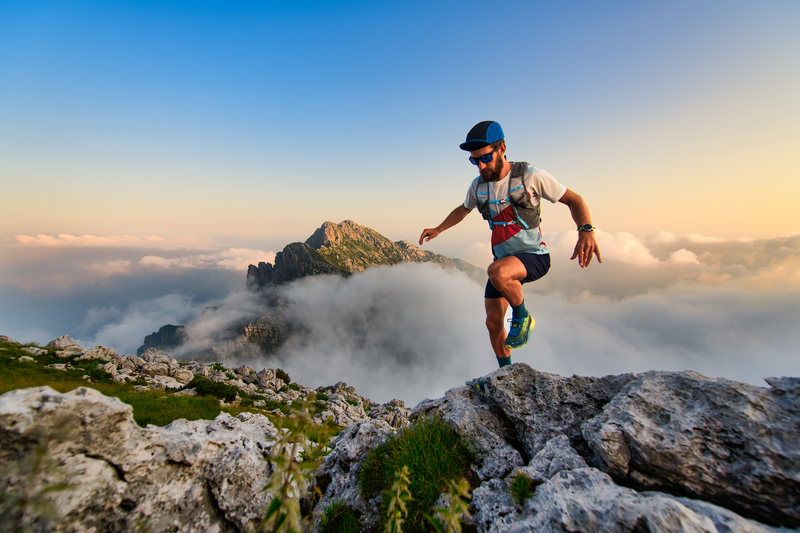
95% of researchers rate our articles as excellent or good
Learn more about the work of our research integrity team to safeguard the quality of each article we publish.
Find out more
ORIGINAL RESEARCH article
Front. Insect Sci. , 20 October 2022
Sec. Invasive Insect Species
Volume 2 - 2022 | https://doi.org/10.3389/finsc.2022.1006092
This article is part of the Research Topic Focus on Popillia japonica View all 11 articles
Remote sensing has been shown to be a promising technology for the detection and monitoring of plant stresses including insect feeding. Popillia japonica Newman, is an invasive insect species in the United States, and a pest of concern to soybean, Glycine max (L.) Merr., in the upper Midwest. To investigate the effects of P. japonica feeding injury (i.e., defoliation) on soybean canopy spectral reflectance and yield, field trials with plots of caged soybean plants were established during the summers of 2020 and 2021. In each year, field-collected P. japonica adults were released into some of the caged plots, creating a gradient of infestation levels and resulting injury. Estimates of injury caused by P. japonica, ground-based hyperspectral readings, total yield, and yield components were obtained from the caged plots. Injury was greatest in the upper canopy of soybean in plots infested with P. japonica. Overall mean canopy injury (i.e., across lower, middle, and upper canopy) ranged from 0.23 to 6.26%, which is representative of injury levels observed in soybean fields in the Midwest United States. Feeding injury from P. japonica tended to reduce measures of soybean canopy reflectance in near infra-red wavelengths (~700 to 1000 nm). These results indicate that remote sensing has potential for detection of injury from P. japonica and could facilitate scouting for this pest. Effects of P. japonica injury on total yield were not observed, but a reduction in seed size was detected in one of the two years. The threat to soybean yield posed by P. japonica alone appears minimal, but this pest adds to the guild of other defoliating insects in soybean whose combined effects could threaten yield. The results of this research will guide refinement of management recommendations for this pest in soybean and hold relevance for other cropping systems.
Japanese beetle, Popillia japonica Newman (Coleoptera: Scarabaeidae), is native to Japan and known to feed on more than 300 plant species (1). Popillia japonica has expanded its geographic range and became an invasive species in the United States in the early 1900s and Canada in 1998 (1, 2). More recently, P. japonica was also reported in mainland Europe (3) and it is now present in at least three European countries (4). Additionally, P. japonica has the potential to expand its range even further and invade Central and South America, Africa, and Oceania (5). In its region of origin, P. japonica is a minor agricultural pest, probably due to unfavorable environmental conditions and the presence of natural enemies (1). However, in invaded regions, P. japonica is an economically important pest of ornamental plants and turf in landscapes, and horticultural and field crops (1, 2, 6).
Soybean, Glycine max (L.) Merrill (Fabales: Fabaceae), is one of the most valuable crops worldwide due to its seed composition (i.e., oil and protein content) and versatility of end-use (7). The United States is the second largest soybean producer worldwide (8), but its production is compromised by the attack of insect pests (7). Defoliation caused by insect feeding is a common injury seen in soybean fields that can potentially lead to yield losses due to the reduction of plant photosynthetic area and disturbance of physiological processes (9). In soybean, P. japonica adults feed on leaves, creating a characteristic lace-like pattern of defoliation (6). Infestations of P. japonica alone can cause up to 20% loss of soybean yield (10), but P. japonica damage to soybeans can be even more problematic when combined with other defoliating insects (11). However, impacts of P. japonica feeding have not been well quantified in contemporary soybean varieties.
Current management of defoliating insects in soybean generally relies on the presence of the pest and estimation of percent defoliation across the whole field based on visual assessment of leaves from the top, middle, and bottom of plants selected from throughout the field (6). For P. japonica, assessment of the entire canopy of the crop is of particular importance because adults aggregate on the upper leaves of the plants and abundance tends to be higher at the edge of the fields, which can lead to overestimation of defoliation, especially at the field edges (6). Overall, traditional scouting and decision making for defoliating pests, like P. japonica, in soybean can be time consuming and therefore increase the overall cost of management.
