- 1Vectech, LLC, Baltimore, MD, United States
- 2Placer County Mosquito and Vector Control District, Roseville, CA, United States
Effective mosquito surveillance and control relies on rapid and accurate identification of mosquito vectors and confounding sympatric species. As adoption of modified mosquito (MM) control techniques has increased, the value of monitoring the success of interventions has gained recognition and has pushed the field away from traditional ‘spray and pray’ approaches. Field evaluation and monitoring of MM control techniques that target specific species require massive volumes of surveillance data involving species-level identifications. However, traditional surveillance methods remain time and labor-intensive, requiring highly trained, experienced personnel. Health districts often lack the resources needed to collect essential data, and conventional entomological species identification involves a significant learning curve to produce consistent high accuracy data. These needs led us to develop MosID: a device that allows for high-accuracy mosquito species identification to enhance capability and capacity of mosquito surveillance programs. The device features high-resolution optics and enables batch image capture and species identification of mosquito specimens using computer vision. While development is ongoing, we share an update on key metrics of the MosID system. The identification algorithm, tested internally across 16 species, achieved 98.4 ± 0.6% % macro F1-score on a dataset of known species, unknown species used in training, and species reserved for testing (species, specimens respectively: 12, 1302; 12, 603; 7, 222). Preliminary user testing showed specimens were processed with MosID at a rate ranging from 181-600 specimens per hour. We also discuss other metrics within technical scope, such as mosquito sex and fluorescence detection, that may further support MM programs.
1 Introduction
In this perspectives paper, we present an overview of critical needs in mosquito surveillance that frame the use case for an automated identification system in the context of modified mosquito populations. These needs have influenced our development of MosID: a device that allows for systematic, high-accuracy mosquito species identification to enhance capability and capacity of mosquito surveillance programs. A brief update on MosID’s state of development is provided and we discuss future directions that we are considering as we aim to develop MosID as a product available to the public health community.
The control of mosquito populations has for many years proven to be an effective strategy in preventing the transmission of mosquito-borne disease (1). Mosquito control programs depend on vector surveillance to optimize these control efforts and monitor efficacy (2). Although traditionally used broad-spectrum adulticides have demonstrated great success as control methods (3), shifting public perception and increased insecticide resistance (4–7) have pushed organizations to adopt alternative control techniques. Many alternative control techniques have been developed and are in various stages of testing or deployment, including sterile insect technique (SIT) (8), genetically modified (GM) populations (9, 10), Wolbachia-induced cytoplasmic incompatibility (11), and disease refractory strains (12, 13). Modified mosquito (MM) methods rely on a detailed understanding of the natural population with accurate species-level data. When implementing a program involving release of insects, it is important to collect baseline data that can inform the parameters of the program, as well as to monitor the success of that program. Often, organizations require a significant scale-up in surveillance activities in order to effectively evaluate and implement these control techniques (14).
Vector control programs are conducted by a variety of organizations across the United States including local health departments, mosquito control districts, and other local governmental agencies (15). As an umbrella term, these will all be referred to as Mosquito Control Organizations (MCOs) moving forward. Despite the recognized need for routine high-accuracy data, surveillance efforts remain a time consuming and labor-intensive process, and MCOs often lack the resources needed to collect essential data. A 2017 survey showed that only 52% of districts performed routine surveillance, and just 61% were targeting mosquito control based on surveillance data (15). Limited public funding for US MCOs is recognized as a main reason for these gaps, causing a lack of preparedness for the Zika outbreak and contributing to the scale of the outbreak internationally (16). In 2020, our team participated in the I-Corps program, an effort sponsored by the National Science Foundation to identify societal needs and potential research technologies that could address these gaps. Through interviews with over one-hundred entomologists, technicians, and managers of vector control organizations, we identified the following specific needs:
1. Capability: Enhance entomological capabilities in public health labs. Smaller public health departments typically do not have a professional entomologist on staff. If equipped with tools capable of accurately identifying vector species, aggregating data, and guiding decision making, smaller public health districts could potentially predict increased risk of vector-borne disease outbreaks and enhance the impact of their vector control programs.
2. Capacity: Build surveillance capacity in the field and the lab for conventional best practices. Even medium and large-sized MCOs, who have the capability of entomological expertise, sometimes have limited capacity to gather sufficient vector surveillance data. Technologies that decrease the time and personnel or training required in the field or lab would be highly beneficial as they would enable districts to generate data more quickly and accurately, increasing the effective allocation of control resources.
3. Modernization and standardization: Modernization and standardization of surveillance data for historical and regional comparisons, reporting to state agencies, and research. Traditional data visualization programs still require data to be manually input or uploaded to each system. Poor centralization of data limits vector control district abilities to review and compare historical data, restricts the ability of state and federal agencies to understand local vector control needs, and hinders research insights. Though some regions have adopted advanced systems, such as California’s VectorServ (https://vectorsurv.org/), many regions do not.
