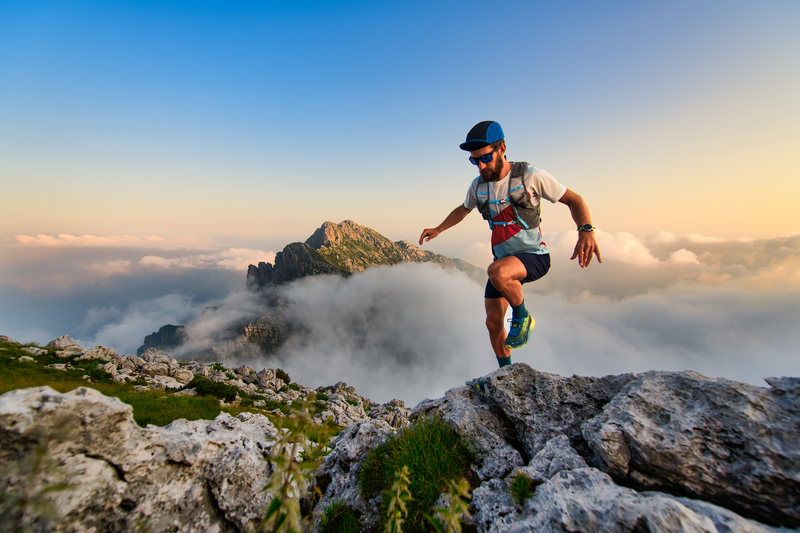
95% of researchers rate our articles as excellent or good
Learn more about the work of our research integrity team to safeguard the quality of each article we publish.
Find out more
ORIGINAL RESEARCH article
Front. Immunol. , 07 March 2025
Sec. Cancer Immunity and Immunotherapy
Volume 16 - 2025 | https://doi.org/10.3389/fimmu.2025.1561333
Purpose: In this study, we aimed to develop a predictive model for patients receiving chemotherapy and immunotherapy for extensive-stage small cell lung cancer
Methods: We retrospectively analyzed 112 extensive-stage small cell lung cancer patients treated with first-line immunotherapy and chemotherapy. The relevant clinical data were collected to evaluate the changes during the treatment. The best subset regression, univariate analysis, and LASSO regression with cross-validation were applied for variable selection and model establishment. The nomograms for 1- and 2-year survival probabilities were established, and the calibration curve was utilized to evaluate the correspondence between actual and predicted survival. The model prediction capacity was assessed using decision curve analysis, calibration curves, and receiver operating characteristic curves. Moreover, five-fold cross-validation was conducted for internal validation. According to risk score, the patients were assigned to high- and low-risk groups, and survival curves were generated for each group.
Results: The LASSO regression model was established based on the variables such as age, ECOG, metastatic sites, NLR, and immunotherapy cycles. This predictive model displayed robust performance, evidenced by the Area Under the Curve of 0.887 and concordance index of 0.759. The nomogram effectively predicted 1- and 2-year survival probabilities and demonstrated a high degree of calibration. The decision curve analysis displayed that the model possessed superior predictive capability. The risk stratification for patients with high- and low-risk categories facilitated more individualized survival assessment.
Conclusion: The study successfully developed a prognostic model for extensive-stage small cell lung cancer patients undergoing immunotherapy and chemotherapy, demonstrating the good accuracy and predictability.
Approximately 15% of worldwide lung cancer cases are small cell lung cancer (SCLC), exhibiting a high degree of invasiveness and rapid progression (1, 2). It is distinguished by its poor prognosis and early metastatic potential. According to the feasibility of covering an irradiation field, SCLC patients are frequently categorized into limited-stage disease and extensive-stage disease. Approximately 70% of all SCLC cases are classified as extensive-stage (3). For extensive-stage SCLC (ES-SCLC) patients, first-line platinum-based chemotherapy regimens have served as the standard treatment option. Over the past 20 years, the median overall survival (mOS) for patients with ES-SCLC following conventional treatment has maintained between 9 and 11 months (4). Immunotherapy has revolutionized the cancer treatment paradigm and has shown promising activity in numerous solid malignancies, including SCLC. The results of CASPIAN and IMpower133 trials suggested that the combination of chemotherapy and immunotherapy improved mOS by two months compared to chemotherapy alone in ES-SCLC patients (5, 6). As a result, according to the National Comprehensive Cancer Network (NCCN) guidelines, platinum-based chemotherapy with etoposide and durvalumab or atezolizumab was considered as the standard first-line treatment for ES-SCLC (7).
