- 1Personalized Diet Research Group, Korea Food Research Institute, Wanju-gun, Jeollabuk-do, Republic of Korea
- 2Department of Molecular Biology, Jeonbuk National University, Jeonju-si, Jeollabuk-do, Republic of Korea
Immunotherapy, especially immune checkpoint inhibitor (ICI) therapy, has yielded remarkable outcomes for some patients with solid cancers, but others do not respond to these treatments. Recent research has identified the gut microbiota as a key modulator of immune responses, suggesting that its composition is closely linked to responses to ICI therapy in cancer treatment. As a result, the gut microbiome is gaining attention as a potential biomarker for predicting individual responses to ICI therapy and as a target for enhancing treatment efficacy. In this review, we discuss key findings from human observational studies assessing the effect of antibiotic use prior to ICI therapy on outcomes and identifying specific gut bacteria associated with favorable and unfavorable responses. Moreover, we review studies investigating the possibility of patient outcome prediction using machine learning models based on gut microbiome data before starting ICI therapy and clinical trials exploring whether gut microbiota modulation, for example via fecal microbiota transplantation or live biotherapeutic products, can improve results of ICI therapy in patients with cancer. We also briefly discuss the mechanisms through which the gut microbial-derived products influence immunotherapy effectiveness. Further research is necessary to fully understand the complex interactions between the host, gut microbiota, and immunotherapy and to develop personalized strategies that optimize responses to ICI therapy.
1 Introduction
Immunotherapy harnesses the body’s immune system to target and eliminate cancer cells (1). The various forms of immunotherapy include immune checkpoint inhibitors (ICIs)—such as programmed cell death protein 1 (PD-1), programmed cell death ligand 1 (PD-L1), and cytotoxic T-lymphocyte–associated antigen 4 (CTLA-4) inhibitors—, adoptive cell therapies—such as chimeric antigen receptor T-cell therapy, tumor-infiltrating lymphocyte therapy, and natural killer (NK) cell therapy—, cancer vaccines, and cytokine therapies (2). These therapies work by either enhancing the immune system’s ability to detect and destroy cancer cells or infusing cancer-fighting immune cells that effectively target cancer cells (3).
Among various types of cancer immunotherapy, ICI therapy has emerged as a promising therapeutic approach for a variety of solid tumors, including for non-small cell lung cancer (NSCLC) and melanoma (4). ICIs are monoclonal antibodies that can target and block immune checkpoints, such as PD-1 and CTLA-4 on T cells or PD-L1 on tumor cells, thus inhibiting tumor immune escape and enhancing the antitumor function of T cells (5). ICIs offer clear advantages such as high accuracy, response durability, wide adaptability, and low toxicity (2). However, the effectiveness of ICI therapy can vary greatly between individuals. For instance, in patients with NSCLC with high PD-L1 expression treated with pembrolizumab (a PD-1 inhibitor), the five-year overall survival (OS) rate is approximately 25–30% (6). A recent study estimated that only 19.60% of US patients with cancer responded to ICI treatment in 2023. This high variability in ICI response has been linked to several factors, including tumor mutational burden, PD-L1 expression, immune infiltration, altered interferon (IFN)-γ signaling, and epigenetic modifications (7, 8). Nevertheless, the factors determining the efficacy of ICI therapy remain poorly understood, posing considerable challenges for improving response rates and developing effective, patient-specific ICI treatment strategies.
Recent studies have shown that the gut microbiota plays a significant role in shaping drug responses by either modifying drug structures or affecting host physiological functions (9–11). Moreover, the gut microbiota can modulate local and systemic immune responses via microbial metabolites or components (12). For example, gut microbiota can affect T-cell expression profiles (e.g., regulatory T cells (Tregs) and CD8+ T cells) and regulate the differentiation of monocytes into immunogenic dendritic cells (DCs) (13, 14). Furthermore, short-chain fatty acid (SCFA) butyrate produced by gut microbiota can enhance CD8+ T-cell effector function and promote the transition of activated CD8+ T cells to T memory cells (15, 16). These immunomodulatory capacities of the gut microbiota suggest its possible influence on the efficacy of ICI therapy. In 2015, Vétizou et al. showed that antibiotic-treated or germ-free mice with tumors exhibited poor responses to CTLA-4 blockade, and the supplementation of specific Bacteroides spp. restored treatment efficacy by activating T-helper (Th) 1 cells in tumor-draining lymph nodes and promoting the maturation of intratumoral DCs (17). Importantly, three studies in 2018 have revealed a strong link between the gut microbiota and outcomes of ICI therapy in human (18–20), positioning it as a potential biomarker for predicting immunotherapy response and a modifiable target for enhancing treatment efficacy.
This review focuses on the past and current state of research on role of the gut microbiota in the responses to ICI therapy in human patients with solid tumors (Figure 1). We explore findings from studies investigating whether gut microbial dysbiosis due to antibiotic treatment is associated with ICI therapy outcomes in patients with solid tumors and determining which specific gut microbes are differentially abundant in responders and non-responders. We also examine research on outcome prediction using machine learning models based on gut microbiome data before starting ICI therapy, as well as clinical trials determining whether gut microbiota modulation can improve ICI treatment outcomes. In addition, we briefly outline proposed mechanisms by which the gut microbiota impacts the efficacy of ICI therapy via cell components or metabolites. As most human studies on this subject have been conducted specifically on gut bacteria, the term “gut microbiome” in this review will refer to the gut bacterial microbiome.
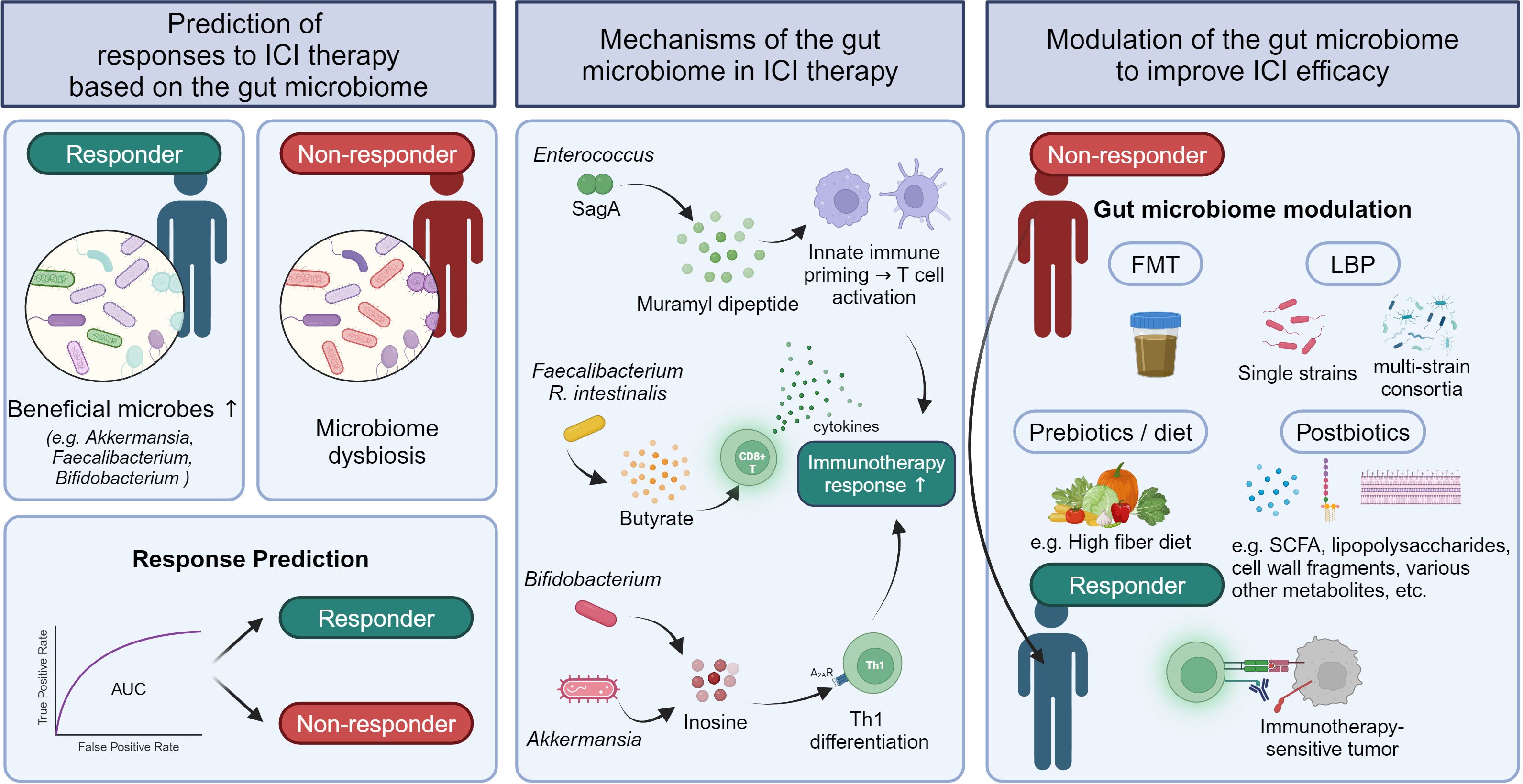
Figure 1. Strategies to predict ICI responses and improve ICI efficacy based on the influence of the gut microbiome in solid tumor responses to ICI therapy.
