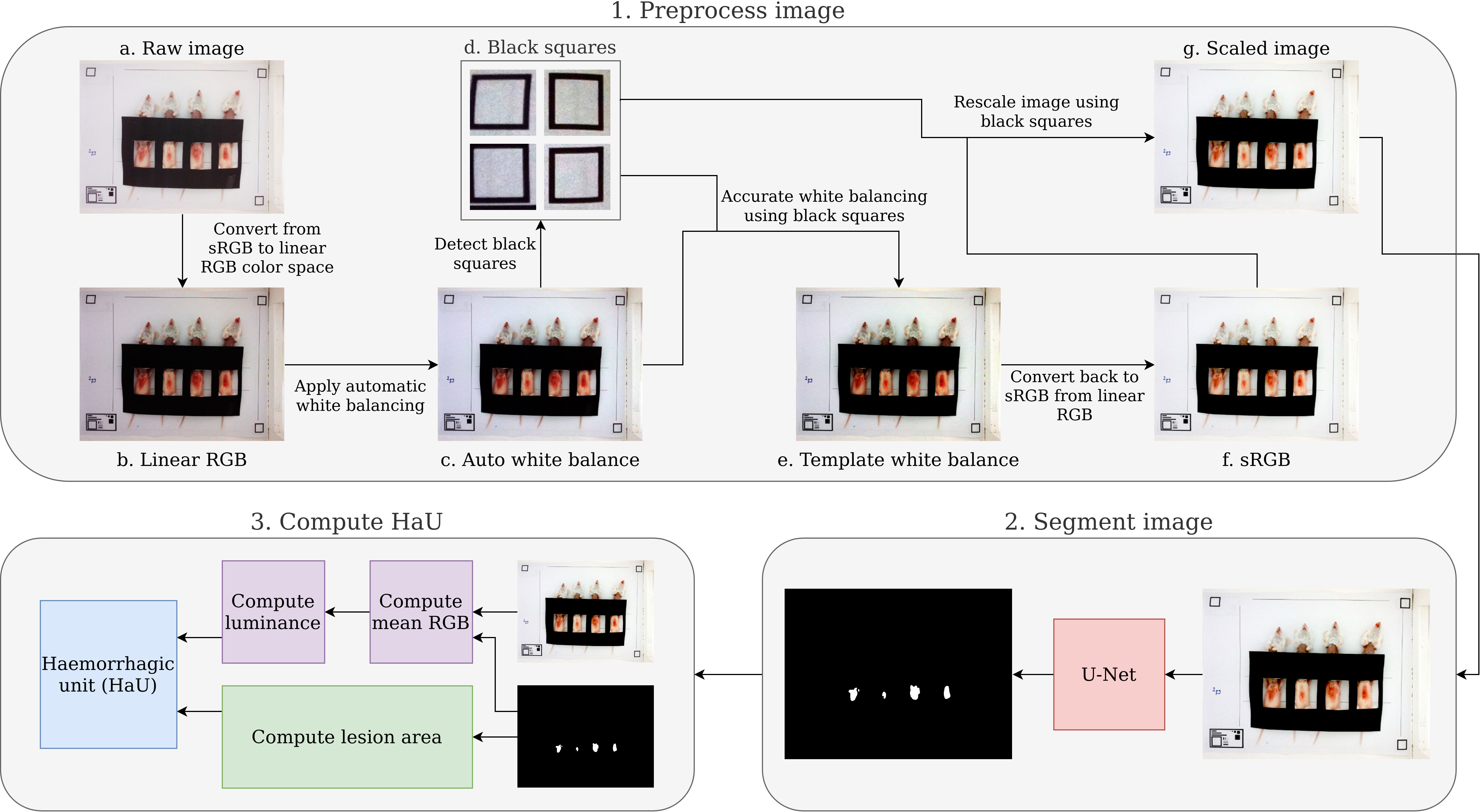
Figure 2 Overview of the workflow for AHA. First, the raw image is imported and converted from sRGB to linear RGB. Thereafter, the image is white balanced and subsequently further white balanced using the colour of the paper detected via the scaling squares. In parallel, the image is rescaled using the same squares. This processed image is then used for segmentation and automatic identification of the haemorrhagic lesions. Together, this information is used to compute the lesion area and luminance, which is then combined into a HaU score to assess the overall haemorrhagic lesion.
2.4.1 Conversion to linear RGB and white balancing
To create reproducible results across images, it is necessary to white balance the images prior to computing the HaUs. First, the images were converted to a linear RGB colour space as in Jenkins et al., 2017 (21), then auto white balancing, based on the method used in the GNU Image Manipulation Program (https://www.gimp.org), was applied. For images that use our template, we applied a second white balancing step for higher accuracy using information extracted from the template image. Firstly, we detected the black boxes in the four corners of the image using a template matching algorithm. We then computed a white point based upon the mean colour of the pixels located within each of the four boxes and a black point based upon the mean colour of the pixels in the black borders of these boxes. The image is then white balanced via the following formula:
This formula does require the assumption that the paper which the template has been printed on is some value of grey, (i.e. the RGB values are all identical).
2.4.2 Segmentation
To identify and segment the haemorrhagic lesions in images, a deep learning method based on the widely-used U-Net architecture was applied (22). We also included a few modifications based upon more recent findings. Namely, we replaced the deconvolution layers in the expanding path with bilinear upsampling followed by a 2x2 convolution and included batch normalisation layers (23, 24). The resulting architecture contains approximately 31 million trainable parameters.
Our dataset consisted of 29 training images taken via smartphone. Each image contains between 1 and 32 mice displaying varying levels of haemorrhagic damage for a total of 217 mice. To limit annotator bias, each image was annotated by two different annotators, resulting in two masks per image. For evaluating performance, we set aside 20% of the images at random as a validation test set. Thereafter, a 5-fold cross-validation was performed on the remaining 23 images, evaluating model performance on the validation test set for each fold. This was repeated 5 times to avoid test-set bias.
At the time of training, the images were split into samples of size 256 x 256 pixels and fed into the model in batches of 32 samples. Batches were created such that each sample had a 75% chance of having a masked section of haemorrhagic tissue according to at least one annotator. The masks used for training were sampled from the set of annotators at random. Data augmentations included flips, rotations, noise, blurring, sharpening, distortions, brightness, contrast, hue, and saturation adjustments. Augmentations were selected to simulate the possible variation lighting environments.