Remote sensing became prominent in the past decades as a promising method for the detection and monitoring of plant stresses (e.g., insect feeding) (12–15). Remote sensing may be preferable to conventional scouting methods because it is faster and offers better coverage of the field (13, 14). Furthermore, remote sensing allows for early detection of diseases and pests (13). Typical applications of remote sensing consist of using sensors for contactless measurement of the electromagnetic radiation reflected from plants (12, 13). Numerous studies have documented the effects of pest injury and diseases on spectral reflectance of crops, mainly in the visible and near infra-red ranges of the electromagnetic spectrum (16–21). Studies assessing the effects of defoliation on plant spectral reflectance have focused mainly on forest areas (13–15), but field crops have also been investigated (22–26). In soybean, the normalized difference vegetation index (NDVI) was proposed for the detection of defoliation with simulated (22) and actual insect feeding by lepidopteran pests (25). More recently, Iost Filho et al. (2022) (26) evaluated the effects of defoliation by two lepidopteran pests on soybean leaf reflectance using individual wavelengths from the visible and near infra-red spectrum.
There is a lack of information of the effects of defoliation on the spectral reflectance of soybean that include individual wavelengths from the visible and near-infrared spectrum for other defoliators, such as P. japonica, especially under field conditions. Additionally, as abovementioned, a better understanding of the impacts of P. japonica feeding on yield of contemporary soybean varieties is also required. Thus, this study was done to assess the effects of feeding injury from P. japonica on the spectral reflectance, total yield, and yield components of soybean. Results of this study will help advance integrated pest management programs for P. japonica in soybean fields.
This study was done in soybean fields of approximately 1 ha located at the University of Minnesota (UMN) Saint Paul campus (44.9898369° N, 93.1802096° W), and at the UMN Research and Outreach Center (44.7113597° N, 93.1041755° W) in Rosemount, Minnesota, United States, during 2020 and 2021, respectively. The soybean variety Stine ‘19EA32’ was planted on 15 May 2020 and the variety Golden Harvest ‘1012E3’ on 15 June 2021 with a seeding rate of 370,000 seeds/ha and row spacing of 0.76 m. When plants were at the V3 growth stage (plants with three fully expanded trifoliate leaves (27), plots of soybean were caged for manipulation of insect populations. Individual plots comprised two rows of soybean that were 1.5 m long (approximately 80 plants per plot), and caged with a 1.5×1.5×1.5-m polyvinyl chloride (PVC) frame covered with white no-see-um mesh (Quest Outfitters, Sarasota, FL, USA). A total of 32 and 24 plots (i.e., cages), arranged in 8 and 6 blocks, were caged in 2020 and 2021, respectively. Before caging, plants were visually inspected for the presence of insects and any individuals found on the plants were manually removed.
In each year, half of the plots in each block were randomly selected for infestation with field-collected P. japonica adults on four dates to create a gradient of insect injury. Adult P. japonica were collected from soybean fields using dual-lures (female sex pheromone and floral attractant) attached to Trécé Pherocon® standard traps (yellow top and green vented catch can) (Trécé Inc., Adair, OK, USA). Two days prior to each infestation, P. japonica were collected from traps 4-5 times per day to reduce insect mortality due to excess heat inside the traps. Trapped P. japonica adults were transferred to 34.29×34.29×60.96-cm pop-up insect cages (Bioquip, Rancho Dominguez, CA, USA). As a food source, each cage contained 3-4 field-collected soybean stems cut at the soil level with the cut end of the stems placed inside 20-mL tubes with pierceable caps containing water. For infestations, live (i.e., actively moving) P. japonica adults were manually collected from the pop-up cages and placed in containers to be transported to the field. The number of P. japonica adults in each container was estimated based on fresh biomass using the methods of Ebbenga et al. (2022) (28) and an analytical scale (Sartorius ENTRIS224-1S, Sartorius Lab Instruments GmbH & Co. KG, Goettingen, Germany). In 2020, P. japonica were released on 28 July (1037 individuals per plot), 3 August (224 individuals per plot), 13 August (212 individuals per plot), and 24 August (39 individuals per plot) for a total of 1512 individuals per plot. Similarly, in 2021, P. japonica were released on 4 August (831 individuals per plot), 9 August (877 individuals per plot), 16 August (945 individuals per plot), and 24 August (80 individuals per plot) for a total of 2734 individuals per plot.
Spectral measurements were recorded within 2 h of solar noon (to reduce atmospheric and solar angle effects), with clear sky conditions or with low cloud cover (< 20%) and a clear view between the sun and the field. Measurements of canopy spectral reflectance were taken on 30 July and 4 September of 2020, and 17 August and 30 August of 2021, using a hyperspectral spectroradiometer (FieldSpec® HandHeld 2™ VNIR spectroradiometer, ASD Inc., Boulder, CO, USA) able to detect wavelengths ranging from 325 to 1075 nm with accuracy of ±1 nm. On each sample date, the spectroradiometer was calibrated immediately before the beginning of measurements and every 7-10 minutes throughout data collection with a Spectralon® reference standard (Labsphere, Inc. Sutton, NH, USA). In each plot, four spectral measurements were manually taken, two from each row, at approximately 0.5 m above the canopy. Cages were opened immediately before and closed immediately after measurements. Canopy-level spectral reflectance data were processed using the software ViewSpec Pro version 6.2.0 (ASD Inc., Boulder, CO, USA) and individual measures were averaged for each plot. Four vegetation indices were calculated from canopy-level spectral reflectance (Table 1) for each plot. These indices were selected because they were used in previous studies investigating the effects of insect feeding and diseases on soybean spectral reflectance (20, 29, 30).
