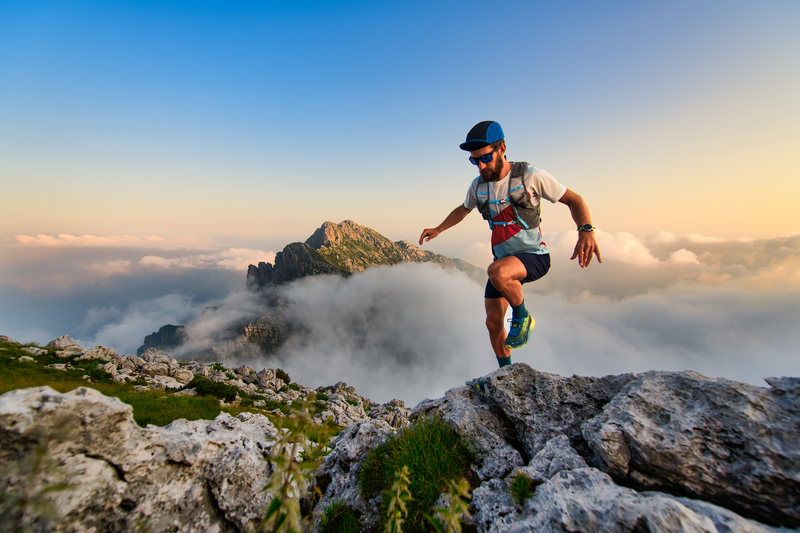
95% of researchers rate our articles as excellent or good
Learn more about the work of our research integrity team to safeguard the quality of each article we publish.
Find out more
ORIGINAL RESEARCH article
Front. Immunol. , 05 December 2024
Sec. Viral Immunology
Volume 15 - 2024 | https://doi.org/10.3389/fimmu.2024.1503118
Background: Epstein-Barr virus-associated hemophagocytic lymphohistiocytosis (EBV-HLH) is a severe hyperinflammatory disorder induced by overactivation of macrophages and T cells. This study aims to identify the risk factors for the progression from infectious mononucleosis (EBV-IM) to EBV-HLH, by analyzing the laboratory parameters of patients with EBV-IM and EBV-HLH and constructing a clinical prediction model. The outcome of this study carries important clinical value for early diagnosis and treatment of EBV-HLH.
Methods: A retrospective analysis was conducted on 60 patients diagnosed with EBV-HLH and 221 patients diagnosed with EBV-IM at our hospital between November 2018 and January 2024. Participants were randomly assigned to derivation and internal validation cohorts in a 7:3 ratio. LASSO regression and logistic regression analyses were employed to identify risk factors and construct the nomogram.
Results: Ferritin (OR, 213.139; 95% CI, 8.604-5279.703; P=0.001), CD3-CD16+CD56+% (OR, 0.011; 95% CI, 0-0.467; P=0.011), anti-EBV-NA-IgG (OR, 57.370; 95%CI, 2.976-1106.049; P=0.007), IL-6 (OR, 71.505; 95%CI, 2.118-2414.288; P=0.017), IL-10 (OR, 213.139; 95% CI, 8.604-5279.703; P=0.001) were identified as independent predictors of EBV-HLH. The prediction model demonstrated excellent discriminatory capability evidenced by an AUC of 0.997 (95% CI,0.993-1.000). When visualized using a nomogram, the ROC curves for the derivation and validation cohorts exhibited AUCs of 0.997 and 0.993, respectively. These results suggested that the model was highly stable and accurate. Furthermore, calibration curves and clinical decision curves indicated that the model possessed good calibration and offered significant clinical benefits.
Conclusions: The nomogram, which was based on these five predictors, exhibited robust predictive value and stability, thereby can be used to aid clinicians in the early detection of EBV-HLH.
The Epstein-Barr virus (EBV), classified within the subfamily γ of the Herpesviridae family, is a double-stranded DNA virus. Transmission of EBV primarily occurs through saliva, although it can also be transmitted via blood transfusion, sexual intercourse, and allografts. Initial infections in individuals under the age of 6 often present as asymptomatic or with mild upper respiratory symptoms, while approximately 50% of adolescents develop infectious mononucleosis (IM) (1). IM is a benign, self-limiting disease resulting from EBV infection. Common clinical presentations include fever, tonsillitis, and enlarged cervical lymph nodes, often accompanied by hepatosplenomegaly and peripheral blood heterogeneous lymphocytosis (2). The majority of cases have a favorable prognosis, although a minority of patients may develop multi-systemic complications, such as EBV-associated hemophagocytic syndrome(HPS) (3).
