- 1Department of Urology, The Second Affiliated Hospital of Anhui Medical University, Hefei, China
- 2Center for Cell Lineage and Development, Guangzhou Institutes of Biomedicine and Health, Chinese Academy of Sciences Guangzhou, Guangzhou, China
- 3Department of Urological Surgery, Daping Hospital, Army Medical Center of PLA, Army Medical University, Chongqing, China
Background: The etiology of interstitial cystitis/painful bladder syndrome (IC/BPS) remains elusive, presenting significant challenges in both diagnosis and treatment. To address these challenges, we employed a comprehensive approach aimed at identifying diagnostic biomarkers that could facilitate the assessment of immune status in individuals with IC/BPS.
Methods: Transcriptome data from IC/BPS patients were sourced from the Gene Expression Omnibus (GEO) database. We identified differentially expressed genes (DEGs) crucial for gene set enrichment analysis. Key genes within the module were revealed using weighted gene co-expression network analysis (WGCNA). Hub genes in IC/BPS patients were identified through the application of three distinct machine-learning algorithms. Additionally, the inflammatory status and immune landscape of IC/BPS patients were evaluated using the ssGSEA algorithm. The expression and biological functions of key genes in IC/BPS were further validated through in vitro experiments.
Results: A total of 87 DEGs were identified, comprising 43 up-regulated and 44 down-regulated genes. The integration of predictions from the three machine-learning algorithms highlighted three pivotal genes: PLAC8 (AUC: 0.887), S100A8 (AUC: 0.818), and PPBP (AUC: 0.871). Analysis of IC/BPS tissue samples confirmed elevated PLAC8 expression and the presence of immune cell markers in the validation cohorts. Moreover, PLAC8 overexpression was found to promote the proliferation of urothelial cells without affecting their migratory ability by inhibiting the Akt/mTOR/PI3K signaling pathway.
Conclusions: Our study identifies potential diagnostic candidate genes and reveals the complex immune landscape associated with IC/BPS. Among them, PLAC8 is a promising diagnostic biomarker that modulates the immune response in patients with IC/BPS, which provides new insights into the future diagnosis of IC/BPS.
1 Introduction
Interstitial cystitis/painful bladder syndrome (IC/BPS) encompasses a spectrum of disorders primarily distinguished by bladder pain and discomfort, accompanied by lower urinary tract symptoms (1). Currently, IC/BPS is classified into two primary types: those with Hunner’s lesions (HIC) and those without (NHIC) (2). Researchers have identified distinct clinical symptoms and diverse treatment responses between these subtypes, indicating potential variations in pathologic mechanisms. While IC/BPS is not fatal, it imposes a considerable burden on affected individuals, particularly women. The etiology of IC/BPS remains elusive, with mounting evidence suggesting a multifaceted pathogenesis involving genetic predisposition, hormonal factors, history of urinary tract infections, autoimmune conditions, and other comorbidities (3–5). The absence of sensitive and specific biomarkers complicates the diagnosis of IC/BPS, underscoring the imperative for reliable markers to enhance accuracy and differentiate IC/BPS from similar diseases.
Clinical pathology strongly supports the classification of IC/BPS as a chronic inflammatory disease that progressively affects the entire bladder wall. The initial stage is primarily characterized by pain symptoms, while advanced stages may involve a significant reduction in bladder capacity (2). Increasing evidence highlights the critical roles of chronic inflammation and immune dysfunction in the progression of IC/BPS (6). Recent studies, utilizing single-cell sequencing analysis, have emphasized the involvement of the inflammatory immune microenvironment and immune cell crosstalk in the pathogenic mechanisms of IC/BPS (7, 8). Neutrophils are key players in early immune responses, typically involved in bacterial infections and acute inflammation. In the bladder tissue of IC/BPS patients, neutrophil infiltration is often elevated, exacerbating the local inflammatory response by releasing various cytokines and chemokines (9). Overactivation of Th1 cells may further drive inflammatory responses, particularly in areas with Hunner’s lesions (10). Additionally, Moldwin et al. observed abnormal immune cell infiltration in IC/BPS tissue samples, alongside elevated levels of pro-inflammatory cytokines in urine samples from these patients (11). For instance, the expression of the chemokine CXCL-10 is significantly elevated in the bladder tissue of IC/BPS patients compared to that of healthy individuals. Despite previous bioinformatics analyses identifying potential molecular markers in IC/BPS patients, a thorough exploration and validation of diagnostic markers and immunological effects are warranted (12–14). Further comprehensive analysis is necessary to unveil differences in the immune landscape between IC/BPS patients and healthy populations.
In this study, we integrated bioinformatics analysis and machine-learning algorithms to identify diagnostic biomarkers contributing to assessing the immune status in IC/BPS patients. Additionally, we validated the expression of key genes and unveiled their relevance to the immune landscape in IC/BPS tissues.
2 Methods
2.1 Data collection
Transcriptome data for IC/BPS patients were obtained from the Gene Expression Omnibus (GEO) database, encompassing GSE11783 (15), GSE28242 (16), and GSE57560 (17). Key information about these datasets is summarized in Table 1. Due to differences in sequencing platforms, methodologies, and experimental designs across the datasets, we applied the “Combat” function to adjust for batch effects arising from these variations. Before applying “Combat,” we first preprocessed the data by removing outliers. The method was then used to correct for batch effects, ensuring that subsequent analyses more accurately reflected biological variations rather than experimental design differences. Following established protocols, the datasets were integrated using the “sva” package in R software, effectively eliminating batch effects for subsequent analysis (18, 19).
2.2 Identification of differentially expressed genes
DEGs between IC/BPS samples and normal samples were identified using the “limma” package in the combined dataset (20). A threshold of P-value < 0.05 and |log2FC| > 1 was applied to screen for DEGs. Adjusted P-value < 0.05 and FDR (Q-value) < 0.25 were set as the cut-off criteria. The expression patterns of these DEGs in both patient groups were then visualized using the “pheatmap” package.
2.3 Functional enrichment analysis
To further investigate the biological role of these DEGs, we performed functional enrichment analyses of “clusterProfiler” packages (P-value < 0.05), including the Gene Ontology (GO) and Kyoto Encyclopedia of Genes and genes Genomes (KEGG) (21). Additionally, Gene Set Enrichment Analysis (GSEA) was undertaken in both patient groups, utilizing the “clusterProfiler” and “enrichplot” packages. Detailed descriptions of these methods can be found in previous studies (22, 23).
2.4 Weighted gene co-expression network analysis
To further analyze potential pathogenic genes in IC/BPS patients, we used the “WGCNA” and “limma” R packages to construct gene co-expression networks and analyze correlations between gene modules and specific phenotypes (20, 24). The key gene of the relevant module is defined as the first principal component gene of each module and is adopted as a representative of all genes in each module (Module membership > 0.80 and P < 0.05).
2.5 Construction and verification of the diagnostic prediction model
To pinpoint the key pathogenic genes, we employed three machine-learning algorithms—random forest (RF) (25), loss absolute shrinkage and selection operator (LASSO) (26) and support vector machine -recursive feature elimination (SVM-RFE) (27). The analyses for SVM-RFE, RF, and LASSO regression were conducted using the R packages “glmnet,” “kernlab,” and “randomForest” (28–30). Genes situated in the overlapping regions of the three algorithms were deemed crucial in IC/BPS diagnosis. Subsequently, a diagnostic nomogram model was constructed based on these identified genes. To further evaluate the predictive model’s capability in distinguishing between normal and IC/BPS tissue, we conducted decision curve analysis (DCA), calibration curve, and clinical impact curve (CIC) using the R packages “pROC” and “rmda” (31, 32). Additionally, we assessed the studies based on receiver operating characteristic (ROC) curves, calculating the area under the curve (AUC) to measure the predictive power of the key genes. Detailed methodologies are referenced in several prior studies (33).
2.6 Immune cells infiltration analysis
Immune cells play a crucial role in the crosstalk that influences disease progression and treatment response. To assess each immune cell across all samples, we utilized the ssGSEA algorithm, incorporating the “GSVA,” “GSEABase,” and “limma” packages. This analysis was based on 28 immune cell gene sets, allowing us to scrutinize and compare the differences between normal and IC/BPS tissues (34).
2.7 Collection of clinical samples
Between January 2022 and August 2023, we collected 8 bladder tissue samples from individuals diagnosed with interstitial cystitis/bladder pain syndrome (IC/BPS) and 10 bladder tissue samples from unaffected controls (UC), following previously established methods (11, 35). Cystoscopy was performed on all IC/BPS patients, and biopsies were taken from tissue suspected to contain Hunner’s lesions. UC samples were obtained from patients with benign bladder tumors, with biopsies confirming the absence of inflammatory changes. Laboratory test results, including hemoglobin (Hb), white blood cell (WBC) count, neutrophil-to-lymphocyte ratio (NLR), lymphocyte-to-monocyte ratio (LMR), and absolute lymphocyte count (ALC), were collected within 12 hours prior to biopsy. Additionally, clinical data on age, sex, history of urinary surgery, diabetes, hypertension, smoking, and alcohol consumption were recorded. The classification of samples into IC/BPS or UC groups was independently verified by two pathologists.
2.8 Immunohistochemistry assay
The specific immunohistochemical staining methods employed in this study were referenced from our previous studies (22, 36). All tissues were transformed into pathological sections and then processed using an IHC kit as per the manufacturer’s instructions (cat. SP-9001; ZSGB-BIO, China). The primary antibodies utilized in this study included PLAC8 (cat. 12284-1-AP, Proteintech, China), CXCL10 (cat. DF6417, Affinity, USA), c-Kit (CD117) (cat. AF6153, Affinity, USA), SDC1 (CD138) (cat. DF6367, Affinity, USA), CD163 (cat. DF8235, Affinity, USA), CD20 (cat. DF13319, Affinity, USA), CD14 (cat. DF13278, Affinity, USA), FucT-IV (CD15) (cat. DF8547, Affinity, USA), and CD3D (cat. DF6370, Affinity, USA). Two senior pathologists independently evaluated the IHC staining results. The percentage of positive cells was categorized as follows: 1 = 0-25%, 2 = 26%-50%, 3 = 51%-75%, and 4 = 76%-100%. Staining intensity was defined as 0 = no staining; 1 = weak staining; 2 = medium staining; 3 = strong staining. The final IHC score was derived by multiplying the staining percentage and staining intensity. Immune cell staining was assessed based on the number of positive cells within the field of vision.
2.9 Cell culture and transfection
Normal human urothelial cell line (SV-HUC-1) was purchased from Procell Life Science & Technology Co., Ltd. (China) using Ham’s F-12 (F-12K) medium modified by Kaighn (Gibco, USA) for cultivation. SV-HUC-1 cell lines were treated with lipopolysaccharide (LPS, 10ug/L, MedChemExpress) and adenosine triphosphate (ATP, 2.5 mM, MedChemExpress) to induce inflammatory damage. We overexpressed PLAC8 using PB-EF1a-FLAG-PLAC8-IRES-Hygromycin vector, and selected PB-EF1a-3FLAG-GFP-IRES-Hygromycin vector as a negative control group. These vectors were purchased from Sigma-Aldrich according to the manufacturer’s instructions.