Table 1 Vegetation indices tested in this study for the detection of effects of Popillia japonica feeding injury in plots of caged soybean plants in the field during 2020 and 2021 in Saint Paul, MN and Rosemount, MN, respectively.
To avoid effects on canopy reflectance measures caused by disturbing the soybean plant canopies, inspection of plants for other insects was performed one day before or one day after collection of spectral data. To do so, whole-plant counts of other insects were recorded for five plants per plot, which were later averaged for each plot. Insects observed on the plants were not removed. Estimates of injury from P. japonica were done one day before or after measurements of canopy spectral reflectance. Five leaflets were randomly selected and collected from the lower, middle, and upper portions of the canopy of each plot (i.e., 15 leaflets/plot). These leaflets were placed in individually-labeled 17×17-cm resealable plastic bags, which were placed in a cooler with ice packs for transportation to the laboratory where they were stored in a refrigerator at 5°C to avoid desiccation. To quantify P. japonica feeding injury (i.e., percentage of leaflet area removed), leaflets were placed individually on a white surface and were fully extended and flattened under a transparent glass circle (180 mm diameter). Measurements of injury were performed on pictures of each individual leaflet using the software LeafByte version 1.3.0 (31) with an iPad (A1893, Apple Inc., Cupertino, CA, USA). Mean canopy injury (%) for lower, middle, and upper canopy and total canopy (i.e., across lower, middle, and upper canopy) were obtained for each plot.
On 9 October 2020, plants were hand-harvested and seeds obtained with a threshing machine (LPR UMB, Almaco, Nevada, IA, USA). On 19 October 2021, plots were harvested with a small plot combine. Seeds were then manually inspected to remove debris, placed in individual paper bags for each plot and brought back to the laboratory for assessment of yield. Total yield (ton per ha) was obtained by weighing all the seeds from a plot on a scale (Scout Pro SP 4001, Ohaus Corp., Pine Brook, NJ, USA). Seed size (g) was estimated by separately weighing three sub-samples of 100 seeds from each plot (i.e., 100-seed weight). Total number of seeds for each plot (i.e., seed number) was calculated using the total weight of seeds for each plot and the 100-seed weight.
As mentioned, plants in the caged soybean plots were inspected for the presence of other insects one day before or one day after collection of spectral data. Aphis glycines Matsumura (Hemiptera: Aphididae) was present in some of the cages, but no honeydew was observed on the plants. For the purposes of the present study, plots with average A. glycines densities above 20 aphids/plant were removed to avoid potential confounding effects of A. glycines feeding on soybean spectral reflectance. This threshold was used because previous research indicated that densities lower than 20 aphids/plant have negligible effects on soybean canopy reflectance (20, 32). A total of 3, 8 and 7 plots with average A. glycines densities above 20 aphids/plant were removed from 4 September 2020, 17 August 2021 and 30 August 2021, respectively. Thus, the total number of plots analyzed was 16 uninfested and 16 infested with P. japonica adults on 30 July 2020, 15 uninfested and 14 infested on 04 September 2020, 7 uninfested and 9 infested on 17 August 2021, and 10 uninfested and 7 infested on 30 August 2021. However, to account for the potential effects of A. glycines, even at low numbers (i.e., densities lower than 20 aphids/plant), on the spectral reflectance of soybean, A. glycines density was also included in the analyses (see below).
All analyses were performed and graphs made using the software R version 3.5.1 (33) and RStudio Desktop version 1.1.463 (34). For each date in each year, stratum-specific injury (i.e., lower, middle, and upper canopy), total canopy injury, and spectral reflectance of the canopy were evaluated using variable dispersion beta regression models with a logit link function (package, code: betareg, betareg (35)). These response variables were included in the models as proportions (i.e., values between 0 and 1). For stratum-specific injury, infestation status (i.e., uninfested or infested with P. japonica), canopy stratum (i.e., lower, middle, or upper canopy) and their interaction were included as explanatory variables; and infestation status was included as an additional regressor for the estimation of the model precision parameter. For total injury, infestation status was used both as the explanatory variable and for the estimation of the model precision parameter. For spectral reflectance of the canopy, wavelengths and vegetation indices were analyzed separately, with total canopy injury used both as the explanatory variable and for the estimation of the model precision parameter. The inclusion of a precision parameter significantly improved the models, which was checked via a likelihood-ratio test comparing the full model with and without this parameter (lmtest, lrtest (36)). Block, Aphis glycines density and its interaction with total canopy injury were initially included in the models as explanatory variables, but they were overall non-significant (P > 0.05) and therefore removed from the models. Model assumptions were assessed with diagnostic plots of residuals. Similarly to Geissinger et al. (2022) (37), the significance of explanatory variables for stratum-specific injury was obtained via sequential nested likelihood-ratio tests (lmtest, lrtest (36)), and mean separation tests with P-values of pairwise comparisons adjusted with the Tukey method were done using estimated marginal means (α = 0.05) (emmeans, emmeans (38)). The significance of explanatory variables for total canopy injury and spectral reflectance of the canopy was estimated with partial Wald tests (stats, summary (33)).