Only a few biomarkers, such as programmed death-ligand 1 (PD-L1) immuno-histochemistry (IHC) expression, tumor mutational burden (TMB), and circulating tumor DNA (ctDNA), have been investigated in relation to immunotherapy to predict the clinical prognosis of SCLC (3, 8, 9).
The principal objective of this study is to develop a predictive model for assessing the response of patients with ES-SCLC to initial chemotherapy and immunotherapy treatments. The proposed conceptual model aims to aid healthcare professionals in devising personalized treatment strategies by identifying potential biomarkers and clinical predictors tailored to the individual needs of each patient. This approach has the potential to enhance therapeutic outcomes and improve the overall prognosis of the disease.
The study participants included patients diagnosed with ES-SCLC at Tianjin Medical University Cancer Institute between 2018 and 2022. Inclusion criteria: (1) patients had to be 18 years and above; (2) diagnosis with an extensive stage based on the Veterans Administration Lung Cancer Group (VALG) staging criteria; (3) Eastern Cooperative Oncology Group (ECOG) performance status of 0-2; (4) receipt of chemotherapy and immunotherapy as their initial treatment. Exclusion criteria: (1) prior neoplastic or autoimmune disease. (2) receipt of anti-tumor treatment in the past, including chemotherapy, radiotherapy, and immunotherapy. This investigation was authorized by the Institutional Review Board (IRB) of Tianjin Medical University Cancer Institute and Hospital (approval number: bc2022244).
Collection of clinical data in this study included ECOG performance status (PS), age, smoking history, gender, co-morbidities (such as hypertension, diabetes, and coronary heart disease), a familial predisposition to lung cancer, weight loss, disease stage, tumor localization (specifically left or right lung and lung lobe location), presence of oligometastases, metastatic sites (including brain, bone, liver, adrenal gland, abdomen, pleural fluid, and pleural nodules), neutrophil-to-lymphocyte ratio (NLR), monocyte-to-lymphocyte ratio (MLR), lactate dehydrogenase (LDH) levels, albumin (ALB) levels, platelet-to-lymphocyte ratio (PLR), number of immunotherapy cycles, systemic immune-inflammation index (SII), and the response to first-line immunotherapy. Overall survival (OS) was the primary endpoint, defined as the time interval between initiation of immunotherapy and death or the final follow-up date.
Variable selection was performed using three methods: Simple regression analysis, best subset regression analysis, and LASSO regression analysis using cross-validation techniques. All the selected variables were employed to develop a survival probability model for survival rates of 1 and 2 years. In order to measure the performance of the model, three tools were used: the calibration curve, the decision curve analysis (DCA), and the receiver operating characteristic (ROC) curve analysis. After analyzing the model’s performance based on the ROC curve using the variables selected via three distinct approaches, the best model was determined according to the maximum area under the curve (AUC). The outcomes for patients were evaluated using the nomogram. The decision, calibration, and ROC curves were utilized for assessing the model’s discriminative capacity. The internal validation was performed utilizing cross-validation presented as scatter plot. All patients were rated using the previously indicated model, and the R software was utilized to identify the optimal cutoff value. The final model classified patients into high- or low-risk categories. To compare the survival probabilities for these groups, the Kaplan-Meier curve was plotted as well.
R version 4. 1. 3 was utilized to perform the statistical analysis in this study. P value less than 0.05 is considered to be statistically significant. By dividing the medians using Kaplan-Meier, the variables, which included LDH, ALB, NLR, PLR, MLR, and SII, were stratified into high- and low-risk groups. These estimates comprised survival rates at one and two years and the corresponding 95% CI, produced using the Kaplan–Meier analysis.
