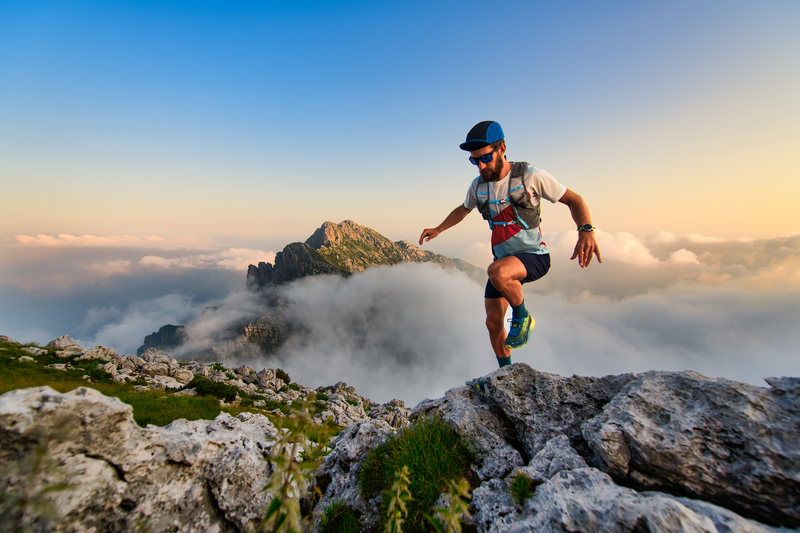
95% of researchers rate our articles as excellent or good
Learn more about the work of our research integrity team to safeguard the quality of each article we publish.
Find out more
ORIGINAL RESEARCH article
Front. Immunol. , 07 November 2024
Sec. Cancer Immunity and Immunotherapy
Volume 15 - 2024 | https://doi.org/10.3389/fimmu.2024.1472433
This article is part of the Research Topic Community Series in Cell Network in Antitumor Immunity of Pediatric and Adult Solid Tumors: Volume II View all 9 articles
Introduction: Pancreatic ductal adenocarcinoma (PDAC) represents the complexity of interaction between cancer and cells of the tumor microenvironment (TME). Immune cells affect tumor cell behavior, thus driving cancer progression. Cancer-associated fibroblasts (CAFs) are responsible of the desmoplastic and fibrotic reaction by regulating deposition and remodeling of extracellular matrix (ECM). As tumor-promoting cells abundant in PDAC ECM, CAFs represent promising targets for novel anticancer interventions. However, relevant clinical trials are hampered by the lack of specific markers and elusive differences among CAF subtypes. Indeed, while single-cell transcriptomic analyses have provided important information on the cellular constituents of PDACs and related molecular pathways, studies based on the identification of protein markers in tissues aimed at identifying CAF subtypes and new molecular targets result incomplete.
Methods: Herein, we applied multiplexed Imaging Mass Cytometry (IMC) at single-cell resolution on 8 human PDAC tissues to depict the PDAC composing cells, and profiling immune cells, endothelial cells (ECs), as well as endocrine cells and tumor cells.
Results: We focused on CAFs by characterizing up to 19 clusters distinguished by phenotype, spatiality, and interaction with immune and tumor cells. We report evidence that specific subtypes of CAFs (CAFs 10 and 11) predominantly are enriched at the tumor-stroma interface and closely associated with tumor cells. CAFs expressing different combinations of FAP, podoplanin and cadherin-11, were associated with a higher level of CA19-9. Moreover, we identified specific subsets of FAP+ and podoplanin+/cadherin-11+ CAFs enriched in patients with negative prognosis.
Discussion: The present study provides new general insights into the complexity of the PDAC microenvironment by defining phenotypic heterogeneities and spatial distributions of CAFs, thus suggesting different functions of their subtypes in the PDAC microenvironment.
Pancreatic ductal adenocarcinoma (PDAC) is one of the most lethal types of cancer, with a 5-years survival rate of 11% only (1). This poor prognosis is mainly due to the inability to detect the disease until late, often metastatic, tumor stage (2). Further, diagnosis is complicated by the asymptomatic evolution of the disease, the lack of diagnostic biomarkers, the absence of attributable risk factors for the majority of patients and the difficult-to-access anatomical location of the pancreas, which limits the routine screening intervention (3, 4). Although 10-15% of cases can be ascribed to germline mutations or known risk factors, the majority of PDAC develops as a consequence of accumulating mutations in several genes, including KRAS, p53, SMAD4 and CDKN2A, which results in the formation of pre-cancerous lesions, such as Pancreatic Intraepithelial Neoplasia (PanIN) and Intrapapillary Mucinous Neoplasia (IPMN), that possibly evolve to invasive cancer (5–8). Beside mutations that drive the neoplastic morphological alterations of pancreatic epithelial cells, PDAC is characterized by a massive infiltration of activated cancer-associated fibroblasts (CAFs), responsible for the deposition of extracellular matrix components and leading to a desmoplastic reaction, that shapes a tumor microenvironment (TME) composed by a dense stroma, a leaky vascular system and suppressive immune cell populations (9–11). The resulting TME, which can develop up to the 90% of the entire tumor mass, is indeed the main responsible of the heterogeneity, aggressive biology and resistance to therapy of the disease (9, 12). Although the limit in the 5-years survival rate, PDAC survival statistics have doubled over the past decades, due to the improved therapeutic approaches and clinical care (13).
Cell heterogeneity in PDAC has been widely investigated using single-cells transcriptomic approaches, but only few studies analyzed the protein expressed by the different cell subpopulations that compose the PDAC microenvironment (14–16). In the last decade, multiplexed Imaging Mass Cytometry (IMC) has emerged as a powerful technology to dissect the cell landscape of several TME (17–19). IMC combines conventional histology with mass cytometry to identify up to 35-40 metal-tagged antibodies, avoiding limitations related to fluorescence-based imaging technologies, including autofluorescence and spectral overlap (17, 20). Although several studies used conventional immunohistochemistry or fluorescence-based imaging to target markers of PDAC microenvironment (15, 16, 21, 22), fewer are the investigations conducted using the multiplexed IMC technology (23, 24). In addition, these studies mainly focused on the immune cell composition in the PDAC microenvironment, with a limited analysis of the CAF phenotype and localization.
In this manuscript, we applied a 31-antibody panel to define the organization and composition of the PDAC tumor microenvironment by IMC. We focused on the phenotype and the spatial localization of different CAF subpopulations, together with their relationship with immune, endothelial cells (ECs) and tumor cells. With this approach, we provide a comprehensive analysis of the PDAC microenvironment with the aim of better defining its cellular complexity, thereby identifying subtypes and cell signatures of relevance and useful in diagnosis and instrumental for new treatment strategies.
The analyzed cohort includes 8 patients diagnosed with PDAC surgically resected at the Humanitas Research Hospital between 2022 and 2023. Patients’ histopathological and clinical features are listed in Supplementary Table 1. Patients had not received any therapy before resection. Written informed consent was obtained for each patient included in the study. The study protocol was in accordance with ethical guidelines established in the 1975 Declaration of Helsinki and was approved by the local ethical committee (Authorization n° 3801).
5μm-thick formalin-fixed, paraffin-embedded (FFPE) sections from PDAC tissue blocks were deparaffinized in xylen and rehydrated through a graded alcohol series. Tissue sections were stained with Hematoxylin (Histo-Line Laboratories, Pantigliate (MI) - Italy) for 15 minutes, extensively washed in H2O for 10 minutes, and then stained with Eosin (Histo-Line Laboratories, Pantigliate (MI) - Italy) for 7 minutes. After a rapid wash in H2O, slides were dehydrated through a graded alcohol series, washed in xylene and then mounted with Eukitt (Sigma-Aldrich, St. Louis, Missouri, USA). Whole-slide scans were acquired by a ZEISS Axio Scan Z1 Slide Scanner and visualized with QuPath software (version 0.5.1).
2μm-thick FFPE sections from PDAC tissue blocks were deparaffinized in xylen and rehydrated through a graded alcohol series. Slides were then incubated with EDTA, pH 9 antigen retrieval solution (Agilent Technologies, Santa Clara, CA 95051, USA) in a water bath at 98°C for 20 minutes, followed by a 10-minutes cooling down in antigen retrieval solution and by an additional 10-minutes cooling down in distilled water. To prevent non-specific antibody binding, slides were incubated in PBS supplemented with Ca2+ and Mg2+ (PBS2+) (Lonza, Basel, Switzerland) supplemented with 0.1% Triton X-100, 3% BSA (Sigma-Aldrich, St. Louis, Missouri, USA), 5% Normal Mouse (Biosera, Cholet, France)/Rat(Sigma-Aldrich, St. Louis, Missouri, USA)/Rabbit(Dako, Agilent Technologies, Santa Clara, CA 95051, USA)/Goat(Sigma-Aldrich, St. Louis, Missouri, USA)/Sheep (Sigma-Aldrich, St. Louis, Missouri, USA) serum, for 45 minutes at room temperature in a humified chamber. Slides were then incubated with the metal-conjugated antibody mix, diluted in PBS2+ supplemented with 0.01% Triton X-100, 0.3% BSA (Sigma-Aldrich, St. Louis, Missouri, USA), 0.5% Normal Mouse (Biosera, Cholet, France)/Rat(Sigma-Aldrich, St. Louis, Missouri, USA)/Rabbit(Dako, Agilent Technologies, Santa Clara, CA 95051, USA)/Goat(Sigma-Aldrich, St. Louis, Missouri, USA), Sheep (Sigma-Aldrich, St. Louis, Missouri, USA) Serum, overnight at 4°C in a humified chamber. Slides were washed 4 times, 5 minutes each, in PBS2+ 0.05% Tween–20 (Merck, Darmstadt, Germany). For nuclear staining, tissues were then incubated with 0.3 µM Ir191/193 (Standard Biotools, South San Francisco, CA, USA) in PBS2+ for 30 minutes at room temperature. After incubation, tissue sections were washed 3 times, 3 minutes each, in PBS2+ 0.05% Tween-20. Finally, sections were washed for 30 seconds in ultrapure H2O to remove salt leftovers and quickly airdried. The list of 31 metal-conjugated antibodies used in this study is reported in Supplementary Table 2. Metal-tagged antibodies recognizing alpha smooth muscle actin (αSMA), CD163, CD20, CD66b and collagen-I were purchased from Standard Biotools. The remaining antibodies were conjugated to lanthanide isotypes using the Maxpar® X8 Antibody Labelling Kit (Standard Biotools, South San Francisco, CA, USA) according to the manufacturer’s instructions and resuspended in PBS2+ and 0.05% NaN3. Titration tests were performed for each metal-conjugated antibody to optimize the staining protocol.
Images were acquired with a Hyperion Imaging System (Standard Biotools, South San Francisco, CA, USA). To ensure system stability, the Hyperion Imaging System was routinely calibrated following the manufacturer’s instructions. For each patient, 2 consecutive sections were cut and stained for H&E and IMC staining, respectively, as previously described. On the H&E-dedicated slides, 3 to 5 regions of interest (ROIs), corresponding to tumor regions, were selected by a specialized pathologist. The same regions were then identified on the IMC dedicated slides and 1 mm2 ROIs were ablated with a UV laser, with a frequency of 200Hz, at a resolution of approximately 1µm2. IMC acquired regions were then revised by a specialized pathologist to confirm the presence of the neoplastic tissue. Antibodies that showed high level of background signal in tumor tissue or did not exhibit a clear staining pattern were excluded from the analysis, resulting in a final panel of 31 metal-tagged antibodies.
