- 1School of Clinical Medicine, The Affiliated Hospital, Southwest Medical University, Luzhou, China
- 2Intensive Care Unit, Xichong People’s Hospital, Nanchong, China
- 3Department of Anaesthesiology, Southwest Medical University, Luzhou, China
- 4Department of Oncology, Chongqing General Hospital, Chongqing University, Chongqing, China
- 5Department of Respiratory and Critical Care Medicine, The Affiliated Hospital, Southwest Medical University, Luzhou, China
- 6Department of Oncology, The Affiliated Hospital, Southwest Medical University, Luzhou, China
Lung cancer is one of the most common malignant tumours worldwide and its high mortality rate makes it a leading cause of cancer-related deaths. To address this daunting challenge, we need a comprehensive understanding of the pathogenesis and progression of lung cancer in order to adopt more effective therapeutic strategies. In this regard, integrating multi-omics data of the lung provides a highly promising avenue. Multi-omics approaches such as genomics, transcriptomics, proteomics, and metabolomics have become key tools in the study of lung cancer. The application of these methods not only helps to resolve the immunotherapeutic mechanisms of lung cancer, but also provides a theoretical basis for the development of personalised treatment plans. By integrating multi-omics, we have gained a more comprehensive understanding of the process of lung cancer development and progression, and discovered potential immunotherapy targets. This review summarises the studies on multi-omics and immunology in lung cancer, and explores the application of these studies in early diagnosis, treatment selection and prognostic assessment of lung cancer, with the aim of providing more personalised and effective treatment options for lung cancer patients.
1 Introduction
Lung cancer has been one of the most common malignant tumours globally over the past decades. Despite the widespread use of conventional treatments such as surgery, radiotherapy, chemotherapy and targeted drug therapy, the five-year survival rate for lung cancer is usually less than 20% (1). Additionally, at all stages, less than 7% of patients survive more than ten years after diagnosis (2). In recent years, the emergence of immunotherapy has marked a revolution in cancer treatments, which not only has an acceptable safety profile, but also produces durable therapeutic responses in a wide range of patient populations (3). Nonetheless, lung cancer exhibits significant histological heterogeneity, diverse genomic profiles, and differential responses to therapy (4), and still poses significant challenges for immunotherapy and prevention.
With the rapid development of multi-omics technology, covering genomics, transcriptomics, proteomics and metabolomics, our understanding of lung cancer is deepening (5, 6). Multi-omics technology has constructed a progressive analysis framework from the genetic basis to the effect of environmental exposure (7), and has deeply analysed the pathogenesis, pathophysiological process and molecular basis of immunotherapy of lung cancer, which has provided a strong support for the scientific formulation of precise treatment strategies.
The aim of this review is to explore recent advances in multi-omics studies of lung cancer and their potential applications in early diagnosis, treatment selection and prognostic assessment. By integrating immunotherapy and multi-omics data in order to better understand the complex molecular network of lung cancer, it provides new ideas and methods for individualised treatment and precision medicine of lung cancer.
2 Lung cancer immunotherapy and genomics
Lung cancer, as a highly heterogeneous disease, has been profoundly influenced by molecular biology in its pathogenesis and therapeutic strategies. In non-small cell lung cancer (NSCLC) and small cell lung cancer (SCLC), unique molecular features of different histological subtypes have been revealed through the identification of specific genetic variants and epigenetic modifications, thus providing new directions for individualised treatment of lung cancer.
In NSCLC, histological subtypes frequently dominated by lung adenocarcinoma (LUAD) and squamous cell carcinoma are common (8). The complexity of NSCLC is reflected in its variable genetic variants. Common target gene driver mutations include genes such as epidermal growth factor receptor (EGFR), KRAS, MET, BRAF, ALK, ROS proto-oncogene 1 (ROS1) and RET (9) (Figure 1A). Through combined whole exome sequencing (WES) technology, it was found that common mutations in LUAD include tumour suppressor genes TP53 (46%), STK11 (17%), KEAP1 (17%), NF1 (11%), RB1 (4%) and CDKN2A (4%), as well as chromatin modification genes SETD2 (9%), ARID1A (7%), SMARCA4 (6%) and RNA splicing genes RBM10 (8%) and U2AF1 (3%) (10). Mutations in the genes FGFR1, NRF2, AKT1 and DDR2 are particularly prominent in lung squamous cell carcinoma (10).