This research examined 112 individuals with ES-SCLC. The median age of the participants was 63 years (IQR 57.7, 68.0), with 81.2% of them being male. An ECOG PS score of 1 (n = 102, 91.1%) was obtained for the majority of patients. As shown in Table 1, the baseline characteristics of these participants were summarized. By the date of last follow-up, 54 patients died, 24 patients were alive with disease progression, and 34 patients were alive without disease progression. A median progression-free survival (PFS) of 4.7 months (95% CI, 4.0-5.7) and an overall survival (OS) of 12.1 months (95% CI, 10.4-15.4) were reported. The OS rates at 1 and 2 years were 60.1% and 29.4%, respectively.
Three methods were used for variable screening: univariate analysis, optimal subset regression, and LASSO regression with cross-validation. All significant variables had a p-value < 0.05 in the univariate analysis. Age, LDH, NLR, SII, hepatic metastases, and the number of immunotherapy rounds were among the significant variables. The best subset regression model was determined based on specific criteria, which included a minimum CP value of 12, a maximum adjusted R2 value of 0.3, and a minimum Bayesian Information Criterion value of -6.1. Nine variables were shown to be the most effective combination (Figure 1). These were weight loss, N3, M3, stage 3, stage 4, bone metastasis, hepatic metastasis, adrenal metastasis, and partial response (PR).
A model was constructed through Lasso regression, with the initial variables comprising elderly, ECOG PS, brain metastases, hepatic metastasis, NLR, and immunotherapy cycles (Figure 2). The Akaike’s Information Criteria (AIC) values of the three models were compared using COX regression, and the ROC curve analysis was utilized for assessing the models’ performance. The model with the highest AUC value and the lowest AIC was deemed optimal (Figure 3). Furthermore, the variables identified through LASSO regression screening were used to construct the nomogram.
Figure 3. Receiver operating characteristic (ROC) curves for the three models: “COX”, “BSR” and “Lasso”.
To determine the probability of survival at one and two years, a nomogram was generated using the variables previously mentioned (Figure 4). The nomogram was titled “Predictive Model for Survival in Extensive-Stage SCLC Patients Undergoing Immunotherapy and Chemotherapy” (PMSSIC). Furthermore, an online tool was created based on the PMSSIC framework for the efficient operation of individual clinical predictions. The tool can be accessed at: https://gaoyunbinjiayou.shinyapps.io/DynNomapp/.
Figure 4. Nomogram for the predictive model for survival in ES-SCLC patients undergoing immunotherapy and chemotherapy (PMSSIC).
ROC analysis, calibration plots, and DCA were used to evaluate the nomogram’s stability (Figures 5A–C). This model’s 2-year survival probability showed that it had a robust discriminant power, as evidenced by the AUC value of 0.887 (with variance 0.777-0.997). DCA, which demonstrated a positive net benefit based on the probability of 2-year survival on the application of the model, was used to show the utility of the design. The model’s performance was further corroborated by internal cross-validation results, which show mean AUC values at the 1- and 2-year of 0.832 (range 0.686-0.940) and 0.906 (range 0.733-1.000), respectively (Figure 5D).
Figure 5. The evaluation and validation of the model. (A) Receiver operating curve for model prediction of 2-year survival. (B) Calibration curve for model prediction of 2-year survival. (C) Decision curve analysis for model prediction of 2-year survival. (D) Violin plot depicting the area under the curve (AUC) and C-index for 1- and 2-year intervals.
Therefore, the patients were allocated to high- and low-risk groups based on the RS that PMSSIC determined. Moreover, the result of Kaplan-Meier survival analysis indicated that the survival rate of the patients in low-risk group was significantly longer than that of high-risk group (P < 0.001, Figure 6). The high-risk group’s 1- and 2-year survival rates were 49.6% (95% CI: 38.7% to 63.4%) and 14.2% (95% CI: 6.8% to 29.7%), respectively, while the corresponding survival rates in the low-risk group were 100% (95% CI: 6.8% to 29.7%) and 90% (95% CI: 73.2% to 100%).
