- 1Center for Translational Science, Florida International University, Port Saint Lucie, FL, United States
- 2Department of Cellular and Molecular Medicine, Florida International University, Miami, FL, United States
- 3Department of Environmental Health Sciences, Florida International University, Miami, FL, United States
Sepsis is a severe and life-threatening medical syndrome that can lead to organ failure and death. Despite advances in medical treatment, current therapies are often inadequate, with high septic mortality rates. Therefore, there is a critical need for reliable prognostic markers to be used in clinical settings to improve the management and outcomes of patients with sepsis. Recent studies have suggested that mitochondrial dynamics, including the processes of mitochondrial fission and fusion, are closely related to the severity of sepsis and the status of inflammation. By monitoring transcriptomic signals related to mitochondrial dynamics, new and reliable biomarkers can be engineered to more accurately predict sepsis survival risk. Such biomarkers would be invaluable in clinical settings, aiding healthcare providers in the early identification of high-risk patients and improving treatment strategies. To achieve this goal, we utilized the major mitochondrial fission regulatory protein dynamin-related protein 1 (Drp1, gene code DNM1L) and identified Drp1-associated genes that are enriched with sepsis survival genes. A 12-gene signature (GS) was established as a differentially expressed gene (DEG)-based GS. Next, we compared genes of proteins that interact with Drp1 to sepsis survival genes and identified 7 common genes, establishing a GS we term as protein-protein interaction (PPI)-based GS. To evaluate if these GSs can predict sepsis survival, we used publicly available human blood transcriptomic datasets from sepsis patients. We confirmed that both GSs can successfully predict sepsis survival in both discovery and validation cohorts with high sensitivity and specificity, with the PPI-based GS showing enhanced prognostic performance. Together, this study successfully engineers a new and validated blood-borne biomarker (PPI-based 7-gene GS) for sepsis survival risk prediction. This biomarker holds the potential for improving the early identification of high-risk sepsis patients and optimizing personalized treatment strategies to reduce sepsis mortality.
1 Introduction
Sepsis is a deadly syndrome comprised of many dysregulated pathways in response to infection or injury, ultimately leading to organ dysfunction and damage (1). Sepsis remains a major public health concern due to its high incidence, mortality rate, and strain on the hospital care system (2). The mortality rate is estimated to be 41.9% for intensive care unit (ICU) septic patients (3). Unfortunately, increasing sepsis survival is not the only hurdle in treating sepsis; but quality of life after sepsis is a major concern (4–6). It is estimated that nearly 75% of sepsis survivors have deficits in at least one activity of daily life (7). Currently, the only treatment for sepsis is the administration of intravenous broad-spectrum antibiotics, with the ideal administration within an hour of sepsis recognition (8). Sepsis survival drops 7.6% every hour that administration of antibiotics is delayed (9). This short window for antibiotic administration means early detection of sepsis is critical.
Sepsis treatment includes supportive care, which involves the initiation of vasopressors and aggressive fluid therapy (10, 11). However, supportive care only offers limited/modest success (12, 13). This means that the only proven treatment to reduce sepsis mortalities is early detection and immediate administration of antibiotics. Unfortunately, there are no reliable biomarkers or metrics that have been proven to detect sepsis early. Therefore, the only recourse for clinicians is to perform repeated blood cultures to monitor serum lactic acid levels and blood pressure in the hope of identifying sepsis or septic shock cases early (8), causing significant strain on hospital resources. Septic shock is the most severe form of sepsis and patients with septic shock have dire metabolic and cellular abnormalities that are associated with higher mortality rates than sepsis alone (1).
Historically, sepsis progression has been divided into two inflammatory stages. Originally, the first stage was believed to be a pro-inflammatory stage where a cytokine storm induces a profound and intense immune response, followed by a compensatory, anti-inflammatory stage. Early investigations targeted the presumed first, pro-inflammatory stage and evaluated anti-inflammatory treatments. These strategies were unsuccessful in clinical trials (14–16). Newer evidence suggests that sepsis is not biphasic, with a distinct pro-then anti-inflammatory stage, but rather occurs simultaneously (17). This is likely due to the complexity of immune responses, where pathways constantly interact and modulate one another (18). Immunomodulation has been the predominant target tested in sepsis clinical trials. Despite over 100 randomized clinical trials testing immunomodulation, they have failed to yield even one successful drug or strategy (19). This failure likely stems from the cross-talk of the immune response; thus, simply augmenting or inhibiting specific immunomodulators does not have the intended effect as the system responds to this modification. Although further testing is needed to verify why immunomodulation has failed to clinically demonstrate efficacy, the overwhelming failure of immunomodulatory clinical trials emphasizes that this is not a valid strategy for sepsis treatment; therefore, research effort needs to be allocated to other approaches.
Sepsis is known to be the cumulation of multiple dysregulated pathways; therefore, it is critical to identify therapeutics that target multiple pathways (20, 21). Mitochondria are known to play critical roles in a multitude of pathways involved in maintaining homeostasis (22, 23), suggesting that targeting mitochondria may modulate multiple aberrant pathways associated with sepsis progression. For example, mitochondria supply the energy and metabolic intermediates that are needed for immune cell activation and function and influence inflammatory and cell death pathways (24). Dysfunctional mitochondria are well documented in sepsis (25–28), although modulation of mitochondria remains primarily untested. Of importance, mitochondrial dysfunction is evident during the early stages of sepsis and is believed to play a critical role in the initiation of organ damage (29).
Mitochondria are highly dynamic organelles that exist in networks rather than individually. Mitochondrial networks can modulate their function by combining (fusion) or separating (fission). The cell contains a multitude of mitochondrial networks that are constantly performing fusion or fission to modulate their function to adapt to cellular demands, a process referred to as mitochondrial dynamics (23, 30). Since patients with the highest mortality rates have pronounced metabolic and cellular abnormalities (1), it is likely that these patients also exhibit greater mitochondrial dysfunction, although tests are needed to evaluate this postulation. For that reason, we hypothesize that altered mitochondrial function plays a critical role in regulating immune and cell responses, which lead to more severe cases of sepsis and may present an opportunity for earlier diagnosis. Because of the close relationship between mitochondrial dynamics and overall mitochondrial function, our study evaluated dynamin-related protein 1 (Drp1), which is recognized as one of the major regulators of fission and is one of the most studied mitochondrial network mediators (31). Therefore, the aim of this study was first to evaluate the expression of genes related to mitochondrial dynamics to determine if there is a relationship between these genes and sepsis survival. Moreover, we aim to establish a new circulating (Drp1-related) gene signature to predict sepsis survival and validate it as a powerful and independent prognostic tool for further clinical application.
2 Materials and methods
2.1 Microarray datasets and sepsis survival-related genes
We previously have identified genes that are differentially expressed between patients with a low or high risk of sepsis-related death (32). These genes were identified using two human peripheral blood mononuclear cell datasets from ArrayExpress datasets, E-MTAB-4421 and E-MTAB-4451. Samples from these datasets were collected from patients at 4 weeks after intensive care unit admission. Discovery cohort E-MTAB-4421 includes 187 sepsis survivors and 78 non-survivors, with a male-to-female ratio of 145:120 and a mean age of 62 ± 16 years. Validation cohort E-MTAB-4451 comprises 50 sepsis survivors and 56 non-survivors, with a male-to-female ratio of 79:27 and a mean age of 69 ± 14 years. Differentially expressed genes (DEGs) were only considered significant if the fold change was greater than 1.5 and the false discovery rate (FDR) was less than 5%. Here, we use E-MTAB-4421 as the discovery cohort and E-MTAB-4451 as the validation cohort to test this Drp1-gene signature.
2.2 Sepsis survival score
To generate a practical sepsis survival score, we used a linear combination of gene expression values and their corresponding weight values in the Drp1-associated gene signature. The sepsis survival score is as follows:
Wherein n is the number of genes in the Drp1-associated gene signature for each dataset, Wi shows the weighted value of each gene, ei is the expression level of each gene, and μi and Si are the mean and standard deviation values, respectively, for the corresponding gene compared the whole sample. Scores above the mean sepsis survival score were classified into the high sepsis survival score cohort, while the values below the mean were classified into the low sepsis survival score cohort.
2.3 Enrichment analysis
To generate a list of Drp1-related genes, we first identified genes that significantly changed during Drp1 upregulation using the Gene Expression Omnibus (GEO) dataset GSE182710, referred to as differentially expressed genes (DEGs). To be classified as a DEG, the genes met the following criteria: fold change > 1.5, FRD < 0.05. Here, we refer to these genes as the DEGs-based Drp1-associated genes. Next, we performed a protein-protein interaction (PPI) analysis of the protein, Drp1, to identify proteins that are predicted to interact with Drp1 using the STRING database. The STRING database identifies functional protein association networks. In our analysis, we only used the primary protein interactors (also known as 1st shell interactors), which are proteins that are directly associated with Drp1. Proteins with interaction scores greater than 0.7 were selected and henceforth referred to as PPI-based Drp1-associated genes. The protein network was visualized by Cytoscape (3.10.2).
The two Drp1-associated gene signatures (GS) were obtained by identifying the common genes between sepsis survival DEGs and the two Drp1-associated genes described above (DEGs-based and PPI-based, Figure 1). This enrichment resulted in a 12-gene DEG-based GS and 7-gene PPI-based GS.
