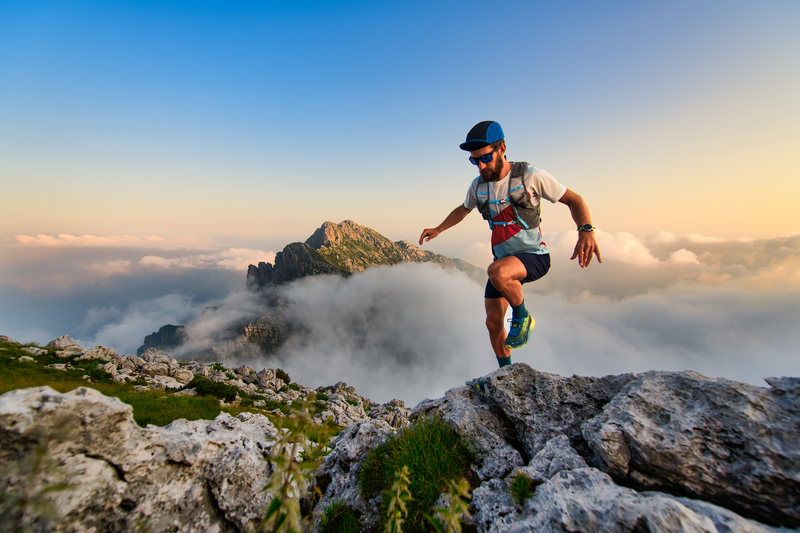
95% of researchers rate our articles as excellent or good
Learn more about the work of our research integrity team to safeguard the quality of each article we publish.
Find out more
ORIGINAL RESEARCH article
Front. Immunol. , 10 January 2025
Sec. Cancer Immunity and Immunotherapy
Volume 15 - 2024 | https://doi.org/10.3389/fimmu.2024.1521930
This article is part of the Research Topic Harnessing Big Data for Precision Medicine: Revolutionizing Diagnosis and Treatment Strategies View all 36 articles
Introduction: Breast cancer (BC) is the most prevalent malignant tumor in women, with triple-negative breast cancer (TNBC) showing the poorest prognosis among all subtypes. Glycosylation is increasingly recognized as a critical biomarker in the tumor microenvironment, particularly in BC. However, the glycosylation-related genes associated with TNBC have not yet been defined. Additionally, their characteristics and relationship with prognosis have not been deeply investigated.
Methods: Transcriptomic analyses were used to identify a glycosylation-related signature (GRS) associated with TNBC prognosis. A machine learning-based prediction model was constructed and validated across multiple independent datasets. The model's predictive capability was extended to evaluate the prognosis of TNBC individuals, tumor immune microenvironment and immunotherapy response. LMAN1L (Lectin, Mannose Binding 1 Like) was identified as a novel prognostic marker in TNBC, and its biological effects were validated through experimental assays.
Results: The GRS showed significant prognostic relevance for TNBC patients. The risk model effectively predicted molecular features, including immune cell infiltration and potential responses to immunotherapy. Experimental validation confirmed LMAN1L as a novel glycosylation-related prognostic gene, with low expression significantly inhibiting TNBC cell proliferation and migration.
Discussion: Our GRS risk model demonstrates robust predictive capability for TNBC prognosis and immunotherapy response. This model offers a promising strategy for personalized treatment and improved clinical outcomes in TNBC.
Breast cancer (BC) is the most common malignant tumor in women (1), with triple-negative breast cancer (TNBC) accounting for 15-20% of BC cases. TNBC is characterized by poor prognosis, high recurrence, and limited treatment options, including surgery, chemotherapy, and radiotherapy (2, 3). Despite advances, metastatic TNBC has a median overall survival of less than two years, highlighting the need for improved therapies (4, 5).
Glycosylation, a crucial post-translational modification, plays a key role in cellular functions such as protein stability, immune evasion, and signal transduction (6–8). Tumor cells exhibit abnormal glycosylation patterns, which contribute to cancer progression, metastasis, and drug resistance (9–13). In BC, altered glycosylation affects cell signaling, adhesion, and immune recognition, promoting tumor aggressiveness (14–17). Suppressing protein glycosylation levels has also been shown to reduce drug resistance in malignant BC cells (18, 19). Presently, biomarkers for TNBC prognosis and therapy lack accuracy and suitability. However, glycosylation patterns in TNBC offer promising biomarkers for early diagnosis, prognosis, and treatment monitoring, as well as new therapeutic targets, though further in-depth research is essential to realize their full clinical potential.
Our study confirmed the value of glycosylation in TNBC, defined a set of glycosylation-related genes affecting TNBC prognosis, and used various machine learning algorithms to construct a clinical prediction model that can assist in predicting clinical prognosis and treatment, as well as evaluating the associated immune environment, responsiveness to immunotherapy, and the targeted drugs for high- and low-risk groups. Additionally, we conducted a series of experimental validations and preliminarily demonstrated its function and value, and innovatively discovered the potential of the glycosylation-related gene LMAN1L in TNBC.
RNA-seq data and corresponding clinicopathological information for the SCANB cohort obtained sourced from Mendeley Data (https://data.mendeley.com/datasets/yzxtxn4nmd/3) (20, 21). Additionally, genome-wide expression data and clinicopathological information for two other TNBC cohorts were retrieved from The Cancer Genome Atlas (TCGA-TNBC) (22) and Gene Expression Omnibus (GSE103091) (23, 24). RNA-seq data for BC cell lines were obtained from the Cancer Cell Line Encyclopedia (CCLE, https://sites.broadinstitute.org/ccle/) (25). Complete RNA-seq data and clinical characteristics for the IMvigor210 cohort, which focuses on anti-PD-L1 immunotherapy in bladder cancer, were retrieved from (http://research-pub.gene.com/IMvigor210CoreBiologies/). RNA-seq read counts from SCANB, TCGA, and GSE103091 were converted to transcripts per kilobase million (TPM) and subsequently log2-transformed. Similarly, RNA-seq read counts from the IMvigor201 cohort were converted to TPM and log2-transformed.
Differentially expressed genes (DEGs) were identified (p < 0.05, log2FC > 1) using the ‘DESeq2’ package in R (26), comparing TNBC tumor samples with normal breast tissue samples from the TCGA database. Prognosis-related genes (PRGs) were identified in all three cohorts (SCANB, TCGA, and GSE103091) via univariate Cox regression analysis (p < 0.05). Glycosylation-related genes (GRGs) were sourced from the KEGG BRITE Database (https://www.kegg.jp/kegg/brite.html) and previous literature (27), with the complete list provided in Supplementary Table S1. A total of 35 glycosylation-related genes that were both differentially expressed and prognostically significant were designated as glycosylation-related signature (GRS) for TNBC. The complete list of GRS is provided in Supplementary Table S2. The molecular pathways related to these genes were analyzed using the ‘clusterProfiler’ package (28) in R for Gene Ontology (GO) and Kyoto Encyclopedia of Genes and Genomes (KEGG) enrichment analysis.