Figure 6. The Kaplan-Meier survival curves for overall survival (OS) in the high- and low-risk groups.
The chemotherapy-immunotherapy combination is considered one of the most effective multimodal treatment strategies for ES-SCLC, which has demonstrated significant effectiveness. By blocking the immune escape pathways, this approach increases the immune system’s response to cancer cells (10). However, this translates into a long-term survival benefit only in a minority of patients, with a lack of clinical predictive models developed for immunotherapy era in ES-SCLC. The limitations of the historical approach in assessing the efficacy of chemotherapy in terms of anti-tumor activity and overall survival as well as its inability to take into account the interactions and responses generated by immunotherapy have been widely acknowledged (11). Therefore, it is essential to develop more efficient predictive models to identify potentially promising candidates for chemo-immunotherapy combinations. By including possible biomarkers and combining clinical indicators like patient demographics and tumor features, these models can improve patient outcomes. Among the potential predictors that were considered in the present predictive model for ES-SCLC patients receiving first-line chemo-immunotherapy, several factors were revealed to be significant in terms of outcomes of the treatment. These variables include older people, ECOG PS, brain metastases, hepatic metastasis, NLR, and immunotherapy cycles.
Earlier investigations have shown the impact of these parameters on the treatment response and overall prognosis in ES-SCLC patients. For instance, elderly patients have lower rates of OS, most likely due to co-morbidities and treatment tolerance. Our study suggests a shorter survival for older people, corroborating the results from prior research (12, 13). Moreover, Longo et al. established an association between increased age and poor prognosis disease, although this was not confirmed by multivariate analysis (14). ECOG PS, referring to a measure of functional status, has been used as an independent predictive factor, with higher scores being associated with poorer outcomes. ECOG PS 1-2 patients had a medium-term survival rate of 68.1% and a long-term survival rate of 65.1%, while ECOG PS 3-4 patients had a medium-term survival rate of 18.1% and a long-term survival rate of 0% (15). Another study also demonstrated that patients with PS 2-3 showed significantly poorer OS compared to patients with PS 0-1 (16). Both the univariate and multivariate analyses found that PS was a significant prognostic factor; the present model showed a negative connection between PS score and OS.
The presence of brain as well as hepatic metastases is a hallmark of increased disease volume and reduced therapeutic efficacy. Individual research has demonstrated that liver and brain metastases are negative prognostic factors; however, these findings are limited to individuals treated with first-line systemic chemotherapy (15, 16). Moreover, there has not been a detailed investigation of the immunotherapy impacts on this component. The current study augmented this information and included it in the model to serve as a clinical point of reference.
It has been reported that inflammatory prognostic biomarkers have prognostic significance in a range of tumor types. Among NLR, PLR, SII, and other factors, only NLR was related to prognosis in this investigation. NLR represents the balance between the innate and adaptive immune systems. Among these, neutrophils release chemokines and cytokines, which are crucial for the advancement of cancer. In response to malignancy, lymphocytes can stimulate a cytotoxic immune response as well. It has been found that raised NLR is linked to poor prognosis after immunotherapy for ES-SCLC (17, 18), while a sharp decline in NLR is consistent with exceptional outcomes in patients. This study further confirms the role and value of NLR in immunotherapy.