4. Access to new precision variables: Develop tools capable of generating or facilitating generation of new variables or layers of resolution to precision surveillance data, such as the abdominal status of specimens (blood-fed vs starved), specimen age, detection of pathogens, and species-level surveillance of larvae. This information is largely limited to research practices, but would provide valuable data that may enhance effective vector control decision making.
The most prominent alternatives to manual mosquito species identification include DNA based methods, wing beat frequency analysis, and computer vision based image analysis. Although DNA based methods such as DNA barcoding (17) are highly accurate, they require significant training to implement and are expensive, limiting their use operationally to research institutions and large, well-funded MCOs. Analysis of wing beat frequency using deep learning methods has been successful in lab environments (18–21), however has faced significant barriers when deployed in the field due to the high variability exhibited by field caught specimens (22). In the past few years, advances in image processing and deep learning, namely convolutional neural networks (CNNs), have shown great promise in adult mosquito species identification nearing 99% accuracy across large numbers of species (23–26). The most notable works have used primarily wild caught specimens in various states of physical damage (24, 25), assessed differences between cryptic species within a species complex (23), identified the sex of specimens (23), and determined when a species was novel to the CNN system, flagging it as an unknown species (25). Although using relatively small datasets, reducing the variability in the images through standardized imaging likely contributed to the success of these works, as compared to other more massive computer vision datasets such as ImageNet (27) or iNaturalist (28), which include dramatic variability in image resolution, background, lighting, and data source. These advances in computer vision based mosquito species identification warrant practical implementation of new surveillance tools which address the needs described above.
2 MosID Development
2.1 Hardware and System Design
In response to the need to enhance capability and capacity of mosquito surveillance programs, we present MosID: a device that allows for systematic, high-accuracy adult mosquito species identification using high-fidelity computer vision techniques (see Figure 1). The device features high-resolution optics to resolve fine morphological features and batch image capture. The device’s imaging chamber is enclosed to maintain consistent lighting independent of the external setting. Light emitting diodes (LEDs) are arranged above and lateral to the image plane to optimize illumination while minimizing shadows that may obscure features. High-resolution imaging is achieved with a 12MP camera and a high resolution 35mm effective focal length lens, suspended ~250mm or ~12in from the object plane where specimens are inserted into the device. Thus, the system can achieve at least 32 lp/mm throughout a 40mm x 30mm field of view and a 3mm depth of focus, validated in each device using the USAF 1951 resolution target.