2 Impact of antibiotic treatment on efficacy of ICI therapy
Patients with cancers are often prescribed antibiotics to treat infectious diseases such as dental, respiratory tract, and urinary tract infections. Antibiotic use is necessary to control infections, but it can disrupt gut microbial homeostasis by altering microbial composition and function (21). The gut dysbiosis induced by antibiotic use can affect the host immune system (22) and has been linked to increased risks of various immunological diseases such as allergy, asthma, and rheumatoid arthritis (23–25). These findings indicate that antibiotic use in patients with cancers may influence the efficacy of ICI therapy by impairing immune responses. Since the first study reporting worsened responses to ICI therapy in antibiotic-treated mice (17), many studies have examined the impact of antibiotic use on outcomes of ICI therapy in human cohorts (Table 1).
In a large European cohort of patients with advanced NSCLC (n = 140), renal cell carcinoma (RCC) (n = 67), and urothelial carcinoma (UC) (n = 42) who received PD-1/PD-L1 inhibitors, progression-free survival (PFS) and/or OS were significantly shorter in the antibiotic-treated group within two months before or one month after the start of ICI treatment, compared to the untreated group (PFS: 3.5 months for antibiotic users vs. 4.1 months for non-users, OS: 11.5 vs. 20.6 months) (26). Similarly, detrimental effects of antibiotic use within 30 days of the first ICI administration on therapeutic efficacy were observed in patients with advanced RCC (n = 121) and NSCLC (n = 239) treated with anti-PD-L1 inhibitor (PFS: 1.9 vs. 7.4 months, OS: 17.3 vs. 30.6 months) (18). In a cohort of Korean patients with solid tumors (n = 234), including NSCLC and melanoma, antibiotic treatment within 60 days of starting ICI therapy was associated with significantly reduced PFS (2 vs. 4 months) and OS (5 vs. 17 months) (27). However, other studies have shown minimal or no significant effects of antibiotic use on outcomes of ICI therapy (28, 29). Despite these mixed findings, a meta-analysis of 23 studies on NSCLC patients reported that antibiotic use before or during ICI treatment reduced median PFS (n = 2208) by 1.2 months and median OS (n = 5560) by 6.7 months (30). Another meta-analysis of 37 studies across 13 cancer types also found that antibiotic use was significantly associated with poorer prognosis (OS and PFS) in cancer patients treated with ICIs (31).
These findings suggest that the gut microbiota plays a critical role in determining responses to ICI therapy and underscore the importance of considering a patient’s antibiotic treatment history when planning ICI treatment. More detailed studies are needed to explore the effects of different class of antibiotics, doses, treatment durations, and time of administration on the efficacy of ICI therapy in various cancer types and stages.
3 Gut bacterial features related with outcomes of ICI therapy
Identifying the specific microbes that play pivotal roles in influencing responses to ICI therapy is crucial for understanding their immune modulation mechanisms, predicting the efficacy of ICI therapy, and developing personalized microbiota modulation strategies to enhance outcomes of ICI therapy. In 2018, three landmark studies were published. Routy et al. found that Akkermansia muciniphila, Enterococcus faecium, and Alistipes indistinctus were significantly enriched in responders compared to non-responders among patients with NSCLC and RCC treated with PD-1 inhibitors (n = 100) (26). Matson et al. reported enrichment of Bifidobacterium longum, Collinsella aerofaciens, and E. faecium in responders (n = 42), while Gopalakrishnan et al. found enrichment of Faecalibacterium in responders (n = 43) in melanoma patients treated with PD-1/CTLA-4 inhibitors (19, 20).
Since these initial studies, many others have reported distinct gut microbial features associated with responses to ICI therapy (Table 2). In patients with advanced NSCLC treated with anti-PD-1 blockade (n = 25), responders had a higher abundance of Alistipes putredinis, Prevotella copri, and B. longum, while non-responders had more Ruminococcus (32). A large cohort study of NSCLC patients receiving ICIs (n = 338) found that baseline A. muciniphila presence was associated with higher objective response rates (ORR) and OS (33). Another study of NSCLC patients (n = 65) undergoing ICI therapy reported significant enrichment of Ruminococcus, Akkermansia, and Faecalibacterium in responders (34). In addition to NSCLC, several studies have been conducted on melanoma. In melanoma patients receiving ICI therapy (n = 27), prolonged PFS was linked to higher abundances of Faecalibacterium prausnitzii, Coprococcus eutactus, and Prevotella stercorea, while shorter PFS was associated with higher levels of Bacteroides ovatus, B. dorei, B. massiliensis, Ruminococcus gnavus, and Blautia producta (35). Another study of advanced melanoma patients treated with combined CTLA-4 and PD-1 blockade (n = 40) found that responders had enriched levels of Bacteroides stercoris, Parabacteroides distasonis, and Fournierella massiliensis (36). In RCC (n = 31), Bifidobacterium adolescentis, Barnesiella intestinihominis, Odoribacter splanchnicus, and Bacteroides eggerthii were enriched in responders (37). Another RCC study (n = 58) showed that responders to PD-1 inhibitors had higher levels of A. muciniphila, Bacteroides salyersiae, and Eubacterium siraeum (38). In hepatocellular carcinoma (HCC), an increase in Faecalibacterium was observed in patients who responded to ICI therapy (39, 40).
Taken together, a wide range of individual gut microbes have been associated with outcomes of ICI therapy, with Akkermansia and Faecalibacterium frequently linked to favorable responses across different cancers. However, there is no clear consensus, making it difficult to use these microbes as universally applicable biomarkers. Recently, Derosa et al. proposed a biomarker based on the ecological topology of the gut microbiome that predicts responses to ICI therapy in various cancers (41). Using co-abundance networks, they identified two species-interacting groups (SIGs) related to PD-1 blockade response in NSCLC patients. SIG1 comprised bacteria associated with reduced OS, such as the Enterocloster genus and Streptococcaceae, Veillonellaceae, and Lactobacillaceae families, while SIG2 consisted of longer OS-related bacteria, such as F. prausnitzii and Ruminococcus bicirculans. The topological score (TOPOSCORE), which is a combination of the SIG1/SIG2 ratio and A. muciniphila abundance, was significantly associated with immunotherapy outcome in the NSCLC discovery cohort and could predict patients’ responses to ICI therapy with an area under the receiver operating characteristic curve (AUC) of 0.66. The association of the TOPOSCORE with responses to ICI was also validated in patients with NSCLC and those with genitourinary cancer, suggesting the potential of TOPOSCORE as a robust biomarker for predicting immunotherapy response (41). These findings highlight the importance of considering not only individual bacteria but also bacterial interactions when predicting ICI responses based on the gut microbiome.
4 Machine learning models for predicting responses to ICI therapy based on the gut microbiome
The identification of significant associations between the gut microbiome and ICI response have led researchers to investigate machine learning models to stratify patients into responders and non-responders to immunotherapy using the gut microbial profiles before starting treatment (Table 3).
