- 1Department of Clinical Laboratory Sciences, College of Applied Medical Sciences, Jouf University, Sakaka, Al-Jouf, Saudi Arabia
- 2Department of Oral Medicine and Diagnostic Sciences, College of Dentistry, King Saud University, Riyadh, Saudi Arabia
- 3Department of Pharmacology, College of Pharmacy, Jouf University, Sakaka, Al-Jouf, Saudi Arabia
- 4Department of Pharmaceutics, College of Pharmacy, Jouf University, Sakaka, Al-Jouf, Saudi Arabia
- 5Clinical Pharmacy Department, College of Pharmacy, Jouf University, Sakaka, Al-Jouf, Saudi Arabia
- 6Department of Family and Community Medicine, College of Medicine, Jouf University, Sakaka, Al-Jouf, Saudi Arabia
- 7Department of Pediatric, Domat Aljandal General Hospital, Ministry of Health, Domat Aljandal, Al-Jouf, Saudi Arabia
- 8Sustainable Development Research and Innovation Center, Deanship of Graduate Studies and Scientific Research, Jouf University, Sakaka, Saudi Arabia
Fasciolosis is a zoonotic infection and is considered a developing deserted tropical illness threatening ruminant productivity and causing financial losses. Herein, we applied immunoinformatics and biophysics studies to develop an epitopes vaccine against Fasciola hepatica using glutathione transferase and Cathepsin L-like proteinase as possible vaccine candidates. Using the selected proteins, B- and T-cell epitopes were predicted. After epitopes prediction, the epitopes were clarified over immunoinformatics screening, and only five epitopes, EFGRWQQEKCTIDLD, RRNIWEKNVKHIQEH, FKAKYLTEMSRASDI, TDMTFEEFKAKYLTE, and YTAVEGQCR were selected for vaccine construction; selected epitopes were linked with the help of a GPGPG linker and attached with an adjuvant through another linker, EAAAK linker. Cholera toxin B subunit was used as an adjuvant. The ExPASy ProtParam tool server predicted 234 amino acids, 25.86257 kDa molecular weight, 8.54 theoretical pI, 36.86 instability index, and −0.424 grand average of hydropathicity. Molecular docking analysis predicted that the vaccine could activate the immune system against F. hepatica. We calculated negative binding energy values. A biophysics study, likely molecular docking molecular dynamic simulation, further validated the docking results. In molecular dynamic simulation analysis, the top hit docked compounds with the lowest binding energy values were subjected to MD simulation; the simulation analysis showed that the vaccine and immune cell receptors are stable and can activate the immune system. MMGBSA of −146.27 net energy (kcal/mol) was calculated for the vaccine–TLR2 complex, while vaccine–TLR4 of −148.11 net energy (kcal/mol) was estimated. Furthermore, the C-ImmSim bioinformatics tool predicted that the vaccine construct can activate the immune system against F. hepatica, eradicate the infection caused by F. hepatica, and reduce financial losses that need to be spent while protecting against infections of F. hepatica. The computational immune simulation unveils that the vaccine model can activate the immune system against F. hepatica; hence, the experimental scientist can validate the finding accomplished through computational approaches.
Introduction
Fasciola hepatica is one of the leading causes of fasciolosis in animals and humans (1). Along with Fasciola gigantica, it is a widely disseminated species of liver fluke. A major zoonotic trematode parasite instigates food-borne fasciolosis in livestock and humans. Adult F. hepatica fluke are flatworms, measuring 20–40 mm long and 8–13mm wide (2). Domestic ruminants of tropical and temperate regions are susceptible to the disease caused by F. hepatica. The worm/parasite can infect new hosts, such as wild animals, contributing to its worldwide transmission. Traditionally, fasciolosis is known as livestock disease, but it has become a significant emerging disease in humans (3).
The epidemiological surveys reported that human fasciolosis occurs in the region where the animal fascioliasis is endemic. The number of human cases reported was <3,000 before 1992, whereas F. hepatica infected approximately 17 million people worldwide in 2005. Furthermore, more than 91.1 million people were considered to have the risk of developing liver fluke infection. On the contrary, some countries, such as China, have rare cases of human infection, although veterinary F. hepatica infection is of considerable importance (4).
Fascioliasis due to F. hepatica poses a notable threat to the growth of the farming industry and public health in developing and developed countries. The continuous rise in morbidity and mortality cases led researchers to come up with solutions to combat life-threatening parasitic infections (5).
Climate change and environmental conditions play a critical role in the life cycle and transmittance of F. hepatica. Humidity, oxygen tension, and vegetative conditions also affect the liver fluke lifecycle. These factors provide favorable conditions for the development and reproduction of its larvae. The helminth is endemic in areas with a mean temperature above 10°C for 6 months, with reports of snail infections (6). The incidence of infection in humans is also severely aggregated by the dietary habits of the individuals who intake aquatic plants during animal husbandry (7).
The large leaf-shaped endoparasite has an intermediate host, Lymnaea, a freshwater snail, while the definitive hosts of F. hepatica are goats, cattle, sheep, and humans (8). Parasites reside in the bile duct of the mammalian liver, where their eggs leave the host through feces after entering the duodenum. The optimum conditions promote the growth of ciliated larvae (miracidium) inside the egg within 2–3 weeks. The larvae then escape from the egg and swim to reach the intermediate host, freshwater snail (Lymnaea truncatula) (9). Once it gets penetrated, it forms a sporocyst by losing ciliated covering. The germinal cells inside the sporocyst grow and divide to form the redia. The sporocyst then burst out with the growth of rediae to mature into the final larval form known as cercariae (10). The cercariae have a large tail, which helps it to leave the snail and swim in water to settle on aquatic plants within 2 h. The larva loses its tail afterwards to form metacercariae, which cause infection when animals and humans ingest them. The host’s intestinal fluid digests the metacercariae’s cyst wall to release the juvenile flukes. The flukes of F. hepatica become highly infective when they reach the liver within 4–6 days (11).
Consequently, they cause fascioliasis when they arrive in liver parenchymal cells in 5–6 weeks. The flukes lay eggs into the bile duct after getting sexually matured on week 7 after infection. The eggs leave the definitive host on week 8 after infection through the bile duct and in feces. Humans allow juvenile flukes to mature within 3–4 months (12).
The signs and symptoms of human fascioliasis are divided into two stages: the hepatic phase, which lasts 1–3 months, and the biliary phase. During the hepatic phase, patients experience fever, abdominal pain, cramps, eosinophilia, and abnormal liver function tests. The biliary phase of F. hepatica results in cholestasis with right upper quadrant pain in infected patients. The hepatic phase is diagnosed by computer tomography imaging (CT scan), while ultrasonographic methods detect the biliary phase of F. hepatica. Infected patients’ stool and blood samples are taken to confirm fascioliasis (13). Fascioliasis is also a zoonotic infection that can infect humans and is considered a significant source of morbidity and mortality rate (14).
Anemia, malnutrition, liver abscess, liver cirrhosis, and liver fibrosis are the complications seen in patients with acute and chronic infections. Triclabendazole is a drug that treats acute and chronic human fascioliasis (15). However, liver flukes of F. hepatica have developed resistance against Triclabendazole. Various antigens and biomarkers have been discovered to detect the resistance that has emerged from this drug (16). Re-purposing anti-helminthic drugs, such as nitroxinil, albendazole, and closantel, is in progress to effectively treat flukicide-resistant infections. Moreover, plant extracts and multiomic studies propagated the discovery of new targets and drugs to combat the rapidly spreading infection. All the treatments go a long way to surpass clinical trials, as they are seen to be successful only in laboratory practice. Lastly, preventive measures should be adopted to control the F. hepatica fluke (16).