The models were trained using the Adam optimizer with a learning rate of 0.0001 for 100 epochs. We used a loss function based upon a combination of the Matthews correlation coefficient (MCC) and cross-entropy (25). We report the average F1 (Dice), MCC, and accuracy for each model as computed on the test set. F1/Dice is the harmonic mean of precision and recall and ranges between 0 and 1, where 1 is a perfect score. MCC ranges from -1 to 1, with 1 being a perfect score.
2.4.3 Scaling
It is essential for computing an accurate HaU that the scale of the images is determined. With the template, this can be done automatically by first detecting the black squares (via template matching) in the corners and then determining the number of white pixels inside the black square via thresholding. The inner white region is a 10 mm x 10 mm square, so the pixel resolution can be computed with the formula below.
For improved accuracy, we then averaged the results across the four black squares.
For images without the template, the scale was determined manually by using FIJI’s measure tool on a known distance in the image (26). Once the image scale was determined, we resized all images to ensure a resolution of 5 pixels per mm, which also allows for rapid computation.
2.4.4 Calculation of HaU and minimum haemorrhagic dose
Haemorrhagic units were calculated as described in Jenkins et al., 2017 (21). Briefly, the RGB values and area of a given lesion were extracted and colour/scale adjusted. Thereafter, the luminance (i.e., intensity) was calculated, combined with the area of the lesion, and expressed as HaUs. Thereafter, the minimum haemorrhagic dose (MHD) of B. asper venom was calculated. This calculation was carried out using linear regression on the HaU means. From the resulting function, we calculated the venom dose needed for a 50 HaU signal by replacing the Y with 50 and calculating X. The software used was GraphPad Prism version 9.2.0.
2.4.5 Implementation in GUI
Using Streamlit (https://github.com/streamlit/streamlit) and localtunnel (https://github.com/localtunnel/localtunnel) with Google Colab, a simple web-based application to automatically analyse images was developed (https://github.com/laprade117/AHA). A web-based application seems to be the most efficient way to quickly analyse data while working in the lab. Users can take a photo with a smartphone and upload it to the web-based tool (accessible via a smartphone browser) for an immediate result (Supplementary Figure 3). A full standard operating procedure sheet has also been provided (Supplementary Figure 4).
3 Results
In this study, we primarily present the results for the six images that used the template to evaluate the performance of our fully automated method, AHA, and we compared these results by analysing the same mice using our original method (21). We used, as a model, the haemorrhagic lesions induced by the venom of B. asper on mice.
3.1 White balancing
To address the potential impact of lighting differences, the tool automatically performs white balancing. The white balancing works as expected and produces comparable results across images (Figure 3).
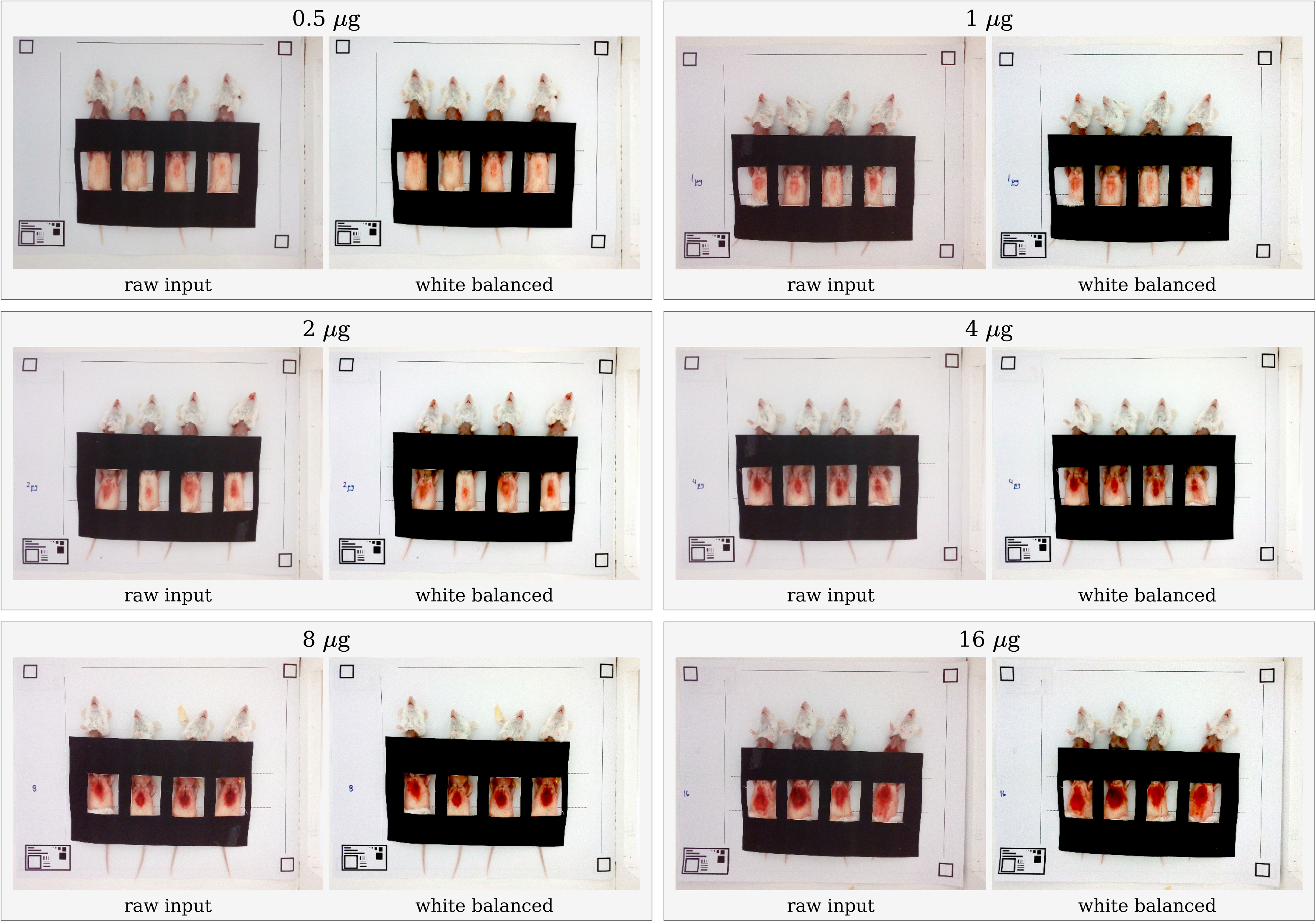
Figure 3 An overview of AHA’s automatic white balancing output across the different test images. The amount of Bothrops asper venom injected into the mice is indicated above each batch of images, where the left represents pre- and the right post-white balancing.