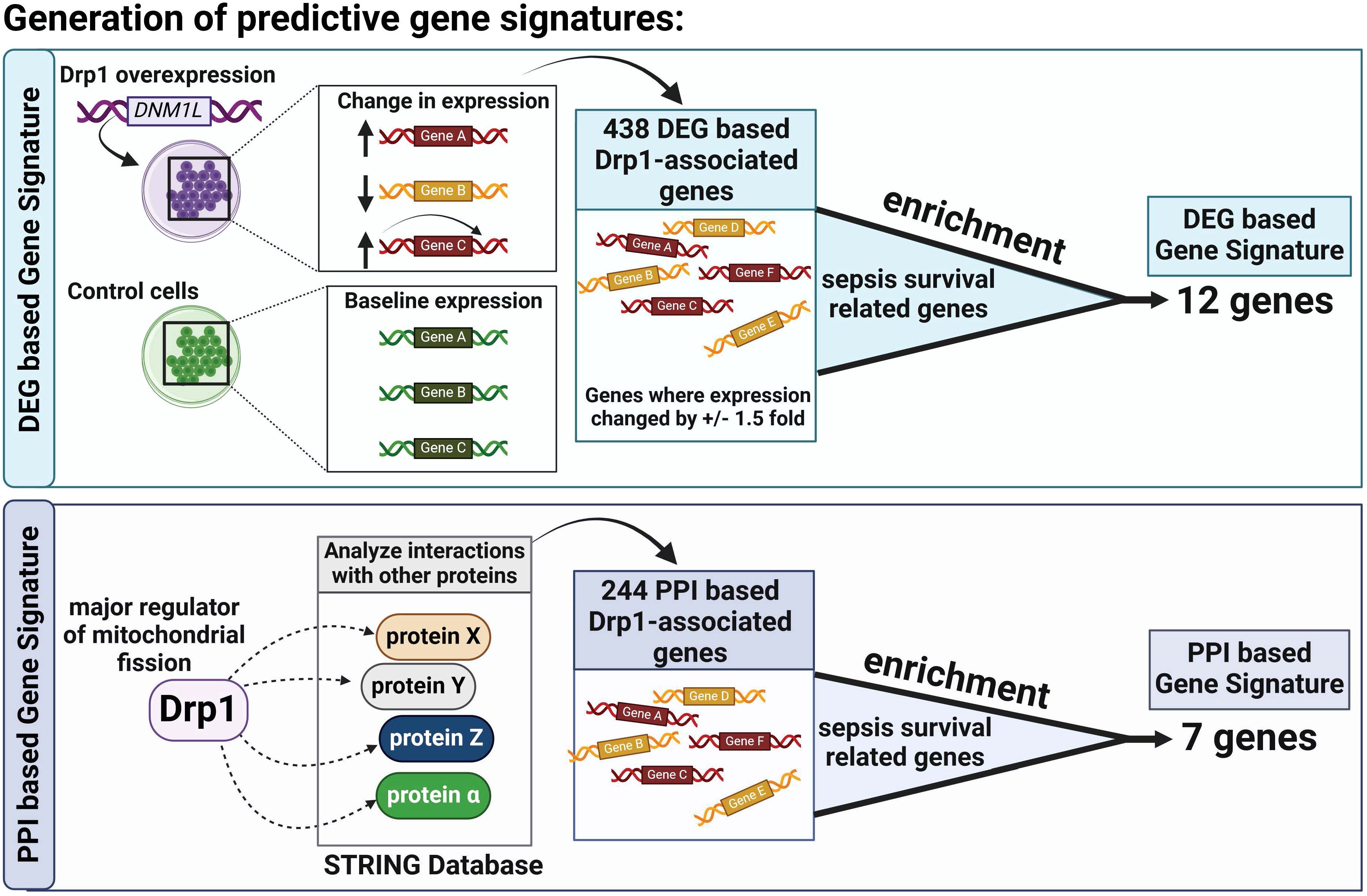
Figure 1. Overview of Gene Signature (GS) Generation. First, we compared Drp1-associated genes to sepsis survival genes and identified 12 common genes, establishing a GS we term as DEG-based GS (A). Next, we compared genes of proteins that interact with Drp1 to sepsis survival genes and identified 7 common genes, establishing a GS we term as PPI-based GS (B). Drp1, Dynamin-related protein 1; DEGs, differentially expressed genes; PPI, protein-protein interaction; GS, gene signature.
Next, we performed Kyoto Encyclopedia of Genes and Genomes (KEGG) analysis of the generated Drp1-associated genes using the database for annotation, visualization, and integrated discovery (DAVID, version 6.8, https://david.ncifcrf.gov/tools.jsp). DAVID is a biological and functional annotation database that aids in the interpretation of gene/signaling pathway interactions. We repeated KEGG analysis for the sepsis-survival genes. We excluded irrelevant pathways, such as diseases that are not associated with sepsis. Adjusted p-values less than 0.05 were considered to be significant.
To validate the accuracy of our PPI analysis, we generated correlation matrixes for both GS using the R package “corrplot” (version 0.92). The genomic annotation file for humans (Genome assembly GRCh37) was obtained from the UCSC Genome Browser. The circular plot was generated by the R package “circlize” (version 0.4.10). All sepsis survival-related genes were visualized as dots, and genes in two gene signatures were marked in the inner circle.
2.4 Evaluating prognostic capabilities of GS
To validate that these GSs have the prognostic capability of distinguishing between high and low sepsis risk groups, we performed a variety of assessments. First, we generated gene expression heatmaps of both GS and grouped patients by their sepsis-risk group. The heatmaps were visualized using the R package “gplots” (version 3.1.3.1). The method “complete” was used for hierarchical clustering, and the method “euclidean” was utilized for distance calculation. The survival scores for each GS were calculated and then plotted in violin plots using the R package “ggplot2” (version 3.5.1). The ROC curves were generated by R package “pROC” (version 1.18.5), and the AUC values were calculated by the function “auc”.
2.5 Running enrichment score
To determine the running enrichment score, the gene expression matrix of the discovery cohort and gene sets derived from the KEGG pathway were used as input for gene set enrichment analysis (GSEA). The analysis was performed using the default parameters in R package GSEA_R (version 1.2) and adjusted p-values less than 0.25 were considered to be significant. An unsupervised method called Gene Set Variation Analysis (GSVA) by the R package GSVA (version 3.18) was also performed on the discovery cohort and gene sets derived from the KEGG pathway to estimate the variation of KEGG gene sets enrichment across the samples.
2.6 CIBERSORT
We estimated the immune cell proportions in the sepsis datasets and evaluated if there were different immune cell proportions between the low- and high-score cohorts generated from the Drp1-PPI GS. We used CIBERSORT (version 1.04) (33) to assess the proportions of immune cell populations in the discovery dataset.
2.7 Statistical analysis
The R packages, ade4 (Version: 1.7-22) (34) and pROC (Version: 1.18.5) (35) were used to generate the PCA plots and the ROC curves. Values with false discovery rates (FDR) less than 0.05 were considered to be significant.
3 Results
First, we sought to identify genes related to Drp1 protein expression. To accomplish this, we used the publicly available GEO dataset containing the gene expression data from human cells where Drp1 was overexpressed and from control cells (GSE182710). With this, we identified 438 genes differentially expressed (DEGs) after Drp1 overexpression, henceforth referred to as the DEG-based Drp1-associated genes (Supplementary Table 1). Next, we performed a protein-protein interaction (PPI) analysis of the protein, Drp1, to identify proteins predicted to interact with Drp1 using the STRING database. This method resulted in an additional 244 genes associated with Drp1, designated as PPI-based Drp1-associated genes (Supplementary Table 2). We then enriched these two sets of Drp1-associated genes with genes related to sepsis survival. This revealed 12 and 7 common genes between the sepsis survival genes and the DEG- or PPI-based Drp1-associated gene sets, respectively (Figures 2A, B, Table 1). We designated the 12 and 7 gene sets as DEGs and PPI Drp1-gene signatures (GS), respectively.