Seed number, 100-seed weight, and total yield were analyzed with general linear models (stats, lm (33)) with injury (%) as the explanatory variable. Block was initially included in the models, but it was overall non-significant and therefore removed from the models. Linear model assumptions were visually checked with residual and quantile-quantile scatterplots, and formally with a global validation test (gvlma, gvlma (39)). The presence of outliers was assessed a priori with a Bonferroni outlier test (car, outlierTest (40)). One observation was indicated as an outlier and model assumptions were accepted after its removal.
The interaction between P. japonica infestation status and canopy stratum was significant on 4 September 2020 and 17 August 2021 (P < 0.001) (Table 2). However, on 30 July 2020 and 30 August 2021, the interaction between P. japonica infestation status and canopy stratum was not significant. On these two dates, canopy injury of soybean was significantly affected by P. japonica infestation status (P < 0.001) and canopy stratum (i.e., lower, middle, and upper canopy) (P < 0.001) (Table 2). Overall, injury in uninfested plots did not differ among canopy strata, but injury in plots infested with P. japonica was greater in the upper stratum of the soybean canopy (Figure 1). Across the two years, mean canopy injury ranged from 0.13 to 0.37%, 0.59 to 0.97%, and 0.25 to 1.40% in the lower, middle, and upper strata, respectively, in uninfested plots (Figure 1). For plots infested with P. japonica adults, mean canopy injury ranged from 0.95 to 1.35%, 1.58 to 5.45%, and 4.46 to 12.00% in the lower, middle, and upper strata, respectively, across the two years (Figure 1). Similarly, mean total canopy injury (i.e., across lower, middle, and upper strata of the canopy) ranged from 0.42 to 0.91% and from 2.28 to 6.51% in uninfested and infested plots, respectively, and was significantly higher in plots infested with P. japonica adults (P < 0.001) (Table 2 and Figure 1).
Table 2 Likelihood ratio tests of beta regression models testing the effect of Popillia japonica infestation status (i.e., infested or unifested), canopy stratum (i.e., lower, middle, or upper canopy) and their interaction on feeding injury within the canopy, and of P. japonica infestation status on total feeding injury in plots of caged soybean plants in the field during 2020 and 2021 in Saint Paul, MN and Rosemount, MN, respectively.
Figure 1 Percentage of leaf area injured in the lower (Low), middle (Mid), upper (Up) and total (i.e., across lower, middle, and upper) canopy in plots of caged soybean plants in the field that were uninfested or infested with Popillia japonica on two sample dates in each of 2020 and 2021. Different letters within each graph indicate significant differences among lower, middle, and upper canopy strata according to the Tukey’s test (P < 0.05). Asterisks indicate significant differences within each graph between infestation treatments for total canopy injury according to the Tukey’s test (P < 0.05).
On 30 July 2020, spectral reflectance of the soybean canopy was not affected by injury from P. japonica. On 4 September 2020, a significant decrease in reflectance was observed at wavelengths above 723 nm. In 2021, a significant increase in reflectance was observed at wavelengths below 420 nm, and a significant decrease in reflectance from 722 to 898 nm on 17 August. On 30 August 2021, a significant increase in reflectance was generally observed from 427 to 529 nm and from 563 to 698 nm, and a significant decrease in reflectance from 735 to 944 nm (Figure 2). Across the three sample dates with significant effects of injury from P. japonica on spectral reflectance, the highest pseudo coefficients of determination (R2) were observed for wavelengths around the 780 nm region (Figure 2).
Figure 2 Estimates and 95% confidence intervals of intercepts and slopes, and pseudo coefficients of determination (R2) from beta regression models testing the effect of feeding injury from Popillia japonica on canopy spectral reflectance in plots of caged soybean plants in the field across 676 narrowband wavelengths on two sample dates in each of 2020 and 2021. Black horizontal bars within graphs in the middle column indicate that estimates of slopes for wavelengths differ from zero according to partial Wald tests (P < 0.05).
The vegetation indices were generally not affected by injury from P. japonica in 2020, except for MCARI on 4 September 2020 (Table 3). On this date, injury from P. japonica significantly decreased MCARI. In 2021, a significant reduction was also observed for NDVI, GNDVI (Green Normalized Difference Vegetation Index) and NDRE (Normalized Difference Red Edge) with increasing injury on 30 August (Table 3). Pseudo coefficients of determination (R2) values ranged from 0.001 to 0.172 in 2020, and from 0.003 to 0.304 in 2021 (Table 3). Overall, higher pseudo R2 were observed around 780 nm.
Table 3 Summary outputs of beta regression models testing the effects of Popillia japonica feeding injury on vegetation indices from canopy spectral reflectance in plots of caged soybean plants in the field during 2020 and 2021 in Saint Paul, MN and Rosemount, MN, respectively.