Fluke control is impossible with the preventive measures and the above treatment options; therefore, effective vaccine development is crucial. Although various vaccination assays have been developed previously, only a few were tested in animals to evaluate their efficacy against fascioliasis. The candidate antigens, including the native and recombinant antigens secreted from F. hepatica, were checked to assess their effectiveness. The vaccines were based on cathepsins secreted from juvenile F. hepatica worms. The results obtained from clinical trials of the vaccines were not satisfactory (17). Hence, multi-epitopes vaccine is needed as a promising treatment for human and livestock fascioliasis. This study aims to utilize multi-informatics methods to design an in silico-based vaccine against F. hepatica.
Research methodology
Sequence retrieval of target protein selection
The protein sequence of the glutathione transferase and Cathepsin L-like proteinase was retrieved from UniProtKB by using ID A0A890CT21 and Q24940 (18). The target protein sequence was subjected to antigenicity analysis; antigenicity was by Vaxijen 2.0 server by selecting a target organism as a parasite with a threshold value of 0.4 (19). Furthermore, the physiochemical properties analysis and allergenicity were done through AllerTOP v. 2.0 and the expasy tools (20). Figure 1 depicts the steps followed to design epitope based vaccine against Fasciola hepatica.
Assessment and prediction of epitopes
The immune epitope database and tool (IEDB) sequence base searching tool for exposing and searching immune epitopes was utilized for the forecasting of epitopes in targeted protein sequences; in B-cell epitopes targeting, a piped linear epitope prediction 2.0 tool was used, and a set of full HLA alleles were selected (21). After B-cell epitope selection, T-cell epitopes were predicted from B-cell epitopes (22). The predicted epitopes were ranked based on the lowest percentile scoring and best overlapping. The predicted epitopes were subjected to allergenicity, toxicity, and water solubility analysis using Vaxijen (23), ToxinPred (24), and peptide calculator bioinformatics tools, respectively (25), and only non-toxic, non-allergen, and suitable water-soluble epitopes were selected.
Designing and processing of vaccine construct
The chosen epitopes were utilized in developing the epitope vaccine construct; the epitopes were linked by “GPGPG” connectors (26). Furthermore, the model vaccine was combined with the cholera toxin B subunit adjuvant to enhance its potency (27). After making the linear sequence of the model vaccine, its physiochemical properties were analyzed using the protparam tool. Afterwards, physiochemical properties analysis and immunoinformatics screening were done using Vaxijen 2.0, peptide calculator, and Allertop 2.0 bioinformatics tools (28). After immunoinformatics screening, the sequence was used in 3D structure modeling using an online 3D scratch predictor bioinformatics tool (29). The model vaccine was subjected to loop refinement using the galaxy web refinement tool https://bio.tools/galaxyrefine. After the refinement of the vaccine structure, the structure was subjected to disulfide engineering by design 2.0, and the engineered structure was generated (30). The world population coverage analysis of the selected epitopes was predicted using the population analysis coverage analysis tool (27, 31, 32) in the immune epitopes database resources tool (33).
Molecular base interaction of vaccine and immune cell receptor, molecular dynamic simulation analysis, and binding free energies estimation
The molecular docking analysis was used to evaluate the binding affinity and interaction pattern of the vaccine construct with receptor TLR2 and TLR4 using their specific PDB ID (TLR2-PDB: ID2Z7X and TLR4-PDB: 4FXI); the crystal structure of the target receptor was retrieved from Protein Data Bank PDB (34). After receptor retrieval, the vaccine construct and receptor were uploaded into the protein–protein molecular docking ClusPro 2.0 web tool (35). Furthermore, the interactive residues of the docking complexes were validated through the PDBsum tool (36). After molecular docking analysis, the docking complexes were subjected to molecular dynamic simulation analysis of 500-ns simulation time (37). In simulation investigation, root mean square fluctuation (RMSF) and root mean square deviation (RMSD) were calculated, using AMBER21 packages (38). Furthermore, using the iMODS server, the motion and stability of the docked conformation were evaluated (39). To further confirm the docking results and the docked complexes’ stability, the complexes’ binding free energy was assessed using MMGBSA and MMPBSA analyses (40).
Results
Protein sequences retrieval and processing for epitope selection
Fasciola hepatica glutathione transferase and Cathepsin L1 protein sequences were retrieved from UniProtKB using A0A890CT21 and Q24940 uniprotkb_accession numbers, respectively. The Vaxijen 2.0 webserver predicted both the proteins as probable antigenic with 0.4576 and 0.5697 antigenic values, respectively; next, the Allerton 2.0 bioinformatics tool predicted both the proteins as non-allergic. The ExPASy ProtParam tool predicted 218, 25.40237, 7.63, and −0.388 number of amino acids, molecular weight, theoretical pI, and GRAVY, respectively, for glutathione transferase, while for Cathepsin L1, the number of amino acids, molecular weight, theoretical pI, and GRAVY of 326, 36.89645, 6.71, and −0.503 were calculated, respectively. In the physiochemical properties analysis, we observed that both the selected proteins are physicochemically stable in the nature and subject for epitopes prediction and prioritization. In the epitopes selection and prioritization phase, the first B-cell epitopes were predicted, as mentioned in Table 1.
The predicted B-cell epitopes were utilized for MHC-I and MHC-II epitopes to make the vaccine construct to activate both humoral and cellular immunity against the target pathogens; the MHC-I and MHC-II predicted epitopes are shown in Table 2.
Immunoinformatics screening
In immunoinformatics screening, the antigenicity, allergenicity, water solubility, and toxicity of the predicted epitopes were analyzed, and only antigenic, non-allergic, non-toxic, and suitable water-soluble epitopes were shortlisted for vaccine designing; the shortlisted epitopes and antigenic values and other immunoinformatics parameters are mentioned in Table 3.
Analysis of worldwide population coverage
The population coverage analysis predicted that the selected epitopes have shown coverage of 99.74% of the worldwide population, and 99.46%, 58.09%, 99.18%, 99.98%, 99.71%, 99.79%, and 99.88% of United States Asian, United States Austronesian, United States Black, United States Caucasoid, United States Hispanic, United States Mestizo, and United States Polynesian, respectively. The other countries and its worldwide population harbor are mentioned in Supplementary Table S1.
Vaccine construct formation and processing
In the vaccine construction phase, the selected epitopes were linked with the help of a GPGPG linker and attached with an adjuvant through another linker, the EAAAK linker; the cholera toxin B subunit was used as an adjuvant. After vaccine construction, the physiochemical properties of the vaccine construct were analyzed through the ExPASy ProtParam tool. The server predicted 234 amino acids, 25,862.57 kDa molecular weight, 8.54 theoretical pI, 36.86 instability index, and −0.424 grand average of hydropathicity (GRAVY); the ExPASy ProtParam tool showed that the model vaccine is stable. Furthermore, the Vaxijen 2.0 bioinformatics tool evaluates the model vaccine as a probable antigen with 0.5000 (probable ANTIGEN) predicted value. Moreover, the Allertop 2.0 tool predicted that the sequence of the model vaccine is non-antigenic by nature. The peptide solubility calculator and ToxinPred 2.0 bioinformatics tools predicted that the sequence of the vaccine construct is good, water soluble, and non-toxic in nature. The physiochemical properties and immunoinformatics findings are mentioned in Table 4.
