- 1Programa da Pós-Graduação em Ecologia e Evolução, Universidade Federal de Goiás, Goiânia, Brazil
- 2Departamento de Ecologia, Instituto de Ciências Biológicas, Universidade Federal de Goiás, Goiânia, Brazil
Dengue is an ongoing problem, especially in tropical countries. Like many other vector-borne diseases, the spread of dengue is driven by a myriad of climate and socioeconomic factors. Within developing countries, heterogeneities on socioeconomic factors are expected to create variable conditions for dengue transmission. However, the relative role of socioeconomic characteristics and their association with climate in determining dengue prevalence are poorly understood. Here we assembled essential socioeconomic factors over 5570 municipalities across Brazil and assessed their effect on dengue prevalence jointly with a previously predicted temperature suitability for transmission. Using a simultaneous autoregressive approach (SAR), we showed that the variability in the prevalence of dengue cases across Brazil is primarily explained by the combined effect of climate and socioeconomic factors. At some dengue seasons, the effect of temperature on transmission potential showed to be a more significant proxy of dengue cases. Still, socioeconomic factors explained the later increase in dengue prevalence over Brazil. In a heterogeneous country such as Brazil, recognizing the transmission drivers by vectors is a fundamental issue in effectively predicting and combating tropical diseases like dengue. Ultimately, it indicates that not considering socioeconomic factors in disease transmission predictions might compromise efficient surveillance strategies. Our study shows that sanitation, urbanization, and GDP are regional indicators that should be considered along with temperature suitability on dengue transmission, setting effective directions to mosquito-borne disease control.
1 Introduction
The presence and prevalence of many infectious diseases have clear geographic structures. These health threats vary from country to country and cause the loss of millions of lives annually (1, 2). Identifying patterns and drivers of infectious diseases has become a fundamental concern (3). For example, understanding why some regions have a higher richness of pathogens than others might help identify hotspots for outbreaks (2). A multitude of factors determines infectious disease geographical distribution and potential outbreaks, spanning from socioeconomic (e.g., urbanization; population density) to environmental (e.g., temperature; precipitation) and biotic (e.g., vectors competition) (1, 4). Acknowledgment of the variation of these drivers over space and time may help identify regions of potential transmission once the disease dynamic is as tightly linked with exogenous factors as with endogenous mechanisms (5, 6).
Dengue, a mosquito-borne disease (MBD), is a global public health concern (7). The incidence of dengue has increased thirty-fold over the last five decades, and it is estimated that approximately one hundred million new infections occur annually (8). In the Americas, the disease is present in almost all countries, with a high number of cases (hereafter referred to as prevalence) (9), where rapid urban expansion led to favorable conditions for dengue vectors (10). The geographic distribution of vectors and the probability of virus transmission to humans are likewise driven by climatic factors, given its influence on mosquito traits (10, 11).
Climate is an important ecological driver of most vector-borne diseases (VBDs) (12–14), of which biological cycles are directly affected (15). For instance, the temperature can either reduce the transmission effectiveness by lowering the vector lifespan or increase it by shortening the extrinsic incubation period (EIP) (5, 12). In VBDs, the geographical transmission range is thus outlined by climate features due to its implication in reproduction, survival, and EIP (2, 16, 17). Accordingly, the physiology of the vectors and their interaction with pathogens are constrained by temperature, generally fitting in a thermal optimum (i.e., between the maximum and minimum tolerated) (18). On the other hand, socioeconomic factors are likewise critical for VBDs (1, 4, 19). For example, studies have shown an association between regional socioeconomic status and vector infestation (20, 21). In this sense, socioeconomic status correlates with factors that include sanitation, education, and health assistance, often associated with disease prevalence (1, 19). In addition, demographic characteristics such as population size and density affect transmission by facilitating the contact between vectors and hosts (see Supplementary Material for a list of detailed assumptions) (15, 22). Consequently, VBDs might prevail where socioeconomic characteristics create favoring conditions for transmission outcomes (19).
Distinct approaches have been used to address the presence and prevalence of VBDs, such as mechanistic (i.e., process-based) and statistical models (23, 24). Mechanistic models rely on empirical information on disease transmission to estimate parameters in a bottom-up procedure (24). For instance, Brady et al. (25) used a mechanistic model to estimate the thermal limits of dengue through the relationship between temperature and Aedes spp. fitness. Most mechanistic approaches in MBDs have integrated the temperature effect on transmission traits to predict global geographical patterns, not considering lower-scale socioeconomic variations (26). Recently, a multi-model climate-driven approach has been proposed to forecast Aedes-borne diseases and support surveillance operations (27). Albeit integrating many transmission-related socioeconomic factors might turn intractable in a process-based procedure, the absence of these critical drivers still brings uncertainty to transmission potential estimation (26).
Although dengue is present in almost all tropical and subtropical countries (8), Brazil has experienced a higher-than-expected number of cases in the last century (9, 28). Since the ‘80s, the reintroduction of dengue in the country has led to its rampant geographic expansion (29). Initially, the presence of the dengue virus was greater in large urban centers, but since the ‘90s, it has spread to small towns and the countryside. In the 2000s, the dengue vectors (i.e., Aedes aegypti and Aedes albopictus) were already present in 72% of Brazilian municipalities, dramatically increasing disease cases and overloading the Brazilian health system (30). Even with dengue being pervasive in Brazil, preparedness for dengue season is difficult due to variations of its incidences across the country and the burden of other infectious and chronic diseases (30, 31).
In this paper, we evaluate the relative impact of socioeconomic conditions and temperature suitability on the spatial pattern of dengue fever prevalence over Brazil. We used a previously estimated temperature suitability for dengue transmission (25) and 7 socioeconomic variables to understand drivers of variation on the prevalence of dengue disease in 5570 municipalities across Brazil. We also sought to understand the fit between estimated temperature suitability for transmission and the effective prevalence of dengue. We predict that in a highly heterogeneous country, such as Brazil, socioeconomic factors are the primary source of high levels of dengue prevalence. In Brazil, regions with the highest dengue prevalence are not those with the highest estimated temperature suitability for transmission, although suitability is still a good indicator of the disease occurrence.