HPS, also known as hemophagocytic lymphohistiocytosis (HLH), is a severe condition characterized by immune system overactivation, leading progressive deterioration with immune dysfunction in multiple organs. HLH is distinguished by the excessive activation of macrophages and T cells, which results in the release of high levels of inflammatory cytokines (4). Clinical presentations of HLH include persistent fever, hepatosplenomegaly, pancytopenia, and phagocytosis within the bone marrow (1, 5).HLH is classified as either “primary” or “secondary” based on the underlying factor. Primary HLH is an autosomal or sex chromosome recessive disorder resulting from potential gene mutations, with 12 causative genes currently identified in association with primary HLH (6, 7). Based on the specific gene defects, primary HLH is classified as familial HLH (FHL) and immune-deficiency syndrome-associated HLH. Conversely, Secondary HLH is mainly linked to infection, malignancy, and autoimmunity, which are referred to as infection-associated hemophagocytic syndrome (IAHS), malignancy-associated hemophagocytic syndrome (MAHS), and macrophage activation syndrome (MAS), respectively (8). Infection-related HLH represents the most prevalent form of secondary HLH, with infectious agents such as bacteria, fungi, viruses, and protozoa implicated. Herpesvirus infections, specifically EBV infection-associated HLH (EBV-HLH), are a prominent form of secondary HLH. They account for approximately 70% of infection-associated HLH cases, with a higher prevalence among Asian children and adolescents (9).
In clinical practice, the initial symptoms of EBV-HLH patients are often atypical and challenging to identify, with some patients presenting with symptoms resembling infectious mononucleosis. However, the disease progresses rapidly, leading to high mortality rates among patients who delay seeking medical treatment or receive an unclear diagnosis. Therefore, it is imperative to compile and analyze the clinical readouts to improve the identification and diagnosis of EBV-HLH (10, 11).
In this retrospective study, an analysis of laboratory data was conducted to identify potential predictors of EBV-HLH in pediatric patients diagnosed with EBV-HLH or EBV-IM (12). Recognizing patients at risk of developing EBV-HLH is crucial for timely intervention. Therefore, examining the clinical risk factors associated with the development of EBV-HLH in patients with EBV infection is essential for early diagnosis and treatment.
This retrospective study examined pediatric patients of EBV-IM and EBV-HLH at the Children’s Hospital Affiliated to Shandong University from November 2018 to January 2024. Clinical data was collected, including gender, age, serum cytokines (IL-2, IL-4, IL-6, IL-10, IFN-γ, and TNF-α), lymphocyte subsets, EBV DNA copy number, ferritin (FRT), lactate dehydrogenase (LDH), hydroxybutyrate dehydrogenase (HBDH) and EBV specific antibodies (anti-EBV-VCA-IgM, anti-EBV-VCA-IgG, anti-EBV-EA-IgG, and anti-EBV-NA-IgG). All patients’ blood samples were obtained for laboratory testing upon their first visits to the hospital. The EBV-HLH disease development happened in a time frame of 5-14 days. The study protocol was approved by our hospital’s institutional review boards (IRB number: SDFE-IRB/T-2024061) and followed the guidelines outlined in the Declaration of Helsinki.
Patients meeting the HLH-2004 criteria and displaying active EBV infection were classified as having EBV-HLH (13). Diagnosis of HLH required the meeting at least five of the eight criteria: (1) fever; (2) splenomegaly; (3) cytopenia affecting two or more blood cell lineages (hemoglobin<90g/L, platelets<100×109/L, and/or neutrophils <1.0×109/L); (4) hypertriglyceridemia(≥265mg/dL) and/or hypofibrinogenemia (≤150g/dL); (5) hematophagy has been discovered in the bone marrow, spleen, liver, and lymph nodes; (6) natural killer (NK) cell activity is low or undetectable; (7) ferritin levels≥500μg/L; (8) increased interleukin-2 receptor levels (soluble CD25). Patients with primary HLH were excluded from the study, as it encompasses familial HLH and HLH caused by genetic variants of RAB27A, LYST, AP3B1, SH2D1A, and BIRC4.
Diagnosis of EBV-IM involves a combination of clinical symptoms and laboratory results (14), with key clinical indicators being: (1) fever; (2) pharyngeal tonsillitis; (3) cervical lymph node enlargement; (4) splenomegaly; (5) hepatomegaly; (6) eyelid edema. Biomarkers of EBV-IM include: (1) positivity for anti-EBV-VCA-IgM and anti-EBV-VCA-IgG antibodies and negativity for anti-EBV-NA-IgG anti-bodies; (2) negativity for anti-EBV-VCA-IgM antibodies but positivity for anti-EBV-VCA-IgG and low-affinity antibodies; (3) anti-EBV-VCA-IgG levels increased≥4-fold in two serum samples; (4) positivity for EBV DNA by polymerase chain reaction. A combination of three clinical indicators and one nonspecific laboratory test must be met for the diagnosis of EBV-IM.