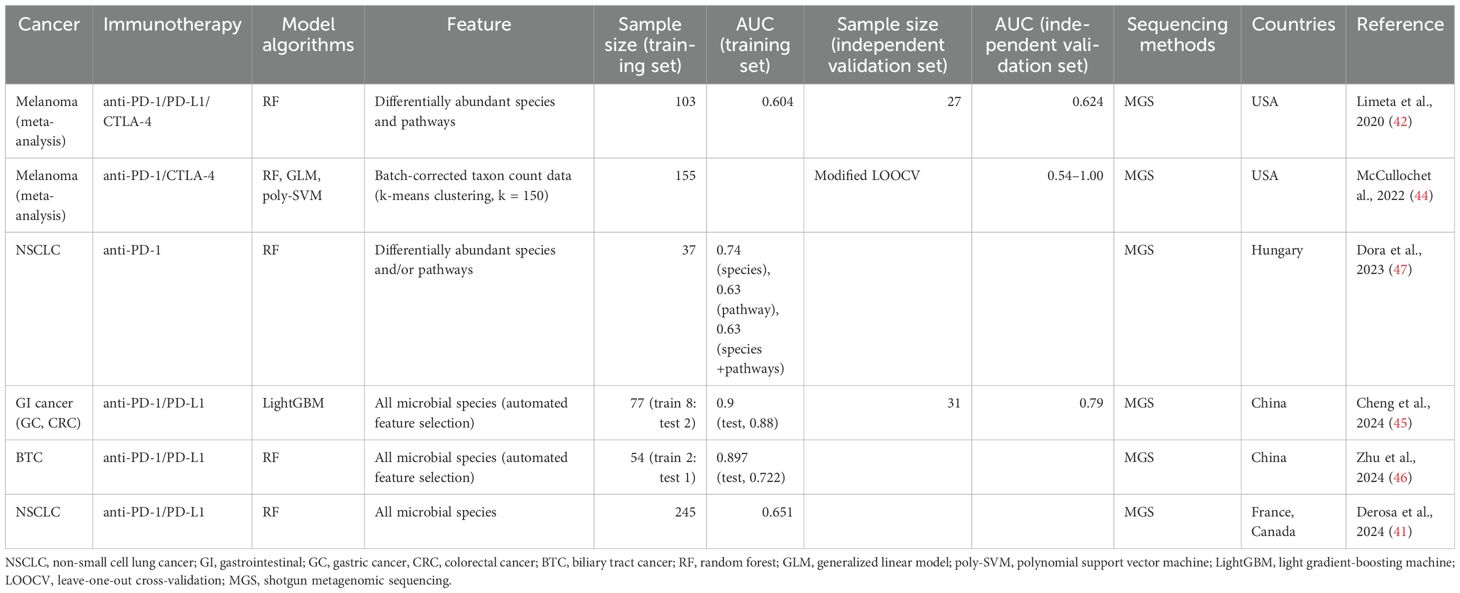
Table 3. Predictive machine learning models distinguishing responders from non-responders based on the gut microbiome.
Limeta et al. (42) developed a predictive model using three previously published gut microbiome datasets from 103 patients with melanoma undergoing ICI therapy (19, 20, 43). They used 49 differentially abundant species and functional pathways between responders and non-responders, including unknown Ruminococcaceae species, unknown Faecalibacterium species, Bacteroides thetaiotaomicron, and aerobic respiration, as input for training a random forest (RF) classifier. The AUC of the model on the training data was 0.604. They also evaluated the classification performance of the model on the independent validation dataset (n = 27) (35), achieving an AUC of 0.624 (42). McCullochet et al. (44) also developed machine learning models to predict the PD-1 response of patients with melanoma using 155 metagenomic datasets from a new cohort and four published independent cohorts (19, 20, 35, 43). In this study, batch-effect-corrected taxon count data were used to reduce the inter-study heterogeneity of metagenomic data, and a modified leave-one-out cross-validation method, where models were trained on the four cohorts and tested on the remaining cohort, was applied to overcome the limitation of small sample size in each cohort. Three machine learning algorithms, including RF, a generalized linear model, and a polynomial support vector machine, were used, and the resulting AUC values were between 0.54 and 1.00 (44). This study showed that machine learning models trained on gut microbiome data can consistently predict the efficacy of PD-1 therapy in patients with melanoma prior to ICI treatment.
A machine learning model for gastrointestinal (GI) cancer was developed using microbial species data from 18 patients with gastric cancer (GC) and 59 patients with colorectal cancer (CRC) undergoing anti-PD-1/PD-L1 treatment (45). The light gradient-boosting machine model showed high predictive performance (AUC of 0.90 in the training dataset), and the performance of this model was confirmed in the independent validation set (n = 31) with an AUC of 0.79. In this model, Bacteroides caccae, Veillonella parvula, V. atypica, and Clostridiales bacteria significantly contributed to distinguishing responders from non-responders (45). Recently, it was reported that the outcome of anti-PD-1/PD-L1 immunotherapy can be also predicted in biliary tract cancer (BTC) (46). This study used microbiome data from 77 patients with BTC and built three different RF models based on bacteria, metabolites, and a combination of bacteria and metabolites. The model trained only with six bacteria, including Streptococcus anginosus, Olsenella profusa, and A. putredinis, showed high predictive performance with an AUC of 0.897 for the training dataset and of 0.722 for the test dataset (46).
For NSCLC, the aforementioned study introducing the TOPOSCORE approach (41) also developed a machine learning model based on the relative abundance of all microbial species to compare the predictive performance of TOPOSCORE across 245 patients with NSCLC. The TOPOSCORE distinguished the responders and non-responders with an AUC of 0.66, while the RF model using microbiome data achieved an AUC of 0.651, indicating that these two approaches have similar performance in predicting the outcome of ICI therapy in patients with NSCLC (41). Another study including small number of patients with NSCLC (n = 37) also demonstrated RF models based on differentially abundant species and/or pathways between patients with long and short PFS. The model based on species could discriminate between long- and short-PFS patients with an AUC of 0.74 (47).
These models demonstrated moderate prediction performances, indicating the potential for gut microbial profiles to serve as markers for treatment response. Notably, the models for cancers of the GI system (GC, CRC, and BTC) exhibited higher predictive performances than those for other cancers, suggesting that ICI therapy for GI cancers may be more directly influenced by the gut microbiota. Given the complexity and variability of the gut microbiome, it is evident that further validation is necessary to enhance the clinical applicability of these predictive models.
5 Modulation of the gut microbiota to improve the efficacy of ICI therapy
5.1 Observational studies
The link between the gut microbiome and responses to ICI therapy suggests the potential to enhance the therapeutic efficacy by modulating the gut microbiota. Key strategies to do this include fecal microbiota transplantation (FMT), live biotherapeutic products (LBP: single taxa or microbial consortia), probiotics, prebiotics, postbiotics, and dietary modifications. Several observational studies have explored the relationship between probiotic or dietary fiber intake and outcomes of ICI therapy.
Interestingly, research on the effect of probiotic intake on the efficacy of solid tumor immunotherapy is underway in Japan with a particular focus on the Clostridium butyricum MIYAIRI 588 strain (CBM588). CBM588 is an anaerobic, butyrate-producing, Gram-positive bacteria widely used for the treatment of diarrhea in Japan (48). Recent studies have revealed that CBM588-based probiotics can promote the growth of Bifidobacterium and Lactobacillus, enhance the intestinal barriers, and modulate immune function and inflammation (49, 50). A retrospective study investigated whether the intake of strain CBM588 before and/or during ICI therapy affected survival and response to ICI in 118 Japanese patients with advanced NSCLC. Probiotic intake significantly prolonged PFS (250 days for probiotic users vs. 101 days for non-users) and OS (not reached vs. 361 days). The use of strain CBM588 was also associated with improved ICI efficacy in a subgroup analysis of patients who received antibiotic therapy prior to treatment initiation (51). Another study of 294 Japanese patients with NSCLC found that PFS was significantly longer in those taking probiotics, including Bifidobacterium (BIOFERMIN and LAC-B), CBM588 (MIYA-BM), and antibiotic-resistant lactic acid bacteria, at the time of anti-PD-1 therapy initiation compared to those not taking probiotics (52). Similarly, among 482 Japanese NSCLC patients receiving ICI monotherapy, those who took probiotics before or during ICI therapy had longer PFS (7.9 months for probiotic users vs. 2.9 months for non-users) and better OS (not reached vs. 13.1 months). In this study, the patients who took probiotics were further divided into two groups according to the probiotic types that they took, CBM588 vs non-spore-forming bacteria only, and no significant differences in PFS and OS depending on the probiotic types were observed (53). These studies indicate a potential role for probiotics in improving the efficacy of ICI therapy in NSCLC patients.
However, findings in melanoma patients are less conclusive. In a cohort of 158 melanoma patients, probiotic use was not significantly associated with outcomes of ICI therapy (54). In this study, all patients who reported any frequency of probiotic supplement use were designated as probiotic users, and they consumed various types of probiotic formulas and foods. Instead, patients who consumed sufficient dietary fiber (≥ 20 g/day) had longer PFS than those consuming less (not reached vs. 13 months) (n = 128). Notably, the most significant benefit was observed in patients with adequate dietary fiber intake and no probiotic use. Preclinical models of melanoma immunotherapy confirmed these results, highlighting the harmful effects of probiotics and the beneficial effects of dietary fiber on immunotherapy outcomes (54). The discrepancies in the effectiveness of probiotics across different studies may stem from variations in probiotic strains, administration duration, dosage, or cancer types, emphasizing the need for further research.