To develop a high-accuracy, stable GRS risk model, we applied ten machine learning algorithms and 101 algorithmic combinations, including Lasso, Ridge regression, stepwise Cox regression, Elastic net (Enet), CoxBoost, random survival forest (RSF), supervised principal components (SuperPC), partial least squares regression for Cox (plsRcox), generalized boosted regression modeling (GBM), and survival support vector machine (survival-SVM).
The signature development process involved: (a) applying 101 algorithmic combinations to the GRS to construct prediction models based on leave-one-out cross-validation (LOOCV) within the SCANB cohort; (b) evaluating all models across the SCANB training dataset and two validation datasets (TCGA-TNBC and GSE103091); and (c) selecting the optimal model based on Harrell’s concordance index (C-index) across the validation datasets. The optimal model was defined using regression coefficients, with the GRS risk score formula as GRS risk score =. Survival modeling and Kaplan-Meier (KM) analyses were performed across all datasets using the ‘survival’ and ‘survminer’ packages in R.
Patients in the SCANB cohort were divided into high- and low-risk groups based on the median GRS risk score. Using PAM50 subtyping, the SCANB and TCGA cohorts were categorized into basal and non-basal groups. Differences in clinicopathological features between the two groups were compared across both the training and validation datasets and visualized using the ‘ggplot2’ package in R. Molecular pathways associated with DEGs between the two groups [p < 0.05, log2FC > 1, screened using ‘limma’ package in R (29)] further explored through GO and KEGG pathway analysis. Additionally, the GSEA software (https://www.gsea-msigdb.org/gsea/login.jsp/) was employed to analyze significantly enriched pathways of these DEGs.
Somatic mutation and copy number variation data for the SCANB cohort were retrieved (30). The ‘maftools’ R package (31) was used to analyze and visualize MAF files of somatic mutation data for the two groups and calculate the tumor mutation burden (TMB) score for SCANB patients.
The immune cell infiltration for TNBC patients in the SCANB cohort was estimated using CIBERSORT (32), XCELL (33), and TIMER (34). The immune, stromal, and ESTIMATE scores, along with tumor purity scores, were calculated using ‘estimate’ package in R (35). Immunomodulators, including major histocompatibility complex (MHC) molecules, immunostimulators, immunostimulatory receptors, immunoinhibitors, and immunoinhibitory receptor markers, were collected from a previous study (36). The results were visualized using stacked graphs, heat maps, violin plots, and box plots generated with ‘ggplot2’ package in R. The Hematoxylin-eosin (HE) staining immunophenotype pathology image data (Formalin-fixed paraffin-embedding) in TCGA datasets were obtained from the Cancer Digital Slide Archive (CDSA, https://cancer.digitalslidearchive.org/).
The SubMap algorithm (https://cloud.genepattern.org/gp) was employed to estimate the potential response of SCANB samples to immunotherapy. Predicted responses to anti-PD-1 and anti-CTLA-4 therapies were compared between high- and low-risk groups using annotation file subtype data (37). Additionally, the GRS risk model was applied to the IMvigor210 cohort to explore its predictive value for anti-PD-L1 therapy. Patients exhibiting stable disease (SD) or progressive disease (PD) were categorized as non-responders, whereas those showing complete response (CR) or partial response (PR) were categorized as responders. To validate the predictive robustness of the GRS risk model, we also analyzed a cohort of 144 melanoma patients treated with anti-PD-1 immune checkpoint blockade (ICB) (38).
Expression profile data of human cancer cell lines (CCLs) were downloaded from the Broad Institute Cancer Cell Line Encyclopedia (CCLE) project (39). Drug sensitivity analysis was performed using estimated AUC and IC50 values from the Genomics of Drug Sensitivity in Cancer (GDSC) database (40). Using the ‘lolR’ package in R, a nearest centroid classifier was developed to predict the risk group classification of BC cell lines based on the expression of essential genes from the SCANB cohort. Prior to drug sensitivity comparison, missing AUC values were imputed using K-nearest neighbor (k-NN) imputation, with compounds having over 20% missing data excluded. The intersections of potential drug candidates and comparisons between high- and low-risk groups were visualized using Venn diagrams, box plots, and bar plots created with ‘ggplot2’ package in R.
MDA-MB-231 cells were cultured in Leibovitz L-15 medium with 10% fetal bovine serum (FBS, Sigma, F2442). The LM2 cell line, derived from MDA-MB-231, was cultured in Dulbecco’s modified Eagle’s medium (DMEM) with 10% FBS. SUM159PT cells were maintained in Ham’s F12 medium supplemented with 5% FBS, 5μg/mL insulin, 1μg/mL hydrocortisone, and 10mM HEPES. MCF-10A cells were maintained in DMEM/F12 medium supplemented with 5% HS, 10μg/mL insulin, 0.5μg/mL hydrocortisone, 20ng/ml EGF, and 100ng/ml Cholera toxin. Cell lines BT-549, SUM149PT, and HCC1937 were cultured in RPMI 1640 with 10% FBS. All cell lines were cultured in a humidified 5% CO2 atmosphere at 37°C, except MDA-MB-231, which was maintained in 100% air. All cell lines were authenticated by short tandem repeat (STR) profiling.
The sequences of small interfering RNA (siRNA) oligonucleotides (provided in Supplementary Table S3) were purchased from Shanghai GenePharma Co. Ltd. Oligo RNA transfection (100nM) was performed using Lipofectamine 2000 following the Reverse Transfection Protocol.
Total RNA was extracted using TRIzol reagent (Invitrogen, Carlsbad, CA) following the manufacturer’s instructions. First-strand cDNA was synthesized using a Reverse Transcriptase kit (Tiangen, China). Quantitative Real-Time PCR (RT-qPCR) was performed using the SYBR Green method (Applied Biosystems, USA) on the 7900 Real-Time PCR System with the SDS 2.4 software sequence detection system (Applied Biosystems, USA). Primer sequences are listed in Supplementary Table S4. β-actin was used as an internal control to quantify mRNA levels. The relative expression levels of RNA were calculated using the 2−ΔΔCT method.
Cell migration ability was assessed using Corning transwell insert chambers (8μm pore size; Corning). A chemoattractant (600μl of medium containing 10% FBS) was added to the lower well of each chamber. Approximately 1.5 × 10^4 cells were seeded into each chamber and incubated for 20–22 hours at 37°C.