2.10 Western blot analysis
Total proteins were extracted from cells using RIPA lysis buffers of protease and phosphatase inhibitors (Beyotime Biotechnology, China). Pierce BCA protein detection kit was used to determine the protein concentration (Beyotime Biotechnology, China). Protein samples were isolated by sodium dodecyl sulfate polyacrylamide gel electrophoresis and transferred to PVDF membranes (Beyotime Biotechnology, China). Incubate overnight with primary antibodies, including PLAC8 (1:1000; Proteintech, China), Flag (1:1000; Sigma-Aldrich, USA), α-tubulin (1:10000, Sigma-Aldrich, USA), AKT (1:1000; Proteintech, China), p-AKT (1:1000; Proteintech, China), mTOR (1:1000; Proteintech, China), p-mTOR (1:1000; Proteintech, China), and PI3K (1:1000; Proteintech, China). The PVDF membrane was then incubated with the horseradish peroxide-coupled secondary antibody (Beyotime Biotechnology, China). Protein bands were detected using an enhanced chemiluminescence kit (Thermo Fisher, USA).
2.11 Cell proliferation assays
Cell proliferation ability was detected using cell counting kit-8 (Beyotime Biotechnology, China). 5000 cells were mixed and inoculated into a 96-well plate incubator for 24h. The absorbance value of 450 nm was measured according to the manufacturer’s instructions.
2.12 Wound healing assays
SV-HUC-1 cells were inoculated into the 6-well plate, and subsequent experiments were carried out when the cell confluence reached 100%. Wounds were formed with a 200 μL pipette suction and cell migration was assessed with photographs taken every 12 hours.
2.13 Statistical analysis
The statistical analysis was conducted using R software (version 4.2.1), and data processing followed established literature protocols. The unpaired Student’s t test was used for statistical comparison between the two groups. The Kruskal-Wallis test is used for statistical comparison of non-normally distributed data. A significance level of P-value < 0.05 was considered statistically significant.
3 Results
3.1 Identification of DEGs in IC/BPS patients and functional enrichment analysis
We acquired three IC/BPS datasets, GSE11783, GSE28242, and GSE57560, from the GEO database, comprising 14 normal samples and 31 IC/BPS samples. Our data analysis process is shown in Figure 1. A total of 87 DEGs were identified, comprising 43 up-regulated genes and 44 down-regulated genes (Figure 2A; Supplementary Table 1). To elucidate the biological functions of these DEGs, we conducted functional enrichment analysis. The results of GO analysis indicated that these DEGs were significantly enriched in processes such as extracellular matrix organization, connective tissue development, collagen fibril organization, collagen-containing extracellular matrix, myofibril, contractile fiber, extracellular matrix structural constituent, peptidase regulator activity, and endopeptidase regulator activity (Figure 2B; Supplementary Table 2). These biological functions involve the changes of extracellular matrix tissue, connective tissue development, collagen fiber tissue and other factors, which are one of the pathological features of IC/BPS. Furthermore, KEGG analysis unveiled the predominant involvement of DEGs in pathways related to the IL-17 signaling pathway, AGE-RAGE signaling pathway in diabetic complications, and TGF-beta signaling pathway (Figure 2C and Supplementary Table 3). Abnormal activation of these signaling pathways is a key step in the pathogenesis of interstitial cystitis, exacerbating bladder inflammation and injury by promoting local inflammatory responses and infiltration of immune cells. DO analysis revealed associations with various medical conditions, including cell type benign neoplasm, renal cell carcinoma, myopathy, esophageal cancer, cerebrovascular disease, esophageal carcinoma, urinary bladder cancer, aortic aneurysm, aortic disease, endometriosis, cholestasis, and prostate carcinoma (Figure 2D; Supplementary Table 4). GSEA was performed on both normal and IC/BPS samples to explore signaling pathways (Supplementary Table 5). In the control group, the top three terms included arachidonic acid metabolism, linoleic acid metabolism, and ribosome (Figure 2E). In the treatment group, the top five terms comprised cell cycle, chemokine signaling pathway, complement and coagulation cascades, cytokine-cytokine receptor interaction, and oocyte meiosis (Figure 2F). The interaction and abnormal activation of these signaling pathways together drive the development of chronic inflammation, fibrosis, and bladder dysfunction of IC/BPS, providing potential targets for treatment.
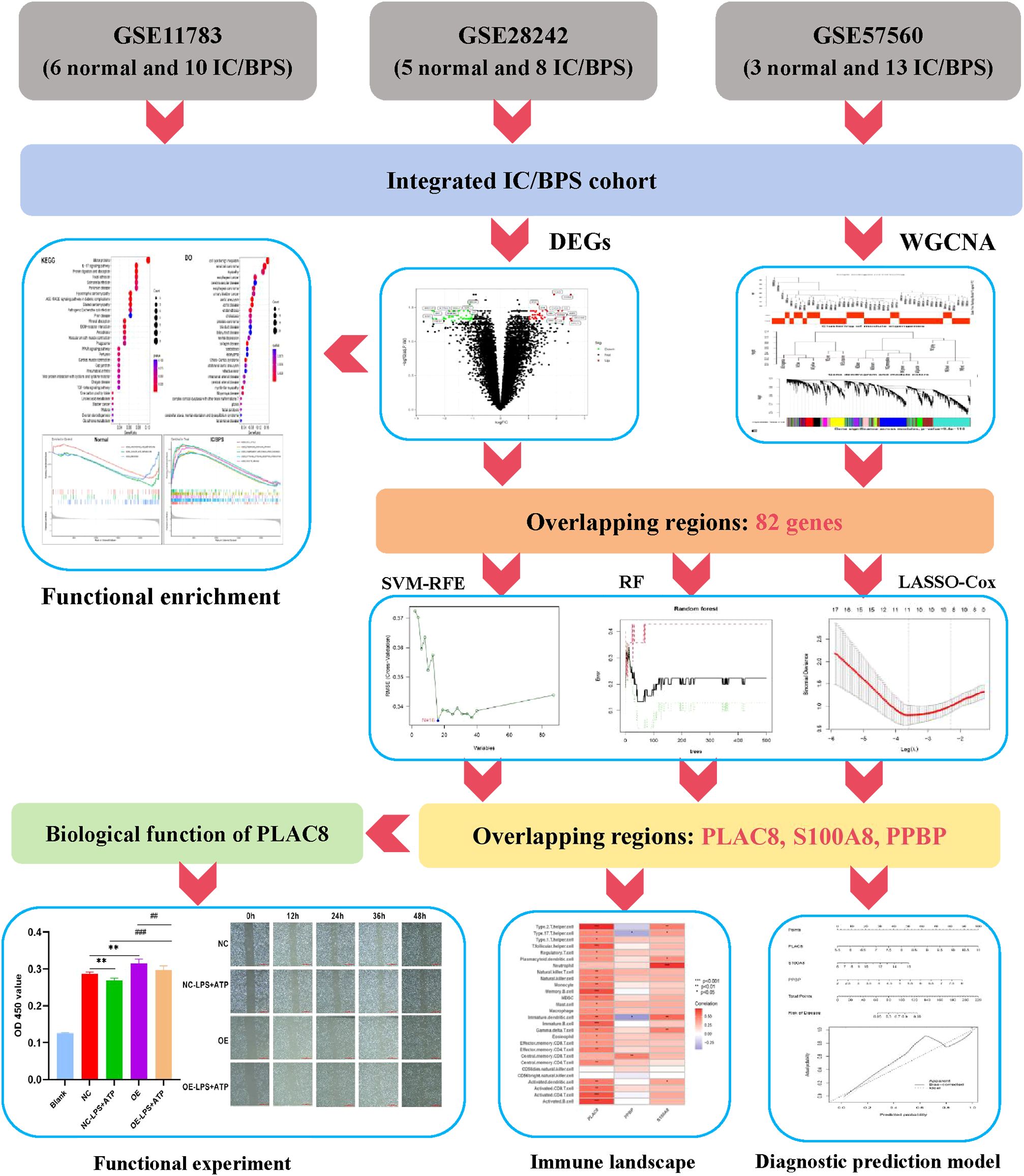
Figure 1. The flowchart depicting the investigation procedure. IC/BPS, interstitial cystitis/painful bladder syndrome; DEGs, Differentially expressed genes; WGCNA, weighted gene co-expression network analysis; RF, random forest; LASSO, loss absolute shrinkage and selection operator; SVM-RFE, support vector machine -recursive feature elimination.