Figure 2A shows the 3D structure of the engineered vaccine construct; the salmon color represents the adjuvant. Furthermore, the Ramachandran plot and secondary structure are mentioned in Figures 2B, C, respectively.
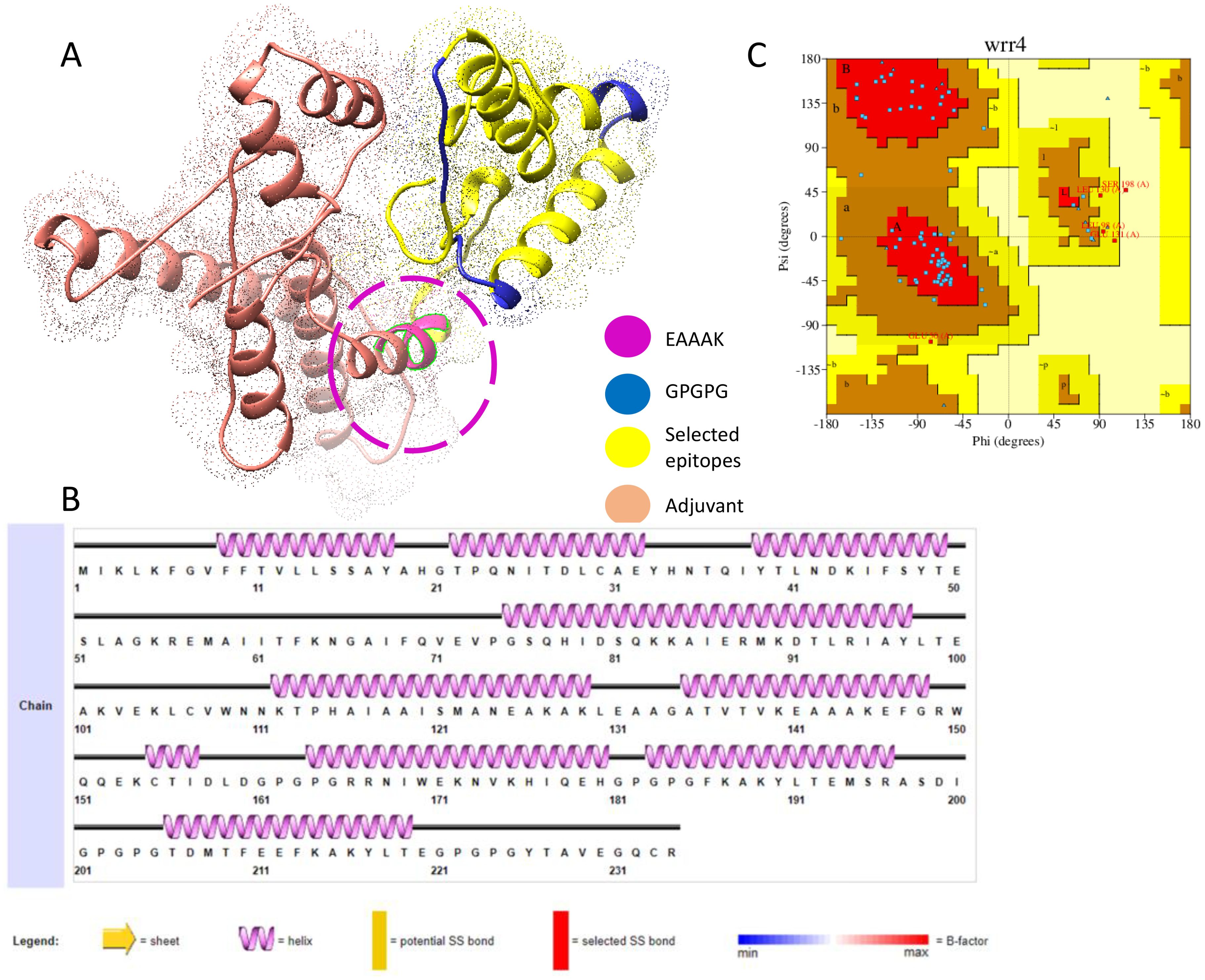
Figure 2. (A) 3D representation of multi epitopes vaccine construct. (B) Secondary structure. (C) Ramachandran plot.
Structure refinement
The structure of the vaccine construct was refined to remove the unwanted loops in the protein structure, and overall, the steric clashes were removed. The refined structure is presented in Figure 3A. The galaxy refinement tool generated the top 10 refine structure based on several parameters, and the calculated values are mentioned in Supplementary Table S2. The model refine structure was considered deemed fit for further processing. For further improvement, disulfide engineering was performed on the structure of the vaccine construct, in which disulfide bonds were incorporated in the refine structure of the vaccine construct. The vaccine construct was further subjected to disulfide engineering, and a total of 25 pairs of amino acid residues Leu4-Thr11, Gly7-Val229, Val8-Thr11, Phe9-His34, Val12-Thr27, Ser15-Pro23, Ile384-Leu41, Lys44-Lys55, Met58-Asn65, Ala59-Thr62, Pro74-Gln77, Ile95-Ser121, Leu98-Lys102, Val103-Ile120, Ala123-Glu141, Lys154-Ile157, Asp160-Arg166, Ile177-Phe186, Tyr190-Pro204, Leu191-Gly203, Asp199-Pro202, Pro202-Glu212, Gly205-Met108, Tyr217-Gly223, and Tyr226-Val229 were chosen to be replaced with cysteine amino acid and the refined structure generated; the original and mutated structures are presented in Figures 3B, C.
Molecular docking analysis and docked confirmation visualization
In molecular docking analysis, we observed the vaccine’s interaction with TLR2 and TLR4. The docking served different binding energy scores between the vaccine and receptor, and the energy was predicted based on the blinding docking approach. The docking scores of the vaccine and TLR2 and TLR4 are presented in Supplementary Tables S3, S4, respectively. The intermolecular docking visualizations are shown in Figures 4, 5.
Intermolecular visualization of docked molecules
The intermolecular interaction of top 1 docked complexes, based on different amino acid residue levels, is visualized by pdbsum generated in the amino acid residues. Other types of bonding interactions have been observed in vaccine and TLR2 and TLR4 docked complexes, as presented in Figures 6, 7.
iMODS analysis
In iMODS simulation, the vibration motion of the docked complexes is increasingly used to study the dynamics of the docked complexes; we predicted eigenvalues of 9.663726 and 1.924031 in the case of vaccine and TLR2 and TLR4, respectively, which correlate that docked complexes have been showing stability in the dynamic environment. Furthermore, the residues and atomic indices also showed that the vaccine and receptors presented stable docked molecules, as the normal modes analysis plots are mentioned in Figures 8, 9.