For plate colony-forming assay, cells were seeded into 3.5 cm culture dishes (800 cells per dish) and incubated for 7–12 days, with the medium changed every 3 days. The colonies were stained with crystal violet (1.5%, w/v; Sigma, St Louis, MO, USA).
For the CCK-8 assay, cells were seeded in 96-well plates (3 × 10^3 cells per well) and incubated lasting 3 days at 37°C. The changes of cell proliferation were monitored daily using CCK-8 reagent (Dojindo, Kumamoto, Japan), and the absorbance values were measured at 450 nm using a Hybrid Reader (BioTek, Winooski, VT, USA).
Cells were seeded in 6-well plates at a density of 3×10^4 cells per well and cultured for 24 hours. Subsequently, 5μM EdU (Meilunbio, Dalian, China) was added and incubated for 2 hours. The remaining steps, including fixation, glycine incubation, and dilution, were carried out following standard protocols in a light-protected environment. Fluorescence images were captured using a fluorescent cell imager (Bio-Rad, Hercules, USA), and at least three random fields per well were photographed.
Cells were lysed in 1× SDS lysis buffer for 15 minutes. Proteins were separated via SDS-PAGE and transferred to nitrocellulose membranes (Axygen, Union City, CA). After blocking with 5% non-fat milk, membranes were incubated overnight at 4°C with primary antibodies, followed by incubation with horseradish peroxidase-conjugated secondary antibodies. Signals were detected using an ECL detection kit (Millipore) and visualized using the ImageQuant LAS 4000 mini system (GE Healthcare, Piscataway, NJ, USA).
TNBC patient tissue samples were obtained from our previous study (41). Tissue sections (4-5 µm) were cut from paraffin-embedded blocks and mounted on slides. The slides were deparaffinized in xylene and rehydrated through a graded ethanol series. Antigen retrieval was performed by heating the slides in citrate buffer (pH 6.0) in a microwave for 20 minutes. Endogenous peroxidase activity was blocked with 3% hydrogen peroxide for 10 minutes. After blocking non-specific binding sites with normal serum, the sections were incubated overnight at 4°C with the primary antibody, followed by incubation with a fluorescent secondary antibody. The slides were then mounted and analyzed using microscopy.
All statistical analyses were performed using R software (version 3.6.0) or GraphPad Prism version 10 software. Significance was calculated using unpaired two-tailed Student’s t test. Data are represented as mean ± SEM or ± SD with at least three independent experiments. For all figures, statistical significance was represented as *P < 0.05, **P < 0.01, and ***P < 0.001.
The flowchart of our study was shown in Figure 1A. Initially, based on the expression profiles of three cohorts (SCANB, TCGA-TNBC, and GSE103091), we identified 4,434 prognostic-related genes (PRGs) through univariate Cox regression analysis. In addition, 9,589 differentially expressed genes (DEGs) were identified between TNBC tumor samples and adjacent normal tissues with statistical significance (p < 0.05 and log2FC > 1). We then integrated glycosylation-related genes from the KEGG BRITE Database and a previous study (27), resulting in a gene set of 481 glycosylation-related genes. By intersecting the three gene sets, we identified a glycosylation-related signature (GRS) with prognostic significance in TNBC (Figure 1B). Further Gene Ontology (GO) and Kyoto Encyclopedia of Genes and Genomes (KEGG) enrichment analyses revealed that the GRS is involved in multiple glycosylation-associated pathways, including glycoprotein metabolic processes, protein N-linked glycosylation, O-glycan processing, oligosaccharide metabolic process and amino sugar metabolic process (Figure 1C).
Figure 1. Construction of a glycosylation-related signature. (A) Study flow chart. (B) Venn diagram showing the intersection of prognosis-related genes (PRG), differentially expressed genes (DEG), and glycosylation-related genes (GRG). (C) Gene Ontology (GO) and Kyoto Encyclopedia of Genes and Genomes (KEGG) pathway analysis of the glycosylation-related signature (GRS).
To ensure high accuracy and stability, we utilized multiple machine learning algorithms to develop a risk model using GRS. In the SCANB dataset, 101 prediction models were fitted using the leave-one-out cross-validation (LOOCV) framework, and the C-index of each model was calculated across all validation datasets (Figure 2A). Notably, the optimal model combined Lasso regression with the Random Survival Forest (RSF), achieving the highest average C-index (0.752). This combination consistently outperformed other models in all validation datasets. In the Lasso regression, the optimal λ was determined when the partial likelihood deviance minimized, based on the LOOCV framework (Figures 2B, C). Using these two algorithms, we performed gene selection and model construction, identifying the 21 genes with the highest variable importance (Figures 2D, E). A risk score for each patient was then calculated using the expression levels of the 21 genes, weighted by their regression coefficients. Patients were stratified into high- and low-risk groups based on the optimal cut-off value, determined by the ‘survminer’ package. Patients in the high-risk group had significantly worse overall survival (OS) compared to those in the low-risk group across the SCANB training dataset and validation datasets (all P < 0.05) (Figures 2F–H). Using the basal-like phenotype, we categorized TNBC patients into basal and non-basal groups and observed that our model maintained consistent predictive accuracy across the subtypes (Figures 2I, J, Supplementary Figures S1A, B).
Figure 2. Construction of a GRS risk model via machine learning algorithms. (A) 101 prediction models were evaluated using the leave-one-out cross-validation (LOOCV) framework, with the concordance index (C-index) calculated for each model across all validation datasets. (B) The optimal λ value in the SCANB cohort was identified at the minimum partial likelihood deviance. (C) Lasso coefficients were derived for the most informative prognostic genes. (D) Error rate and (E) variable importance were assessed using random survival forest (RSF) analysis. Kaplan-Meier survival curves for overall survival (OS) were stratified by GRS risk groups in (F) SCANB, (G) TCGA-TNBC, (H) GSE103091, (I) SCANB-Basal and (J) SCANB-Non-Basal cohorts.
In addition, we investigated the relationship between the risk score and clinical features. In the SCANB cohort, the results suggested a significant difference in overall stage, N stage, and T stage (Figure 3A). In the GSE103091 database, we also observed a significant correlation between risk scores and clinical staging (Figure 3B). Unfortunately, due to limitations in sample size in the TCGA database, we did not observe a significant difference in clinical characteristics between high- and low-risk groups. However, it can be seen that the patients in the high-risk group had more advanced tumor progression, which is consistent with the results of the other two databases (Figure 3C). Furthermore, we explored the biological functions and enriched pathways between low- and high-risk groups. The high-risk group was significantly enriched for regulation of lymphocyte activation, regulation of immune response and cytokine production by GO analysis (Figure 3D). KEGG analysis indicated that the top three pathways were cytokine-cytokine receptor interaction, NF-kappa B signaling pathway and Th17 cell differentiation (Figure 3E). To further confirm the correlation between risk scores and biological function, we performed Gene Set Enrichment Analysis (GSEA). The results showed that the risk score was positively correlated with oxidative phosphorylation and organic acid catabolic process (Figures 3F, G), and was negatively correlated with adaptive immune response, lymphocyte receptor signaling pathway, and cytokine-cytokine receptor interaction (Figures 3H, I).