5.2 Intervention studies
In addition to observational studies, numerous clinical trials are underway to assess whether gut microbiota-targeted interventions can improve immunotherapy efficacy in cancer patients. Below, we summarize results from published clinical trials to date (Table 4).
Baruch et al. conducted a clinical trial (NCT03353402) investigating FMT in 10 patients with immunotherapy-refractory melanoma. FMT improved the response to anti-PD-1 immunotherapy in three patients. Interestingly, all responding patients received FMT from the same donor, whose gut microbiome had a high relative abundance of B. adolescentis (55). Another trial by Davar et al. (NCT03341143) in 15 patients with refractory melanoma found that six patients showed improved clinical responses to anti-PD-1 therapy after FMT. Responders demonstrated a gut microbiome shift toward the donor’s profile, increased CD8+ T cell activation, and decreased interleukin (IL)-8-expressing myeloid cells (56). FMT combined with ICI also showed promising results in a trial with 20 previously untreated patients with advanced melanoma (NCT03772899), where 65% of patients (13/20) achieved a response. In responders, donor–recipient gut microbiome similarity increased over time (57). A recent trial (NCT04264975) examined the combined therapeutic effect of anti-PD-1 and FMT on 13 non-responders to anti-PD-(L)1 therapy with advanced solid tumors, including GC, HCC, or esophageal squamous cell carcinoma (58). Clinical benefits were observed, with an ORR of 7.7% and a disease control rate of 46.2%. In the study, the authors isolated Prevotella merdae Immunoactis from a responder to FMT and found that it promoted T-cell activity and suppressed tumor growth (58). These findings suggest FMT could be a viable strategy to enhance the efficacy of solid cancer immunotherapy.
In the previous section, we discussed several retrospective studies associating the use of CBM588 before and/or during ICI therapy with better therapeutic outcomes in patients with NSCLC (51–53). In line with the results of the retrospective studies, two phase 1 clinical trials using CBM588 have shown encouraging results (59, 60). In treatment-naïve patients with metastatic RCC (mRCC), the addition of CBM588 to nivolumab–ipilimumab therapy significantly improved PFS compared to controls (12.7 months vs. 2.5 months) (NCT03829111) (59). Another trial added CBM588 to cabozantinib (a vascular endothelial growth factor receptor inhibitor) and nivolumab therapy in treatment-naïve mRCC patients, leading to a significantly higher ORR (74% vs. 20%) (NCT05122546) (60). In these two trials, the patients in the experimental arm received CBM588 at a dose of 80 mg orally twice daily in addition to ICI therapy, where each 40 mg sachet consisted of approximately 2.0 × 108 colony-forming units of C. butyricum and pharmaceutical excipients such as corn starch, calcium carbonate, and lactose. These studies indicate that CBM588 may enhance ICI efficacy in mRCC, though larger trials are necessary to validate these results.
Recently, Glitza et al. reported a phase 1 trial (NCT03817125) involving patients with ICI-naïve metastatic melanoma treated with SER-401 (61). SER-401 is a proprietary formulation of bacterial spores from Ruminococcaceae and other spore-forming microbes derived from a stool sample provided by a healthy donor with a gut microbial profile similar to that of immunotherapy responders (19). In this trial, the patients in the SER-401 arm received vancomycin preconditioning followed by SER-401 and then nivolumab with SER-401 maintenance, while the patients in the placebo arm received placebo preconditioning followed by placebo microbiota modulation and then nivolumab with placebo maintenance. This trial was initiated before studies connected antibiotic use before ICI treatment with worse responses to therapy (26, 61), and vancomycin preconditioning was used to prime the gut microbiota for the expansion of SER-401 bacteria in the intestine. In terms of safety and response, the combination of SER-401 and nivolumab was safe, but the ORR in the SER-401 group was lower than in the placebo group (25% vs. 66.7%). In the SER-401 group, the relative abundance of Ruminococcaceae was significantly reduced after vancomycin preconditioning, and it was restored to near-baseline levels after the administration of SER-401. However, no further increase occurred, indicating the suboptimal colonization of target species. Vancomycin preconditioning led to a decrease in the enrichment of ICI response-associated pathways (e.g., butyrate biosynthesis pathway) and an increase in that of ICI resistance-associated pathways in the gut microbiome of the SER-401 group. In addition, antibiotic treatments increased the proportion of peripheral blood mononuclear cell-derived Tregs and circulating serum proteins, which are involved in cellular stress/death, T-cell receptor (TCR) signaling, and Toll-like receptor (TLR) signaling, indicating increased systemic inflammation (61). Several meta-analyses have reported that a high systemic immune inflammatory index or neutrophil-to-lymphocyte ratio (NLR > 3.0), as quantitative measurements of systemic inflammation in the human body, are associated with worse clinical outcomes of OS and PFS in patients with cancer who receive ICI treatment (62, 63). Additionally, in melanoma patients treated with anti-PD-1, the gut microbiome of non-responders was enriched with Gram-negative bacteria and lipopolysaccharide synthesis-related microbial genes, and the host genes encoding pro-inflammatory cytokines were more highly expressed in stool specimens from non-responders than in those from responders. Also, patients with a high NLR had shorter OS and PFS than those with a low NLR (44). Vancomycin, which was used for antibiotic preconditioning in the SER-401 trial, is not effective against Gram-negative bacteria (64). Thus, vancomycin preconditioning might have hindered efforts to determine whether SER-401 could enhance the efficacy of ICI therapy, as it can affect the outcomes of ICI therapy by inducing changes in the intestinal environment and systemic immune responses. This underscores the importance of carefully considering preconditioning regimens when designing clinical trials.
6 Mechanisms by which the gut microbiota influences ICI responses
Applications using the gut microbiota to enhance ICI therapy outcomes are of considerable clinical interest. However, a comprehensive understanding of the molecular mechanisms underlying this effect is crucial for deepening our knowledge and developing more effective strategies to harness the gut microbiota for ICI therapy. In this context, we will briefly explore the molecular mechanisms by which the gut microbiota may impact the efficacy of ICI therapy, with a particular focus on the bacterial cell components and metabolites.
6.1 Bacterial cell components
Mechanistic studies of Enterococcus and Bacteroides have revealed the involvement of microbe-associated molecular patterns in enhancing the efficacy of ICI therapy. For instance, immunotherapy-active Enterococcus strains secrete the peptidoglycan hydrolase secreted antigen A, which can generate muramyl dipeptide (MDP) peptidoglycan fragments by breaking down the bacterial cell wall. These MDP fragments activate nucleotide-binding oligomerization domain 2-dependent signaling pathways, induce the expression of pro-inflammatory genes, and promote cytotoxic T-lymphocyte (CTL) activation within the tumor microenvironment, consequently resulting in the enhancement of anti-PD-L1 antitumor efficacy (65). Vétizou et al. showed that the administration of B. fragilis-purified polysaccharide in antibiotic-treated mice could improve the efficacy of CTLA-4 blockade by inducing IL-12-dependent Th1 immune responses. However, a polysaccharide purified from another Bacteroides species, B. distasonis, showed lower efficacy in reducing tumor size than that from B. fragilis (17). These results indicate that bacterial cell components may improve the efficacy of ICI therapy in a species- or strain-specific manner.
Another type of microbial component, cyclic diadenylate AMP (cdAMP), may also modulate ICI responses. Akkermansia-derived cdAMP can act as stimulator of interferon genes (STING) agonist in the tumor microenvironment and improve the antitumor response by inducing IFN-I production and activating NK cells (66). A combination of anti-PD-1 and a synthetic STING agonist, MSA-2, led to significantly inhibited tumor growth compared to that with anti-PD-1 monotherapy in mouse tumor models by inducing CD8+ T-cell infiltration in tumors (67). These findings provide some insight into the mechanism by which Akkermansia may promote ICI response.