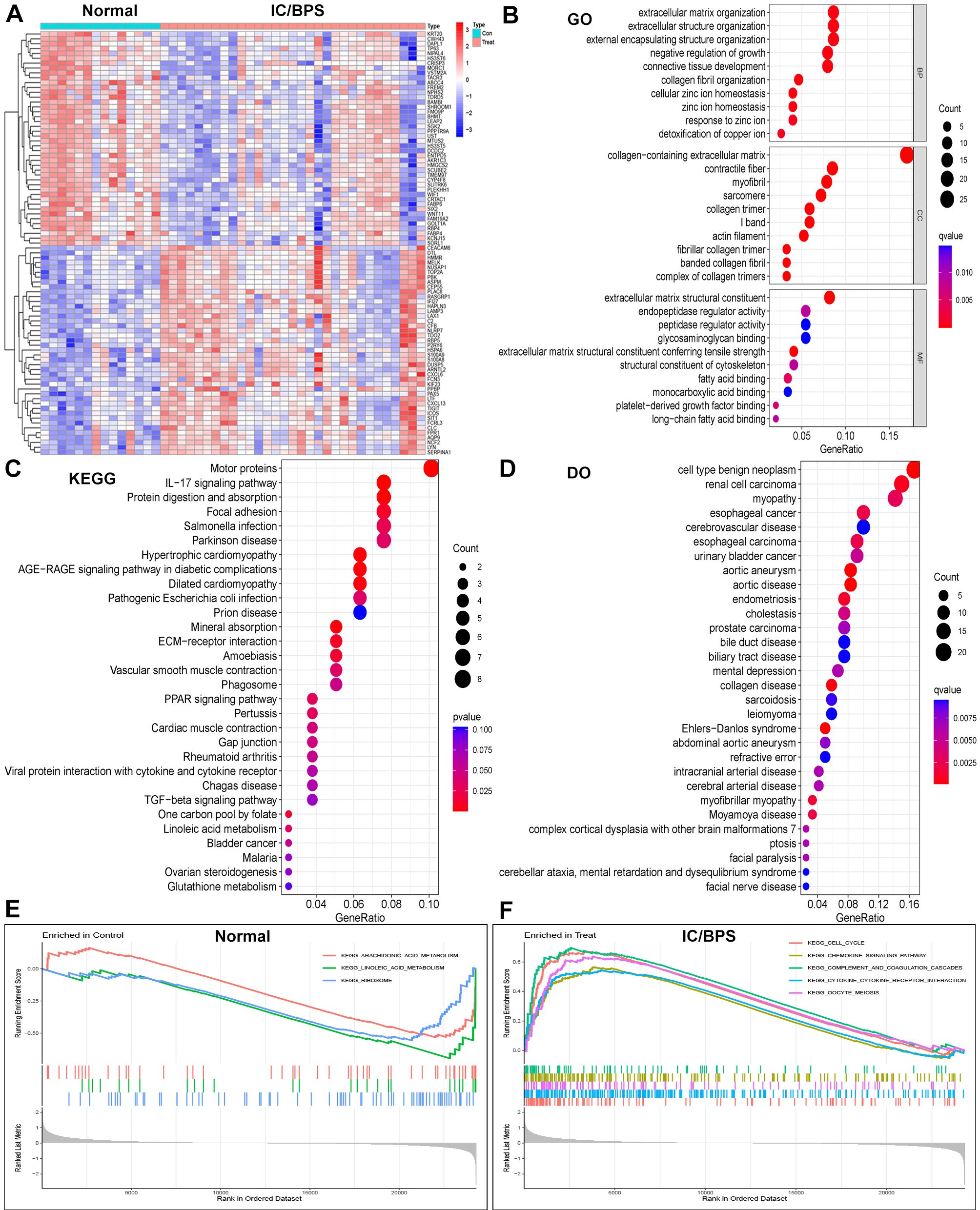
Figure 2. Identification of DEGs in IC/BPS patients and functional enrichment analysis. (A) Heatmap showing up-regulated or down-regulated DEGs in IC/BPS samples compared to normal samples (bule: down-regulated; red: up-regulated). (B) GO analysis of these DEGs in IC/BPS patients. (C) KEGG analysis of these DEGs in IC/BPS patients. (D) DO analysis of these DEGs in IC/BPS patients. (E) GSEA was performed in normal patients. (F) GSEA was performed in IC/BPS patients. IC/BPS, interstitial cystitis/painful bladder syndrome; DEGs, Differentially expressed genes; GO, Gene Ontology; KEGG, Kyoto Encyclopedia of Genes and genes Genomes; DO, Disease Ontology; GSEA, Gene Set Enrichment Analysis.
3.2 Identification of key genes by WGCNA analysis in IC/BPS patients
Subsequently, we delved into the identification of key pathogenic genes in IC/BPS through the WGCNA method. The IC/BPS dataset was stratified into two distinct groups—comprising the control group (normal samples) and the treatment group (IC/BPS samples)—with no discernible outliers (Figure 3A). Employing a scale-free R2 value of 0.95, we ascertained the soft thresholding capability of WGCNA, ultimately selecting 6 as the soft thresholding power β (Figure 3B). Subsequent to employing average link hierarchy clustering and evaluating soft threshold capability, we identified 9 modules, with a particular focus on the turquoise, blue, greenyellow, and tan modules due to their significant associations with IC/BPS (Figures 3C–E). The correlation between these selected modules and IC/BPS is visually represented in the scatter plot (Figures 3F–I). A comprehensive total of 2612 genes exhibited significant associations with IC/BPS in terms of gene significance and functional modules (Supplementary Table 6).
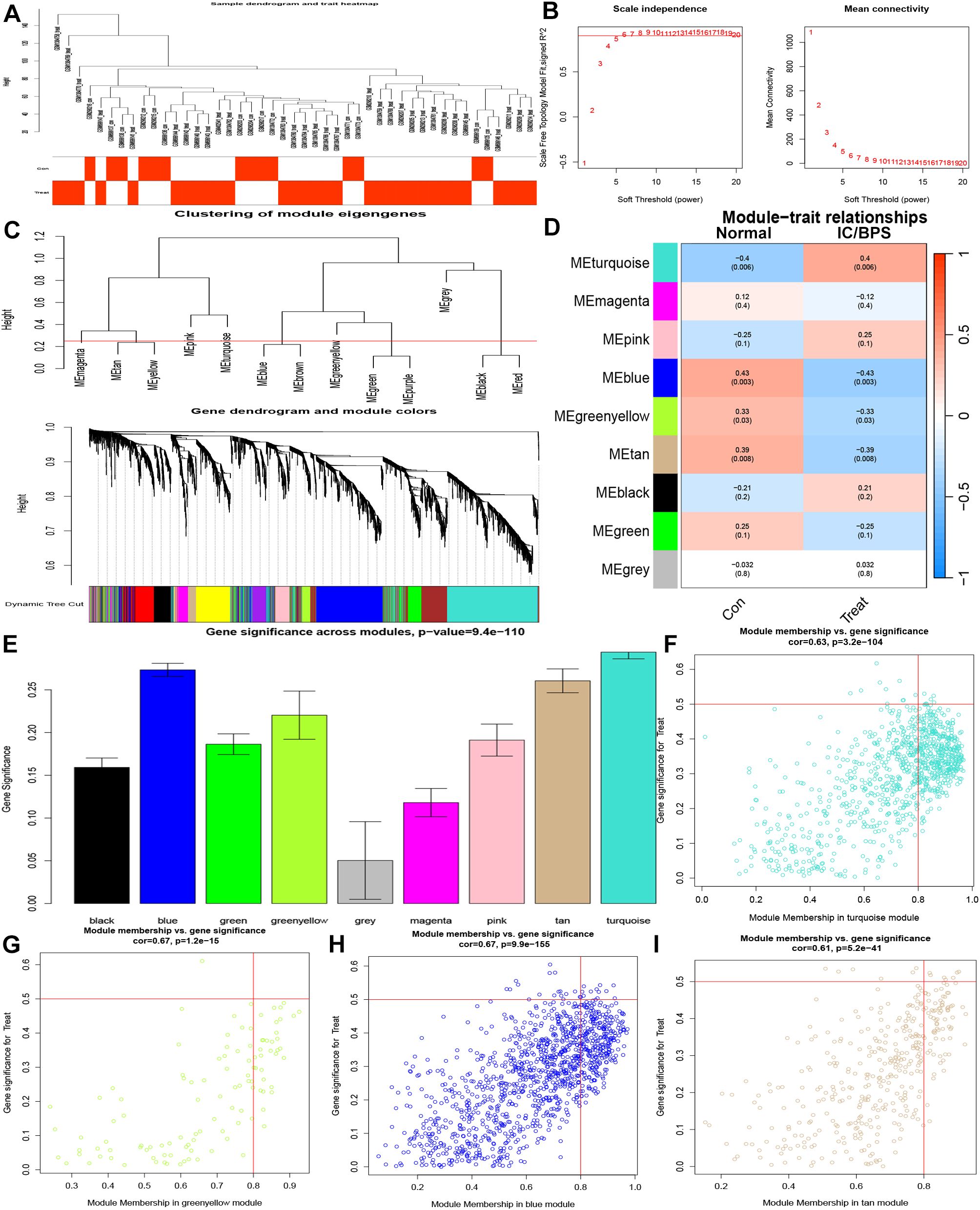
Figure 3. Identification of key genes by WGCNA analysis in IC/BPS patients. (A) Clustering dendrogram of 45 samples. (B) Analysis of network topology for various soft thresholds (β). (C) A dendrogram of the differentially expressed genes clustered based on different metrics. (D) Module-trait relationships. (E) Distribution of average gene significance and errors in the modules. (F) Associations between turquoise module membership and gene importance is depicted in a scatter plot. (G) Associations between greenyellow module membership and gene importance is depicted in a scatter plot. (H) Associations between blue module membership and gene importance is depicted in a scatter plot. (I) Associations between tan module membership and gene importance is depicted in a scatter plot. IC/BPS, interstitial cystitis/painful bladder syndrome; WGCNA, weighted gene co-expression network analysis.
3.3 Screening and verification of diagnostic markers by bioinformatics and machine-learning algorithm in IC/BPS patients
In order to enhance the precision of data processing, we employed a Venn diagram to identify the overlapping genes between DEGs and key module genes in IC/BPS patients. This analysis revealed a total of 82 genes within the overlapping regions (Figure 4A; Supplementary Table 7). Subsequently, for the identification of potential diagnostic markers in IC/BPS patients, we applied three machine-learning algorithms to pinpoint crucial genes. Initially, the SVM-RFE algorithm identified 16 key genes in IC/BPS patients (Figure 4B). Following this, the RF algorithm, coupled with the distinctive characteristics of IC/BPS patients, selected 12 key genes (Figures 4C, D). Lastly, utilizing LASSO regression analysis, we identified 7 key genes from statistically significant univariate variables in IC/BPS patients (Figures 4E, F).