Figure 3. Association of GRS risk model with clinical characteristics in TNBC patients. Circos plots showing survival and pathological differences between two risk groups in the (A) SCANB, (B) GSE103091, and (C) TCGA-TNBC cohorts (Chi-square test). (D) GO and (E) KEGG analysis of differentially expressed genes between high- and low-risk groups. (F-I) GO and KEGG terms enriched by differentially expressed genes using GSEA analysis between the two risk groups.
The above GSEA revealed that several immune-related pathways were highly enriched in the low-risk group, and we consequently investigated the immune landscape and expression of immune-related markers between the two groups. We employed a variety of algorithms, including TIMER, XCELL, and CIBERSORT to comprehensively investigate the infiltration of immune cells across two groups within the SCANB cohort (Figure 4A). We found that the low-risk group exhibited a relatively higher infiltration abundance of immune cell types, including B cells, CD4+ naïve T cells, CD8+ T cells and neutrophils (all p<0.05). However, the high-risk group exhibited lower immune cell infiltration, with a higher presence of tumor parenchymal cells. The HE staining immunophenotype also revealed that high-risk scores were associated with reduced immune cell infiltration, whereas low-risk scores were correlated with increased immune cell infiltration (Figures 4B, C). In addition, among the immune-related markers, the low-risk group had significantly higher relative expression levels, such as NECTIN2, CD70, TNFSF4, TNFSF14, TNFSF18, and CD27 (Figure 4D). In terms of genomic heterogeneity, we observed that the high-risk group had a higher tumor mutation burden (TMB) (Figure 4E). Further, after comparing the two groups of genes with high mutation frequencies we found that oncogene PIK3CA was relatively high mutated in the high-risk group and the classical tumor suppressor gene TP53 was mutated at a higher frequency in the low-risk group (Figures 4F, G).
Figure 4. Correlation of immune microenvironment with GRS risk model. (A) Thermogram displaying relationships between GRS risk groups (top 20% samples) and tumor immune microenvironment components based on CIBERSORT, XCELL, TIMER, and ESTIMATE analyses (Wilcoxon test). The representative images show the variations in pathological HE staining between the (B) high- and (C) low-risk groups. (D) Boxplot illustrating the association between GRS risk groups and the mRNA expression levels of various immune-related markers (Wilcoxon test). (E) Histogram depicting differences in tumor mutation burden (TMB) between risk groups (Wilcoxon test). Waterfall plots of genetic alterations in common mutant genes for the (F) high- and (G) low-risk groups in the SCANB cohort. *P < 0.05, **P < 0.01, ***P < 0.001.
Given that patients in the low-risk group had higher levels of immune cell infiltration, we hypothesized that they would be more sensitive to immunotherapy. The results of the Subclass Mapping (Submap) suggested that the expression patterns of patients in the low-risk group were similar to those of TNBC patients who responded to anti-PD-1 immunotherapy (Figure 5A). In addition, to further explore the predictive efficacy of GRS for anti-PD-L1 therapies, the GRS risk model was applied to the IMvigor210 cohort. Similar to previous results, we observed a high-risk score in immune desert type and IC0 (lowest immune cell score) type (Figures 5B, C). We also examined differences in risk scores among the TC groups (Figure 5D). The low-risk group also showed better immunotherapy response rates and better prognosis (Figures 5E–G). Overall, these results demonstrated that the low-risk group was more likely to benefit from immunotherapy.
Figure 5. Predictive value of GRS risk model for immunotherapy and identification of potential therapeutic agents. (A) Prediction of immunotherapy responses between two GRS risk groups in the SCANB cohort. (B) Histogram showing differences in GRS scores across immune phenotypes in the IMvigor210 cohort (Kruskal-Wallis test). (C, D) Histograms displaying variations in PD-L1 expression across immune cell (IC) and tumor cell (TC) subsets in the IMvigor210 cohort (Wilcoxon test). (E) Stacked histogram of anti-PD-L1 responsiveness between GRS risk groups in the IMvigor210 cohort. Kaplan-Meier survival curves for GRS risk groups in the IMvigor210 cohort (F) and Liu’s cohort (G). (H) Histogram of area under the curve (AUC) values between high- and low-risk groups from the GDSC dataset (Wilcoxon test). (I) Boxplot showing variations in IC50 values between risk groups from the GDSC dataset (Wilcoxon test). (J) Venn diagram showing compounds with significant differences in both AUC and IC50 values in the GDSC dataset. *P < 0.05, **P < 0.01, ***P < 0.001.
To explore the potential relationship between our GRS risk model and drug sensitivity, we analyzed half-maximal inhibitory concentration (IC50) values and AUC values for several drugs from the Genomics of Drug Sensitivity in Cancer (GDSC) database (Figures 5H, I). The results demonstrated that four compounds (including GS-9973, TRAMETINIB, PD-0325901, and REFAMETINIB) could be the potential therapeutic agents for the high-risk group (Figure 5J). Among them, TRAMETINIB, PD-0325901, and REFAMETINIB are all MEK inhibitors. Trametinib is the first FDA-approved MEK inhibitor. When taken alone or in combination with other treatments, MEK inhibitors have been shown to have good anti-tumor activity in melanoma, lung cancer, and colorectal cancer (42, 43). Thus, our study may provide guidance for the treatment of specific TNBC patient subgroups in the clinic.
We utilized RT-qPCR analysis to validate the differential mRNA expression of 21 model genes in TNBC cell lines (Figure 6A). Several genes were up-regulated in all six TNBC cell lines compared to MCF-10A, including LMAN1L, DERL3, CHST1, HS6ST3, FUT2, BCAN, VEGFB, FUT7, GCNT2, GALNT13, and NEU4. We also observed that the expression of two genes (LMAN1L and NEU4) was highest in the more malignant TNBC cell lines (LM2 and SUM159PT), suggesting that they may play a role in TNBC progression. MAN1A1, CHST7, BGN, GAS2, and SCGB1A1 were also up-regulated in most TNBC cell lines. But, the gene SRD5A3 showed a significant down-regulation in all TNBC cell lines. Additionally, we verified the expression of the model genes at the protein level between BC and adjacent normal tissues using the Human Protein Atlas (HPA) database (https://www.proteinatlas.org). The majority of genes showed higher staining in BC samples (Figure 6B).
