- 1Jiangxi Medical College, Nanchang University, NanChang, China
- 2Department of Thoracic Surgery, Jiangxi Cancer Hospital, The Second Affiliated Hospital of Nanchang Medical College, Jiangxi Cancer Institute, Nanchang, China
- 3Jiangxi Key Laboratory of Oncology, Jiangxi Cancer Hospital, Nanchang, Jiangxi, China
- 4Medical College, Jiangxi University of Chinese Medicine, Nanchang, Jiangxi, China
- 5Zhejiang-Jiangxi Joint Thoracic Oncology Research Laboratory, Jiangxi Cancer Hospital, Nanchang, Jiangxi, China
Esophageal squamous cell carcinoma is a cancer with high morbidity and mortality. The advent of immune checkpoint inhibitors has significantly increased complete response rates and postoperative R0 resection rates after neoadjuvant therapy. These drugs can largely reverse the suppression of the immune system caused by the tumor microenvironment, allowing the reactivation of anti-tumor immune infiltrating cells, significantly improving the patient’s tumor microenvironment, and thus preventing tumor development. However, there are still some patients who respond poorly to neoadjuvant combined immunotherapy and cannot achieve the expected results. It is now found that exploring changes in the tumor microenvironment not only elucidates patient responsiveness to immunotherapy and identifies more reliable biomarkers, but also addresses the limitations of prediction with imaging examination such as CT and the instability of existing biomarkers. In light of these considerations, this review aims to delve into the alterations within the tumor microenvironment and identify potential predictive biomarkers ensuing from neoadjuvant immunotherapy in the context of esophageal squamous cell carcinoma.
1 Introduction
Esophageal cancer has a high incidence and mortality rate, with esophageal squamous cell carcinoma(ESCC) being the predominant histological subtype (1–3). Neoadjuvant chemotherapy is widely applied in the perioperative management of ESCC, yet the outcomes have not been entirely satisfactory. Since the introduction of immune checkpoint inhibitors (ICI), they have been extensively applied in the treatment of various cancers. In the field of ESCC, numerous large-scale clinical trials have shown that neoadjuvant immunotherapy improves the pathological response rate(pCR) and extends patient survival. Moreover, neoadjuvant chemotherapy and immunotherapy (nCIT) can increase the chances of surgery and enhance the rate of R0 resections, offering hope to patients.
Surgery following neoadjuvant chemoimmunotherapy (nCIT) is considered the optimal treatment approach (4). However, because esophageal cancer is a hollow viscus tumor, there are certain limitations when using the immune-related Response Evaluation Criteria in Solid Tumors (iRECIST) to assess the efficacy of neoadjuvant therapy before surgery for resectable esophageal cancer. The invasiveness of endoscopy and its costs also restrict the widespread application of endoscopic assessment (5). Concurrently, there remains a subset of patients who do not benefit from this combined therapy, delaying surgical opportunities and leading to a poorer prognosis (6, 7). Therefore, it is significant to investigate the alterations and potential biomarkers in the tumor microenvironment (TME) of ESCC following nCIT. Recently, Existing biomarkers predictive of nCIT efficacy, including the tumor proportion score (TPS) for PD-L1, have shown limited sensitivity or specificity in predicting therapeutic outcomes (8). Researchers are increasingly focusing on the impact of TME after nCIT and utilizing Single-cell RNA-sequence to analyze various immune cells. Thus, cells and genes with significant alterations are expected to become more specific and sensitive biomarkers. Therefore, We provide a brief overview of the current status of research on nCIT, summarize the alterations and potential biomarkers on the TME of ESCC after nCIT, and discuss future research directions in this field (Figure 1).
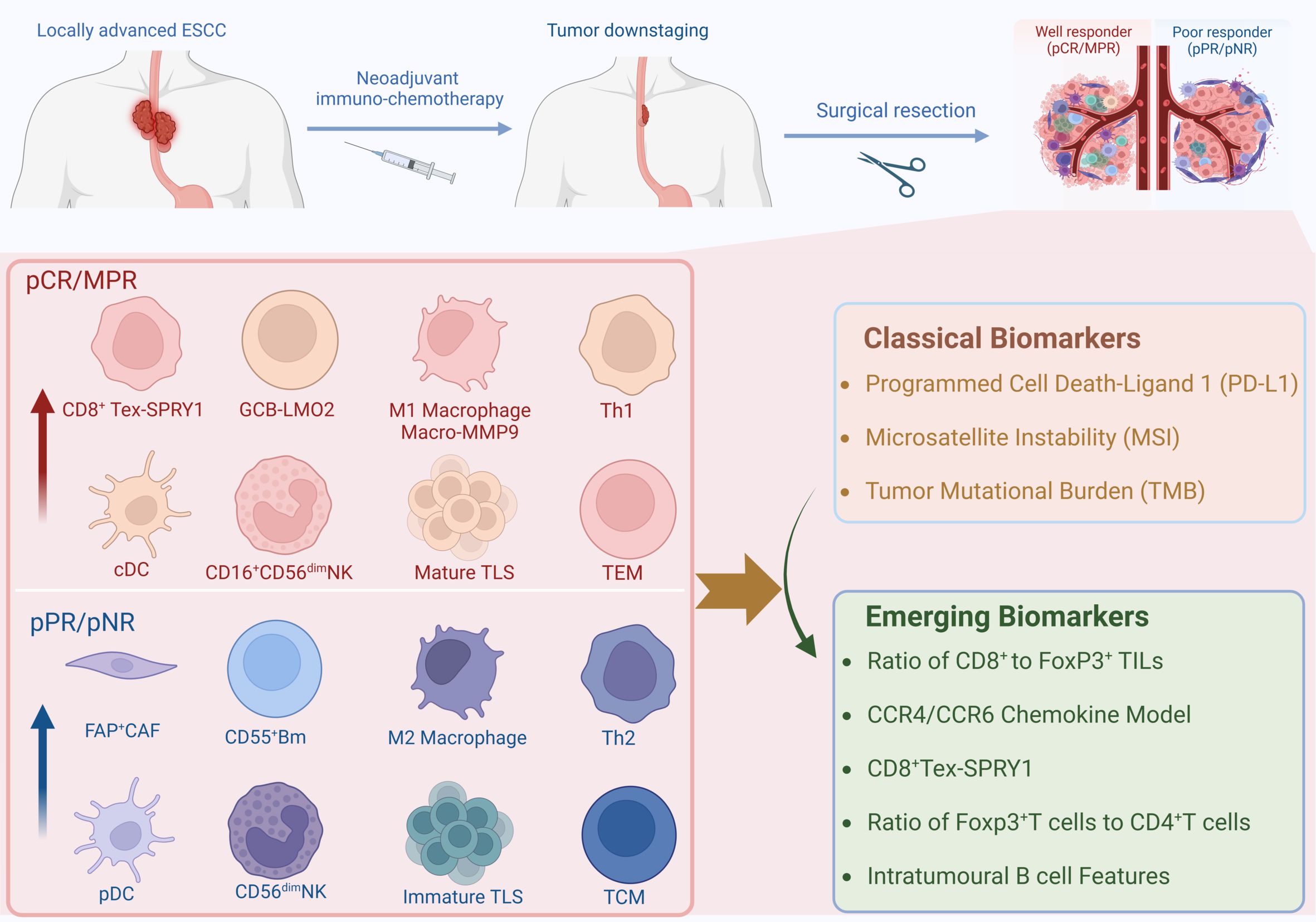
Figure 1. Graphical Introduction. Created in BioRender. Ka, X. (2024) https://BioRender.com/v51n660.
2 Current status of neoadjuvant immunotherapy research
Following the introduction of neoadjuvant therapy, large-scale clinical trials, such as the CROSS trial (9) and the NEOCRTEC5010 trial (10), have established neoadjuvant chemoradiotherapy (nCRT) as a first-line treatment new option for resectable locally advanced esophageal cancer. Furthermore, clinical studies including CheckMate 648, ESCORT, and KEYNOTE-181 have revealed the excellent efficacy of immune checkpoint inhibitors (ICIs) in unresectable advanced or recurrent esophageal cancer, prompting researchers to focus on the nCIT (11–13).
At present, prospective studies, such as NICE (14)and Neo-PLANET (15), have demonstrated excellent pathological complete response (pCR) rates for nCIT in esophageal squamous cell carcinoma (ESCC), ranging from 21.2% to 40.0% (16). Xu et al. included 9 trials in a meta-analysis, pointing out that the pCR rate of nCIT significantly reached 26.9% (OR, 4.24; 95% CI, 2.84-6.32), which is more than three times higher than the 8.3% observed in the group receiving chemotherapy alone (nCT) (17). Similarly, in the field of lung cancer, Sorin et al. included 43 trials in a meta-analysis and indicated that in comparison with nCT, nCIT has significantly increased the pCR rate to the range of 20% to 40%(RR, 5.52; 95% CI, 4.25-7.15) (18). In the gastric cancer field, the combined pCR rate increased to 24% (95% CI: 19%–28%) (19). In the field of melanoma, the pCR rate increased to 57%, with a 2-year RFS range of 94% to 100% (20). Regarding the pCR rate of ESCC is lower than that of melanoma, most researchers believe that melanoma has a higher immunogenicity, making immune cells easily activated and having a good response rate. However, the efficacy of ESCC is similar to that of NSCLC and gastric cancer, with significant improvements including other observed indicators such as pCR, MPR, and OS. At the same time, these studies indicate that nCIT has controllable treatment-related toxic effects, and the rate of R0 resection after surgery has also increased, further demonstrating that nCIT is a better choice for neoadjuvant therapy. To this end, we have also summarized the ongoing clinical trials on neoadjuvant therapy for ESCC, as shown in Table 1.
3 Alterations in the tumor microenvironment
Numerous studies indicate a potential correlation between TME and pathological response (21, 22). In the TME, immune checkpoint blockade (ICB) or immune checkpoint inhibitor (ICI) targets immunosuppressive molecules of tumor cells, thereby reactivating exhausted T cells to resist tumor cells (23). However, the composition of the TME in ESCC is complex. Although tumor-infiltrating CD8+ T cells and NK cells exert antitumor effects, the regulatory T cells (24) and M2 macrophages exert immunosuppressive effects, leading to deficiencies in immune surveillance (25). Furthermore, extensive fibrosis and extracellular matrix deposition also augment the tumor’s resistance to immunotherapy (26). Post-treatment with ICB, the interactions and changes among various cells within TME remain insufficiently elucidated. The response to immunotherapy does not always correlate with PD-L1 expression (27, 28). Therefore, it is crucial to explore the alteration of TME before and after immunotherapy and identify relevant biomarkers.