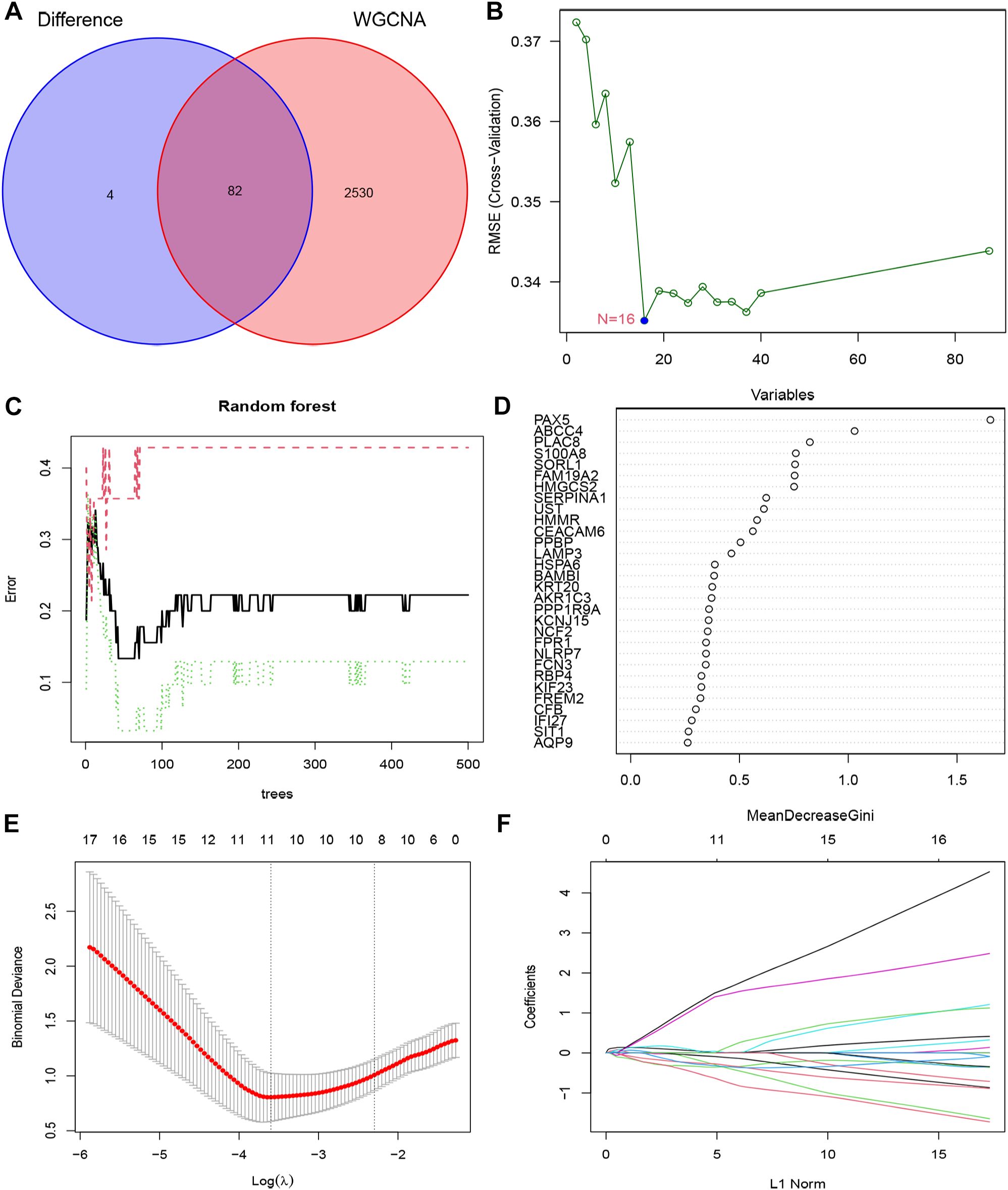
Figure 4. Screening key genes is based on biological analysis and machine-learning algorithms in IC/BPS patients. (A) The overlapping regions of key module genes and DEGs. (B) Screening of key genes was based on SVM-RFE algorithm. (C, D) Screening of key genes was based on RF algorithm. (E, F) Screening of key genes was based on LASSO regression analysis. IC/BPS, interstitial cystitis/painful bladder syndrome; DEGs, Differentially expressed genes; RF, random forest; LASSO, loss absolute shrinkage and selection operator; SVM-RFE, support vector machine -recursive feature elimination.
The integration of the predictions from these three machine-learning algorithms highlighted three key genes: PLAC8, S100A8, and PPBP (Figure 5A). To assess the clinical significance of these key genes, we constructed a nomogram model for diagnosing IC/BPS based on these predictive genes (Figure 5B). Decision curve analysis (DCA), calibration curve, and clinical impact curve (CIC) results demonstrated a favorable clinical benefit of our nomogram model (Figures 5C–E). Additionally, ROC curve analysis indicated that PLAC8 (AUC: 0.887), S100A8 (AUC: 0.818), and PPBP (AUC: 0.871) may serve as valuable biomarkers for IC/BPS patients (Figures 5F–H). Furthermore, the mRNA expression levels of PLAC8, S100A8, and PPBP were significantly upregulated in IC/BPS patients compared to normal patients (Figures 5I–K).
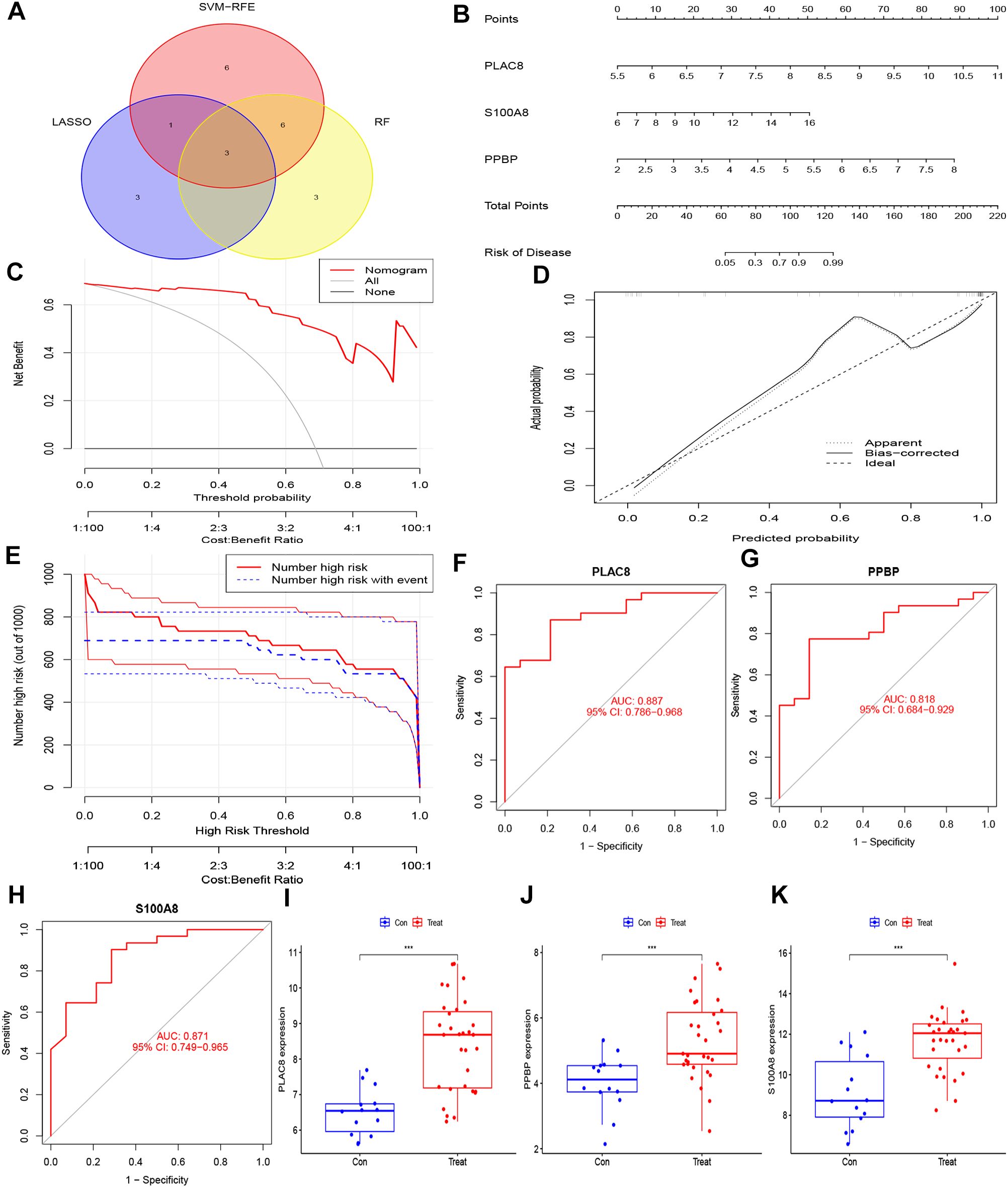
Figure 5. Hub genes for pediatric sepsis diagnosis in IC/BPS patients. (A) Venn diagram showed the intersection of diagnostic markers obtained by the three algorithms. (B) Nomogram is used to predict the occurrence of IC/BPS. (C) DCA curves of the diagnostic efficacy verification; (D) Calibration curve of the diagnostic efficacy verification; (E) Clinical impact curve of the diagnostic efficacy verification. (F) The ROC curve of PLAC8 in IC/BPS dataset; (G) The ROC curve of PPBP in IC/BPS dataset. (H) The ROC curve of S100A8 in IC/BPS dataset. (I) Boxplot showed the expression of PLAC8 between IC/BPS group and control group. (J) Boxplot showed the expression of PPBP between IC/BPS group and control group. (K) Boxplot showed the expression of S100A8 between IC/BPS group and control group. IC/BPS, interstitial cystitis/painful bladder syndrome; DCA, decision curve analysis; CIC, clinical impact curve; ROC, receiver operating characteristic. ***, P < 0.001.
3.4 Immune cell landscape and its associations with diagnostic genes in IC/BPS patients
We employed the ssGSEA algorithm to assess the landscape of immune cell infiltration in IC/BPS compared to normal samples. Our findings revealed a notable increase in the infiltration levels of 28 types of immune cells in the majority of IC/BPS samples as opposed to normal samples (Figure 6A). Specifically, the infiltration levels of 23 types of immune cells in IC/BPS samples were significantly higher than those in normal samples, encompassing Activated B cells, Activated CD4 T cells, Activated CD8 T cells, Activated dendritic cells, Eosinophils, Gamma delta T cells, Immature B cells, Immature dendritic cells, MDSCs, Macrophages, Mast cells, Monocytes, Natural killer T cells, Natural killer cells, Neutrophils, Plasmacytoid dendritic cells, Regulatory T cells, T follicular helper cells, Type 1 T helper cells, Type 2 T helper cells, Effector memory CD4 T cells, Memory B cells, and Central memory CD4 T cells (Figure 6B).