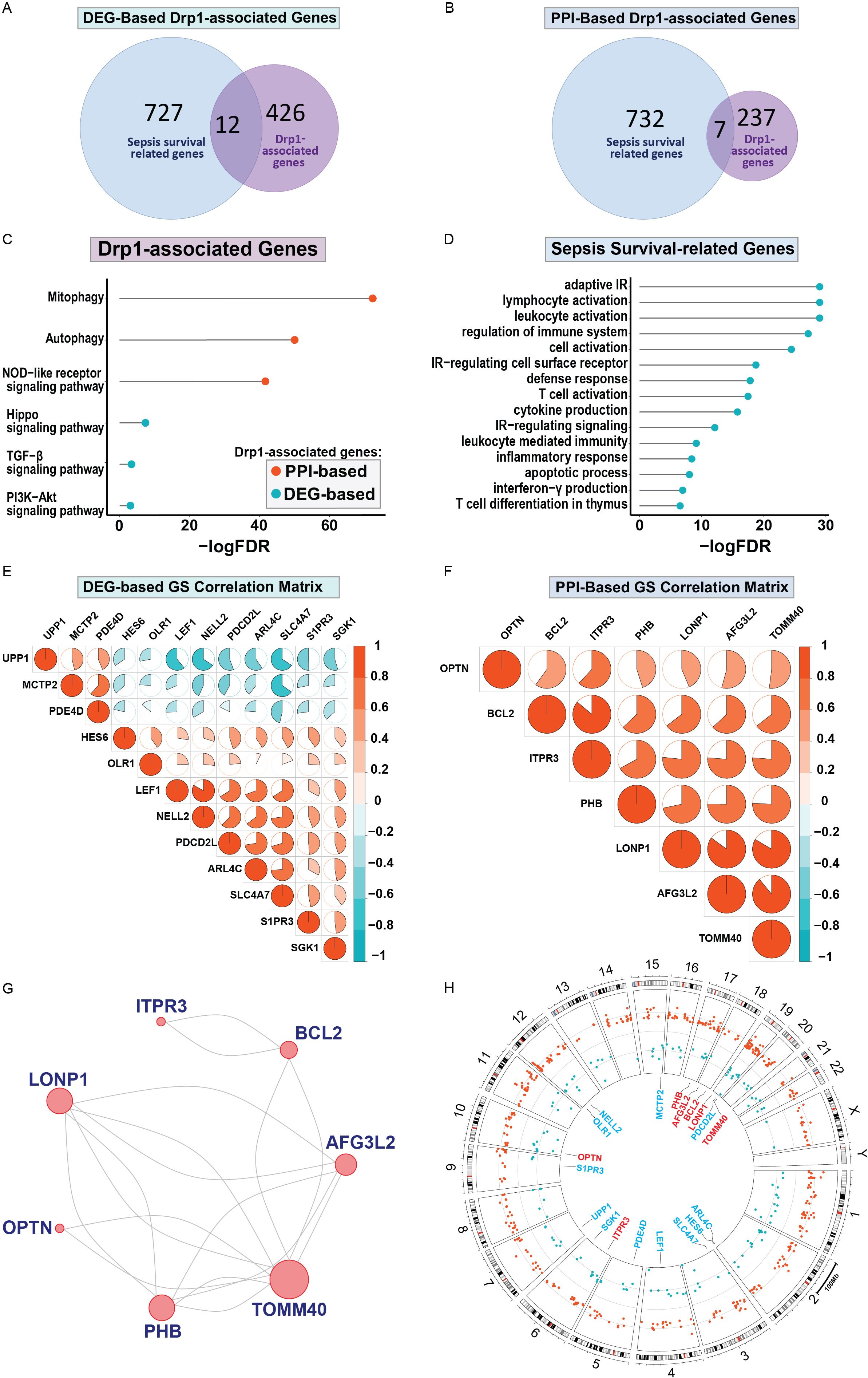
Figure 2. Differentially expressed genes and protein-protein interaction analysis revealed a 12 and 7 Drp1-associated gene signature that corresponds with sepsis survival genes. 12 of 438 Drp1-associated genes (generated by identifying DEG after Drp1 overexpression) match genes related to sepsis survival (A), these 12 genes are referred to as DEG-based GS. PPI analysis of Drp1 identified 244 Drp1-associated genes. 7 of these genes are common between the Drp1-associated genes and sepsis survival genes (B), these 7 genes are referred to as PPI-based GS. KEGG analysis was used to identify pathways enriched with both Drp1-associated GS (C) and pathways enriched with the sepsis survival genes (D). The correlation matrix of Drp1-DGEs (E) and Drp1-PPI GS (F) shows the relationship of individual genes compared to the other genes in the GS. PPI network for the genes in the PPI-based GS (G), and DEG-based GS have no interactions. Genomic locations for both GS are shown in a circular plot (H), with the DEG-based GS represented by blue lettering, and the PPI-based GS represented by red lettering. Red dots represent upregulated genes and blue dots represent downregulated genes related to sepsis survival. Drp1, Dynamin-related protein 1; DEGs, differentially expressed genes; PPI, protein-protein interaction; KEGG, Kyoto Encyclopedia of Genes and Genomes; FDR, adjusted p-value; GS, gene signature; IR, immune response.
We then performed KEGG pathway analysis using both the Drp1 DEGs and PPI gene signatures (Figure 2C). This revealed 6 pathways that are enriched in these genes: mitophagy, autophagy, the NOD-like receptor, TGF-β, and PI3K-Akt signaling pathways. We have previously published a gene signature that differentiates between patients with a low or high risk of sepsis-related death and compiled a list of genes related to sepsis survival (32). KEGG analysis of these genes reveals 15 pathways enriched in sepsis-survival genes, consisting of primarily immunomodulatory pathways (Figure 2D). We then assessed the correlation of the Drp1-associated genes with the other genes in their respective GS to validate that PPI GS was generated correctly (Figures 2E, F). As expected, the Drp1-DEGs GS consists of genes with relatively low correlation with one another (Figure 2E). On the other hand, the Drp1-PPI GS consists of genes that are highly correlated with one another (Figure 2F). The PPI network of this GS is shown in Figure 2G, meanwhile, no connection was found within Drp1-DEGs GS. The location of the genes of both GS are shown in Figure 2H, with the Drp1-DEGs GS represented by blue lettering, and the Drp1-PPI GS represented by red lettering in the inner circle. The dots in the middle circle represent the locations of genes related to sepsis survival, with red dots representing upregulated and blue dots representing downregulated sepsis survival-related genes.
Next, we sought to evaluate both GS for their capabilities of distinguishing high and low sepsis risk groups. To accomplish this, we first compiled the expression levels of the individual genes within the GS and separated the low and high-risk patients. We obtained heatmaps of the DEGs-based (Figure 3A) and PPI-based (Figure 3B) GS, which both show expression patterns that differentiate low and high-risk sepsis groups from the discovery cohort. To confirm this differentiation, we generated violin plots of the sepsis survival scores of both gene signatures in the discovery and validation cohorts. To calculate sepsis survival scores, we used our previously published formula (32), which assesses the probability of septic death in each patient. Lower score values indicate a worse prognosis and survival rate, we labeled this group as the high-risk septic patients. Higher scores were classified as low-risk septic patients. Both sepsis survival score violin plots demonstrate that the DEG-based (Figure 3C) and the PPI-based (Figure 3D) GS successfully differentiate between low and high-risk sepsis patients, with the PPI-based GS providing greater separation between the low and high-risk groups. To evaluate both the specificity and sensitivity of the gene signatures, we generated a receiver operating characteristic curve (ROC) for the DEG-based (Figure 3E) and PPI-based (Figure 3F) GS. These ROC curves reveal an AUC value of 0.89 for the discovery cohort using the DEG-based GS and 0.97 using the PPI-based GS. For the validation cohort, the DEG-based GS has an AUC value of 0.69 and the PPI-based GS has a value of 0.91, indicating that the PPI-based GS is more specific and sensitive compared to the DEG-based GS. Principal component analysis (PCA) of both gene signatures shows that the DEG-based (Figure 4A) and PPI-based (Figure 4C) GS can completely differentiate high-risk sepsis patients from low-risk patients in both the discovery and validation cohorts. The PCA analysis for DEG-based GS represents 78.5-84.2% of the variable expression data. The PCA analysis for PPI-based GS represents 52.6-59.3% of the variable expression data.
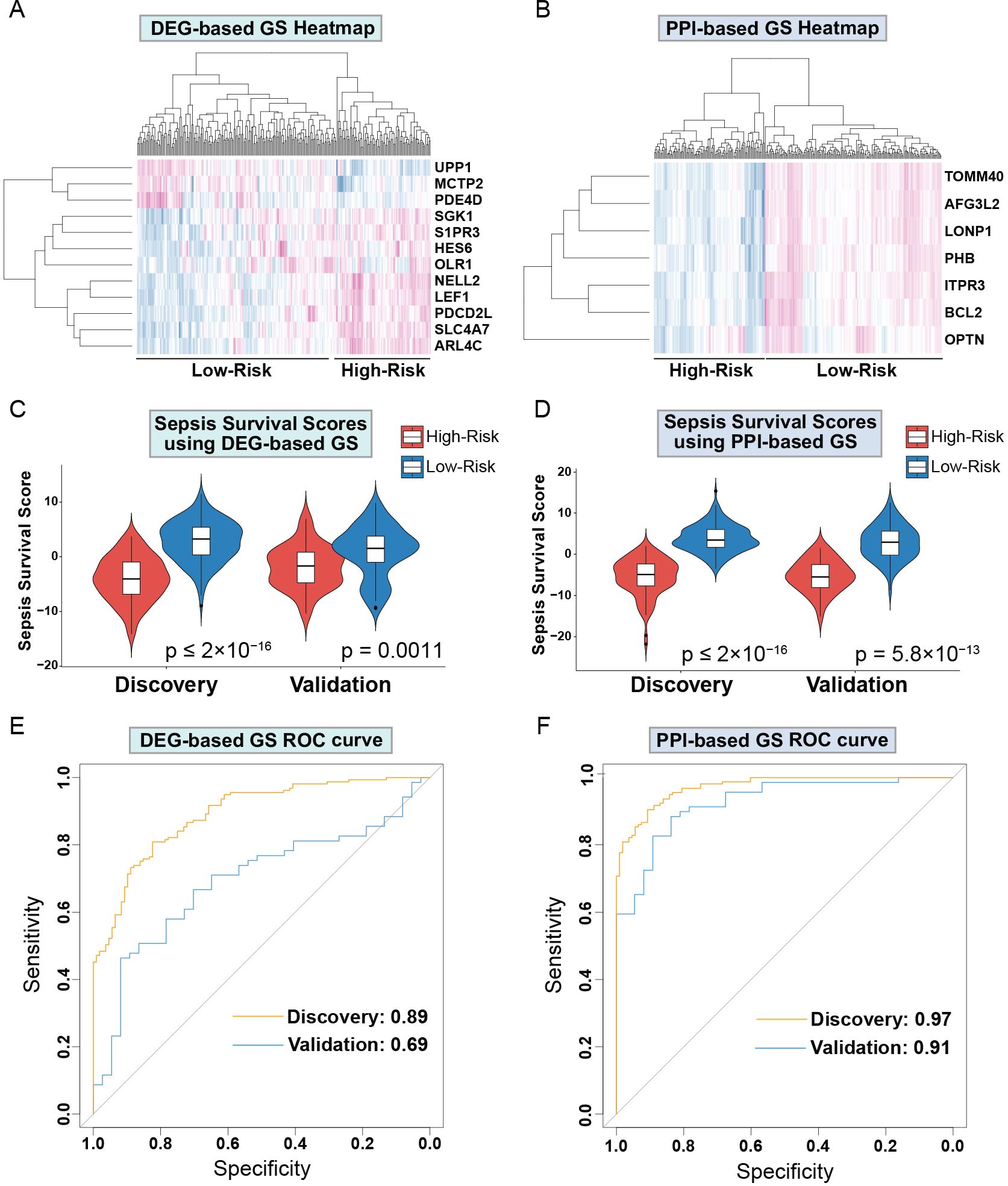
Figure 3. Drp1-associated gene signatures differentiate between low and high-risk sepsis groups in both the discovery and validation cohort. Heatmap of the DEG-based (A) and PPI-based GS (B) reveal expression patterns that differentiate low and high-risk sepsis groups. Violin plots of the sepsis survival scores from DEG-based (C) and the PPI-based (D) GS show that these gene signatures successfully differentiate between low and high-risk sepsis patients in both the discovery and validation cohorts. ROC curves of the DEG-based (E) and PPI-based (F) GS exhibit the sensitivity and specificity of these gene signatures. Drp1, Dynamin-related protein 1; DEGs, differentially expressed genes; PPI, protein-protein interaction; GS, gene signature; ROC, receiver operating characteristic curve.