2 Materials And Methods
2.1 Data
2.1.1 Dengue Cases
Notified dengue cases in all 5570 Brazilian municipalities from 2007 to 2016 were obtained from DATASUS through Notifiable Diseases Information System, or SINAN, a database maintained by Brazilian Health System with public access (32). Dengue cases are reported based on clinical (e.g., vomiting, rash, myalgia, headache, retroorbital pain) and epidemiological evidence and are carried out by the local health surveillance team (33).
2.1.2 Temperature Suitability
Here we used simulated dengue transmission suitability maps by Brady et al. (25) as a predictor of dengue presence and prevalence in Brazil. We extracted the raster information regarding each municipality. Brady et al. (25) estimated dengue transmission suitability given the temperature influence on survivorship and extrinsic incubation period (EIP) of Aedes aegypti and Aedes albopictus. The EIP represents the viral incubation period between the mosquito biting an infected host and becoming infectious after processing the pathogen into the gut (34, 35). Brady et al.’s (25) mechanistic model considered the dynamic between EIP and adult vector survival - both temperature-dependent - over the basic reproductive number (i.e., R0) (see 36, 37). The model outcome was then combined with spatially explicit temperature data from WorldClim (38), producing predictive maps of suitability for persistence and competence of dengue transmission for both vectors (25). In our analyses, we used the mean suitability between both vector species.
2.1.3 Socioeconomic Drivers
For each Brazilian municipality, we gathered critical socioeconomic predictors to distribution and prevalence of MBDs, which were: human population density, urbanization, population size, amount of health facilities, gross domestic product (GDP), education, and sanitation (see Supplementary Material). The referred socioeconomic variables and the political-administrative division map of Brazilian municipalities were obtained from the Brazilian Institute of Geography and Statistics (IBGE). This public institution is the primary provider of geographic and census information in Brazil (39, 40). Brazilian political-administrative extension comprises 5570 municipalities, which were all included in analyses.
We estimated population density as the ratio among population size and area of each municipality. We accounted for population size as the census of the total number of people within each city, opposed to estimation. To access the proportion of urbanization within municipalities, we estimated the ratio between urbanized areas [maps based on satellite images (39)] and each municipality’s political-administrative extent. Also, to account for the effect of medical diagnosis, notification, and local investments, we used the number of people assisted by educational and health assistance in each municipality (15, 30). Finally, to represent economic development, we also considered GDP (log scale) and the presence of the basic sanitation system (i.e., sewage treatment and rainfall water management) (see Table A in Supplementary Material).
2.2 Analyses
We employed linear correlations among predictors to assess their collinearity. In a stepwise procedure, we evaluated the non-independence between predictors by measuring the Variance Inflation Factor (VIF) among variables and set apart the most inflated. We started with a full model and iterated the procedure until all predictors had a VIF lower than 10, a threshold commonly used to indicate excessive collinearity (41, 42). Following this procedure, population size and education showed excessive inflation and, therefore, were withdrawn from analyses. Albeit relevant to infectious disease transmission overall, population size as a predictor in our study exhibits a confounding association with GDP in Brazil as a consequence of regional migration patterns to economically developed areas (43).
Since our analysis is spatially explicit, we accounted for spatial autocorrelation that inflates the Type-I error in statistical inferences (44). We used a simultaneous autoregressive model (SAR), which comprises linear regressions with the addition of an autoregressive term specifying the strength of dependence between each pair of locations. Given its reliability and better performance, we used the SARerror model (45). Also, we applied the standardized row coding for the spatial weight matrix, all using the R packages spdep (46) and spatialreg (47). We then examined the Moran’s I correlogram from the model’s residuals to ensure the effective control of spatial autocorrelation. To test for the dissimilarity between putative drives of dengue prevalence, once the period of 2015-2016 had higher disease prevalence than previous years (9), we implemented two separate SARerror models using the log of dengue cases from 2007-2014 and 2015-2016.
Because our SARerror models are estimated by maximum likelihood, the calculation of the coefficient of determination is different from standard Ordinary Least Square (OLS) regression (48). We, therefore, estimated coefficients of determination (R2) to appraise the amount of variation explained by each model through the following formula:
where, n = sample size, Lfull= likelihood of the fitted model, Lnull = likelihood of the null model- the model containing no autoregressive term -. All analyses and maps were performed using R 3.5.0 (49).
3 Results
Dengue cases are unevenly distributed across Brazil, both in occurrence and prevalence (i.e., number of cases) (Figure 1). Over the last years, most dengue cases showed to be concentrated in the Southeast and Midwest regions of Brazil but were also less frequently present in the North and Northeast. From 2007-2014 (Figure 1A), there were fewer reported dengue cases when compared with a later epidemic period (2015-2016; Figure 1B), albeit reaching the Northern region with a higher prevalence. From 2015 to 2016, dengue prevalence was higher in Brazil’s Southeast, Midwest, and Northeast regions. The number of cases almost doubled proportionally to the previous seven years on which dengue was predominant in the Southeast.