To further stress test the extent to which the white balancing is able to adjust for difficult lighting situations we manipulated the images, randomly simulating different colours. In each simulation, the tool was able to recover a comparable image (Figure 4).
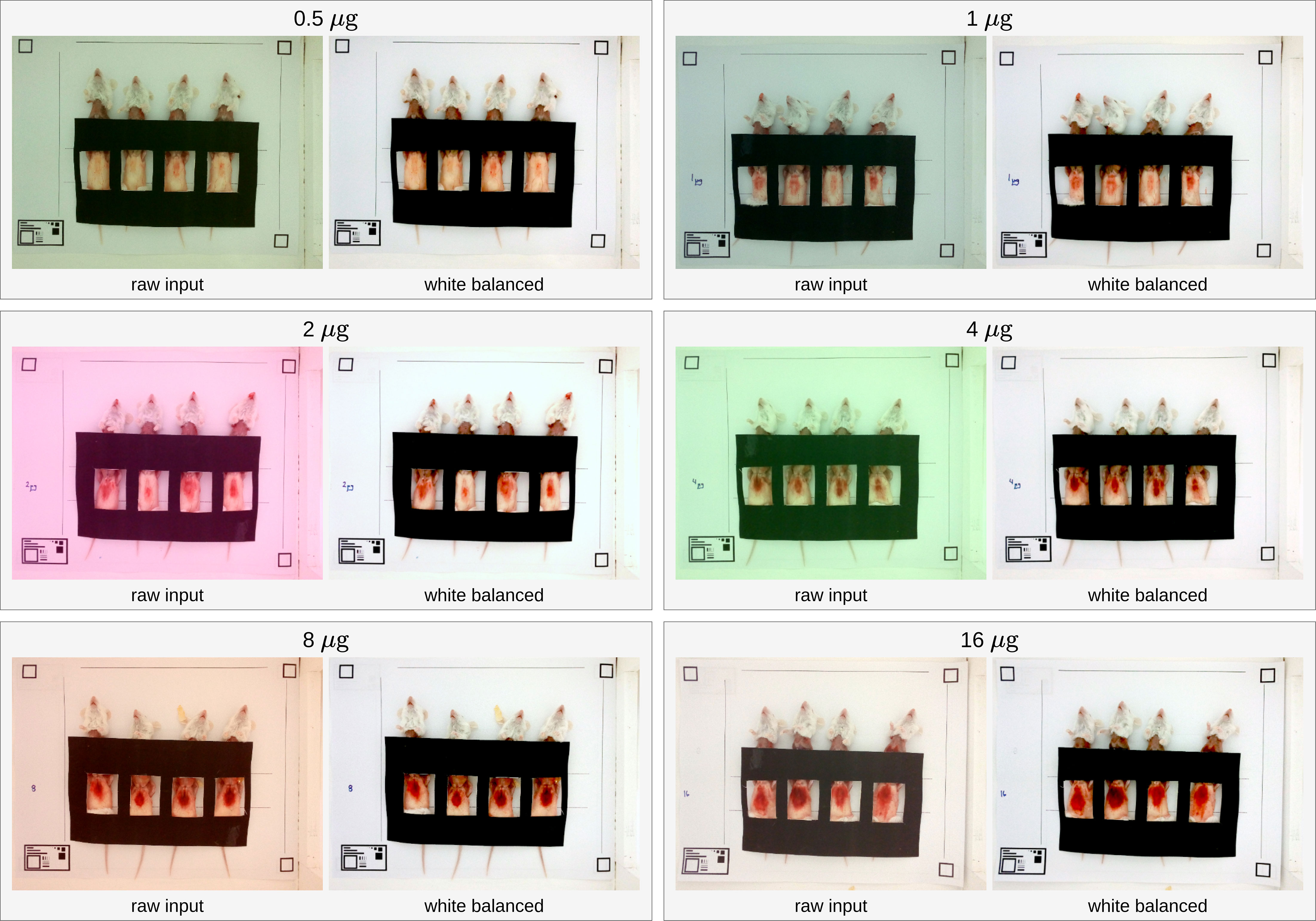
Figure 4 Stress testing AHA’s automatic white balancing capacity. Images were taken and colours randomly manipulated to assess white balancing performance.
3.2 Scaling
Using the scaling method outlined in 2.4.3, the tool automatically detects the image scales and reports them (Table 1).

Table 1 Table outlining the input dimensions, detected scale, target scale, and output dimensions across six test images.
3.3 Segmentation
To automatically identify lesion areas, the tool uses a machine learning guided segmentation approach. Overall, an average MCC score of 0.8612 and an average F1 (Dice) score of 0.9064 was achieved, and we were able to predict 99.84% of the pixels correctly across 25 runs (Figure 5).