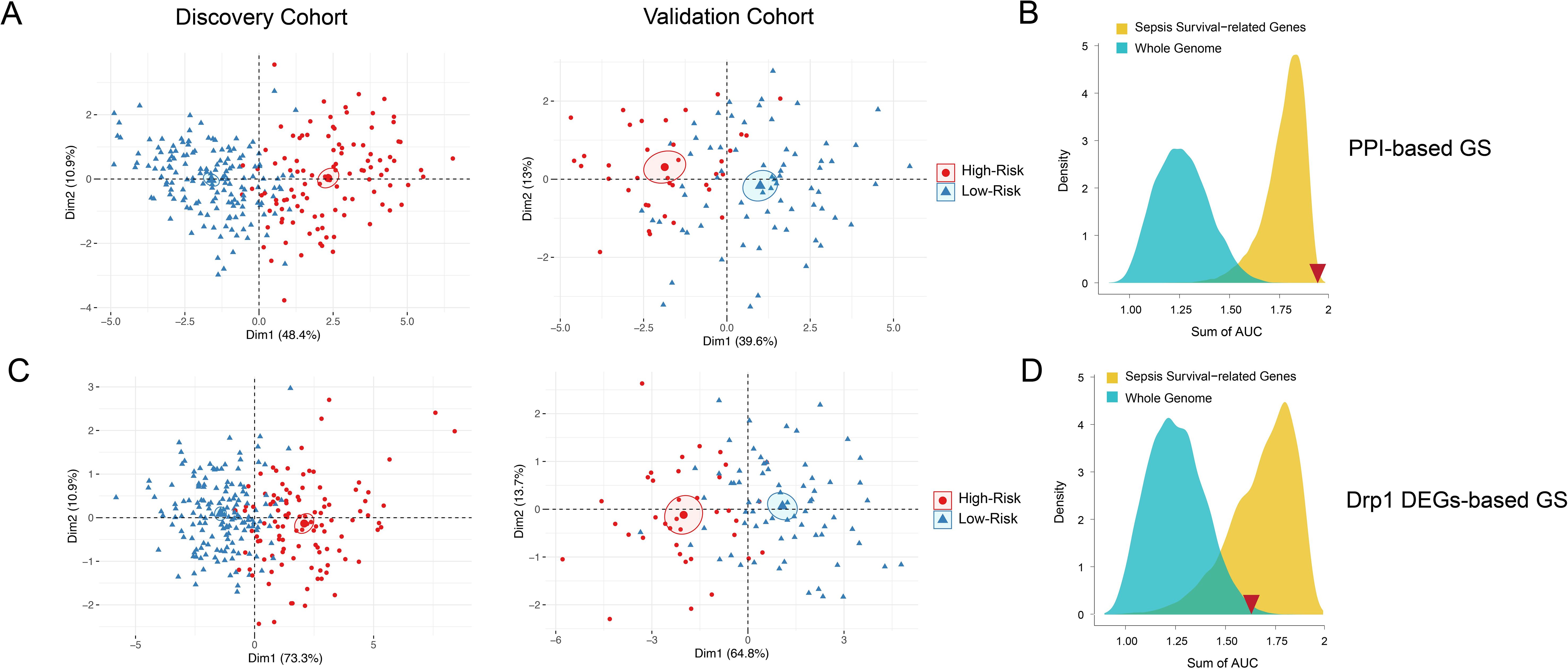
Figure 4. PCA and density distribution plots of both Drp1-associated gene signatures. PCA of both DEG-based (A) and PPI-based (C) GS were performed to reduce dimensionality and assess the similarity between each individual sample. The PCA showed that both gene signatures can entirely differentiate high-risk sepsis patients and low-risk sepsis patients. The PCA analysis for DEG-based GS represents 78.5-84.2% of the variable expression data. The PCA analysis for PPI-based GS represents 52.6-59.3% of the variable expression data. The density distribution of the AUC of random genes (blue) and sepsis survival-related genes (yellow) shows that both DEG-based GS (B) and PPI-based GS (D) have a prognostic power greater than random genes from the whole genome. The PPI-based GS (D) prognostic power is greater than that of other random genes related to sepsis survival. The sum of the DEG-based GS AUC value (1.58) and the sum of the PPI-based GS AUC value (1.88) is indicated by the red inverted triangles. Drp1, Dynamin-related protein 1; DEGs, differentially expressed genes; PPI, protein-protein interaction; GS, gene signature; PCA, principal component analysis; AUC, area under the curve.
To confirm that both gene signatures are more predictive of sepsis risk than a random set of whole genome genes (blue) or a random set of sepsis-survival genes (yellow), we plotted the density distribution of the AUC for the whole genome and sepsis-survival genes (Figures 4B, D). The sum of the DEG-based GS AUC value (1.58) and the sum of the Drp1-PPI GS AUC value (1.88) are indicated by the red inverted triangles. These results indicate that both the DEG-based and PPI-based GS have a prognostic power greater than random genes from the whole genome. However, the PPI-based GS has prognostic power that is greater than that of other random genes related to sepsis survival, while the DEG-based GS does not. Therefore, we selected the PPI-based GS to calculate sepsis survival scores for the patients in the discovery cohort.
In order to understand the cellular and molecular mechanism of the prognostic power of the PPI-GS, we compared the transcriptomic and cellularity differences between the high-score and low-score groups. Using the PPI-based GS, we then re-clustered the patients into high-survival score (Supplementary Table 3) or low-survival score (Supplementary Table 4) cohorts based on whether the survival scores of these samples were higher or lower than the medium survival score across all samples. With the new cohorts generated from the PPI-based GS, we identified DEGs in the high-survival score cohorts in comparison to the low-survival score cohorts, where low-survival scores indicate a higher-risk group for sepsis severity. KEGG analysis shows that the upregulated genes in the high-score, low-risk cohort are enriched in the ribosome, DNA replication, T cell, and RNA pathways. While the downregulated genes in this lower-risk group are enriched in the metabolic and the complement and coagulation pathways (Figure 5A). We then utilized Gene Set Variation Analysis (GSVA) to identify additional pathways enriched in the high scores, lower-risk cohort (Figure 5B). This is highly consistent with the KEGG analysis in DEGs. We then generated a heatmap of the pathways identified by GSVA. This revealed pathway enrichment patterns that could differentiate between low- and high-sepsis survival score patients (Figure 5C).