Cytokine levels were assessed using the Cytokine Combination Assay Kit II (CEGER, China) (15).Lymphocyte subpopulation levels were determined with the Lymphocyte Subpopulation Assay Kit (BD, USA). Lactate dehydrogenase levels were measured through Lactate Dehydrogenase Assay Kit (Zhongya, China). HBDH levels were determined using the α-hydroxybutyrate dehydrogenase assay kit (Zhongya, China). EB-specific antibodies were measured by an EBV antibody detection kit (LIAISON, Italy). Ferritin was measured by a ferritin assay kit (SIEMENS, Germany). All measurement results were derived from the patients’ initial visits to our hospital.
We performed a univariate analysis to analyze clinicopathological characteristics differences between the HLH and IM groups. Variables with statistically significant differences from the above analysis were used as candidate variables for least absolute shrinkage and selection operator (LASSO) regression and multivariate logistic regression to create a nomogram.
The appropriate cutoff values for variables were determined by receiver operating characteristic (ROC) analysis and the maximal Youden index. The area under the curve (AUC) was calculated to evaluate the nomogram’s discriminative ability. Meanwhile, the calibration curve and Hosmer–Lemeshow test were used to reduce the overfitting bias and assess the goodness of fit by comparing the actual probabilities and the probabilities predicted by our nomogram. To estimate the net benefits of the nomogram model under different threshold probabilities, decision curve analysis (DCA) was performed.
SPSS 26.0 (SPSS Inc., Chicago, IL, USA) and R software (v4.0.1) were used for statistical analysis. The difference was statistically significant when the P-value was <0.05. The Wilcoxon rank sum test (continuous variables with skewed distributions) and the Pearson chi-square test (categorical variables) were used to determine statistical differences between the EBV-HLH and EBV-IM groups.
In order to filter the variables for prediction, LASSO regression was performed in the derivation cohort and the importance of these variables was compared by using the “glment package” and the “DALEX package”. The minimum error of lambda (l) values in LASSO regression were obtained as a criterion to screen the variables by ten‐fold cross‐validation. Multivariate logistic regression analysis was performed to estimate odds ratio (OR) with 95% confidence intervals (95% CI) and to identify independent predictor variables for EBV-HLH. The performance of this predictive model was evaluated by using ROC curves (“pROC” package), calibration curves (“RMS” package), and DCA (“rmda” package). A nomogram was constructed based on the five predictors.
Out of the 281 children in the study, 60 were diagnosed with EBV-HLH and 221 with only EBV-IM. These patients were divided into derivation and internal validation cohorts using simple randomization at a ratio of 7:3, with 203 cases in the derivation cohort and 78 cases in the internal validation cohort. Patient characteristics can be found in Supplementary Table S1. The incidence of HLH was 20.2% in the derivation cohort and 24.36% in the validation cohort, with no significant difference in variables between the two cohorts (Supplementary Table S1).
We performed univariate regression analysis in the derivation cohort and identified predictors that were highly associated with EBV-HLH. All the variables showed statistically significant differences, with the exception of gender and IL-2 (Table 1). To build the optimal EBV-HLH risk prediction model, we conducted LASSO regression analysis of these variables, with twelve variables selected through cross-validation: ferritin, CD3+CD8+ T cell ratio (%), the ratio of CD4+ and CD8+T cells (CD4+/CD8+), CD3-CD16+CD56+ T cell ratio (%), HBDH, EBV-DNA copy number, anti-EBV-VCA-IgM, anti-EBV-VCA-IgG, anti-EBV-NA-IgG, IL-6, IL-10 and IFN-γ (Figure 1).
Figure 1. Screening of 21 variables based on Lasso regression. (A) The variation characteristics of the coefficient of variables; (B) the selection process of the optimum value of the parameter log(λ) in the Lasso regression model and 12 variables were selected for further logistic regression analysis.