IMC image analysis was performed using a custom pipeline as previously described (25). Briefly, hot pixel removal (radius=2, threshold=50) was performed on single–channel images extracted from mcd files. For each channel, low–intensity thresholds were manually settled based on visual inspection and a cutoff was set to at the top 99.99% percentile of expression (or at least at an intensity value of 10 dual counts) calculated over all the considered ROIs. Gaussian filter (r=2) was applied exclusively to estimate of pan-Cytokeratin+ (Pan-Ck+), CD45+, CD31+ and fibroblast activation protein (FAP+) positive area to avoid bias due to missing nuclear signal and small debrids.
Tiffs substacks containing the complete list of channels relevant for segmentation and cell classification were created. Ilastik (v1.3.3post3) (26) and CellProfiler (v4.2.1) (27) were used to perform single–pixel classification and cell segmentation. R EBImage package (v4.36) (28) was used to obtain channel intensity and shape parameters for each cell. Objects with area < 10µm2, area larger than 1000µm2, mean intensity higher than 2 in more than 15 markers, and lower than 0.01 in the markers used for Uniform Manifold Approximation and Projection (UMAP) analysis were discarded. No more than 2% of the cells were discarded based on these criteria.
Following inverse hyperbolic sine transformation and normalization of the data between 1% and 99% of the overall signal, UMAP (https://CRAN.R-project.org/package=umap, v0.2.8) and PhenoGraph (v 0.99) (k=60) algorithms were used for dimensional reduction and clustering analysis. Clusters were assigned to five different cellular populations (tumor, immune, ECs, CAFs, pancreatic islets). PhenoGraph analysis (k=20) was then performed on the five subsets separately, in order to identify clusters of cells misannotated. After reassignment of all the cells to the correct populations, cells were re-clustered (k=20) and annotated into more specific subpopulations, as described in the Results section.
Neighboring cells were identified as those located within 30μm from cells borders using 3D interaction Fiji plugin (mcib3D v4.1.5). Interaction counts score were determined using patch method (p=1) from the imcRtools package (v1.9.0, permutation test n=5000). Patch method gives for a reference subpopulation, and each ROI, the fraction of its cells that have at least one neighbor in the target subpopulation. Cells subpopulations with less than 10 cells for ROI were not included in the statistics. For each pair of cell subtypes, permutation test over their positions allows to obtain an estimate of p-value associated to the observed number of interactions compared to those expected by chance. Interactions were tested separately for each ROI, and considered significant when p-value < 0.01. A score +1 or -1 was associated to each significantly positive or negative (more or less associated than expected by random model) interaction. Non-significant interactions were given score zero. The resulting scores, averaged over all the considered ROIs, were represented in heatmaps. For CAF subtypes, the minimum distance from each cell and tumor cells was evaluated with Cdist function (Rdist v0.05) using center of mass. For each cell we calculated the abundance of different neighboring cells (identified, as above, in a radius <30μm) subpopulation. We considered 29 classes, taking into account tumors and endothelial cells as aggregated macro populations, while subpopulations of CAFs and immune cells were considered in detail. The abundance normalized vectors were used as input for kmeans clustering (k=10, 1500 iterations, 10 initialization sets). The resulting clusters were manually annotated, based on center coordinates and cell subtype enrichment, to identify neighborhoods regions similar in composition (Supplementary Table 3).
Relative enrichment of CAFs subtypes in the groups identified by clinical parameters was defined ad –Log10 of the false discovery rate (fdr) from hypergeometric test for the good prognosis group and +Log10 of fdr for bad prognosis group. Thus, larger positive values indicate enrichment in the good prognosis group, while large negative values are indicative of enrichment in the bad prognosis group. Values of fdr were capped to 10-10. Values fdr > 0.001 were set to 1, corresponding to enrichment score 0.
Representative images were prepared using ImageJ (Fiji, version 1.54f) software. Gaussian filter was applied to representative images to increase their quality. GraphPad Prism software (version 9.0.2), dittoSeq (v1.6.0) and ggplot2 (v3.4) R packages were used to prepare graphs and to performed statistical analysis. Two-sample, two sided Kolmogorov-Smirnov (KS) test was used to compare distances distribution. Hypergeometric test was performed based on HypeR package (29). False discovery correction was applied to all p-values and reported as p-adjusted (padj).
Multiple staining protocols combined with IMC technology at single-cell resolution (Figure 1A) were applied to human tissues of PDAC (n=8) (Supplementary Table 1). A total of 34 ROIs (1mm2 range of n=3-5 per patient) were selected for acquisition, based on histopathological evaluation, including both neoplastic glands from PDAC (with various grades of differentiation) and stromal tissue, with the aim of acquiring regions similarly divided between PDAC and remaining TME (23±11% Pan-Ck+ area, n=34 PDAC). As stated in Supplementary Table 2 and shown in Supplementary Figure 1A, tissue sections were stained with 31 metal-tagged antibodies detecting classical markers of tumor cells (Pan-Ck, Ck-7), pancreatic islets (peptide C); monocytic (CD45, CD68), polymorphonuclear (CD66b, MMP-9) and lymphoid (CD45, CD3, CD8, CD20) immune cells; cells composing the blood vessel wall, including vascular ECs (CD31, CD34), smooth muscle cells (CD146) and pericytes (CD146, αSMA); lymphatic ECs (CD31, podoplanin). Detection of differential markers subtyped the cells of mesenchymal origin (vimentin, desmin, cadherin-11, podoplanin, CD74, S100A4, CD44, FAP), which vary according to the functional differentiation and specialization in sites of cancer tissue (30–32). In addition, a definition of the diversity of ECM components (collagen-I, collagen-3A, collagen-IV, fibrinogen, pentraxin 3 (PTX3)) served to assess a functional association with different cell types in the proximity, such as functionality and stability of tumor blood vessels by measuring collagen IV-rich coverage (33). Additional markers included CD206, CD163 and HLA-DR, to reveal a functional state of tumor-associated macrophage (TAM) (M1 versus M2 polarization), whereas evaluation of carbonic anhydrase 9 (CA-IX) expression defined the cancer cell capable in sustaining local acidosis, and hence in favoring cancer progression (34) (Supplementary Figure 1A).
Figure 1. Cellular landscape in PDAC by IMC. (A) Schematic view of the IMC analysis workflow. (B) Representative images out of 34 acquired ROIs (n=8 PDAC) showing extracted signal contribution of Pan-Ck (cyan), vimentin (green), αSMA (magenta), CD45 (red), CD31 (yellow) and correspondent merged image. Bar: 200µm. (C) Upper panel, representative images out of 34 acquired ROIs (n=8 PDAC) showing signal contribution of Pan-Ck (green) and Collagen I (blue). Bar: 200µm. Lower panel, representative images out of 34 acquired ROIs (n=8 PDAC) showing Pan-Ck (magenta) and Collagen 3A (green). Bar: 200µm. (D) Representative images out of 34 acquired ROIs (n=8 PDAC) showing Pan-Ck (cyan), peptide C (red) and FAP (yellow). White arrow in the inset indicates the pancreatic islet expressing peptide C (red) and FAP (yellow). Bar: 200µm. (E) UMAP representation of PDAC cells annotated into tumor cells, immune cells, CAFs, ECs, pancreatic islet and other cells. (F) Representative reconstructed images showing the localization within the PDAC tissues of the segmented cells (black contours) corresponding to tumor cell (green), immune cells (yellow), CAF (blue), ECs (cyan), pancreatic islets (red) and other (gray) cell clusters. (G) Frequency of cells belonging to tumor cell (green), immune cell (yellow), CAF (blue), ECs (cyan), pancreatic islets (red) and other cell (gray) clusters, as in the legend. Bar: 200µm. (H) Heatmap referring to the normalized expression of each single markers of the acquired images (n=34 ROIs; n=8 PDAC) after PhenoGraph analysis, among the different clusters.
In all PDAC tissues analyzed, Pan-Ck+ regions are randomly arranged and surrounded by a dense desmoplastic and collagenous stroma enriched of αSMA and vimentin. Dispersed blood vessels (2.1±1.5% CD31+ area n=34 ROIs) and immune cells (15.7±8.2% CD45+ area; n=34 ROIs) enclosed the tumor cells (Figures 1B, C). Isolated epithelial peptide C+/FAP+ pancreatic islets (35) (0.4±0.6% peptide C+ area, range 0-2.3%, 0.8±1.9% FAP+ range 0-9%; n=34 ROIs) were distinguished from Pan-Ck+ cells (Figure 1D).
In a single-cell segmentation analysis, we generated a mask for each ROI of PDAC identifying a total number of 122827 cells (range 12080-18643 cells for n=8 PDAC) (a representative image of single-cell segmentation is shown in Supplementary Figure 1B). PhenoGraph analysis generated 32 different clusters, subdivided in tumor cells (Pan-Ck+ and Ck-7+), immune cells (CD45+, CD3+, CD68+, CD66b+, CD20+), ECs (CD31+, CD34+, podoplanin+), CAFs (CD45-, Pan-Ck-, Ck-7-, CD31-, αSMA+, vimentin+, CD74+, CD44+, S100A4+, FAP+, podoplanin+, cadherin-11+, desmin+), pancreatic islets (peptide C+) and stated as other cells for not expressing specific markers. UMAP representation of the annotated cluster is shown in in Figure 1E; Supplementary Figure 2A and, per single patient, in Supplementary Figure 2B. Representative images of the distribution of annotated cell subtypes in PDAC tissue are shown in Figure 1F.
CAFs represented the most abundant cell population identified (n=41339 cells, n=8 PDAC; range 21-43.6% per PDAC) and corresponded to 33.6% of the annotated cells, compared with tumor cells (n=38284; 31.2%, n=8; range 22.5-37.2% per PDAC), immune cells (n=28661 cells, 23.3%, n=8; range 12.7-45.5% per PDAC) and ECs (n=8596 cells, 7%, n=8; range 2.9-12.2% per PDAC) (Figure 1G). 4.1% of cells (n=5070; n=8; range 0.6-10.4% per PDAC) were not specifically annotated. The clusters identified were homogeneously represented among the different PDAC patients, with a diversity of relative abundance more associated with specific subtypes of CAFs and tumor cells (Supplementary Figure 2B).
As shown in the averaged intensity-based heatmap (Figure 1H) and in line with transcriptomic studies in PDAC (36), tumor cells were further reclassified into 7 different subtypes, based on the expression of Pan-Ck, Ck-7, CD44, S100A4 and CA-IX. Although PTX3 was recognized as a molecule predominantly associated with cells of mesenchymal origin in PDAC (37), two clusters of tumor cells (Tumor 3, Pan-Ck+ PTX3+; Tumor 5, Pan-Ck+ Ck-7+ PTX3+) were identified based on its high expression. Immune cells were identified as myeloid cells (CD45+, CD68-, CD206+) and as a whole subtyped in M1-like (CD45+, CD68+, HLA-DR+, CD74+), M2-like macrophages (CD45+, CD68+, CD206+, CD163+) or CD44-expressing macrophages (CD45+, CD68+, CD44+); neutrophils (CD45+, CD66b+); CD4+ T cells (CD45+, CD3+, CD8-) and CD8+ T cells (CD45+, CD3+, CD8+); B cells (CD45+, CD20+) (Figure 1H). On the basis on the selective expression of markers of pericytes (αSMA, CD146) and coverage of a collagen-IV+ basement (38), blood vessels were distinguished into functioning and stabilized (CD31+, CD34+, Collagen-IV+, CD146+, αSMA+, cadherin-11+) from non-stabilized (CD31+, CD34+, collagen-IV-, CD146-) tumor neo-angiogenesis, as well as in CD31+ podoplanin+ lymphatic vessels (Figure 1H). As reported, CAFs represent a multitude of potentially dynamic and plastic subgroups that change their gene expression profiles based on the stimuli from the environment (39), thereby influencing tumor progression through the tissue fibrotic reaction, the regulation of tissue biomechanical property and the modulation of the immune response to chemotherapy (11). Therefore, detection of multiple CAF markers, including αSMA, vimentin, S100A4, CD74, FAP, desmin, cadherin-11, CD34, CD146, CD44, CA-IX, podoplanin, collagen-I, collagen-3A and PTX3, served to discriminate the subpopulation of CAFs having a different functional impact in PDAC. As shown in Figure 1H, CAFs were classified into 19 different clusters.