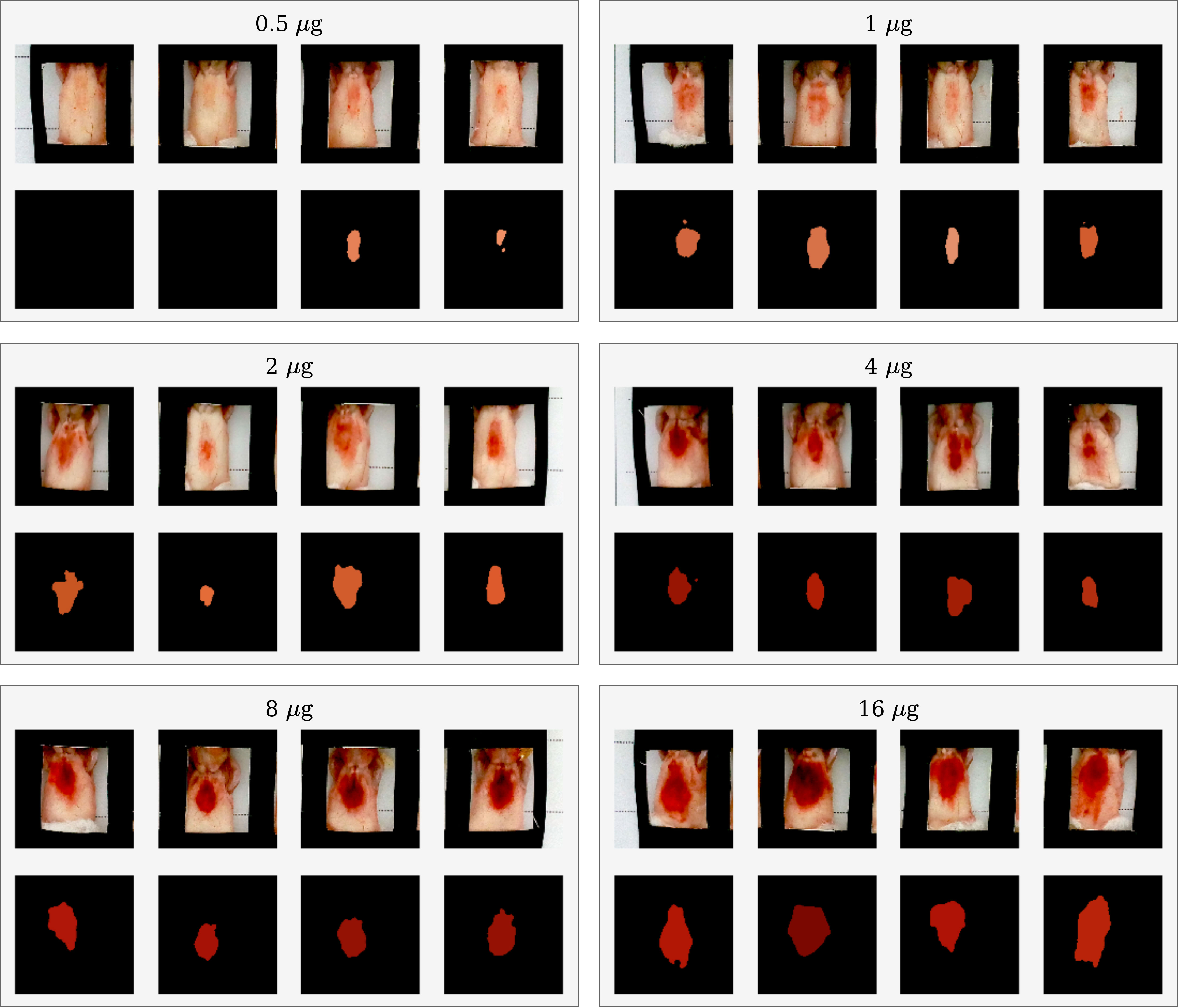
Figure 5 Segmentation of all haemorrhagic lesions across the test images. The segmentations are coloured using the average RGB values detected within the lesion area and the amount of Bothrops asper venom injected into the mice indicated above each batch of images.
3.4 Haemorrhagic units
To assess the severity of each lesion, the tool automatically computes the real-world area, luminance, and HaU for each mouse in all of the test images (Tables 2–4). To ensure consistency with our original method we also assessed the lesions of the same mice (different photos including a pantone) using our original method (21) and established that there were no statistically significant differences between our two methods (Table 5).
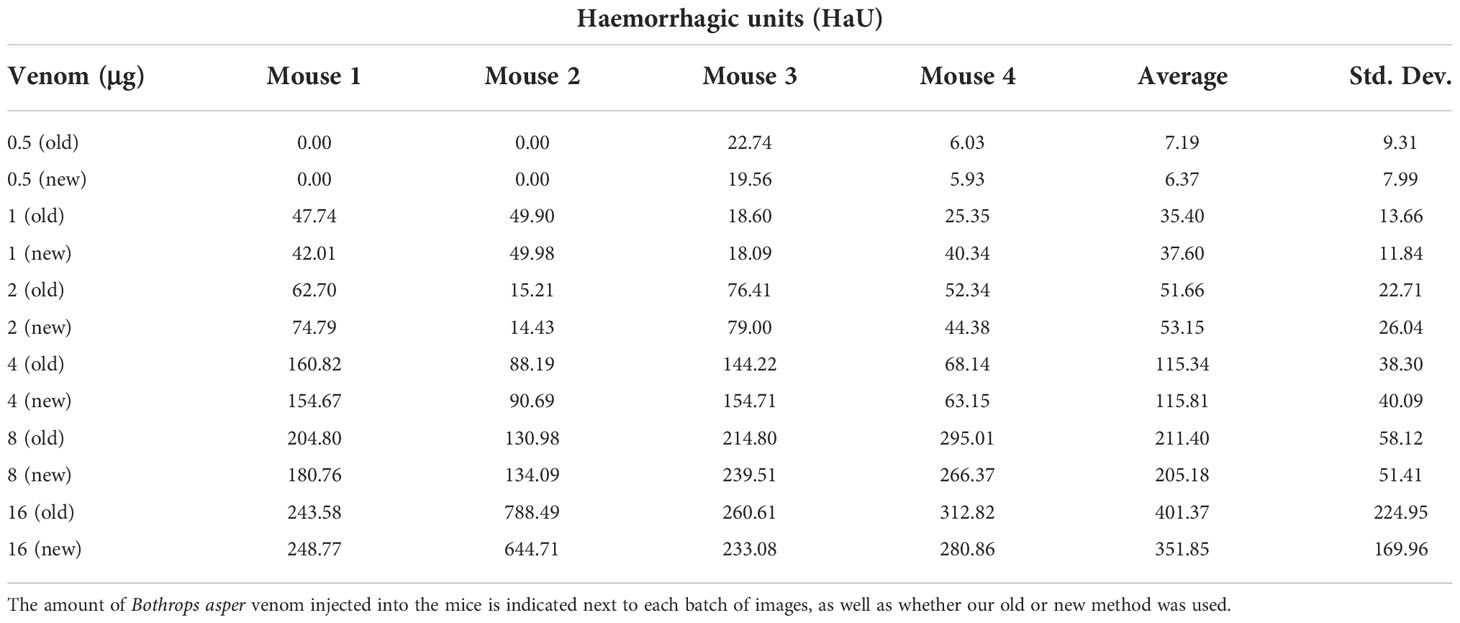
Table 4 Individual and average (across one image) lesion haemorrhagic units for all of the test images.
3.4 Calculation of minimum haemorrhagic dose
Using linear regression (Figure 6), we obtained the function Y=21.83(∓1.18)X+13.73(∓8.91), from which we calculated the MHD on the proposed method from our earlier work (50 HaU) (21). This resulted in a MHD of 1.66 (SD: -0.34, +0.30).