We subjected these variables to multivariate logistic regression analysis and identified five variables to be included in the predictive model: ferritin (OR, 213.139; 95% CI, 8.604-5279.703; P=0.001), CD3-CD16+CD56+% (OR, 0.011; 95% CI, 0-0.467; P=0.011), anti-EBV-NA-IgG (OR, 57.370; 95%CI, 2.976-1106.049; P=0.007), IL-6 (OR, 71.505; 95%CI, 2.118-2414.288; P=0.017), IL-10 (OR, 213.139; 95% CI, 8.604-5279.703; P=0.001) (Table 2). We have made dotplots to show the different distributions of these parameters between EBV-IM and EBV-HLH (Supplementary Figure S1). These predictors were represented in a nomogram (Figure 2), where each was assigned a point value between 0 and 100. By summing these points and locating them on the total point scale, the corresponding probability of EBV-HLH can be determined. The generated ROC curve for the derivation cohort demonstrated the predictive model’s excellent discriminatory ability, with an AUC of 0.997 (95% CI, 0.993-1.000) (Figure 3A). The calibration curves showed good agreement between predicted and actual EBV-HLH presence (Figure 4A), and the Hosmer-Lemeshow test showed no deviation from a good fit. The cutoff value to distinguish the presence of EBV-HLH in the derivation cohort is 0.141.
Figure 3. ROC curves show excellent discrimination ability for predicting EBV-HLH in the derivation (A) and internal validation cohorts (B). The cutoff values were 0.141 (AUC:0.997, sensitivity: 0.981, specificity: 1.000), and 0.420 (AUC:0.993, sensitivity: 0.983, specificity: 0.947).
The internal validation cohort was employed to evaluate the predictive accuracy of the model. Figure 3B illustrated the ROC curve, which demonstrated an AUC of 0.993 (95% CI, 0.980-1.000). The calibration curves indicated a high degree of concordance between the observed and predicted probabilities of EBV-HLH (Figure 4B). The Hosmer-Lemeshow test yielded a P-value of 0.985, indicating no significant difference. Decision curve analysis indicated that the nomogram was likely to yield substantial net benefits across a range of threshold probabilities for EBV-HLH (Figure 5).
Based on the constructed nomogram, a novel risk stratification model for EBV-HLH was constructed (Table 3). The risk point assigned to various predictors were as follows: ferritin>250ug/L (13 points), CD3-CD16+CD56+%>6.295 (-22 points), anti-EBV-NA-IgG≥5U/ml (10 points), IL-6≥15.95pg/ml (11 points) and IL-10≥26.515pg/ml (10 points). The total risk points for each patient were calculated by summing the individual risk points of these predictors. Based on the distribution characteristics of EBV-HLH prevalence, a cutoff value was established to stratify patients into three distinct subgroups: patients with total points of -22, -12, -11, -1, or 0 were assigned to the low-risk subgroup; patients with total points>11 were classified as the high-risk subgroup; and the remaining patients were defined as the moderate-risk subgroup. A significant difference was found among the three subgroups in terms of EBV-HLH prevalence.
Most primary EBV infections manifest as IM in pediatric populations, with a minority of these cases progressing to EBV-associated hemophagic syndrome (16). The clinical course of hemophagic syndrome is often rapid and severe, with a notably poor prognosis. However, early diagnosis and prompt therapeutic interventions can significantly improve survival outcomes (17).
It has been postulated that deficiencies in natural killer (NK) cell and cytotoxic T lymphocyte (CTL)-mediated cytotoxicity may precipitate an exaggerated or inadequate immune response, culminating in which could give rise to a cytokine storm and multisystem-involved inflammation inflammatory involvement (18). Impaired immune regulation can cause an excessive proliferation of immunoreactive cells, producing a surge of inflammatory cytokines that lead to If the patient’s immune regulation is compromised, resulting in an excessive proliferation of immunoreactive cells, these cells can produce a surge of inflammatory cytokines, culminating in HLH (19).
Lymphocyte subpopulation analysis serves as a crucial indicator for assessing both cellular and humoral immunity. This analysis can provide a snapshot of the current state of the immune system, aiding in the diagnosis of specific diseases and contributing to etiological analysis and prognostic evaluation (20). CD3+ T cells encompass the total population of T cells. CD4+ T cells, also known as helper T cells, play a crucial role in both cellular and humoral immunity. CD8+ T cells, which include CTLs and suppressor T cells, primarily function to eliminate target cells and secrete suppressor factors, thereby modulating the immune response. The CD4+/CD8+ ratio serves as a sensitive indicator for clinical diagnosis of human immune dysfunction (21–23).CD3-CD16+CD56+ cells refer to natural killer (NK) cells, which are vital components of the immune system. NK cells are not only involved in anti-tumor and anti-viral activities, but also implicated in hypersensitivity reactions and autoimmune diseases. NK cells are adept at recognizing target cells and mediating cytotoxic responses. CD3-CD19+ cells, also known as B lymphocytes, are responsible for antibody secretion, antigen presentation, and cytokine production. They play a critical role in regulating the immune system and inflammatory responses.