Tumor cells (n=38284; 31.2%, n=8; range 22.5-37.2% per PDAC) were annotated into 7 different subtypes, based on the expression of Pan-Ck, cytokeratin 7 (Ck-7), CD44, S100A4, PTX3, CA-IX, CD74 (Figures 2A, B). Although the expression levels changed among the subtypes, all tumor cells expressed Pan-Ck. A cluster with an exclusive expression of Pan-Ck alone corresponded to 18.8% (n=8; range 1.1-73.5% per PDAC) of tumor cells (Tumor 1), whereas majority of tumor cells expressed both Pan-Ck and Ck-7 (Tumor 2, n=8; range 1.1-74.0% per PDAC). Some clusters expressed a combination of markers associated with tumor proliferation and invasiveness (40), such as CD44, S100A4, CD74 and MMP-9 (Tumor 7, 29.1%, n=8; range 0.7-81.5% per PDAC) (Figure 2A–C). In particular, expression of CD44, a classical marker associated with epithelial-to-mesenchymal transition and poor prognosis of PDAC progression and metastasis (41, 42), was found associated with Tumor 3 (3.6%, range 0.2-9.0% per PDAC), Tumor 4 (2.2%, range 0-10.0% per PDAC) and Tumor 7 subtypes (Figures 2A–C). Few cells in the Tumor 6 subtype (4.1%, n=8; range 0.2-11.5% per PDAC) expressed the hypoxic marker CA-IX (Figures 2B, D). Therefore, as shown by IMC analysis, PDAC consists of a phenotypic diversity (n=7 identified clusters) of tumor cells potentially associated with different capacity for tumorigenesis and metastasis. As shown by UMAP in Supplementary Figure 3, for the same clusters, heterogeneity in the expression of the same markers was observed among the PDAC patients analyzed.
Figure 2. Profiling of tumor cells in PDAC. (A) UMAP representation of PDAC tumor cells annotated to 7 tumor cell subtypes, colors as in legend. (B) UMAP representation of the normalized expression of single markers in segmented cells annotated as tumor cells. (C) Representative images out of 34 acquired ROIs (n=8 PDAC) showing Pan-Ck (magenta) signal in combination with CD44 (green, left panel), S100A4 (green, middle panel) and CA-IX (green, right panel). White arrowheads on the onset (bottom) indicate tumor cells expressing CD44 (left panel), S100A4 (middle panel) and CA-IX (right panel). Bar: 200µm. (D) Frequency of cells belonging to the identified tumor subtypes, over the total number of tumor cells, as in legend. (E) UMAP representation of the subset of tumor cells in PDAC #1 and #3. Black-dotted line is a guide for the eye. Colors and UMAP coordinates as in (A). (F) Representative images of Pan-Ck (yellow), Ck-7 (magenta) and PTX3 (green) signal in the PDAC #1. White arrows and white arrowheads in the inset show tumor cells co-expressing Pan-Ck/Ck-7/PTX3 (Tumor 5) or Pan-Ck/PTX3 alone, respectively. Bar: 200µm. (G) Representative images of the same region represented in (F), showing the tissue localization of segmented cells (black contours) annotated as Tumor 5 (green) in PDAC #1. Red arrows and red arrowheads in the inset show tumor cells co-expressing Pan-Ck/Ck-7/PTX3 (Tumor 5) or Pan-Ck/PTX3 alone, respectively. Bar: 200µm.
PTX3 is a humoral innate immune molecule produced by macrophages (43) and mesenchymal cells (37), which plays a role of extrinsic oncosuppressor of cancer by regulating complement-dependent tumor-promoting inflammation (43). On the other hand, PTX3 was found elevated in PDAC tissue and associated with an increased capacity of cancer cells to invade ECM (37). As observed in other tumors, PTX3 produced by cancer cells promote tumor progression by promoting invasiveness and migration (44, 45). Interestingly, we found that the Tumor 5 (12.9%, range 0-43.7% per PDAC, Figure 2D), expressing Pan-Ck, Ck-7 and PTX3 was almost exclusively found in PDAC #1 and #3 only, counting for the 90% of all identified Tumor 5 cells (Figure 2E), who were diagnosed with distant metastasis at the time of surgery (Supplementary Table 1). Co-expression of Pan-Ck, Ck-7 and PTX3 is restricted to a specific subset of cells (34.5% in PDAC#1; 43.7% in PDAC#3) (Figure 2F, white arrows and Figure 2G, red arrows), compared to the neighbor cells which lack the expression of Ck-7 (Figure 2F, white arrowheads and Figure 2G, red arrowheads), thus suggesting in an attempt to speculate the identification of subpopulation of PDAC cells associated with high tumor metastatic potential.
In immune cell population (n=28661 cells), a functional specialization of macrophage was defined based on the expression of classical M1 (CD68+, HLA-DR+, CD74+) or M2 (CD68+, CD163+, CD206+) markers. The percentage of M2-like macrophages (14.7%, range 6.5-26.8% per PDAC) was higher compared to M1-like macrophages (8.2%, range 1.7-24.4% per PDAC), thus indicating a propensity for M2 deviation in the tumor microenvironment of PDAC, and hence sustained tumorigenesis, immune evasion, and metastasis formation (46, 47) (Figures 3A, B). A distinguished cluster of macrophages showed exclusive enrichment in CD44 (10.8%, range 1.5-35.9% per PDAC), and lower expression of HLA-DR and CD74, or of CD163 and CD206 (Figures 1H, 3E, F, yellow arrowheads). In PDAC, the same macrophage subtype was recently described to belong to the vascular niche and to be distinguished by a pro-angiogenic gene signature (24). Noteworthy, distribution of macrophages around Pan-Ck tumor cells is shown in Figure 3F, with M2-like macrophages expressing higher levels of CD163 and CD206 (Figure 3F, white arrows) and M1-like macrophages expressing higher levels of HLA-DR and CD74 (Figure 3F, white arrowheads). In addition, as previously shown in Figure 1H, S100A4 expression was higher in M2-like macrophages compared to M1-like macrophages, suggesting their pro-tumorigenic activity (48). The remaining subtypes of immune cells identified were found to be CD66b+ neutrophils; CD3+, CD4+ (CD8-) T cells; CD3+, CD8+ T cells; CD20+ B cells; and eventually CD68-, CD206+ myeloid dendritic cells (Figure 3A; Supplementary Figures 4A, B). T cells represent the 34.8% of the tumor infiltrating cells (range 19.7-51.3%; n=8 PDAC), with CD8+ and CD4+ T cells counting for the 19.6% (range 8.4-32.2%; n=8) and 15.19% (range 5.1-26.2%; n=8) of T lymphocytes, respectively (Figures 3B, C: CD3+, CD8-, white arrowheads, CD3+, CD8+, white arrows). On the contrary, B cells poorly infiltrate tumor tissue (4.46%; range 0.1-13.3%; n=8) (Figures 3B, C; yellow arrowheads). Neutrophil cluster (10.2%; range 0.2-29.3%; n=8) (Figure 3B) infiltrating tumor tissues were identified based on the expression of CD66b and high levels MMP-9 stored into their tertiary granules (Figures 3D, E). As observed in tumor cell profiling, immune cell composition of the TME is heterogeneous among the analyzed patients: neutrophil abundance was higher in PDAC #1, (29.4%) #2 (20.5%), #3 (9.8%) and #6 (15.9%); PDAC #2 showed a highest frequency of both M1-like (24.4%) and M2-like (26.8%) macrophages; CD44+ were abundant in PDAC #4 (35.9%) and #8 (19.5%) (Supplementary Figure 4C).
Figure 3. Profiling of immune cells in PDAC. (A) UMAP representation of immune cells in PDAC (n=8 PDAC), as in legend. (B) Frequency of cells belonging to the identified immune cell subpopulations, over the total number of immune cells, as in legend. (C) Representative images out of 34 acquired ROIs (n=8 PDAC) showing signal contribution of Pan-Ck (green) CD3 (magenta) CD8 (cyan) and CD20 (yellow). White arrowheads, white arrows and yellow arrows in the inset indicate CD4 T cells (identified as CD3+/CD8- T cells), CD8+ T cells and B cells respectively. Bar: 200µm. (D) Representative images out of 34 acquired ROIs (n=8 PDAC) showing Pan-Ck (green), CD66b+ neutrophils (magenta) and MMP-9+ (cyan). Arrowheads in the inset indicate CD66b+ neutrophils expressing MMP-9. Bar: 200µm. (E) UMAP representation of the normalized expression of immune cell markers for the identification of macrophages subpopulation (M1 macrophages as CD68+/HLA-DRhigh/CD74high, M2 macrophages as CD68+/CD163high/CD206high and CD44+ macrophages as CD68+/CD44+) and neutrophils (CD66b+/MMP-9+). (F) Representative images out of 34 acquired ROIs (n=8 PDAC) showing the extracted signal contribution of Pan-Ck (green), CD68 (magenta), HLA-DR (blue) CD74 (gray), CD163 (cyan), CD206 (red) and CD44 (yellow). White arrowheads indicate M1 macrophages as CD68+/HLA-DRhigh/CD74high, white arrows indicate M2 macrophages as CD68+/CD163high/CD206high, yellow arrows indicate CD44+ macrophages as CD68+/CD44+. Bar: 200µm. (G) Results of the neighboring cell analysis, as heatmap, showing the average percentage of each indicated cell subtype (Reference) that are in proximity (≤ 30μm distance) to each indicated cell subpopulations (Target).
Analysis of the frequency of cells (Reference) in close proximity (<30μm) with other cell types (Target) showed a preferential neighboring of B cells to both CD4+ (72 ± 28% of B cells) and CD8+ T cells (81 ± 21% of B cells) whereas no interaction was observed between immune cells and PDAC, with the exception of a weak association with CD44+ macrophages (59 ± 24% of cells vs all the Tumor subtypes), M1-like macrophages (67 ± 20% of cells vs all the Tumor subtypes) and neutrophils (65 ± 25% of cells vs all tumor subtypes) (Figure 3G; Supplementary Figure 5).