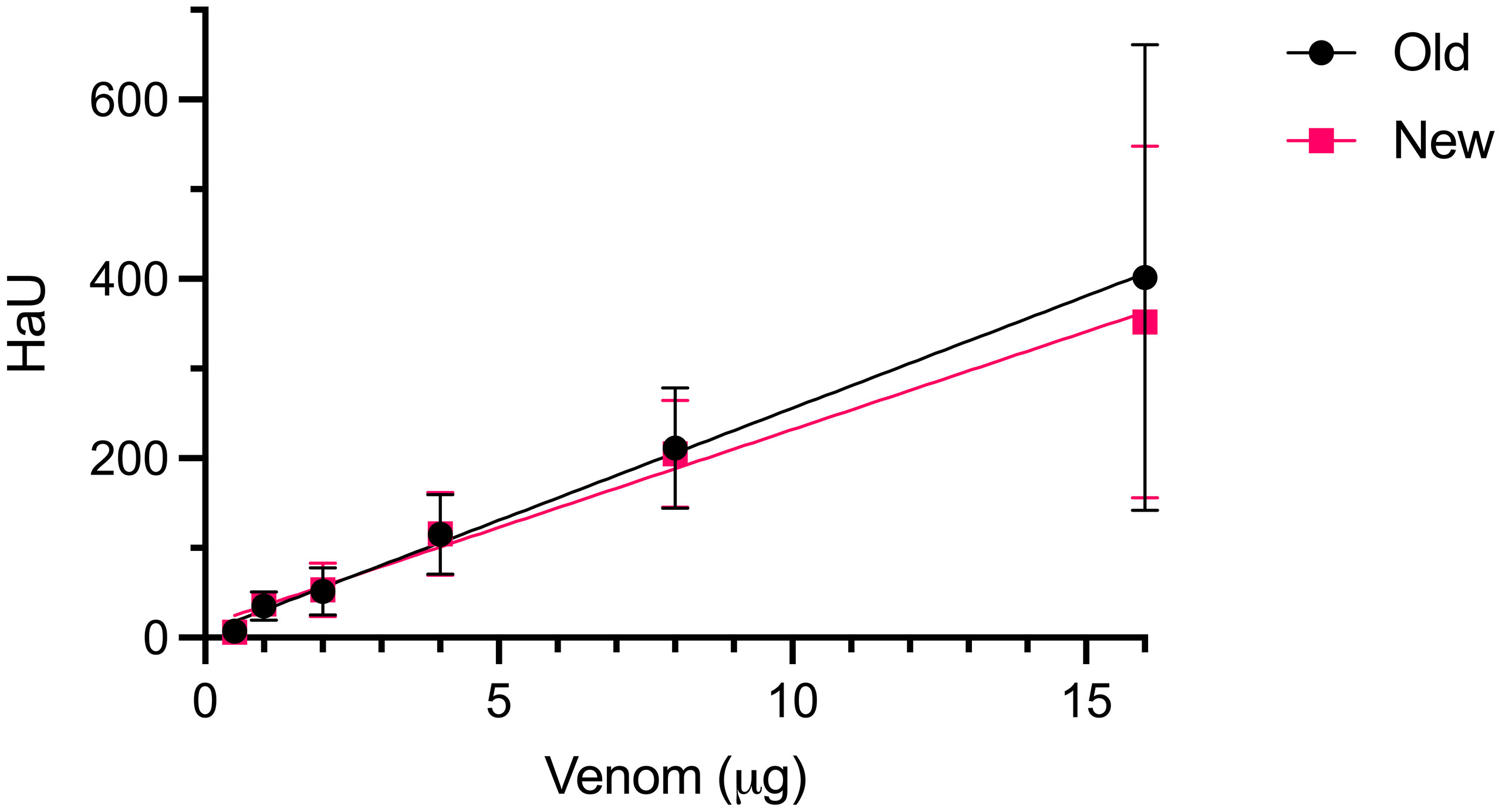
Figure 6 Linear regression of the means calculated from Table 4, with standard deviations shown as bars. The y-axis shows the HaUs and the x-axis shows the amount of venom used for the corresponding HaU level. Each point corresponds to the mean of the 4 mice used and that the bars correspond to the standard deviation. Analysis was carried out using GraphPad Prism version 9.2.0.
3.5 Tool GUI
To ensure accessibility and easy implementation of AHA across research, production, and quality control laboratories, a graphical user interface was developed (https://github.com/laprade117/AHA). Our tool can be used to quickly upload an image and receive statistics on the lesion area, luminance, and HaU for each mouse in the image (Figure 7).