Our results indicate that CD3-CD16+CD56+ cells (NK cells) are important within this predictive model. NK cells constitute a major component of the non-specific immune system. The proportion of NK cell immunity in EBV-HLH is significantly lower compared to EBV-IM, indicating a reduced non-specific NK cell response in patients with EBV-HLH. NK cells eliminate virus-infected or malignant cells using a variety of mechanisms, some of which rely on cytotoxic granule-mediated killing. Granule-mediated cytotoxicity is carried out through the polarized delivery of cytotoxic granule contents to the immunologic synapse, extrusion of contents into the space shared with a target cell, and perforin-mediated entry into the target cell where cytotoxic granule contents induce target-cell apoptosis.
In patients with familial hemophagocytic lymphohistiocytosis (HLH) types 2 to 5, as well as those with pigmentary disorders associated with HLH, granule-mediated cytotoxicity is compromised. The prolonged duration of synapse formation between cytotoxic lymphocytes—deficient in perforin or granzymes—and target cells results in the excessive production of inflammatory cytokines. Furthermore, antigen-presenting cells accumulate and persistently stimulate T cells, thereby exacerbating T-cell activation and proliferation. This initiates a self-perpetuating cycle of lymphohistiocytic proliferation and hypercytokinemia, ultimately culminating in extensive tissue damage and hyperinflammatory syndrome of HLH (24). On the other hand, the percentage of CD4+ and CD8+ T cells, the CD4+/CD8+ cell ratio, and the percentage of CD3-CD19+ cells were not effective in distinguishing between the two disorders due to their lower sensitivity, supporting the previous findings. Further research is needed to elucidate the molecular mechanism underlying the immune responses in IM and HLH upon EBV infection.
The pathophysiological abnormalities in the immune mechanism of HLH are characterized by the dysregulated activation of T cells, particularly CD8+ cytotoxic T cells, and macrophages. This dysregulation results in the excessive production of cytokines, contributing to the clinical manifestations of the disease (25, 26). Raj et al. elucidated that EBV infection led to the augmented synthesis and secretion of cytokines, including IFN-γ, IL-10, and granulocyte-macrophage colony-stimulating factor (GM-CSF). These elevated cytokine levels further stimulated the activation of cytotoxic T-cell (CTL) and macrophages (27–30). The activated macrophages, in turn, secreted substantial quantities of pro-inflammatory cytokines such as IFN-γ and TNF-α, thereby initiating a “cytokine storm” that induced persistent hyperthermia and resulted in immune- mediated damage to normal tissues. Previous studies have demonstrated that patients with HLH exhibited specific cytokine profiles characterized by significantly elevated levels of IFN-γ and IL-10, along with moderately elevated levels of IL-6 (4). Our findings validated these observations, by demonstrating elevated IL-6 and IL-10 levels in EBV-HLH compared to EBV-IM. IL-6 has been demonstrated to attenuate the cytolytic activity of NK cells. IL-10, expressed by a variety of immune cells, plays a significant role in anti-inflammatory processes. IL-10 disrupts the functionality of antigen-presenting cells (APCs) and downregulates the expression of major histocompatibility complex class II (MHC-II), thereby impairing antigen presentation. Additionally, IL-10 inhibits T-cell proliferation, compromises the secondary responses of CD8+ T cells, and diminishes the production of inflammatory mediators by neutrophils (31). Consequently, it is critical to delineate the cytokine changes in EBV-IM and EBV-HLH following EBV infection, which can aid clinicians in the early identification of EBV-HLH.
Specimens from patients with EBV-HLH exhibit diverse EBV antibody responses, which may indicate either primary EBV infection or reactivation of a previous infection. In this study, anti-EBV-NA-IgG was identified as a significant factor in the predictive model. It is well-established that the host organism sequentially produces antibodies against various EBV antigens (CA, EA, NA) following the infection, with IgM and IgG antibodies against EBV-CA being generated at an early stage, followed by antibodies against EBV-EA. The anti-EBV-NA-IgG typically manifested at a later stage of the infection (32). Consequently, anti-EBV-NA-IgG served as a critical marker for clinicians, not only in pinpointing of the infection timeline, but also in recognition of the progression of EBV-IM into EBV-HLH.
A hallmark of HLH is the hepatic injury-induced release of ferritin, which acts as an acute phase reactant. The diagnostic and prognostic utility of ferritin cut-off values in pediatric HLH has been extensively investigated. The 2004 HLH guidelines, which are widely implemented in routine pediatric practice, include a ferritin level of ≥500 ng/ml as one of the diagnostic criteria for HLH. This threshold was subsequently adjusted to 10,000ng/ml by Allen et al., achieving a sensitivity of 90% (95% CI: 71-100%) and a specificity of 96% (95% CI: 94-98%) (33). In our study, we integrated ferritin with four additional parameters to develop a predictive model for HLH, which proved to be more effective than assessing ferritin levels alone. It is important to note that elevated ferritin is not exclusive to HLH. It is also observed in conditions such as kidney failure, liver disease, infections, and malignancies.