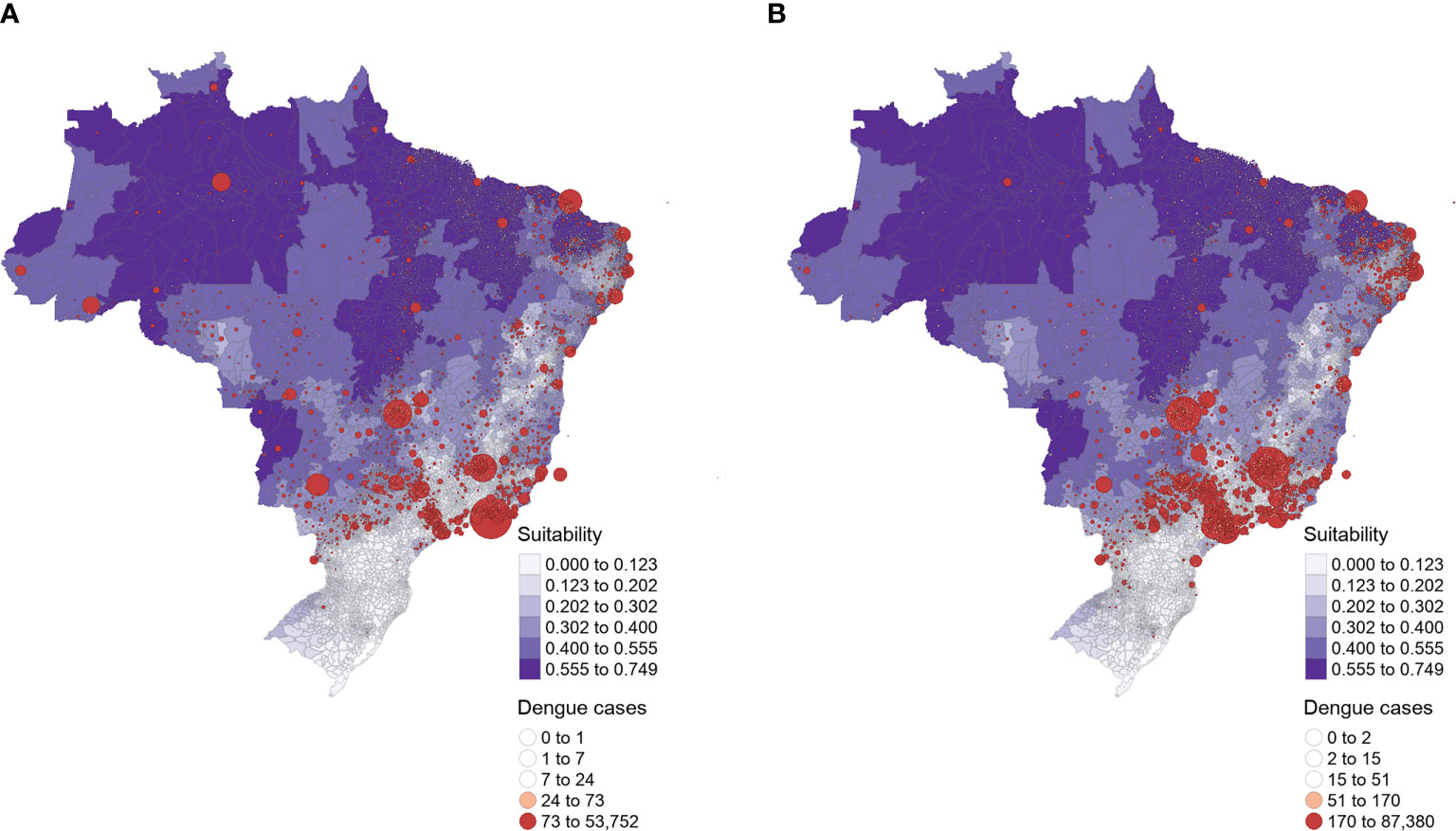
Figure 1 Brazilian dengue cases distribution of (A) 2007 to 2014 and (B) 2015 to 2016. The size of the red circles corresponds to the magnitude of dengue prevalence (i.e., the mean number of cases). The intensity of purple indicates the mean temperature suitability for the dengue transmission by Aedes spp. vectors (i.e., Ae. aegypti and Ae. albopictus), increasing values represent higher suitability for transmission (25).
The graphical comparison between the distribution of actual dengue cases (Figure 1, red circles) and estimated temperature suitability for potential dengue transmission (Figure 1, purple shades) showed a lack of correspondence in both periods. Although the model by Brady et al. predicts high suitability for dengue transmission in the North and Northeast regions of Brazil, fewer dengue cases indeed occurred within this extensive area. Conversely, most dengue cases were reported in the Southeast and Midwest regions, where the model estimated lower temperature suitability. Notably, most dengue cases are concentrated in areas where the model did not predict environmental suitability for dengue transmission. However, the temperature suitability model accurately points to the decreased potential for dengue outbreaks in the south of Brazil, where autochthonous dengue cases were lower from 2007 to 2016.
The autoregressive model revealed the relative importance of socioeconomic factors and estimated temperature suitability for dengue transmission in Brazil (Table 1). From 2007 to 2014, urbanization (and its association with temperature suitability), health facilities, and GDP were the socioeconomic features that best explained the number of dengue cases across Brazil. In contrast, GDP and sanitation were the unique socioeconomic aspects that accounted for the disease distribution and prevalence from 2015 to 2016. However, in 2007-2014 (z = 67.423) and 2015-2016 (z = 58.691), GDP was the predictor that best explained the reported dengue cases across the country. Albeit in less magnitude, temperature suitability for dengue transmission also showed higher explanatory power for distribution of dengue cases in 2007-2014 (z = 14.825) relative to 2015-2016 (z = 7.145).