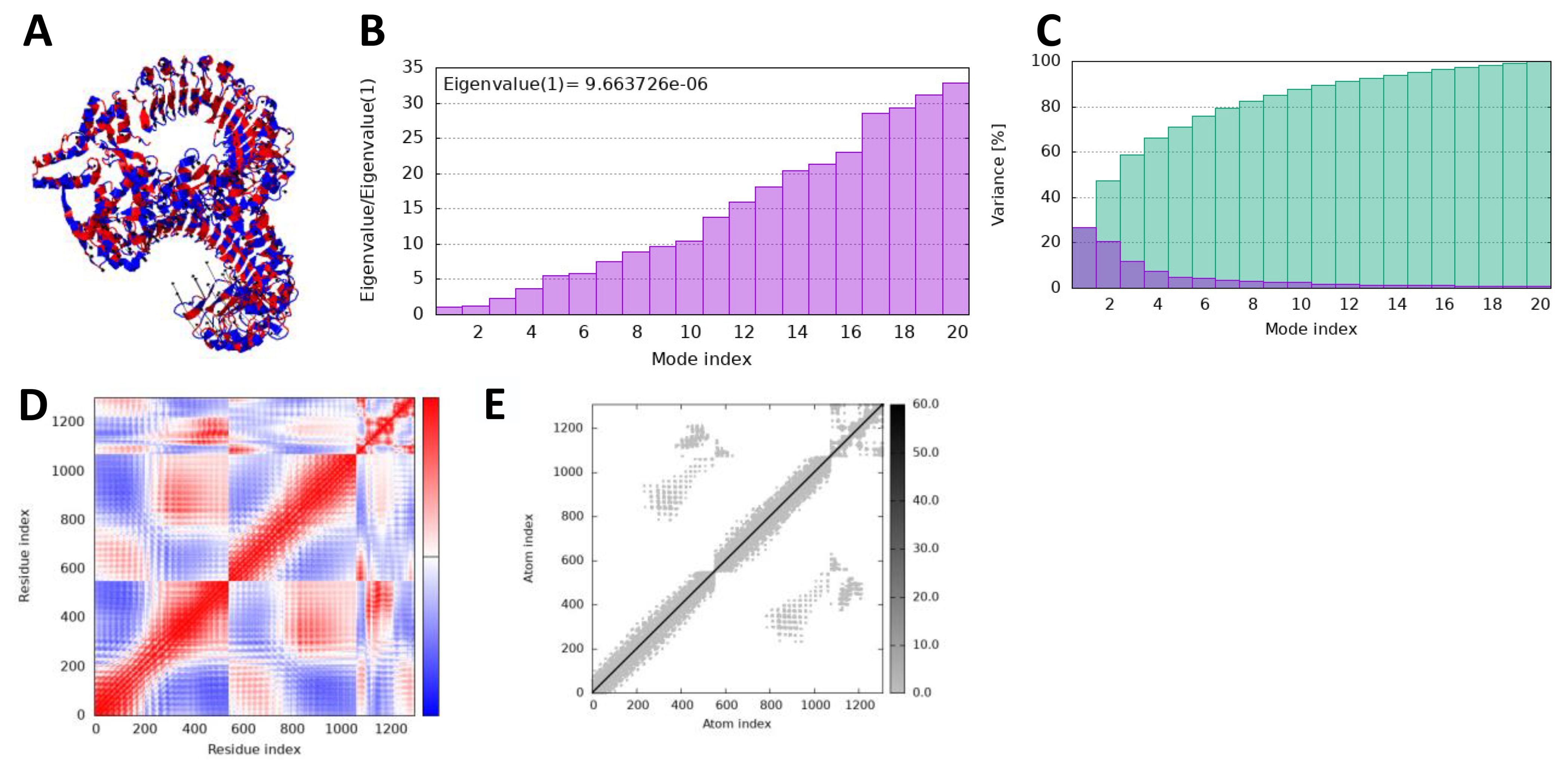
Figure 8. iMODS simulation of vaccine_TLR4. (A) Docked structure. (B) Eigenvalue. (C) Variance. (D) Covaiance. (E) Elastic network model.
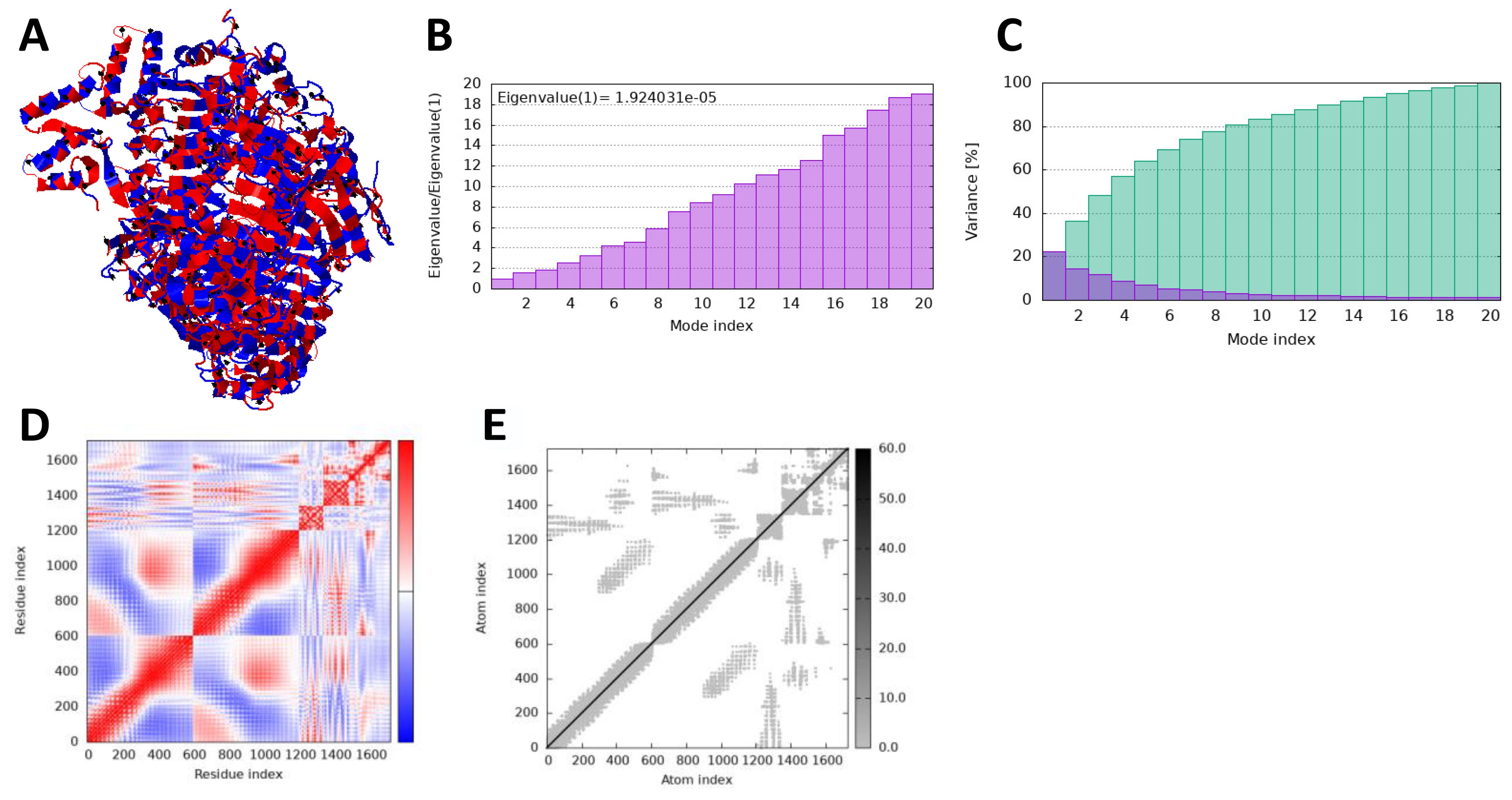
Figure 9. iMODS simulation of vaccine_TLR4. (A) Docked structure. (B) Eigenvalue. (C) Variance. (D) Covaiance. (E) Elastic network model.