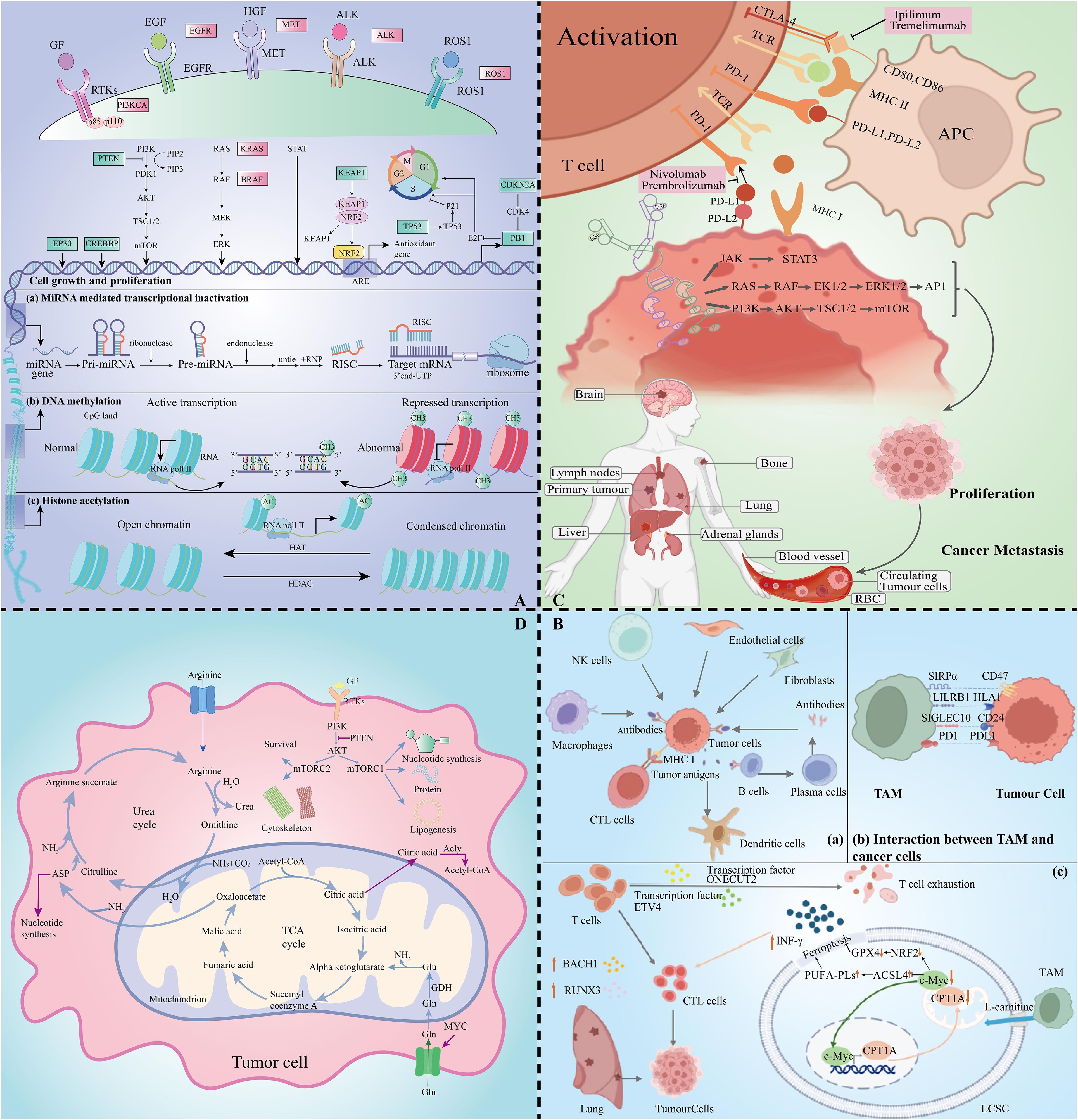
Figure 1. Lung cancer multidimensional histologic analysis map. (A) Exploratory mapping of changes in metabolomic profiles in lung cancer pathogenesis. (B) Immune cell profiles revealed by transcriptomics in the tumour microenvironment. (C) Critical mapping of proteomic changes during lung cancer progression. (D) Resolution of metabolic profiles in lung cancer.