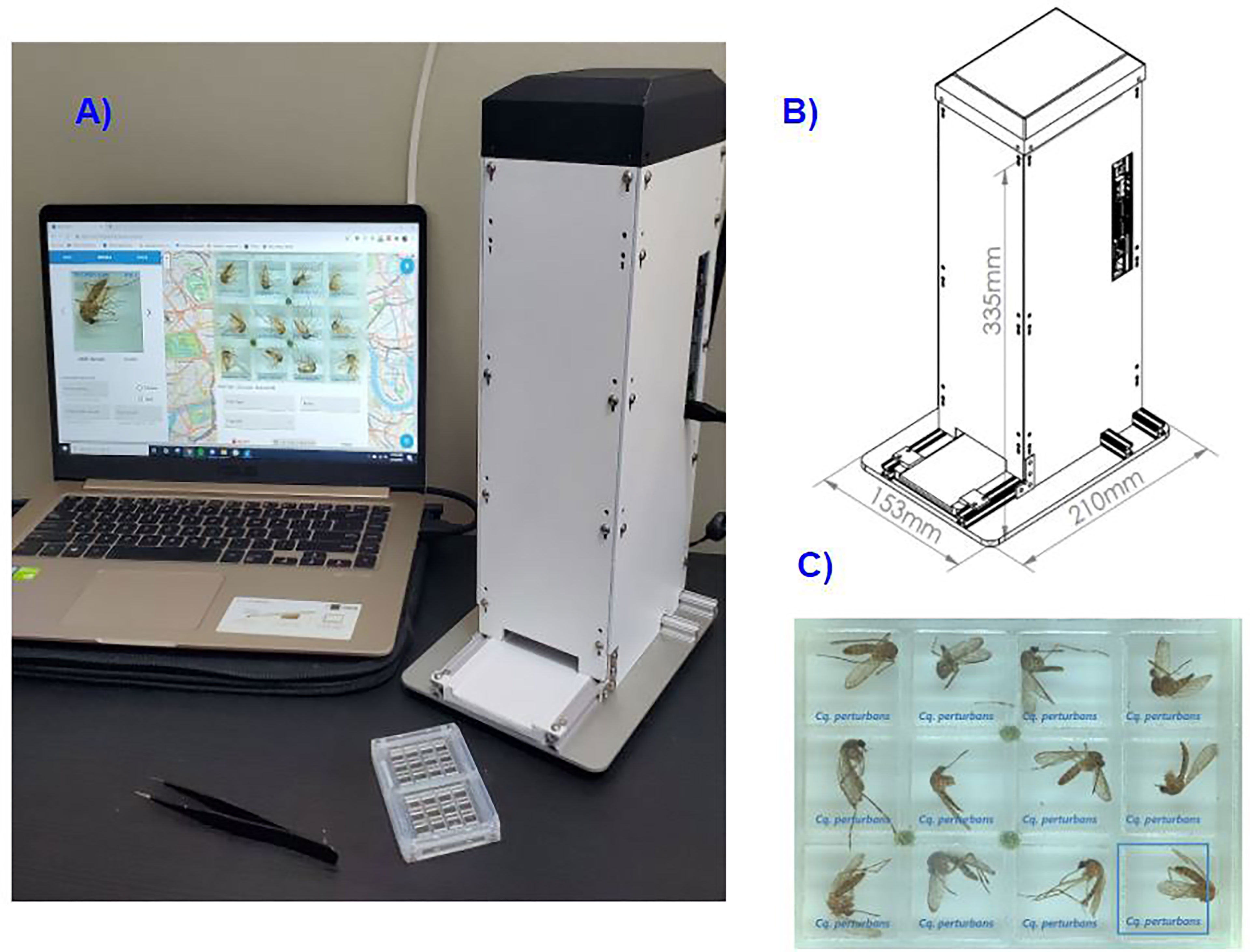
Figure 1 MosID is a tabletop tool for mosquito identification. (A) The MosID system consists of: a specimen tray, which is loaded with specimens and inserted into the device; a device, which takes images and processes them prior to sending them to cloud servers; and a web dashboard which is used to control the device and view the resulting data. (B) The MosID device measures 153mm x 210mm x 335mm. (C) Example of image captured with MosID. Each specimen is placed individually into a well in the tray prior to inserting into the device.
A custom tray was designed to maximize specimen processing speed while maintaining resolution and depth of focus requirements. The tray is transparent, allowing the tray to be flipped and specimens to be imaged from both sides, thereby providing more information for identification. Current computer vision methods in MosID rely on a single view for identification.
The user places a mosquito sample within each of the 12 individual cells, replaces the cover, and inserts the tray into the device. A website is used to direct the device to take an image, receive the image and identification data, and display the result to the user. Users can enter associated data and have the option of contributing their own species identifications for the specimens they imaged. The system is designed to require minimal training.
2.2 Species Identification Algorithm Development
Wild specimens exhibit more variations of phenotype and physical damage than a homogenous lab colony population. Wild specimens were captured and identified by various partner MCOs throughout the United States, then mailed to Vectech for processing with MosID. We tested the function of the novel species detection algorithm described by Goodwin et. al (25)., which uses a three tiered system that analyzes the features output by the identification CNN, an unmodified xception network (29). Novel species detection here refers to detecting a species previously unseen by the CNN species classification algorithm and classifying it as “unknown”. This feature enables deployment of an identification algorithm in a region prior to including every species in the algorithm. The species identification algorithm, tested across 16 species considered relevant by partners, achieved a macro F1 score of 98.4 ± 0.6% across five experiments where the data was folded five times, with each experiment reserving one fold for the test set, resulting in a 80:20 ratio of training data to test data (see Table 1). Image preprocessing and CNN architecture training used were described by Goodwin et al. (25) in the Tier I architecture section of that work. Species and number of specimens included in the identification CNN were: Ae. aegypti, n=95; Ae. dorsalis, n=70; Ae. melanimon, n=96; Ae. nigromaculis, n=62; Ae. sierrensis, n=59; Ae. taeniorhynchus, n=67; Ae. vexans, n=96; An. freeborni, n=88; An punctipennis, n=66; Coquillettidia perturbans, n=96; Culiseta incidens, n=98; Cx. erraticus, n=102; Cx. pipiens sl, n=141; Cx. salinarius, n=96; Cx. tarsalis, n=128; and Psorophora ferox, n=63.