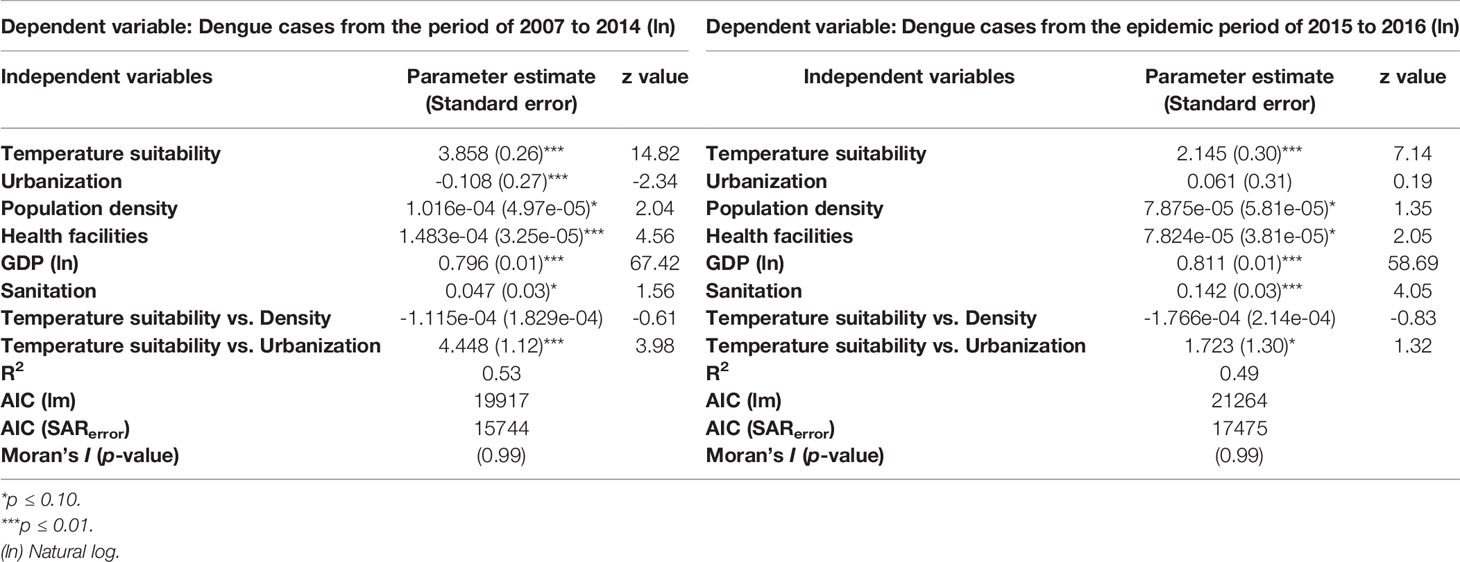
Table 1 SARerror results for distinct periods of dengue prevalence magnitude, 2007 to 2014 and 2015 to 2016.
The human population density was not a significant explanatory factor for the number of reported dengue cases in both periods. Neither was its interaction with estimated temperature suitability for dengue transmission. However, urbanization, a proxy for expanding human-modified areas, was a significant predictor of dengue cases from 2007 to 2014 (Table 1). Urbanization and its interaction with temperature suitability for transmission were crucial from 2007 to 2014 but not significant between 2015 and 2016. The coefficients of determination (R2) of the SARerror models varied between periods, suggesting that the same socioeconomic variables and the temperature suitability for dengue transmission have higher explanatory power during years of lower transmission (R2 = 0.53) than in periods of an increased outbreak (R2 = 0.49). The SAR model had lower AICs than its Ordinary Least Squares (OLS) counterpart (Table 1).
The spatial autocorrelation was successfully controlled by the SAR model (Figure A in Supplementary Material). Patterns of residuals of the models across cities revealed a minor variation in the range of values, and there were no marked spatial patterns of residuals across Brazil (Figure 2). In the southern region, residuals are minimal in many municipalities, indicating that model predictions were accurate in these areas. In contrast, some cities in the Amazon region had negative residuals (overestimated number of dengue cases), whereas some central and southeast Brazil municipalities showed positive residuals (underestimated number of dengue cases) (Figure 2).
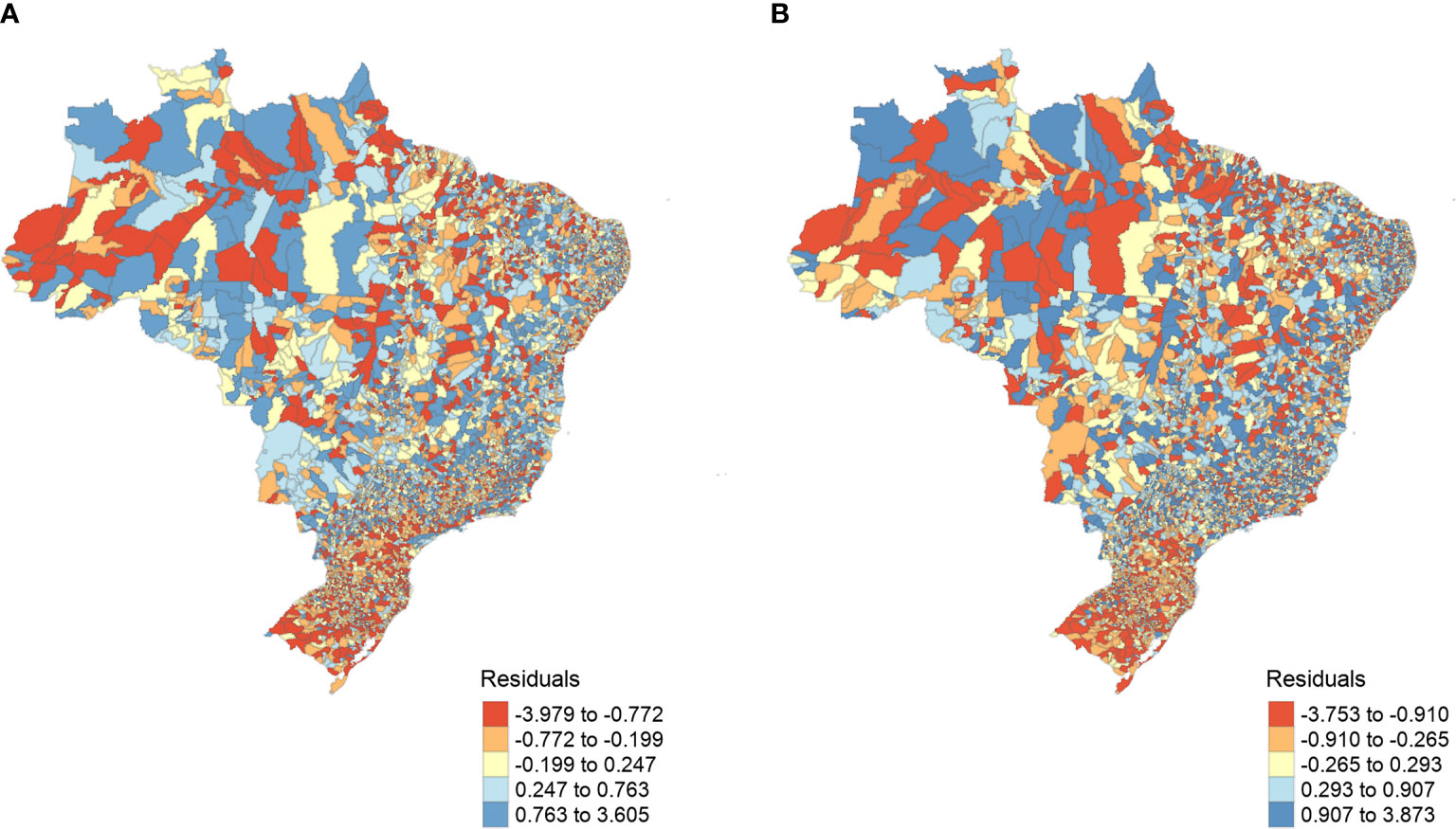
Figure 2 SARerror models’ resulting residuals. (A) 2007 to 2014 and (B) 2015 to 2016, spatially distributed by municipalities. The model’s residuals represent the dengue prevalence that was not fully explained by the model covariates, ranging between ~0.8 and ~ -3.8.