In 2020, injury from P. japonica adults did not affect seed number (mean: 2.52 x 107; range: 1.86 x 105 – 3.04 x 107), 100-seed weight (mean: 16.60 g; range: 13.92 – 18.70 g), or total yield (mean: 4.17 ton per ha; range: 3.21 – 5.05 ton per ha) of soybean (Table 4). A similar result was found in 2021 for seed number (mean: 1.90 x 107; range: 1.39 x 107 – 2.28 x 107) and total yield (mean: 3.24 ton per ha; range: 2.55 – 4.01 ton per ha). However, a significant effect was detected for 100-seed weight (mean: 17.06 g; range: 15.55 – 18.36 g), which decreased 1.6 g for every 10% increase in injury (Table 4).
Table 4 Summary outputs from general linear models estimating the effects of feeding injury (%) from Popillia japonica adults on seed number (seeds/ha x 106), 100-seed weight (g) and total yield (ton/ha) in plots of caged soybean plants in the field during 2020 (n = 28) and 2021 (n = 17) in Saint Paul, MN and Rosemount, MN, respectively.
Popillia japonica is an invasive insect species of global concern for food crops in North America (1, 2) and, more recently, Europe (4). In this study, feeding injury by P. japonica was detectable and greater in the upper stratum of the canopy of soybean, with potential effects on canopy spectral reflectance and minimal effects on total yield and seed quality. This is consistent with the top-down feeding patterns well documented for P. japonica on a range of host plants (1).
Overall mean canopy injury ranged from 0.23 to 6.26%. The levels of injury attained in this study are representative of levels of P. japonica injury observed in soybean fields in the Midwest (41, 42). Such levels of injury (i.e., overall mean canopy injury < 15%) are also commonly observed in other crops fed on by P. japonica (28, 43). In soybean, typical infestation levels of P. japonica may not be a threat to yield (11). However, P. japonica is part of a complex of defoliating pests that, in combination, can result in defoliation greater than the economic thresholds currently adopted in the Midwest (i.e., 30% before bloom or 20% from bloom to pod fill (6)).
Leaf injury reduces photosynthetic area of plants and can cause disturbance of physiological processes including water and nutrient transportation, as well as eliciting the expression of defense responses, which are energetically costly and therefore reduce plant efficiency (9). Popillia japonica feeding was previously found to increase transpiration and consequently water loss in soybean leaflets without affecting carbon assimilation rates or photosynthetic efficiency (9). However, physiological effects observed at the leaf level are not necessarily reflected at the canopy level. For example, Ostlie and Pedigo (1984) (44) observed higher transpiration from soybean leaflets following artificial defoliation; but artificial defoliation or actual feeding by the green cloverworm, Hypena scabra (Fabricius) (Lepidoptera: Noctuidae), and the cabbage looper, Trichoplusia ni (Hubner) (Lepidoptera: Noctuidae), actually decreased canopy transpiration. Similarly, Klubertanz et al. (1996) (45) observed higher soil moisture in potted soybean plants following artificial defoliation.
The morphophysiological changes of plants caused by biotic or abiotic stresses can alter the spectral reflectance of plants including the visible and near infra-red ranges of the electromagnetic spectrum (15). For insect pests, this effect seems to be density-dependent as shown for A. glycines (20) and two lepidopteran pests (26) in soybean. In this study, a significant increase in soybean canopy reflectance of plants fed on by P. japonica at wavelengths in the visible range was observed, but this effect was inconsistent. However, a decrease in reflectance in the near infra-red associated with an increase in canopy injury from P. japonica was observed in three of the four dates across the two years. Lack of spectral response on the first sample date in 2020 was likely due to the low levels of injury observed on this date (Figure 1). Similarly, feeding injury by two lepidopteran species increased the visible and decreased near infra-red reflectance of soybean leaves in a greenhouse experiment (26). In contrast, an increase in both visible and near infra-red reflectance of leaves of peanut, Arachis hypogaea (L.) (Fabales: Fabaceae), was observed following injury by Stegasta bosqueella (Chambers) (Lepidoptera: Gelechiidae) and S. cosmioides (Walker) in the greenhouse (23).
Feeding injury from P. japonica to soybean tended to reduce spectral reflectance in the canopy in near infra-red wavelengths (~700 to 1000 nm). Furthermore, higher pseudo coefficients of determination (R2) around 780 nm indicate that this region is optimal for the detection of P. japonica injury in soybean. Canopy reflectance at 780 nm has also been shown to be optimal for the detection of A. glycines in soybean (46). Although A. glycines was present at densities lower than 20 aphids per plant in some plots used in this study, this effect was non-significant across all dates. For this reason, the results presented here are due to the effects of P. japonica injury alone. This corroborates previous findings indicating that A. glycines at densities lower than 20 aphids per plant result in negligible effects on soybean canopy reflectance (20, 32). Nevertheless, such overlapping effects on canopy spectral reflectance around 780 nm suggests that P. japonica feeding resulting in mean canopy injury of ≥ 5% may confound the detection of A. glycines or other herbivores in soybean. Thus, further investigation of spectral data that includes P. japonica injury coincident with other pests such as A. glycines is needed.