CAFs are tumor-promoting cells abundant in the ECM with a multifaceted phenotype (49, 50) and promising targets for new anticancer interventions (11, 51). In the present study, major efforts were therefore directed towards the identification of profiling markers of the different functional activities of CAFs in PDAC. Single-cell segmentation and PhenoGraph analysis identified n=41339 total CAFs (33.8% on total cells, n=8 PDAC; range 21-43.5% per PDAC tissue) annotated into 19 different subtypes, based on the differential expression of CD44, CA-IX, CD74, S100A4, αSMA, vimentin, FAP, desmin, cadherin-11, CD34, CD146, podoplanin, collagen I, collagen 3A and PTX3 (Figure 4A; Supplementary Figure 5A). As with immune cells, a phenotypic heterogeneity in the CAF population was found among the analyzed patients, although no specific CAF subtype was restricted to individual patients (Supplementary Figure 5B). According to the literature (49), most of the CAFs identified belonged to clusters 2 (12.7%, n=8; range 4.6-44.4%), 6 (26.4%, n=8; range 7-43.6%) and 15 (10.7%, n=8; range 2.4-30.6%) which express almost exclusively αSMA and vimentin (Figures 4A, B) and correspond to myofibroblast-like CAFs (myCAFs). In a further dissection of myCAFs, while CAFs 6 show a concomitant expression of αSMA and vimentin (Figures 4A, C, red arrows), CAFs 2 and CAFs 15 were expressing αSMA or vimentin alone, respectively (Figures 4A, C, red arrowhead: αSMA+ CAFs 2; yellow arrowhead: vimentin+ CAFs 15). Distinctly, the αSMA+ vimentin+ clusters CAFs 1 (1.2%, n=8; range 0.2-2.5%) and CAFs 3 (0.7%, n=8; range 0.2-2.9%) were patently identified as pericytes, exhibiting the expression of markers of mesenchymal origin, included cadherin-11 and CD146, and are associated CD31+ ECs (Figure 4D). In addition to the expression of αSMA and vimentin, CAFs 9 (6.3%, n=8; range 0.04-20.4%), 10 (3.5%, n=8; range 0.4-9.2%), 11 (4.8%, n=8; range 0.2-18%) and 14 (3.4%, n=8; range 0.3-12.9%) showed higher expression of FAP, also in combination with S100A4 and PTX3 (CAFs 10) or cadherin-11 (CAFs 11), thus identifying phenotypically different subtypes and emphasizing their functional evolution and plasticity in PDAC (e.g. myCAF vs. inflammartory CAFs (iCAFs)) (52, 53) (Figure 4E). Interestingly, FAP+ αSMA+ subtypes were localized closer to tumor cells compared to the previously identified myCAFs (Figure 4E) (average minimal distance from the tumor cells: CAFs 9, 29.7 ± 25.5µm, n=2591 cells; CAFs 10, 36.6 ± 60.2µm, n=1437 cells; CAFs 11, 32.5 ± 36.9µm, n=1980 cells; vs. CAFs 2, 68.0 ± 63.3µm, n=5254 cells; CAFs 6, 95.7 ± 108.0µm, n=10905 cells; CAFs 15, 61.3 ± 79.7µm; n=4430 cells; p-value < 10-15 KS test for all conditions), as well as to the FAP+ αSMA- CAFs 14 subpopulation (56.4 ± 54.7µm, n= 1389; p-value < 10-15 KS test). Other subtypes identified included CAFs 19 distinguished by higher expression of desmin (2.0%, n=8; range 0.3-4.7%) (Figures 4A, B); CAFs 16 (6.3%, n=8; range 3.2-9.2%), the only cluster that showed a distinctive expression of CD74 and HLA-DR but lacking of CD45 expression (Figure 4F, white arrows), thus suggesting the overt identification of CAFs having immunological properties in PDAC, the so-called antigen-presenting CAFs (apCAFs) (54); the poorly represented CAFs 18 (1.1%, n=8; range 0.04-4.7%) and CAFs 8 (3.5%, n=8; range 0.3-6.7%) expressed high levels of CD44, alone (CAFs 18), or in combination with elevated αSMA and Vimentin (CAF 8) (Figures 4A, B); CAFs 5 (1.5%, n=8; range 0-7.7%), CAFs 7 (1.8%, n=8; range 0.2-7.2%) and CAFs 12 (2.8%, n=8; range 0-15.2%) showed expression of podoplanin, a well-defined CAF predictive marker of PDAC progression (55), in combination with αSMA (CAFs 5), αSMA and vimentin (CAFs 7), or with αSMA and vimentin and cadherin-11 (CAFs 12), (Figures 4A, B). In contrast to proteomic studies on CAFs in breast cancer (56), no consistent association between CD34 expression and CAFs was observed (Figure 4A). As expected, many of the clusters of CAFs that included CAFs 2, 6, 11, 12 and 13 (Figure 4A), were associated with collagen I and 3A, pointing to them as major players involved in a continuous interaction with the regions of the tumor tissue associated with deposition and remodeling of ECM (57).
Figure 4. Profiling of CAFs in PDAC. (A) Dot plot showing each CAF marker average normalized expression and the relative percentage of positive cells, among the annotated CAF subtypes. (B) Barplot showing the relative percentage of cells among the identified subtypes on total CAFs. (C) Representative images out of 34 acquired ROIs (n=8 PDAC) of Pan-Ck (gray), vimentin (green) and αSMA (blue). Red arrows indicate cells co-expressing vimentin and αSMA. Red arrowheads and yellow arrowheads indicate cell expressing vimentin or αSMA only. Bar, 200µm. (D) Representative images out of 34 acquired ROIs (n=8 PDAC) showing the extracted signal contribution of Pan-Ck (gray), vimentin (green), αSMA (blue), CD31 (yellow), cadherin-11 (red) and CD146 (cyan). Red arrow indicates CD31+ blood vessel (yellow) covered by vimentin+/αSMA+/cadherin-11+/CD146+ pericytes. Bar, 200µm. (E) Representative images out of 34 acquired ROIs (n=8 PDAC) showing the extracted signal contribution of Pan-Ck (gray), FAP (magenta), vimentin (green) and αSMA (blue). Yellow arrowheads indicate CAFs co-expressing FAP, vimentin and αSMA surrounding Pan-Ck+ tumor cells. Bar, 200µm. (F) Representative images out of 34 acquired ROIs (n=8 PDAC) showing the extracted signal contribution of Pan-Ck (gray), CD45 (red), CD74 (green) and HLA-DR (magenta). White arrowheads indicate immune cells (CD45+) expressing CD74 and HLA-DR, while white arrows indicate CAFs (CD45-) expressing CD74 and HLA-DR. Bar: 200µm.
A spatial association between cell subpopulations was evaluated by neighboring analysis, highlighting pairwise association between specific cell types, and neighborhood enrichment, aimed to identify larger regions homogeneous in cell composition (Figure 5). In association with a high phenotype diversity of CAFs, neighboring cell analysis revealed the presence of high heterogeneity in their spatial relationship with other cells in PDAC (Figure 5A). Except for CAFs 6, which showed an association with CAFs 2, 4, 8, 13 and 16 (fraction of CAFs 6 neighboring CAFs 2, 51 ± 27%; CAFs 4, 30 ± 19%; CAFs 8, 34 ± 18%; CAFs 13, 36 ± 23%; CAFs 16, 50 ± 20%; n= 10905 cells), no specific relationship was found between them. As expected, CAFs 1 and 3, identified as αSMA+ vimentin+ CD146+ pericytes, (Figures 4A, D) were spatially associated with ECs (Figure 5A). In particular, CAFs 1 significantly associated with CD146+ and collagen IV+ CD31+ ECs (Figure 1E; Supplementary Figure 5, score 0.57 ± 0.51), thus suggesting a role in controlling blood vessel functionality.
Figure 5. Neighborhood analysis of CAFs in PDAC. (A) Results of the single cell neighboring analysis, as heatmap, showing the average percentage of each indicated cell subtype (Reference) that are in proximity (≤ 30μm radius) to cells of each indicated subpopulation (Target). (B) Heatmap showing the average proximity score for each pair of cell phenotypic subpopulations of CAF (Reference) to immune cells (Target). Positive (red) or negative (blue) values indicate that a specific pair of phenotypes is neighboring significantly more often or significantly less often, respectively, than expected from a randomized placement, as described in Material and Methods. 30μm radius is considered for cell-to-cell proximity, as in (A). (C) Heatmap, showing the average proximity score for each pair of cell phenotypic subpopulations, as in B, assuming immune cells as Reference populations and CAF subtypes as Target. (D) Representative spatial distribution of cellular neighborhoods, identified as regions with similar cellular composition, as described in Material and Methods, into two different ROIs (out of n=34). Each dot represents the center of mass of a single cell, dot color corresponds to annotated neighborhood, as in legend. Annotation was performed based on enrichment analysis reported in Supplementary Table 3. (E) Relative abundance of cellular populations across the annotated cellular neighborhoods as in (D) Values are normalized by column.
In addition, CAF FAP+ subtypes (CAFs 9, 10 and 11) preferentially localized in proximity with Pan-CK+ cells (CAFs 9 86 ± 10%, n=2575 cells; CAFs 10 76 ± 19%, n=1405 cells; CAFs 11 80 ± 17%, n=1929 cells) (Figure 5A).
A consistent spatial association between CAFs and immune cells was observed (Figure 5A). Considering the subtypes of immune cells identified (Figures 5B, C), CAFs 1 and CAFs 3, identified as pericytes, showed an interaction, respectively, with CD4+ T cells (Figure 5B, score 0.07 ± 0.47) and myeloid cells (Figure 5B, score 0.14 ± 0.38). Moreover, CAFs 3 were associated with CD44+ macrophages (Figure 5C, score 0.29 ± 0.49) and M2-like macrophages (Figure 5C, score 0.43 ± 0.53). Of note, CAFs 1 and 3 mainly differ by the expression of cadherin-11 (Figure 1E), whose expression has been associated to anti-tumor immune response in a genetic mouse model of PDAC (58). Similarly, podoplanin+ CAFs 7 were found in association with CD4+ T cells (Figure 5B, score 0.06 ± 0.44) and CD44+ macrophages (Figure 5B, 0.06 ± 0.25). FAP+ CAFs 10 and 11 interacted preferentially with CD44+ macrophages (Figure 5B, CAFs 10, score 0.04 ± 0.36; CAFs 11, score 0.1 ± 0.3) while FAP+ CAFs 14 showed relationship with M1-like macrophages (Figure 5B, score 0.11 ± 0.47). These CAF subtypes were observed in the peritumoral niche around Pan-Ck+ cells, in correspondence with an enrichment of macrophages (Figures 3H, 4G, E). As evidence for an identification of a CAF cluster with immunoregulatory properties (apCAFs) in PDAC, CD4+ T cells were observed significantly close to CAFs 16 (Figure 5C, score 0.09 ± 0.445).
We then investigated the presence of patterns of localized enrichment resulting in the identification of 10 classes of regions similar in cellular composition (neighborhoods, Figures 5D, E). Thus, each ROI can be divided into sub-regions highlighting spatial adjacent cells belonging to different spatial context (59) (Figure 5D). The analysis shows that regions identified as tumor-stroma interface are particularly enriched of FAP+ CAFs 10 and CAFs 11 (padj<0.001, Figure 5E; Supplementary Table 3), in agreement with the evidence resulting from the neighboring analysis (Figures 4E, 5A). In addition, CAFs 1, expressing pericyte markers, were enriched in perivascular region (padj<0.001). We also identified three neighborhoods of immune cells, enriched either in B cells and CD8+ T cells (Lymphoid Cell Enriched Stroma), or neutrophils and CD44+ and M1-like macrophages (Myeloid Enriched Stroma 1) or myeloid cells and CD44+ macrophages (Myeloid Enriched Stroma 2), with distinct spatial distribution in the ROIs (Figures 5D, E). Several CAF subtypes were associated with the immune enriched neighborhoods. Among them, apCAFs (CAFs 16) were associated with Lymphoid Cell Enriched Stroma and Myeloid Cell Enriched Stroma 2 (padj<0.001, Figure 5D; Supplementary Table 3).
To evaluate an association between CAF subtypes and PDAC progression, we evaluate their distribution in patients with circulating levels of carbohydrate antigen 19-9 (CA19-9) (60), disease-free survival (DFS) and survival status (Supplementary Table 1). We divided patients into high CA19-9 (n=5) and low CA19-9 (n=3), setting a threshold level of 100IU/L, as previously reported (61). We found that FAP+ CAFs, namely CAFs 9, CAFs 10, CAFs 11 and CAFs 14, as well as podoplanin+ cadherin-11+ CAFs12 were enriched in patients with higher level of CA19-9 (padj<0.001) (Figures 6A, D–F; Supplementary Figure 6A). Interestingly, CAFs 12, as well as podoplanin+ CAFs 5 are also associated with a worst DFS (n= 4 patients, DFS<13.5 months, padj <0.001) (Figures 6B, D; Supplementary Figure 6B). Similarly, CAFs 12 were associated to a shorter survival (n=3 patients, survival<18 months, padj<0.001)(Figures 6C, D; Supplementary Figure 6C). Higher frequency of CAFs 12 (81%) was found in the metastatic patient #1. Figure 6F show the concomitant infiltration of podoplanin+ cadherin-11- CAFs 5 (white arrow) and podoplanin+ cadherin-11+ CAFs 12 (white arrowheads), close to tumor cells. Finally, CAFs 1, expressing the conventional pericyte markers, are associated with higher CA19-9 levels and worst patients prognosis (padj<0.001)(Figures 6A-D; Supplementary Figure 6). On the other hand, CD44+ CAFs 8, vimentin+ CAFs 15 and apCAFs (CAFs 16) are associated with lower CA19-9 levels, as well as longer DFS and survival (padj<0.001) (Figures 6A-D; Supplementary Figure 6).