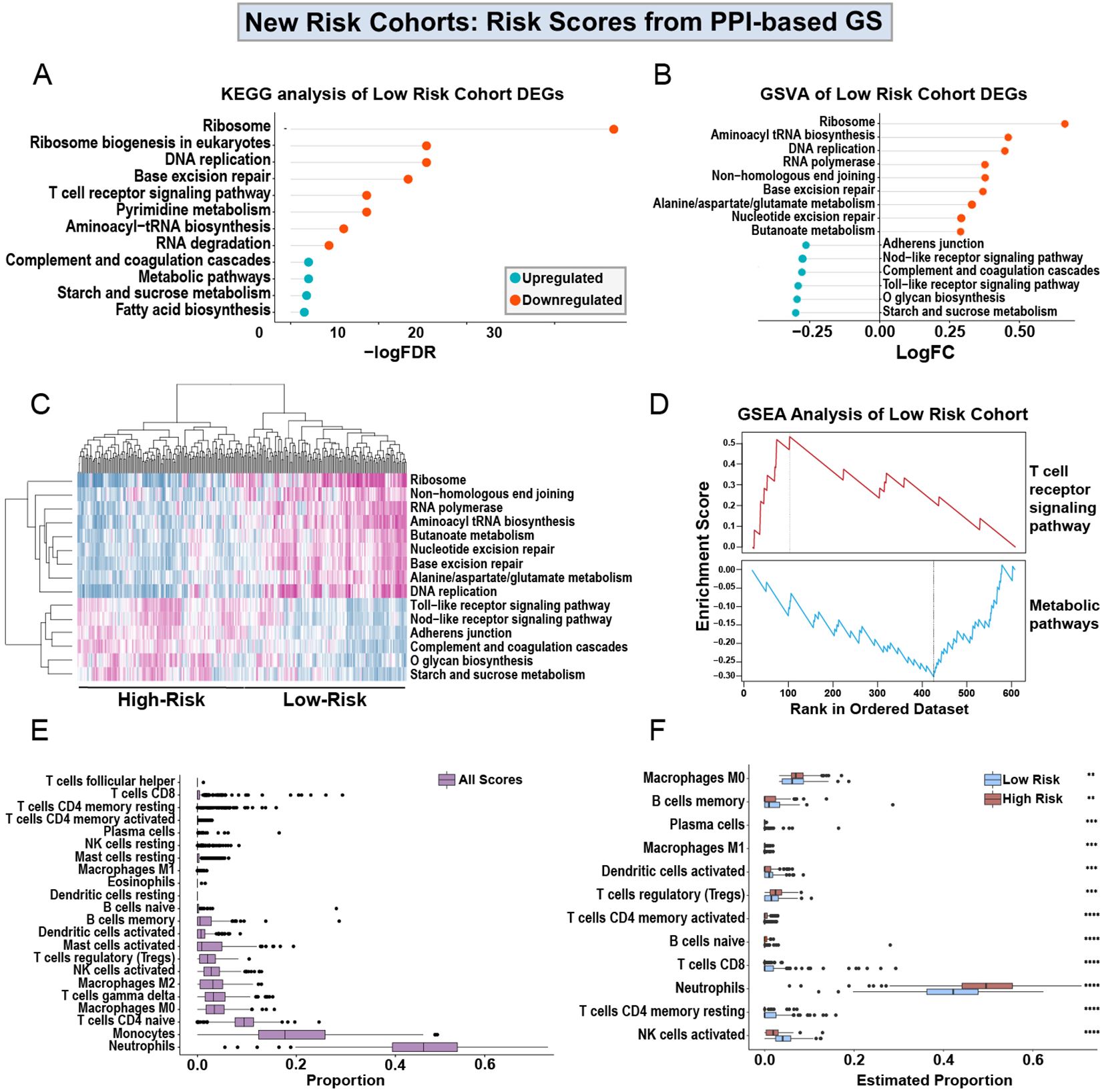
Figure 5. Analysis of low- and high-scores obtained from PPI-based GS. KEGG analysis of the upregulated and downregulated DEGs from high-score, lower-risk patients in the discovery cohort (A), using the Drp1-PPI GS to generate the sepsis survival scores (Supplementary Tables 3, 4). Gene set variation analysis (GSVA) shows the logFC of the pathways enriched in the DEGs from the high-score, lower-risk cohort (B). The heatmap generated from the GSVA shows pathway expression patterns that differentiate the low- and high-score cohorts (C). GSEA shows that the high-score, lower-risk cohort has increased T cell receptor signaling and decreased expression of metabolic pathways (D). Using CIBERSORT, immune cell proportions in PBMCs were estimated in the entire discovery cohort (E). Separating the low- and high-risk cohorts shows a predicted immune profile shift between the groups (F). Specifically, the high-score, lower-risk cohort has decreased levels of neutrophils and increased activation of NK cells. Drp1, Dynamin-related protein 1; DEGs, differentially expressed genes; PPI, protein-protein interaction; KEGG, Kyoto Encyclopedia of Genes and Genomes; FDR, adjusted p-value; FC, fold change; GS, gene signature; GSVA, gene set variation analysis; GSEA, gene set enrichment analysis (GSEA).
Using gene set enrichment analysis (GSEA), we found that the high-score, lower-risk cohort has increased T-cell signaling and decreased metabolic signaling (Figure 5D). Because the results from KEGG, GSVA, and GSEA all indicate an alteration in immune pathways (Figures 5A–D), we used CIBERSORT to estimate the proportions of immune cells in the entire discovery cohort (Figure 5E). Lastly, we separated the low- and high-risk cohorts from one another (Figure 5F). This separation reveals a predicted immune profile shift between the low and high-risk groups. Specifically, the lower-risk patients are predicted to have decreased levels of neutrophils and increased activation of NK cells.
4 Discussion
Despite immense research efforts, there are still no available therapeutics for treating sepsis other than general antibiotics and source control. Early recognition and action are the only courses of action for physicians to improve patient outcomes (12, 36). This necessitates a quick diagnosis to reduce sepsis mortality. Unfortunately, the identification of patients at risk of septic death may not be sufficient to reduce mortality rates. This is exemplified in a retrospective cohort study of 6 hospitals between 2014 and 2015, where only 3.7% of sepsis-associated deaths were considered to be either moderately or definitively preventable (37). This suggests that even early identification of high-risk patients and subsequent implementation of aggressive treatments will only minimally improve sepsis outcomes. This highlights the urgency to identify new strategies to test and implement to improve sepsis survival. In this study, we identified Drp1-associated genes as a predictor of sepsis survival, which not only reveals a novel biomarker but also calls attention to mitochondrial dynamics as a pathway that should be investigated for therapeutic development.
Recent research efforts have called attention to the potential and likely connection between septic organ failure and mitochondrial function (27, 38). For example, it has been proposed that mitochondrial dysfunction may explain why there is minimal cell death observed during sepsis-induced organ failure. Additionally, mitochondrial dysfunction could also explain how tissues maintain oxygen levels during sepsis. Moreover, mitochondria have been speculated to mediate the rapid recovery of organ function in survivors, even in organs notoriously poor at regeneration (39–43). Since mitochondria are the leading harnessers of oxygen, it is plausible that mitochondrial function may explain these seemingly paradoxical findings. At the center of mitochondrial function is mitochondrial dynamics, in which networks of mitochondria alter their shape to modulate their function. Importantly, mitochondrial dynamics connects a multitude of pathways (23) and has vast implications for diseases with multiple perturbed pathways, including sepsis. Here, we provide evidence that mitochondrial dynamics is connected to sepsis severity by demonstrating the prognostic capability of the major regulator of fission, Drp1. Additionally, we demonstrate that Drp1 and its associated proteins likely influence multiple signaling pathways.
Historically, sepsis pathobiology was credited to excessive inflammation. However, this is now widely disputed, resulting in sepsis being redefined (1). It is now accepted that both pro- and anti-inflammatory responses are activated during the early phases of sepsis (17) and that a multitude of nonimmunological pathways are perturbed in addition to abnormal immune responses (44–46). Activation of the Toll-Like Receptor 4 (TLR4) signaling pathway is regarded as a critical component of sepsis (47), releasing pro-inflammatory mediators and reactive oxygen and nitrogen species (48). Attempts to antagonize TLR4 have failed to improve mortality rates (16), suggesting that TLR4 activation may contribute to sepsis development; however, severity may be determined by a more downstream component. In support of this, we recently published that TLR4 agonists increase mitochondrial fission through activation of Drp1 (49). This and our previously published findings lead us to conjecture that mitochondrial dynamics play a critical role in determining sepsis severity. It is tempting to speculate that pathways activated during sepsis may modulate mitochondrial dynamics, leading to dysfunction that perpetuates mitochondrial damage and disease progression. Postulations aside, this study demonstrates that Drp1-associated proteins can predict sepsis severity, implicating mitochondrial dynamics in sepsis progression.
Interestingly, the PPI-based GS predicted sepsis survival better than the DEG-based GS, as indicated by higher specificity and sensitivity values. A plausible explanation for this is that the PPI-based GS consists of proteins that interact with Drp1. Therefore, we may be identifying genes that have more functional outcomes than genes identified by Drp1 overexpression. Although further testing is needed to evaluate this, it does support further evaluation of mitochondrial dynamics during sepsis. In this study, we used the STRING database to identify proteins that interact with Drp1, which generates functional protein association networks grouped into shells. In our analysis, we only used the primary protein interactors. The primary interactors represent proteins that are directly associated with Drp1. On the other hand, the secondary protein interactors (or the 2nd shell) contain proteins that associate with primary protein interactors. In this study, we hypothesized that mitochondrial dynamics are capable of predicting sepsis survival. For that reason, we evaluated the expression of proteins that are directly associated with Drp1. It would be interesting to perform further analysis using the 2nd shell to elucidate which proteins may be enhancing or inhibiting Drp1 activity. Additionally, it would be of value to identify specific pathways these 2nd shell proteins interact with to isolate a gene signature with even greater prognostic ability.