In recent years, immunotherapy has made remarkable progress in cancer treatment, and the optimization of treatment strategies represents an important field of research (19). By understanding the patient’s immune status and tumor microenvironment, personalized treatment plans can be better developed, thus improving the effectiveness of immunotherapy (20). Previously, the duration of immunotherapy has primarily been determined based on expert judgment and estimation rather than a standardized prognostic model. The inclusion of immunotherapy cycles in the present predictive model represents a progression in mapping therapies for each patient. Accurately determining the optimal number of immunotherapy cycles is crucial for maximizing treatment efficacy and minimizing potential adverse effects. However, it is feasible to uncover studies that suggest a positive OS when many CT lines are used. There is a lack of information on the impact of the number of cycles of immunotherapy. By analyzing patient data, the results suggest that specific cohorts may benefit from extended immunotherapy regimens, while others may not derive such benefit in terms of long-term survival after a certain number of cycles. This nuanced approach allows for personalized treatment strategies that encompass both patient-specific characteristics and treatment duration, ultimately guiding clinical decision-making.
The model provides several potential benefits in the context of ES-SCLC. The present model incorporates multitudes of clinical variables, indicating a comprehensive method for predicting treatment outcomes. This aids physicians in evaluating the best suitable therapy and customizing treatment procedures. Furthermore, this model demonstrates a broad interest in ES-SCLC, particularly in the context of emerging guidelines of treatment involving first-line chemotherapy and immunotherapy. This model provides beneficial assistance for clinicians in improving therapeutic approaches by offering insights into the elements that influence treatment response in this unique scenario.
Based on our results, using the nomogram, patients with ED-SCLC who were treated with chemo-immunotherapy can be classified into good- and poor-responder groups. However, the role of immunotherapy in the different respond groups is not the purpose of our study. We cannot conclude that immunotherapy only add financial toxicity to poor-responder group of patients. Due to the limited number of patients included in this study, we cannot further evaluated the role of immunotherapy and obtain a sounded conclusion. So further study should be performed to evaluate the role of immunotherapy in the poor-responder group of patients and the role of other treatment modality such as thoracic radiation in this kind of patients also need to be studied.
However, it is important to emphasize some limitations that are related to this study model. First, this model was derived from historical data, and therefore, the information it provides may be susceptible to biases and other variables that might distort the actual situation. Further studies with more extensive patient data should be undertaken to validate the findings and improve the model. Moreover, it is essential to acknowledge that the data upon which the model was developed and evaluated was from a single-center population. Therefore, it would be necessary to conduct external validation to apply the model to different populations.
In conclusion, this model for immunotherapy combined with first-line chemo-therapy in ES-SCLC patients has potential as a method for predicting treatment results. These characteristics enhance the ability to evaluate the clinical situation and assist physicians in making more informed treatment choices. However, further investigation is needed to ascertain the dependability and efficacy of the proposed model to ensure its effectiveness in clinics and improve patient outcomes.
The original contributions presented in the study are included in the article/Supplementary Material. Further inquiries can be directed to the corresponding author.
The studies involving humans were approved by the Institutional Review Board (IRB) of Tianjin Medical University Cancer Institute and Hospital. The studies were conducted in accordance with the local legislation and institutional requirements. The ethics committee/institutional review board waived the requirement of written informed consent for participation from the participants or the participants’ legal guardians/next of kin because the retrospective nature of the study, the requirement for obtaining informed consent was waived.
YG: Conceptualization, Funding acquisition, Investigation, Methodology, Resources, Visualization, Writing – original draft, Writing – review & editing. LXZ: Data curation, Software, Visualization, Writing – review & editing. MY: Investigation, Methodology, Software, Validation, Writing – review & editing. ZS: Data curation, Formal Analysis, Project administration, Software, Validation, Writing – review & editing. HZ: Formal Analysis, Methodology, Project administration, Writing – review & editing. LJZ: Conceptualization, Funding acquisition, Investigation, Project administration, Resources, Supervision, Visualization, Writing – original draft, Writing – review & editing.
The author(s) declare that financial support was received for the research and/or publication of this article. This research was funded by Key R&D Program of Jining, grant number 2023YXNS195 and The APC was funded by Key R&D Program of Jining.
I wish to extend my sincere gratitude to Dr. Dawei Zhao and Dr. Xiaoyue Wu for their guidance on R programming language during the data processing phase of this paper.
The authors declare that the research was conducted in the absence of any commercial or financial relationships that could be construed as a potential conflict of interest.