For SCLC, deep sequencing of key oncogenes by advanced integrated mutational profiling (MSK-IMPACT) technology (11) revealed inactivating mutations or deletion of tumour suppressor genes such as TP53, RB1, KMT2D, PTEN, NOTCH1, CREBBP, FAT1, NF1 and APC, and inactivating mutations in PIK3A, EGFR and KRAS activating mutations in oncogenes (12). Unlike NSCLC, SCLC is often accompanied by the expression of MYC oncogenes, which contribute to rapid cell proliferation and lead to DNA replication stress (13). In addition, epigenetic modifications play a key role in lung carcinogenesis, and heritable chromatin modifications such as DNA methylation, histone modifications, and non-coding RNA regulation regulate gene expression without altering the DNA sequence (9, 10, 14–16) (Figure 1A). Epigenetic mutations and disruptions are strongly associated with multiple tumour types, providing new ideas for targeted lung cancer therapy based on molecular subtype differences.
Genomics plays an important role in the classification, treatment and prognostic assessment of lung cancer. Traditionally, lung cancer classification was based on histological patterns, whereas advances in genomics have allowed lung cancer to be characterised also by tumour biomarkers and genetic alterations. For example, Stephen J Murphy et al. defined a common origin or lineage of lung cancer by analysing genomic rearrangements and somatic DNA linkages, and used these specific DNA linkages as precise tumour markers to differentiate between primary and metastatic lung cancer (17).
Genome sequencing technology has revealed key genetic variants in lung cancer, facilitating the development of personalised treatment strategies. The study noted that in non-small cell lung cancer (NSCLC), aberrant activation of the PI3K-AKT-mTOR pathway is closely associated with resistance to EGFR tyrosine kinase inhibitors (EGFR-TKIs), and that its activation is mainly caused by PIK3CA, AKT1 mutations and PTEN deletion. This discovery led to the development of drugs targeting mTOR (e.g., everolimus and temsirolimus) and EGFR-TKIs targeting EGFR and ALK (e.g., ositinib, gefitinib, ceritinib, and loratinib), which have demonstrated clinical efficacy in the treatment of lung cancer (18) (Table 1).
Immune checkpoint inhibitors (ICIs) have become key agents in tumour immunotherapy, especially in the treatment of lung cancer. However, their potential to cause immune-related adverse effects makes the search for biomarkers that predict response to ICI therapy crucial (9). Investigators assessed early predictors of anti-PD-L1 therapy by analysing circulating tumour DNA (ctDNA), and their study showed that a reduction in the frequency of the variant allele was associated with tumour shrinkage after 6 weeks of treatment, providing a valuable non-invasive method for predicting the effectiveness of treatment (44).
3 Lung cancer immunotherapy and transcriptomics
In the field of tumour immunotherapy, targeting the tumour microenvironment (TME) for precision medicine is one of the latest research directions. In this process, immune cells play a key role (45). Immune cell interactions are regulated by transcription factors and further contribute to the immune response. Wu et al. identified interactions between cancer cells and endothelial cells, fibroblasts, and macrophages by single-cell RNA sequencing analysis of NSCLC patient samples, revealing multiple signalling pathways (e.g., EGFR, NOTCH, WNT, and PDGF, etc.) that are associated with carcinogenesis (Figure 1B). These findings shed more light on the molecular interactions and immunoregulatory mechanisms of NSCLC and provide a new perspective on the treatment of lung cancer (46).