4 Discussion
The higher prevalence of dengue in Brazil compared to other countries has intrigued researchers for decades, revealing that distinctive factors might regulate the transmission of this arboviral disease within particular countries (30). Constant reemergence and maintenance of a high number of dengue cases in Brazil remains unclear, which is justified due to the complex nature of biological features of virus (e.g., circulating serotypes, viral lineages), host (e.g., immune system), and vectors (e.g., vector competence, reproduction rates) (50). In addition, broad-scale patterns of the magnitude of arboviral disease incidence fluctuate according to a myriad of exogenous factors such as climate and socioeconomic status. Accordingly, our results showed that, although temperature suitability for transmission is a good indicator of dengue occurrence, regional socioeconomic characteristics are fundamental determinants of spatial patterns in dengue prevalence in Brazil.
Similar to other tropical nations, Brazil is a heterogeneous country that has undergone substantial urban growth in recent decades. This urban expansion, along with favorable climatic conditions, creates an ideal scenario for the spread of infectious diseases, especially those carried by mosquitoes (24). However, dengue presence and the number of cases differ substantially among regions within Brazil. Dengue vectors are pervasive over Brazil, but mosquito surveillance data are lacking, highly skewed by region, and subject to variations depending on the local surveillance infrastructure. Thus, predicting the geographical potential of MBDs transmission requires incorporating environmental and socioeconomic heterogeneities delimiting its capacity to transmit the disease, especially in countries where an endemic scenario is well established (30). Temperature is a physical factor known to affect the physiology of mosquitoes (e.g., vector competence) and is a suitable proxy for an MBD occurrence (17). However, here we demonstrated that the predicted environmental suitability for dengue transmission, based solely on the temperature influence on the competence of vectors, does not account for most dengue cases in Brazil. In contrast, socioeconomic heterogeneity across cities proved fundamental in determining dengue occurrence and prevalence patterns.
Because temperature is a critical exogenous driver for disease transmission by vectors (27), global climate change may substantially alter the spatial pattern in distribution and prevalence of dengue (11, 51). In countries where the autochthonous transmission of dengue is established, increasing temperatures may intensify transmission by favoring vectors’ survival, reproduction, and biting rates (6). Estimates of dengue transmission suitability under global temperature trends are made in an attempt to anticipate VBD spread and plan mitigation strategies (24, 25, 52). Frequently, such forecasts point to a potential increase of dengue burden under current and future temperature scenarios. Under lower spatial and temporal scales, the relationship between temperature and other exogenous drivers, such as urbanization, GDP, and sanitation, should be more appropriate to describe potential transmission patterns (15, 17). For instance, we showed that Brady’s et al. model points to a high dengue transmission potential in the north of Brazil (e.g., Amazon region), although few cases were reported there. This mismatch reveals that, under the temperature perspective, their model may correctly predict the potential of dengue transmission in this area. Still, observed transmission depends on other factors, such as the interactions between viruses and hosts. Moreover, thermal optima for transmission are limited considering the unimodal effect of temperature on vectors (6, 11). Therefore, increasing temperature over the tolerance of vectors (e.g., through urban heat island effect) might also decrease MBD transmission (31), which would explain the overestimated dengue suitability in some cities.
After controlling for the role of temperature for dengue transmission suitability, our findings highlight that socioeconomic conditions contribute substantially to dengue prevalence. Jointly, GDP and urbanization surpassed the importance of temperature suitability from 2007 to 2014, whereas GDP and sanitation were determinants of an increase in dengue cases between 2015 and 2016. Although temperature constrains the vectorial capacity of Ae. aegypti and Ae. albopictus (53), we show that socioeconomic aspects ultimately determine dengue burden.
Urban centers with higher socioeconomic status are usually equipped with more health facilities, which might bias dengue diagnosis and reports; however, health facilities had a low explanation weight overall. Nevertheless, some studies suggested that socioeconomic developed areas might reduce MBD burden by expanding the sanitation system and the vector combating strategies (19, 54). Conversely, here we found a positive relationship between the sanitation system and GDP with the prevalence of dengue, indicating that the presence of these factors by itself may not attest to the benefits of socioeconomic development in reducing dengue disease cases in Brazil.
The demography in urban environments is thought to be an important driver of dengue prevalence (55). For instance, a temporal analysis of the dengue outbreak in Singapore found that the population demography is the main driver for dengue increase in last years (56). This finding is usually accurate given the expected mixed contact between hosts and vectors. The increase in individual density is expected to increase contact rates between hosts and vectors (36). However, after controlling for other covariates, our model did not find a substantial effect of demography on the prevalence of dengue across Brazil. Although demography may be a good proxy for the number of susceptible individuals, natural immunization likely reduces this proportion in dengue-endemic countries such as Brazil (57). Still, population density may have significant importance at the local scale (e.g., among neighborhoods) once it increases the probability of vector contact with hosts when the virus is established (58). The more significant relation of GDP with dengue prevalence could also indicate the interchange between larger populations, herd immunity, and different serotypes circulating, once Brazilian cities with higher income grew faster by historically being attractive for migration (59).