The author(s) declare that no Generative AI was used in the creation of this manuscript.
All claims expressed in this article are solely those of the authors and do not necessarily represent those of their affiliated organizations, or those of the publisher, the editors and the reviewers. Any product that may be evaluated in this article, or claim that may be made by its manufacturer, is not guaranteed or endorsed by the publisher.
The Supplementary Material for this article can be found online at: https://www.frontiersin.org/articles/10.3389/fimmu.2025.1561333/full#supplementary-material
1. Bray F, Ferlay J, Soerjomataram I, Siegel RL, Torre LA, Jemal A. Global cancer statistics 2018: GLOBOCAN estimates of incidence and mortality worldwide for 36 cancers in 185 countries. CA Cancer J Clin. (2018) 68:394–424. doi: 10.3322/caac.21492
2. Rudin CM, Brambilla E, Faivre-Finn C, Sage J. Small-cell lung cancer. Nat Rev Dis Primers. (2021) 7:3. doi: 10.1038/s41572-020-00235-0
3. Zhu L, Qin J. Predictive biomarkers for immunotherapy response in extensive-stage SCLC. J Cancer Res Clin Oncol. (2024) 150:22. doi: 10.1007/s00432-023-05544-x
4. Farago AF, Keane FK. Current standards for clinical management of small cell lung cancer. Transl Lung Cancer Res. (2018) 7:69–79. doi: 10.21037/tlcr.2018.01.16
5. Horn L, Mansfield AS, Szczesna A, Havel L, Krzakowski M, Hochmair MJ, et al. First-line atezolizumab plus chemotherapy in extensive-stage small-cell lung cancer. N Engl J Med. (2018) 379:2220–9. doi: 10.1056/NEJMoa1809064
6. Goldman JW, Dvorkin M, Chen Y, Reinmuth N, Hotta K, Trukhin D, et al. Durvalumab, with or without tremelimumab, plus platinum-etoposide vers us platinum-etoposide alone in first-line treatment of extensive-stage small-cell lung cancer (CASPIAN): updated results from a randomised, controlled, open-label, phase 3 trial. Lancet Oncol. (2021) 22:51–65. doi: 10.1016/S1470-2045(20)30539-8
7. Megyesfalvi Z, Gay CM, Popper H, Pirker R, Ostoros G, Heeke S, et al. Clinical insights into small cell lung cancer: Tumor heterogeneity, di agnosis, therapy, and future directions. CA: Cancer J Clin. (2023) 73:620–52. doi: 10.3322/caac.21785
8. Liu SA-O, Reck MA-O, Mansfield AA-O, Mok T, Scherpereel AA-O, Reinmuth N, et al. Updated overall survival and PD-L1 subgroup analysis of patients with extensive-stage small-cell lung cancer treated with atezolizumab, carboplatin, and etoposide (IMpower133). J Clin Oncol. (2021) 39:619–30. doi: 10.1200/JCO.20.01055
9. Yu H, Batenchuk C, Badzio A, Boyle TA, Czapiewski P, Chan DC, et al. PD-L1 expression by two complementary diagnostic assays and mRNA in situ hybridization in small cell lung cancer. J Thorac Oncol. (2017) 12:110–20. doi: 10.1016/j.jtho.2016.09.002
10. Heinhuis KM, Ros W, Kok M, Steeghs N, Beijnen JH, Schellens JHM. Enhancing antitumor response by combining immune checkpoint inhibitors with chemotherapy in solid tumors. Ann Oncol. (2019) 30:219–35. doi: 10.1093/annonc/mdy551
11. Ni J, Zhang X, Wang H, Si X, Xu Y, Zhao J, et al. Clinical characteristics and prognostic model for extensive-stage small cell lung cancer: A retrospective study over an 8-year period. Thorac Cancer. (2022) 13:539–48. doi: 10.1111/1759-7714.14289
12. Foster NR, Mandrekar SJ, Schild SE, Nelson GD, Rowland KM, Deming RL, et al. Prognostic factors differ by tumor stage for small cell lung cancer. Cancer. (2009) 115:2721–31. doi: 10.1002/cncr.v115:12