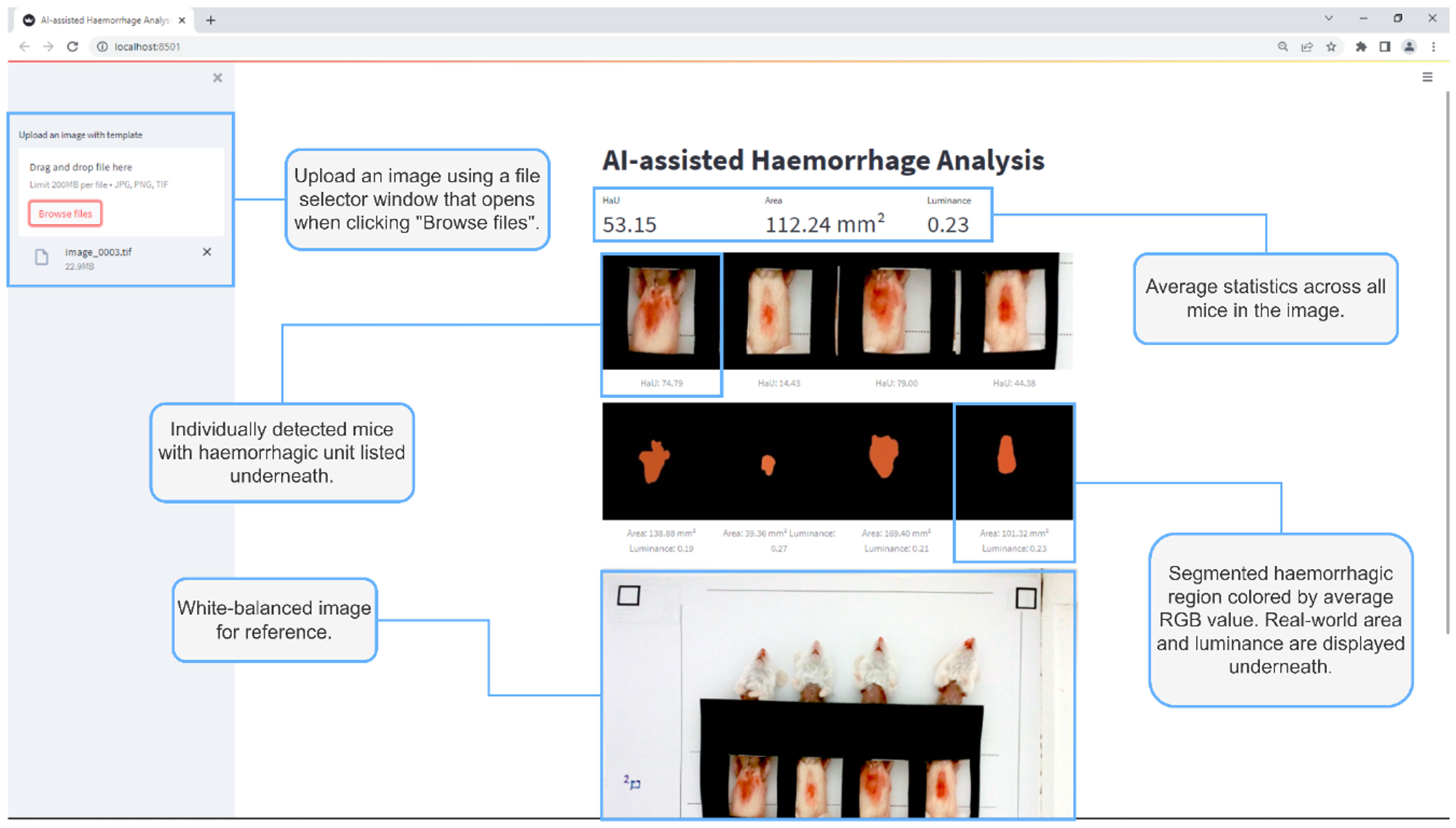
Figure 7 Image of AHA’s web interface. By uploading an image file of the experiment, the tool will, within seconds, provide the white balanced reference image, the individual lesions it detected, and how it decided to segment them, as well as all relevant data on lesion area, luminance, and HaUs.
4 Discussion
Haemorrhage is one of the key pathophysiological manifestations of snakebite envenomings, particularly those inflicted by species of the family Viperidae (1, 11). Therefore, the preclinical assessment of antivenom efficacy includes the evaluation of the neutralisation of haemorrhagic activity (27). This results in both a need for robust and reliable, but also rapid assay approaches, while limiting required resources, which would allow their implementation in diverse laboratory settings. In our earlier study, we were able to improve the classical rodent skin test that either uses calliper measurements or manual lesion outlining by increasing the accuracy of haemorrhage characterisation and reducing the time required for analysis in comparison to the original approach, as well to establish that HaUs accurately reflect haemoglobin content analysis (21). Therefore, our previous tool has been used in a series of later publications (28–36). Nevertheless, our prior approach still remained time consuming and required familiarisation with a new software and access to a colour pantone. Furthermore, it was subject to human error as lesions were manually annotated, which required training and would still differ from one individual to another.
In this study, we aimed to address these shortcomings by implementing a fully automated analysis pipeline, aided by vision AI, i.e. U-Net. We found that our tool, AHA, was able to rapidly and robustly assess the training images that covered a range of haemorrhagic lesion severities. We observed consistent white balancing, error-free scaling, and accurate segmentation. To further stress-test the white balancing, the images were computationally manipulated to represent different lighting scenarios and colour settings on for example different phones. Remarkably, the tool was still able to accurately white balance these images, indicating strong experimental robustness. Segmentation was conducted on a limited dataset to minimise animal usage prior to validation of this approach. Nevertheless, U-Net has demonstrated, across many studies (22, 37–39), its robustness despite small training datasets, as was the case in our study; segmentation consistently aligned with our expert opinion, even when artificially manipulating the images to simulate a range of different laboratory/lighting conditions for a harsher image testing environment. Additionally, the area, luminance, and HaU values calculated across the test images were not significantly different to the ones generated via our previously validated original method and fell within the same range as prior findings (21, 28, 31, 36), thus upholding our prior suggestion of 50 HaU as MHD (21). This also suggests that the AHA tool is validated to be at least as reliable as expert human assessment, whilst being substantially quicker (seconds vs hours). Furthermore, while the tool was trained on B. asper venom induced haemorrhage images, the tool will be able to assess any snake venom induced haemorrhage as long as the background template is used.
To ensure optimal accessibility and ease of implementation into existing workflows, we developed a GUI-based web tool that allows users to conduct fast and accurate analyses of haemorrhagic lesions. Using a smartphone, it takes less than a minute to take a photo, upload the image, and receive accurate information on the severity of a venom-induced haemorrhagic lesion in mice. This substantially decreases the analysis time required from hours to just a few minutes. Furthermore, the ease-of-use significantly boosts the accessibility of our method and provides a standard tool to be used across labs that does not require training or prior knowledge on lesion assessment.
Despite the benefits that AHA holds, some possible limitations may exist. In properly illuminated environments, the white balancing performs accurately. However, in shadowed or strangely lit environments, the white balancing may not perform as well. To mitigate this, it is recommended to photograph in bright, uniformly lit environments. It is especially important to avoid casting shadows or covering the black squares on the template paper for optimal results. Furthermore, due to the translucency of a single sheet of paper, it is best to avoid placing the template on a brightly colored table or desk when photographing. Scaling is computed via information obtained from the black squares at the corners of the template paper. This computation assumes that the black squares are perfect 10 mm x 10 mm squares. Thus, for the most accurate results, it is best to use flat unwrinkled paper and photograph directly from above, so that there is the least amount of distortion applied to the black squares.
5 Conclusion
With AHA, we introduce a new tool for the assessment of venom-induced skin haemorrhage in mice by relying on machine learning guided image analysis approaches. We found it to be at least as accurate as our previously validated approach, while eliminating the risk of human biases in assessing lesion areas and increases the speed of analysis substantially. Furthermore, its open access web-based graphical user interface makes it easy to use and implement in laboratories across the globe. This markedly decreases the resources required for a given analysis, such as analysis and training time, and ensures reproducibility of the results.