MD simulation analysis
In the molecular dynamic simulation, the plot represents that the vaccine and the immune cell receptor stability remains in the dynamic environment; root mean square deviation analysis was done to observe time-dependent deviation in docked complexes, while root mean square deviation was done to achieve residue level fluctuation in the docked molecules. In the RMSD, we noticed that the vaccine–TLR4 is more stable followed by vaccine–TLR2 in 200 simulation times. Moreover, the RMSF plot also represents that the immunization and TLR4 are more stable, followed by vaccine and TLR4, as shown in Figures 10A, B.
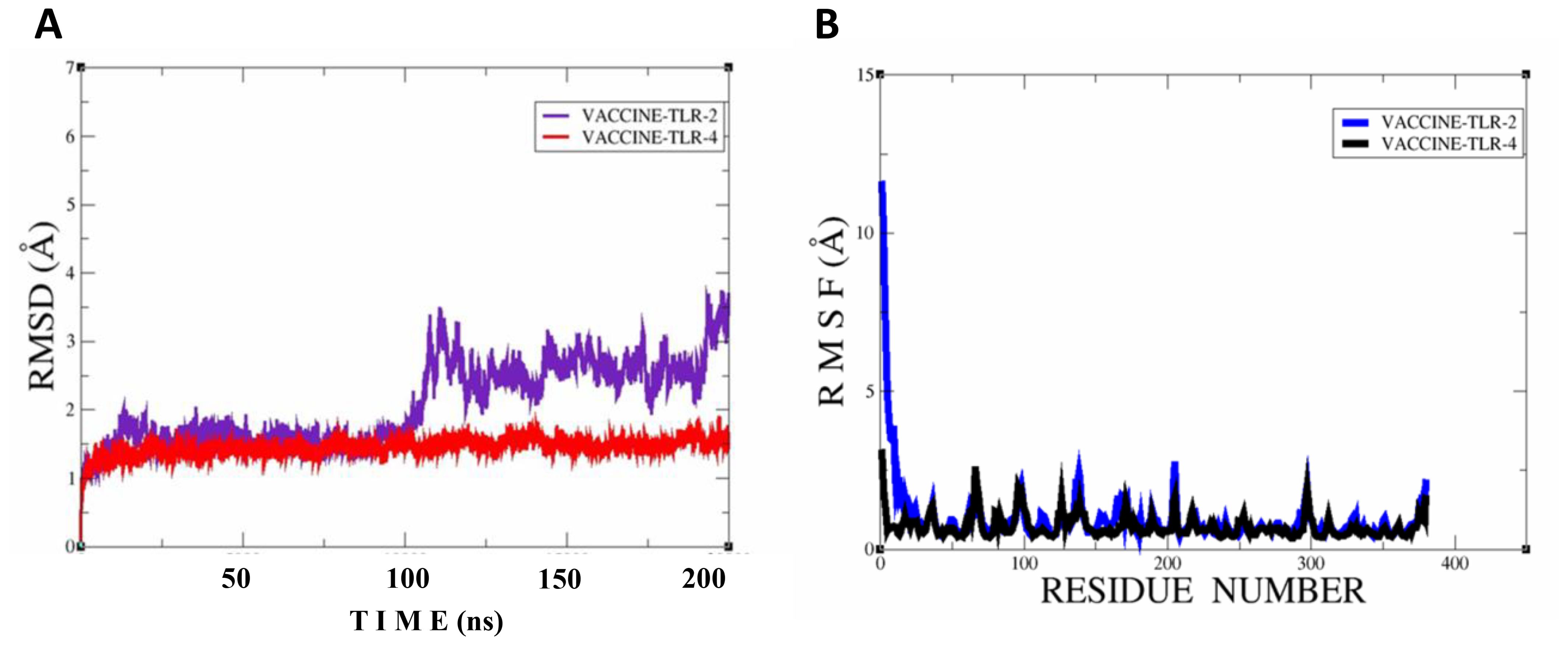
Figure 10. Simulation plots of vaccine–TLR2 and vaccine–TLR4. (A) RMSD plot for both docked complexes; vaccine-TLR2 and vaccine-TLR4. (B) RMSF plot for both docked complexes; vaccine-TLR2 and vaccine-TLR4.
Estimation of binding energy
The calculation of binding free energy predicted that the vaccine and receptor binding affinity is stable, and the MMGB/PBSA analysis predicted the negative binding energy, unveiling that the net binding energy is a negative value, hence representing that the complexes are stable. Negative biding energies of −148.11 and −146.27 were predicted via MMGBSA for vaccine–TLR4 and vaccine–TLR2 receptors, the MM-PBSA predicted −145.98 and −164.78, which was calculated by MMPBSA vaccine–TLR4 and vaccine–TLR2, respectively, as mentioned in Table 5.
Computational immune simulation
In computational immune simulation, we observed that the model vaccine against F. hepatica can activate the immune response in the host and efficiently tackle the pathogenesis. We analyzed different types of B and T cells’ immune response toward the F. hepatica vaccine, as presented in Figures 11A-D.
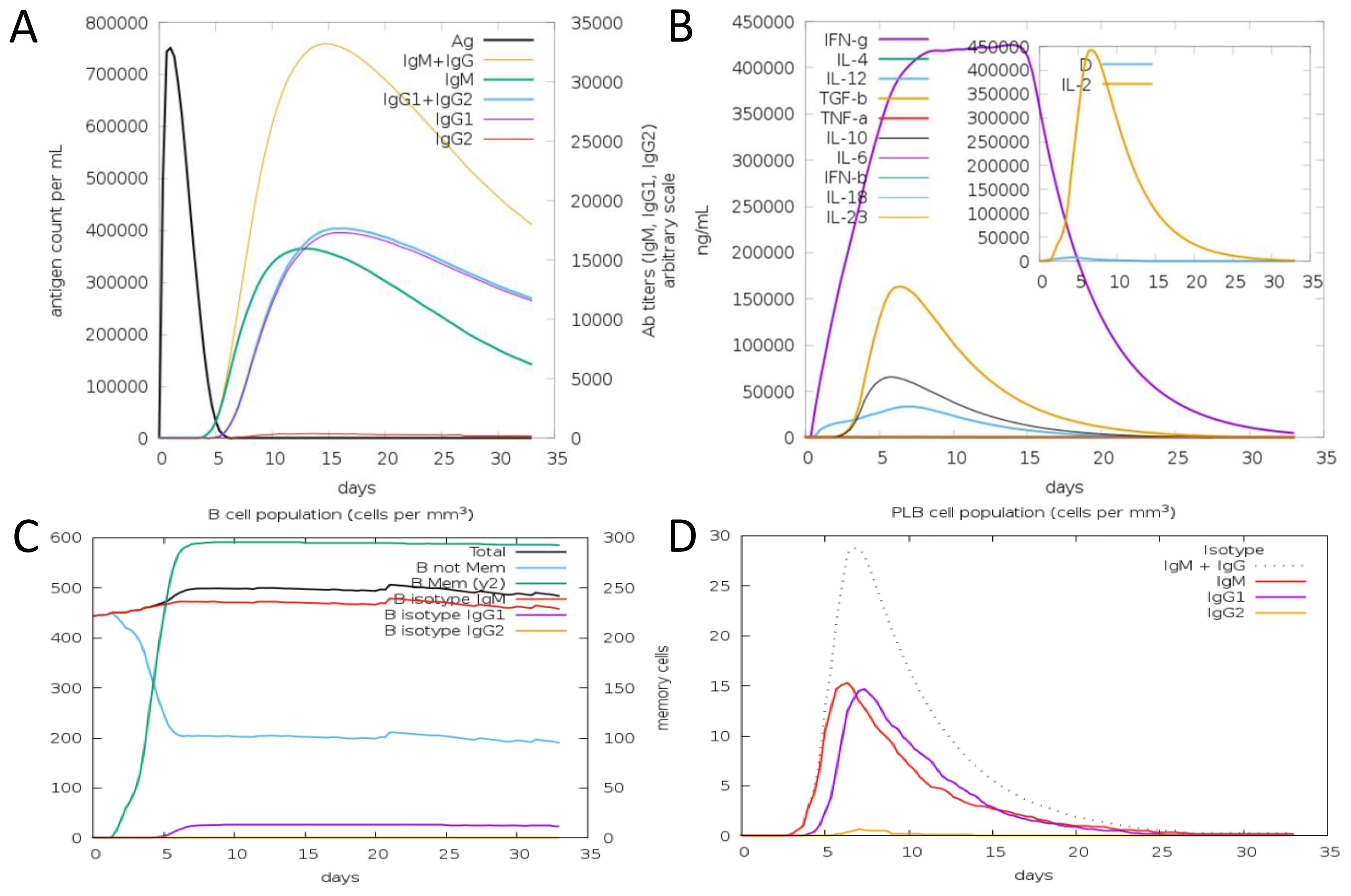
Figure 11. Antibodies and cytokines plots toward a model vaccine. (A) Antibody production level with number of days after vaccine. (B) Concentration of Cytokines and Interleukins against vaccine. (C) B cell population. (D) PLB cell population.