3.1 CD8+ T cell
CD8+ T cells are a crucial subset of T cells, showing the most significant difference after neoadjuvant chemoimmunotherapy (nCIT) (29). CD8+ T cells are T cells that have undergone differentiation and maturation to express CD8 molecules. They can secrete large amounts of IFN-γ and granzyme B to synergize against cancer cells (30). Numerous studies have found that nCIT increases CD8+ T cell infiltration more significantly than neoadjuvant chemotherapy (28), prolonging overall patient survival (31). And the same trend can be observed before and after nCIT, whereas regulatory T cells (Treg) show the opposite trend. One of the studies has indicated that the density of CD8+ tumor-infiltrating lymphocytes (TILs) in the pCR and major pathological response (MPR) groups is higher than in the pathological partial response (pPR) and pathological non-response (pNR) groups. Additionally, the ratio of CD8+ to FoxP3+ TILs in the pCR/MPR group is significantly greater than in the pPR/pNR group, suggesting that this ratio could emerge as a novel biomarker after nCIT in ESCC. Interestingly, in the patients not achieving pCR, CD8+ TILs were observed to be mostly distributed in the periphery of the tumor, correlating with a worse prognosis, while the same cellular distribution was found in the lymph nodes (32).
Based on the expression of antigenic clusters, CD8+ T cells are classified into different T cell subpopulations. In addition to the regulatory CD8+ T cell subset (33), there are Tc1 cells with the most typical cytotoxic function (34). In the tumor microenvironment, CD8+ T cells generally resist cancer cells by differentiating into cytotoxic T cells (29). However, T cells chronically exposed to persistent antigens or chronic inflammatory environments are prevented from acquiring memory cell homeostatic responsiveness (34–36). The immediate protection and recall response to antigens conferred by memory lymphocytes constitutes a critical component of the organism’s immune defense (37). Nowadays, studies have shown a decrease in the ratio of central memory cells (TCM) to effector memory T cells (TEM) in samples of patients who have achieved pCR/MPR, reversing the high pre-treatment TCM/TEM ratio (28). The process of TCM-to-TEM, which occurs on antigenic restimulation, produces greater anti-tumor capacity (38, 39).
T cells, during their process of progressive exhaustion, upregulate the expression of inhibitory pathway molecules, including PD-1 and CTLA-4 (40). Thus, ICB facilitates the reactivation of exhausted CD8+ T cells by blocking the inhibitory molecules. However, with an in-depth study, it was found that even with ICB, a large number of exhausted T cells (Tex) still existed in the TME (41) and it could be divided into ICI-permissive and ICI-resistant subpopulations (42, 43). However, two clusters of cells, CD8+ Tex-SPRY1 and CD8+ Tex-XAF1, were found to respond significantly to ICI treatment. Furthermore, the anti-tumor signature genes ENTPD1, CXCL13, and HLA-DR genes were enriched in these two CD8+ Tex subsets. The CD8+ Tex-SPRY1 cells were in the early stage of exhausted T cells and showed high similarity to progenitor CD8+ T cells. Moreover, these samples from patients who achieved pCR had higher expression of SPRY1 and CD8 in the tumor stroma and intra-tumoral regions before and after nCIT, which was not observed in patients who did not achieve pCR (Figure 2). It is suggested that patients with high CD8+ Tex-SPRY1 cell infiltration have a higher sensitivity to nCIT and that CD8+ Tex-SPRY1 may be a potential biomarker for nCIT in ESCC.
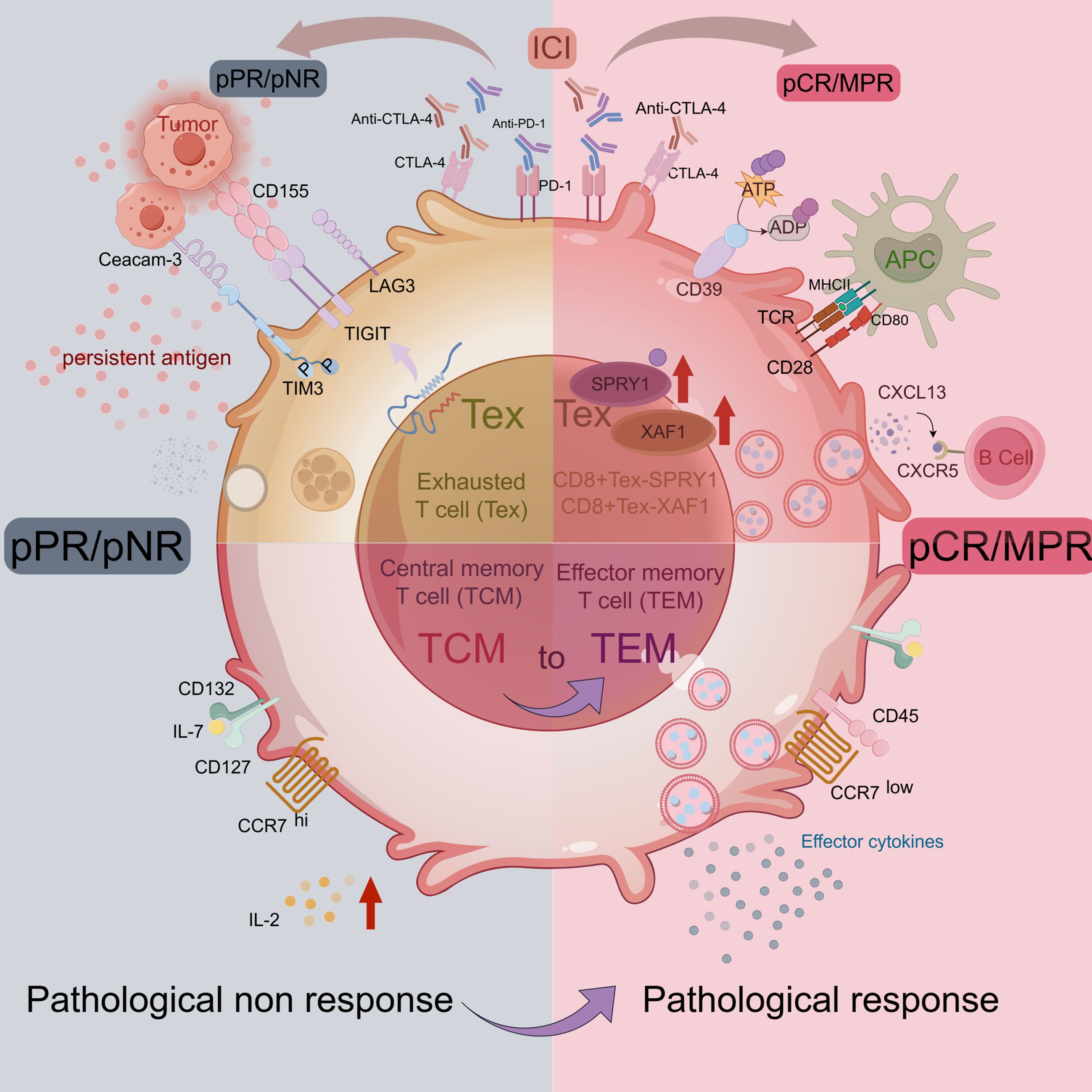
Figure 2. T cell in TME of ESCC. Image created with figdraw.com,ID: IUIUT533a6.
3.2 CD4+ T cell
CD8+ T cells showed a significant correlation with nCIT, but the heterogeneity of the TME also limits its stability as a biomarker. CD4+ T cells, unlike CD8+ T cells that can directly kill tumor cells, function mainly by releasing different cytokines to regulate other immune cells and play an equally important role as CD8+ T cells. CD4+ T cells represent a heterogeneous subset of T cells, and different levels of CD4+ T cell numbers are associated with different outcomes. Some studies have noted that a significant increase in CD4+ T cells is observed after nCIT compared to neoadjuvant chemotherapy alone and that higher CD4+/CD8+ ratios are associated with better pathological response (44). However, it has also been noted that the high level of CD4+ T cell distribution within a distance of 0-10 μm from the tumor cells predicts a low survival rate (45). Tumor-infiltrating CD4+ T cells exert their function by undergoing differentiation into various subsets directed by functional polarization. Moreover, the differentiation of these subsets is significantly influenced by the characteristics of the TME in which they exist. For example, Th1 cells exert antitumor effects, whereas Th2 cells counteract the effects of Th1 cells, and the Th9 is unchanged after nCIT (28).
Recently, a single-cell sequencing study classified CD4+ T cells into six major subsets based on specific markers. Among them, CD4-C1-CCR7 carries naïve signature genes, including TCF7, CCR7, LEF1, and SELL. CD4-C6-FOXP3 has the most distinctive Treg profile, expressing high levels of the Treg signature genes FOXP3, IKZF2, IL2RA, and CTLA-4. The study noted that Tregs are heavily enriched in ESCC and that Tregs accounted for more than 50% of the total CD4+ T cells in the tumors, compared to only 25% in the adjacent normal tissues (46). At the same time, numerous studies have indicated that Treg cells can significantly inhibit T cell-mediated antitumor effects (47). The density of Treg cells in ESCC tissues was higher than that in normal tissues. However, after nCIT, the number of Treg decreased while CD8+ T cells increased (28). FoxP3+ TILs were found in higher quantities in tumor samples from patients who achieved pNR compared to those who achieved pCR (32). It was also found that there exists a subset of T cells expressing CD4+CD25-CD69+Foxp3-LAP+, which inhibits T cell proliferation and promotes tumor immune escape by secreting TGF-β. This subset was positively correlated with the pathologic response (48). However, only high Treg density in ESCC tissues is not predictive of patient survival (45). The calculation of the ratio between Treg and CD4+ T cells is required to observe a positive correlation with better pathological response (49). Thus, the ratio of FoxP3+ T cells to CD4+ T cells is expected to be a biomarker for predicting the response to nCIT, as mentioned previously for CD8+ TILs/FoxP3+ TILs.
3.3 Macrophage
Macrophages are known as the “scavengers” of the human organism. They can not only phagocytose pathogens but also exert the function of presenting antigens by processing antigens to form MHC complexes. Macrophages migrate into the TME and become tumor-associated macrophages (TAMs), which play an important role in the initiation and regulation of antitumor immune responses (50). Recently, it was found that dendritic cells and macrophages were mostly distributed within 30 μm from tumor cells and that patients with closer spatial distance of TAM’s distribution tend to have better immune responses and longer survival (45). This is because chemotherapy causes tumor cells to release more tumor-associated antigens, and the closer the distance between TAMs and tumor cells, the better for TAMs to be exposed to these more new antigens, which provide immunomodulatory signals to the T cells to promote anti-tumor immune responses (51). This suggests that spatial distances between TAMs and tumor cells are potential predictive biomarkers (52).