In TME, individual cells are precisely regulated by transcription factors. Through the regulation of transcription factors, the killing of cells can be modulated. ONECUT2 and ETV4 were found to be likely potential regulators of CD8 T cell depletion in the blood of NSCLC patients, whereas the transcription factors BACH1 and RUNX3 were up-regulated in CD8 T cytotoxic subpopulations. Thus, regulation of these transcription factors may drive cytotoxic immune responses in NSCLC (47). Immune cell macrophages (TAM) in TME are among the most common immunosuppressive cells. Increased TAM in TME has been associated with immunotherapy resistance by transcriptomic analysis, and its expression is regulated by genes such as CD27, ITGAM and CCL5 (48). Recent studies have shown that TAM interacts with carnitine palmitoyltransferase 1A (CPT1A), increasing resistance to iron death and inactivation of CD8 T cells in lung cancer. Therefore, the use of CPT1 inhibitors enhances the killing of tumour cells by chemotherapy or immunotherapy (49) (Figure 1B).
In the field of lung cancer treatment, transcriptomics is often combined with other histological approaches to extend its application. By combining transcriptomics and metabolomics, the researchers analysed the effects of AZD-6482 (a PI3Kβ-targeted inhibitor) on 28 metabolite-related genes in LUAD. They found that the expression of three genes, LDHA, PPAT, and SMS, was increased in untreated LUAD samples; whereas after treatment with AZD-6482, the expression of these genes was significantly decreased, suggesting that the inhibitor may improve the prognosis of LUAD patients (50). By joining forces with proteomics, Qing et al. used Cancer Cell Line Encyclopaedia (CCLE) RNA sequencing and proteomics profiles in human NSCLC cell lines to identify genes that are pan-sensitive and pan-resistant to drugs used in the treatment of NSCLC with systemic or targeted therapies (51).
4 Lung cancer immunotherapy and proteomics
With the rapid development of mass spectrometry (MS) technology, large-scale protein analysis has become a hotspot in scientific research, in which proteomics has achieved remarkable results in the study of protein phosphorylation, interaction, structure and function (52–55). In particular, proteomics has shown great potential for the discovery of new therapies and biomarkers. These biomarkers come from a wide range of sources, including body fluids and specific samples from lung cancer studies, such as breath condensate (56–59).
In the study of lung cancer proteomics, proteins in receptor tyrosine kinases such as EGFR and ALK and their downstream signalling pathways play a key role in the pathological process of lung cancer (60–62). EGFR, as a member of the ErbB family, promotes malignant cell survival, proliferation, etc. through a series of biochemical processes, making EGFR and its downstream signalling pathway an important target for lung cancer therapy.
In addition, the discovery of immune checkpoints (ICP) has led to a major breakthrough in the field of immunotherapy (63). In normal physiology, ICP maintains immune system homeostasis, but tumour cells evade immune attack by expressing ICP proteins (3). Among them, the interaction of programmed death receptor 1 (PD-1) with programmed cell death ligand-1 (PD-L1), cytotoxic T-lymphocyte-associated protein-4 (CTLA-4) and CD80/86 is the main mechanism of tumour cell escape (Figure 1C). Significant progress has been made in the development of targeted therapeutic agents for lung cancer against these immune checkpoint proteins (64). By blocking the function of these proteins, the immune system in the patient’s body is activated to recognize and attack tumour cells more effectively, bringing new therapeutic hope to lung cancer patients. Over the past decade, tyrosine kinase inhibitors (TKIs) have made significant advances in the treatment of cancer, especially NSCLC.EGFR-TKI, as a potent agent for the treatment of over-activation of EGFR signalling, has been developed for multiple generations with remarkable efficacy (54, 65).