6.2 SCFAs
SCFAs (butyrate, acetate, and propionate) are major metabolites produced by the gut microbiota and play various roles in regulating host physiology. In particular, SCFAs heavily modulate the host immune system by regulating intestinal barrier integrity as well as innate immune cells (e.g., neutrophils, macrophages, and DCs) and adaptive immune cells (e.g., Tregs, Th cells, and CTLs) (68). Preclinical and clinical studies have also reported that SCFAs are involved in ICI responses. In patients with solid tumors, responders to anti-PD-1 inhibitor therapy had higher levels of serum and fecal SCFAs than non-responders (69, 70). In melanoma-bearing mouse models, butyrate supplementation with anti-PD-1 therapy significantly reduced tumor volume and increased the percentage of IFN-γ+ and tumor necrosis factor (TNF)-α+ cells among tumor infiltrating CD4+ and CD8+ T cells. In addition, in vitro experiments showed that butyrate promoted the production of antitumor-related cytokines such as IFN-γ, TNF-α, and IL-2 in CD8+ T cells through the TCR-dependent signaling pathway (70). Among gut microbiota, microbes belonging to the Clostridium cluster, such as Faecalibacterium, Ruminococcus, and Roseburia, are major producers of butyrate (71). Kang et al. found that R. intestinalis abundance was significantly higher in responders to anti-PD-1 therapy than in non-responders among patients with NSCLC. Moreover, in a mouse model of CRC with the microsatellite stable phenotype, R. intestinalis or sodium butyrate improved anti-PD-1 efficacy by enhancing cytotoxic CD8+ T-cell function via TLR5-dependent NF-κB pathway activation (72). However, there are conflicting findings regarding the effect of SCFAs on ICI efficacy. Coutzac et al. reported that in patients with metastatic melanoma, low baseline serum butyrate levels were associated with better outcomes of ICI therapy. In mice, sodium butyrate inhibited the anti-CTLA-4-induced maturation of DCs and accumulation of tumor-specific T cells and memory T cells and diminished the antitumor effect of CTLA-4 blockade treatment (73). Therefore, further studies are needed on the role and mechanisms of action of SCFAs in modulating cancer immunity and ICI efficacy for various doses and cancer types.
6.3 Inosine
In murine tumor models, Bifidobacterium pseudolongum significantly improved the therapeutic efficacy of ICI treatment. Inosine, derived from B. pseudolongum, was identified as a key metabolite contributing to this effect. Mechanistically, inosine binds to the adenosine A2A receptor on T cells, which promotes IL12Rβ2 and IFN-γ transcription and Th1-cell activation (74). In addition to B. pseudolongum, A. muciniphila can produce inosine via inosine–A2A receptor signaling, enhancing the efficacy of ICI treatment (74).
6.4 Tryptophan metabolites
Tryptophan metabolites from gut microbes have been studied for their role in immunotherapy responses. Indole-3-carboxylic acid (ICA), produced by Lactobacillus gallinarum, inhibits indoleamine 2,3-dioxygenase expression and kynurenine (Kyn) production in tumors. ICA also inhibits Kyn binding to the aryl hydrocarbon receptor in T cells, reducing Treg differentiation and improving anti-PD-1 therapy response in CRC (75). Additionally, ICA from Lactobacillus johnsonii has been shown to promote the generation of progenitor-exhausted CD8+ T cells, significantly improving the efficacy of CD8+ T cell-mediated anti-PD-1 immunotherapy across multiple cancer types (76).
6.5 Secondary bile acids
Gut microbe-produced secondary bile acids can directly regulate adaptive immune cells. For example, 3-oxolithocholic acid inhibits Th17 cell differentiation by binding to retinoid-related orphan receptor-γt, while isoallolithocholic acid enhances Treg cell differentiation by inducing mitochondrial reactive oxygen species production in vitro. These effects were also confirmed in mouse models, indicating that these bile acids may have a detrimental effect on immunotherapy response (77). Another study revealed that deoxycholic acid (DCA) suppresses CD8+ T cell responses by inhibiting Ca2+-nuclear factor of activated T cells 2 signaling, promoting tumor growth in mice. Disrupting DCA could mitigate tumor growth (78).
These studies underline the immunological connection between the gut microbiota and cancer, suggesting that specific gut microbes, their components, and metabolites could improve immunotherapy responses. However, the exact mechanisms by which the gut microbiota influences cancer progression and immunotherapy outcomes remain to be fully elucidated.
7 Discussion
The reviewed studies make it evident that the gut microbiota plays a significant role in influencing patients’ responses to ICI therapy. While the potential for utilizing the gut microbiome to predict or enhance outcomes of ICI therapy is promising, several challenges must be addressed before it can be widely implemented in clinical practice.
A recurring issue is the lack of consensus regarding gut microbial features associated with responses to ICI therapy. Many studies have identified specific microbes which were enriched in responders and non-responders to ICI treatment in various types of solid cancer, but an individual’s gut microbial compositions are influenced by numerous intrinsic and extrinsic factors such as age, diet, geography, medication, and co-morbidities (79). These factors may results in differences in ICI response-related microbes among the study populations. Therefore, large-scale research projects including diverse populations are needed to identify robust biomarkers applicable to diverse populations. It is also important to collect comprehensive patient metadata such as antibiotic use, co-morbidities, co-medications, and dietary records. These metadata can be used to account for confounding factors in statistical analyses and to identify ICI response-associated features specific to certain subgroups.
Studies investigating gut microbiome-based machine learning models to predict patient responses to ICI therapy have shown promising results. To determine whether a developed model can be applied to other populations, the predictive performance of the model should be evaluated in independent validation cohorts (80). However, it may be inappropriate to use microbiome data produced using different protocols for sample collection, storage, processing, and/or sequencing for validation, as these differences can introduce systemic bias in sequence-based microbiome data (81). Therefore, standardized protocols for microbiome analysis are required in this research area. McCulloch et al. used an empirical Bayesian framework to reduce between-cohort heterogeneity when integrating and analyzing microbiome data from five independent cohorts (44). This method may represent a solution that enables data produced by various protocols to be utilized in the creation and validation of predictive models. Additionally, most predictive models reported to date have been generated using only gut microbiome information. Research is also needed to determine whether adding other known biomarkers for responses to ICI therapy—such as tumor mutational burden and PD-L1 expression—or other omics data can improve the predictive performance of machine learning models.
The gut microbiota holds potential as a modifiable target to enhance the efficacy of ICI therapy. Gut microbes enriched in responders could serve as candidates for LBPs to improve treatment outcomes. Given that strains from the same species can have different functional capabilities (82), high-resolution microbiome studies are necessary to identify specific strains with the greatest potential for boosting the therapeutic efficacy. Additionally, verifying the functional characteristics of candidate microbes through in vitro and in vivo experiments is critical.
One challenge in gut microbiota interventions is the pre-existing microbial environment. As demonstrated by the SER-401 trial, where the effectiveness of ICI therapy was influenced by antibiotic preconditioning (61), the gut microbial dysbiosis induced by antibiotic treatment before intervention may impact the intervention outcome. Among the four FMT clinical trials mentioned in the previous section, those by Baruch et al. and Kim et al. included the administration of oral antibiotics before FMT treatment for 3 and 5 days, respectively, to remove the native gut microbiota (55, 58). However, the studies by Davar et al. and Routy et al. did not include any antibiotic treatment prior to FMT (56, 57). As these four FMT studies have shown the clinical benefits of FMT combined with ICI therapy regardless of antibiotic preconditioning, the procedure for native microbiota depletion may be omitted in combined treatment using ICIs and gut microbiota manipulation. Further research is needed to determine preconditioning regimens for preparing the gut microbial environment to maximize the effect of the combination therapy. In addition, understanding the relationship between the baseline gut microbial composition and intervention outcomes could help develop microbiome-based stratification strategies for patient treatment.
In addition to FMT and LBPs, ongoing clinical studies are investigating the role of diet and prebiotics in modulating the gut microbiota to enhance the efficacy of ICI therapy. However, our understanding of the mechanisms through which the gut microbiota influences ICI therapy outcomes is still limited. Therefore, large-scale observational and intervention studies, combined with mechanistic investigations, are essential for advancing gut microbiome-based personalized therapeutic strategies applicable in real-world clinical settings.
Author contributions
ML: Conceptualization, Data curation, Funding acquisition, Investigation, Visualization, Writing – original draft, Writing – review & editing. SH: Data curation, Investigation, Visualization, Writing – original draft, Writing – review & editing. Y-DN: Conceptualization, Writing – review & editing, Funding acquisition.
Funding
The author(s) declare financial support was received for the research, authorship, and/or publication of this article. This research was supported by the Main Research Program (E0170600-08) of the Korea Food Research Institute (KFRI) funded by the Ministry of Science and ICT, and the Bio&Medical Technology Development Program of the National Research Foundation (NRF) funded by the Korean government (MSIT) (No. NRF-2024M3A9E4036869).
Conflict of interest
The authors declare that the research was conducted in the absence of any commercial or financial relationships that could be construed as a potential conflict of interest.
Generative AI statement
The author(s) declare that no Generative AI was used in the creation of this manuscript.
Publisher’s note
All claims expressed in this article are solely those of the authors and do not necessarily represent those of their affiliated organizations, or those of the publisher, the editors and the reviewers. Any product that may be evaluated in this article, or claim that may be made by its manufacturer, is not guaranteed or endorsed by the publisher.