13. Sculier JP, Chansky K, Crowley JJ, Meerbeeck JV, Goldstraw P, International Staging Committee and Participating Institutions. The impact of additional prognostic factors on survival and their relationship with the anatomical extent of disease expressed by the 6th Edition of the TNM Classification of Malignant Tumors and the proposals for the 7th Edition. J Thorac Oncol. (2008) 3:457–66. doi: 10.1097/JTO.0b013e31816de2b8
14. Longo V, Pizzutilo P, Catino A, Montrone M, Pesola F, Marerch I, et al. Prognostic factors for survival in extensive-stage small cell lung cancer: An Italian real-world retrospective analysis of 244 patients treated over the last decade. Thorac Cancer. (2022) 13:3486–95. doi: 10.1111/1759-7714.14712
15. Dawe DE, Rittberg R, Syed I, Shanahan MK, Moldaver D, Bucher O, et al. Real-world predictors of survival in patients with extensive-stage small-cell lung cancer in Manitoba, Canada: a retrospective cohort study. Front Oncol. (2023) 13:1191855. doi: 10.3389/fonc.2023.1191855
16. Ma X, Zhang Z, Chen X, Zhang J, Nie J, Da L, et al. Prognostic factor analysis of patients with small cell lung cancer: Real-world data from 988 patients. Thorac Cancer. (2021) 12:1841–50. doi: 10.1111/1759-7714.13846
17. Ito F, Sato T, Emoto K, Kaizuka N, Yagi K, Watanabe R, et al. Standard therapy-resistant small cell lung cancer showing dynamic tran sition of neuroendocrine fate during the cancer trajectory: A case report. Mol Clin Oncol. (2021) 15:261. doi: 10.3892/mco.2021.2423
18. Yuan G, Liu X, Zhang X, Song W, Lu J, Ding Z, et al. Remarkable response to PD-1 inhibitor in a patient with extensive-stag e small cell lung cancer: a case report and literature review. Front Immunol. (2023) 14:1267606. doi: 10.3389/fimmu.2023.1267606
19. de Vries NL, Mahfouz A, Koning F, de Miranda N. Unraveling the complexity of the cancer microenvironment with multidimensional genomic and cytometric technologies. Front Oncol. (2020) 10:1254. doi: 10.3389/fonc.2020.01254
Keywords: immunotherapy, chemotherapy, prognosis, nomogram, extensive-stage small cell lung cancer
Citation: Gao Y, Zhang L, Yan M, Sun Z, Zhao H and Zhao L (2025) Development of a prognostic model for patients with extensive-stage small cell lung cancer undergoing immunotherapy and chemotherapy. Front. Immunol. 16:1561333. doi: 10.3389/fimmu.2025.1561333
Received: 20 January 2025; Accepted: 25 February 2025;
Published: 07 March 2025.
Edited by:
Salvatore Grisanti, University of Brescia, ItalyReviewed by:
Jael Obiero, Institute of Primate Research, KenyaCopyright © 2025 Gao, Zhang, Yan, Sun, Zhao and Zhao. This is an open-access article distributed under the terms of the Creative Commons Attribution License (CC BY). The use, distribution or reproduction in other forums is permitted, provided the original author(s) and the copyright owner(s) are credited and that the original publication in this journal is cited, in accordance with accepted academic practice. No use, distribution or reproduction is permitted which does not comply with these terms.
*Correspondence: Lujun Zhao, emhhb2x1anVuQHRqbXVjaC5jb20=
Disclaimer: All claims expressed in this article are solely those of the authors and do not necessarily represent those of their affiliated organizations, or those of the publisher, the editors and the reviewers. Any product that may be evaluated in this article or claim that may be made by its manufacturer is not guaranteed or endorsed by the publisher.
Research integrity at Frontiers
Learn more about the work of our research integrity team to safeguard the quality of each article we publish.