TAMs can be activated by M1 and M2 types. M1-TAMs exert proinflammatory and antitumor effects (53), enhancing the antigen-presenting capacity of DC and augmenting tumoricidal effects of infiltrating T cells (54). Tumor tissues in the pCR group were observed to have a significant M1 polarization level before treatment and a significantly higher M1/M2 ratio than in the non-pCR group after treatment (29, 55). Among M1-type macrophages, Macro-MMP9 and Macro-FOLR2 subset produced large amounts of cytokines such as IL-15, CXCL1, and CXCL9, which induced the activation of CD8+ Tex-SPRY1 subset. Thus Macro-MMP9 and CD8+ Tex-SPRY1 form a positive feedforward cycle and anti-tumor ability (29). Whereas M2-TAMs could inhibit M1-TAMs effects to promote immunesuppression (56), the number of PD-L1+CD163+ cells infiltrated was significantly increased in the non-pCR group (57). Recently, it has been shown that macrophage migration inhibitory factor (MIF) promotes the conversion of M1-type TAMs to M2-type, and patients with high baseline MIF levels are significantly associated with adverse pathologic responses.
Macrophages exhibit high plasticity and heterogeneity (58). CCR4+CCR6+ TAM were found to be significantly reduced after nCIT, while the percentage of total macrophages in the tumor microenvironment was not changed. The CCR4/CCR6 chemokine system score was lower in tumor regression grading (TRG) of grade 0/1 compared to patients with TRG2/3, suggesting that the CCR4/CCR6 chemokine system would be a potential biomarker (28). Another subset of TAM, TAM-TREM2, has an immunosuppressive phenotype and is functionally close to the M2 subtype (59). Their high level of infiltration is associated with worse OS (60). Further studies showed that TAM-TREM2 expressing key genes of the complement system, C1Q, APOE, and SPP1, promote infiltration of Tex and Tregs as well as tumor proliferation (61) (Figure 3). Meanwhile, the TIDE algorithm predicted that signature genes of TAM-TREM2 are associated with immune resistance. It is proposed that this subset is expected to be a predictive biomarker for nCIT (60).
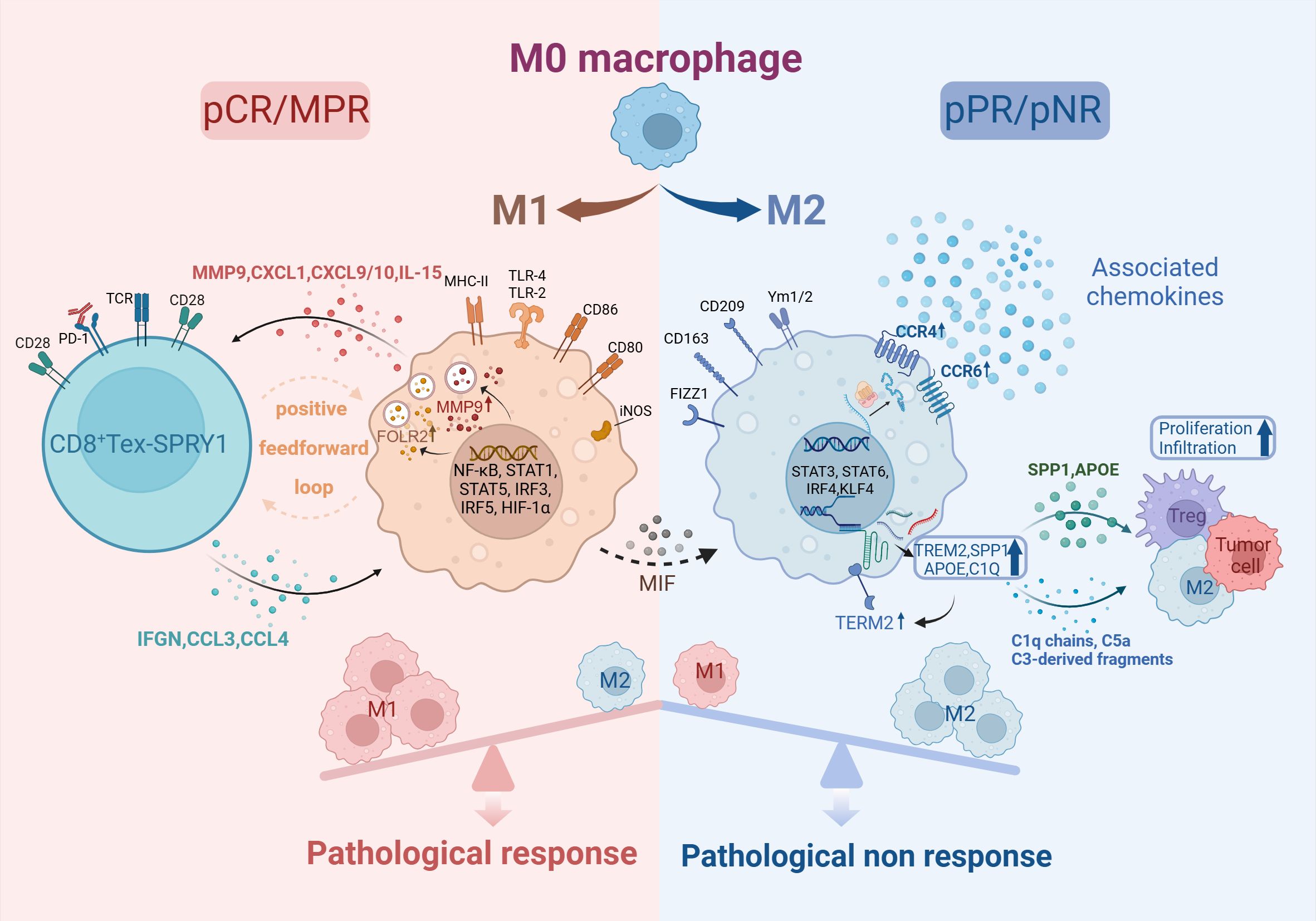
Figure 3. Macrophage in TME of ESCC. Created in BioRender. Q, Z. (2024) https://BioRender.com/b87g297.
3.4 Dendritic cell
In TME, dendritic cells (DCs), similar to macrophages, exert antigen-presenting function and regulate the immune response, thereby influencing tumor immune surveillance and escape (62). Among long non-coding RNAs, lncRNA ENST00000560647 has been shown to inhibit dendritic cell-mediated antigen presentation, leading to the inactivation of CD8+ T cells and the facilitation of immune evasion (63). DCs can differentiate into classic dendritic cell cDC and plasma cell-like dendritic cells pDC in TME (64). The current study found that the density of pDC in tumor tissues of patients not treated with nCIT is higher than in adjacent normal tissues, and then significantly decreased after receiving nCIT. This is consistent with the fact that pDCs produce IFN-α which leads to differentiation of Tregs and immune escape (65). The TCGA database survival analysis also illustrated that the characterized genes of pDC can differentiate patient survival well. Another DC subset, cDC, whose quantitative changes are mainly influenced by classical cDC type 1. cDC1 can activate CD4+ and CD8+ effector T cells, which are potentially responsive to immunotherapy (66). Therefore, the regulation of DC to exert its antitumor effects has become one of the hotspots in immunotherapy, including the use of tumor vaccines. Tumor vaccines utilize anti-CLEC9A antibodies to specifically deliver the immunogenic tumor antigen NY-ESO-1 to human CD141+ DC cells for anticancer therapy (67). Meanwhile, overexpression of MAGE-A3 and CALR on DCs to study their potential for generating anti-tumor immune responses is also one of them (68).
3.5 Natural killer cell
NK cells are the core cells of the organism’s innate immune cells. NK cells have a broader-spectrum immune role that not only is limited to fending off viruses but also has a crucial role in killing tumor cells (69). CD56+ is the characteristic gene of NK cells, and NK cells can be further categorized into two major subsets based on CD56 expression: CD56bright and CD56dim NK cells (70). CD56bright NK is the precursor cell of CD56dim NK (71), but CD56dim NK expresses higher levels of FCγ receptor III (CD16) and possesses stronger natural cytotoxicity and antitumor effects (72, 73). The present study found that CD16+ cells were less in tumor tissues than in normal tissues and were significantly higher after nCIT, while CD16- cells exhibited higher exhaustion scores (28). Meanwhile, CD56dim NK cells were significantly reduced in the responders compared to non-responders within the nCIT group, whereas no significant change was observed in the neoadjuvant chemotherapy group. And, the pCR group had more abundant CD56dim NK cells in the stromal area than the non-pCR group. These results show that CD16+CD56dim NK cells are associated with a better pathological response (55). ICB could activate numerous PD-1-expressing NK cells (74, 75), and CD16 could strongly self-activate NK cells (76). These all support an immunotherapeutic approach using modified NK cells to resist tumors, known as chimeric antigen receptor-NK (CAR-NK) cell therapy.
CAR-NK cell therapy, from adoptive cell therapy (ACTs), involves the transduction of a specially designed chimeric antigen receptor into NK cells. This enables CAR-NK cells to specifically recognize antigens on the surface of tumor cells and be activated, subsequently releasing cytotoxic molecules to eliminate tumor cells (77). Research on adoptive cell therapy, which began with tumor-infiltrating T cells, has demonstrated promising efficacy in hematological malignancies. However, the development of CAR-T therapy is constrained by issues related to infiltration into tumor tissues, as well as resistance to the tumor microenvironment (TME). Compared to CAR-T therapy, CAR-NK therapy can reduce the cytokine release syndrome (CRS) and neurological toxicities associated with CAR-T treatment (78). These advantages position CAR-NK as a potential “universal” product (79). CD19, CD20, and CD33 are the main targets for CAR-NK cells, which are frequently expressed in hematological malignancies (80). Additionally, CAR-NK cells have been shown to be effective against solid tumor targets such as Her2, EpCAM, and EGFR, which are common in colorectal and ovarian cancers (81–83). This broadens the applicability of CAR-NK cell therapy. Currently, CAR-NK cell therapy faces challenges such as viral transduction difficulties. Researchers are developing CAR-NK cells capable of recognizing multiple antigens to reduce the risk of tumor escape due to the loss of a single antigen (84). Moreover, CAR-NK cell therapy is being explored in combination with other treatment modalities to expand the applicability of immunotherapy. It is anticipated that ACTs will also become a milestone in immunological treatment.
3.6 B cells and tertiary lymphoid structure
B cells are the major humoral immune cells, exerting antibody-dependent cytotoxicity (ADCC) and antibody-dependent cellular phagocytosis (ADCP) (85). There is growing evidence that tumor-infiltrating B cells (TIL-B) and plasma cells have a crucial role in immunomodulation (86). One study showed a significant infiltration of B cells in a subgroup of “immune activation-dominant” samples. COL19A1, as a receptor for TIL-B, positively correlates with pathological responses and is expected to be a biomarker predicting the prognosis of nCIT (87). The present study classified TIL-B into five major subsets: naïve B cells (NBCs); activated B cells (ABCs); memory B cells (MBCs); germinal center B cells (GCBs), and the ASC (88). Two of these B cell subsets are sensitive to immunotherapy, GCB-LMO2 and CD55+ Bm. First, patients with a pathological response showed higher GCB cell infiltration than pathological non-response patients, and a significant increase in the interaction between the GCB-LMO2 and the CD4+ T-CXCL13 subsets was observed. GCB-LMO2 expresses the conventional GCB cell markers GL-7 and CD23, which are distributed within TLS and promote an antitumor immune response. CD55+ Bm belongs to the group of memory B cells that express relevant genes such as CD20, which are distributed outside the TLS. The expression of its signature gene, CD55, is down regulated with increased expression of LMO2. CD55+ Bm expresses ADGRE5, which inhibits CD8+ T cells and releases cytokines that interact with Treg cells to inhibit responsiveness to immunotherapy (Figure 4). It is illustrated that comprehensive B cell profile characteristics can predict nCIT responsiveness (89).