In this study, we used datasets from two different biological sources: primary human peripheral blood mononuclear cells (PBMC) and human cell lines. Because the aim of this study was to evaluate the effects of altered mitochondrial dynamics on sepsis survival, we chose to focus our study on the major fission regulator, Drp1, and proteins that are directly affected by Drp1. To identify genes directly related to Drp1, human cell lines are necessary, as Drp1 deletion causes embryonic lethality in mice (50). Moreover, by using data from cells, we are only gathering genes directly affected by Drp1 upregulation. Global upregulation of Drp1 in mice causes immense damage, which would convolute our analysis and cause such a high degree of dysfunction that the resulting data would no longer have biological applicability to sepsis (51–53). We then used existing PBMC expression data to confirm that these genes are detectable and capable of differentiating between low- and high-risk patients. By using PBMC from septic patients, we validated that the identified genes derived from the human cell lines are relevant in a clinical setting.
One major limitation of this study is the low number of septic patients with available genetic data. Small data sets are inherently more prone to bias that may alter or even hide important genetic evidence. In our study, we used the data set E-MTAB-4421 as the discovery cohort, consisting of 45.6% female samples, while the validation cohort E-MTAB-4451 has only 26.3% female samples. Sex biases in medicine and research are well documented (54–59), and sex disparity in sepsis severity and survival is also noted in the field (60). An analysis of sepsis data from 2017 indicates that the global incidence of sepsis is higher among females (61). This presents a critical unmet need for studying the sex differences in the response to sepsis and highlights the necessity to specifically study sepsis and sex, and include pregnancy status (62, 63). Further, sepsis is well recognized as a heterogeneous disease state (64), meaning that the pathogenesis of sepsis is highly influenced by the pathogen, site of infection, co-morbidities, race, and sex (65–69). Therefore, the current transcriptome databases are substantially inadequate, and major effort should be allocated to obtaining larger datasets that include information on all of these factors for identification of the expression differences between groups that may be hidden by aggregating all the different groups together. Additionally, these datasets lack detailed patient information and the treatments they received. Because of this lack of information, there may be confounding factors between the patients who survive and those who do not. Despite these limitations, we still believe GS could be a valuable tool to identify high-risk patients.
Another consideration while interpreting the results from our study is that the generation of this GS was a computational analysis using data from a retrospective study. Therefore, to confirm our findings, a prospective study is needed where GS is used at ICU admission to determine if the high-risk group identified by the GS has a higher mean length of ICU stay or mortality rate than the low-risk group. If successful, this may lead to the development of precision medicine for this group that counters this expression pattern. Alternatively, this could provide an opportunity to test therapeutics that have previously failed to demonstrate significance in clinical trials. Specifically, if our hypothesis of mitochondrial dynamics being a critical mediator of sepsis severity is correct, that could mean that drug candidates were tested in a population with too diverse of sepsis presentations. Sepsis consists of many perturbed pathways that interact with one another and with a person’s specific genetics or comorbidities. Thus, an individual’s presentation of sepsis can vary. Therefore, identifying high-risk patients with GS could identify a sub-population that would be responsive to therapies that target mitochondrial dynamics. Although more testing is needed to confirm the validity of those speculations, it is clear that the early evidence we provide here provides support that this generated gene signature may provide novel clinical opportunities shown in Figure 6.
This current gene signature, can propel the field forward in understanding the complexity that is sepsis and understand the clinical implications of mitochondrial health. Moreover, as expected, this gene signature might be applied to other chronic and acute diseases due to its nature as a composite biomarker (70–73). Mitochondrial dysfunction is closely associated with a variety of diseases, including SARS-CoV-2 and cancer (74–77). However, it is essential to recognize that the gene signature will be disease-specific and will require careful adjustment and validation for each specific condition. This aligns with the principles of precision medicine. In addition, it is important to recognize that many challenges exist in translating results from bioinformatic analyses into the clinic. For example, one significant challenge is mitigating errors during computational analysis. Overfitting during bioinformatics analyses can lead to false-positive results, making the gene signature cohort-specific and lacking the precision and accuracy needed for reliable disease prognosis in independent cohorts. To address this, two critical steps should be employed: (a) validation in independent cohorts and (b) comparison of the gene signature’s performance against randomized gene signatures, as illustrated in our Figure 3. However, even while taking these precautions, it is essential that gene signatures are tested clinically before widespread use.
In summary, septic organ dysfunction, regardless of severity, is not associated with cell death (41). This suggests that sepsis involves changes in functional signaling rather than organ cell death. A leading theory is that cells are unable to maintain homeostasis during sepsis (46). Specifically, cells are unable to counteract perturbed pathways to return to normal physiological function. Mitochondria are known to play critical roles in maintaining cellular homeostasis (22) and are implicated in sepsis (25–28). Therefore, mitochondria may present an opportunity to restore multiple dysregulated pathways using a single strategy. In this study, we demonstrate that genes related to the major mitochondrial shaping protein, Drp1, can predict sepsis severity. For that reason, mitochondrial dynamics not only offers a prognostic opportunity but also suggests a novel therapeutic strategy that should be investigated further.
5 Conclusions
By engineering and validating gene signatures linked to Drp1, we demonstrated that peripheral blood gene expression can accurately predict sepsis survival. With further studies, this innovative sepsis prognostic biomarker (Drp1-PPI GS) can be developed for clinical application.
Data availability statement
The original contributions presented in the study are included in the article/Supplementary Material. Further inquiries can be directed to the corresponding author.
Ethics statement
Ethical approval was not required for the studies involving humans because Existing public available datasets from NIH GEO are used. The studies were conducted in accordance with the local legislation and institutional requirements. The human samples used in this study were acquired from Only de-identified datasets from NIH-GEO are used. Written informed consent to participate in this study was not required from the participants or the participants’ legal guardians/next of kin in accordance with the national legislation and the institutional requirements.
Author contributions
MP: Conceptualization, Formal analysis, Investigation, Visualization, Writing – original draft, Writing – review & editing. AF: Conceptualization, Data curation, Formal analysis, Investigation, Methodology, Software, Visualization, Writing – original draft, Writing – review & editing. YL: Writing – review & editing. WM: Investigation, Writing – review & editing. SA: Writing – review & editing. HU: Writing – review & editing. SB: Writing – review & editing. TW: Conceptualization, Funding acquisition, Methodology, Supervision, Writing – original draft, Writing – review & editing.
Funding
The author(s) declare financial support was received for the research, authorship, and/or publication of this article. This study is supported in part by grants from the National Institutes of Health: HL134610, HL146369, and HG013615.
Conflict of interest
The authors declare that the research was conducted in the absence of any commercial or financial relationships that could be construed as a potential conflict of interest.
Generative AI statement
The author(s) declare that no Generative AI was used in the creation of this manuscript.
Publisher’s note
All claims expressed in this article are solely those of the authors and do not necessarily represent those of their affiliated organizations, or those of the publisher, the editors and the reviewers. Any product that may be evaluated in this article, or claim that may be made by its manufacturer, is not guaranteed or endorsed by the publisher.
Supplementary material
The Supplementary Material for this article can be found online at: https://www.frontiersin.org/articles/10.3389/fimmu.2024.1516145/full#supplementary-material
References
1. Singer M, Deutschman CS, Seymour CW, Shankar-Hari M, Annane D, Bauer M, et al. The third international consensus definitions for sepsis and septic shock (Sepsis-3). JAMA. (2016) 315:801–10. doi: 10.1001/jama.2016.0287
2. Torio CM, Andrews RM. National inpatient hospital costs: the most expensive conditions by paye. In: Healthcare Cost and Utilization Project (HCUP) Statistical Briefs. gency for Healthcare Research and Quality (US, Rockville (MD (2006).