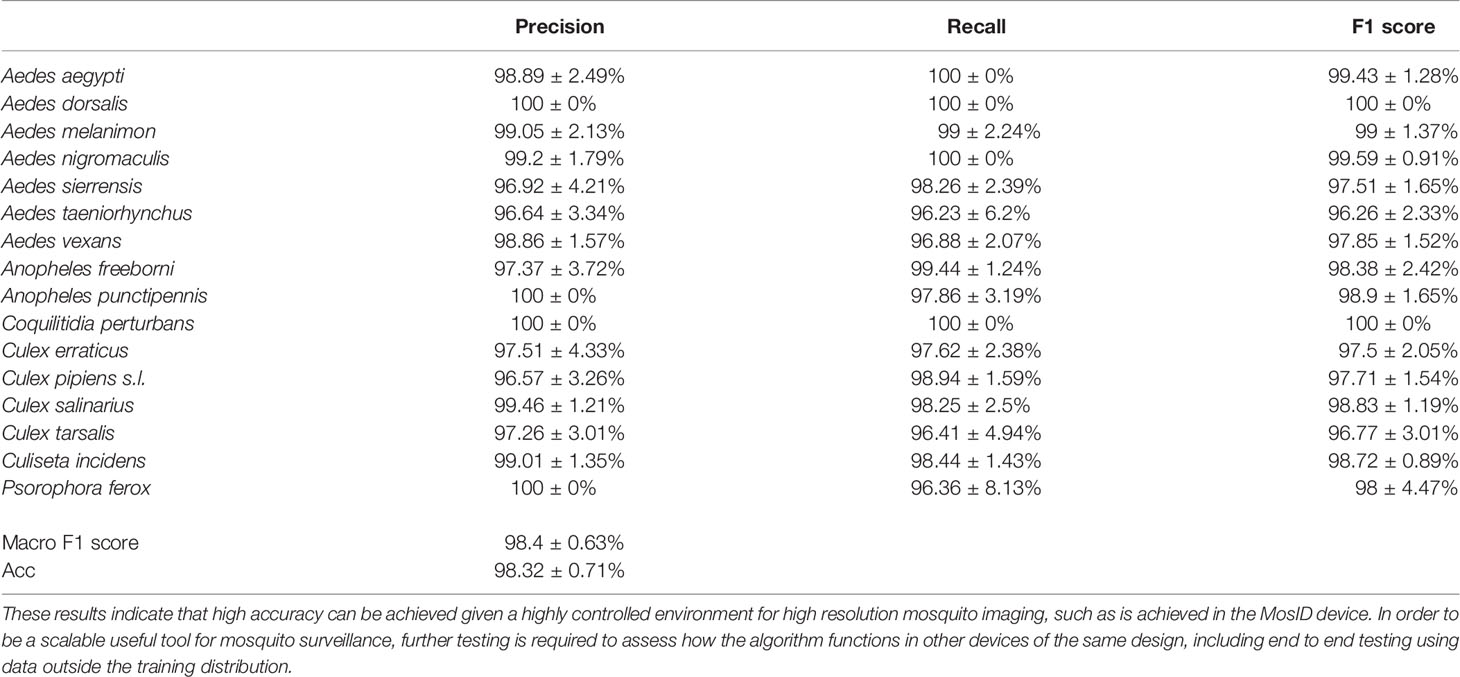
Table 1 Below is the F1-score, precision, and recall for the algorithm test described in Section 2.2, replicating the Tier I closed set architecture of the species classification CNN used in Goodwin et al. (25) on the image database of mosquitoes developed using MosID for this work.
In a separate test, the novel species detection algorithm was trained and tested on a single fold on a data split with 12 known species, 12 unknown species used in training, and 7 unknown species reserved for testing and thus novel to the CNN, following the same methodology as Goodwin et al. (25). The novel species detection architecture was modified to omit the open set CNN portion of tier I and tier II for simplicity. All other portions of the architecture and training methodology were unchanged. Accuracy, F1-score, precision, and recall were as follows: 88.6%, 92.7%, 90.4%, 95.1%. Known species and specimen counts were as follows: Ae. aegypti, n=127; Ae. albopictus, n=143; Ae. melanimon, n=96; Ae. sierrensis, n=72; Ae. vexans, n=98; An. freeborni, n=88; Cq. perturbans, n=110; Cx. erraticus, n=103; Cx. pipiens sl, n=144; Cx. salinarius, n=90; Cx. tarsalis, n=131; Cs. incidens, n=100. Unknown species used in training and the specimen counts were as follows: Ae. dorsalis, n=69; Ae. japonicus, n=58; Ae. nigromaculis, n=56; Ae. taeniorhynchus, n=72; An. punctipennis, n=72; Cx. nigripalpus, n=94; Cx. restuans, n=12; Cx. stigmatosoma, n=13; Cs. inornata, n=41; Cs. particeps, n=1; Ps. ferox, n=70; Uranotaenia sapphirina, n=45. Unknown novel species and specimen counts were as follows: Ae. canadensis, n=31; Ae. increpitus, n=48; Ae. triseriatus, n=16; An. quadrimaculatus, n=30; Cx. territans, n=9; Cs. melanura, n=52; Mansonia titillans, n=36.
2.3 Specimen Processing Speed
Given the large volume of specimens gathered by MCOs during normal operations, which can be further increased with the implementation of MM programs, processing speed of batch collections is a critical metric. This was preliminarily tested by projecting probable operational workflows of MosID based on normal MCO identification workflows. Two workflows were assessed: Process A, where specimens are identified and then discarded; and Process B, where specimens are identified, and then certain specimens are sorted by species for other types of testing such as pathogen detection. Internal testing with MosID showed a processing speed of 600 specimens per hour for identification only (process A), and 430 specimens per hour for identification with sorting (process B). Partner MCOs also tested the speed of these processes using MosID. The processing speeds of MCOs using MosID for identification only and identification with sorting, respectively, were as follows: Placer County, CA (330 specimens per hour, and 229 specimens per hour); Beaufort County, SC (214 specimens per hour, and 182 specimens per hour). MCOs reported the primary rate limiting factors to be the placement of specimens within each well and the sorting of specimens after imaging, especially for trap catches with high species diversity. A significant reduction in processing speed for a portion of the timed trap catches is attributed to the entry of specimen identification data during processing (see Supplementary Information). Other reported factors included some minor manufacturing issues and occasional generation of static electricity.