Figure 6. Association between CAFs and patients’ levels of CA19-9, disease-free survival and survival status. (A-C) Barplot showing frequency of each CAF subpopulation in association with patients’ pre-operative levels of CA19-9 [(A), blue >100IU/l, green ≤100IU/l], disease-free survival [(B), blue ≤13.5 months, green >13.5 months] and overall survival [(C), blue ≤18 months, green >18 months) (PDAC n=8 total)]. Data are reported as frequency normalized over the total number of cells in each CAF group and annotated according to patient status. Number of patient in each group is reported in legend. (D) Relative enrichment score of each CAF subtype in the good (red) or bad (lightblue) prognosis group for three different clinical parameters as above. Higher absolute values correspond to more significant enrichment, values closer to zero are less significant. P-value adjusted > 0.001 are set to enrichment 0. (E) Representative images out of 34 acquired ROIs (n=8 PDAC) showing the extracted signal contribution of Pan-Ck (yellow), FAP (green), αSMA (magenta) and vimentin (cyan). White arrowheads indicate CAFs co-expressing FAP, vimentin and αSMA surrounding Pan-Ck+ tumor cells. Bar, 200µm. (F) Representative images out of 34 acquired ROIs (n=8 PDAC) showing the extracted signal contribution of Pan-Ck (yellow), cadherin-11 (green) and podoplanin (magenta) (Upper panel). White arrows and arrowheads in close up images (lower panel) show podoplanin+ CAFs and cadherin-11+ podoplanin+ CAFs, respectively. Bar, 200µm.
Overall, single-cell resolution IMC analyses shed light on the phenotypic and spatial complexity of associated PDAC infiltrating CAFs, reflecting their possible functional differences that contribute to disease progression.
Over the last years, several transcriptomic studies described the heterogeneity of CAFs in PDAC, underlying their pivotal role in disease progression and resistance to therapy. Analyses of gene expression profile and spatial location led to the identification of three main CAF subtypes, named myCAFs, iCAFs and apCAFs (52, 62, 63). Although transcriptomic approaches have the potential to identify thousands of genes and new signatures in tissues, a correlation between mRNA and protein can be limited by several factors, including post-transcriptional machinery (64). For this reason, several studies combined single-cell RNA analysis and multiplexed imaging to provide a comprehensive analysis of diseased tissue. Recent studies report the relevance of using IMC complementary to transcriptomics for the purpose of defining the phenotype of cell subpopulations previously revealed by transcriptional approaches (54, 56, 65). On the other hand, while transcriptomic analysis has provided important information on the molecular pathways involved in the activation and differentiation of CAFs in PDAC, only a few studies have investigated their phenotype profiling by protein detection.
In the present work, we applied IMC to investigate cellular composition of 8 PDAC patients. By using a 31-antibody panel, IMC allowed us to describe the tissue architecture, identifying different subtypes of cancer cells, immune cells, ECs and CAFs. In addition, neighborhood analysis implemented the phenotypic data, providing information about the spatial localization of distinct cellular subtypes and their relationship within tumor tissue.
PDACs are characterized by a marked degree of both inter-tumor and intra-tumor heterogeneity in the histomorphology of tumor cells (66). These morphological differences coexist in the same tissues and are associated with distinct gene profiles and generally correlate with disease outcome (67, 68). In addition, morphological heterogeneity can be randomly observed even between immediately adjacent tumor cells, with a gradual transition from one morphological type to another (66). Recently, the concept of morpho-biotypes has been introduced to describe the diverse morphological and spatial organization of PDAC cells with different gene expression profiles, leading to the classification of PDAC into “glandular”, “transitional” and “undifferentiated” (69). In our IMC analysis, we identified 7 different tumor cell subtypes. Beside the common expression of Pan-Ck, tumor cells were characterized by the expression of markers associated with tumor progression, epithelial-to-mesenchymal transition, and resistance to therapy, such as CD44, S100A4 and CA-IX (41, 70, 71). As reported (72) we also observed a marked degree of inter-tumor variability, with some subtypes of tumor cells whose expression was limited to specific patients in our cohort. We identified a subset of tumor cells expressing Pan-Ck, Ck-7 and PTX3, associated with the only two patients in our cohort having distant metastasis at the time of the diagnosis and surgery. Interestingly, a cancer-derived PTX3 production has been associated with tumor progression in several type of cancer, including melanoma, cervical cancer, hepatocellular carcinoma and glioma (44, 45, 73, 74), possible consequence of its role in remodeling ECM (75–77) that occurs in acidic microenvironments (76), an hallmark in cancer (78).
PDAC is generally considered an “immunologically cold” tumor, showing intrinsic properties that lead to the evasion of an effective immune response (79, 80). Almost 90% of PDACs show mutation in KRAS, which is associated to the secretion of granulocyte-macrophages colony-stimulating factor (GM-CSF) and the consequent recruitment of immunosuppressive myeloid cells, as well as the upregulation of programmed death ligand-1 (PD-L1) (81, 82). In addition, the recruitment of regulatory immune cells and the secretion of chemokine and cytokine, such as CXCL12, IL-10 and TGFβ, contribute to the generation of an immunosuppressive microenvironment (83). However, we identified that PDAC is predominantly accompanied by infiltration of M2-like macrophages, consistent with their association with tumor progression, recurrence and metastatic spread in PDAC patients (46). In addition, we identified a distinct population of CD44+ macrophages. Recently, CD44+ macrophages have been described in PDAC tissue as a subtype of HLA-DRLow macrophages enriched in the vascular niche and possibly promoting neovascularization (24). Our analysis confirmed the presence of this phenotype, with lower HLA-DR expression compared to M1-like macrophages: differently, we found that, compared to the other immune cells, CD44+ macrophages, together with M1-like macrophages and neutrophils, are the only immune cells showing a weak spatial interaction with tumor cells. Moreover, our neighborhood analysis (Figures 3G, 5D) showed that immune cells are generally excluded from tumor cell-enriched areas, confirming the immune suppressive microenvironment of PDAC tissue.
As widely described (12, 49, 84), CAFs play a pivotal role in the PDAC development and therapeutic response, by regulating the composition and the structure of the ECM, contributing to the generation of an immune-suppressive environment and influencing PDAC cell proliferation, invasion and drug resistance (11, 84). CAFs have been classified on the basis of their phenotype and function into myCAFs, having high expression of αSMA and located in proximity to tumor cells, iCAFs, showing IL-6high inflammatory feature and located away from neoplastic cells, and apCAFs, expressing MHC-II and CD74, whose function is still matter of study (49, 54). Beside this classification, a variety of markers have been used to define CAFs, but most of them are shared among CAF and non-CAF cell type, thus requiring a further identification of subtype specific markers (85). In our study, we combined the expression of 11 established CAF markers (56, 65), resulting in the definition of 19 different CAF subtypes having distinct phenotype, tissue localization and relationship with other cells. We found that the vast majority of CAFs expressed αSMA and vimentin, thus suggesting the identification of the myCAF phenotype. αSMA+ Vimentin+ myCAFs can be further distinguished into distinct subtypes, based on the differential expression of other CAF markers, such as CD146, S100A4, podoplanin, FAP, CD44 and cadherin-11. αSMA and vimentin can be concomitantly (CAFs 6) or alternatively expressed (CAFs 2 and CAFs 15). Recently, it has been demonstrated that higher vimentin expression in CAFs is associated with a significantly shorter patient survival. In addition, CAFs expressing vimentin alone, without the expression of αSMA, represent an independent predictor of poor prognosis (86). In addition to myCAFs, we identified a subtype of CAF expressing CD74 and HLA-DR (CAFs 16), likely corresponding to apCAFs (54). Unlike professional antigen-presenting cells, apCAFs did not express costimulatory molecules, such as CD40, CD80 and CD86: it has been hypothesized that MHC-II expressed by apCAFs might act as a decoy receptor for CD4+ T cells, inhibiting their clonal expansion, inducing anergy and promoting differentiation into T-regulatory cells, thus contributing to the generation of an immunosuppressive microenvironment (62, 87). In line with this observation, our neighborhood analysis revealed a spatial relationship between CD4+ T cells and CAFs 16 (apCAFs), suggesting a possible immunomodulatory activity.
Generally, CAFs localized in proximity to different immune cell-enriched regions associated with M2-like macrophages, CD44+ macrophages, T cells and myeloid cells. Recently, in mouse models of PDAC, is has been demonstrated that Cdh11 deficiency alters the molecular profile of fibroblasts, reduces the expression of immunosuppressive cytokines and increases the anti-tumor immunity (58, 88).
Podoplanin has emerged as a robust marker for CAFs in PDAC, showing a correlation with worst patients’ prognosis (54, 89). More recently, it has been described a role of podoplanin-positive CAFs in the regulation of immune cell infiltration in PDAC and other tumors (90, 91). In our study, we identified 3 different CAF subtypes expressing podoplanin: among them, CAFs 7 also showed a significant spatial relationship with CD4+ T cells and CD44+ macrophages, suggesting a possible immune modulatory activity of this CAF population.
FAP is considered another well-defined CAF marker in PDAC. FAP+ CAFs are the main repository for CXCL12 in PDAC tumor microenvironment: CXCL12 promotes T cell spatial exclusion, and pharmacological inhibition of CXCL12-CXCR4 interaction results in T cell accumulation in tumor tissue and fostering of immune checkpoint blockade (92). In addition, FAP+ CAFs contribute to ECM desmoplasia, leading to the formation of a dense ECM which limit T cell proximity to PDAC tumor cells (93–96). In our analysis, we found 4 distinct CAF subtypes expressing FAP (CAFs 9, 10, 11 and 14): among this, CAFs 10 and 11 were the only CAF subtypes significantly enriched in tumor-stroma interface region and strictly associated to tumor cells. Moreover, we observed a significant spatial relationship between CAFs 10 and CD44+ macrophages, possibly regulating their recruitment and activity. Given the described role of FAP+ CAFs in regulating desmoplastic reaction and CD44+ macrophages as highly phagocytic cells, in an attempt to speculate these cells can cooperate to a ECM remodeling niche that promotes tumor cell invasion and PDAC progression (42, 94, 97).
CA19-9 is a validated marker to establish PDAC progression, with a good sensitivity (61, 98). Even if the number of patients included in this study is not sufficient to provide reliable correlation between CAF subtypes and patients prognosis, we report that CAFs expressing different combination of FAP, podoplanin and cadherin-11, were associated with higher level of CA19-9.
Recently, expression of αSMA by pericytes, induced by cancer cell-derived exosomes, has been associated with an alteration to the morphology and bio-mechanical properties of pericytes, which significantly correlate with vascular leakiness and hypoxia in PDAC (99), thus compromising the stability of tumor vasculature and hence affecting therapy efficacy. Interestingly, CAFs 1, which expressed higher levels of αSMA compared to the other identified pericyte subtype (CAFs 3), were enriched in perivascular regions, and resulted associated with a worst patients conditions.