3. Fleischmann-Struzek C, Mellhammar L, Rose N, Cassini A, Rudd KE, Schlattmann P, et al. Incidence and mortality of hospital- and ICU-treated sepsis: results from an updated and expanded systematic review and meta-analysis. Intensive Care Med. (2020) 46:1552–62. doi: 10.1007/s00134-020-06151-x
4. Karlsson S, Ruokonen E, Varpula T, Ala-Kokko TI, Pettilä V. Long-term outcome and quality-adjusted life years after severe sepsis. Crit Care Med. (2009) 37:1268–74. doi: 10.1097/CCM.0b013e31819c13ac
5. Iwashyna TJ, Ely EW, Smith DM, Langa KM. Long-term cognitive impairment and functional disability among survivors of severe sepsis. JAMA. (2010) 304:1787–94. doi: 10.1001/jama.2010.1553
6. Winters BD, Eberlein M, Leung J, Needham DM, Pronovost PJ, Sevransky JE. Long-term mortality and quality of life in sepsis: a systematic review. Crit Care Med. (2010) 38:1276–83. doi: 10.1097/CCM.0b013e3181d8cc1d
7. Iwashyna TJ, Cooke CR, Wunsch H, Kahn JM. Population burden of long-term survivorship after severe sepsis in older Americans. J Am Geriatr Soc. (2012) 60:1070–7. doi: 10.1111/j.1532-5415.2012.03989.x
8. Levy MM, Evans LE, Rhodes A. The surviving sepsis campaign bundle: 2018 update. Intensive Care Med. (2018) 44:925–8. doi: 10.1007/s00134-018-5085-0
9. Kumar A, Roberts D, Wood KE, Light B, Parrillo JE, Sharma S, et al. Duration of hypotension before initiation of effective antimicrobial therapy is the critical determinant of survival in human septic shock. Crit Care Med. (2006) 34:1589–96. doi: 10.1097/01.CCM.0000217961.75225.E9
10. Rhodes A, Evans LE, Alhazzani W, Levy MM, Antonelli M, Ferrer R, et al. Surviving sepsis campaign: international guidelines for management of sepsis and septic shock: 2016. Intensive Care Med. (2017) 43:304–77. doi: 10.1007/s00134-017-4683-6
11. Suliman S, Price J, Cahill M, Young T, Furmanek S, Galvis J, et al. Bedside evaluation for early sepsis intervention: addition of a sepsis response team leads to improvement in sepsis bundle compliance. Crit Care Explor. (2021) 3. doi: 10.1097/CCE.0000000000000312
12. Ferrer R, Martin-Loeches I, Phillips G, Osborn TM, Townsend S, Dellinger RP, et al. Empiric antibiotic treatment reduces mortality in severe sepsis and septic shock from the first hour: results from a guideline-based performance improvement program. Crit Care Med. (2014) 42:1749–55. doi: 10.1097/CCM.0000000000000330
13. Kim HI, Park S. Sepsis: early recognition and optimized treatment. Tuberc Respir Dis (Seoul). (2019) 82:6–14. doi: 10.4046/trd.2018.0041
14. Abraham E, Glauser MP, Butler T, Garbino J, Gelmont D, Laterre PF, et al. p55 Tumor necrosis factor receptor fusion protein in the treatment of patients with severe sepsis and septic shock. A randomized controlled multicenter trial. Ro 45-2081 Study Group. JAMA. (1997) 277:1531–8. doi: 10.1001/jama.1997.03540430043031
15. Osuchowski MF, Welch K, Siddiqui J, Remick DG. Circulating cytokine/inhibitor profiles reshape the understanding of the SIRS/CARS continuum in sepsis and predict mortality. J Immunol. (2006) 177:1967–74. doi: 10.4049/jimmunol.177.3.1967
16. Opal SM, Laterre PF, Francois B, Larosa SP, Angus DC, Mira JP, et al. Effect of eritoran, an antagonist of MD2-TLR4, on mortality in patients with severe sepsis: the ACCESS randomized trial. JAMA. (2013) 309:1154–62. doi: 10.1001/jama.2013.2194
17. Hotchkiss RS, Monneret G, Payen D. Sepsis-induced immunosuppression: from cellular dysfunctions to immunotherapy. Nat Rev Immunol. (2013) 13:862–74. doi: 10.1038/nri3552
18. Cao M, Wang G, Xie J. Immune dysregulation in sepsis: experiences, lessons and perspectives. Cell Death Discovery. (2023) 9:465. doi: 10.1038/s41420-023-01766-7
19. Marshall JC. Why have clinical trials in sepsis failed? Trends Mol Med. (2014) 20:195–203. doi: 10.1016/j.molmed.2014.01.007
20. Iskander KN, Osuchowski MF, Stearns-Kurosawa DJ, Kurosawa S, Stepien D, Valentine C, et al. Sepsis: multiple abnormalities, heterogeneous responses, and evolving understanding. Physiol Rev. (2013) 93:1247–88. doi: 10.1152/physrev.00037.2012
21. Zhang YY, Ning BT. Signaling pathways and intervention therapies in sepsis. Signal Transduct Target Ther. (2021) 6:407. doi: 10.1038/s41392-021-00816-9
22. Ma K, Chen G, Li W, Kepp O, Zhu Y, Chen Q. Mitophagy, mitochondrial homeostasis, and cell fate. Front Cell Dev Biol. (2020) 8. doi: 10.3389/fcell.2020.00467
23. Pokharel MD, Garcia-Flores A, Marciano D, Franco MC, Fineman JR, Aggarwal S, et al. Mitochondrial network dynamics in pulmonary disease: Bridging the gap between inflammation, oxidative stress, and bioenergetics. Redox Biol. (2024) 70:103049. doi: 10.1016/j.redox.2024.103049
24. Lira Chavez FM, Gartzke LP, Van Beuningen FE, Wink SE, Henning RH, Krenning G, et al. Restoring the infected powerhouse: Mitochondrial quality control in sepsis. Redox Biol. (2023) 68:102968. doi: 10.1016/j.redox.2023.102968
25. Exline MC, Crouser ED. Mitochondrial mechanisms of sepsis-induced organ failure. Front Biosci. (2008) 13:5030–41. doi: 10.2741/3061
26. Islam MN, Das SR, Emin MT, Wei M, Sun L, Westphalen K, et al. Mitochondrial transfer from bone-marrow-derived stromal cells to pulmonary alveoli protects against acute lung injury. Nat Med. (2012) 18:759–65. doi: 10.1038/nm.2736
27. Singer M. The role of mitochondrial dysfunction in sepsis-induced multi-organ failure. Virulence. (2014) 5:66–72. doi: 10.4161/viru.26907
28. Mccall CE, Zhu X, Zabalawi M, Long D, Quinn MA, Yoza BK, et al. Sepsis, pyruvate, and mitochondria energy supply chain shortage. J Leukoc Biol. (2022) 112:1509–14. doi: 10.1002/JLB.3MR0322-692RR
29. Nedel W, Deutschendorf C, Portela LVC. Sepsis-induced mitochondrial dysfunction: A narrative review. World J Crit Care Med. (2023) 12:139–52. doi: 10.5492/wjccm.v12.i3.139
30. Yu SB, Pekkurnaz G. Mechanisms orchestrating mitochondrial dynamics for energy homeostasis. J Mol Biol. (2018) 430:3922–41. doi: 10.1016/j.jmb.2018.07.027
31. Qi Z, Huang Z, Xie F, Chen L. Dynamin-related protein 1: A critical protein in the pathogenesis of neural system dysfunctions and neurodegenerative diseases. J Cell Physiol. (2019) 234:10032–46. doi: 10.1002/jcp.v234.7
32. Feng A, Simpson E, Wu J, Robinson T, Ma W, Tieu K, et al. NAMPT-associated gene signature in the prediction of severe sepsis. Am J Transl Res. (2022) 14:7090–7.
33. Newman AM, Steen CB, Liu CL, Gentles AJ, Chaudhuri AA, Scherer F, et al. Determining cell type abundance and expression from bulk tissues with digital cytometry. Nat Biotechnol. (2019) 37:773–82. doi: 10.1038/s41587-019-0114-2
34. Jombart T. adegenet: a R package for the multivariate analysis of genetic markers. Bioinformatics. (2008) 24:1403–5. doi: 10.1093/bioinformatics/btn129
35. Robin X, Turck N, Hainard A, Tiberti N, Lisacek F, Sanchez JC, et al. pROC: an open-source package for R and S+ to analyze and compare ROC curves. BMC Bioinf. (2011) 12:77. doi: 10.1186/1471-2105-12-77
36. Amland RC, Hahn-Cover KE. Clinical decision support for early recognition of sepsis(). Am J Med Qual. (2019) 34:494–501. doi: 10.1177/1062860619873225
37. Rhee C, Jones TM, Hamad Y, Pande A, Varon J, O’brien C, et al. Prevalence, underlying causes, and preventability of sepsis-associated mortality in US acute care hospitals. JAMA Network Open. (2019) 2:e187571–e187571. doi: 10.1001/jamanetworkopen.2018.7571
38. Arulkumaran N, Deutschman CS, Pinsky MR, Zuckerbraun B, Schumacker PT, Gomez H, et al. MITOCHONDRIAL FUNCTION IN SEPSIS. Shock. (2016) 45:271–81. doi: 10.1097/SHK.0000000000000463
39. Boekstegers P, Weidenhöfer S, Pilz G, Werdan K. Peripheral oxygen availability within skeletal muscle in sepsis and septic shock: comparison to limited infection and cardiogenic shock. Infection. (1991) 19:317–23. doi: 10.1007/BF01645355
40. Rosser DM, Stidwill RP, Jacobson D, Singer M. Oxygen tension in the bladder epithelium rises in both high and low cardiac output endotoxemic sepsis. J Appl Physiol (1985). (1995) 79:1878–82. doi: 10.1152/jappl.1995.79.6.1878
41. Hotchkiss RS, Swanson PE, Freeman BD, Tinsley KW, Cobb JP, Matuschak GM, et al. Apoptotic cell death in patients with sepsis, shock, and multiple organ dysfunction. Crit Care Med. (1999) 27:1230–51. doi: 10.1097/00003246-199907000-00002