3 Discussion
3.1 Future Work
There has been a considerable amount of successful research in species identification of mosquitoes using computer vision (23–26) but the technology has yet to be translated for operational use. To function well in the practical context, learning algorithms like CNNs need to be exposed during development to data representative of the diversity they will encounter when deployed, an extremely difficult task in the context of scale. The barriers to achieving consistent deployment accuracy faced by the BG-Counter smart mosquito trap (22) provide evidence of this challenge. However, many MCOs are interested in identification of a small number of key species and therefore limited species coverage may be acceptable. Focusing on key species may limit the required data and accelerate deployment. Additionally, minor variations in images, as may be seen between imaging devices even of the same design and assembly, can produce errors in a CNN (26). Further development is required to scale and deploy an algorithm that is increasingly robust to expected data variations, such as: minute differences between devices; differences between specimen capture, handling, and storage methods prior to imaging; and regional differences in populations within a species.
Algorithm development thus far has focused on species recognition and validating that species identification is feasible. However, similar computer vision techniques can be deployed to identify additional characteristics within technical scope, such as mosquito sex and fluorescence detection, that may further support MM programs.
Sex classification is of particular importance to most MM programs where the method of intervention is dependent on release of males. Measuring the ratio of males to females in the population can serve as a metric for the effectiveness of the intervention in reducing the size of the population (30). Species classification requires an individual to have far more training and expertise than sex determination, as the features dividing certain species from each other can be cryptic (e.g., the coloration of proboscis, the presence of certain hairs on the legs or body) (31), while those which distinguish sex are more distinct and visible at a more macro level. Successful determination of mosquito sex using computer vision has been shown in literature (23) and is currently in development for MosID.
Altering mosquitoes so that they fluoresce under a particular wavelength of light can be another method used to monitor GM program reach. By this method, an organization can monitor the proportion of a population which stems from the released specimens, or in the case of gene drive technologies, monitor the spread of the gene throughout the population (32, 33). Detecting fluorescence with a device such as MosID follows the same principle as a fluorescence microscope or macroscope. To this end, we envision future versions of MosID altered for the detection of certain fluorescent molecules of interest to MM programs.
Additional opportunities exist to further enhance the utility of MosID. For sex classification, fluorescence detection, and species identification, the ability to rapidly assess thousands of specimens is highly desirable to enable surveillance at large scale. Thus, hardware and software workflow modifications to improve processing speed are in development. Beyond the needs of enhanced data collection, streamlined and standardized data entry and presentation stand to expand the impact of surveillance. Manual data entry slows processing time and limits the flexibility and impact of the collected data. Recent advancements in data presentation and data analytics have been used to provide clear historical and regional comparisons, combining inputs beyond traditional mosquito surveillance data, and could be used to produce actionable outputs (34, 35). Furthermore, improvements in centralization of data would provide more research level insights into region-wide trends and provide better visibility on the part of state and federal agencies to understand local vector control needs. Lastly, MosID in its current form is a species identification tool and does not attempt to provide or tie-in additional surveillance data beyond simple metrics such as trap type, location, and collection date; to be accurate and reliable as a stand-alone surveillance tool for users with limited training, predictive variables could be included, such as species variation due to trap type, habitat association, and geographic distribution.
3.2 Conclusion
Advancements in computer vision are well-positioned to push vector surveillance beyond the capability and capacity limitations currently facing MCOs. MosID, a lab device designed to implement computer vision based mosquito species identification, enables species identification without the need for review by a trained professional, thus eliminating a significant capability hurdle in mosquito surveillance. We have shown that high accuracy species identification with this device can be achieved, with the potential to serve MCOs that monitor a wide variety of species, as well as mosquito release programs that are often concerned with the identification of a single species.
Development of MosID thus far represents the transition of computer vision based mosquito species identification from a research topic to an operational tool designed to increase organizational capacity and capability. Of the four critical surveillance needs of MCOs identified by our team in the NSF Innovation Core program, MosID provides species identification expertise, increasing the 1) capacity of organizations that otherwise would not have this skill in-house. For some larger or medium sized MCOs where 2) capacity is a limiting factor, MosID may de-skill the identification process, enabling anyone with basic computer literacy to identify specimens without intensive training. Other innovations may address remaining capacity gaps, such as automating sorting alongside identification as has been done by Verily in the mass production of Wolbachia infected male mosquitoes (36), or smart traps that remotely transmit identification and trap catch data (21, 37). Depending on the organization, MosID may serve as an improvement in 3) modernization and standardization of data due to the inherent digitization of each specimen during processing. With discussed improvements, MosID has the potential to introduce 4) access to new precision variables, such as detection of fluorescence or colorants. This would be powerful in the context of monitoring the dynamics and efficacy of control techniques using mass release of insects, which were previously only accessible to research organizations and very large MCOs.