Figure 6. Validation of mRNA and protein expression in GRS risk model. (A) The expression of 21 gene mRNA in TNBC cell lines experimented by RT-qPCR analysis: MAN1A1, LMAN1L, DERL3, CHST1, HS6ST3, FUT2, BCAN, XXYLT1, VEGFB, FUT7, ALG3, CHST7, BGN, MFNG, GCNT2, GALNT13, GAS2, B4GALNT4, SRD5A3, NEU4, and SCGB1A1. (B) Protein expression in breast cancer and normal tissues validated by the immunohistochemistry analysis of the HPA database. *P < 0.05, **P < 0.01, ***P < 0.001.
Among the top five genes ranked in the GRS risk model, MAN1A1 (44, 45), DERL3 (46), CHST1 (47), and HS6ST3 (48) have all been reported for their roles in BC, whereas the role of LMAN1L in various cancers, especially TNBC, has not been investigated. LMAN1L ranks second in importance, making a major contribution to the model. The qPCR results also demonstrate a substantial difference in its expression between TNBC cell lines and normal breast epithelial cells. Therefore, we performed a series of in vitro experiments to examine the role of LMAN1L in TNBC. First, we silenced LMAN1L expression in LM2 and SUM159PT cells, which have high levels of LMAN1L, using two distinct LMAN1L-targeting small interfering RNAs (siRNAs), siLMAN1L#1 and siLMAN1L#2 (Figure 7A). Subsequently, we assessed the overall levels of intracellular O-GlcNAcylation after LMAN1L knockdown in these cells and observed that LMAN1L likely inhibits O-GlcNAcylation (Figure 7B). Compared to the NC group we also observed that inhibition of LMAN1L expression significantly suppressed the proliferation of LM2 and SUM159PT (Figure 7C). The plate colony-forming assay and transwell migration assay brought similar results, finding that knockdown of LMAN1L resulted in a significant attenuation of clone formation and migration of TNBC cells (Figures 7D–I). Additionally, we carried out further validation by EdU experiments (Figures 7J–L). We also analyzed immune cell infiltration in tumor samples from TNBC patients with different LMAN1L expression levels and found that high LMAN1L expression correlated with reduced immune cell infiltration (Figure 7M). In summary, we found that LMAN1L being a glycosylation-related gene may play an important role in TNBC. All data were presented as means with standard deviations from three independent experiments.
Figure 7. LMAN1L promotes the malignant characteristics of TNBC. (A) RT-qPCR analyzing the knockdown efficiency of LMAN1L in LM2 cells and SUM159PT cells. (B) The level of overall intracellular O-GlcNAcylation in LM2 cells and SUM159PT cells following treatment with siRNA sequences (siNC, siLMAN1L#1, siLMAN1L#2). (C) Cell proliferation assays using CCK-8 in LM2 cells and SUM159PT cells with LMAN1L knockdown. (D) Representative images (scale bar 0.5cm) and quantification (E, F) of plate colony formation assay. (G) Representative images (scale bar 100μm) and quantification (H, I) of transwell migration assay in LM2 cells and SUM159PT cells with LMAN1L knockdown. (J) Representative images and quantification (K, L) of EdU assay in LM2 cells and SUM159PT cells with LMAN1L knockdown. EdU (red) and Hoechst 33342 (blue). (M) Representative images of immunofluorescence assay in TNBC patient samples grouped based on LMAN1L protein expression. LMAN1L (red), DAPI (blue), CD3 (white), CD19 (green), and CD68 (yellow). ***P < 0.001.
Glycosylation plays a critical role in tumor biology by influencing key processes such as cell adhesion, extracellular matrix interactions, and cell signaling. These processes are essential for tumor development, including invasion, angiogenesis, metastasis, and immune response regulation (49–51). In the tumor microenvironment (TME), abnormal glycosylation often contributes to immune evasion, leading to immune tolerance.
We observed significant differences in immune cell infiltration between high- and low-risk groups using the GRS risk model. Immune cell infiltration, including B cells, CD4+ naïve T cells, and CD8+ T cells, was higher in the low-risk group. Tumor-infiltrating lymphocytes (TILs) are increasingly recognized for their role in TNBC prognosis, and TME remodeling (recruitment of CD4+ T cells, CD8+ T cells, and NK cells) can enhance the efficacy of immunotherapy (52–55). These findings suggest that low-risk group patients may benefit more from immunotherapy. In the immunotherapy cohort, responders had lower risk scores, likely due to more effective immune responses, reinforcing that the GRS risk model can assess the tumor immune microenvironment and identify glycosylation as a potential biomarker for TNBC.
Our study also highlights the role of glycosylation-related genes in TNBC. MAN1A1, upregulated in most TNBC cell lines, participates in the maturation of Asn-linked oligosaccharides. Low expression of MAN1A1 often leads to abnormal N-glycosylation and is associated with cell adhesion and brain metastasis in BC (44). DERL3, another gene highly expressed in TNBC cell lines, targets misfolded glycoproteins in the ER and is associated with lymph node metastasis (56) and poor prognosis (46). The expression of sulfotransferase CHST1 is related to the sensitivity of Siglec ligands to carbohydrate sulfation inhibition, and its high expression is generally associated with poor prognosis in specific cancers (47). Notably, we identified LMAN1L, a glycosylation-related gene previously unreported in BC, which plays a role in TNBC malignancy. LMAN1L is a type I transmembrane protein involved in transporting glycoproteins from the ER to the Golgi apparatus. Our results show that inhibiting LMAN1L suppresses TNBC cell proliferation, clonogenicity, and migration, and modulates the immune microenvironment. High expression of LMAN1L is also associated with poor prognosis in pancreatic cancer (57). These findings suggest that LMAN1L may contribute significantly to TNBC progression, though further studies are needed to elucidate its precise mechanisms.
While our study provides valuable insights, several limitations must be addressed. TNBC is highly heterogeneous, with diverse histological phenotypes, necessitating more comprehensive mechanistic and clinical research on the roles of glycosylation-related genes in various TNBC subtypes. Although RT-qPCR confirmed differential mRNA expression of model genes in TNBC cell lines, protein expression remains unvalidated. Further investigation into the molecular mechanisms of glycosylation-related genes in TNBC is essential to fully understand their impact on tumor biology and treatment responses.
We developed and validated a glycosylation-related risk model for prognostic stratification in TNBC patients. This model effectively predicts clinical outcomes and immunotherapy responses. Future research should explore the mechanisms of glycosylation-related genes in TNBC, potentially guiding novel therapeutic strategies.
The original contributions presented in the study are included in the article/Supplementary Material. Further inquiries can be directed to the corresponding authors.
Ethical approval was not required for the studies on humans in accordance with the local legislation and institutional requirements because only commercially available established cell lines were used.