In the development of the field of immunotherapy, it is particularly important to achieve selective destruction of tumours by activating the immune response of T cells (64, 65). PD-1/PD-L1 inhibitors in combination with chemotherapy have become the standard of care in advanced NSCLC (62, 66). In clinical study finds,PD-1 antibodies such as Nivolumab and Pembrolizumab demonstrate durable efficacy in a variety of cancers (67, 68) (Figure 1C). In addition, anti-CTLA-4 antibodies such as Lpilimumab and Tremelimumab play an important role in immunotherapy.
Immunotherapy has great potential in the field of cancer treatment, including checkpoint inhibitors, monoclonal antibodies, and over-the-counter cell transplantation (69). Scholars such as Wang and Chiu emphasized that the combination of multiple therapies is the key to enhancing the effectiveness of cancer treatment and is expected to significantly improve patient survival rates (70, 71).
5 Lung cancer immunotherapy and metabolomics
Metabolomics delves into metabolite changes in organisms, providing new insights into the pathology and drug mechanisms of lung cancer. By analysing lung cancer samples and identifying metabolic markers closely related to lung cancer, it brings new perspectives for early diagnosis, treatment planning and prognosis assessment (72–74).
In the oncogenic transformation of lung cancer cells, there are significant metabolic changes that are particularly dependent on energy sources such as ATP. Among these, the Warburg effect is particularly pronounced in lung cancer cells, where their metabolic needs are met by increased glucose uptake and support nucleotide and amino acid biosynthesis (75). SCLC is significantly dependent on exogenous arginine, which is associated with the deficiency or low expression of arginine succinyl synthase 1 (ASS1) (76, 77). The study found that up to 45% of SCLC samples and 50% of cell lines exhibited ASS1-negativity, highlighting the importance of arginine biosynthesis downregulation in the progression of lung carcinogenesis (78, 79).
The interaction between signalling and metabolism is critical in lung cancer research. mTOR kinases in the PI3K/Akt/mTOR pathway form the mTORC1 and mTORC2 complex, which influences protein, nucleotide, and lipid metabolism (80) (Figure 1D). MYC gene changes affect bioenergetic processes (81). Changes in metabolic state can also inversely regulate signalling pathway activity, e.g., mTORC1 activity is reduced during energy shortage (82).
Developing more effective lung cancer treatment regimens by modulating metabolic pathways or monitoring the disease using metabolic markers. In particular, lung cancer immunotherapy is closely linked to metabolomics, an important cornerstone of lung cancer treatment (83). The markers provided by metabolomics support personalized strategies for immunotherapy and improve treatment efficiency. In addition, the study by Ma et al. revealed the relationship between the regulation of amino acid metabolism, hypoxia-inducible factor-1 (HIF-1) and PI3K-Akt pathways and ositinib resistance, providing new perspectives for understanding the mechanism of drug resistance (84). These studies emphasize the critical role of metabolomics in monitoring marker changes after treatment (85).
For SCLC, polyethylene glycolated arginine deiminase (ADI-PEG20) and human recombinant polyethylene glycolated arginase (e.g., rhArgPEG, BCT-100, etc.) have been regarded as potential therapeutic targets due to arginine nutritional deficiency. And the combination of arginine with PD-1/PD-L1 inhibitors has also demonstrated efficacy in the clinic (76).
The combination of immunotherapy and metabolomics in lung cancer provides patients with more effective and personalized treatment options that are expected to improve their quality of life.
6 Discussion
In recent years, immunotherapies, particularly targeted therapies, have transformed the management and prognosis of lung cancer by providing personalized treatment options for lung cancer patients (86). And the integration of multi-omics data offers the unique advantage of aiming for a comprehensive assessment of each patient through extracted features, which promises a more complete picture of this complex immune ecosystem.