Data Availability Statement
The datasets presented in this article are not readily available because the image data is the proprietary intellectual property of Vectech, LLC. The data will be made available to academic researchers for noncommercial use upon request. Example images from the dataset and speed testing data are available in the Supplementary Material. A comparable open-source dataset used by Goodwin et al. is available here: https://novel-species-detection-paper.s3.us-east-2.amazonaws.com/ImageBase.zip. Requests to access the datasets should be directed to jewell@vectech.io.
Author Contributions
JB, BS, TF, AH, and AG contributed to writing the manuscript. JB, KG, SP, and AG contributed to the design of MosID. BS, JB, TF, and AG contributed to design of algorithm experiments. BS and AG implemented the algorithm experiments. JB, KG, JW, MG, TF, and AG contributed to the design and implementation of speed testing. JB, BS, AH, and AG contributed to the discussion of previous work, impact of the current work, and possible future work. All authors contributed to the article and approved the submitted version.
Funding
This material is based upon work supported by the National Science Foundation under Grant No. 203953 and the Maryland Innovation Initiative Convertible Note Purchase Agreement #0120-001.
Conflict of Interest
JB, BS, TF, MG, SP, and AG are owners of Vectech, a company developing precision environmental health tools, including MosID, the patent-pending device described in this work. KG was employed by Vectech, and AH is a consultant for Vectech.
The remaining authors declare that the research was conducted in the absence of any commercial or financial relationships that could be construed as a potential conflict of interest.
Publisher’s Note
All claims expressed in this article are solely those of the authors and do not necessarily represent those of their affiliated organizations, or those of the publisher, the editors and the reviewers. Any product that may be evaluated in this article, or claim that may be made by its manufacturer, is not guaranteed or endorsed by the publisher.
Acknowledgments
The authors sincerely thank and acknowledge the many individuals and organizations who supported this work: Nicholas Durr and Michelle Zwernemann in advising the team on optical and mechanical design; SP, George Constantine, and Rongjun Wu for their contributions as a part of the Vectech software team; all the MCOs and research institutions who have donated time, identification expertise, and mosquito specimens, in particular Placer County Mosquito and Vector Control District and Beaufort County Mosquito Control District who contributed to the speed testing, the Johns Hopkins School of Public Health, and the Florida Medical Entomology Lab.
Supplementary Material
The Supplementary Material for this article can be found online at: https://www.frontiersin.org/articles/10.3389/fitd.2021.810062/full#supplementary-material
References
1. World Health Organization. Handbook for Integrated Vector Management. In: Drexler A, editor. Geneva: WHO Press (2012). p. vi–67.
2. Erlank E, Koekemoer LL, Coetzee M. The Importance of Morphological Identification of African Anopheline Mosquitoes (Diptera: Culicidae) for Malaria Control Programmes. Malar J (2018) 17(1):43. doi: 10.1186/s12936-018-2189-5
3. Housset P, Dickmann R. A Promise Fulfilled – Pyrethroid Development and the Benefits for Agriculture and Human Health. Bayer Crop (2009) 62(2):135–144.
4. Tantely ML, Tortosa P, Alout H, Berticat C, Berthomieu A, Rutee A, et al. Insecticide Resistance in Culex Pipiens Quinquefasciatus and Aedes Albopictus Mosquitoes From La Réunion Island. Insect Biochem Mol Biol (2010) 40(4):317–24. doi: 10.1016/j.ibmb.2010.02.005
5. Vontas J, Kioulos E, Pavlidi N, Morou E, della Torre A, Ranson H. Insecticide Resistance in the Major Dengue Vectors Aedes Albopictus and Aedes Aegypti. Pestic Biochem Physiol (2012) 104(2):126–31. doi: 10.1016/j.pestbp.2012.05.008
6. Gan SJ, Leong YQ, Barhanuddin MFH, Wong ST, Wong SF, Mak JW, et al. Dengue Fever and Insecticide Resistance in Aedes Mosquitoes in Southeast Asia: A Review. Parasites Vectors (2021) 14(1):1–19. doi: 10.1186/s13071-021-04785-4
7. Amelia-Yap ZH, Chen CD, Sofian-Azirun M, Low VL. Pyrethroid Resistance in the Dengue Vector Aedes Aegypti in Southeast Asia: Present Situation and Prospects for Management. Parasites Vectors (2018) 11(1):1–17. doi: 10.1186/s13071-018-2899-0
8. Knipling EF. Sterile-Male Method of Population Control. Science (80- ) (1959) 130(3380):902–4. doi: 10.1126/science.130.3380.902