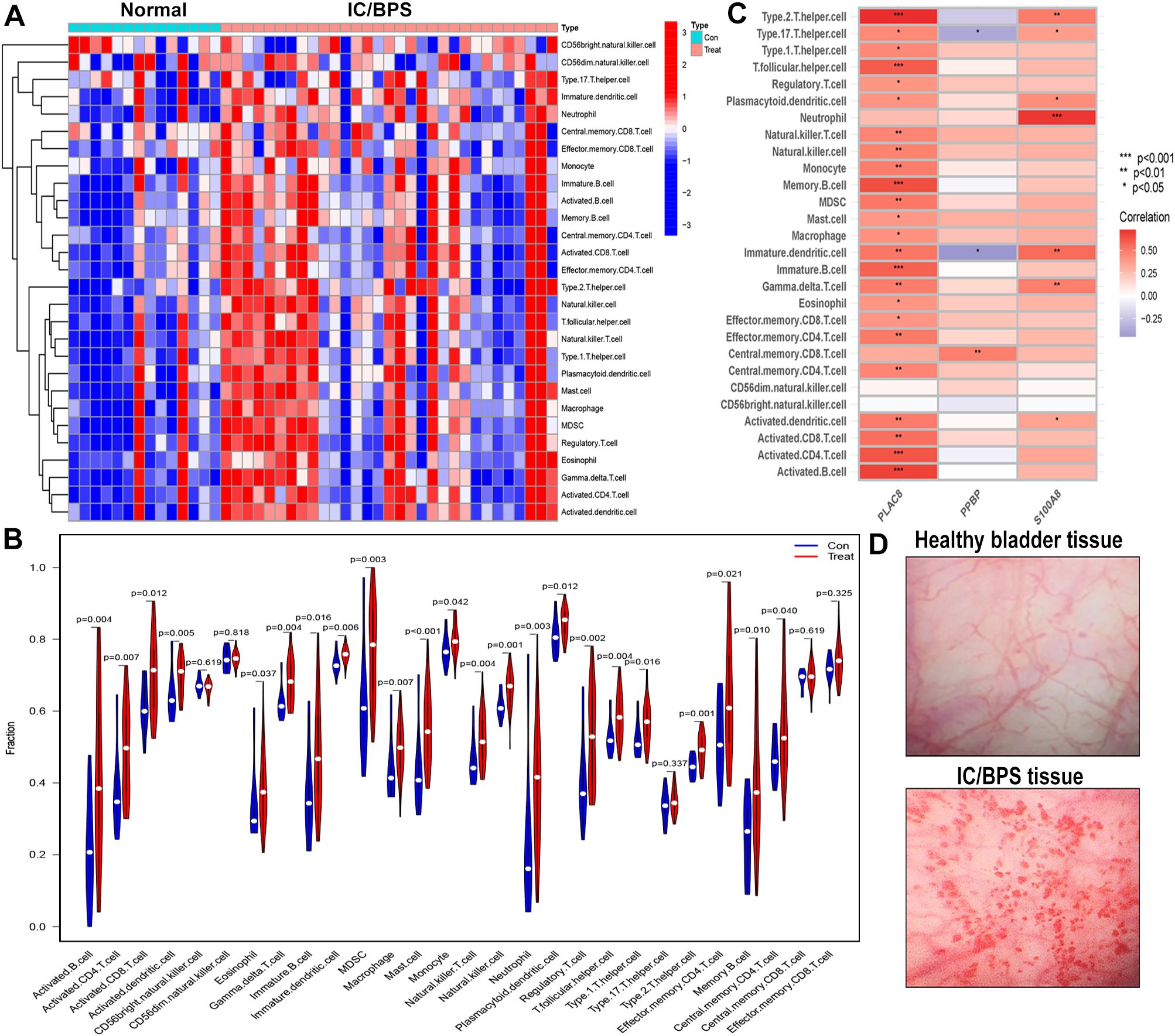
Figure 6. Evaluation and visualization of immune cell infiltration in IC/BPS cohort. (A) Heat maps confirm that most IC/BPS samples have higher immune cells compared to normal samples. (B) The immune landscape reveal the infiltration levels of 23 types of immune cells in IC/BPS samples were significantly higher than those in normal samples. (C) Correlation between three hub genes and immune cell in IC/BPS dataset. (D) Representative cystoscopic results of healthy bladder tissue and IC/BPS tissue with Hunner’s lesions in one case. Diagnosis is made via cystoscopy and confirmed by biopsy. IC/BPS, interstitial cystitis/painful bladder syndrome. *P < 0.05; **P < 0.01; ***P < 0.001.
Furthermore, our analysis indicated significant positive associations between three key genes and multiple immune cells in IC/BPS patients, particularly PLAC8 (Figure 6C). To validate the role of these key genes and immune cells in IC/BPS patients, we gathered 18 tissue samples (10 UC samples and 8 IC/BPS samples) for subsequent analysis, confirmed through cystoscopy and tissue biopsy (Figure 6D). There was no significant difference in clinical features between the two groups (Table 2). In IC/BPS tissues, we observed significantly upregulated expression of PLAC8, CXCL10, CD14 (Monocytes), CD15 (Neutrophils), and CD117 (Mast cells) compared to UC tissues (Figures 7A–D). The relative proportions of each immune cell marker’s protein expression in the UC and IC/BPS cohorts exhibited distinctions, with a notable increase in mast cells and monocytes in IC/BPS tissues (Figure 7E).
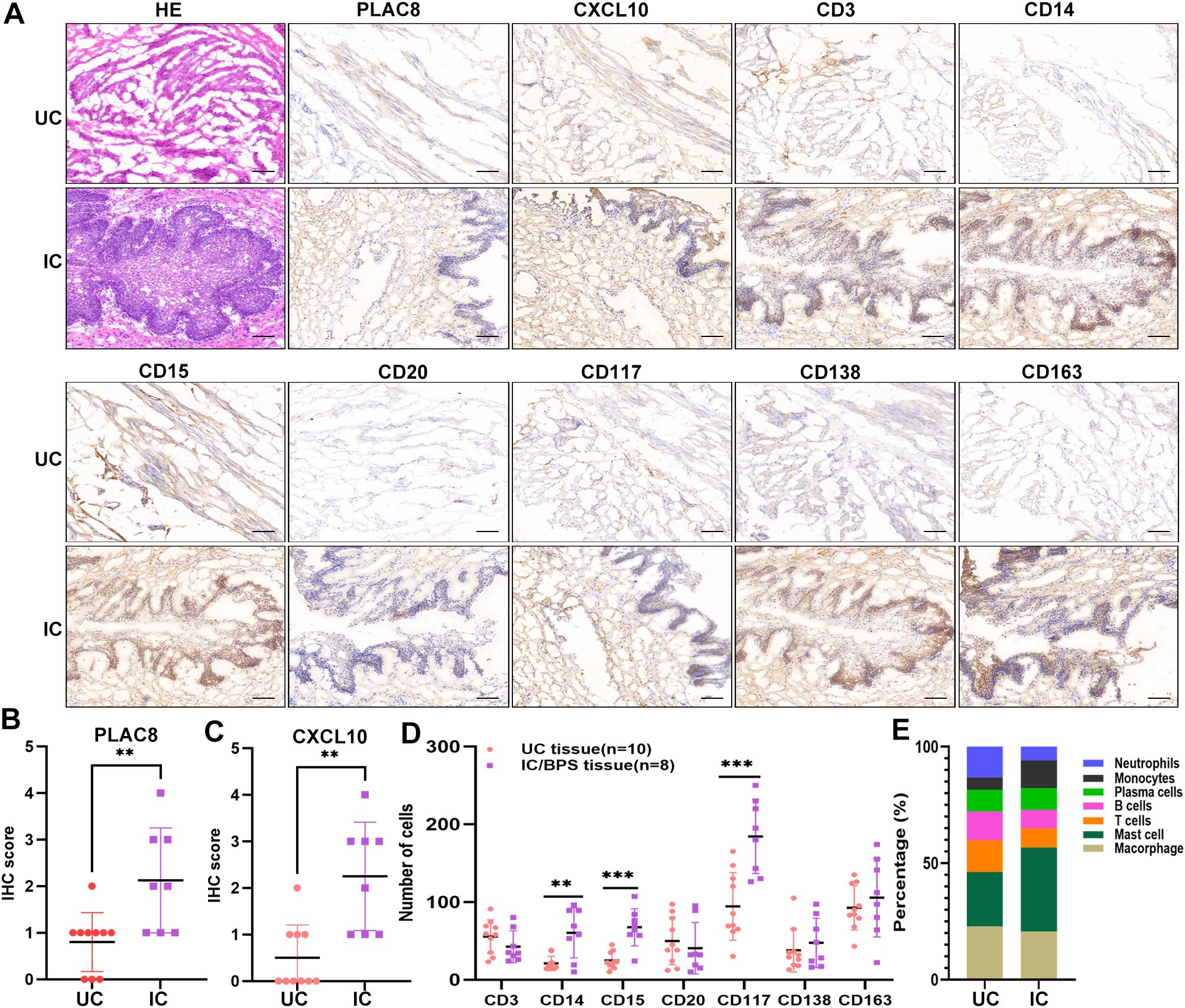
Figure 7. Verification of PLAC8 expression and immune cell infiltration in verified cohort. (A) Representative results of protein expression levels of PLAC8, CXCL10 and 7 immune cell markers in IC/BPS tissue and UC tissue (Microscale: 100 μm). (B) The IHC score of PLAC8 in IC/BPS tissues and UC tissues. (C) The IHC score of CXCL10 in IC/BPS tissues and UC tissues. (D) Number of positive cells for seven immune cell marker in IC/BPS tissues and UC tissues. (E) The relative proportions of seven immune cell marker’s protein expression in IC/BPS tissues and UC tissues. IC/BPS, interstitial cystitis/painful bladder syndrome; UC, unaffected control; IHC, Immunohistochemistry. **P < 0.01; ***P < 0.001.
3.5 Effects of PLAC8 expression on the biological function of urothelial cells in vitro and in vivo
PLAC8 protein expression levels were significantly upregulated in inflammatory cell models induced by LPS and ATP compared to controls (Figure 8A). Following transfection, PLAC8 was overexpressed in SV-HUC-1 cells, as confirmed by western blot analysis, compared to control cells (Figure 8B). We observed that the expression of key proteins in the AKT/mTOR/PI3K signaling pathway—such as AKT, mTOR, and PI3K—was inhibited in PLAC8-overexpressing cells compared to the control group, including in the inflammatory cell models (Figure 8C). PLAC8 overexpression significantly enhanced the proliferative capacity of SV-HUC-1 cells compared to untreated cells (Figure 8D). However, a wound healing assay revealed that PLAC8 had no significant effect on the migration ability of SV-HUC-1 cells, including in the inflammatory cell models (Figure 8E).