In addition to CAFs 1, CAFs 12 cells, expressing podoplanin and cadherin-11, are associated with higher levels of CA19-9 as well as shorter DFS and overall survival. This result is in accordance with the recently observed association between the expression of podoplanin and cadherin-11 and the expansion of mesothelial cells that contribute to stromal deposition and desmoplastic reaction in early neoplastic lesions in mouse (87). In our cohort, majority of CAFs 12 were expressed by the metastatic patient#1, suggesting a possible correlation with PDAC metastatic capability. In line with this observation, podoplanin expression by CAFs has been associated with PDAC progression and invasion (55). On the other side, CAFs 15 and CAFs 16 are associated with lower CA19-9 levels and better patient prognosis. As previously reported, we identified CAFs 16 as apCAFs, based on the concomitant expression of CD74 and HLA-DR (54). Although apCAFs have been generally linked to the generation of an immunomodulatory microenvironment (62, 87), they may exert more complicated immune-regulating functions. For example, in breast cancer, MHC-II+ CAFs were associated with T regulatory cells and resistance to immunotherapy but also correlated with patient survival (100–102). In addition, in lung cancer, MHC-II+ CAFs enhanced CD4+ T cell cancer immunity (103).
In conclusion, our IMC analysis provided an overview of the complexity of the PDAC tumor microenvironments, showing how cancer and stromal cells with different phenotypic characteristics co-exist within the same tissue. In particular, the classification of 19 distinct CAF subtypes, characterized by different combination of fibroblast markers and by a peculiar spatial localization and relationship with surrounding cells, underlies the high plasticity of CAFs in PDAC and their complex role in PDAC progression, leading to the potential identification of new targets for the diagnosis and the treatment of PDAC patients.
The raw data supporting the conclusions of this article will be made available by the authors, without undue reservation.
The studies involving humans were approved by Comitato Etico Territoriale Lombardia 5, Via Alessandro Manzoni 56, 20089 Rozzano (Milano). The studies were conducted in accordance with the local legislation and institutional requirements. The participants provided their written informed consent to participate in this study.
ME: Conceptualization, Data curation, Formal analysis, Investigation, Methodology, Validation, Visualization, Writing – original draft. MF: Data curation, Formal analysis, Methodology, Software, Validation, Visualization, Writing – original draft. RD: Data curation, Formal analysis, Software, Writing – review & editing. MS: Resources, Writing – review & editing. SB: Resources, Writing – review & editing. GN: Resources, Writing – review & editing. DZ: Investigation, Writing – review & editing. RP: Investigation, Writing – review & editing. CG: Funding acquisition, Supervision, Writing – review & editing. LR: Writing – review & editing, Resources. LT: Resources, Supervision, Writing – review & editing. SB: Supervision, Writing – review & editing. AZ: Resources, Supervision, Writing – review & editing. AM: Supervision, Writing – review & editing. AD: Conceptualization, Funding acquisition, Project administration, Supervision, Writing – original draft, Writing – review & editing.
The author(s) declare financial support was received for the research, authorship, and/or publication of this article. This study was funded by Ministero degli Affari Esteri e della Cooperazione Internazionale (MAECI; Project no. 2023-23683652-ITALIA-SINGAPORE 2023-2026), ITALY -SINGAPORE SCIENCE AND TECHNOLOGY COOPERATION, A*STAR (Grant No. R22I0IR118) and EU funding within the MUR PNRR Italian network of excellence for advanced diagnosis (Project no. PNC-E3-2022-23683266 PNC-HLS-DA). This work was partially supported by “Ricerca Corrente” funding from Italian Ministry of Health to IRCCS Humanitas Research Hospital.
IMC workflow in Figure 1A has been created with BioRender.com.
LR reports grant/research funding to institution from Agios, AstraZeneca, BeiGene, Eisai, Exelixis, Fibrogen, Incyte, IPSEN, Lilly, MSD, Nerviano Medical Sciences, Roche, Servier, Taiho Oncology, TransThera Sciences, and Zymeworks; consulting fees from AbbVie, AstraZeneca, Basilea, Bayer, Bristol Myers Squibb, Elevar Therapeutics, Exelixis, Genenta, Hengrui, Incyte, IPSEN, IQVIA, Jazz Pharmaceuticals, MSD, Nerviano Medical Sciences, Roche, Servier, Taiho Oncology, and Zymeworks; lecture fees from AstraZeneca, Bayer, Bristol Myers Squibb, Guerbet, Incyte, IPSEN, Roche, and Servier; and travel expenses from AstraZeneca.
The remaining authors declare that the research was conducted in the absence of any commercial or financial relationships that could be construed as a potential conflict of interest.
The author(s) declared that they were an editorial board member of Frontiers, at the time of submission. This had no impact on the peer review process and the final decision.
The reviewer MM declared a shared affiliation, with no collaboration, with the authors to the handling editor at the time of the review.
All claims expressed in this article are solely those of the authors and do not necessarily represent those of their affiliated organizations, or those of the publisher, the editors and the reviewers. Any product that may be evaluated in this article, or claim that may be made by its manufacturer, is not guaranteed or endorsed by the publisher.
The Supplementary Material for this article can be found online at: https://www.frontiersin.org/articles/10.3389/fimmu.2024.1472433/full#supplementary-material
1. Siegel RL, Miller KD, Fuchs HE, Jemal A. Cancer statistics, 2022. CA Cancer J Clin. (2022) 72:7–33. doi: 10.3322/caac.21708
2. Halbrook CJ, Lyssiotis CA, Pasca di Magliano M, Maitra A. Pancreatic cancer: advances and challenges. Cell. (2023) 186:1729–54. doi: 10.1016/j.cell.2023.02.014
3. Petersen GM. Familial pancreatic cancer. Semin Oncol. (2016) 43:548–53. doi: 10.1053/j.seminoncol.2016.09.002
4. Cronin KA, Scott S, Firth AU, Sung H, Henley SJ, Sherman RL, et al. Annual report to the nation on the status of cancer, part 1: national cancer statistics. Cancer. (2022) 128:4251–84. doi: 10.1002/cncr.34479
5. Kanda M, Matthaei H, Wu J, Hong SM, Yu J, Borges M, et al. Presence of somatic mutations in most early-stage pancreatic intraepithelial neoplasia. Gastroenterology. (2012) 142:730–3.e9. doi: 10.1053/j.gastro.2011.12.042
6. Waddell N, Pajic M, Patch AM, Chang DK, Kassahn KS, Bailey P, et al. Whole genomes redefine the mutational landscape of pancreatic cancer. Nature. (2015) 518:495–501. doi: 10.1038/nature14169
7. Cancer Genome Atlas Research Network. Electronic address aadhe, Cancer Genome Atlas Research N. Integrated Genomic Characterization of Pancreatic Ductal Adenocarcinoma. Cancer Cell. (2017) 32:185–203 e13. doi: 10.1016/j.ccell.2017.07.007
8. Fujikura K, Hosoda W, Felsenstein M, Song Q, Reiter JG, Zheng L, et al. Multiregion whole-exome sequencing of intraductal papillary mucinous neoplasms reveals frequent somatic KLF4 mutations predominantly in low-grade regions. Gut. (2021) 70:928–39. doi: 10.1136/gutjnl-2020-321217
9. Ho WJ, Jaffee EM, Zheng L. The tumour microenvironment in pancreatic cancer - clinical challenges and opportunities. Nat Rev Clin Oncol. (2020) 17:527–40. doi: 10.1038/s41571-020-0363-5
10. Liot S, Balas J, Aubert A, Prigent L, Mercier-Gouy P, Verrier B, et al. Stroma involvement in pancreatic ductal adenocarcinoma: an overview focusing on extracellular matrix proteins. Front Immunol. (2021) 12:612271. doi: 10.3389/fimmu.2021.612271
11. Zhang T, Ren Y, Yang P, Wang J, Zhou H. Cancer-associated fibroblasts in pancreatic ductal adenocarcinoma. Cell Death Dis. (2022) 13:897. doi: 10.1038/s41419-022-05351-1
12. Pereira BA, Vennin C, Papanicolaou M, Chambers CR, Herrmann D, Morton JP, et al. Caf subpopulations: A new reservoir of stromal targets in pancreatic cancer. Trends Cancer. (2019) 5:724–41. doi: 10.1016/j.trecan.2019.09.010
13. Siegel RL, Miller KD, Wagle NS, Jemal A. Cancer statistics, 2023. CA Cancer J Clin. (2023) 73:17–48. doi: 10.3322/caac.21763
14. Tsujikawa T, Kumar S, Borkar RN, Azimi V, Thibault G, Chang YH, et al. Quantitative multiplex immunohistochemistry reveals myeloid-inflamed tumor-immune complexity associated with poor prognosis. Cell Rep. (2017) 19:203–17. doi: 10.1016/j.celrep.2017.03.037
15. Steele NG, Carpenter ES, Kemp SB, Sirihorachai VR, The S, Delrosario L, et al. Multimodal mapping of the tumor and peripheral blood immune landscape in human pancreatic cancer. Nat Cancer. (2020) 1:1097–112. doi: 10.1038/s43018-020-00121-4
16. Yousuf S, Qiu M, Voith von Voithenberg L, Hulkkonen J, Macinkovic I, Schulz AR, et al. Spatially resolved multi-omics single-cell analyses inform mechanisms of immune dysfunction in pancreatic cancer. Gastroenterology. (2023) 165:891–908.e14. doi: 10.1053/j.gastro.2023.05.036
17. Giesen C, Wang HA, Schapiro D, Zivanovic N, Jacobs A, Hattendorf B, et al. Highly multiplexed imaging of tumor tissues with subcellular resolution by mass cytometry. Nat Methods. (2014) 11:417–22. doi: 10.1038/nmeth.2869
18. Le Rochais M, Hemon P, Pers JO, Uguen A. Application of high-throughput imaging mass cytometry hyperion in cancer research. Front Immunol. (2022) 13:859414. doi: 10.3389/fimmu.2022.859414
19. Zhao C, Germain RN. Multiplex imaging in immuno-oncology. J Immunother Cancer. (2023) 11:e006923. doi: 10.1136/jitc-2023-006923
20. Chang Q, Ornatsky OI, Siddiqui I, Loboda A, Baranov VI, Hedley DW. Imaging mass cytometry. Cytometry A. (2017) 91:160–9. doi: 10.1002/cyto.a.23053
21. Byrne KT, Betts CB, Mick R, Sivagnanam S, Bajor DL, Laheru DA, et al. Neoadjuvant selicrelumab, an agonist Cd40 antibody, induces changes in the tumor microenvironment in patients with resectable pancreatic cancer. Clin Cancer Res. (2021) 27:4574–86. doi: 10.1158/1078-0432.CCR-21-1047