9. Labbé GMC, Scaife S, Morgan SA, Curtis ZH, Alphey L. Female-Specific Flightless (fsRIDL) Phenotype for Control of Aedes Albopictus. PLoS Negl Trop Dis (2012) 6(7):e1724. doi: 10.1371/journal.pntd.0001724
10. Thomas DD, Donnelly CA, Wood RJ, Alphey LS. Insect Population Control Using a Dominant, Repressible, Lethal Genetic System. Science (80) (2000) 287(5462):2474–6. doi: 10.1126/science.287.5462.2474
11. Zabalou S, Apostolaki A, Livadaras I, Franz G, Robinson AS, Savakis C, et al. Incompatible Insect Technique: Incompatible Males From a Ceratitis Capitata Genetic Sexing Strain. Entomol Exp Appl (2009) 132(3):232–40. doi: 10.1111/j.1570-7458.2009.00886.x
12. Gray CE, Coates CJ. High-Level Gene Expression in Aedes Albopictus Cells Using a Baculovirus Hr3 Enhancer and IE1 Transactivator. BMC Mol Biol (2004) 5:1–8. doi: 10.1186/1471-2199-5-1
13. Sánchez-Vargas I, Scott JC, Poole-Smith BK, Franz AWE, Barbosa-Solomieu V, Wilusz J, et al. Dengue Virus Type 2 Infections of Aedes Aegypti Are Modulated by the Mosquito’s RNA Interference Pathway. PLoS Pathog (2009) 5(2):1–11. doi: 10.1371/journal.ppat.1000299
14. Iyaloo DP, Elahee KB, Bheecarry A, Lees RS. Guidelines to Site Selection for Population Surveillance and Mosquito Control Trials: A Case Study From Mauritius. Acta Trop (2014) 132(1):S140–9. doi: 10.1016/j.actatropica.2013.11.011
15. National Association of County and City Health Officials. Mosquito Control Capabilities in the U. (2017). Available at: https://www.naccho.org/uploads/downloadable-resources/Mosquito-control-in-the-U.S.-Report.pdf.
16. Hotez PJ. Zika in the United States of America and a Fateful 1969 Decision. PLoS Negl Trop Dis (2016) 10(5):7–10. doi: 10.1371/journal.pntd.0004765
17. Hebert PDN, Cywinska A, Ball SL, DeWaard JR. Biological Identifications Through DNA Barcodes. Proc R Soc B Biol Sci (2003) 270(1512):313–21. doi: 10.1098/rspb.2002.2218
18. Fanioudakis E, Geismar M, Potamitis I. Mosquito Wingbeat Analysis and Classification Using Deep Learning. In: 26th European Signal Processing Conference (EUSIPCO). (2018). p. 2424–8.
19. Chen Y, Why A, Batista G, Mafra-Neto A, Keogh E. Flying Insect Classification With Inexpensive Sensors. J Insect Behav (2014) 27(5):657–77. doi: 10.1007/s10905-014-9454-4
20. Genoud AP, Basistyy R, Williams GM, Thomas BP. Optical Remote Sensing for Monitoring Flying Mosquitoes, Gender Identification and Discussion on Species Identification. Appl Phys B Lasers Opt (2018) 124(3):46. doi: 10.1007/s00340-018-6917-x
21. Geier M, Weber M, Rose A, Obermayr U, Abadam C, Kiser J, et al. The BG-Counter: A Smart Internet of Things (IoT) Device for Monitoring Mosquito Trap Counts in the Field While Drinking Coffee at Your Desk. In: American Mosquito Control Association Conference. Savannah, Georgia, United States (2016). p. 1–2.
22. Day CA, Richards SL, Reiskind MH, Doyle MS, Byrd BD. Context-Dependent Accuracy of the Bg-Counter Remote Mosquito Surveillance Device in North Carolina. J Am Mosq Control Assoc (2020) 36(2):74–80. doi: 10.2987/19-6903.1
23. Couret J, Moreira D, Bernier D, Loberti A, Dotson EM, Alvarez M. Delimiting Cryptic Morphological Variation Among Human Malaria Vector Species Using Convolutional Neural Networks. PLoS Negl Trop Dis (2020) 14(12):e0008904. doi: 10.1371/journal.pntd.0008904
24. Park J, Kim DI, Choi B, Kang W, Kwon HW. Classification and Morphological Analysis of Vector Mosquitoes Using Deep Convolutional Neural Networks. Sci Rep (2020) 10(1):1–12. doi: 10.1038/s41598-020-57875-1
25. Goodwin A, Padmanabhan S, Hira S, Glancey M, Slinowsky M, Immidisetti R, et al. Mosquito Species Identification Using Convolutional Neural Networks With a Multitiered Ensemble Model for Novel Species Detection. Sci Rep (2021) 11(1):1–15. doi: 10.1038/s41598-021-92891-9
26. Okayasu K, Yoshida K, Fuchida M, Nakamura A. Vision-Based Classification of Mosquito Species: Comparison of Conventional and Deep Learning Methods. Appl Sci (2019) 9(18):1–11. doi: 10.3390/app9183935
27. Deng J, Dong W, Socher R, Li L-J, Kai Li, Li F-F. ImageNet: A Large-Scale Hierarchical Image Database. Institute Electrical Electron Engineers (IEEE) (2010):248–55. doi: 10.1109/CVPR.2009.5206848
28. Van Horn G, Mac Aodha O, Song Y, Cui Y, Sun C, Shepard A, et al. The Inaturalist Species Classification and Detection Dataset. In: 2018 IEEE/CVF Conference on Computer Vision and Pattern Recognition. Piscataway, NJ: IEEE. pp. 8769–78. doi: 10.1109/CVPR.2018.00914
29. Chollet F. Xception: Deep Learning With Depthwise Separable Convolutions. In: 2017 IEEE Conference on Computer Vision and Pattern Recognition (CVPR). Honolulu, HI, USA: IEEE (2017). p. 1800–7.