Inconsistent results were observed for the vegetation indices evaluated for the effects of P. japonica injury on soybean canopy reflectance. This lack of a consistent effect is probably because the vegetation indices tested here incorporate reflectance from the visible spectrum. Previous studies found the vegetation index NDVI is associated with the distribution of three lepidopteran pests, but not with their feeding injury in soybean fields (25). In cotton, differences in NDVI were observed for plants fed on by Spodoptera exigua (Hubner) (Lepidoptera: Noctuidae), but not for T. ni in the field (47).
Taken together, these findings indicate that plant spectral responses to defoliation are likely species-dependent, and care should be taken when generalizing across species of plants and defoliators. Furthermore, some of the results documented in the cited literature come from leaf measurements performed in the laboratory. Comparisons of canopy spectral reflectance obtained in the field to laboratory measurements can further create discrepancies between studies.
In this study, effects of P. japonica feeding injury on total yield were not observed, but a reduction in seed size was detected in one of the two years. Although soybean is tolerant to defoliation (48), the spatiotemporal distribution of canopy defoliation seems to have a differential impact on soybean yield. The intensity of feeding injury from P. japonica was quantified among strata of the soybean canopy more thoroughly than in previous studies. The lack of an effect of P. japonica feeding on total soybean yield confirms that contemporary soybean varieties likely respond similarly to this pest as those studied in the past.
In conclusion, near infra-red wavelengths may hold promise for remote sensing of P. japonica feeding injury in soybean. Because remote sensing can also be affected by other soybean pests, further studies incorporating near infra-red wavelengths and standard red, green and blue (RGB) imagery to differentiate P. japonica injury from that of other defoliators in soybean are needed. These results can facilitate refinement of management recommendations for P. japonica in soybean.
The raw data supporting the conclusions of this article will be made available by the authors, without undue reservation.
AR, investigation, formal analysis, writing (original draft, review, and editing). TC, conceptualization, methodology, investigation, writing (review and editing). IM, conceptualization, writing (review and editing). RK, project administration, funding acquisition, conceptualization, methodology, writing (original draft, review, and editing). All authors contributed to the article and approved the submitted version.
Funding for this research was provided by the Minnesota Soybean Research and Promotion Council and the Minnesota Invasive Terrestrial Plants and Pests Center through the Minnesota Environment and Natural Resources Trust Fund.
We thank Rosa (Tina) Lozano, Rafael Carlesso Aita, James Menger, Grace Doyle, Samantha Janecek, Gunnar Morris, Ellen Adjeiwaa and Gloria Melotto for their help during data collection.
The authors declare that the research was conducted in the absence of any commercial or financial relationships that could be construed as a potential conflict of interest.
All claims expressed in this article are solely those of the authors and do not necessarily represent those of their affiliated organizations, or those of the publisher, the editors and the reviewers. Any product that may be evaluated in this article, or claim that may be made by its manufacturer, is not guaranteed or endorsed by the publisher.
1. Potter DA, Held DW. Biology and management of the japanese beetle. Annu Rev Entomol (2002) 47:175–205. doi: 10.1146/annurev.ento.47.091201.145153
2. Althoff ER, Rice KB. Japanese Beetle (Coleoptera: Scarabaeidae) invasion of north America: History, ecology, and management. J Integr Pest Manag (2022) 13:2. doi: 10.1093/jipm/pmab043
3. EPPO. First report of popillia japonica in Italy. EPPO Report Service (2014) 10:179. Available at: https://gd.eppo.int/reporting/article-3272.
4. EPPO. Popillia japonica. EPPO global database (2022). Available at: https://gd.eppo.int/taxon/POPIJA/distribution.
5. Kistner-Thomas EJ. The potential global distribution and voltinism of the Japanese beetle (Coleoptera: Scarabaeidae) under current and future climates. J Insect Sci (2019) 19:16. doi: 10.1093/jisesa/iez023
6. Shanovich HN, Dean AN, Koch RL, Hodgson EW. Biology and management of Japanese beetle (Coleoptera: Scarabaeidae) in corn and soybean. J Integr Pest Manag (2019) 10:9. doi: 10.1093/jipm/pmz009
7. Shea Z, Singer WM, Zhang B. “Soybean production, versatility, and improvement”. In: Hasanuzzaman M, editor. Legume crops - prospects, production and uses. London: IntechOpen (2020). doi: 10.5772/intechopen.91778
8. FAO. FAOSTAT statistical database (2022). Available at: https://www.fao.org/faostat/en/#data/QCL.
9. Aldea M, Hamilton JG, Resti JP, Zangerl AR, Berenbaum MR, DeLucia EH. Indirect effects of insect herbivory on leaf gas exchange in soybean. Plant Cell Environ (2005) 28:402–11. doi: 10.1111/j.1365-3040.2005.01279.x
10. Gould GE. Japanese Beetle damage to soybeans and corn. J Econ Entomol (1963) 56:776–81. doi: 10.1093/jee/56.6.776
11. Hammond RB. “Japanese beetle”. In: Higley LG, Boethel DJ, editors. Handbook of soybean insect pests. Lanham, MD: Entomological Society of America (1994). p. 64–5.