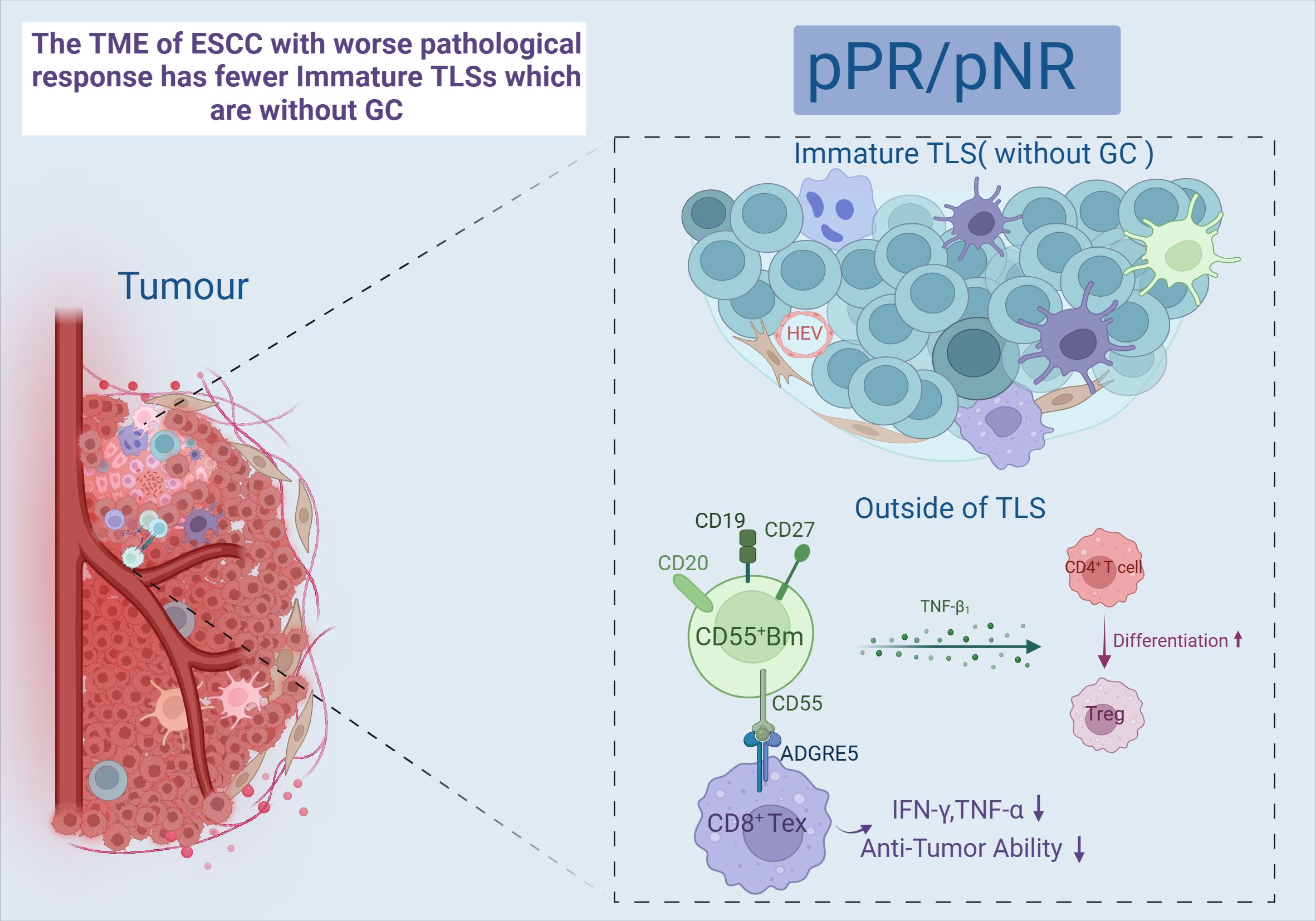
Figure 4. Immature TLS and CD55+Bm. Created in BioRender. Q, Z. (2024) https://BioRender.com/a41h264.
Tertiary lymphoid structure (TLS) refers to the organization of immune cell aggregates that resemble lymphoid tissues, formed within non-lymphoid tissues. In the physiological state, TLS is not present in normal tissues and is mainly found in the infiltrative margins of tumors (90). In recent years, TLS has been found to have a positive effect on the prognosis of many cancers, including lung cancer (91) and ovarian cancer (92). However, both RFS and OS were higher in the TLS-positive group than in the TLS-negative group, and the mature TLS group containing germinal centers (GCs) had better long-term efficacy and prognosis (93, 94). After nCIT, TME with abundant TLS was the most common type among the patients who achieved pCR or MPR (95, 96). Moreover, a study has developed a pathology image model using deep learning to quantitatively calculate the TLS ratio for predicting the pathological response to nCIT in ESCC. In TME, TLS is constructed by GCBs, T cells, and B cells (7). And it can only be activated in the presence of GCB-LMO2 cells. Meanwhile, patients with TLS high in CD55+ Bm cells and low in GCB-LMO2 cells were stratified as having the worst clinical outcome (89). This indicates that the antigen-presenting role of GCB cells contributes to the maturation of cytotoxic T cell (97). Follicular B cells (Bfo-NEIL1), which originate from GCB and express NEIL1, co-localize with CD8+ Tex-SPRY1 within TLS and exhibit better pathological responses. This co-localization demonstrates a synergistic interaction that significantly improves RFS. Bfo-NEIL1 cells express TNF and IL-23 to activate CD8+ Tex-SPRY1 and promote the positive feedforward cycle between Macro-MMP9 cells and CD8+ Tex-SPRY1. Additionally, CD4+ T-CXCL13 cells were induced by B-T interaction to activate the Tfh phenotype, which in turn activated CD8+ Tex-SPRY1 again. (Figure 5) Meanwhile, CD8+ Tex-SPRY1 induced the activity of germinal centers and the TLS, coinciding with the recent studies that the CD8+ response is present in the TLS (90, 98).
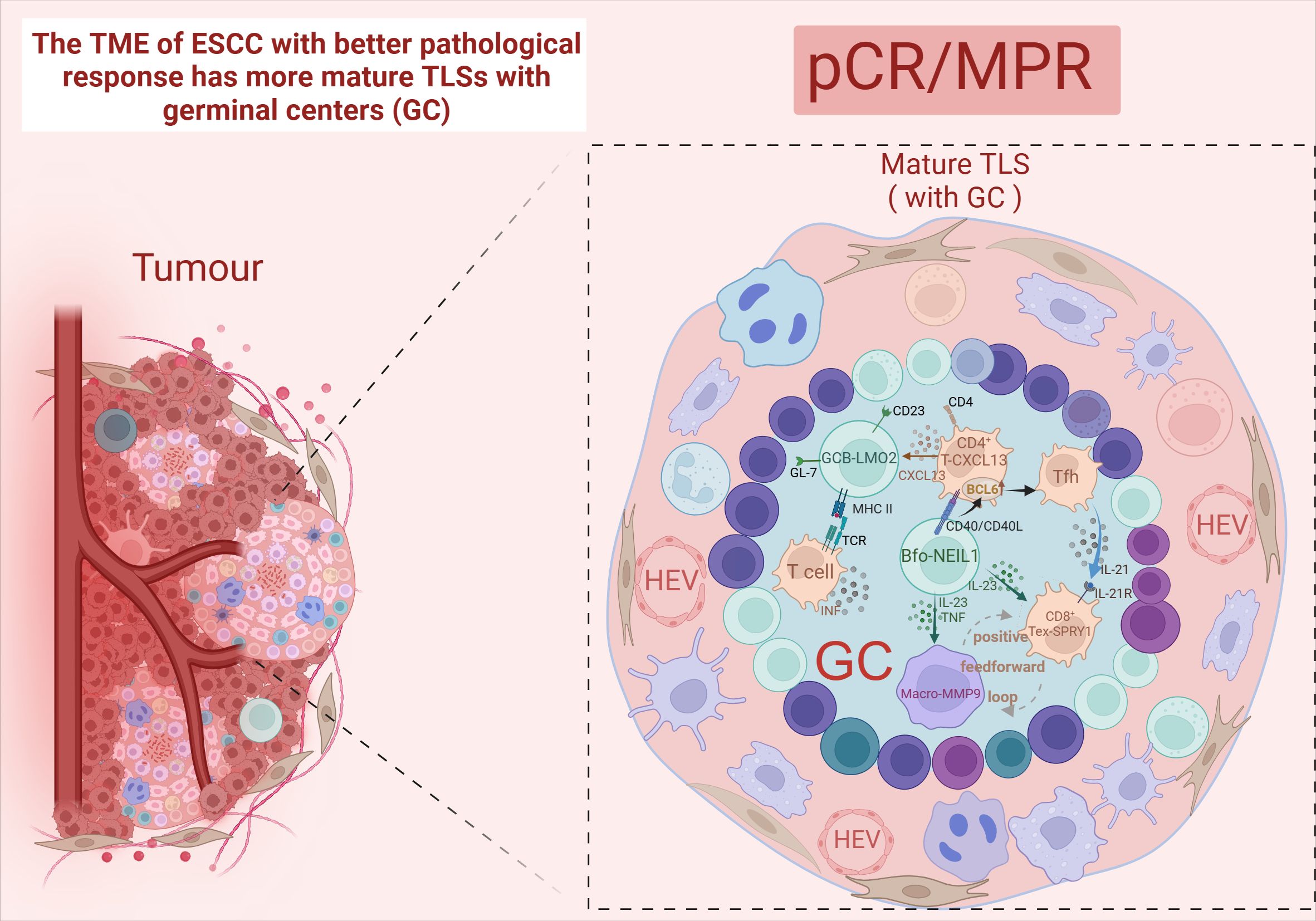
Figure 5. Mature TLS. Created in BioRender. Q, Z. (2024) https://BioRender.com/l91q142.