22. Wattenberg MM, Colby S, Garrido-Laguna I, Xue Y, Chang R, Delman D, et al. Intratumoral cell neighborhoods coordinate outcomes in pancreatic ductal adenocarcinoma. Gastroenterology. (2024) 166:1114–29. doi: 10.1053/j.gastro.2024.01.013
23. Yoon S, Li H, Quintanar L, Armstrong B, Rossi JJ. Uncovering differently expressed markers and heterogeneity on human pancreatic cancer. Transl Oncol. (2020) 13:100749. doi: 10.1016/j.tranon.2020.100749
24. Sussman J, Kim NY, Kemp SB, Traum D, Katsuda T, Kahn BM, et al. Multiplexed imaging mass cytometry analysis characterizes the vascular niche in pancreatic cancer. Cancer Res. (2024) 84(14):2364–76. doi: 10.1158/0008-5472.CAN-23-2352
25. Erreni M, Fumagalli MR, Zanini D, Candiello E, Tiberi G, Parente R, et al. Multiplexed imaging mass cytometry analysis in preclinical models of pancreatic cancer. Int J Mol Sci. (2024) 25:1389. doi: 10.3390/ijms25031389
26. Berg S, Kutra D, Kroeger T, Straehle CN, Kausler BX, Haubold C, et al. Ilastik: interactive machine learning for (Bio)Image analysis. Nat Methods. (2019) 16:1226–32. doi: 10.1038/s41592-019-0582-9
27. Stirling DR, Swain-Bowden MJ, Lucas AM, Carpenter AE, Cimini BA, Goodman A. Cellprofiler 4: improvements in speed, utility and usability. BMC Bioinf. (2021) 22:433. doi: 10.1186/s12859-021-04344-9
28. Pau G, Fuchs F, Sklyar O, Boutros M, Huber W. Ebimage–an R package for image processing with applications to cellular phenotypes. Bioinformatics. (2010) 26:979–81. doi: 10.1093/bioinformatics/btq046
29. Federico A, Monti S. Hyper: an R package for geneset enrichment workflows. Bioinformatics. (2020) 36:1307–8. doi: 10.1093/bioinformatics/btz700
30. Kalluri R, Zeisberg M. Fibroblasts in cancer. Nat Rev Cancer. (2006) 6:392–401. doi: 10.1038/nrc1877
31. Uccelli A, Moretta L, Pistoia V. Mesenchymal stem cells in health and disease. Nat Rev Immunol. (2008) 8:726–36. doi: 10.1038/nri2395
32. Kalluri R. The biology and function of fibroblasts in cancer. Nat Rev Cancer. (2016) 16:582–98. doi: 10.1038/nrc.2016.73
33. Carmeliet P, Jain RK. Molecular mechanisms and clinical applications of angiogenesis. Nature. (2011) 473:298–307. doi: 10.1038/nature10144
34. McDonald PC, Chafe SC, Dedhar S. Overcoming hypoxia-mediated tumor progression: combinatorial approaches targeting pH regulation, angiogenesis and immune dysfunction. Front Cell Dev Biol. (2016) 4:27. doi: 10.3389/fcell.2016.00027
35. Segerstolpe A, Palasantza A, Eliasson P, Andersson EM, Andreasson AC, Sun X, et al. Single-cell transcriptome profiling of human pancreatic islets in health and type 2 diabetes. Cell Metab. (2016) 24:593–607. doi: 10.1016/j.cmet.2016.08.020
36. Zhang S, Fang W, Zhou S, Zhu D, Chen R, Gao X, et al. Single cell transcriptomic analyses implicate an immunosuppressive tumor microenvironment in pancreatic cancer liver metastasis. Nat Commun. (2023) 14:5123. doi: 10.1038/s41467-023-40727-7
37. Goulart MR, Watt J, Siddiqui I, Lawlor RT, Imrali A, Hughes C, et al. Pentraxin 3 is a stromally-derived biomarker for detection of pancreatic ductal adenocarcinoma. NPJ Precis Oncol. (2021) 5:61. doi: 10.1038/s41698-021-00192-1
38. van Splunder H, Villacampa P, Martinez-Romero A, Graupera M. Pericytes in the disease spotlight. Trends Cell Biol. (2024) 34:58–71. doi: 10.1016/j.tcb.2023.06.001
39. Brichkina A, Polo P, Sharma SD, Visestamkul N, Lauth M. A quick guide to CAF subtypes in pancreatic cancer. Cancers (Basel). (2023) 15:2614. doi: 10.3390/cancers15092614
40. Jordan AR, Racine RR, Hennig MJ, Lokeshwar VB. The role of CD44 in disease pathophysiology and targeted treatment. Front Immunol. (2015) 6:182. doi: 10.3389/fimmu.2015.00182
41. Zhao S, Chen C, Chang K, Karnad A, Jagirdar J, Kumar AP, et al. CD44 expression level and isoform contributes to pancreatic cancer cell plasticity, invasiveness, and response to therapy. Clin Cancer Res. (2016) 22:5592–604. doi: 10.1158/1078-0432.CCR-15-3115
42. Chen C, Zhao S, Zhao X, Cao L, Karnad A, Kumar AP, et al. Gemcitabine resistance of pancreatic cancer cells is mediated by igf1r dependent upregulation of CD44 expression and isoform switching. Cell Death Dis. (2022) 13:682. doi: 10.1038/s41419-022-05103-1
43. Bonavita E, Gentile S, Rubino M, Maina V, Papait R, Kunderfranco P, et al. PTX3 is an extrinsic oncosuppressor regulating complement-dependent inflammation in cancer. Cell. (2015) 160:700–14. doi: 10.1016/j.cell.2015.01.004
44. Ying TH, Lee CH, Chiou HL, Yang SF, Lin CL, Hung CH, et al. Knockdown of pentraxin 3 suppresses tumorigenicity and metastasis of human cervical cancer cells. Sci Rep. (2016) 6:29385. doi: 10.1038/srep29385
45. Rathore M, Girard C, Ohanna M, Tichet M, Ben Jouira R, Garcia E, et al. Cancer cell-derived long pentraxin 3 (PTX3) promotes melanoma migration through a toll-like receptor 4 (Tlr4)/Nf-Kappab signaling pathway. Oncogene. (2019) 38:5873–89. doi: 10.1038/s41388-019-0848-9
46. Yang S, Liu Q, Liao Q. Tumor-associated macrophages in pancreatic ductal adenocarcinoma: origin, polarization, function, and reprogramming. Front Cell Dev Biol. (2020) 8:607209. doi: 10.3389/fcell.2020.607209
47. Mantovani A, Allavena P, Marchesi F, Garlanda C. Macrophages as tools and targets in cancer therapy. Nat Rev Drug Discovery. (2022) 21:799–820. doi: 10.1038/s41573-022-00520-5
48. Liu S, Zhang H, Li Y, Zhang Y, Bian Y, Zeng Y, et al. S100a4 enhances protumor macrophage polarization by control of PPAR-gamma-dependent induction of fatty acid oxidation. J Immunother Cancer. (2021) 9:e002548. doi: 10.1136/jitc-2021-002548
49. Geng X, Chen H, Zhao L, Hu J, Yang W, Li G, et al. Cancer-associated fibroblast (CAF) heterogeneity and targeting therapy of CAFs in pancreatic cancer. Front Cell Dev Biol. (2021) 9:655152. doi: 10.3389/fcell.2021.655152
50. Stouten I, van Montfoort N, Hawinkels L. The tango between cancer-associated fibroblasts (CAFs) and immune cells in affecting immunotherapy efficacy in pancreatic cancer. Int J Mol Sci. (2023) 24:8707. doi: 10.3390/ijms24108707
51. Yamashita K, Kumamoto Y. CAFs-associated genes (Cafgs) in pancreatic ductal adenocarcinoma (PDAC) and novel therapeutic strategy. Int J Mol Sci. (2024) 25:6003. doi: 10.3390/ijms25116003
52. Ohlund D, Handly-Santana A, Biffi G, Elyada E, Almeida AS, Ponz-Sarvise M, et al. Distinct populations of inflammatory fibroblasts and myofibroblasts in pancreatic cancer. J Exp Med. (2017) 214:579–96. doi: 10.1084/jem.20162024
53. Xu Y, Li W, Lin S, Liu B, Wu P, Li L. Fibroblast diversity and plasticity in the tumor microenvironment: roles in immunity and relevant therapies. Cell Commun Signal. (2023) 21:234. doi: 10.1186/s12964-023-01204-2
54. Elyada E, Bolisetty M, Laise P, Flynn WF, Courtois ET, Burkhart RA, et al. Cross-species single-cell analysis of pancreatic ductal adenocarcinoma reveals antigen-presenting cancer-associated fibroblasts. Cancer Discovery. (2019) 9:1102–23. doi: 10.1158/2159-8290.CD-19-0094
55. Shindo K, Aishima S, Ohuchida K, Fujiwara K, Fujino M, Mizuuchi Y, et al. Podoplanin expression in cancer-associated fibroblasts enhances tumor progression of invasive ductal carcinoma of the pancreas. Mol Cancer. (2013) 12:168. doi: 10.1186/1476-4598-12-168
56. Cords L, Tietscher S, Anzeneder T, Langwieder C, Rees M, de Souza N, et al. Cancer-associated fibroblast classification in single-cell and spatial proteomics data. Nat Commun. (2023) 14:4294. doi: 10.1038/s41467-023-39762-1
57. Perez VM, Kearney JF, Yeh JJ. The PDAC extracellular matrix: A review of the ECM protein composition, tumor cell interaction, and therapeutic strategies. Front Oncol. (2021) 11:751311. doi: 10.3389/fonc.2021.751311
58. Peran I, Dakshanamurthy S, McCoy MD, Mavropoulos A, Allo B, Sebastian A, et al. Cadherin 11 promotes immunosuppression and extracellular matrix deposition to support growth of pancreatic tumors and resistance to gemcitabine in mice. Gastroenterology. (2021) 160:1359–72 e13. doi: 10.1053/j.gastro.2020.11.044
59. Schurch CM, Bhate SS, Barlow GL, Phillips DJ, Noti L, Zlobec I, et al. Coordinated cellular neighborhoods orchestrate antitumoral immunity at the colorectal cancer invasive front. Cell. (2020) 182:1341–59 e19. doi: 10.1016/j.cell.2020.07.005
60. van Oosten AF, Groot VP, Dorland G, Burkhart RA, Wolfgang CL, van Santvoort HC, et al. Dynamics of serum CA19-9 in patients undergoing pancreatic cancer resection. Ann Surg. (2024) 279:493–500. doi: 10.1097/SLA.0000000000005977
61. Ballehaninna UK, Chamberlain RS. The clinical utility of serum CA 19-9 in the diagnosis, prognosis and management of pancreatic adenocarcinoma: an evidence based appraisal. J Gastrointest Oncol. (2012) 3:105–19. doi: 10.3978/j.issn.2078-6891.2011.021
62. Hosein AN, Huang H, Wang Z, Parmar K, Du W, Huang J, et al. Cellular heterogeneity during mouse pancreatic ductal adenocarcinoma progression at single-cell resolution. JCI Insight. (2019) 5:e129212. doi: 10.1172/jci.insight.129212
63. Dominguez CX, Muller S, Keerthivasan S, Koeppen H, Hung J, Gierke S, et al. Single-cell RNA sequencing reveals stromal evolution into lrrc15(+) myofibroblasts as a determinant of patient response to cancer immunotherapy. Cancer Discovery. (2020) 10:232–53. doi: 10.1158/2159-8290.CD-19-0644
64. Haider S, Pal R. Integrated analysis of transcriptomic and proteomic data. Curr Genomics. (2013) 14:91–110. doi: 10.2174/1389202911314020003
65. Cords L, Engler S, Haberecker M, Ruschoff JH, Moch H, de Souza N, et al. Cancer-associated fibroblast phenotypes are associated with patient outcome in non-small cell lung cancer. Cancer Cell. (2024) 42:396–412 e5. doi: 10.1016/j.ccell.2023.12.021
66. Verbeke C. Morphological heterogeneity in ductal adenocarcinoma of the pancreas - does it matter? Pancreatology. (2016) 16:295–301. doi: 10.1016/j.pan.2016.02.004
67. NK S, Wilson GW, Grant RC, Seto M, O'Kane G, Vajpeyi R, et al. Morphological classification of pancreatic ductal adenocarcinoma that predicts molecular subtypes and correlates with clinical outcome. Gut. (2020) 69:317–28. doi: 10.1136/gutjnl-2019-318217
68. Juiz N, Elkaoutari A, Bigonnet M, Gayet O, Roques J, Nicolle R, et al. Basal-like and classical cells coexist in pancreatic cancer revealed by single-cell analysis on biopsy-derived pancreatic cancer organoids from the classical subtype. FASEB J. (2020) 34:12214–28. doi: 10.1096/fj.202000363RR