In a study, researchers have found that IL-10, IFN-γ, ferritin and D-dimer levels were significantly different between EBV-HLH and EBV-IM. In the present study, we also found ferritin and IL-10 were independent predictors of EBV-HLH. IFN-γ was subjected to multivariate logistic regression analysis (P=0.809) but was not included in the predictive model. The coagulation related indicators of the most EBV-IM patients were not collected, so D-dimer levels were not adopted in our study (12).
This study has several limitations. First, as a retrospective analysis, we were unable to monitor patients from the onset of fever to the diagnosis of EBV-HLH. Second, due to the single-center nature of this study, the reproducibility of our results necessitates validation through multicenter studies with a larger sample size.
In summary, IL-6, IL-10, CD3-CD16+CD56+ cells, anti-EBV-NA-IgG and ferritin are potential biomarkers for the early differentiation between EBV-HLH and EBV-IM.
The raw data supporting the conclusions of this article will be made available by the authors, without undue reservation.
The studies involving humans were approved by The institutional review boards of Jinan Children’s Hospital. The studies were conducted in accordance with the local legislation and institutional requirements. The human samples used in this study were acquired from a by- product of routine care or industry. Written informed consent for participation was not required from the participants or the participants’ legal guardians/next of kin in accordance with the national legislation and institutional requirements.
RH: Formal analysis, Software, Validation, Writing – original draft. DW: Data curation, Investigation, Writing – original draft. LW: Methodology, Visualization, Writing – original draft. PL: Funding acquisition, Investigation, Writing – review & editing. XZ: Data curation, Resources, Writing – original draft. LH: Data curation, Writing – original draft, Investigation. MC: Methodology, Visualization, Writing – review & editing. XL: Conceptualization, Writing – review & editing.
The author(s) declare that financial support was received for the research, authorship, and/or publication of this article. This study was supported by the Science and Technology Development Program of Jinan Municipal Health Commission (2024308010, 2024308012).
The authors declare that the research was conducted in the absence of any commercial or financial relationships that could be construed as a potential conflict of interest.
The author(s) declare that no Generative AI was used in the creation of this manuscript.
All claims expressed in this article are solely those of the authors and do not necessarily represent those of their affiliated organizations, or those of the publisher, the editors and the reviewers. Any product that may be evaluated in this article, or claim that may be made by its manufacturer, is not guaranteed or endorsed by the publisher.
The Supplementary Material for this article can be found online at: https://www.frontiersin.org/articles/10.3389/fimmu.2024.1503118/full#supplementary-material
1. Marsh RA, Canna SW. Pediatric hemophagocytic lymphohistiocytosis. Blood. (2020) 135:1332–43. doi: 10.1182/blood.2019000936
2. Gao W-b, Hu L-j, Ma X-l, Shi M-j, Wang C-y, Ma Y, et al. A predictive model for identifying secondary underlying diseases of hemophagocytic lymphohistiocytosis. Front Immunol. (2023) 14. doi: 10.3389/fimmu.2023.1143181
3. Darteyre S, Ludwig C, Jeziorski E, Schved JF, Rodière M. Syndrome d’activation macrophagique et infection à virus d’Epstein-Barr chez l’enfant. Médecine Maladies Infectieuses. (2010) 40:18–26. doi: 10.1016/j.medmal.2009.06.004
4. Zhang H-q, Yang S-W, Fu Y-C, Chen M-C, Yang C-H, Yang M-H, et al. Cytokine storm and targeted therapy in hemophagocytic lymphohistiocytosis. Immunologic Res. (2022) 70:566–77. doi: 10.1007/s12026-022-09285-w