4 Interactions and spatial distribution of immune cells
It is well known that tumors can be viewed as complex systems (99). The response of tumor cells to treatment is closely related to the interaction of TME (100). In TME of ESCC, tumor-associated fibroblasts CAF expressing fibroblast activating protein (FAP) are distributed around the tumor. CAF attracts inhibitory TILs through paracrine signals and influences T cell penetration into the tumor center through direct physical interactions (101, 102). Within the TME, secretion of suppressive cytokines by the tumor leads to a progressive reduction in cytotoxic CD8+ T cells and NK cells, while concurrently promoting the expansion of Tex, Tregs, and regulatory B cells. Also, DCs exhibit maturation and functional defects. In contrast, patient samples that achieved pCR/MPR showed substantial immune activation and enrichment, which was able to reverse this TME. From this TME, we can observe the reactivation of Tex, self-activation of NK cells, positive feedforward loops of M1 macrophages with CD8+ T cells, and B-cell and T-cell interactions in the TLS. However, such reversal is rarely seen in samples of patients who achieved pPR/pNR (Figure 6). Therefore, some studies have classified TME into metabolic subtypes, mesenchymal, immune activated, and epithelial subtypes by characterized genes and noted that immune activated were associated with better prognosis and better pathological response (87).
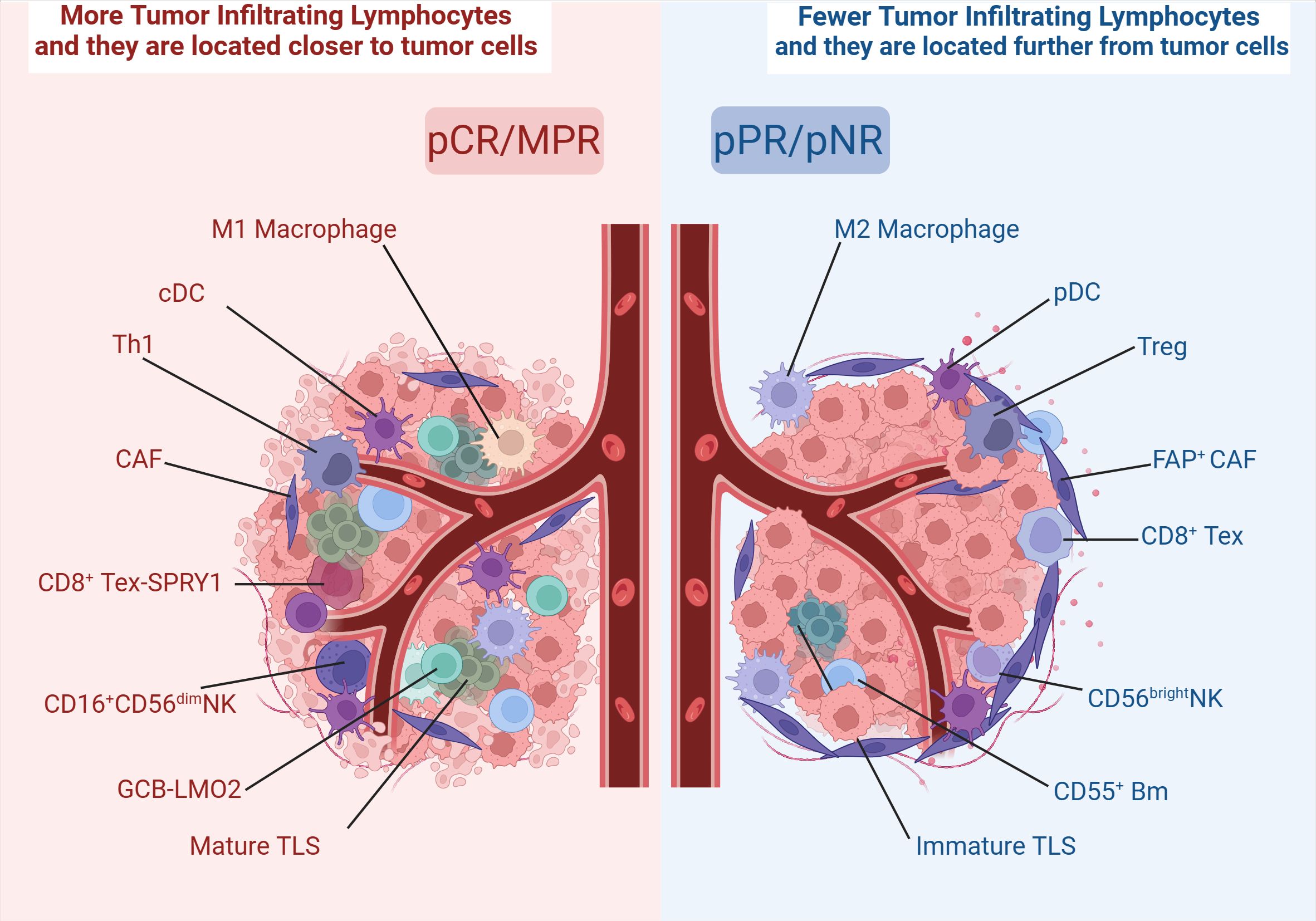
Figure 6. Different distribution of immune cells in TME. Created in BioRender. Q, Z. (2024) https://BioRender.com/r37r267.
To more finely delineate the landscape of TME in ESCC following nCIT, Yang et al. employed scRNA-seq and scTCR-seq to analyze samples from 18 patients with ESCC who underwent nCIT. The study divided the samples into four groups for analysis: pre-treatment, post-treatment, responders, and non-responders. Comparing post-treatment to pre-treatment, the study indicated minimal changes in the relative proportions of T cells, B cells, myeloid cells, and mast cells across all cellular constituents. However, significant changes were observed in epithelial cells, fibroblasts, endothelial cells, smooth muscle cells, and neurons. Among these, fibroblasts, endothelial cells, neurons, and smooth muscle cells increased significantly, while epithelial cells decreased. Similarly, when comparing responders to non-responders, the same effects were observed. This validated the overarching viewpoint of the paper. Furthermore, the study pointed out that in samples with responses and post-treatment, cell subpopulations CD8+ Tex-CXCL13, CD8+ Tex-STMN1, CD4+ Treg-TNFRSF4, tCAF-MMP11, Macro-SPP1, Macro-EREG, and Macro-STMN1 were significantly reduced, while CD8+ Tn-IL7R, Bmem_TNFSF13B cells, TLS, iCAF-CFD, iCAF-CXCL12, and iCAF-PLA2G2A were significantly increased in the parent cell populations (103).
On the other hand, spatial distance is also the basis for ensuring immune interactions within the tumor. Before nCIT, PD-1-positive cells were closer to tumor cells than PD-1- negative cells. CD4+ and CD8+ T cells that were 100 μm away from the tumor center were more likely to be activated with better immune response as well as having better correlation. Moreover, most DC and macrophage were distributed within 30 μm from the tumor cells, and patients with closer spatial proximity tended to have better immune responses (45). Wang et al. classified the post-treatment primary tumor into four patterns: Type I, Type II, Type III, and Type IV. In MPR or pCR cases, residual tumor cells were mainly found in the mucosa and submucosa, and less frequently in the lamina propria and epithelium, presenting a Type I pattern. In contrast, in pPR and pNR cases, most residual tumor cells were distributed in four layers, presenting Type IV (32).
5 Biomarker
The progression of cancer is characterized by heterogeneity, and the prospects for antitumor immunity within TME may vary among individual patients (31). Typical biomarkers, such as PD-L1 expression, microsatellite instability, and tumor mutation burden, seem to be inconsistent in predicting pathological responses in neoadjuvant therapies (37). We discuss recent studies on the alteration of TME in ESCC after nCIT and have compiled a list of the most recent relevant biomarkers. See Table 2.
Nowadays, many articles have identified certain genes or constructed models to predict pathological responses or clinical prognoses from existing databases. Jia et al. identified that eRNAAC005515.1, an enhancer eRNA, is associated with the local immune environment of ESCC and maybe a new biomarker for ESCC prognosis (104). Ma et al. analyzed patients’ clinical information and proposed AST, d -d-dimer, and CEA as independent predictors of objective remission rate after nCIT (105). There are also some researchers, who proposed different biomarkers from new fields. Huang et al. analyzed baseline respiratory samples from patients and proposed to predict the pathological response of ESCA patients by detecting volatile organic compounds (VOCs) in exhaled gas (106). Xu et al. analyzed stool samples from patients for 16S ribosomal ribonucleic acid (rRNA) V3-V4 sequencing and proposed the classification characteristics of the gut microbiome as a potential biomarker for predicting pathological responses and adverse reactions (107). Given the limitations of existing biomarkers, these emerging and novel biomarkers undoubtedly fill an exciting gap in this area.
6 Discussion and future prospects
Here, we discuss the alterations in TME following neoadjuvant therapy combined with immunotherapy and potential biomarkers (108). Nowadays, advances in sequencing technology provide more details about immune infiltrating cell interactions and spatial distribution. Thus, we have summarized and discussed the major alterations and interactions of immune cells in the TME of ESCC. We also compiled predictive biomarkers for the prognosis of ESCC based on these alterations. These will better and more finely stratify patients, providing a clear direction for effective anti-tumor therapy.
Author contributions
ZQ: Conceptualization, Data curation, Formal analysis, Investigation, Software, Visualization, Writing – original draft, Writing – review & editing. CYG: Funding acquisition, Methodology, Project administration, Writing – original draft, Writing – review & editing. ZL: Investigation, Supervision, Writing – original draft, Writing – review & editing. XL: Data curation, Software, Writing – review & editing. RZ: Formal analysis, Resources, Visualization, Writing – review & editing. YL: Formal analysis, Writing – review & editing. YB: Conceptualization, Formal analysis, Software, Supervision, Visualization, Writing – review & editing. CGG: Investigation, Methodology, Software, Writing – review & editing. XM: Software, Visualization, Writing – review & editing. MZ: Conceptualization, Formal analysis, Writing – review & editing.
Funding
The author(s) declare financial support was received for the research, authorship, and/or publication of this article. This study was supported by grants from the Natural Science Foundation of Jiangxi Province (#20203BBGL73151) and the Investigator Initiated Trial (IIT) Project of Jiangxi Cancer Hospital (#IIT2023YB01). The publication fee for this paper, 3,295$, is covered by project #IIT2023YB01, and the costs associated with producing the paper's charts and figures, are covered by #2023BBGL73151.
Conflict of interest
The authors declare that the research was conducted in the absence of any commercial or financial relationships that could be construed as a potential conflict of interest.
Publisher’s note
All claims expressed in this article are solely those of the authors and do not necessarily represent those of their affiliated organizations, or those of the publisher, the editors and the reviewers. Any product that may be evaluated in this article, or claim that may be made by its manufacturer, is not guaranteed or endorsed by the publisher.