There is no doubt that the burden of dengue is heavier in some regions than in others. In Brazil, where dengue cases significantly vary across space and time, we highlight that the combined effect of climate and socioeconomic factors are strong drivers of dengue occurrence and prevalence patterns. Albeit not the focus of our study, on a local scale, factors related to population immunization, virus serotype, and vector density are also important drivers of dengue transmission. They should be considered when tracking real-time spread. Still, due to the lack of reliable reports on serological data, most predictive models emphasize the role of temperature on dengue transmission on large scales (24). Indeed, dengue reports assessed in our study might be influenced by the outbreaks of Zika and Chikungunya virus during 2013-2014, once these diseases were then unknown but were already circulating in Brazil, and symptoms are similar to those related to dengue (60).
By accounting for the effect of socioeconomic drivers in a highly heterogeneous country, we showed that the spatial and temporal patterns of dengue prevalence are determined not only by the temperature suitability on the vectorial capacity for transmission but also by social and economic factors. Highly urbanized centers - with high income - were epicenters of dengue transmission in Brazil, aligned with other infectious diseases (61). Consequently, dengue risk projections under current or future climatic conditions should include socioeconomic covariates for reliable predictions on the disease burden, especially when considering that dengue season might come when other infectious (e.g., SARS-CoV-2) or chronic diseases are already overloading the health system. Here we emphasize the need to consider social, economic, and cultural differences between Brazilian regions along with ecological variations for effective decision making for MBDs control.
Data Availability Statement
The original contributions presented in the study are included in the article/Supplementary Material. Further inquiries can be directed to the corresponding author.
Author Contributions
LS and TR conceived the project. LS managed the project. LS conducted the analyses. All authors contributed to the article and approved the submitted version.
Funding
This study was financed in part by the Coordenação de Aperfeiçoamento de Pessoal de Nível Superior - Brasil (CAPES) - Finance Code 001. LS was supported by CAPES fellowship over PROEX PhD and the Sandwich Doctoral Program Abroad-PDSE.
Conflict of Interest
The authors declare that the research was conducted in the absence of any commercial or financial relationships that could be construed as a potential conflict of interest.
Publisher’s Note
All claims expressed in this article are solely those of the authors and do not necessarily represent those of their affiliated organizations, or those of the publisher, the editors and the reviewers. Any product that may be evaluated in this article, or claim that may be made by its manufacturer, is not guaranteed or endorsed by the publisher.
Acknowledgments
We thank the Coordenação de Aperfeiçoamento de Pessoal de Nível Superior - Brasil (CAPES).
Supplementary Material
The Supplementary Material for this article can be found online at: https://www.frontiersin.org/articles/10.3389/fitd.2021.758393/full#supplementary-material
References
1. Bonds MH, Dobson AP, Keenan DC. Disease Ecology, Biodiversity, and the Latitudinal Gradient in Income. Hochberg ME, Editor. PloS Biol (2012) 10(12):e1001456. doi: 10.1371/journal.pbio.1001456
2. Guernier V, Hochberg ME, Guégan J-F. Ecology Drives the Worldwide Distribution of Human Diseases. Paul Harvey, Editor. PloS Biol (2004) 2(6):e141. doi: 10.1371/journal.pbio.0020141
3. Stensgaard A-S, Dunn RR, Vennervald BJ, Rahbek C. The Neglected Geography of Human Pathogens and Diseases. Nat Ecol Evol (2017) 1(7):0190. doi: 10.1038/s41559-017-0190
4. Dunn RR, Davies TJ, Harris NC, Gavin MC. Global Drivers of Human Pathogen Richness and Prevalence. Proc R Soc B Biol Sci (2010) 277(1694):2587–95. doi: 10.1098/rspb.2010.0340
5. Bødker R, Akida J, Shayo D, Kisinza W, Msangeni HA, Pedersen EM, et al. Relationship Between Altitude and Intensity of Malaria Transmission in the Usambara Mountains, Tanzania. J Med Entomol (2003) 40(5):706–17. doi: 10.1603/0022-2585-40.5.706
6. Siraj AS, Oidtman RJ, Huber JH, Kraemer MUG, Brady OJ, Johansson MA, et al. Temperature Modulates Dengue Virus Epidemic Growth Rates Through its Effects on Reproduction Numbers and Generation Intervals. Althouse B, Editor. PloS Negl Trop Dis (2017) 11(7):e0005797. doi: 10.1371/journal.pntd.0005797
7. Harapan H, Michie A, Sasmono RT, Imrie A. Dengue: A Minireview. Viruses (2020) 12(8):829. doi: 10.3390/v12080829
8. WHO. Epidemiology. Geneva: WHO. World Health Organization. (2021) Available at: http://www.who.int/denguecontrol/epidemiology/en/.