5. Griffin G, Shenoi S, Hughes GC. Hemophagocytic lymphohistiocytosis: An update on pathogenesis, diagnosis, and therapy. Best Pract Res Clin Rheumatol. (2020) 34:101515. doi: 10.1016/j.berh.2020.101515
6. Yao S, He L, Zhang R, Liu M, Hua Z, Zou H, et al. Improved hemophagocytic lymphohistiocytosis index predicts prognosis of adult Epstein-Barr virus-associated HLH patients. Ann Med. (2022) 55:89–100. doi: 10.1080/07853890.2022.2149850
7. El-Mallawany NK, Curry CV, Allen CE. Haemophagocytic lymphohistiocytosis and Epstein–Barr virus: a complex relationship with diverse origins, expression and outcomes. Br J Haematology. (2021) 196:31–44. doi: 10.1111/bjh.17638
8. Zhang J, Qin S, Jin Z, Chen Q, Xing L, Qiu T, et al. The clinical significance and prognostic role of whole-blood Epstein-Barr virus DNA in lymphoma-associated hemophagocytic lymphohistiocytosis. J Clin Immunol. (2023) 43:1302–10. doi: 10.1007/s10875-023-01493-9
9. Ponnatt TS, Lilley CM, Mirza KM. Hemophagocytic lymphohistiocytosis. Arch Pathol Lab Med. (2022) 146:507–19. doi: 10.5858/arpa.2020-0802-RA
10. Sepulveda FE, de Saint Basile G. Hemophagocytic syndrome: primary forms and predisposing conditions. Curr Opin Immunol. (2017) 49:20–6. doi: 10.1016/j.coi.2017.08.004
11. Li X, Yan H, Xiao Z, Luo T, Xie L, Yang Y, et al. A three-step screening procedure for early identification of children at high risk of hemophagocytic lymphohistiocytosis. J Clin Immunol. (2023) 43:989–98. doi: 10.1007/s10875-023-01458-y
12. Cai L, Xing Y, Xia Y, Zhang Z, Luo Z, Tang Y, et al. Comparative study of biomarkers for the early identification of Epstein–Barr virus-associated hemophagocytic lymphohistiocytosis in infectious mononucleosis. BMC Infect Dis. (2023) 23(1):728. doi: 10.1186/s12879-023-08654-6
13. Henter JI, Horne A, Aricó M, Egeler RM, Filipovich AH, Imashuku S, et al. HLH-2004: Diagnostic and therapeutic guidelines for hemophagocytic lymphohistiocytosis. Pediatr Blood Cancer. (2006) 48:124–31. doi: 10.1002/pbc.21039
14. t.S.o.P.The Subspecialty Group of Infectious Diseases CMA, The National Group of EpsteinBarr Virus Associated Diseases in Children. Principle suggestions for diagnosis and treatment of main nontumorous EpsteinBarr virusassociated diseases in children. Zhonghua Er Ke Za Zhi. (2016) 54:563–8. doi: 10.3760/cma.j.issn.0578-1310.2016.08.002
15. Tang Y, Xu X, Song H, Yang S, Shi S, Wei J, et al. Early diagnostic and prognostic significance of a specific Th1/Th2 cytokine pattern in children with haemophagocytic syndrome. Br J Haematology. (2008) 143:84–91. doi: 10.1111/j.1365-2141.2008.07298.x
16. Taylor GS, Long HM, Brooks JM, Rickinson AB, Hislop AD. The immunology of Epstein-Barr virus–induced disease. Annu Rev Immunol. (2015) 33:787–821. doi: 10.1146/annurev-immunol-032414-112326
17. Ababneh E, Saad AM, Crane GM. The role of EBV in haematolymphoid proliferations: emerging concepts relevant to diagnosis and treatment. Histopathology. (2021) 79:451–64. doi: 10.1111/his.14379
18. Liu M, Wang R, Xie Z. T cell-mediated immunity during Epstein–Barr virus infections in children. Infection Genet Evol. (2023) 112:105443. doi: 10.1016/j.meegid.2023.105443
19. Brisse E, Wouters CH, Matthys P. Hemophagocytic lymphohistiocytosis (HLH): A heterogeneous spectrum of cytokine-driven immune disorders. Cytokine Growth Factor Rev. (2015) 26:263–80. doi: 10.1016/j.cytogfr.2014.10.001
20. Dalal BI, Vakil AP, Khare NS, Wang SY, Richards MJ, Chen LYC. Abnormalities of the lymphocyte subsets and their immunophenotype, and their prognostic significance in adult patients with hemophagocytic lymphohistiocytosis. Ann Hematol. (2015) 94:1111–7. doi: 10.1007/s00277-015-2350-y
21. An Q, Wang Y, Hu S, Fang D, Xuan C, Xu S, et al. Clinical significance of lymphocyte subset changes in hemophagocytic lymphohistiocytosis of children. Exp Ther Med. (2016) 12:3549–52. doi: 10.3892/etm.2016.3809
22. Zhang Y, Huang C, Zhang H, Duan Z, Liu Q, Li J, et al. Characteristics of immunological events in Epstein-Barr virus infection in children with infectious mononucleosis. Front Pediatr. (2023) 11. doi: 10.3389/fped.2023.1060053