References
1. Chen W, Zheng R, Baade PD, Zhang S, Zeng H, Bray F, et al. Cancer statistics in China, 2015. CA Cancer J Clin. (2016) 66:115–32. doi: 10.3322/caac.21338
2. Gao Z, Huang S, Wang S, Tang D, Xu W, Zeng R, et al. Efficacy and safety of immunochemotherapy, immunotherapy, chemotherapy, and targeted therapy as first-line treatment for advanced and metastatic esophageal cancer: a systematic review and network meta-analysis. Lancet Reg Health West Pac. (2023) 38:100841. doi: 10.1016/j.lanwpc.2023.100841
3. Zhu H, Ma X, Ye T, Wang H, Wang Z, Liu Q, et al. Esophageal cancer in China: Practice and research in the new era. Int J Cancer. (2023) 152:1741–51. doi: 10.1002/ijc.v152.9
4. Leng X-F, Daiko H, Han Y-T, Mao Y-S. Optimal preoperative neoadjuvant therapy for resectable locally advanced esophageal squamous cell carcinoma. Ann N Y Acad Sci. (2020) 1482:213–24. doi: 10.1111/nyas.v1482.1
5. Ulla M, Gentile EMJ, Cavadas D, Yeyati EL, Frank L, Argerich JI, et al. Esophageal cancer characterization with pneumo-64-MDCT. Abdom Imaging. (2012) 37:501–11. doi: 10.1007/s00261-011-9784-z
6. Topalian SL, Taube JM, Pardoll DM. Neoadjuvant checkpoint blockade for cancer immunotherapy. Science. (2020) 367:eaax0182. doi: 10.1126/science.aax0182
7. Liu J, Chen H, Qiao G, Zhang J-T, Zhang S, Zhu C, et al. PLEK2 and IFI6, representing mesenchymal and immune-suppressive microenvironment, predicts resistance to neoadjuvant immunotherapy in esophageal squamous cell carcinoma. Cancer Immunol Immunother. (2023) 72:881–93. doi: 10.1007/s00262-022-03288-0
8. Park R, Da Silva LL, Saeed A. Immunotherapy predictive molecular markers in advanced gastroesophageal cancer: MSI and beyond. Cancers (Basel). (2021) 13:1715. doi: 10.3390/cancers13071715
9. Shapiro J, van Lanschot JJB, Hulshof MCCM, van Hagen P, van Berge Henegouwen MI, Wijnhoven BPL, et al. Neoadjuvant chemoradiotherapy plus surgery versus surgery alone for oesophageal or junctional cancer (CROSS): long-term results of a randomised controlled trial. Lancet Oncol. (2015) 16:1090–8. doi: 10.1016/S1470-2045(15)00040-6
10. Yang H, Liu H, Chen Y, Zhu C, Fang W, Yu Z, et al. Neoadjuvant chemoradiotherapy followed by surgery versus surgery alone for locally advanced squamous cell carcinoma of the esophagus (NEOCRTEC5010): A phase III multicenter, randomized, open-label clinical trial. J Clin Oncol. (2018) 36:2796–803. doi: 10.1200/JCO.2018.79.1483
11. Huang J, Xu J, Chen Y, Zhuang W, Zhang Y, Chen Z, et al. Camrelizumab versus investigator's choice of chemotherapy as second-line therapy for advanced or metastatic oesophageal squamous cell carcinoma (ESCORT): a multicentre, randomised, open-label, phase 3 study. Lancet Oncol. (2020) 21:832–42. doi: 10.1016/S1470-2045(20)30110-8
12. Kojima T, Shah MA, Muro K, Francois E, Adenis A, Hsu C-H, et al. Randomized phase III KEYNOTE-181 study of pembrolizumab versus chemotherapy in advanced esophageal cancer. J Clin Oncol. (2020) 38:4138–48. doi: 10.1200/JCO.20.01888
13. Doki Y, Ajani JA, Kato K, Xu J, Wyrwicz L, Motoyama S, et al. Nivolumab combination therapy in advanced esophageal squamous-cell carcinoma. N Engl J Med. (2022) 386:449–62. doi: 10.1056/NEJMoa2111380
14. Liu J, Yang Y, Liu Z, Fu X, Cai X, Li H, et al. Multicenter, single-arm, phase II trial of camrelizumab and chemotherapy as neoadjuvant treatment for locally advanced esophageal squamous cell carcinoma. J Immunother Cancer. (2022) 10:e004291. doi: 10.1136/jitc-2021-004291
15. Tang Z, Wang Y, Liu D, Wang X, Xu C, Yu Y, et al. The Neo-PLANET phase II trial of neoadjuvant camrelizumab plus concurrent chemoradiotherapy in locally advanced adenocarcinoma of stomach or gastroesophageal junction. Nat Commun. (2022) 13:6807. doi: 10.1038/s41467-022-34403-5
16. Liu Y. Perioperative immunotherapy for esophageal squamous cell carcinoma: Now and future. World J Gastroenterol. (2023) 29:5020–37. doi: 10.3748/wjg.v29.i34.5020
17. Xu J, Cai Y, Hong Z, Duan H, Ke S. Comparison of efficacy and safety between neoadjuvant chemotherapy and neoadjuvant immune checkpoint inhibitors combined with chemotherapy for locally advanced esophageal squamous cell carcinoma: a systematic review and meta-analysis. Int J Surg. (2024) 110:490–506. 10.1097/JS9.0000000000000816
18. Sorin M, Prosty C, Ghaleb L, Nie K, Katergi K, Shahzad MH, et al. Neoadjuvant chemoimmunotherapy for NSCLC: A systematic review and meta-analysis. JAMA Oncol. (2024) 10:621–33. doi: 10.1001/jamaoncol.2024.0057
19. Wang J, Tong T, Zhang G, Jin C, Guo H, Liu X, et al. Evaluation of neoadjuvant immunotherapy in resectable gastric/gastroesophageal junction tumors: a meta-analysis and systematic review. Front Immunol. (2024) 15:1339757. doi: 10.3389/fimmu.2024.1339757
20. Long GV, Menzies AM, Scolyer RA. Neoadjuvant checkpoint immunotherapy and melanoma: the time is now. J Clin Oncol. (2023) 41:3236–48. doi: 10.1200/JCO.22.02575
21. Noma T, Makino T, Ohshima K, Sugimura K, Miyata H, Honma K, et al. Immunoscore signatures in surgical specimens and tumor-infiltrating lymphocytes in pretreatment biopsy predict treatment efficacy and survival in esophageal cancer. Ann Surg. (2023) 277:e528–e37. doi: 10.1097/SLA.0000000000005104
22. Fassan M, Cavallin F, Guzzardo V, Kotsafti A, Scarpa M, Cagol M, et al. PD-L1 expression, CD8+ and CD4+ lymphocyte rate are predictive of pathological complete response after neoadjuvant chemoradiotherapy for squamous cell cancer of the thoracic esophagus. Cancer Med. (2019) 8:6036–48. doi: 10.1002/cam4.v8.13
23. Li Q, Liu T, Ding Z. Neoadjuvant immunotherapy for resectable esophageal cancer: A review. Front Immunol. (2022) 13:1051841. doi: 10.3389/fimmu.2022.1051841
24. Ellis GI, Riley JL. How to kill Treg cells for immunotherapy. Nat Cancer. (2020) 1:1134–5. doi: 10.1038/s43018-020-00155-8
25. Fares CM, Van Allen EM, Drake CG, Allison JP, Hu-Lieskovan S. Mechanisms of resistance to immune checkpoint blockade: why does checkpoint inhibitor immunotherapy not work for all patients? Am Soc Clin Oncol Educ Book. (2019) 39:147–64. doi: 10.1200/EDBK_240837
26. Galbo PM, Zang X, Zheng D. Molecular features of cancer-associated fibroblast subtypes and their implication on cancer pathogenesis, prognosis, and immunotherapy resistance. Clin Cancer Res. (2021) 27:2636–47. doi: 10.1158/1078-0432.CCR-20-4226
27. Fuchs CS, Doi T, Jang RW, Muro K, Satoh T, Machado M, et al. Safety and efficacy of pembrolizumab monotherapy in patients with previously treated advanced gastric and gastroesophageal junction cancer: phase 2 clinical KEYNOTE-059 trial. JAMA Oncol. (2018) 4:e180013. doi: 10.1001/jamaoncol.2018.0013
28. Han D, Han Y, Guo W, Wei W, Yang S, Xiang J, et al. High-dimensional single-cell proteomics analysis of esophageal squamous cell carcinoma reveals dynamic alterations of the tumor immune microenvironment after neoadjuvant therapy. J Immunother Cancer. (2023) 11:e007847. doi: 10.1136/jitc-2023-007847
29. Liu Z, Zhang Y, Ma N, Yang Y, Ma Y, Wang F, et al. Progenitor-like exhausted SPRY1+CD8+ T cells potentiate responsiveness to neoadjuvant PD-1 blockade in esophageal squamous cell carcinoma. Cancer Cell. (2023) 41:1852–70.e9. doi: 10.1016/j.ccell.2023.09.011
30. St Paul M, Ohashi PS. The roles of CD8+ T cell subsets in antitumor immunity. Trends Cell Biol. (2020) 30:695–704. doi: 10.1016/j.tcb.2020.06.003
31. Huang T-X, Fu L. The immune landscape of esophageal cancer. Cancer Commun (Lond). (2019) 39:79. doi: 10.1186/s40880-019-0427-z
32. Wang H, Jiang Z, Wang Q, Wu T, Guo F, Xu Z, et al. Pathological response and prognostic factors of neoadjuvant PD-1 blockade combined with chemotherapy in resectable oesophageal squamous cell carcinoma. Eur J Cancer. (2023) 186:196–210. doi: 10.1016/j.ejca.2023.03.008
33. Yu Y, Ma X, Gong R, Zhu J, Wei L, Yao J. Recent advances in CD8+ regulatory T cell research. Oncol Lett. (2018) 15:8187–94. doi: 10.3892/ol.2018.8378
34. Zhang S, Fujita H, Mitsui H, Yanofsky VR, Fuentes-Duculan J, Pettersen JS, et al. Increased Tc22 and Treg/CD8 ratio contribute to aggressive growth of transplant associated squamous cell carcinoma. PLoS One. (2013) 8:e62154. doi: 10.1371/journal.pone.0062154
36. Doering TA, Crawford A, Angelosanto JM, Paley MA, Ziegler CG, Wherry EJ. Network analysis reveals centrally connected genes and pathways involved in CD8+ T cell exhaustion versus memory. Immunity. (2012) 37:1130–44. doi: 10.1016/j.immuni.2012.08.021
37. Zhou Q, Yuan J, Wu K, Yin J, Tan L. Neoadjuvant immunotherapy for resectable esophagal squamous cell carcinoma: An ace up the sleeve. Clin Transl Med. (2023) 13:e1426. doi: 10.1002/ctm2.v13.9
38. Yang S, Gattinoni L, Liu F, Ji Y, Yu Z, Restifo NP, et al. In vitro generated anti-tumor T lymphocytes exhibit distinct subsets mimicking in vivo antigen-experienced cells. Cancer Immunol Immunother. (2011) 60:739–49. doi: 10.1007/s00262-011-0977-7