42. Dyson A, Rudiger A, Singer M. Temporal changes in tissue cardiorespiratory function during faecal peritonitis. Intensive Care Med. (2011) 37:1192–200. doi: 10.1007/s00134-011-2227-z
43. Takasu O, Gaut JP, Watanabe E, To K, Fagley RE, Sato B, et al. Mechanisms of cardiac and renal dysfunction in patients dying of sepsis. Am J Respir Crit Care Med. (2013) 187:509–17. doi: 10.1164/rccm.201211-1983OC
44. Singer M, De Santis V, Vitale D, Jeffcoate W. Multiorgan failure is an adaptive, endocrine-mediated, metabolic response to overwhelming systemic inflammation. Lancet. (2004) 364:545–8. doi: 10.1016/S0140-6736(04)16815-3
45. Angus DC, van der Poll T. Severe sepsis and septic shock. N Engl J Med. (2013) 369:840–51. doi: 10.1056/NEJMra1208623
46. Deutschman CS, Tracey KJ. Sepsis: current dogma and new perspectives. Immunity. (2014) 40:463–75. doi: 10.1016/j.immuni.2014.04.001
47. Kuzmich NN, Sivak KV, Chubarev VN, Porozov YB, Savateeva-Lyubimova TN, Peri F. TLR4 signaling pathway modulators as potential therapeutics in inflammation and sepsis. Vaccines (Basel). (2017) 5. doi: 10.3390/vaccines5040034
48. Kumar V. Toll-like receptors in sepsis-associated cytokine storm and their endogenous negative regulators as future immunomodulatory targets. Int Immunopharmacol. (2020) 89:107087. doi: 10.1016/j.intimp.2020.107087
49. Pokharel MD, Fu P, Garcia-Flores A, Yegambaram M, Lu Q, Sun X, et al. Inflammatory lung injury is associated with endothelial cell mitochondrial fission and requires the nitration of RhoA and cytoskeletal remodeling. Free Radic Biol Med. (2024) 221:125–35. doi: 10.1016/j.freeradbiomed.2024.05.019
50. Wakabayashi J, Zhang Z, Wakabayashi N, Tamura Y, Fukaya M, Kensler TW, et al. The dynamin-related GTPase Drp1 is required for embryonic and brain development in mice. J Cell Biol. (2009) 186:805–16. doi: 10.1083/jcb.200903065
51. Touvier T, De Palma C, Rigamonti E, Scagliola A, Incerti E, Mazelin L, et al. Muscle-specific Drp1 overexpression impairs skeletal muscle growth via translational attenuation. Cell Death Dis. (2015) 6:e1663–3. doi: 10.1038/cddis.2014.595
52. Hu C, Huang Y, Li L. Drp1-dependent mitochondrial fission plays critical roles in physiological and pathological progresses in mammals. Int J Mol Sci. (2017) 18. doi: 10.3390/ijms18010144
53. Giovarelli M, Zecchini S, Martini E, Garrè M, Barozzi S, Ripolone M, et al. Drp1 overexpression induces desmin disassembling and drives kinesin-1 activation promoting mitochondrial trafficking in skeletal muscle. Cell Death Differentiation. (2020) 27:2383–401. doi: 10.1038/s41418-020-0510-7
54. Beery AK, Zucker I. Sex bias in neuroscience and biomedical research. Neurosci Biobehav Rev. (2011) 35:565–72. doi: 10.1016/j.neubiorev.2010.07.002
55. Clayton JA, Arnegard ME. Taking cardiology clinical trials to the next level: A call to action. Clin Cardiol. (2018) 41:179–84. doi: 10.1002/clc.2018.41.issue-2
56. Feldman S, Ammar W, Lo K, Trepman E, Van Zuylen M, Etzioni O. Quantifying sex bias in clinical studies at scale with automated data extraction. JAMA Netw Open. (2019) 2:e196700. doi: 10.1001/jamanetworkopen.2019.6700
57. Mamlouk GM, Dorris DM, Barrett LR, Meitzen J. Sex bias and omission in neuroscience research is influenced by research model and journal, but not reported NIH funding. Front Neuroendocrinol. (2020) 57:100835. doi: 10.1016/j.yfrne.2020.100835
58. Al Hamid A, Beckett R, Wilson M, Jalal Z, Cheema E, Al-Jumeily Obe D, et al. Gender bias in diagnosis, prevention, and treatment of cardiovascular diseases: A systematic review. Cureus. (2024) 16:e54264. doi: 10.7759/cureus.54264
59. Goldstein KM, Kung LCY, Dailey SA, Kroll-Desrosiers A, Burke C, Shepherd-Banigan M, et al. Strategies for enhancing the representation of women in clinical trials: an evidence map. Syst Rev. (2024) 13:2. doi: 10.1186/s13643-023-02408-w
60. Klein SL. Sex influences immune responses to viruses, and efficacy of prophylaxis and treatments for viral diseases. Bioessays. (2012) 34:1050–9. doi: 10.1002/bies.201200099
61. Rudd KE, Johnson SC, Agesa KM, Shackelford KA, Tsoi D, Kievlan DR, et al. Global, regional, and national sepsis incidence and mortality 1990-2017: analysis for the Global Burden of Disease Study. Lancet. (2020) 395:200–11. doi: 10.1016/S0140-6736(19)32989-7
62. Say L, Chou D, Gemmill A, Tunçalp Ö, Moller AB, Daniels J, et al. Global causes of maternal death: a WHO systematic analysis. Lancet Glob Health. (2014) 2:e323–333. doi: 10.1016/S2214-109X(14)70227-X
63. Greer O, Shah NM, Sriskandan S, Johnson MR. Sepsis: precision-based medicine for pregnancy and the puerperium. Int J Mol Sci. (2019) 20. doi: 10.3390/ijms20215388
64. Stanski NL, Wong HR. Prognostic and predictive enrichment in sepsis. Nat Rev Nephrol. (2020) 16:20–31. doi: 10.1038/s41581-019-0199-3
65. Dimeglio M, Dubensky J, SChadt S, Potdar R, Laudanski K. Factors underlying racial disparities in sepsis management. Healthcare (Basel). (2018) 6. doi: 10.20944/preprints201810.0192.v1
66. Leligdowicz A, Matthay MA. Heterogeneity in sepsis: new biological evidence with clinical applications. Crit Care. (2019) 23:80. doi: 10.1186/s13054-019-2372-2
67. Engoren M, Arslanian-Engoren C. Race and sex based disparities in sepsis. Heart Lung. (2022) 52:37–41. doi: 10.1016/j.hrtlng.2021.11.001
68. Black LP, Hopson C, Puskarich MA, Modave F, Booker SQ, Devos E, et al. Racial disparities in septic shock mortality: a retrospective cohort study. Lancet Regional Health – Americas. (2024) 29. doi: 10.1016/j.lana.2023.100646
69. Bladon S, Ashiru-Oredope D, Cunningham N, Pate A, Martin GP, Zhong X, et al. Rapid systematic review on risks and outcomes of sepsis: the influence of risk factors associated with health inequalities. Int J Equity Health. (2024) 23:34. doi: 10.1186/s12939-024-02114-6
70. Qian Z, Zhou T, Gurguis CI, Xu X, Wen Q, Lv J, et al. Nuclear factor, erythroid 2-like 2-associated molecular signature predicts lung cancer survival. Sci Rep. (2015) 5:16889. doi: 10.1038/srep16889
71. Qian Z, Lv J, Kelly GT, Wang H, Zhang X, Gu W, et al. Expression of nuclear factor, erythroid 2-like 2-mediated genes differentiates tuberculosis. Tuberculosis (Edinb). (2016) 99:56–62. doi: 10.1016/j.tube.2016.04.008
72. Feng A, Ma W, Faraj R, Kelly GT, Black SM, Fallon MB, et al. Identification of S1PR3 gene signature involved in survival of sepsis patients. BMC Med Genomics. (2021) 14:43. doi: 10.1186/s12920-021-00886-2
73. Feng A, Caro YM, Gardner C, Grischo G, Liang Y, Wickremasinghe PD, et al. PTK2-associated gene signature could predict the prognosis of IPF. Respir Res. (2023) 24:304. doi: 10.1186/s12931-023-02582-4
74. Moro L. Mitochondrial dysfunction in aging and cancer. J Clin Med. (2019) 8. doi: 10.3390/jcm8111983
75. Georgieva E, Ananiev J, Yovchev Y, Arabadzhiev G, Abrashev H, Abrasheva D, et al. COVID-19 complications: oxidative stress, inflammation, and mitochondrial and endothelial dysfunction. Int J Mol Sci. (2023) 24. doi: 10.3390/ijms241914876
76. Wang SF, Tseng LM, Lee HC. Role of mitochondrial alterations in human cancer progression and cancer immunity. J BioMed Sci. (2023) 30:61. doi: 10.1186/s12929-023-00956-w
Keywords: DRP1, fission, mitochondria, sepsis survival, inflammation
Citation: Pokharel MD, Feng A, Liang Y, Ma W, Aggarwal S, Unwalla H, Black SM and Wang T (2025) Drp1–associated genes implicated in sepsis survival. Front. Immunol. 15:1516145. doi: 10.3389/fimmu.2024.1516145
Received: 23 October 2024; Accepted: 12 December 2024;
Published: 08 January 2025.
Edited by:
Artur Trancoso Lopo De Queiroz, Gonçalo Moniz Institute (IGM), BrazilReviewed by:
Ricardo Khouri, Gonçalo Moniz Institute (IGM), BrazilDhanasekaran Sivakumaran, University of Bergen, Norway
Copyright © 2025 Pokharel, Feng, Liang, Ma, Aggarwal, Unwalla, Black and Wang. This is an open-access article distributed under the terms of the Creative Commons Attribution License (CC BY). The use, distribution or reproduction in other forums is permitted, provided the original author(s) and the copyright owner(s) are credited and that the original publication in this journal is cited, in accordance with accepted academic practice. No use, distribution or reproduction is permitted which does not comply with these terms.
*Correspondence: Ting Wang, dGlud2FuZ0BmaXUuZWR1
†These authors have contributed equally to this work and share first authorship