23. Chaturvedi V, Marsh RA, Zoref-Lorenz A, Owsley E, Chaturvedi V, Nguyen TC, et al. T-cell activation profiles distinguish hemophagocytic lymphohistiocytosis and early sepsis. Blood. (2021) 137:2337–46. doi: 10.1182/blood.2020009499
24. Crayne CB, Albeituni S, Nichols KE, Cron RQ. The immunology of macrophage activation syndrome. Front Immunol. (2019) 10. doi: 10.3389/fimmu.2019.00119
25. Zhao X-X, Lian H-Y, Zhang L, Ma H-H, Wang D, Zhao Y-Z, et al. Significance of serum Th1/Th2 cytokine levels in underlying disease classification of childhood HLH. Cytokine. (2022) 149:155729. doi: 10.1016/j.cyto.2021.155729
26. Chen Y, Wang Z, Luo Z, Zhao N, Yang S, Tang Y. Comparison of Th1/Th2 cytokine profiles between primary and secondary haemophagocytic lymphohistiocytosis. Ital J Pediatr. (2016) 42(1):50. doi: 10.1186/s13052-016-0262-7
27. Yanagimachi M, Goto H, Miyamae T, Kadota K, Imagawa T, Mori M, et al. Association of IRF5 polymorphisms with susceptibility to hemophagocytic lymphohistiocytosis in children. J Clin Immunol. (2011) 31:946–51. doi: 10.1007/s10875-011-9583-x
28. Maruyama A, Takahashi N, Gunji Y, Morimoto A, Yada Y, Koike Y, et al. Congenital hemophagocytic lymphohistiocytosis in a preterm infant. J Pediatr Hematology/Oncology. (2011) 33:e209–12. doi: 10.1097/MPH.0b013e318219fd63
29. Ou W, Zhao Y, Wei A, Ma H, Zhang Q, Zhang L, et al. Serum cytokine pattern in children with hemophagocytic lymphohistiocytosis. Ann Hematol. (2023) 102:729–39. doi: 10.1007/s00277-023-05132-6
30. Xu X-J, Luo Z-B, Song H, Xu W-Q, Henter J-I, Zhao N, et al. Simple evaluation of clinical situation and subtypes of pediatric hemophagocytic lymphohistiocytosis by cytokine patterns. Front Immunol. (2022) 13. doi: 10.3389/fimmu.2022.850443
31. Tang Y, Xu Q, Luo H, Yan X, Wang G, Hu L, et al. Excessive IL-10 and IL-18 trigger hemophagocytic lymphohistiocytosis–like hyperinflammation and enhanced myelopoiesis. J Allergy Clin Immunol. (2022) 150:1154–67. doi: 10.1016/j.jaci.2022.06.017
32. Akpolat N, Gedik M, Nergiz S, Bilek H. Determination of serological profiles and avidity of specific antibodies in the sera of patients with potential Epstein-Barr virus (EBV) infection. Bratislava Med J. (2013) 114:460–3. doi: 10.4149/BLL_2013_096
Keywords: Epstein-Barr virus infections, lymphohistiocytosis, hemophagocytic, infectious mononucleosis, pediatrics, nomograms
Citation: Huang R, Wu D, Wang L, Liu P, Zhu X, Huang L, Chen M and Lv X (2024) A predictive model for Epstein-Barr virus-associated hemophagocytic lymphohistiocytosis. Front. Immunol. 15:1503118. doi: 10.3389/fimmu.2024.1503118
Received: 28 September 2024; Accepted: 20 November 2024;
Published: 05 December 2024.
Edited by:
Anders Wallqvist, U.S. Army Medical Research and Development Command, United StatesReviewed by:
Wendy Lee, Singapore Immunology Network (A*STAR), SingaporeCopyright © 2024 Huang, Wu, Wang, Liu, Zhu, Huang, Chen and Lv. This is an open-access article distributed under the terms of the Creative Commons Attribution License (CC BY). The use, distribution or reproduction in other forums is permitted, provided the original author(s) and the copyright owner(s) are credited and that the original publication in this journal is cited, in accordance with accepted academic practice. No use, distribution or reproduction is permitted which does not comply with these terms.
*Correspondence: Xin Lv, ZXR5eWp5a2x2eGluQDE2My5jb20=; Mengmeng Chen, MTg4NjYxMTU4MjhAMTYzLmNvbQ==
Disclaimer: All claims expressed in this article are solely those of the authors and do not necessarily represent those of their affiliated organizations, or those of the publisher, the editors and the reviewers. Any product that may be evaluated in this article or claim that may be made by its manufacturer is not guaranteed or endorsed by the publisher.
Research integrity at Frontiers
Learn more about the work of our research integrity team to safeguard the quality of each article we publish.