9. Dengue - PAHO/WHO. Pan American Health Organization. Available at: https://www.paho.org/en/topics/dengue.
10. Phillips ML. Dengue Reborn: Widespread Resurgence of a Resilient Vector. Environ Health Perspect (2008) 116(9):A382–8. doi: 10.1289/ehp.116-a382
11. Brady OJ, Johansson MA, Guerra CA, Bhatt S, Golding N, Pigott DM, et al. Modelling Adult Aedes Aegypti and Aedes Albopictus Survival at Different Temperatures in Laboratory and Field Settings. Parasit Vectors (2013) 6(1):351. doi: 10.1186/1756-3305-6-351
12. Lafferty KD. The Ecology of Climate Change and Infectious Diseases. Ecology (2009) 90(4):888–900. doi: 10.1890/08-0079.1
13. Harapan H, Yufika A, Anwar S, Te H, Hasyim H, Nusa R, et al. Effects of El Niño Southern Oscillation and Dipole Mode Index on Chikungunya Infection in Indonesia. Trop Med Infect Dis (2020) 5(3):119. doi: 10.3390/tropicalmed5030119
14. Dhewantara PW, Jamil KF, Fajar JK, Saktianggi PP, Nusa R, Garjito TA, et al. Decline of Notified Dengue Infections in Indonesia in 2017: Discussion of the Possible Determinants. Narra J (2021) 1(1):1–16. doi: 10.52225/narraj.v1i1.23
15. Kuno G. Review of the Factors Modulating Dengue Transmission. Epidemiol Rev (1995) 17(2):321–35. doi: 10.1093/oxfordjournals.epirev.a036196
16. Kyle JL, Harris E. Global Spread and Persistence of Dengue. Annu Rev Microbiol (2008) 62:71–92. doi: 10.1146/annurev.micro.62.081307.163005
17. Chandrasegaran K, Lahondère C, Escobar LE, Vinauger C. Linking Mosquito Ecology, Traits, Behavior, and Disease Transmission. Trends Parasitol (2020) 36(4):393–403. doi: 10.1016/j.pt.2020.02.001
18. Dell AI, Pawar S, Savage VM. Systematic Variation in the Temperature Dependence of Physiological and Ecological Traits. Proc Natl Acad Sci (2011) 108(26):10591–6. doi: 10.1073/pnas.1015178108
19. Wood CL, McInturff A, Young HS, Kim D, Lafferty KD. Human Infectious Disease Burdens Decrease With Urbanization But Not With Biodiversity. Philos Trans R Soc B Biol Sci (2017) 372(1722):20160122. doi: 10.1098/rstb.2016.0122
20. LaDeau SL, Leisnham PT, Biehler D, Bodner D. Higher Mosquito Production in Low-Income Neighborhoods of Baltimore and Washington, DC: Understanding Ecological Drivers and Mosquito-Borne Disease Risk in Temperate Cities. Int J Environ Res Public Health (2013) 10(4):1505–26. doi: 10.3390/ijerph10041505
21. Dowling Z, Armbruster P, LaDeau SL, DeCotiis M, Mottley J, Leisnham PT. Linking Mosquito Infestation to Resident Socioeconomic Status, Knowledge, and Source Reduction Practices in Suburban Washington, Dc. EcoHealth (2013) 10(1):36–47. doi: 10.1007/s10393-013-0818-6
22. Anderson RM, May RM. Regulation and Stability of Host-Parasite Population Interactions: I. Regulatory Processes. J Anim Ecol (1978) 47(1):219. doi: 10.2307/3933
23. Parham PE, Waldock J, Christophides GK, Hemming D, Agusto F, Evans KJ, et al. Climate, Environmental and Socio-Economic Change: Weighing Up the Balance in Vector-Borne Disease Transmission. Philos Trans R Soc B Biol Sci (2015) 370(1665):20130551–20130551. doi: 10.1098/rstb.2013.0551
24. Messina JP, Brady OJ, Pigott DM, Golding N, Kraemer MUG, Scott TW, et al. The Many Projected Futures of Dengue. Nat Rev Microbiol (2015) 13(4):230–9. doi: 10.1038/nrmicro3430
25. Brady OJ, Golding N, Pigott DM, Kraemer MUG, Messina JP, Reiner RC Jr., et al. Global Temperature Constraints on Aedes Aegypti and Ae. Albopictus Persistence and Competence for Dengue Virus Transmission. Parasit Vectors (2014) 7(1):338. doi: 10.1186/1756-3305-7-338
26. Carlson CJ, Dougherty E, Boots M, Getz W, Ryan SJ. Consensus and Conflict Among Ecological Forecasts of Zika Virus Outbreaks in the United States. Sci Rep (2018) 8(1):4921. doi: 10.1038/s41598-018-22989-0
27. Muñoz ÁG, Chourio X, Rivière-Cinnamond A, Diuk-Wasser MA, Kache PA, Mordecai EA, et al. AeDES: A Next-Generation Monitoring and Forecasting System for Environmental Suitability of Aedes-Borne Disease Transmission. Sci Rep (2020) 10(1):12640. doi: 10.1038/s41598-020-69625-4
28. Bhatt S, Gething PW, Brady OJ, Messina JP, Farlow AW, Moyes CL, et al. The Global Distribution and Burden of Dengue. Nature (2013) 496(7446):504–7. doi: 10.1038/nature12060
29. Barcellos C, Lowe R. Expansion of the Dengue Transmission Area in Brazil: The Role of Climate and Cities. Trop Med Int Health (2014) 19(2):159–68. doi: 10.1111/tmi.12227
30. Teixeira MG, Costa M da CN, Barreto F, Barreto ML. Dengue: Twenty-Five Years Since Reemergence in Brazil. Cad Saúde Pública (2009) 25(suppl 1):S7–18. doi: 10.1590/S0102-311X2009001300002
31. Araujo RV, Albertini MR, Costa-da-Silva AL, Suesdek L, Franceschi NCS, Bastos NM, et al. São Paulo Urban Heat Islands Have a Higher Incidence of Dengue Than Other Urban Areas. Braz J Infect Dis Off Publ Braz Soc Infect Dis (2015) 19(2):146–55. doi: 10.1016/j.bjid.2014.10.004
32. DATASUS. Available at: http://www2.datasus.gov.br/DATASUS/index.php?area=02.
33. Siqueira JB, Martelli CMT, Coelho GE, Simplicio AC da R, Hatch DL. Dengue and Dengue Hemorrhagic Fever, Brazil, 1981-2002. Emerg Infect Dis (2005) 11(1):48–53. doi: 10.3201/eid1101.031091
34. Chan M, Johansson MA. The Incubation Periods of Dengue Viruses. Vasilakis N, Editor. PloS One (2012) 7(11):e50972. doi: 10.1371/journal.pone.0050972
35. Gabrieli P, Caccia S, Varotto-Boccazzi I, Arnoldi I, Barbieri G, Comandatore F, et al. Mosquito Trilogy: Microbiota, Immunity and Pathogens, and Their Implications for the Control of Disease Transmission. Front Microbiol (2021) 12:633. doi: 10.3389/fmicb.2021.630438
36. Anderson RM, May RM. Infectious Diseases of Humans: Dynamics and Control. OUP Oxford (1992) 772.
37. Gething PW, Van Boeckel TP, Smith DL, Guerra CA, Patil AP, Snow RW, et al. Modelling the Global Constraints of Temperature on Transmission of Plasmodium Falciparum and P. vivax Parasit Vectors (2011) 4:92. doi: 10.1186/1756-3305-4-92