39. Terrén I, Orrantia A, Vitallé J, Zenarruzabeitia O, Borrego F. NK cell metabolism and tumor microenvironment. Front Immunol. (2019) 10:2278. doi: 10.3389/fimmu.2019.02278
40. Blackburn SD, Shin H, Haining WN, Zou T, Workman CJ, Polley A, et al. Coregulation of CD8+ T cell exhaustion by multiple inhibitory receptors during chronic viral infection. Nat Immunol. (2009) 10:29–37. doi: 10.1038/ni.1679
41. Kallies A, Zehn D, Utzschneider DT. Precursor exhausted T cells: key to successful immunotherapy? Nat Rev Immunol. (2020) 20:128–36. doi: 10.1038/s41577-019-0223-7
42. McLane LM, Abdel-Hakeem MS, Wherry EJ. CD8 T cell exhaustion during chronic viral infection and cancer. Annu Rev Immunol. (2019) 37:457–95. doi: 10.1146/annurev-immunol-041015-055318
43. Topalian SL, Hodi FS, Brahmer JR, Gettinger SN, Smith DC, McDermott DF, et al. Safety, activity, and immune correlates of anti-PD-1 antibody in cancer. N Engl J Med. (2012) 366:2443–54. doi: 10.1056/NEJMoa1200690
44. Xu X, Sun Z, Liu Q, Zhang Y, Shen L, Zhang C, et al. Neoadjuvant chemoradiotherapy combined with sequential perioperative toripalimab in locally advanced esophageal squamous cell cancer. J Immunother Cancer. (2024) 12:e008631. doi: 10.1136/jitc-2023-008631
45. Yan C, Huang H, Zheng Z, Ma X, Zhao G, Zhang T, et al. Spatial distribution of tumor-infiltrating T cells indicated immune response status under chemoradiotherapy plus PD-1 blockade in esophageal cancer. Front Immunol. (2023) 14:1138054. doi: 10.3389/fimmu.2023.1138054
46. Zheng Y, Chen Z, Han Y, Han L, Zou X, Zhou B, et al. Immune suppressive landscape in the human esophageal squamous cell carcinoma microenvironment. Nat Commun. (2020) 11:6268. doi: 10.1038/s41467-020-20019-0
47. Huang AC, Postow MA, Orlowski RJ, Mick R, Bengsch B, Manne S, et al. T-cell invigoration to tumour burden ratio associated with anti-PD-1 response. Nature. (2017) 545:60–5. doi: 10.1038/nature22079
48. Han Y, Guo Q, Zhang M, Chen Z, Cao X. CD69+ CD4+ CD25- T cells, a new subset of regulatory T cells, suppress T cell proliferation through membrane-bound TGF-beta 1. J Immunol. (2009) 182:111–20. doi: 10.4049/jimmunol.182.1.111
49. Duan H, Shao C, Pan M, Liu H, Dong X, Zhang Y, et al. Neoadjuvant pembrolizumab and chemotherapy in resectable esophageal cancer: an open-label, single-arm study (PEN-ICE). Front Immunol. (2022) 13:849984. doi: 10.3389/fimmu.2022.849984
50. Song Q, Hawkins GA, Wudel L, Chou P-C, Forbes E, Pullikuth AK, et al. Dissecting intratumoral myeloid cell plasticity by single cell RNA-seq. Cancer Med. (2019) 8:3072–85. doi: 10.1002/cam4.2019.8.issue-6
51. Collin M, Bigley V. Human dendritic cell subsets: an update. Immunology. (2018) 154:3–20. doi: 10.1111/imm.2018.154.issue-1
52. Ma X, Guo Z, Wei X, Zhao G, Han D, Zhang T, et al. Spatial distribution and predictive significance of dendritic cells and macrophages in esophageal cancer treated with combined chemoradiotherapy and PD-1 blockade. Front Immunol. (2021) 12:786429. doi: 10.3389/fimmu.2021.786429
53. Bi K, He MX, Bakouny Z, Kanodia A, Napolitano S, Wu J, et al. Tumor and immune reprogramming during immunotherapy in advanced renal cell carcinoma. Cancer Cell. (2021) 39:649–61.e5. doi: 10.1016/j.ccell.2021.02.015
54. Jimenez O, Barros MH, De Matteo E, Garcia Lombardi M, Preciado MV, Niedobitek G, et al. M1-like macrophage polarization prevails in young children with classic Hodgkin Lymphoma from Argentina. Sci Rep. (2019) 9:12687. doi: 10.1038/s41598-019-49015-1
55. Wang S, Xu G, Li M, Zheng J, Wang Y, Feng X, et al. M1 macrophage predicted efficacy of neoadjuvant camrelizumab combined with chemotherapy vs chemotherapy alone for locally advanced ESCC: A pilot study. Front Oncol. (2023) 13:1139990. doi: 10.3389/fonc.2023.1139990
56. Vitale I, Manic G, Coussens LM, Kroemer G, Galluzzi L. Macrophages and metabolism in the tumor microenvironment. Cell Metab. (2019) 30:36–50. doi: 10.1016/j.cmet.2019.06.001
57. Yang W, Xing X, Yeung S-CJ, Wang S, Chen W, Bao Y, et al. Neoadjuvant programmed cell death 1 blockade combined with chemotherapy for resectable esophageal squamous cell carcinoma. J Immunother Cancer. (2022) 10:e003497. doi: 10.1136/jitc-2021-003497
58. Xiang X, Wang J, Lu D, Xu X. Targeting tumor-associated macrophages to synergize tumor immunotherapy. Signal Transduct Target Ther. (2021) 6:75. doi: 10.1038/s41392-021-00484-9
59. Liu Z, Gao Z, Li B, Li J, Ou Y, Yu X, et al. Lipid-associated macrophages in the tumor-adipose microenvironment facilitate breast cancer progression. Oncoimmunology. (2022) 11:2085432. doi: 10.1080/2162402X.2022.2085432
60. Li H, Miao Y, Zhong L, Feng S, Xu Y, Tang L, et al. Identification of TREM2-positive tumor-associated macrophages in esophageal squamous cell carcinoma: implication for poor prognosis and immunotherapy modulation. Front Immunol. (2023) 14:1162032. doi: 10.3389/fimmu.2023.1162032
61. Afshar-Kharghan V. The role of the complement system in cancer. J Clin Invest. (2017) 127:780–9. doi: 10.1172/JCI90962
62. Liu Z, Wang H, Li Z, Dress RJ, Zhu Y, Zhang S, et al. Dendritic cell type 3 arises from Ly6C+ monocyte-dendritic cell progenitors. Immunity. (2023) 56:1761–77.e6. doi: 10.1016/j.immuni.2023.07.001
63. Liu J, Zhou W-Y, Luo X-J, Chen Y-X, Wong C-W, Liu Z-X, et al. Long noncoding RNA Regulating ImMune Escape regulates mixed lineage leukaemia protein-1-H3K4me3-mediated immune escape in oesophageal squamous cell carcinoma. Clin Transl Med. (2023) 13:e1410. doi: 10.1002/ctm2.v13.9
64. Satpathy AT, Wu X, Albring JC, Murphy KM. Re(de)fining the dendritic cell lineage. Nat Immunol. (2012) 13:1145–54. doi: 10.1038/ni.2467
65. Kießler M, Plesca I, Sommer U, Wehner R, Wilczkowski F, Müller L, et al. Tumor-infiltrating plasmacytoid dendritic cells are associated with survival in human colon cancer. J Immunother Cancer. (2021) 9:e001813. doi: 10.1136/jitc-2020-001813
66. Spranger S, Dai D, Horton B, Gajewski TF. Tumor-residing batf3 dendritic cells are required for effector T cell trafficking and adoptive T cell therapy. Cancer Cell. (2017) 31:711–23.e4. doi: 10.1016/j.ccell.2017.04.003
67. Masterman K-A, Haigh OL, Tullett KM, Leal-Rojas IM, Walpole C, Pearson FE, et al. Human CLEC9A antibodies deliver NY-ESO-1 antigen to CD141+ dendritic cells to activate naïve and memory NY-ESO-1-specific CD8+ T cells. J Immunother Cancer. (2020) 8:e000691. doi: 10.1136/jitc-2020-000691
68. Liu X, Song N, Liu Y, Liu Y, Li J, Ding J, et al. Efficient induction of anti-tumor immune response in esophageal squamous cell carcinoma via dendritic cells expressing MAGE-A3 and CALR antigens. Cell Immunol. (2015) 295:77–82. doi: 10.1016/j.cellimm.2015.03.011
69. Perricone R, Perricone C, De Carolis C, Shoenfeld Y. NK cells in autoimmunity: a two-edg'd weapon of the immune system. Autoimmun Rev. (2008) 7:384–90. doi: 10.1016/j.autrev.2008.03.002
70. Zhou Y, Cheng L, Liu L, Li X. NK cells are never alone: crosstalk and communication in tumour microenvironments. Mol Cancer. (2023) 22:34. doi: 10.1186/s12943-023-01737-7
71. Romagnani C, Juelke K, Falco M, Morandi B, D'Agostino A, Costa R, et al. CD56brightCD16- killer Ig-like receptor- NK cells display longer telomeres and acquire features of CD56dim NK cells upon activation. J Immunol. (2007) 178:4947–55. doi: 10.4049/jimmunol.178.8.4947
72. Jin M, Günther R, Akgün K, Hermann A, Ziemssen T. Peripheral proinflammatory Th1/Th17 immune cell shift is linked to disease severity in amyotrophic lateral sclerosis. Sci Rep. (2020) 10:5941. doi: 10.1038/s41598-020-62756-8
73. Van Acker HH, Van Acker ZP, Versteven M, Ponsaerts P, Pende D, Berneman ZN, et al. CD56 homodimerization and participation in anti-tumor immune effector cell functioning: A role for interleukin-15. Cancers (Basel). (2019) 11:1029. doi: 10.3390/cancers11071029
74. Hsu J, Hodgins JJ, Marathe M, Nicolai CJ, Bourgeois-Daigneault M-C, Trevino TN, et al. Contribution of NK cells to immunotherapy mediated by PD-1/PD-L1 blockade. J Clin Invest. (2018) 128:4654–68. doi: 10.1172/JCI99317
75. Pahl JHW, Koch J, Götz J-J, Arnold A, Reusch U, Gantke T, et al. CD16A activation of NK cells promotes NK cell proliferation and memory-like cytotoxicity against cancer cells. Cancer Immunol Res. (2018) 6:517–27. doi: 10.1158/2326-6066.CIR-17-0550
76. Bryceson YT, March ME, Ljunggren H-G, Long EO. Activation, coactivation, and costimulation of resting human natural killer cells. Immunol Rev. (2006) 214:73–91. doi: 10.1111/j.1600-065X.2006.00457.x
77. Khawar MB, Sun H. CAR-NK cells: from natural basis to design for kill. Front Immunol. (2021) 12:707542. doi: 10.3389/fimmu.2021.707542