References
1. Couzin-Frankel J. Breakthrough of the year 2013. Cancer Immunother Sci. (2013) 342:1432–3. doi: 10.1126/science.342.6165.1432
2. Tan S, Li D, Zhu X. Cancer immunotherapy: pros, cons and beyond. BioMed Pharmacother. (2020) 124:109821. doi: 10.1016/j.biopha.2020.109821
3. Wang DR, Wu XL, Sun YL. Therapeutic targets and biomarkers of tumor immunotherapy: response versus non-response. Signal Transduct Target Ther. (2022) 7:331. doi: 10.1038/s41392-022-01136-2
4. Meng L, Wu H, Wu J, Ding P, He J, Sang M, et al. Mechanisms of immune checkpoint inhibitors: insights into the regulation of circular rnas involved in cancer hallmarks. Cell Death Dis. (2024) 15:3. doi: 10.1038/s41419-023-06389-5
5. He X, Xu C. Immune checkpoint signaling and cancer immunotherapy. Cell Res. (2020) 30:660–9. doi: 10.1038/s41422-020-0343-4
6. Garon EB, Hellmann MD, Rizvi NA, Carcereny E, Leighl NB, Ahn MJ, et al. Five-year overall survival for patients with advanced non-Small-cell lung cancer treated with pembrolizumab: results from the phase I keynote-001 study. J Clin Oncol. (2019) 37:2518–27. doi: 10.1200/JCO.19.00934
7. Pilard C, Ancion M, Delvenne P, Jerusalem G, Hubert P, Herfs M. Cancer immunotherapy: it’s time to better predict patients’ Response. Br J Cancer. (2021) 125:927–38. doi: 10.1038/s41416-021-01413-x
8. Lu S, Stein JE, Rimm DL, Wang DW, Bell JM, Johnson DB, et al. Comparison of biomarker modalities for predicting response to pd-1/pd-L1 checkpoint blockade: A systematic review and meta-analysis. JAMA Oncol. (2019) 5:1195–204. doi: 10.1001/jamaoncol.2019.1549
9. Zimmermann M, Zimmermann-Kogadeeva M, Wegmann R, Goodman AL. Mapping human microbiome drug metabolism by gut bacteria and their genes. Nature. (2019) 570:462–7. doi: 10.1038/s41586-019-1291-3
10. Weersma RK, Zhernakova A, Fu J. Interaction between drugs and the gut microbiome. Gut. (2020) 69:1510–9. doi: 10.1136/gutjnl-2019-320204
11. Cai J, Auster A, Cho S, Lai Z. Dissecting the human gut microbiome to better decipher drug liability: A once-forgotten organ takes center stage. J Adv Res. (2023) 52:171–201. doi: 10.1016/j.jare.2023.07.002
12. Rooks MG, Garrett WS. Gut microbiota, metabolites and host immunity. Nat Rev Immunol. (2016) 16:341–52. doi: 10.1038/nri.2016.42
13. Behary J, Amorim N, Jiang XT, Raposo A, Gong L, McGovern E, et al. Gut microbiota impact on the peripheral immune response in non-alcoholic fatty liver disease related hepatocellular carcinoma. Nat Commun. (2021) 12:187. doi: 10.1038/s41467-020-20422-7
14. Radojevic D, Tomic S, Mihajlovic D, Tolinacki M, Pavlovic B, Vucevic D, et al. Fecal microbiota composition associates with the capacity of human peripheral blood monocytes to differentiate into immunogenic dendritic cells in vitro. Gut Microbes. (2021) 13:1–20. doi: 10.1080/19490976.2021.1921927
15. Bachem A, Makhlouf C, Binger KJ, de Souza DP, Tull D, Hochheiser K, et al. Microbiota-derived short-chain fatty acids promote the memory potential of antigen-activated cd8(+) T cells. Immunity. (2019) 51:285–97 e5. doi: 10.1016/j.immuni.2019.06.002
16. Trompette A, Gollwitzer ES, Pattaroni C, Lopez-Mejia IC, Riva E, Pernot J, et al. Dietary fiber confers protection against flu by shaping ly6c(-) patrolling monocyte hematopoiesis and cd8(+) T cell metabolism. Immunity. (2018) 48:992–1005 e8. doi: 10.1016/j.immuni.2018.04.022
17. Vetizou M, Pitt JM, Daillere R, Lepage P, Waldschmitt N, Flament C, et al. Anticancer immunotherapy by ctla-4 blockade relies on the gut microbiota. Science. (2015) 350:1079–84. doi: 10.1126/science.aad1329
18. Derosa L, Hellmann MD, Spaziano M, Halpenny D, Fidelle M, Rizvi H, et al. Negative association of antibiotics on clinical activity of immune checkpoint inhibitors in patients with advanced renal cell and non-small-cell lung cancer. Ann Oncol. (2018) 29:1437–44. doi: 10.1093/annonc/mdy103
19. Gopalakrishnan V, Spencer CN, Nezi L, Reuben A, Andrews MC, Karpinets TV, et al. Gut microbiome modulates response to anti-pd-1 immunotherapy in melanoma patients. Science. (2018) 359:97–103. doi: 10.1126/science.aan4236
20. Matson V, Fessler J, Bao R, Chongsuwat T, Zha Y, Alegre ML, et al. The commensal microbiome is associated with anti-pd-1 efficacy in metastatic melanoma patients. Science. (2018) 359:104–8. doi: 10.1126/science.aao3290
21. Fishbein SRS, Mahmud B, Dantas G. Antibiotic perturbations to the gut microbiome. Nat Rev Microbiol. (2023) 21:772–88. doi: 10.1038/s41579-023-00933-y
22. Levy M, Kolodziejczyk AA, Thaiss CA, Elinav E. Dysbiosis and the immune system. Nat Rev Immunol. (2017) 17:219–32. doi: 10.1038/nri.2017.7
23. Marra F, Marra CA, Richardson K, Lynd LD, Kozyrskyj A, Patrick DM, et al. Antibiotic use in children is associated with increased risk of asthma. Pediatrics. (2009) 123:1003–10. doi: 10.1542/peds.2008-1146
24. Kim DH, Han K, Kim SW. Effects of antibiotics on the development of asthma and other allergic diseases in children and adolescents. Allergy Asthma Immunol Res. (2018) 10:457–65. doi: 10.4168/aair.2018.10.5.457
25. Sultan AA, Mallen C, Muller S, Hider S, Scott I, Helliwell T, et al. Antibiotic use and the risk of rheumatoid arthritis: A population-based case-control study. BMC Med. (2019) 17:154. doi: 10.1186/s12916-019-1394-6
26. Routy B, Le Chatelier E, Derosa L, Duong CPM, Alou MT, Daillere R, et al. Gut microbiome influences efficacy of pd-1-based immunotherapy against epithelial tumors. Science. (2018) 359:91–7. doi: 10.1126/science.aan3706
27. Kim H, Lee JE, Hong SH, Lee MA, Kang JH, Kim IH. The effect of antibiotics on the clinical outcomes of patients with solid cancers undergoing immune checkpoint inhibitor treatment: A retrospective study. BMC Cancer. (2019) 19:1100. doi: 10.1186/s12885-019-6267-z
28. Nyein AF, Bari S, Hogue S, Zhao Y, Maller B, Sha S, et al. Effect of prior antibiotic or chemotherapy treatment on immunotherapy response in non-small cell lung cancer. BMC Cancer. (2022) 22:101. doi: 10.1186/s12885-022-09210-2
29. Luo WC, Mei SQ, Huang ZJ, Chen ZH, Zhang YC, Yang MY, et al. Correlation of distribution characteristics and dynamic changes of gut microbiota with the efficacy of immunotherapy in egfr-mutated non-small cell lung cancer. J Transl Med. (2024) 22:326. doi: 10.1186/s12967-024-05135-5
30. Lurienne L, Cervesi J, Duhalde L, de Gunzburg J, Andremont A, Zalcman G, et al. Nsclc immunotherapy efficacy and antibiotic use: A systematic review and meta-analysis. J Thorac Oncol. (2020) 15:1147–59. doi: 10.1016/j.jtho.2020.03.002
31. Zhou J, Huang G, Wong WC, Hu DH, Zhu JW, Li R, et al. The impact of antibiotic use on clinical features and survival outcomes of cancer patients treated with immune checkpoint inhibitors. Front Immunol. (2022) 13:968729. doi: 10.3389/fimmu.2022.968729
32. Jin Y, Dong H, Xia L, Yang Y, Zhu Y, Shen Y, et al. The diversity of gut microbiome is associated with favorable responses to anti-programmed death 1 immunotherapy in chinese patients with nsclc. J Thorac Oncol. (2019) 14:1378–89. doi: 10.1016/j.jtho.2019.04.007