Data availability statement
The datasets presented in this study can be found in online repositories. The names of the repository/repositories and accession number(s) can be found below: https://github.com/laprade117/AHA.
Ethics statement
The animal study protocol was reviewed and approved by the Institutional Committee for the Care and Use of Laboratory Animals (CICUA) of the University of Costa Rica.
Author contributions
All authors listed have made a substantial, direct, and intellectual contribution to the work and approved it for publication.
Funding
TJ is the grateful recipient of funding from the European Union’s Horizon 2020 research and innovation program under the Marie Sklodowska-Curie grant agreement no. 713683 (COFUNDfellowsDTU). AL acknowledges funding support from the Villum Foundation (Grant No. 00025302) and the European Research Council (ERC) under the European Union’s Horizon 2020 research and innovation programme (Grant agreement No. 850974).
Conflict of interest
Author TF was employed by company Novozymes.
The remaining authors declare that the research was conducted in the absence of any commercial or financial relationships that could be construed as a potential conflict of interest.
Publisher’s note
All claims expressed in this article are solely those of the authors and do not necessarily represent those of their affiliated organizations, or those of the publisher, the editors and the reviewers. Any product that may be evaluated in this article, or claim that may be made by its manufacturer, is not guaranteed or endorsed by the publisher.
Supplementary material
The Supplementary Material for this article can be found online at: https://www.frontiersin.org/articles/10.3389/fitd.2022.1063640/full#supplementary-material
Supplementary Figure 1 | A4 printout template to be used for haemorrhage assays.
Supplementary Figure 2 | A4 printout cover sheet to be used for haemorrhage assays.
Supplementary Figure 3 | Graphical illustration of how to use the AHA tool. First you open the Google colab tool (1.), then follow the instructions and connect to the server (2.), open the menu bar if not already visible, and select Runtime -> Restart and run all (4.). After a brief wait you use the link provided at the bottom of the page and follow the instructions.
Supplementary Figure 4 | Standard operating procedure sheet for the AHA tool.
References
1. Gutiérrez JM, Calvete JJ, Habib AG, Harrison RA, Williams DJ, Warrell DA. Snakebite envenoming. Nat Rev Dis Primers (2017) 3:17063. doi: 10.1038/nrdp.2017.63
2. Chippaux JP. Estimate of the burden of snakebites in sub-Saharan Africa: A meta-analytic approach. Toxicon (2011) 57:586–99. doi: 10.1016/j.toxicon.2010.12.022
3. Harrison RA, Hargreaves A, Wagstaff SC, Faragher B, Lalloo DG. Snake envenoming: A disease of poverty. PLoS Negl Trop Dis (2009) 3:e569. doi: 10.1371/journal.pntd.0000569
4. Mohapatra B, Warrell DA, Suraweera W, Bhatia P, Dhingra N, Jotkar RM, et al. Snakebite mortality in India: A nationally representative mortality survey. PLoS Negl Trop Dis (2011) 5:e1018. doi: 10.1371/journal.pntd.0001018
5. Chippaux JP. Snake-bites: Appraisal of the global situation. Bull World Health Organ (1998) 76:515–24.
6. Kasturiratne A, Wickremasinghe AR, de Silva N, Gunawardena NK, Pathmeswaran A, Premaratna R, et al. The global burden of snakebite: A literature analysis and modelling based on regional estimates of envenoming and deaths. PLoS Med (2008) 5:e218. doi: 10.1371/journal.pmed.0050218
7. Williams D, Gutiérrez JM, Harrison R, Warrell DA, White J, Winkel KD, et al. The global snake bite initiative: an antidote for snake bite. Lancet (2010) 375:89–91. doi: 10.1016/S0140-6736(09)61159-4
9. Kazandjian TD, Petras D, Robinson SD, van Thiel J, Greene HW, Arbuckle K, et al. Convergent evolution of pain-inducing defensive venom components in spitting cobras. Science (2021) 371:386–90. doi: 10.1126/science.abb9303
10. Calvete JJ. Proteomic tools against the neglected pathology of snake bite envenoming. Expert Rev Proteomics (2011) 8:739–58. doi: 10.1586/epr.11.61
11. Gutiérrez JM, Escalante T, Rucavado A, Herrera C. Hemorrhage caused by snake venom metalloproteinases: A journey of discovery and understanding. Toxins (Basel) (2016) 8:93–3. doi: 10.3390/toxins8040093
12. Escalante T, Rucavado A, Fox JW, Gutiérrez JM. Key events in microvascular damage induced by snake venom hemorrhagic metalloproteinases. J Proteomics (2011) 74:1781–94. doi: 10.1016/j.jprot.2011.03.026
13. Cardoso JLC, França F de S, Wen FH, Malaque CMS. HADDAD jr vidala. animais peçonhentos no brasil: Biologia, clínica e terapêutica dos acidentes. Rev do Instituto Medicina Trop São Paulo (2003) 45:338–8. doi: 10.1590/S0036-46652003000600009
14. White J. Snake venoms and coagulopathy. Toxicon (2005) 45:951–67. doi: 10.1016/j.toxicon.2005.02.030
15. World Health Organization. Rabies and envenomings: A neglected public health issue: report of a consultative meeting, World Health Organization, Geneva, 10 January 2007. World Health Organ (2007).
16. World Health Organization. WHO guidelines for the production, control and regulation of snake antivenom immunoglobulins. In: WHO technical report series. Geneva, Switzerland: WHO (2010). p. 1–134.
17. Gutiérrez JM, Solano G, Pla D, Herrera M, Segura Á, Villalta M, et al. Assessing the preclinical efficacy of antivenoms: From the lethality neutralization assay to antivenomics. Toxicon (2013) 69:168–79. doi: 10.1016/j.toxicon.2012.11.016
18. Kondo H Kondo S, Ikezawa H, Murata R, Ohsaka A. Studies on the quantitative method for determination of hemorrhagic activity of habu snake venom. Japanese J Med Sci Biol (1960) 13:43–51. doi: 10.7883/yoken1952.13.43
19. Theakston R, Reid H. Development of simple standard assay procedures for the characterization of snake venoms. Bull World Health Organ (1983) 61:949.