QY: Formal Analysis, Validation, Writing – original draft, Writing – review & editing. HYZ: Data curation, Methodology, Software, Writing – original draft. XHZ: Investigation, Writing – review & editing. CL: Writing – review & editing. XZ: Writing – review & editing. JW: Writing – review & editing. ZL: Writing – review & editing. SS: Writing – review & editing. HRZ: Writing – review & editing. CZ: Writing – review & editing. QZ: Writing – review & editing, Conceptualization, Funding acquisition.
The author(s) declare that financial support was received for the research, authorship, and/or publication of this article. This work was supported by grants from the Ministry of Science and Technology of China (2020YFA0803403), the National Natural Science Foundation of China (81772831) and Natural Science Foundation of Shanghai (24ZR1442100). This work was also supported by Shanghai Frontiers Science Center of Cellular Homeostasis and Human Diseases.
We thank Yanfei Shao, Xiaodong Fan, and Chaozhao Chen, Department of General Surgery, Ruijin Hospital, Shanghai Jiao Tong University School of Medicine for technical support of bioinformatics analysis.
The authors declare that the research was conducted in the absence of any commercial or financial relationships that could be construed as a potential conflict of interest.
The author(s) declare that no Generative AI was used in the creation of this manuscript.
All claims expressed in this article are solely those of the authors and do not necessarily represent those of their affiliated organizations, or those of the publisher, the editors and the reviewers. Any product that may be evaluated in this article, or claim that may be made by its manufacturer, is not guaranteed or endorsed by the publisher.
The Supplementary Material for this article can be found online at: https://www.frontiersin.org/articles/10.3389/fimmu.2024.1521930/full#supplementary-material
AUC, Areas Under the Curve; BC, Breast Cancer; CCLE, Cancer Cell Line Encyclopedia; DEG, Differentially Expressed Gene; EdU, Ethynyl-2’-deoxyuridine; ER, Estrogen Receptors; GDSC, Genomics of Drug Sensitivity in Cancer; GEO, Gene Expression Omnibus; GRS, Glycosylation-related Signature; GO, Gene Ontology; GSEA, Gene Set Enrichment Analysis; HR, Hazard Ratio; HER2, Human Epidermal Growth Factor Receptor 2; ICB, Immune Checkpoint Blockade; KEGG, Kyoto Encyclopedia of Genes and Genomes; LASSO, Least Absolute Shrinkage and Selection Operator; LMAN1L, Lectin Mannose-Binding 1-Like; LOOCV, Leave-one-out Cross-validation; MHC, Major Histocompatibility Complex; OS, Overall Survival; PR, Progesterone Receptors; PD, Progressive Disease; CR, Complete Response; PR, Partial Response; PRG, Prognosis-related Gene; RNA-seq, RNA Sequencing; RSF, Random Survival Forest; SD, Stable Disease; TCGA, The Cancer Genome Atlas; TIL, Tumor-infiltrating Lymphocyte; TIMER, Tumor Immune Estimation Resource; TMB, Tumor Mutation Burden; TME, Tumor Microenvironment; TNBC, Triple-Negative Breast Cancer; TPM, Transcripts Per Million.
1. Siegel RL, Giaquinto AN, Jemal A. Cancer statistics, 2024. CA Cancer J Clin. (2024) 74:12–49. doi: 10.3322/caac.21820
2. Brown M, Tsodikov A, Bauer KR, Parise CA, Caggiano V. The role of human epidermal growth factor receptor 2 in the survival of women with estrogen and progesterone receptor-negative, invasive breast cancer: the california cancer registry, 1999-2004. Cancer. (2008) 112:737–47. doi: 10.1002/cncr.23243
3. Lin NU, Vanderplas A, Hughes ME, Theriault RL, Edge SB, Wong YN, et al. Clinicopathologic features, patterns of recurrence, and survival among women with triple-negative breast cancer in the national comprehensive cancer network. Cancer. (2012) 118:5463–72. doi: 10.1002/cncr.27581
4. Spring LM, Nakajima E, Hutchinson J, Viscosi E, Blouin G, Weekes C, et al. Sacituzumab govitecan for metastatic triple-negative breast cancer: clinical overview and management of potential toxicities. Oncologist. (2021) 26:827–34. doi: 10.1002/onco.13878
5. Cortes J, Rugo HS, Cescon DW, Im SA, Yusof MM, Gallardo C, et al. Pembrolizumab plus chemotherapy in advanced triple-negative breast cancer. N Engl J Med. (2022) 387:217–26. doi: 10.1056/NEJMoa2202809
6. Ohtsubo K, Marth JD. Glycosylation in cellular mechanisms of health and disease. Cell. (2006) 126:855–67. doi: 10.1016/j.cell.2006.08.019
7. He M, Zhou X, Wang X. Glycosylation: mechanisms, biological functions and clinical implications. Signal Transduct Target Ther. (2024) 9:194. doi: 10.1038/s41392-024-01886-1
8. Xu C, Ng DT. Glycosylation-directed quality control of protein folding. Nat Rev Mol Cell Biol. (2015) 16:742–52. doi: 10.1038/nrm4073
9. Pinho SS, Reis CA. Glycosylation in cancer: mechanisms and clinical implications. Nat Rev Cancer. (2015) 15:540–55. doi: 10.1038/nrc3982
10. Marsico G, Russo L, Quondamatteo F, Pandit A. Glycosylation and integrin regulation in cancer. Trends Cancer. (2018) 4:537–52. doi: 10.1016/j.trecan.2018.05.009
11. Stowell SR, Ju T, Cummings RD. Protein glycosylation in cancer. Annu Rev Pathol. (2015) 10:473–510. doi: 10.1146/annurev-pathol-012414-040438
12. Oliveira-Ferrer L, Legler K, Milde-Langosch K. Role of protein glycosylation in cancer metastasis. Semin Cancer Biol. (2017) 44:141–52. doi: 10.1016/j.semcancer.2017.03.002
13. Singh P, Joon A, Kumari M, Singh T, Bal A, Maan P, et al. Role of a disease-associated st3gal-4 in non-small cell lung cancer. Cell Biochem Biophys. (2022) 80:781–93. doi: 10.1007/s12013-022-01091-3
14. Caval T, Alisson-Silva F, Schwarz F. Roles of glycosylation at the cancer cell surface: opportunities for large scale glycoproteomics. Theranostics. (2023) 13:2605–15. doi: 10.7150/thno.81760