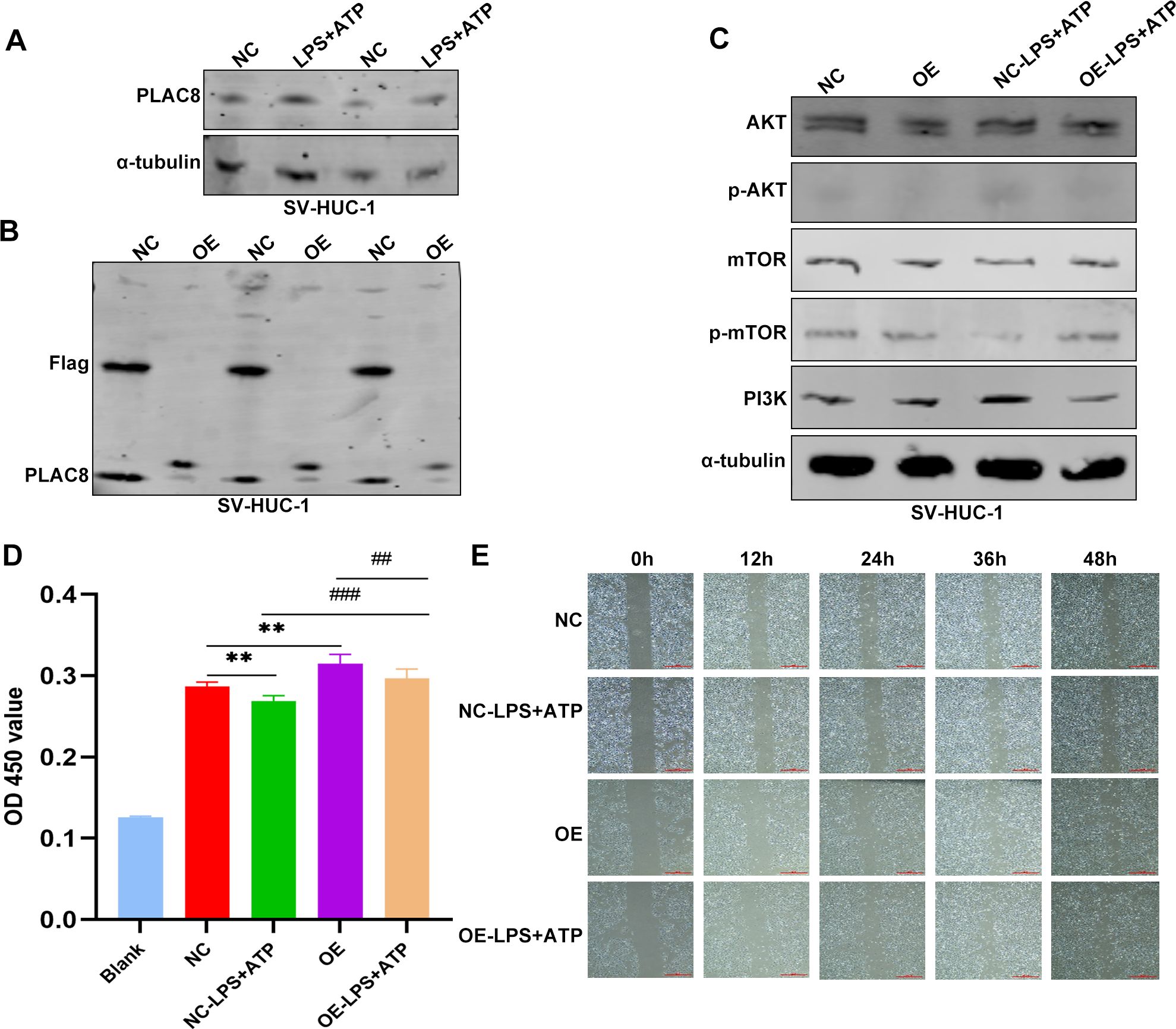
Figure 8. The expression and biological functions of PLAC8 in IC/BPS were further validated through in vitro experiments. (A) Representative results of the expression level of PLAC8 protein in inflammatory cell model was detected by western blot. (B) Representative results of PLAC8 overexpression in SV-HUC-1 cells by western blot. (C) Representative results of AKT/mTOR/PI3K pathway marker proteins were detected by western blot in SV-HUC-1 cells. (D) Proliferation of SV-HUC-1 cells was detected by cell counting kit-8. (E) Representative results of migration ability were measured by wound healing assay in SV-HUC-1 cells (Microscale: 500 μm). OD, optical density; NC, negative control; OE, over expression. **P < 0.01; ##P < 0.01; ###P < 0.001.
4 Discussion
IC/BPS is a chronic condition characterized by pelvic pain, discomfort, and urinary symptoms. Research efforts focused on IC/BPS aim to comprehend its etiology, enhance diagnostic methods, and devise effective treatments (6). Currently, it is noteworthy to recognize that inflammation and immune cell response constitute key features in IC/BPS patients. In this study, the integration of bioinformatics and machine-learning algorithms led to the identification of three upregulated DEGs. The immune landscape analysis unveiled a robust positive association between PLAC8 and various immune cells. Our findings suggest that PLAC8 may serve as a diagnostic biomarker and a potential target for modulating immune responses in individuals with IC/BPS.
In IC/BPS, inflammation is commonly characterized as non-infectious, indicating that it is not attributed to bacteria or other pathogens typically associated with urinary tract infections (37). The diagnostic process is often intricate due to the variability in IC/BPS symptoms and their overlap with other urinary disorders. Pathological diagnosis reports in IC/BPS are typically obtained only after transurethral resection of Hunner’s lesion, which may introduce bias and cause delays in diagnosis. A comprehensive approach to IC/BPS diagnosis, involving clinical evaluation, laboratory tests, and imaging studies, is crucial (38). Ongoing efforts to identify diagnostic biomarkers and develop urine tests are focused on establishing non-invasive methods for diagnosing IC/BPS. For example, chemokines such as CXCL-10 may serve as valuable biomarkers for both diagnosing IC/BPS and assessing its clinical characteristics. In this study, we reported a novel diagnostic biomarker for identifying patients with IC/BPS. Differential analysis identified 43 up-regulated DEGs and 44 down-regulated DEGs in the IC/BPS cohort. Functional enrichment analysis indicated that these genes were associated with extracellular matrix regulation, the IL-17 signaling pathway, and the TGF-beta signaling pathway. To assess the predictive value of these genes in IC/BPS patients, we constructed a nomogram model, demonstrating their efficacy in accurately predicting the occurrence of IC/BPS. Our findings align with several prior studies that identified novel diagnostic biomarkers through integrated approaches (12, 13, 18). Unfortunately, due to a lack of available data, our model could not be validated in independent IC/BPS datasets.
Chronic inflammation and abnormal immune responses are implicated in the occurrence and progression of IC/BPS (11, 39). Employing bioinformatics analysis and machine-learning algorithms, our study identified three hub genes—PLAC8, S100A8, and PPBP—that may be involved in the disease progression of IC/BPS patients. Previous reports indicate that PLAC8 is a multifunctional immunomodulator that was originally discovered in the placenta and has been shown to be valuable in multiple immune responses (40). PLAC8 regulates inflammatory responses and activation of immune cells, affecting the immune system’s response to pathogens or other exogenous stimuli (41, 42). For example, PLAC8 has been observed to mediate T cell regulation in clearing genital chlamydia infection (43). In chronic inflammation, rheumatoid arthritis, and autoimmune diseases, PLAC8 expression is often elevated, potentially exacerbating or modulating the inflammatory response by influencing the function of immune cells and the levels of secreted cytokines (41, 44, 45). Our study revealed a significantly higher expression level of PLAC8 protein than in normal healthy tissues, indicating its potential relevance to the pathogenic process of IC/BPS. S100A8/S100A9 is released by neutrophils and monocytes and is considered to be a key regulator involved in a variety of inflammatory responses (46, 47). Current studies confirm its potential use as a predictive biomarker for inflammatory developments such as juvenile rheumatoid arthritis, autoimmune synovitis, and autoimmune diseases (48–50). Moreover, blocking soluble S100A8/A9 secretion may offer an inspiring therapeutic strategy for inflammatory diseases (51). While these findings underscore the crucial role of S100A8 in inflammatory processes, its specific value in patients with IC/BPS warrants further evaluation. PPBP, identified as a potent neutrophil activator, has been shown to activate substance metabolism and synthesis in various cells (52). Numerous studies associate PPBP disorders with inflammatory conditions like acute lung injury and chronic obstructive pulmonary disease (52, 53). Zhang et al. reported PPBP as a marker of podocyte injury in diabetic nephropathy (54). PPBP may participate in immune response regulation by inducing chronic inflammation or Th2 polarization, suggesting its potential involvement in mediating immune response processes in IC/BPS patients (55). Collectively, these findings propose that the three identified candidate genes—PLAC8, S100A8, and PPBP—may play crucial roles in the disease progression of IC/BPS patients, serving as potential diagnostic markers and therapeutic targets.
Research indicates that the immune system’s response in IC/BPS may be triggered by various factors, including infection, injury, or other unknown elements (1). Several immune cells, such as mast cells and T lymphocytes, are implicated in the pathogenesis of IC/BPS (56). Mast cells release inflammatory mediators that contribute to pain and inflammation, while T lymphocytes play a role in immune responses (11). Abnormalities in the function of these immune cells may contribute to the chronic inflammation observed in IC/BPS patients. In our study, we utilized the ssGSEA algorithm and IHC staining to evaluate the immune cell landscapes in IC/BPS patients. Notably, IC/BPS patients exhibited a significantly higher proportion of innate and adaptive immune cell infiltration compared to normal patients (11, 12). We observed a significant positive correlation between PLAC8 expression and various immune cell types, including T cells, B cells, macrophages, dendritic cells, and mast cells. These findings suggest that PLAC8 may play a role in initiating immune responses, maintaining immune tolerance, and regulating inflammatory responses by modulating the function of multiple immune cell types. Neutrophils, a type of white blood cell, play a crucial role in the body’s immune response, particularly in the early stages of inflammation and infection (57). Neutrophils can directly contribute to epithelial damage in urothelial tissue through the release of a variety of harmful mediators, including proteases, reactive oxygen species (ROS), and inflammatory cytokines (58, 59). Furthermore, the prolonged presence of neutrophils and their ongoing release of inflammatory mediators can contribute to the persistence and exacerbation of the inflammatory response (60). Some studies have suggested that inflammation may contribute to the pathophysiology of interstitial cystitis, and neutrophils could be involved in this inflammatory response (59, 61). However, the precise mechanisms and the extent of neutrophil involvement in interstitial cystitis are not fully understood. It’s important to note that the understanding of the relationship between immune cells and interstitial cystitis is still evolving, and more research is needed to fully elucidate the underlying mechanisms.
The PI3K/Akt/mTOR signaling pathway is a crucial signal transduction pathway in mammals, playing a vital role in various biological processes (62). For instance, the activity of this pathway is essential in tissue repair and inflammatory diseases (63, 64). Activation of the PI3K/Akt/mTOR signaling pathway can inhibit autophagy and promote inflammation, whereas its inhibition can enhance autophagy and reduce inflammation (65, 66). In this study, activation of the PI3K/Akt/mTOR signaling pathway was observed in inflammatory cell models, while PLAC8 overexpression inhibited this activation, suggesting a potential therapeutic role for PLAC8 in the inflammatory process of urothelial cells. The possibility of downregulating PLAC8 to modulate the PI3K/Akt/mTOR signaling pathway and suppress the inflammatory response presents a promising avenue for the treatment and prevention of IC/BPS. Although further clinical and experimental studies are needed to confirm our findings, our results offer valuable insights into IC/BPS biomarkers and lay a solid foundation for future research.
5 Conclusions
In summary, we harnessed genomic data to integrate bioinformatics analysis and machine-learning algorithms, unveiling molecular characteristics and immune landscapes specific to IC/BPS patients. The three hub genes, PLAC8, S100A8, and PPBP, are significantly associated with various immune cell types in IC/BPS. Among them, PLAC8 is a promising diagnostic biomarker that modulates the PI3K/Akt/mTOR signaling pathway in patients with IC/BPS, which provides new insights into the future diagnosis of IC/BPS.
Data availability statement
The raw data supporting the conclusions of this article will be made available by the authors, without undue reservation.
Ethics statement
The studies involving humans were approved by The Research Ethics Committee of the Second Affiliated Hospital of Anhui Medical University. The studies were conducted in accordance with the local legislation and institutional requirements. The participants provided their written informed consent to participate in this study. Ethical approval was not required for the studies on animals in accordance with the local legislation and institutional requirements because only commercially available established cell lines were used.