78. Liu E, Marin D, Banerjee P, Macapinlac HA, Thompson P, Basar R, et al. Use of CAR-transduced natural killer cells in CD19-positive lymphoid tumors. N Engl J Med. (2020) 382:545–53. doi: 10.1056/NEJMoa1910607
79. Marin D, Li Y, Basar R, Rafei H, Daher M, Dou J, et al. Safety, efficacy and determinants of response of allogeneic CD19-specific CAR-NK cells in CD19+ B cell tumors: a phase 1/2 trial. Nat Med. (2024) 30:772–84. doi: 10.1038/s41591-023-02785-8
80. Bahramloo M, Shahabi SA, Kalarestaghi H, Rafat A, Mazloumi Z, Samimifar A, et al. CAR-NK cell therapy in AML: Current treatment, challenges, and advantage. BioMed Pharmacother. (2024) 177:117024. doi: 10.1016/j.biopha.2024.117024
81. Schnalzger TE, de Groot MH, Zhang C, Mosa MH, Michels BE, Röder J, et al. 3D model for CAR-mediated cytotoxicity using patient-derived colorectal cancer organoids. EMBO J. (2019) 38:e100928. doi: 10.15252/embj.2018100928
82. Li T, Niu M, Zhang W, Qin S, Zhou J, Yi M. CAR-NK cells for cancer immunotherapy: recent advances and future directions. Front Immunol. (2024) 15:1361194. doi: 10.3389/fimmu.2024.1361194
83. Maalej KM, Merhi M, Inchakalody VP, Mestiri S, Alam M, Maccalli C, et al. CAR-cell therapy in the era of solid tumor treatment: current challenges and emerging therapeutic advances. Mol Cancer. (2023) 22:20. doi: 10.1186/s12943-023-01723-z
84. He J, Yan Y, Zhang J, Wei Z, Li H, Xing L. Synergistic treatment strategy: combining CAR-NK cell therapy and radiotherapy to combat solid tumors. Front Immunol. (2023) 14:1298683. doi: 10.3389/fimmu.2023.1298683
85. Gilbert AE, Karagiannis P, Dodev T, Koers A, Lacy K, Josephs DH, et al. Monitoring the systemic human memory B cell compartment of melanoma patients for anti-tumor IgG antibodies. PLoS One. (2011) 6:e19330. doi: 10.1371/journal.pone.0019330
86. Laumont CM, Banville AC, Gilardi M, Hollern DP, Nelson BH. Tumour-infiltrating B cells: immunological mechanisms, clinical impact and therapeutic opportunities. Nat Rev Cancer. (2022) 22:414–30. doi: 10.1038/s41568-022-00466-1
87. Liu J-H, Lin J-Z, Qiu Q, Zhu C, Li W, Li Q, et al. COL19A1 is a predictive biomarker for the responsiveness of esophageal squamous cell carcinoma patients to immune checkpoint therapy. Thorac Cancer. (2023) 14:1294–305. doi: 10.1111/1759-7714.14873
88. Ellebedy AH, Jackson KJL, Kissick HT, Nakaya HI, Davis CW, Roskin KM, et al. Defining antigen-specific plasmablast and memory B cell subsets in human blood after viral infection or vaccination. Nat Immunol. (2016) 17:1226–34. doi: 10.1038/ni.3533
89. Zhang H, Wen H, Zhu Q, Zhang Y, Xu F, Ma T, et al. Genomic profiling and associated B cell lineages delineate the efficacy of neoadjuvant anti-PD-1-based therapy in oesophageal squamous cell carcinoma. EBioMedicine. (2024) 100:104971. doi: 10.1016/j.ebiom.2024.104971
90. Schumacher TN, Thommen DS. Tertiary lymphoid structures in cancer. Science. (2022) 375:eabf9419. doi: 10.1126/science.abf9419
91. Germain C, Gnjatic S, Tamzalit F, Knockaert S, Remark R, Goc J, et al. Presence of B cells in tertiary lymphoid structures is associated with a protective immunity in patients with lung cancer. Am J Respir Crit Care Med. (2014) 189:832–44. doi: 10.1164/rccm.201309-1611OC
92. Nielsen JS, Sahota RA, Milne K, Kost SE, Nesslinger NJ, Watson PH, et al. CD20+ tumor-infiltrating lymphocytes have an atypical CD27- memory phenotype and together with CD8+ T cells promote favorable prognosis in ovarian cancer. Clin Cancer Res. (2012) 18:3281–92. doi: 10.1158/1078-0432.CCR-12-0234
93. Posch F, Silina K, Leibl S, Mündlein A, Moch H, Siebenhüner A, et al. Maturation of tertiary lymphoid structures and recurrence of stage II and III colorectal cancer. Oncoimmunology. (2018) 7:e1378844. doi: 10.1080/2162402X.2017.1378844
94. Nakamura S, Ohuchida K, Hayashi M, Katayama N, Tsutsumi C, Yamada Y, et al. Tertiary lymphoid structures correlate with enhancement of antitumor immunity in esophageal squamous cell carcinoma. Br J Cancer. (2023) 129:1314–26. doi: 10.1038/s41416-023-02396-7
95. Wang X, Ling X, Wang C, Zhang J, Yang Y, Jiang H, et al. Response to neoadjuvant immune checkpoint inhibitors and chemotherapy in Chinese patients with esophageal squamous cell carcinoma: the role of tumor immune microenvironment. Cancer Immunol Immunother. (2023) 72:1619–31. doi: 10.1007/s00262-022-03354-7
96. Wu L, Gao Y, Xie S, Ye W, Uemura Y, Zhang R, et al. The level of macrophage migration inhibitory factor is negatively correlated with the efficacy of PD-1 blockade immunotherapy combined with chemotherapy as a neoadjuvant therapy for esophageal squamous cell carcinoma. Transl Oncol. (2023) 37:101775. doi: 10.1016/j.tranon.2023.101775
97. Li R, Huang X, Yang W, Wang J, Liang Y, Zhang T, et al. Tertiary lymphoid structures favor outcome in resected esophageal squamous cell carcinoma. J Pathol Clin Res. (2022) 8:422–35. doi: 10.1002/cjp2.v8.5
98. Thommen DS, Koelzer VH, Herzig P, Roller A, Trefny M, Dimeloe S, et al. A transcriptionally and functionally distinct PD-1+ CD8+ T cell pool with predictive potential in non-small-cell lung cancer treated with PD-1 blockade. Nat Med. (2018) 24:994–1004. doi: 10.1038/s41591-018-0057-z
99. de Visser KE, Joyce JA. The evolving tumor microenvironment: From cancer initiation to metastatic outgrowth. Cancer Cell. (2023) 41:374–403. doi: 10.1016/j.ccell.2023.02.016
100. Deepak KGK, Vempati R, Nagaraju GP, Dasari VR, S N, Rao DN, et al. Tumor microenvironment: Challenges and opportunities in targeting metastasis of triple negative breast cancer. Pharmacol Res. (2020) 153:104683. doi: 10.1016/j.phrs.2020.104683
101. LeBleu VS, Kalluri R. A peek into cancer-associated fibroblasts: origins, functions and translational impact. Dis Model Mech. (2018) 11:dmm029447. doi: 10.1242/dmm.029447
102. Watanabe S, Noma K, Ohara T, Kashima H, Sato H, Kato T, et al. Photoimmunotherapy for cancer-associated fibroblasts targeting fibroblast activation protein in human esophageal squamous cell carcinoma. Cancer Biol Ther. (2019) 20:1234–48. doi: 10.1080/15384047.2019.1617566
103. Yang Z, Tian H, Chen X, Li B, Bai G, Cai Q, et al. Single-cell sequencing reveals immune features of treatment response to neoadjuvant immunochemotherapy in esophageal squamous cell carcinoma. Nat Commun. (2024) 15:9097. doi: 10.1038/s41467-024-52977-0
104. Jia L, Chen J, Zhao J, Yang J. Identification of enhancer RNA AC005515.1 as a novel biomarker for prognosis in esophageal cancer and predictors of immunotherapy response. Transl Cancer Res. (2023) 12:3266–83. doi: 10.21037/tcr-23-777
105. Ma R, Yuan D, Mo C, Zhu K, Dang C, Zhang Y, et al. Factors affecting the ORR after neoadjuvant therapy of TP regimen combined with PD-1 inhibitors for esophageal cancer. Sci Rep. (2023) 13:6080. doi: 10.1038/s41598-023-33038-w
106. Huang Q, Liu Z, Yu Y, Rong Z, Wang P, Wang S, et al. Prediction of response to neoadjuvant chemo-immunotherapy in patients with esophageal squamous cell carcinoma by a rapid breath test. Br J Cancer. (2024) 130:694–700. doi: 10.1038/s41416-023-02547-w
107. Xu L, Qi Y, Jiang Y, Ji Y, Zhao Q, Wu J, et al. Crosstalk between the gut microbiome and clinical response in locally advanced thoracic esophageal squamous cell carcinoma during neoadjuvant camrelizumab and chemotherapy. Ann Transl Med. (2022) 10:325. doi: 10.21037/atm-22-1165
Keywords: esophageal cancer, esophageal squamous cell carcinoma (ESCC), immunotherapy, neoadjuvant therapy, tumor microenvironment (TME), tumor immune microenvironment, tumor-infiltrating lymphocytes (TILs), biomarkers
Citation: Qiu Z, Li Z, Liu X, Zhang R, Li Y, Gao C, Mao X, Bao Y, Zhang M and Guo C (2024) From tumor microenvironment to emerging biomarkers: the reshaping of the esophageal squamous cell carcinoma tumor microenvironment by neoadjuvant chemotherapy combined with immunotherapy. Front. Immunol. 15:1478922. doi: 10.3389/fimmu.2024.1478922
Received: 11 August 2024; Accepted: 15 November 2024;
Published: 05 December 2024.
Edited by:
Yuanyuan Zhang, Broad Institute, United StatesReviewed by:
Mayela Carolina Mendt, University of Texas MD Anderson Cancer Center, United StatesSakshi M., Emory University, United States
Copyright © 2024 Qiu, Li, Liu, Zhang, Li, Gao, Mao, Bao, Zhang and Guo. This is an open-access article distributed under the terms of the Creative Commons Attribution License (CC BY). The use, distribution or reproduction in other forums is permitted, provided the original author(s) and the copyright owner(s) are credited and that the original publication in this journal is cited, in accordance with accepted academic practice. No use, distribution or reproduction is permitted which does not comply with these terms.
*Correspondence: Changying Guo, Z3VvY2hhbmd5aW5nQG5jbWMuZWR1LmNu
†Present address: Xiaoling Mao, School of Medicine, Jishou University, Jishou, China