33. Derosa L, Routy B, Thomas AM, Iebba V, Zalcman G, Friard S, et al. Intestinal akkermansia muciniphila predicts clinical response to pd-1 blockade in patients with advanced non-small-cell lung cancer. Nat Med. (2022) 28:315–24. doi: 10.1038/s41591-021-01655-5
34. Newsome RC, Gharaibeh RZ, Pierce CM, da Silva WV, Paul S, Hogue SR, et al. Interaction of bacterial genera associated with therapeutic response to immune checkpoint pd-1 blockade in a United States cohort. Genome Med. (2022) 14:35. doi: 10.1186/s13073-022-01037-7
35. Peters BA, Wilson M, Moran U, Pavlick A, Izsak A, Wechter T, et al. Relating the gut metagenome and metatranscriptome to immunotherapy responses in melanoma patients. Genome Med. (2019) 11:61. doi: 10.1186/s13073-019-0672-4
36. Andrews MC, Duong CPM, Gopalakrishnan V, Iebba V, Chen WS, Derosa L, et al. Gut microbiota signatures are associated with toxicity to combined ctla-4 and pd-1 blockade. Nat Med. (2021) 27:1432–41. doi: 10.1038/s41591-021-01406-6
37. Salgia NJ, Bergerot PG, Maia MC, Dizman N, Hsu J, Gillece JD, et al. Stool microbiome profiling of patients with metastatic renal cell carcinoma receiving anti-pd-1 immune checkpoint inhibitors. Eur Urol. (2020) 78:498–502. doi: 10.1016/j.eururo.2020.07.011
38. Derosa L, Routy B, Fidelle M, Iebba V, Alla L, Pasolli E, et al. Gut bacteria composition drives primary resistance to cancer immunotherapy in renal cell carcinoma patients. Eur Urol. (2020) 78:195–206. doi: 10.1016/j.eururo.2020.04.044
39. Lee PC, Wu CJ, Hung YW, Lee CJ, Chi CT, Lee IC, et al. Gut microbiota and metabolites associate with outcomes of immune checkpoint inhibitor-treated unresectable hepatocellular carcinoma. J Immunother Cancer. (2022) 10:e004779. doi: 10.1136/jitc-2022-004779
40. Mao J, Wang D, Long J, Yang X, Lin J, Song Y, et al. Gut microbiome is associated with the clinical response to anti-pd-1 based immunotherapy in hepatobiliary cancers. J Immunother Cancer. (2021) 9:e003334. doi: 10.1136/jitc-2021-003334
41. Derosa L, Iebba V, Silva CAC, Piccinno G, Wu G, Lordello L, et al. Custom scoring based on ecological topology of gut microbiota associated with cancer immunotherapy outcome. Cell. (2024) 187:3373–89 e16. doi: 10.1016/j.cell.2024.05.029
42. Limeta A, Ji B, Levin M, Gatto F, Nielsen J. Meta-analysis of the gut microbiota in predicting response to cancer immunotherapy in metastatic melanoma. JCI Insight. (2020) 5:e140940. doi: 10.1172/jci.insight.140940
43. Frankel AE, Coughlin LA, Kim J, Froehlich TW, Xie Y, Frenkel EP, et al. Metagenomic shotgun sequencing and unbiased metabolomic profiling identify specific human gut microbiota and metabolites associated with immune checkpoint therapy efficacy in melanoma patients. Neoplasia. (2017) 19:848–55. doi: 10.1016/j.neo.2017.08.004
44. McCulloch JA, Davar D, Rodrigues RR, Badger JH, Fang JR, Cole AM, et al. Intestinal microbiota signatures of clinical response and immune-related adverse events in melanoma patients treated with anti-pd-1. Nat Med. (2022) 28:545–56. doi: 10.1038/s41591-022-01698-2
45. Cheng S, Han Z, Dai D, Li F, Zhang X, Lu M, et al. Multi-omics of the gut microbial ecosystem in patients with microsatellite-instability-high gastrointestinal cancer resistant to immunotherapy. Cell Rep Med. (2024) 5:101355. doi: 10.1016/j.xcrm.2023.101355
46. Zhu C, Wang Y, Zhu R, Wang S, Xue J, Zhang D, et al. Gut microbiota and metabolites signatures of clinical response in anti-pd-1/pd-L1 based immunotherapy of biliary tract cancer. biomark Res. (2024) 12:56. doi: 10.1186/s40364-024-00607-8
47. Dora D, Ligeti B, Kovacs T, Revisnyei P, Galffy G, Dulka E, et al. Non-small cell lung cancer patients treated with anti-pd1 immunotherapy show distinct microbial signatures and metabolic pathways according to progression-free survival and pd-L1 status. Oncoimmunology. (2023) 12:2204746. doi: 10.1080/2162402X.2023.2204746
48. Seki H, Shiohara M, Matsumura T, Miyagawa N, Tanaka M, Komiyama A, et al. Prevention of antibiotic-associated diarrhea in children by clostridium butyricum miyairi. Pediatr Int. (2003) 45:86–90. doi: 10.1046/j.1442-200x.2003.01671.x
49. Hagihara M, Kuroki Y, Ariyoshi T, Higashi S, Fukuda K, Yamashita R, et al. Clostridium butyricum modulates the microbiome to protect intestinal barrier function in mice with antibiotic-induced dysbiosis. iScience. (2020) 23:100772. doi: 10.1016/j.isci.2019.100772
50. Hagihara M, Ariyoshi T, Kuroki Y, Eguchi S, Higashi S, Mori T, et al. Clostridium butyricum enhances colonization resistance against clostridioides difficile by metabolic and immune modulation. Sci Rep. (2021) 11:15007. doi: 10.1038/s41598-021-94572-z
51. Tomita Y, Ikeda T, Sakata S, Saruwatari K, Sato R, Iyama S, et al. Association of probiotic clostridium butyricum therapy with survival and response to immune checkpoint blockade in patients with lung cancer. Cancer Immunol Res. (2020) 8:1236–42. doi: 10.1158/2326-6066.CIR-20-0051
52. Takada K, Shimokawa M, Takamori S, Shimamatsu S, Hirai F, Tagawa T, et al. Clinical impact of probiotics on the efficacy of anti-pd-1 monotherapy in patients with nonsmall cell lung cancer: A multicenter retrospective survival analysis study with inverse probability of treatment weighting. Int J Cancer. (2021) 149:473–82. doi: 10.1002/ijc.33557
53. Morita A, Ichihara E, Inoue K, Fujiwara K, Yokoyama T, Harada D, et al. Impacts of probiotics on the efficacies of immune checkpoint inhibitors with or without chemotherapy for patients with advanced non-small-cell lung cancer. Int J Cancer. (2024) 154:1607–15. doi: 10.1002/ijc.34842
54. Spencer CN, McQuade JL, Gopalakrishnan V, McCulloch JA, Vetizou M, Cogdill AP, et al. Dietary fiber and probiotics influence the gut microbiome and melanoma immunotherapy response. Science. (2021) 374:1632–40. doi: 10.1126/science.aaz7015
55. Baruch EN, Youngster I, Ben-Betzalel G, Ortenberg R, Lahat A, Katz L, et al. Fecal microbiota transplant promotes response in immunotherapy-refractory melanoma patients. Science. (2021) 371:602–9. doi: 10.1126/science.abb5920
56. Davar D, Dzutsev AK, McCulloch JA, Rodrigues RR, Chauvin JM, Morrison RM, et al. Fecal microbiota transplant overcomes resistance to anti-pd-1 therapy in melanoma patients. Science. (2021) 371:595–602. doi: 10.1126/science.abf3363
57. Routy B, Lenehan JG, Miller WH Jr., Jamal R, Messaoudene M, Daisley BA, et al. Fecal microbiota transplantation plus anti-pd-1 immunotherapy in advanced melanoma: A phase I trial. Nat Med. (2023) 29:2121–32. doi: 10.1038/s41591-023-02453-x
58. Kim Y, Kim G, Kim S, Cho B, Kim SY, Do EJ, et al. Fecal microbiota transplantation improves anti-pd-1 inhibitor efficacy in unresectable or metastatic solid cancers refractory to anti-pd-1 inhibitor. Cell Host Microbe. (2024) 32:1380–93 e9. doi: 10.1016/j.chom.2024.06.010
59. Dizman N, Meza L, Bergerot P, Alcantara M, Dorff T, Lyou Y, et al. Nivolumab plus ipilimumab with or without live bacterial supplementation in metastatic renal cell carcinoma: A randomized phase 1 trial. Nat Med. (2022) 28:704–12. doi: 10.1038/s41591-022-01694-6
60. Ebrahimi H, Dizman N, Meza L, Malhotra J, Li X, Dorff T, et al. Cabozantinib and nivolumab with or without live bacterial supplementation in metastatic renal cell carcinoma: A randomized phase 1 trial. Nat Med. (2024) 30:2576–85. doi: 10.1038/s41591-024-03086-4