Author contributions
TZ: Investigation, Software, Validation, Visualization, Writing – original draft, Writing – review & editing. CZh: Software, Validation, Visualization, Writing – original draft, Writing – review & editing. WZ: Writing – original draft, Writing – review & editing. QW: Data curation, Validation, Visualization, Writing – original draft, Writing – review & editing. MD: Data curation, Validation, Visualization, Writing – original draft, Writing – review & editing. ZJ: Writing – original draft, Writing – review & editing. LP: Writing – original draft, Writing – review & editing. HG: Writing – original draft, Writing – review & editing. ZT: Formal analysis, Investigation, Software, Validation, Visualization, Writing – original draft, Writing – review & editing. CZo: Conceptualization, Funding acquisition, Investigation, Software, Supervision, Validation, Visualization, Writing – original draft, Writing – review & editing.
Funding
The author(s) declare financial support was received for theresearch, authorship, and/or publication of this article. This study is supported by University Natural Science Research Key Project of Anhui Province (Grant No. 2023AH053164), the Research Foundation of Anhui Medical University (Grant No. 2022xkj180), and the Research Fund of Anhui Institute of translational medicine (Grant No. 2023zhyx-C83).
Conflict of interest
The authors declare that the research was conducted in the absence of any commercial or financial relationships that could be construed as a potential conflict of interest.
Generative AI statement
The author(s) declare that no Generative AI was used in the creation of this manuscript.
Publisher’s note
All claims expressed in this article are solely those of the authors and do not necessarily represent those of their affiliated organizations, or those of the publisher, the editors and the reviewers. Any product that may be evaluated in this article, or claim that may be made by its manufacturer, is not guaranteed or endorsed by the publisher.
Supplementary material
The Supplementary Material for this article can be found online at: https://www.frontiersin.org/articles/10.3389/fimmu.2025.1511529/full#supplementary-material
References
1. Duh K, Funaro MG, DeGouveia W, Bahlani S, Pappas D, Najjar S, et al. Crosstalk between the immune system and neural pathways in interstitial cystitis/bladder pain syndrome. Discovery Med. (2018) 25:243–50.
2. Ko KJ, Cho WJ, Lee YS, Choi J, Byun HJ, Lee KS. Comparison of the efficacy between transurethral coagulation and transurethral resection of Hunner lesion in interstitial cystitis/bladder pain syndrome patients: A prospective randomized controlled trial. Eur Urol. (2020) 77:644–51. doi: 10.1016/j.eururo.2020.01.002
3. Homma Y, Akiyama Y, Tomoe H, Furuta A, Ueda T, Maeda D, et al. Clinical guidelines for interstitial cystitis/bladder pain syndrome. Int J Urol. (2020) 27:578–89. doi: 10.1111/iju.14234
4. Adamian L, Urits I, Orhurhu V, Hoyt D, Driessen R, Freeman JA, et al. A comprehensive review of the diagnosis, treatment, and management of urologic chronic pelvic pain syndrome. Curr Pain Headache Rep. (2020) 24:27. doi: 10.1007/s11916-020-00857-9
5. Clemens JQ, Mullins C, Ackerman AL, Bavendam T, van Bokhoven A, Ellingson BM, et al. Urologic chronic pelvic pain syndrome: insights from the MAPP Research Network. Nat Rev Urol. (2019) 16:187–200. doi: 10.1038/s41585-018-0135-5
6. Li J, Yi X, Ai J. Broaden horizons: the advancement of interstitial cystitis/bladder pain syndrome. Int J Mol Sci. (2022) 23:14594. doi: 10.3390/ijms232314594
7. Peng L, Jin X, Li BY, Zeng X, Liao BH, Jin T, et al. Integrating single-cell RNA sequencing with spatial transcriptomics reveals immune landscape for interstitial cystitis. Signal Transduct Target Ther. (2022) 7:161. doi: 10.1038/s41392-022-00962-8
8. Su F, Zhang W, Meng L, Zhang W, Liu X, Liu X, et al. Multimodal single-cell analyses outline the immune microenvironment and therapeutic effectors of interstitial cystitis/bladder pain syndrome. Adv Sci (Weinh). (2022) 9:e2106063. doi: 10.1002/advs.202106063
9. Elgebaly SA, Allam ME, Walzak MJ, Oselinsky D, Gillies C, Yamase H. Urinary neutrophil chemotactic factors in interstitial cystitis patients and a rabbit model of bladder inflammation. J Urol. (1992) 147:1382–87. doi: 10.1016/s0022-5347(17)37578-x
10. Akiyama Y, Harada K, Miyakawa J, Kreder KJ, O’Donnell MA, Daichi M, et al. Th1/17 polarization and potential treatment by an anti-interferon-γ DNA aptamer in Hunner-type interstitial cystitis. Iscience. (2023) 26:108262. doi: 10.1016/j.isci.2023.108262
11. Moldwin RM, Nursey V, Yaskiv O, Dalvi S, Macdonald EJ, Funaro M, et al. Immune cell profiles of patients with interstitial cystitis/bladder pain syndrome. J Transl Med. (2022) 20:97. doi: 10.1186/s12967-022-03236-7
12. Zhang W, Liu X, Wang J, Wang X, Zhang Y. Immunogenic cell death associated molecular patterns and the dual role of IL17RA in interstitial cystitis/bladder pain syndrome. Biomolecules. (2023) 13:421. doi: 10.3390/biom13030421
13. Wang G, Yang TX, Li JM, Huang ZY, Yang WB, Li P, et al. Bruton tyrosine kinase (BTK) may be a potential therapeutic target for interstitial cystitis/bladder pain syndrome. Aging (Albany Ny). (2022) 14:7052–64. doi: 10.18632/aging.204271
14. Saha SK, Jeon TI, Jang SB, Kim SJ, Lim KM, Choi YJ, et al. Bioinformatics approach for identifying novel biomarkers and their signaling pathways involved in interstitial cystitis/bladder pain syndrome with Hunner lesion. J Clin Med. (2020) 9.:1935 doi: 10.3390/jcm9061935
15. Gamper M, Viereck V, Geissbühler V, Eberhard J, Binder J, Moll C, et al. Gene expression profile of bladder tissue of patients with ulcerative interstitial cystitis. BMC Genomics. (2009) 10:199. doi: 10.1186/1471-2164-10-199
16. Blalock EM, Korrect GS, Stromberg AJ, Erickson DR. Gene expression analysis of urine sediment: evaluation for potential noninvasive markers of interstitial cystitis/bladder pain syndrome. J Urol. (2012) 187:725–32. doi: 10.1016/j.juro.2011.09.142
17. Colaco M, Koslov DS, Keys T, Evans RJ, Badlani GH, Andersson KE, et al. Correlation of gene expression with bladder capacity in interstitial cystitis/bladder pain syndrome. J Urol. (2014) 192:1123–29. doi: 10.1016/j.juro.2014.05.047
18. Jiang Y, Zhu X, Al-Danakh AY, Chen Q, Yang D. Identification of immune-related genes and small-molecule drugs in interstitial cystitis/bladder pain syndrome based on the integrative machine learning algorithms and molecular docking. J Immunol Res. (2022) 2022:2069756. doi: 10.1155/2022/2069756
19. Leek JT, Johnson WE, Parker HS, Jaffe AE, Storey JD. The sva package for removing batch effects and other unwanted variation in high-throughput experiments. Bioinformatics. (2012) 28:882–83. doi: 10.1093/bioinformatics/bts034
20. Ritchie ME, Phipson B, Wu D, Hu Y, Law CW, Shi W, et al. limma powers differential expression analyses for RNA-sequencing and microarray studies. Nucleic Acids Res. (2015) 43:e47. doi: 10.1093/nar/gkv007
21. Yu G, Wang LG, Han Y, He QY. clusterProfiler: an R package for comparing biological themes among gene clusters. Omics. (2012) 16:284–87. doi: 10.1089/omi.2011.0118
22. Lin Y, Zhang Y, Tuo Z, Gao L, Ding D, Bi L, et al. ORC6, a novel prognostic biomarker, correlates with T regulatory cell infiltration in prostate adenocarcinoma: a pan-cancer analysis. BMC Cancer. (2023) 23:285. doi: 10.1186/s12885-023-10763-z
23. Zou C, Xu S, Geng H, Li E, Sun W, Yu D. Bioinformatics analysis identifies potential hub genes and crucial pathways in the pathogenesis of asthenozoospermia. BMC Med Genomics. (2022) 15:252. doi: 10.1186/s12920-022-01407-5
24. Langfelder P, Horvath S. WGCNA: an R package for weighted correlation network analysis. BMC Bioinf. (2008) 9:559. doi: 10.1186/1471-2105-9-559
25. Wang H, Yang F, Luo Z. An experimental study of the intrinsic stability of random forest variable importance measures. BMC Bioinf. (2016) 17:60. doi: 10.1186/s12859-016-0900-5
26. Cheung-Lee WL, Link AJ. Genome mining for lasso peptides: past, present, and future. J Ind Microbiol Biotechnol. (2019) 46:1371–79. doi: 10.1007/s10295-019-02197-z
27. Huang S, Cai N, Pacheco PP, Narrandes S, Wang Y, Xu W. Applications of support vector machine (SVM) learning in cancer genomics. Cancer Genomics Proteomics. (2018) 15:41–51. doi: 10.21873/cgp.20063
28. Yang L, Qu Q, Hao Z, Sha K, Li Z, Li S. Powerful identification of large quantitative trait loci using genome-wide R/glmnet-based regression. J Hered. (2022) 113:472–78. doi: 10.1093/jhered/esac006
29. Hengl T, Nussbaum M, Wright MN, Heuvelink G, Gräler B. Random forest as a generic framework for predictive modeling of spatial and spatio-temporal variables. Peerj. (2018) 6:e5518. doi: 10.7717/peerj.5518
30. Scharl T, Grü B, Leisch F. Mixtures of regression models for time course gene expression data: evaluation of initialization and random effects. Bioinformatics. (2010) 26:370–77. doi: 10.1093/bioinformatics/btp686
31. Zhang Z, Rousson V, Lee WC, Ferdynus C, Chen M, Qian X, et al. Decision curve analysis: a technical note. Ann Transl Med. (2018) 6:308. doi: 10.21037/atm.2018.07.02