Discussion
Vaccination is the primary method for preventing infections caused by pathogens (41). However, traditional vaccine development is expensive and labor intensive and carries a significant risk of failure (42). By contrast, immunoinformatics-driven vaccine design can streamline this process by pinpointing potential epitopes from proteins for use in creating vaccine candidates (43). Multi-epitope vaccines are crafted to enhance the collective impact of cellular, humoral, and innate immune responses, giving them an advantage over monovalent candidates or formulations (44). Recently, many efforts have been made to utilize immunoinformatics approaches for developing epitope vaccines against parasites (45).
In this research, a multi-epitope-based vaccine has been projected from two selected proteins, glutathione transferase and Cathepsin L-like proteinase, that play a significant role in the virulence and pathogenesis of parasites; the proteins were selected based on homology search, as they do not show homology with human proteins, which proposed a tremendously lesser possibility of causing autoimmune reactions in the host body (46). Unusually, the selected protein sequences were physicochemically stable, non-allergic, had good water solubility, non-toxic, and had 0 transmembrane helices (TM helices), indicating that the selected proteins are a promising vaccine candidate. Both types of host immunity, namely, the T-lymphocyte effector response and long-lasting B-cell memory, are crucial for protection against the parasite (47). Seven B-cell epitopes were predicted from both selected proteins to generate a humoral immune response against F. hepatica; from B cells, further T-cell epitopes were predicted to accomplish T-cell response as well (48).
Macrophage activation is essential for the elimination of microorganisms, and interferon-gamma is the main macrophage-activating factor. In the immunoinformatics analysis, we observed that the selected epitopes were found to be an IFN-γ activator (49). In addition, the B- and T-cell epitopes were also added in the vaccine construct for the activation of cellular- and humoral-mediated immune system F. hepatica. At the same time, none of the selected epitopes in the designed vaccine showed any similarity to the human proteome (50). This indicates its ability to trigger immune solid responses while avoiding potential harmful allergic reactions.
Keeping the synthetic protein small is essential. Minimizing the size of the artificial protein is vital to lower production costs, simplify the purification process from inclusion bodies, and avoid host organism toxicity. Therefore, only 10 epitopes were selected for the vaccine design, excluding overlapping ones, to optimize the total number of epitopes, without increasing the overall length (51).
Moreover, the docking analysis predicted that the immune system could recognize the model vaccine; hence, it can provoke the immune system and easily create a cellular and antibody-dependent immune system (52). In the dynamic environment, the stability of the docked complex is essential to activate and create long-lasting immunity against F. hepatica. The molecular dynamic simulation finding unveils the binding stability of the immune system and vaccine stability. Moreover, the in silico results estimated that the vaccine model could boost the immune system in the form of the cellular and humoral immune response (28, 29, 53, 54). The MMGBSA and MMPBSA analysis further validated that the vaccine and immune system have stable interaction. Overall, the findings suggest that the vaccine model can activate the immune system and can reduce the pathogenicity of the F. hepatica.
Conclusion
Fasciolosis is not just a zoonotic infection and health issue but also affects social and economic values. Therefore, constructing a powerful and effective vaccine could be crucial in effectively combating this disease. This study mainly focused on epitope-based vaccine constructs against F. hepatica by precisely predicting possible vaccine targets from glutathione transferase and Cathepsin L-like proteinase. The developed vaccine construct exhibits acceptable properties regarding antigenicity, allergenicity, physicochemical characteristics, and structural integrity. Moreover, the interaction of the designed vaccine construct with the immune system indicates that the vaccine construct can activate the immune system and induce proper B- and T-cell response toward the F. hepatica; however, the findings further need to validate its potency against F. hepatica. It can be concluded that despite some structural changes observed after MD simulation, the vaccine construct remains stable in vivo within the biological system.
Data availability statement
The original contributions presented in the study are included in the article/Supplementary Material. Further inquiries can be directed to the corresponding author.
Author contributions
HA: Conceptualization, Data curation, Formal analysis, Funding acquisition, Investigation, Methodology, Project administration, Resources, Software, Supervision, Validation, Visualization, Writing – original draft, Writing – review & editing. MU: Data curation, Writing – review & editing. AN: Data curation, Writing – review & editing. SA: Methodology, Writing – review & editing. OA: Investigation, Writing – review & editing. AIA: Project administration, Writing – review & editing. AsA: Investigation, Writing – review & editing. AbA: Validation, Writing – review & editing. MA: Visualization, Writing – review & editing. YA: Software, Writing – review & editing.
Funding
The author(s) declare financial support was received for the research, authorship, and/or publication of this article. This research is funded by the Deanship of Graduate Studies and Scientific Research at Jouf University (grant number DSR2022-NF-10).
Conflict of interest
The authors declare that the research was conducted in the absence of any commercial or financial relationships that could be construed as a potential conflict of interest.
Publisher’s note
All claims expressed in this article are solely those of the authors and do not necessarily represent those of their affiliated organizations, or those of the publisher, the editors and the reviewers. Any product that may be evaluated in this article, or claim that may be made by its manufacturer, is not guaranteed or endorsed by the publisher.
Supplementary material
The Supplementary Material for this article can be found online at: https://www.frontiersin.org/articles/10.3389/fimmu.2024.1478107/full#supplementary-material
References
2. Nawar AM, Dawood WM, Kadhem NY. The effect of alcoholic extract of Citrullus colocynthis on the structural manifestations of the parasite Fasciola gigantica and its comparison with the drug pendazole. Nabatia. (2024) 12:20–5. doi: 10.21070/nabatia.v12i1.1637
3. Gupta N, Verma I, Singh H. Current Therapeutic Strategies for Food Borne Trematodiases. In: Emerg Approaches to Tack Neglected Dis From Mol to End Prod (2024). p. 200.