61. Glitza IC, Seo YD, Spencer CN, Wortman JR, Burton EM, Alayli FA, et al. Randomized placebo-controlled, biomarker-stratified phase ib microbiome modulation in melanoma: impact of antibiotic preconditioning on microbiome and immunity. Cancer Discovery. (2024) 14:1161–75. doi: 10.1158/2159-8290.CD-24-0066
62. Kou J, Huang J, Li J, Wu Z, Ni L. Systemic immune-inflammation index predicts prognosis and responsiveness to immunotherapy in cancer patients: A systematic review and meta−Analysis. Clin Exp Med. (2023) 23:3895–905. doi: 10.1007/s10238-023-01035-y
63. Zheng M. Systemic inflammation shapes clinical outcomes in response to immune checkpoint blockade treatment: moving toward optimizing antitumor immunity. J Immunother Cancer. (2023) 11:e006462. doi: 10.1136/jitc-2022-006462
64. Nikaido H. Outer membrane barrier as a mechanism of antimicrobial resistance. Antimicrob Agents Chemother. (1989) 33:1831–6. doi: 10.1128/AAC.33.11.1831
65. Griffin ME, Espinosa J, Becker JL, Luo JD, Carroll TS, Jha JK, et al. Enterococcus peptidoglycan remodeling promotes checkpoint inhibitor cancer immunotherapy. Science. (2021) 373:1040–6. doi: 10.1126/science.abc9113
66. Lam KC, Araya RE, Huang A, Chen Q, Di Modica M, Rodrigues RR, et al. Microbiota triggers sting-type I ifn-dependent monocyte reprogramming of the tumor microenvironment. Cell. (2021) 184:5338–56 e21. doi: 10.1016/j.cell.2021.09.019
67. Pan BS, Perera SA, Piesvaux JA, Presland JP, Schroeder GK, Cumming JN, et al. An orally available non-nucleotide sting agonist with antitumor activity. Science. (2020) 369:eaba6098. doi: 10.1126/science.aba6098
68. Deleu S, Machiels K, Raes J, Verbeke K, Vermeire S. Short chain fatty acids and its producing organisms: an overlooked therapy for ibd? EBioMedicine. (2021) 66:103293. doi: 10.1016/j.ebiom.2021.103293
69. Nomura M, Nagatomo R, Doi K, Shimizu J, Baba K, Saito T, et al. Association of short-chain fatty acids in the gut microbiome with clinical response to treatment with nivolumab or pembrolizumab in patients with solid cancer tumors. JAMA Netw Open. (2020) 3:e202895. doi: 10.1001/jamanetworkopen.2020.2895
70. Zhu X, Li K, Liu G, Wu R, Zhang Y, Wang S, et al. Microbial metabolite butyrate promotes anti-pd-1 antitumor efficacy by modulating T cell receptor signaling of cytotoxic cd8 T cell. Gut Microbes. (2023) 15:2249143. doi: 10.1080/19490976.2023.2249143
71. Singh V, Lee G, Son H, Koh H, Kim ES, Unno T, et al. Butyrate producers, “the sentinel of gut”: their intestinal significance with and beyond butyrate, and prospective use as microbial therapeutics. Front Microbiol. (2022) 13:1103836. doi: 10.3389/fmicb.2022.1103836
72. Kang X, Liu C, Ding Y, Ni Y, Ji F, Lau HCH, et al. Roseburia intestinalis generated butyrate boosts anti-pd-1 efficacy in colorectal cancer by activating cytotoxic cd8(+) T cells. Gut. (2023) 72:2112–22. doi: 10.1136/gutjnl-2023-330291
73. Coutzac C, Jouniaux JM, Paci A, Schmidt J, Mallardo D, Seck A, et al. Systemic short chain fatty acids limit antitumor effect of ctla-4 blockade in hosts with cancer. Nat Commun. (2020) 11:2168. doi: 10.1038/s41467-020-16079-x
74. Mager LF, Burkhard R, Pett N, Cooke NCA, Brown K, Ramay H, et al. Microbiome-derived inosine modulates response to checkpoint inhibitor immunotherapy. Science. (2020) 369:1481–9. doi: 10.1126/science.abc3421
75. Fong W, Li Q, Ji F, Liang W, Lau HCH, Kang X, et al. Lactobacillus gallinarum-derived metabolites boost anti-pd1 efficacy in colorectal cancer by inhibiting regulatory T cells through modulating ido1/kyn/ahr axis. Gut. (2023) 72:2272–85. doi: 10.1136/gutjnl-2023-329543
76. Jia D, Wang Q, Qi Y, Jiang Y, He J, Lin Y, et al. Microbial metabolite enhances immunotherapy efficacy by modulating T cell stemness in pan-cancer. Cell. (2024) 187:1651–65 e21. doi: 10.1016/j.cell.2024.02.022
77. Hang S, Paik D, Yao L, Kim E, Trinath J, Lu J, et al. Bile acid metabolites control T(H)17 and T(Reg) cell differentiation. Nature. (2019) 576:143–8. doi: 10.1038/s41586-019-1785-z
78. Cong J, Liu P, Han Z, Ying W, Li C, Yang Y, et al. Bile acids modified by the intestinal microbiota promote colorectal cancer growth by suppressing cd8(+) T cell effector functions. Immunity. (2024) 57:876–89 e11. doi: 10.1016/j.immuni.2024.02.014
79. Schmidt TSB, Raes J, Bork P. The human gut microbiome: from association to modulation. Cell. (2018) 172:1198–215. doi: 10.1016/j.cell.2018.02.044
80. Ho SY, Phua K, Wong L, Bin Goh WW. Extensions of the external validation for checking learned model interpretability and generalizability. Patterns (N Y). (2020) 1:100129. doi: 10.1016/j.patter.2020.100129
81. Nearing JT, Comeau AM, Langille MGI. Identifying biases and their potential solutions in human microbiome studies. Microbiome. (2021) 9:113. doi: 10.1186/s40168-021-01059-0
82. Van Rossum T, Ferretti P, Maistrenko OM, Bork P. Diversity within species: interpreting strains in microbiomes. Nat Rev Microbiol. (2020) 18:491–506. doi: 10.1038/s41579-020-0368-1
83. Vihinen H, Jokinen A, Laajala TD, Wahid N, Peltola L, Kettunen T, et al. Antibiotic treatment is an independent poor risk factor in nsclc but not in melanoma patients who had received anti-pd-1/L1 monotherapy. Clin Lung Cancer. (2023) 24:295–304. doi: 10.1016/j.cllc.2023.01.004
84. Simpson RC, Shanahan ER, Batten M, Reijers ILM, Read M, Silva IP, et al. Diet-driven microbial ecology underpins associations between cancer immunotherapy outcomes and the gut microbiome. Nat Med. (2022) 28:2344–52. doi: 10.1038/s41591-022-01965-2
85. Zhang M, Bzura A, Baitei EY, Zhou Z, Spicer JB, Poile C, et al. A gut microbiota rheostat forecasts responsiveness to pd-L1 and vegf blockade in mesothelioma. Nat Commun. (2024) 15:7187. doi: 10.1038/s41467-024-49842-5
Keywords: gut microbiome, immunotherapy, immune checkpoint inhibitor, solid cancer, response, prediction, intervention
Citation: Lim MY, Hong S and Nam Y-D (2025) Understanding the role of the gut microbiome in solid tumor responses to immune checkpoint inhibitors for personalized therapeutic strategies: a review. Front. Immunol. 15:1512683. doi: 10.3389/fimmu.2024.1512683
Received: 17 October 2024; Accepted: 16 December 2024;
Published: 07 January 2025.
Edited by:
Michael G. White, University of Texas MD Anderson Cancer Center, United StatesReviewed by:
Sergej Tomić, Institute for the Application of Nuclear Energy (INEP), SerbiaDenise Cecil, University of Washington, United States
Copyright © 2025 Lim, Hong and Nam. This is an open-access article distributed under the terms of the Creative Commons Attribution License (CC BY). The use, distribution or reproduction in other forums is permitted, provided the original author(s) and the copyright owner(s) are credited and that the original publication in this journal is cited, in accordance with accepted academic practice. No use, distribution or reproduction is permitted which does not comply with these terms.
*Correspondence: Young-Do Nam, eW91bmdkbzk4QGtmcmkucmUua3I=
†These authors have contributed equally to this work