38. WorldClim global climate data. Data for Current Conditions. Available at: http://www.worldclim.org/current.
39. IBGE. Portal do IBGE | IBGE. Available at: https://www.ibge.gov.br/pt/inicio.html.
40. Sistema IBGE De Recuperação Automática - SIDRA . Available at: https://sidra.ibge.gov.br/home/ipca/brasil.
41. O’brien RM. A Caution Regarding Rules of Thumb for Variance Inflation Factors. Qual Quant (2007) 41(5):673–90. doi: 10.1007/s11135-006-9018-6
42. Belsley DA. Assessing the Presence of Harmful Collinearity and Other Forms of Weak Data Through a Test for Signal-to-Noise. J Econom (1982) 20(2):211–53. doi: 10.1016/0304-4076(82)90020-3
43. Yap L. Internal Migration and Economic Development in Brazil. Q J Econ (1976) 90(1):119–37. doi: 10.2307/1886089
45. Kissling WD, Carl G. Spatial Autocorrelation and the Selection of Simultaneous Autoregressive Models. Glob Ecol Biogeogr (2007) 0(0):070618060123007–??? doi: 10.1111/j.1466-8238.2007.00334.x
46. Bivand R, Altman M, Anselin L, Assunção R, Berke O, Bernat A, et al. Spdep: Spatial Dependence: Weighting Schemes, Statistics (2019). Available at: https://CRAN.R-project.org/package=spdep.
47. Bivand R, Piras G, Anselin L, Bernat A, Blankmeyer E, Chun Y, et al. Spatialreg: Spatial Regression Analysis (2019). Available at: https://CRAN.R-project.org/package=spatialreg.
48. Nagelkerke NJD. A Note on a General Definition of the Coefficient of Determination. Biometrika (1991) 78(3):691–2. doi: 10.1093/biomet/78.3.691
49. R: The R Project for Statistical Computing. Available at: https://www.r-project.org/.
50. Gubler DJ. The Economic Burden of Dengue. Am J Trop Med Hyg (2012) 86(5):743–4. doi: 10.4269/ajtmh.2012.12-0157
51. Kraemer MUG, Sinka ME, Duda KA, Mylne AQN, Shearer FM, Barker CM, et al. The Global Distribution of the Arbovirus Vectors Aedes Aegypti and Ae. albopictus. eLife (2015) 4:e08347. doi: 10.7554/eLife.08347
52. Mordecai EA, Ryan SJ, Caldwell JM, Shah MM, LaBeaud AD. Climate Change Could Shift Disease Burden From Malaria to Arboviruses in Africa. Lancet Planet Health (2020) 4(9):e416–23. doi: 10.1016/S2542-5196(20)30178-9
53. Johansson MA, Dominici F, Glass GE. Local and Global Effects of Climate on Dengue Transmission in Puerto Rico. PloS Negl Trop Dis (2009) 3(2):e382. doi: 10.1371/journal.pntd.0000382
54. Åström C, Rocklöv J, Hales S, Béguin A, Louis V, Sauerborn R. Potential Distribution of Dengue Fever Under Scenarios of Climate Change and Economic Development. EcoHealth (2012) 9(4):448–54. doi: 10.1007/s10393-012-0808-0
55. Schmidt W-P, Suzuki M, Thiem VD, White RG, Tsuzuki A, Yoshida L-M, et al. Population Density, Water Supply, and the Risk of Dengue Fever in Vietnam: Cohort Study and Spatial Analysis. PloS Med (2011) 8(8):e1001082. doi: 10.1371/journal.pmed.1001082
56. Struchiner CJ, Rocklöv J, Wilder-Smith A, Massad E. Increasing Dengue Incidence in Singapore Over the Past 40 Years: Population Growth, Climate and Mobility. PloS One (2015) 10(8):e0136286. doi: 10.1371/journal.pone.0136286
57. Wearing HJ, Rohani P. Ecological and Immunological Determinants of Dengue Epidemics. Proc Natl Acad Sci (2006) 103(31):11802–7. doi: 10.1073/pnas.0602960103
58. Padmanabha H, Correa F, Rubio C, Baeza A, Osorio S, Mendez J, et al. Human Social Behavior and Demography Drive Patterns of Fine-Scale Dengue Transmission in Endemic Areas of Colombia. Paul R, Editor. PloS One (2015) 10(12):e0144451. doi: 10.1371/journal.pone.0144451
59. Salles TS, da Encarnação Sá-Guimarães T, de Alvarenga ESL, Guimarães-Ribeiro V, de Meneses MDF, de Castro-Salles PF, et al. History, Epidemiology and Diagnostics of Dengue in the American and Brazilian Contexts: A Review. Parasit Vectors (2018) 11(1):264. doi: 10.1186/s13071-018-2830-8
60. Souza TML, Vieira YR, Delatorre E, Barbosa-Lima G, Luiz RLF, Vizzoni A, et al. Emergence of the East-Central-South-African Genotype of Chikungunya Virus in Brazil and the City of Rio De Janeiro may Have Occurred Years Before Surveillance Detection. Sci Rep (2019) 9(1):2760. doi: 10.1038/s41598-019-39406-9
Keywords: dengue, temperature suitability, socioeconomic drivers, vector-borne disease, mosquito transmission
Citation: Simon LM and Rangel TF (2021) Are Temperature Suitability and Socioeconomic Factors Reliable Predictors of Dengue Transmission in Brazil? Front. Trop. Dis 2:758393. doi: 10.3389/fitd.2021.758393
Received: 13 August 2021; Accepted: 27 October 2021;
Published: 16 November 2021.
Edited by:
Son H. Nghiem, Griffith University, AustraliaReviewed by:
Emad I. M. Khater, Ain Sham University, EgyptHarapan Harapan, Syiah Kuala University, Indonesia
Copyright © 2021 Simon and Rangel. This is an open-access article distributed under the terms of the Creative Commons Attribution License (CC BY). The use, distribution or reproduction in other forums is permitted, provided the original author(s) and the copyright owner(s) are credited and that the original publication in this journal is cited, in accordance with accepted academic practice. No use, distribution or reproduction is permitted which does not comply with these terms.
*Correspondence: Lorena M. Simon, bG9yZXNzaW1vbkBnbWFpbC5jb20=