30. Harris AF, McKemey AR, Nimmo D, Curtis Z, Black I, Morgan SA, et al. Successful Suppression of a Field Mosquito Population by Sustained Release of Engineered Male Mosquitoes. Nat Biotechnol (2012) 30(9):828–30. doi: 10.1038/nbt.2350
31. Ferreira-De-Freitas L, Thrun NB, Tucker BJ, Melidosian L, Bartholomay LC. An Evaluation of Characters for the Separation of Two Culex Species (Diptera: Culicidae) Based on Material From the Upper Midwest. J Insect Sci (2020) 20(6):1–7. doi: 10.1093/jisesa/ieaa119
32. Hammond A, Galizi R, Kyrou K, Simoni A, Siniscalchi C, Katsanos D, et al. A CRISPR-Cas9 Gene Drive System Targeting Female Reproduction in the Malaria Mosquito Vector Anopheles Gambiae. Nat Biotechnol (2016) 34(1):78–83. doi: 10.1038/nbt.3439
33. Simoni A, Hammond AM, Beaghton AK, Galizi R, Taxiarchi C, Kyrou K, et al. A Male-Biased Sex-Distorter Gene Drive for the Human Malaria Vector Anopheles Gambiae. Nat Biotechnol (2020) 38(9):1054–60. doi: 10.1038/s41587-020-0508-1
34. Sallam MF, Michaels SR, Riegel C, Pereira RM, Zipperer W, Graeme Lockaby B, et al. Spatio-Temporal Distribution of Vector-Host Contact (VHC) Ratios and Ecological Niche Modeling of the West Nile Virus Mosquito Vector, Culex Quinquefasciatus, in the City of New Orleans, LA, USA. Int J Environ Res Public Health (2017) 14(8):1–20. doi: 10.3390/ijerph14080892
35. Shi Y, Liu X, Kok SY, Rajarethinam J, Liang S, Yap G, et al. Three-Month Real-Time Dengue Forecast Models: An Early Warning System for Outbreak Alerts and Policy Decision Support in Singapore. Environ Health Perspect (2016) 124(9):1369–75. doi: 10.1289/ehp.1509981
36. Crawford JE, Clarke DW, Criswell V, Desnoyer M, Cornel D, Deegan B, et al. Efficient Production of Male Wolbachia-Infected Aedes Aegypti Mosquitoes Enables Large-Scale Suppression of Wild Populations. Nat Biotechnol (2020) 38(4):482–92. doi: 10.1038/s41587-020-0471-x
Keywords: computer vision, mosquito surveillance, artificial intelligence (AI), imaging, species identification, modified mosquito, GM mosquito, mosquito control organization
Citation: Brey J, Sai Sudhakar BMM, Gersch K, Ford T, Glancey M, West J, Padmanabhan S, Harris AF and Goodwin A (2022) Modified Mosquito Programs’ Surveillance Needs and An Image-Based Identification Tool to Address Them. Front. Trop. Dis 2:810062. doi: 10.3389/fitd.2021.810062
Received: 05 November 2021; Accepted: 29 December 2021;
Published: 10 February 2022.
Edited by:
Yoosook Lee, University of Florida, United StatesReviewed by:
Eamonn John Keogh, University of California, Riverside, United StatesDongmin Kim, University of Florida, United States
Copyright © 2022 Brey, Sai Sudhakar, Gersch, Ford, Glancey, West, Padmanabhan, Harris and Goodwin. This is an open-access article distributed under the terms of the Creative Commons Attribution License (CC BY). The use, distribution or reproduction in other forums is permitted, provided the original author(s) and the copyright owner(s) are credited and that the original publication in this journal is cited, in accordance with accepted academic practice. No use, distribution or reproduction is permitted which does not comply with these terms.
*Correspondence: Jewell Brey, amV3ZWxsQHZlY3RlY2guaW8=
†These authors share senior authorship