15. Gupta R, Ponangi R, Indresh KG. Role of glycosylation in breast cancer progression and metastasis: implications for mirna, emt and multidrug resistance. Glycobiology. (2023) 33:545–55. doi: 10.1093/glycob/cwad046
16. Chakraborty M, Kaur J, Gunjan, Kathpalia M, Kaur N. Clinical relevance of glycosylation in triple negative breast cancer: A review. Glycoconj J. (2024) 41:79–91. doi: 10.1007/s10719-024-10151-0
17. Singh K, Agrawal L, Gupta R, Singh D, Kathpalia M, Kaur N. Lectins as a promising therapeutic agent for breast cancer: A review. Breast Dis. (2024) 43:193–211. doi: 10.3233/BD-230047
18. Yang M, Li Y, Kong L, Huang S, He L, Liu P, et al. Inhibition of dpagt1 suppresses her2 shedding and trastuzumab resistance in human breast cancer. J Clin Invest. (2023) 133:e164428. doi: 10.1172/JCI164428
19. Tominaga N, Hagiwara K, Kosaka N, Honma K, Nakagama H, Ochiya T. Rpn2-mediated glycosylation of tetraspanin cd63 regulates breast cancer cell Malignancy. Mol Cancer. (2014) 13:134. doi: 10.1186/1476-4598-13-134
20. Staaf J, Hakkinen J, Hegardt C, Saal LH, Kimbung S, Hedenfalk I, et al. Rna sequencing-based single sample predictors of molecular subtype and risk of recurrence for clinical assessment of early-stage breast cancer. NPJ Breast Cancer. (2022) 8:94. doi: 10.1038/s41523-022-00465-3
21. Saal LH, Vallon-Christersson J, Hakkinen J, Hegardt C, Grabau D, Winter C, et al. The Sweden cancerome analysis network - breast (Scan-B) initiative: A large-scale multicenter infrastructure towards implementation of breast cancer genomic analyses in the clinical routine. Genome Med. (2015) 7:20. doi: 10.1186/s13073-015-0131-9
22. Cancer Genome Atlas N. Comprehensive molecular portraits of human breast tumours. Nature. (2012) 490:61–70. doi: 10.1038/nature11412
23. Jezequel P, Loussouarn D, Guerin-Charbonnel C, Campion L, Vanier A, Gouraud W, et al. Gene-expression molecular subtyping of triple-negative breast cancer tumours: importance of immune response. Breast Cancer Res. (2015) 17:43. doi: 10.1186/s13058-015-0550-y
24. Jezequel P, Kerdraon O, Hondermarck H, Guerin-Charbonnel C, Lasla H, Gouraud W, et al. Identification of three subtypes of triple-negative breast cancer with potential therapeutic implications. Breast Cancer Res. (2019) 21:65. doi: 10.1186/s13058-019-1148-6
25. Barretina J, Caponigro G, Stransky N, Venkatesan K, Margolin AA, Kim S, et al. The cancer cell line encyclopedia enables predictive modelling of anticancer drug sensitivity. Nature. (2012) 483:603–7. doi: 10.1038/nature11003
26. Love MI, Huber W, Anders S. Moderated estimation of fold change and dispersion for rna-seq data with deseq2. Genome Biol. (2014) 15:550. doi: 10.1186/s13059-014-0550-8
27. Shi Y, Wang Y, Yang R, Zhang W, Zhang Y, Feng K, et al. Glycosylation-related molecular subtypes and risk score of hepatocellular carcinoma: novel insights to clinical decision-making. Front Endocrinol (Lausanne). (2022) 13:1090324. doi: 10.3389/fendo.2022.1090324
28. Xu S, Hu E, Cai Y, Xie Z, Luo X, Zhan L, et al. Using clusterprofiler to characterize multiomics data. Nat Protoc. (2024) 19:3292–320. doi: 10.1038/s41596-024-01020-z
29. Ritchie ME, Phipson B, Wu D, Hu Y, Law CW, Shi W, et al. Limma powers differential expression analyses for rna-sequencing and microarray studies. Nucleic Acids Res. (2015) 43:e47. doi: 10.1093/nar/gkv007
30. Brueffer C, Gladchuk S, Winter C, Vallon-Christersson J, Hegardt C, Hakkinen J, et al. The mutational landscape of the scan-B real-world primary breast cancer transcriptome. EMBO Mol Med. (2020) 12:e12118. doi: 10.15252/emmm.202012118
31. Mayakonda A, Lin DC, Assenov Y, Plass C, Koeffler HP. Maftools: efficient and comprehensive analysis of somatic variants in cancer. Genome Res. (2018) 28:1747–56. doi: 10.1101/gr.239244.118
32. Newman AM, Liu CL, Green MR, Gentles AJ, Feng W, Xu Y, et al. Robust enumeration of cell subsets from tissue expression profiles. Nat Methods. (2015) 12:453–7. doi: 10.1038/nmeth.3337
33. Aran D, Hu Z, Butte AJ. Xcell: digitally portraying the tissue cellular heterogeneity landscape. Genome Biol. (2017) 18:220. doi: 10.1186/s13059-017-1349-1
34. Li T, Fan J, Wang B, Traugh N, Chen Q, Liu JS, et al. Timer: A web server for comprehensive analysis of tumor-infiltrating immune cells. Cancer Res. (2017) 77:e108–e10. doi: 10.1158/0008-5472.CAN-17-0307
35. Yoshihara K, Shahmoradgoli M, Martinez E, Vegesna R, Kim H, Torres-Garcia W, et al. Inferring tumour purity and stromal and immune cell admixture from expression data. Nat Commun. (2013) 4:2612. doi: 10.1038/ncomms3612
36. Charoentong P, Finotello F, Angelova M, Mayer C, Efremova M, Rieder D, et al. Pan-cancer immunogenomic analyses reveal genotype-immunophenotype relationships and predictors of response to checkpoint blockade. Cell Rep. (2017) 18:248–62. doi: 10.1016/j.celrep.2016.12.019
37. Riaz N, Havel JJ, Makarov V, Desrichard A, Urba WJ, Sims JS, et al. Tumor and microenvironment evolution during immunotherapy with nivolumab. Cell. (2017) 171:934–49 e16. doi: 10.1016/j.cell.2017.09.028
38. Liu D, Schilling B, Liu D, Sucker A, Livingstone E, Jerby-Arnon L, et al. Integrative molecular and clinical modeling of clinical outcomes to pd1 blockade in patients with metastatic melanoma. Nat Med. (2019) 25:1916–27. doi: 10.1038/s41591-019-0654-5
39. Ghandi M, Huang FW, Jane-Valbuena J, Kryukov GV, Lo CC, McDonald ER 3rd, et al. Next-generation characterization of the cancer cell line encyclopedia. Nature. (2019) 569:503–8. doi: 10.1038/s41586-019-1186-3
40. Yang W, Soares J, Greninger P, Edelman EJ, Lightfoot H, Forbes S, et al. Genomics of drug sensitivity in cancer (Gdsc): A resource for therapeutic biomarker discovery in cancer cells. Nucleic Acids Res. (2013) 41:D955–61. doi: 10.1093/nar/gks1111