69. Di Chiaro P, Nacci L, Arco F, Brandini S, Polletti S, Palamidessi A, et al. Mapping functional to morphological variation reveals the basis of regional extracellular matrix subversion and nerve invasion in pancreatic cancer. Cancer Cell. (2024) 42:662–81.e10. doi: 10.1016/j.ccell.2024.02.017
70. Che P, Yang Y, Han X, Hu M, Sellers JC, Londono-Joshi AI, et al. S100a4 promotes pancreatic cancer progression through a dual signaling pathway mediated by Src and focal adhesion kinase. Sci Rep. (2015) 5:8453. doi: 10.1038/srep08453
71. McDonald PC, Chafe SC, Brown WS, Saberi S, Swayampakula M, Venkateswaran G, et al. Regulation of ph by carbonic anhydrase 9 mediates survival of pancreatic cancer cells with activated KRAS in response to hypoxia. Gastroenterology. (2019) 157:823–37. doi: 10.1053/j.gastro.2019.05.004
72. Milan M, Diaferia GR, Natoli G. Tumor cell heterogeneity and its transcriptional bases in pancreatic cancer: A tale of two cell types and their many variants. EMBO J. (2021) 40:e107206. doi: 10.15252/embj.2020107206
73. Tung JN, Ko CP, Yang SF, Cheng CW, Chen PN, Chang CY, et al. Inhibition of pentraxin 3 in glioma cells impairs proliferation and invasion in vitro and in vivo. J Neurooncol. (2016) 129:201–9. doi: 10.1007/s11060-016-2168-z
74. Song T, Wang C, Guo C, Liu Q, Zheng X. Pentraxin 3 overexpression accelerated tumor metastasis and indicated poor prognosis in hepatocellular carcinoma via driving epithelial-mesenchymal transition. J Cancer. (2018) 9:2650–8. doi: 10.7150/jca.25188
75. Erreni M, Manfredi A, Garlanda C, Mantovani A, Rovere-Querini P. The long pentraxin PTX3: A prototypical sensor of tissue injury and a regulator of homeostasis. Immunol Rev. (2017) 280:112–25. doi: 10.1111/imr.12570
76. Doni A, Musso T, Morone D, Bastone A, Zambelli V, Sironi M, et al. An acidic microenvironment sets the humoral pattern recognition molecule PTX3 in a tissue repair mode. J Exp Med. (2015) 212:905–25. doi: 10.1084/jem.20141268
77. Doni A, Stravalaci M, Inforzato A, Magrini E, Mantovani A, Garlanda C, et al. The long pentraxin PTX3 as a link between innate immunity, tissue remodeling, and cancer. Front Immunol. (2019) 10:712. doi: 10.3389/fimmu.2019.00712
78. Boedtkjer E, Pedersen SF. The acidic tumor microenvironment as a driver of cancer. Annu Rev Physiol. (2020) 82:103–26. doi: 10.1146/annurev-physiol-021119-034627
79. Ullman NA, Burchard PR, Dunne RF, Linehan DC. Immunologic strategies in pancreatic cancer: making cold tumors hot. J Clin Oncol. (2022) 40:2789–805. doi: 10.1200/JCO.21.02616
80. Hartupee C, Nagalo BM, Chabu CY, Tesfay MZ, Coleman-Barnett J, West JT, et al. Pancreatic cancer tumor microenvironment is a major therapeutic barrier and target. Front Immunol. (2024) 15:1287459. doi: 10.3389/fimmu.2024.1287459
81. Bayne LJ, Beatty GL, Jhala N, Clark CE, Rhim AD, Stanger BZ, et al. Tumor-derived granulocyte-macrophage colony-stimulating factor regulates myeloid inflammation and T cell immunity in pancreatic cancer. Cancer Cell. (2012) 21:822–35. doi: 10.1016/j.ccr.2012.04.025
82. Coelho MA, de Carne Trecesson S, Rana S, Zecchin D, Moore C, Molina-Arcas M, et al. Oncogenic ras signaling promotes tumor immunoresistance by stabilizing PD-L1 mRNA. Immunity. (2017) 47:1083–99.e6. doi: 10.1016/j.immuni.2017.11.016
83. Karamitopoulou E. Tumour microenvironment of pancreatic cancer: immune landscape is dictated by molecular and histopathological features. Br J Cancer. (2019) 121:5–14. doi: 10.1038/s41416-019-0479-5
84. Rebelo R, Xavier CPR, Giovannetti E, Vasconcelos MH. Fibroblasts in pancreatic cancer: molecular and clinical perspectives. Trends Mol Med. (2023) 29:439–53. doi: 10.1016/j.molmed.2023.03.002
85. Kobayashi H, Enomoto A, Woods SL, Burt AD, Takahashi M, Worthley DL. Cancer-associated fibroblasts in gastrointestinal cancer. Nat Rev Gastroenterol Hepatol. (2019) 16:282–95. doi: 10.1038/s41575-019-0115-0
86. Maehira H, Miyake T, Iida H, Tokuda A, Mori H, Yasukawa D, et al. Vimentin expression in tumor microenvironment predicts survival in pancreatic ductal adenocarcinoma: heterogeneity in fibroblast population. Ann Surg Oncol. (2019) 26:4791–804. doi: 10.1245/s10434-019-07891-x
87. Huang H, Wang Z, Zhang Y, Pradhan RN, Ganguly D, Chandra R, et al. Mesothelial cell-derived antigen-presenting cancer-associated fibroblasts induce expansion of regulatory T cells in pancreatic cancer. Cancer Cell. (2022) 40:656–73.e7. doi: 10.1016/j.ccell.2022.04.011
88. Sebastian A, Martin KA, Peran I, Hum NR, Leon NF, Amiri B, et al. Loss of cadherin-11 in pancreatic ductal adenocarcinoma alters tumor-immune microenvironment. Front Oncol. (2023) 13:1286861. doi: 10.3389/fonc.2023.1286861
89. Hirayama K, Kono H, Nakata Y, Akazawa Y, Wakana H, Fukushima H, et al. Expression of podoplanin in stromal fibroblasts plays a pivotal role in the prognosis of patients with pancreatic cancer. Surg Today. (2018) 48:110–8. doi: 10.1007/s00595-017-1559-x
90. Suzuki J, Aokage K, Neri S, Sakai T, Hashimoto H, Su Y, et al. Relationship between podoplanin-expressing cancer-associated fibroblasts and the immune microenvironment of early lung squamous cell carcinoma. Lung Cancer. (2021) 153:1–10. doi: 10.1016/j.lungcan.2020.12.020
91. Neuzillet C, Nicolle R, Raffenne J, Tijeras-Raballand A, Brunel A, Astorgues-Xerri L, et al. Periostin- and podoplanin-positive cancer-associated fibroblast subtypes cooperate to shape the inflamed tumor microenvironment in aggressive pancreatic adenocarcinoma. J Pathol. (2022) 258:408–25. doi: 10.1002/path.6011
92. Feig C, Jones JO, Kraman M, Wells RJ, Deonarine A, Chan DS, et al. Targeting cxcl12 from fap-expressing carcinoma-associated fibroblasts synergizes with anti-pd-L1 immunotherapy in pancreatic cancer. Proc Natl Acad Sci U.S.A. (2013) 110:20212–7. doi: 10.1073/pnas.1320318110
93. Hartmann N, Giese NA, Giese T, Poschke I, Offringa R, Werner J, et al. Prevailing role of contact guidance in intrastromal T-cell trapping in human pancreatic cancer. Clin Cancer Res. (2014) 20:3422–33. doi: 10.1158/1078-0432.CCR-13-2972
94. Lo A, Wang LS, Scholler J, Monslow J, Avery D, Newick K, et al. Tumor-promoting desmoplasia is disrupted by depleting fap-expressing stromal cells. Cancer Res. (2015) 75:2800–10. doi: 10.1158/0008-5472.CAN-14-3041
95. Jiang H, Hegde S, Knolhoff BL, Zhu Y, Herndon JM, Meyer MA, et al. Targeting focal adhesion kinase renders pancreatic cancers responsive to checkpoint immunotherapy. Nat Med. (2016) 22:851–60. doi: 10.1038/nm.4123
96. Cheng CS, Yang PW, Sun Y, Song SL, Chen Z. Fibroblast activation protein-based theranostics in pancreatic cancer. Front Oncol. (2022) 12:969731. doi: 10.3389/fonc.2022.969731
97. Chen C, Zhao S, Karnad A, Freeman JW. The biology and role of CD44 in cancer progression: therapeutic implications. J Hematol Oncol. (2018) 11:64. doi: 10.1186/s13045-018-0605-5
98. Luo G, Jin K, Deng S, Cheng H, Fan Z, Gong Y, et al. Roles of CA19-9 in pancreatic cancer: biomarker, predictor and promoter. Biochim Biophys Acta Rev Cancer. (2021) 1875:188409. doi: 10.1016/j.bbcan.2020.188409
99. Natarajan V, Ha S, Delgado A, Jacobson R, Alhalhooly L, Choi Y, et al. Acquired alphasma expression in pericytes coincides with aberrant vascular structure and function in pancreatic ductal adenocarcinoma. Cancers (Basel). (2022) 14:2448. doi: 10.3390/cancers14102448
100. Costa A, Kieffer Y, Scholer-Dahirel A, Pelon F, Bourachot B, Cardon M, et al. Fibroblast heterogeneity and immunosuppressive environment in human breast cancer. Cancer Cell. (2018) 33:463–79.e10. doi: 10.1016/j.ccell.2018.01.011
101. Kieffer Y, Hocine HR, Gentric G, Pelon F, Bernard C, Bourachot B, et al. Single-cell analysis reveals fibroblast clusters linked to immunotherapy resistance in cancer. Cancer Discovery. (2020) 10:1330–51. doi: 10.1158/2159-8290.CD-19-1384
102. Friedman G, Levi-Galibov O, David E, Bornstein C, Giladi A, Dadiani M, et al. Cancer-associated fibroblast compositions change with breast cancer progression linking the ratio of S100a4(+) and Pdpn(+) CAFs to clinical outcome. Nat Cancer. (2020) 1:692–708. doi: 10.1038/s43018-020-0082-y
Keywords: multiplexed histopathology, Imaging Mass Cytometry, pancreatic cancer, tumor microenvironment, cancer-associated fibroblasts (CAFs)
Citation: Erreni M, Fumagalli MR, D’Anna R, Sollai M, Bozzarelli S, Nappo G, Zanini D, Parente R, Garlanda C, Rimassa L, Terracciano LM, Biswas SK, Zerbi A, Mantovani A and Doni A (2024) Depicting the cellular complexity of pancreatic adenocarcinoma by Imaging Mass Cytometry: focus on cancer-associated fibroblasts. Front. Immunol. 15:1472433. doi: 10.3389/fimmu.2024.1472433
Received: 29 July 2024; Accepted: 08 October 2024;
Published: 07 November 2024.
Edited by:
Ombretta Melaiu, University of Rome Tor Vergata, ItalyReviewed by:
Marina Macchini, San Raffaele Hospital (IRCCS), ItalyCopyright © 2024 Erreni, Fumagalli, D’Anna, Sollai, Bozzarelli, Nappo, Zanini, Parente, Garlanda, Rimassa, Terracciano, Biswas, Zerbi, Mantovani and Doni. This is an open-access article distributed under the terms of the Creative Commons Attribution License (CC BY). The use, distribution or reproduction in other forums is permitted, provided the original author(s) and the copyright owner(s) are credited and that the original publication in this journal is cited, in accordance with accepted academic practice. No use, distribution or reproduction is permitted which does not comply with these terms.
*Correspondence: Andrea Doni, YW5kcmVhLmRvbmlAaHVtYW5pdGFzcmVzZWFyY2guaXQ=
†These authors have contributed equally to this work
Disclaimer: All claims expressed in this article are solely those of the authors and do not necessarily represent those of their affiliated organizations, or those of the publisher, the editors and the reviewers. Any product that may be evaluated in this article or claim that may be made by its manufacturer is not guaranteed or endorsed by the publisher.
Research integrity at Frontiers
Learn more about the work of our research integrity team to safeguard the quality of each article we publish.