4. Che-Kamaruddin N, Hamid NFS, Idris LH, Yusuff FM, Ashaari ZH, Yahaya H, et al. Prevalence and risk factors of fasciolosis in a bovine population from farms in Taiping, Malaysia. Vet Parasitol Reg Stud Rep. (2024) 49:100998. doi: 10.1016/j.vprsr.2024.100998
5. Thakur S. An overview of fasciolosis in Nepal: epidemiology, diagnosis, and control strategies. J Parasit Dis. (2024), 1–13. doi: 10.1007/s12639-024-01700-3
6. Quinteros ACR, Soler P, Larroza M, Morales JM, Gurevitz JM. Water temperature and microenvironmental factors predict the presence and detection of the snail host of Fasciola hepatica in Andean Patagonia. Vet Parasitol. (2024) 329:110209. doi: 10.1016/j.vetpar.2024.110209
7. Hu Y, Zhan R-J, Lu S-L, Zhang Y-Y, Zhou M-Y, Huang H, et al. Global distribution of zoonotic digenetic trematodes: a scoping review. Infect Dis Poverty. (2024) 13:46. doi: 10.1186/s40249-024-01208-1
8. Lee JS. Fascioliasis: image findings, diagnosis, and treatment. Clin Ultrasound. (2024) 9:18–24. doi: 10.18525/cu.2024.9.1.18
9. Koyee QM, Khailany RA, Rahman ML, Nassraldin LN. Histopathologic changes and molecular characterization of fascioliasis (a zoonotic disease) among slaughtered livestock in Erbil and Halabja Abattoirs, Kurdistan region-Iraq. Baghdad Sci J. (2024) 21:2191. doi: 10.21123/bsj.2023.9099
10. Aftab A, Raina OK, Maxton A, Masih SA. Advances in diagnostic approaches to Fasciola infection in animals and humans: An overviews. J Helminthol. (2024) 98:e12. doi: 10.1017/S0022149X23000950
11. Mahdy OA, Salem MA, Abdelsalam M, Shaheed IB, Attia MM. Immunological and molecular evaluation of zoonotic metacercarial infection in freshwater fish: A cross-sectional analysis. Res Vet Sci. (2024) 172:105239. doi: 10.1016/j.rvsc.2024.105239
12. Chai J-Y, Jung B-K. Pathogenesis of trematode infections (blood, liver and lung flukes). Mol Med Microbiol. (2024) 7:2965–3001. doi: 10.1016/B978-0-12-818619-0.00074-5
13. Tanabe MB, Caravedo MA, Clinton White A Jr, Cabada MM. An update on the pathogenesis of fascioliasis: what do we know? Res Rep Trop Med. (2024) 15:13–24. doi: 10.2147/RRTM.S397138
14. Rosas-Hostos Infantes LR, Paredes Yataco GA, Ortiz-Martínez Y, Mayer T, Terashima A, Franco-Paredes C, et al. The global prevalence of human fascioliasis: a systematic review and meta-analysis. Ther Adv Infect Dis. (2023) 10:20499361231185412. doi: 10.1177/20499361231185413
15. Sewoyo PS, Purwanti NLL, Evan S. Case report: anatomic pathology of Bali cattle liver infested with fasciola gigantica. Veterinary Medicine. (2024) 35:0215–8930. doi: 10.20473/mkh.v35i1.2024.28-35
16. Nguyen M-N, Yeo S-J, Park H. Identification of novel biomarkers for anti-Toxoplasma gondii IgM detection and the potential application in rapid diagnostic fluorescent tests. Front Microbiol. (2024) 15:1385582. doi: 10.3389/fmicb.2024.1385582
17. Shah M, Sitara F, Sarfraz A, Shehroz M, Wara TU, Perveen A, et al. Development of a subunit vaccine against the cholangiocarcinoma causing Opisthorchis viverrini: a computational approach. Front Immunol. (2024) 15:1281544. doi: 10.3389/fimmu.2024.1281544
18. Li C, Sutherland D, Salehi A, Richter A, Lin D, Aninta SI, et al. Mining the UniProtKB/Swiss-Prot database for antimicrobial peptides. bioRxiv. (2024), 2005–24. doi: 10.1101/2024.05.24.595811
19. Praveen M. Multi-epitope-based vaccine designing against Junín virus glycoprotein: immunoinformatics approach. Futur J Pharm Sci. (2024) 10:29. doi: 10.1186/s43094-024-00602-8
20. Dimitrov I, Flower DR, Doytchinova I. AllerTOP-a server for in silico prediction of allergens. BMC Bioinf. (2013) 14, 1–9. doi: 10.1186/1471-2105-14-S6-S4
21. Zhang Q, Wang P, Kim Y, Haste-Andersen P, Beaver J, Bourne PE, et al. Immune epitope database analysis resource (IEDB-AR). Nucleic Acids Res. (2008) 36:W513–8. doi: 10.1093/nar/gkn254
22. Vita R, Mahajan S, Overton JA, Dhanda SK, Martini S, Cantrell JR, et al. The immune epitope database (IEDB): 2018 update. Nucleic Acids Res. (2019) 47:D339–43. doi: 10.1093/nar/gky1006
23. Zaharieva N, Dimitrov I, Flower DR, Doytchinova I. Immunogenicity prediction by VaxiJen: a ten year overview. J Proteomics Bioinforma. (2017) 10:298–310. doi: 10.4172/jpb.1000454
24. Sharma N, Naorem LD, Jain S, Raghava GPS. ToxinPred2: an improved method for predicting toxicity of proteins. Brief Bioinform. (2022) 23:1–12. doi: 10.1093/bib/bbac174
25. Bibi S, Ullah I, Zhu B, Adnan M, Liaqat R, Kong WB, et al. In silico analysis of epitope-based vaccine candidate against tuberculosis using reverse vaccinology. Sci Rep. (2021) 11:1–16. doi: 10.1038/s41598-020-80899-6
26. Mushtaq M, Khan S, Hassan M, Al-Harbi AI, Hameed AR, Khan K, et al. Computational Design of a Chimeric Vaccine against Plesiomonas shigelloides Using Pan-Genome and Reverse Vaccinology. Vaccines. (2022) 10:1886. doi: 10.3390/vaccines10111886
27. Ud-Din M, Albutti A, Ullah A, Ismail S, Ahmad S, Naz A, et al. Vaccinomics to design a multi-epitopes vaccine for acinetobacter baumannii. Int J Environ Res Public Health. (2022) 19:5568. doi: 10.3390/ijerph19095568
28. Gul S, Ahmad S, Ullah A, Ismail S, Khurram M, Tahir ul Qamar M, et al. Designing a Recombinant Vaccine against Providencia rettgeri Using Immunoinformatics Approach. Vaccines. (2022) 10:189. doi: 10.3390/vaccines10020189
29. Ullah A, Shahid FA, Haq MU, Tahir ul Qamar M, Irfan M, Shaker B, et al. An integrative reverse vaccinology, immunoinformatic, docking and simulation approaches towards designing of multi-epitopes based vaccine against monkeypox virus. J Biomol Struct Dyn. (2023) 41:7821–34. doi: 10.1080/07391102.2022.2125441
30. Irfan M, Khan S, Hameed AR, Al-Harbi AI, Abideen SA, Ismail S, et al. Computational Based Designing of a Multi-Epitopes Vaccine against Burkholderia mallei. Vaccines. (2022) 10:1580. doi: 10.3390/vaccines10101580
31. Rida T, Ahmad S, Ullah A, Ismail S, Tahir ul Qamar M, Afsheen Z, et al. Pan-genome analysis of oral bacterial pathogens to predict a potential novel multi-epitopes vaccine candidate. Int J Environ Res Public Health. (2022) 19:8408. doi: 10.3390/ijerph19148408
32. Ullah A, Shahid FA, Haq MU, Tahir ul Qamar M, Irfan M, Shaker B, et al. An integrative reverse vaccinology, immunoinformatic, docking and simulation approaches towards designing of multi-epitopes based vaccine against monkeypox virus. J Biomol Struct Dyn. (2022) 41:1–14. doi: 10.1080/07391102.2022.2125441
33. Malik M, Khan S, Ullah A, Hassan M, Ahmad S, Al-Harbi AI, et al. Proteome-wide Screening of Potential Vaccine Targets against Brucella melitensis. Vaccines. (2023) 11:263. doi: 10.3390/vaccines11020263
34. Abbas G, Zafar I, Ahmad S, Azam SS. Immunoinformatics design of a novel multi-epitope peptide vaccine to combat multi-drug resistant infections caused by Vibrio vulnificus. Eur J Pharm Sci. (2020) 142:105160. doi: 10.1016/j.ejps.2019.105160
35. Kozakov D, Hall DR, Xia B, Porter KA, Padhorny D, Yueh C, et al. The ClusPro web server for protein–protein docking. Nat Protoc. (2017) 12:255–78. doi: 10.1038/nprot.2016.169
36. Laskowski RA. PDBsum: summaries and analyses of PDB structures. Nucleic Acids Res. (2001) 29:221–2. doi: 10.1093/nar/29.1.221
37. Alshammari A, Alasmari AF, Alharbi M, Ali N, Muhseen ZT, Ashfaq UA, et al. Novel Chimeric Vaccine Candidate Development against Leptotrichia buccalis. Int J Environ Res Public Health. (2022) 19:10742. doi: 10.3390/ijerph191710742
38. Case DA, Aktulga HM, Belfon K, Ben-Shalom I, Brozell SR, Cerutti DS, et al. Amber 2021. San Francisco: University of California (2021).