However, although ICI has been widely used in the treatment of lung cancer, there is still a lack of adequate understanding of prognostic biomarkers. We still need to increase research efforts on prognostic biomarkers for lung cancer at the multi-omics level such as proteomics and genomics. For example, genetic mutations in EGFR and KRAS have a key role in individualized therapy, but their specific impact and mechanisms in prognostic assessment need to be further explored (87, 88). In addition, proteomic markers such as CEA and CYFRA 21-1 show potential in disease surveillance, and their correlation with disease progression could provide additional information for disease management (89, 90).
Immune tolerance and therapeutic resistance are also current challenges in the combination of immunology and proteomics for the treatment of lung cancer. In particular, the EGFR T790M mutation leads to resistance to early drug (91–93). Specifically, although the third-generation EGFR-TKI ositinib has successfully treated patients with T790M mutations, new resistance mechanisms such as the EGFR T790M/C797S mutation are still emerging. Currently, there are investigators evaluating fourth-generation EGFR-TKIs clinically for new resistance issues (94, 95).
By integrating these multi-omics data, we can develop a more comprehensive understanding of the biological complexity of lung cancer, leading to the development of more effective therapeutic strategies and improved patient survival (96). Therefore, future research should focus on how to use these biomarkers to optimize treatment pathways, improve the accuracy of prognostic prediction, and ultimately achieve true precision medicine.
Author contributions
YY: Conceptualization, Data curation, Writing – original draft, Writing – review & editing. SS: Data curation, Writing – original draft. JML: Data curation, Writing – original draft. LS: Data curation, Writing – original draft. BW: Validation, Writing – original draft. JZ: Validation, Writing – original draft. JL: Validation, Writing – original draft. HL: Validation, Writing – original draft. PH: Validation, Writing – original draft. KX: Supervision, Writing – original draft. XS: Supervision, Writing – original draft. SH: Supervision, Writing – original draft.
Funding
The author(s) declare financial support was received for the research, authorship, and/or publication of this article. This project is supported by the Doctoral Startup Fund of the Affiliated Hospital of Southwest Medical University (No. 19025), Innovation and Entrepreneurship Training Program of Southwest Medical University (No. 2023393), (Luzhou city- Southwest Medical University) Collaborative Application Foundation Project (No. 2023LZXNYDJ033).
Conflict of interest
The authors declare that the research was conducted in the absence of any commercial or financial relationships that could be construed as a potential conflict of interest.
Publisher’s note
All claims expressed in this article are solely those of the authors and do not necessarily represent those of their affiliated organizations, or those of the publisher, the editors and the reviewers. Any product that may be evaluated in this article, or claim that may be made by its manufacturer, is not guaranteed or endorsed by the publisher.
References
1. Sung H, Ferlay J, Siegel RL, Laversanne M, Soerjomataram I, Jemal A, et al. Global cancer statistics 2020: GLOBOCAN estimates of incidence and mortality worldwide for 36 cancers in 185 countries. CA Cancer J Clin. (2021) 71:209–49. doi: 10.3322/caac.21660
2. Crinò L, Weder W, van Meerbeeck J, Felip E. Early stage and locally advanced (non-metastatic) non-small-cell lung cancer: ESMO Clinical Practice Guidelines for diagnosis, treatment and follow-up. Ann Oncol. (2010) 21 Suppl 5:v103–15. doi: 10.1093/annonc/mdq207