12. Mulla DJ. Twenty five years of remote sensing in precision agriculture: key advances and remaining knowledge gaps. Biosyst Eng (2013) 114:358–71. doi: 10.1016/j.biosystemseng.2012.08.009
13. Zhang J, Huang Y, Pu R, Gonzalez-Moreno P, Yuan L, Wu K, et al. Monitoring plant diseases and pests through remote sensing technology: a review. Comput Electron Agric (2019) 165:104943. doi: 10.1016/j.compag.2019.104943
14. Abd El-Ghany NM, Abd El-Aziz SE, Marei SS. A review: application of remote sensing as a promising strategy for insect pests and diseases management. Environ Sci pollut Res (2020) 27:33503–15. doi: 10.1007/s11356-020-09517-2
15. Rhodes MW, Bennie JJ, Spalding A, ffrench-Constant RH, Maclean IMD. Recent advances in the remote sensing of insects. Biol Rev (2022) 97:343–60. doi: 10.1111/brv.12802
16. Reisig D, Godfrey L. Spectral response of cotton aphid-(Homoptera: Aphididae) and spider mite-(Acari: Tetranychidae) infested cotton: controlled studies. Environ Entomol (2007) 36:1466–74. doi: 10.1603/0046-225X(2007)36[1466:SROCAH]2.0.CO;2
17. Yang C-M, Cheng C-H, Chen R-K. Changes in spectral characteristics of rice canopy infested with brown planthopper and leaffolder. Crop Sci (2007) 47:329–35. doi: 10.2135/cropsci2006.05.0335
18. Huang J, Liao H, Zhu Y, Sun J, Sun Q, Liu X. Hyperspectral detection of rice damaged by rice leaf folder (Cnaphalocrocis medinalis). Comput Electron Agric (2012) 82:100–7. doi: 10.1016/j.compag.2012.01.002
19. Krishna G, Sahoo RN, Pargal S, Gupta VK, Sinha P, Bhagat S, et al. Assessing wheat yellow rust disease through hyperspectral remote sensing. Int Arch Photogramm Remote Sens Spat Inf Sci (2014) XL–8:1413–6. doi: 10.5194/isprsarchives-XL-8-1413-2014
20. Alves TM, Macrae IV, Koch RL. Soybean aphid (Hemiptera: Aphididae) affects soybean spectral reflectance. J Econ Entomol (2015) 108:2655–64. doi: 10.1093/jee/tov250
21. Ramos APM, Gomes FDG, Pinheiro MMF, Furuya DEG, Gonçalvez WN, Marcato Junior J, et al. Detecting the attack of the fall armyworm (Spodoptera frugiperda) in cotton plants with machine learning and spectral measurements. Precis Agric (2022) 23:470–91. doi: 10.1007/s11119-021-09845-4
22. Board JE, Maka V, Price R, Knight D, Baur ME. Development of vegetation indices for identifying insect infestations in soybean. Agron J (2007) 99:650–6. doi: 10.2134/agronj2006.0155
23. Pinto J, Powell S, Peterson R, Rosalen D, Fernandes O. Detection of defoliation injury in peanut with hyperspectral proximal remote sensing. Remote Sens (2020) 12:3828. doi: 10.3390/rs12223828
24. Furuya DEG, Ma L, Pinheiro MMF, Gomes FDG, Gonçalvez WN, Marcato Junior J, et al. Prediction of insect-herbivory-damage and insect-type attack in maize plants using hyperspectral data. Int J Appl Earth Obs Geoinf (2021) 105:102608. doi: 10.1016/j.jag.2021.102608
25. Greene AD, Reay-Jones FPF, Kirk KR, Peoples BK, Greene JK. Spatial associations of key lepidopteran pests with defoliation, NDVI, and plant height in soybean. Environ Entomol (2021) 50:1378–92. doi: 10.1093/ee/nvab098
26. Iost Filho FH, Pazini J de B, de MAD, DL R, Yamamoto PT. Assessment of injury by four major pests in soybean plants using hyperspectral proximal imaging. Agronomy (2022) 12:1516. doi: 10.3390/agronomy12071516
27. Fehr WR, Caviness CE. Stages of soybean development. Ames, IA: Cooperative Extension Service (1977).
28. Ebbenga DN, Burkness EC, Clark MD, Hutchison WD. Impact of adult Popillia japonica (Coleoptera: Scarabaeidae) foliar feeding injury on fruit yield and quality of a temperate, cold-hardy wine grape, ‘Frontenac’’. Front Insect Sci (2022) 2:887659. doi: 10.3389/finsc.2022.887659
29. Liu S, Yu H, Sui Y, Zhou H, Zhang J, Kong L, et al. Classification of soybean frogeye leaf spot disease using leaf hyperspectral reflectance. PloS One (2021) 16:e0257008. doi: 10.1371/journal.pone.0257008
30. Vigier BJ, Pattey E, Strachan IB. Narrowband vegetation indexes and detection of disease damage in soybeans. IEEE Geosci Remote Sens Lett (2004) 1:255–9. doi: 10.1109/LGRS.2004.833776
31. Getman-Pickering ZL, Campbell A, Aflitto N, Grele A, Davis JK, Ugine TA. LeafByte: a mobile application that measures leaf area and herbivory quickly and accurately. Methods Ecol Evol (2020) 11:215–21. doi: 10.1111/2041-210X.13340
32. Marston ZPD, Cira TM, Hodgson EW, Knight JF, Macrae IV, Koch RL. Detection of stress induced by soybean aphid (Hemiptera: Aphididae) using multispectral imagery from unmanned aerial vehicles. J Econ Entomol (2020) 113:779–86. doi: 10.1093/jee/toz306
33. R Core Team. R: a language and environment for statistical computing (2021). Available at: https://www.r-project.org/.