41. Zhou C, Wang D, Li J, Wang Q, Wo L, Zhang X, et al. Tgfb2-as1 inhibits triple-negative breast cancer progression via interaction with smarca4 and regulating its targets tgfb2 and sox2. Proc Natl Acad Sci U S A. (2022) 119:e2117988119. doi: 10.1073/pnas.2117988119
42. Ram T, Singh AK, Kumar A, Singh H, Pathak P, Grishina M, et al. Mek inhibitors in cancer treatment: structural insights, regulation, recent advances and future perspectives. RSC Med Chem. (2023) 14:1837–57. doi: 10.1039/d3md00145h
43. Akinleye A, Furqan M, Mukhi N, Ravella P, Liu D. Mek and the inhibitors: from bench to bedside. J Hematol Oncol. (2013) 6:27. doi: 10.1186/1756-8722-6-27
44. Legler K, Rosprim R, Karius T, Eylmann K, Rossberg M, Wirtz RM, et al. Reduced mannosidase man1a1 expression leads to aberrant N-glycosylation and impaired survival in breast cancer. Br J Cancer. (2018) 118:847–56. doi: 10.1038/bjc.2017.472
45. Rathod M, Chatterjee S, Dutta S, Kalraiya R, Bhattacharyya D, De A. Mannose glycosylation is an integral step for nis localization and function in human breast cancer cells. J Cell Sci. (2019) 132:jcs232058. doi: 10.1242/jcs.232058
46. Shibata M, Kanda M, Tanaka H, Umeda S, Miwa T, Shimizu D, et al. Overexpression of derlin 3 is associated with Malignant phenotype of breast cancer cells. Oncol Rep. (2017) 38:1760–6. doi: 10.3892/or.2017.5800
47. Jung J, Enterina JR, Bui DT, Mozaneh F, Lin PH, Nitin, et al. Carbohydrate sulfation as a mechanism for fine-tuning siglec ligands. ACS Chem Biol. (2021) 16:2673–89. doi: 10.1021/acschembio.1c00501
48. Iravani O, Bay BH, Yip GW. Silencing hs6st3 inhibits growth and progression of breast cancer cells through suppressing igf1r and inducing xaf1. Exp Cell Res. (2017) 350:380–9. doi: 10.1016/j.yexcr.2016.12.019
49. Lau KS, Partridge EA, Grigorian A, Silvescu CI, Reinhold VN, Demetriou M, et al. Complex N-glycan number and degree of branching cooperate to regulate cell proliferation and differentiation. Cell. (2007) 129:123–34. doi: 10.1016/j.cell.2007.01.049
50. RodrIguez E, Schetters STT, van Kooyk Y. The tumour glyco-code as a novel immune checkpoint for immunotherapy. Nat Rev Immunol. (2018) 18:204–11. doi: 10.1038/nri.2018.3
51. Mereiter S, Balmana M, Campos D, Gomes J, Reis CA. Glycosylation in the era of cancer-targeted therapy: where are we heading? Cancer Cell. (2019) 36:6–16. doi: 10.1016/j.ccell.2019.06.006
52. Liu Y, Hu Y, Xue J, Li J, Yi J, Bu J, et al. Advances in immunotherapy for triple-negative breast cancer. Mol Cancer. (2023) 22:145. doi: 10.1186/s12943-023-01850-7
53. Karn T, Denkert C, Weber KE, Holtrich U, Hanusch C, Sinn BV, et al. Tumor mutational burden and immune infiltration as independent predictors of response to neoadjuvant immune checkpoint inhibition in early tnbc in geparnuevo. Ann Oncol. (2020) 31:1216–22. doi: 10.1016/j.annonc.2020.05.015
54. Gianni L, Huang CS, Egle D, Bermejo B, Zamagni C, Thill M, et al. Pathologic complete response (Pcr) to neoadjuvant treatment with or without atezolizumab in triple-negative, early high-risk and locally advanced breast cancer: neotrip michelangelo randomized study. Ann Oncol. (2022) 33:534–43. doi: 10.1016/j.annonc.2022.02.004
55. Schmid P, Salgado R, Park YH, Munoz-Couselo E, Kim SB, Sohn J, et al. Pembrolizumab plus chemotherapy as neoadjuvant treatment of high-risk, early-stage triple-negative breast cancer: results from the phase 1b open-label, multicohort keynote-173 study. Ann Oncol. (2020) 31:569–81. doi: 10.1016/j.annonc.2020.01.072
56. Lin L, Lin G, Lin H, Chen L, Chen X, Lin Q, et al. Integrated profiling of endoplasmic reticulum stress-related derl3 in the prognostic and immune features of lung adenocarcinoma. Front Immunol. (2022) 13:906420. doi: 10.3389/fimmu.2022.906420
Keywords: TNBC, glycosylation, machine learning, prognosis, tumor immune microenvironment
Citation: Yu Q, Zhong H, Zhu X, Liu C, Zhang X, Wang J, Li Z, Shi S, Zhao H, Zhou C and Zhao Q (2025) Glycosylation profiling of triple-negative breast cancer: clinical and immune correlations and identification of LMAN1L as a biomarker and therapeutic target. Front. Immunol. 15:1521930. doi: 10.3389/fimmu.2024.1521930
Received: 03 November 2024; Accepted: 27 December 2024;
Published: 10 January 2025.
Edited by:
Dan Liu, Wuhan University, ChinaReviewed by:
Navkiran Kaur, Amity University, IndiaCopyright © 2025 Yu, Zhong, Zhu, Liu, Zhang, Wang, Li, Shi, Zhao, Zhou and Zhao. This is an open-access article distributed under the terms of the Creative Commons Attribution License (CC BY). The use, distribution or reproduction in other forums is permitted, provided the original author(s) and the copyright owner(s) are credited and that the original publication in this journal is cited, in accordance with accepted academic practice. No use, distribution or reproduction is permitted which does not comply with these terms.
*Correspondence: Cixiang Zhou, emhvdWN4QHNoc211LmVkdS5jbg==; Qian Zhao, cXpoYW9Ac2hzbXUuZWR1LmNu
†These authors have contributed equally to this work and share first authorship
Disclaimer: All claims expressed in this article are solely those of the authors and do not necessarily represent those of their affiliated organizations, or those of the publisher, the editors and the reviewers. Any product that may be evaluated in this article or claim that may be made by its manufacturer is not guaranteed or endorsed by the publisher.
Research integrity at Frontiers
Learn more about the work of our research integrity team to safeguard the quality of each article we publish.