39. López-Blanco JR, Aliaga JI, Quintana-Ortí ES, Chacón P. iMODS: internal coordinates normal mode analysis server. Nucleic Acids Res. (2014) 42:W271–6. doi: 10.1093/nar/gku339
40. Deng Y, Roux B. Computations of standard binding free energies with molecular dynamics simulations. J Phys Chem B. (2009) 113:2234–46. doi: 10.1021/jp807701h
42. Sanae A, Aicha SS. (2024). Data modeling review: the case of vaccine lifecycle management, in: 2024 IEEE 15th International Colloquium on Logistics and Supply Chain Management (LOGISTIQUA). 1–7. Sousse, Tunisia: IEEE, pp. 1–7.
43. Arshad SF, Rehana R, Saleem MA, Usman M, Arshad HJ, Rizwana R, et al. Multi-epitopes vaccine design for surface glycoprotein against SARS-CoV-2 using immunoinformatic approach. Heliyon. (2024) 10:e24186. doi: 10.1016/j.heliyon.2024.e24186
44. Sarvmeili J, Baghban Kohnehrouz B, Gholizadeh A, Shanehbandi D, Ofoghi H. Immunoinformatics design of a structural proteins driven multi-epitope candidate vaccine against different SARS-CoV-2 variants based on fynomer. Sci Rep. (2024) 14:10297. doi: 10.1038/s41598-024-61025-2
45. Larijani A, Kia-Karimi A, Rahmati M, Derakhshan R. Computational prediction of leishmania infantum epitopes: A bioinformatic-based step to leishmaniasis vaccine design. J Vis Med. (2024) 1:e0109. doi: 10.30699/jovm.006.e0109
46. Asad Y, Ahmad S, Rungrotmongkol T, Ranaghan KE, Azam SS. Immuno-informatics driven proteome-wide investigation revealed novel peptide-based vaccine targets against emerging multiple drug resistant Providencia stuartii. J Mol Graph Model. (2018) 80:238–50. doi: 10.1016/j.jmgm.2018.01.010
47. Khan S, Khan A, Rehman AU, Ahmad I, Ullah S, Khan AA, et al. Immunoinformatics and structural vaccinology driven prediction of multi-epitope vaccine against Mayaro virus and validation through in-silico expression. Infect Genet Evol. (2019) 73:390–400. doi: 10.1016/j.meegid.2019.06.006
48. Ullah A, Ullah Khan S, Haq MU, Ahmad S, Irfan M, Asif M, et al. Computational study to investigate Proteus mirabilis proteomes for multi-epitope vaccine construct design. J Biomol Struct Dyn. (2022) 41:1–12. doi: 10.1080/07391102.2022.2153920
49. Tan YL, Al-Masawa ME, Eng SP, Shafiee MN, Law JX, Ng MH. Therapeutic efficacy of interferon-gamma and hypoxia-primed mesenchymal stromal cells and their extracellular vesicles: underlying mechanisms and potentials in clinical translation. Biomedicines. (2024) 12:1369. doi: 10.3390/biomedicines12061369
50. Jha DK, Yashvardhini N, Kumar A, Gaurav M, Sayrav K. Designing of multi-Epitope vaccine against spike glycoprotein of SARS-CoV-2 using immunoinformatics approach. Med Omi. (2024) 11:100036. doi: 10.1016/j.meomic.2024.100036
51. Guo Z, Ren H, Chang Q, Liu R, Zhou X, Xue K, et al. Lactobacilli-derived adjuvants combined with immunoinformatics-driven multi-epitope antigens based approach protects against Clostridium perfringens in a mouse model. Int J Biol Macromol. (2024) 267:131475. doi: 10.1016/j.ijbiomac.2024.131475
52. Banerjee S, Majumder K, Gutierrez GJ, Gupta D, Mittal B. Immuno-informatics approach for multi-epitope vaccine designing against SARS-CoV-2. BioRxiv. (2020). doi: 10.1101/2020.07.23.218529
53. Yousaf M, Ismail S, Ullah A, Bibi S. Immuno-informatics profiling of monkeypox virus cell surface binding protein for designing a next generation multi-valent peptide-based vaccine. Front Immunol. (2022) 13. doi: 10.3389/fimmu.2022.1035924
Keywords: Fasciola hepatica, immunoinformatics, epitopes, molecular docking, molecular dynamic simulation analysis
Citation: Alhassan HH, Ullah MI, Niazy AA, Alzarea SI, Alsaidan OA, Alzarea AI, Alsaidan AA, Alhassan AA, Alruwaili M and Alruwaili YS (2024) Exploring glutathione transferase and Cathepsin L-like proteinase for designing of epitopes-based vaccine against Fasciola hepatica by immunoinformatics and biophysics studies. Front. Immunol. 15:1478107. doi: 10.3389/fimmu.2024.1478107
Received: 09 August 2024; Accepted: 28 August 2024;
Published: 26 September 2024.
Edited by:
Sumra Wajid Abbasi, National University of Medical Sciences (NUMS), PakistanCopyright © 2024 Alhassan, Ullah, Niazy, Alzarea, Alsaidan, Alzarea, Alsaidan, Alhassan, Alruwaili and Alruwaili. This is an open-access article distributed under the terms of the Creative Commons Attribution License (CC BY). The use, distribution or reproduction in other forums is permitted, provided the original author(s) and the copyright owner(s) are credited and that the original publication in this journal is cited, in accordance with accepted academic practice. No use, distribution or reproduction is permitted which does not comply with these terms.
*Correspondence: Hassan H. Alhassan, aC5hbGhhc2FuQGp1LmVkdS5zYQ==