34. RStudio Team. RStudio: integrated development for r (2018). Available at: http://www.rstudio.com.
35. Cribari-Neto F, Zeileis A. Beta regression in r. J Stat Softw (2010) 34:1–24. doi: 10.18637/jss.v034.i02
36. Zeileis A, Hothorn T. Diagnostic checking in regression relationships. R News (2002) 2:7–10. Available at: https://cran.r-project.org/web/packages/lmtest/vignettes/lmtest-intro.pdf.
37. Geissinger EA, Khoo CLL, Richmond IC, Faulkner SJM, Schneider DC. A case for beta regression in the natural sciences. Ecosphere (2022) 13:e3940. doi: 10.1002/ecs2.3940
38. Lenth RV, Buerkner P, Herve M, Love J, Riebl H, Singmann H. Emmeans: estimated marginal means, aka least-squares means. CRAN (2020). Available at: https://cran.r-project.org/web/packages/emmeans/index.html.
39. Pena EA, Slate EH. Gvlma: global validation of linear models assumptions. CRAN (2019). Available at: https://cran.r-project.org/package=gvlma.
40. Fox J, Weisberg S. An r companion to applied regression. Sage: Thousand Oaks (2019). Available at: https://socialsciences.mcmaster.ca/jfox/Books/Companion/.
41. Hodgson EW, VanNostrand GR, Dean A, Berns KR. Japanese Beetle insecticide efficacy evaluation in Northeast Iowa Vol. 1 2019. Ames: Iowa State Univ Res Demonstr Farms Prog Reports (2020). Available at: https://www.iastatedigitalpress.com/farmreports/article/id/11345/.
42. Hodgson EW, VanNostrand GR, Dean A. Japanese Beetle insecticide efficacy evaluation in central Iowa Vol. 1 2020. Ames:Iowa State Univ Res Demonstr Farms Prog Reports (2021). Available at: https://www.iastatedigitalpress.com/farmreports/article/id/12547/.
43. Shanovich HN, Ribeiro AV, Koch RL. Seasonal abundance, defoliation, and parasitism of Japanese beetle (Coleoptera: Scarabaeidae) in two apple cultivars. J Econ Entomol (2021) 114:811–7. doi: 10.1093/jee/toaa315
44. Ostlie KR, Pedigo LP. Water loss from soybeans after simulated and actual insect defoliation. Environ Entomol (1984) 13:1675–80. doi: 10.1093/ee/13.6.1675
45. Klubertanz TH, Pedigo LP, Carlson RE. Soybean physiology, regrowth, and senescence in response to defoliation. Agron J (1996) 88:577–82. doi: 10.2134/agronj1996.00021962008800040014x
46. Alves TM, Moon RD, MacRae IV, Koch RL. Optimizing band selection for spectral detection of Aphis glycines matsumura in soybean. Pest Manag Sci (2019) 75:942–9. doi: 10.1002/ps.5198
47. Sudbrink DL, Harris FA, Robbins JT, English PJ, Willers JL. Evaluation of remote sensing to identify variability in cotton plant growth and correlation with larval densities of beet armyworm and cabbage looper (Lepidoptera:Noctuidae). Florida Entomol (2003) 86:290–4. doi: 10.1653/0015-4040(2003)086[0290:EORSTI]2.0.CO;2
Keywords: herbivory, integrated pest management, Japanese beetle, remote sensing, yield
Citation: Ribeiro AV, Cira TM, MacRae IV and Koch RL (2022) Effects of feeding injury from Popillia japonica (Coleoptera: Scarabaeidae) on soybean spectral reflectance and yield. Front. Insect Sci. 2:1006092. doi: 10.3389/finsc.2022.1006092
Received: 28 July 2022; Accepted: 05 October 2022;
Published: 20 October 2022.
Edited by:
David Held, Auburn University, United StatesReviewed by:
Lara Maistrello, University of Modena and Reggio Emilia, ItalyCopyright © 2022 Ribeiro, Cira, MacRae and Koch. This is an open-access article distributed under the terms of the Creative Commons Attribution License (CC BY). The use, distribution or reproduction in other forums is permitted, provided the original author(s) and the copyright owner(s) are credited and that the original publication in this journal is cited, in accordance with accepted academic practice. No use, distribution or reproduction is permitted which does not comply with these terms.
*Correspondence: Robert L. Koch, a29jaDAxMjVAdW1uLmVkdQ==
†Present address: Theresa M. Cira, Pesticide and Fertilizer Management Division, Department of Agriculture, Saint Paul, MN, United States
Disclaimer: All claims expressed in this article are solely those of the authors and do not necessarily represent those of their affiliated organizations, or those of the publisher, the editors and the reviewers. Any product that may be evaluated in this article or claim that may be made by its manufacturer is not guaranteed or endorsed by the publisher.
Research integrity at Frontiers
Learn more about the work of our research integrity team to safeguard the quality of each article we publish.