32. Robin X, Turck N, Hainard A, Tiberti N, Lisacek F, Sanchez JC, et al. pROC: an open-source package for R and S+ to analyze and compare ROC curves. BMC Bioinf. (2011) 12:77. doi: 10.1186/1471-2105-12-77
33. Zhang WY, Chen ZH, An XX, Li H, Zhang HL, Wu SJ, et al. Analysis and validation of diagnostic biomarkers and immune cell infiltration characteristics in pediatric sepsis by integrating bioinformatics and machine learning. World J Pediatr. (2023) 19:1094–103. doi: 10.1007/s12519-023-00717-7
34. Hänzelmann S, Castelo R, Guinney J. GSVA: gene set variation analysis for microarray and RNA-seq data. BMC Bioinf. (2013) 14:7. doi: 10.1186/1471-2105-14-7
35. Chelimsky G, Heller E, Buffington CA, Rackley R, Zhang D, Chelimsky T. Co-morbidities of interstitial cystitis. Front Neurosci. (2012) 6:114. doi: 10.3389/fnins.2012.00114
36. Wang X, Lu Y, Tuo Z, Zhou H, Zhang Y, Cao Z, et al. Role of SIRT1/AMPK signaling in the proliferation, migration and invasion of renal cell carcinoma cells. Oncol Rep. (2021) 45:109. doi: 10.3892/or.2021.8060
37. Jhang JF, Jiang YH, Kuo HC. Current understanding of the pathophysiology and novel treatments of interstitial cystitis/bladder pain syndrome. Biomedicines. (2022) 10:2380. doi: 10.3390/biomedicines10102380
38. Hanno PM, Erickson D, Moldwin R, Faraday MM. Diagnosis and treatment of interstitial cystitis/bladder pain syndrome: AUA guideline amendment. J Urol. (2015) 193:1545–53. doi: 10.1016/j.juro.2015.01.086
39. Song K, Dayem AA, Lee S, Choi Y, Lim KM, Kim S, et al. Superior therapeutic activity of TGF-β-induced extracellular vesicles against interstitial cystitis. J Control Release. (2022) 348:924–37. doi: 10.1016/j.jconrel.2022.06.045
40. Ledford JG, Kovarova M, Koller BH. Impaired host defense in mice lacking ONZIN. J Immunol. (2007) 178:5132–43. doi: 10.4049/jimmunol.178.8.5132
41. Tsuboi H, Segawa S, Yagishita M, Toko H, Honda F, Kitada A, et al. Activation mechanisms of monocytes/macrophages in adult-onset Still disease. Front Immunol. (2022) 13:953730. doi: 10.3389/fimmu.2022.953730
42. Mao M, Chen Y, Yang J, Cheng Y, Xu L, Ji F, et al. Modification of PLAC8 by UFM1 affects tumorous proliferation and immune response by impacting PD-L1 levels in triple-negative breast cancer. J Immunother Cancer. (2022) 10:e005668. doi: 10.1136/jitc-2022-005668
43. Johnson RM, Kerr MS, Slaven JE. Plac8-dependent and inducible NO synthase-dependent mechanisms clear Chlamydia muridarum infections from the genital tract. J Immunol. (2012) 188:1896–904. doi: 10.4049/jimmunol.1102764
44. Ciraci E, Elgun T, Gok YA, Cagin KH, Ekenoglu MY, Sait TM, et al. Evaluation of serum placenta-specific gene 8 protein, total antioxidant capacity, interleukin-10, interleukin-17A, interleukin-21 and interleukin-33 levels in Turkish women with gestational diabetes mellitus. Endocrinol Diabetes Nutr (Engl Ed). (2024) 71:12–8. doi: 10.1016/j.endien.2024.02.002
45. Wen J, Li H, Zhou Y, Du H, Hu G, Wen Z, et al. Immunoglobin attenuates fulminant myocarditis by inhibiting overactivated innate immune response. Br J Pharmacol. (2024). doi: 10.1111/bph.17372
46. Coveney AP, Wang W, Kelly J, Liu JH, Blankson S, Wu QD, et al. Myeloid-related protein 8 induces self-tolerance and cross-tolerance to bacterial infection via TLR4- and TLR2-mediated signal pathways. Sci Rep. (2015) 5:13694. doi: 10.1038/srep13694
47. Holzinger D, Nippe N, Vogl T, Marketon K, Mysore V, Weinhage T, et al. Myeloid-related proteins 8 and 14 contribute to monosodium urate monohydrate crystal-induced inflammation in gout. Arthritis Rheumatol. (2014) 66:1327–39. doi: 10.1002/art.38369
48. Nistala K, Varsani H, Wittkowski H, Vogl T, Krol P, Shah V, et al. Myeloid related protein induces muscle derived inflammatory mediators in juvenile dermatomyositis. Arthritis Res Ther. (2013) 15:R131. doi: 10.1186/ar4311
49. De Rycke L, Baeten D, Foell D, Kruithof E, Veys EM, Roth J, et al. Differential expression and response to anti-TNFalpha treatment of infiltrating versus resident tissue macrophage subsets in autoimmune arthritis. J Pathol. (2005) 206:17–27. doi: 10.1002/path.1758
50. Frosch M, Strey A, Vogl T, Wulffraat NM, Kuis W, Sunderkötter C, et al. Myeloid-related proteins 8 and 14 are specifically secreted during interaction of phagocytes and activated endothelium and are useful markers for monitoring disease activity in pauciarticular-onset juvenile rheumatoid arthritis. Arthritis Rheum. (2000) 43:628–37. doi: 10.1002/1529-0131(200003)43:3<628::AID-ANR20>3.0.CO;2-X
51. Xu YD, Wang Y, Yin LM, Park GH, Ulloa L, Yang YQ. S100A8 protein attenuates airway hyperresponsiveness by suppressing the contraction of airway smooth muscle. Biochem Biophys Res Commun. (2017) 484:184–88. doi: 10.1016/j.bbrc.2017.01.033
52. Ghasemzadeh M, Kaplan ZS, Alwis I, Schoenwaelder SM, Ashworth KJ, Westein E, et al. The CXCR1/2 ligand NAP-2 promotes directed intravascular leukocyte migration through platelet thrombi. Blood. (2013) 121:4555–66. doi: 10.1182/blood-2012-09-459636
53. Bdeir K, Gollomp K, Stasiak M, Mei J, Papiewska-Pajak I, Zhao G, et al. Platelet-specific chemokines contribute to the pathogenesis of acute lung injury. Am J Respir Cell Mol Biol. (2017) 56:261–70. doi: 10.1165/rcmb.2015-0245OC
54. Zhang F, Jiang N, Gao Y, Fan Z, Li Q, Ke G, et al. PPBP as a marker of diabetic nephropathy podocyte injury via Bioinformatics Analysis. Biochem Biophys Res Commun. (2021) 577:165–72. doi: 10.1016/j.bbrc.2021.08.087
55. Wang YS, Liao KW, Chen MF, Huang YC, Chu RM, Chi KH. Canine CXCL7 and its functional expression in dendritic cells undergoing maturation. Vet Immunol Immunopathol. (2010) 135:128–36. doi: 10.1016/j.vetimm.2009.11.011
56. Neuhaus J, Schwalenberg T. Intravesical treatments of bladder pain syndrome/interstitial cystitis. Nat Rev Urol. (2012) 9:707–20. doi: 10.1038/nrurol.2012.217
57. Herrero-Cervera A, Soehnlein O, Kenne E. Neutrophils in chronic inflammatory diseases. Cell Mol Immunol. (2022) 19:177–91. doi: 10.1038/s41423-021-00832-3
58. Kuhn HW, Hreha TN, Hunstad DA. Immune defenses in the urinary tract. Trends Immunol. (2023) 44:701–11. doi: 10.1016/j.it.2023.07.001
59. Mintz D, Salamon H, Mintz M, Rosenshine I, Shpigel NY. Intraepithelial neutrophils in mammary, urinary and gall bladder infections. Vet Res. (2019) 50:56. doi: 10.1186/s13567-019-0676-5
60. Riaz B, Sohn S. Neutrophils in inflammatory diseases: unraveling the impact of their derived molecules and heterogeneity. Cells. (2023) 12:2621. doi: 10.3390/cells12222621
61. Ke H, Zhu L, Wang Q, Xu K. Neutrophil-to-lymphocyte ratio as a promising non-invasive biomarker for symptom assessment and diagnosis of interstitial cystitis/bladder pain syndrome. BMC Urol. (2023) 23:180. doi: 10.1186/s12894-023-01353-z
62. Tehranian C, Fankhauser L, Harter PN, Ratcliffe C, Zeiner PS, Messmer JM, et al. The PI3K/Akt/mTOR pathway as a preventive target in melanoma brain metastasis. Neuro Oncol. (2022) 24:213–25. doi: 10.1093/neuonc/noab159
63. Xia J, Li CY, Wang H, Zhang QM, Han ZM. Therapeutic effects of scoparone on pilocarpine (Pilo)-induced seizures in mice. BioMed Pharmacother. (2018) 97:1501–13. doi: 10.1016/j.biopha.2017.09.127
64. Lu X, Masic A, Liu Q, Zhou Y. Regulation of influenza A virus induced CXCL-10 gene expression requires PI3K/Akt pathway and IRF3 transcription factor. Mol Immunol. (2011) 48:1417–23. doi: 10.1016/j.molimm.2011.03.017
65. Liu B, Deng X, Jiang Q, Li G, Zhang J, Zhang N, et al. Scoparone improves hepatic inflammation and autophagy in mice with nonalcoholic steatohepatitis by regulating the ROS/P38/Nrf2 axis and PI3K/AKT/mTOR pathway in macrophages. BioMed Pharmacother. (2020) 125:109895. doi: 10.1016/j.biopha.2020.109895
Keywords: IC/BPS, bioinformatics, machine-learning, PLAC8, immune cell landscape
Citation: Zhou T, Zhu C, Zhang W, Wu Q, Deng M, Jiang Z, Peng L, Geng H, Tuo Z and Zou C (2025) Identification and validation of immune and diagnostic biomarkers for interstitial cystitis/painful bladder syndrome by integrating bioinformatics and machine-learning. Front. Immunol. 16:1511529. doi: 10.3389/fimmu.2025.1511529
Received: 07 November 2024; Accepted: 03 January 2025;
Published: 23 January 2025.
Edited by:
Pedro L. Vera, Lexington VA Health Care System, United StatesReviewed by:
Hann-Chorng Kuo, Hualien Tzu Chi Hospital, TaiwanTongxin Yang, The Second Affiliated Hospital of Kunming Medical University, China
Copyright © 2025 Zhou, Zhu, Zhang, Wu, Deng, Jiang, Peng, Geng, Tuo and Zou. This is an open-access article distributed under the terms of the Creative Commons Attribution License (CC BY). The use, distribution or reproduction in other forums is permitted, provided the original author(s) and the copyright owner(s) are credited and that the original publication in this journal is cited, in accordance with accepted academic practice. No use, distribution or reproduction is permitted which does not comply with these terms.
*Correspondence: Hao Geng, MTM3MzkyOTYwODlAMTYzLmNvbQ==; Zhouting Tuo, dHVvemhvdXRpbmdAZ21haWwuY29t; Ci Zou, em91Y2lAYWhtdS5lZHUuY24=
†These authors have contributed equally to this work