20. Gutiérrez J, Gené J, Rojas G, Cerdas L. Neutralization of proteolytic and hemorrhagic activities of Costa Rican snake venoms by a polyvalent antivenom. Toxicon (1985) 23:887–93. doi: 10.1016/0041-0101(85)90380-0
21. Jenkins TP, Sánchez A, Segura Á, Vargas M, Herrera M, Stewart TK, et al. An improved technique for the assessment of venom-induced haemorrhage in a murine model. Toxicon (2017) 139:87–93. doi: 10.1016/j.toxicon.2017.10.005
22. Ronneberger O, Fischer P, Brox T. (2015). U-Net: Convolutional networks for biomedical image segmentation, in: International Conference on Medical image computing and computer-assisted intervention, Cham, Switzerland: Springer Cham. pp. 234–41.
23. Ioffe S, Szegedy C. Batch normalization: Accelerating deep network training by reducing internal covariate shift. In: Bach F, Blei D, editors. Proceedings of the 32nd international conference on machine learning, vol. p. Massachusetts: PMLR (2015). p. 448–56. Available at: https://proceedings.mlr.press/v37/ioffe15.html.
25. Chicco D, Jurman G. The advantages of the matthews correlation coefficient (MCC) over F1 score and accuracy in binary classification evaluation. BMC Genomics (2020) 21:6. doi: 10.1186/s12864-019-6413-7
26. Schindelin J, Arganda-Carreras I, Frise E, Kaynig V, Longair M, Pietzsch T, et al. Fiji: An open-source platform for biological-image analysis. Nat Methods (2012) 9:676–82. doi: 10.1038/nmeth.2019
27. Gutiérrez MJ, Solano G, Pla D, Herrera M, Segura Á, Vargas M, et al. Preclinical evaluation of the efficacy of antivenoms for snakebite envenoming: State-of-the-Art and challenges ahead. Toxins (2017) 9, 163. doi: 10.3390/toxins9050163
28. Mora-Obando D, Pla D, Lomonte B, Guerrero-Vargas JA, Ayerbe S, Calvete JJ. Antivenomics and in vivo preclinical efficacy of six Latin American antivenoms towards south-western Colombian Bothrops asper lineage venoms. PloS Negl Trop Dis (2021) 15:e0009073. doi: 10.1371/journal.pntd.0009073
29. Calderon H, Coronel V, Rey O, Alave E, Duran W, Rojas C, et al. Development of nanobodies against hemorrhagic and myotoxic components of Bothrops atrox snake venom. Front Immunol (2020) 11:655. doi: 10.3389/fimmu.2020.00655
30. Chen Y-C, Wang T-Y, Huang Y-K, Chang K-C, Chen M-H, Liu C-C, et al. Effects of sodium silicate complex against hemorrhagic activities induced by Protobothrops mucrosquamatus venom. Toxins (2021) 13:59. doi: 10.3390/toxins13010059
31. Sánchez Brenes A. Evaluación proteómica y toxinológica del veneno de hemachatus haemachatus y su comparación con venenos de cobras del genero naja sp. en términos de inmunoreactividad y neutralización cruzada para la preparación de un antiveneno poliespecífico para África (2020).
32. Alfaro-Chinchilla A, Segura Á, Gómez A, Díaz C, Corrales G, Chacón D, et al. Expanding the neutralization scope of the central American antivenom (PoliVal-ICP) to include the venom of Crotalus durissus pifanorum. J Proteomics (2021) 246:104315. doi: 10.1016/j.jprot.2021.104315
33. Yong MY, Tan KY, Tan CH. Potential para-specific and geographical utility of Thai green pit viper (Trimeresurus albolabris) monovalent antivenom: Neutralization of procoagulant and hemorrhagic activities of diverse trimeresurus pit viper venoms. Toxicon (2021) 203:85–92. doi: 10.1016/j.toxicon.2021.09.021
34. Albulescu L-O, Hale MS, Ainsworth S, Alsolaiss J, Crittenden E, Calvete JJ, et al. Preclinical validation of a repurposed metal chelator as an early-intervention therapeutic for hemotoxic snakebite. Sci Trans Med (2020) 12:eaay8314. doi: 10.1126/scitranslmed.aay8314
35. Sánchez A, Herrera M, Villalta M, Solano D, Segura Á, Lomonte B, et al. Proteomic and toxinological characterization of the venom of the south African ringhals cobra Hemachatus haemachatus. J Proteomics (2018) 181:104–17. doi: 10.1016/j.jprot.2018.04.007
36. Sachetto ATA, Rosa JG, Santoro ML. Rutin (quercetin-3-rutinoside) modulates the hemostatic disturbances and redox imbalance induced by Bothrops jararaca snake venom in mice. PloS Negl Trop Dis (2018) 12:e0006774. doi: 10.1371/journal.pntd.0006774
37. Dong H, Yang G, Liu F, Mo Y, Guo Y. (2017). Automatic brain tumor detection and segmentation using U-net based fully convolutional networks, in: Annual conference on medical image understanding and analysis, Cham, Switzerland: Cham Springer, Vol. p. pp. 506–17.
38. Laprade WM, Perslev M, Sporring J. (2021). How few annotations are needed for segmentation using a multi-planar U-net?, in: Deep Generative Models, and Data Augmentation, Labelling, and Imperfections, Cham, Switzerland: Cham Springer, pp. 209–16.
Keywords: haemorrhage, venom, artificial intelligence, tool, quantification
Citation: Jenkins TP, Laprade WM, Sánchez A, Tulika T, O’Brien C, Sørensen CV, Stewart TK, Fryer T, Laustsen AH and Gutiérrez JM (2022) AHA: AI-guided tool for the quantification of venom-induced haemorrhage in mice. Front. Trop. Dis 3:1063640. doi: 10.3389/fitd.2022.1063640
Received: 07 October 2022; Accepted: 25 November 2022;
Published: 20 December 2022.
Edited by:
Stuart Ainsworth, Liverpool School of Tropical Medicine, United KingdomReviewed by:
Jacqueline De Almeida Gonçalves Sachett, University of the State of Amazonas, BrazilLeticia Gomes De Pontes, University of São Paulo, Brazil
Copyright © 2022 Jenkins, Laprade, Sánchez, Tulika, O’Brien, Sørensen, Stewart, Fryer, Laustsen and Gutiérrez. This is an open-access article distributed under the terms of the Creative Commons Attribution License (CC BY). The use, distribution or reproduction in other forums is permitted, provided the original author(s) and the copyright owner(s) are credited and that the original publication in this journal is cited, in accordance with accepted academic practice. No use, distribution or reproduction is permitted which does not comply with these terms.
*Correspondence: Timothy P. Jenkins, tpaje@dtu.dk
†These authors have contributed equally to this work