3. Lahiri A, Maji A, Potdar PD, Singh N, Parikh P, Bisht B, et al. Lung cancer immunotherapy: progress, pitfalls, and promises. Mol Cancer. (2023) 22:40. doi: 10.1186/s12943-023-01740-y
4. de Sousa VML, Carvalho L. Heterogeneity in lung cancer. Pathobiology. (2018) 85:96–107. doi: 10.1159/000487440
5. Alyass A, Turcotte M, Meyre D. From big data analysis to personalized medicine for all: challenges and opportunities. BMC Med Genomics. (2015) 8:33. doi: 10.1186/s12920-015-0108-y
6. Ma A, McDermaid A, Xu J, Chang Y, Ma Q. Integrative methods and practical challenges for single-cell multi-omics. Trends Biotechnol. (2020) 38:1007–22. doi: 10.1016/j.tibtech.2020.02.013
7. Mohammadi-Shemirani P, Sood T, Paré G. From ‘Omics to multi-omics technologies: the discovery of novel causal mediators. Curr Atheroscler Rep. (2023) 25:55–65. doi: 10.1007/s11883-022-01078-8
8. Chen P, Liu Y, Wen Y, Zhou C. Non-small cell lung cancer in China. Cancer Commun (Lond). (2022) 42:937–70. doi: 10.1002/cac2.v42.10
9. Abbasian MH, Ardekani AM, Sobhani N, Roudi R. The role of genomics and proteomics in lung cancer early detection and treatment. Cancers (Basel). (2022) 14:5144–60. doi: 10.3390/cancers14205144
10. Saller JJ, Boyle TA. Molecular pathology of lung cancer. Cold Spring Harb Perspect Med. (2022) 12:369–77. doi: 10.1101/cshperspect.a037812
11. MacGregor GA, Markandu ND, Smith SJ, Sagnella GA. Captopril: contrasting effects of adding hydrochlorothiazide, propranolol, or nifedipine. J Cardiovasc Pharmacol. (1985) 7 Suppl 1:S82–7. doi: 10.1097/00005344-198507001-00016
12. Rudin CM, Brambilla E, Faivre-Finn C, Sage J. Small-cell lung cancer. Nat Rev Dis Primers. (2021) 7:3. doi: 10.1038/s41572-020-00235-0
13. Yuan M, Zhao Y, Arkenau H-T, Lao T, Chu L, Xu Q. Signal pathways and precision therapy of small-cell lung cancer. Signal Transduct Target Ther. (2022) 7:187. doi: 10.1038/s41392-022-01013-y
14. Karpinets TV, Foy BD. Tumorigenesis: the adaptation of mammalian cells to sustained stress environment by epigenetic alterations and succeeding matched mutations. Carcinogenesis. (2005) 26:1323–34. doi: 10.1093/carcin/bgi079
15. Ansari J, Shackelford RE, El-Osta H. Epigenetics in non-small cell lung cancer: from basics to therapeutics. Transl Lung Cancer Res. (2016) 5:155–71. doi: 10.21037/tlcr.2016.02.02
16. Bajbouj K, Al-Ali A, Ramakrishnan RK, Saber-Ayad M, Hamid Q. Histone modification in NSCLC: molecular mechanisms and therapeutic targets. Int J Mol Sci. (2021) 22:11701. doi: 10.3390/ijms222111701
17. Murphy SJ, Harris FR, Kosari F, Barreto Siqueira Parrilha Terra S, Nasir A, Johnson SH, et al. Using genomics to differentiate multiple primaries from metastatic lung cancer. J Thorac Oncol. (2019) 14:1567–82. doi: 10.1016/j.jtho.2019.05.008
18. Hamouz M, Hammouz RY, Bajwa MA, Alsayed AW, Orzechowska M, Bednarek AK. A functional genomics review of non-small-cell lung cancer in never smokers. Int J Mol Sci. (2023) 24:13314. doi: 10.3390/ijms241713314
19. Roper N, Brown A-L, Wei JS, Pack S, Trindade C, Kim C, et al. Clonal evolution and heterogeneity of osimertinib acquired resistance mechanisms in EGFR mutant lung cancer. Cell Rep Med. (2020) 1:100007. doi: 10.1016/j.xcrm.2020.100007
20. Liu S-Y, Bao H, Wang Q, Mao W-M, Chen Y, Tong X, et al. Genomic signatures define three subtypes of EGFR-mutant stage II-III non-small-cell lung cancer with distinct adjuvant therapy outcomes. Nat Commun. (2021) 12:6450. doi: 10.1038/s41467-021-26806-7
21. Hou B, Lu X, Gao D-C, Liu Q-X, Zhou D, Zheng H, et al. REPORT- Clinical outcomes of using second - versus first-Generation EGFR-tkis for the First-Line treatment of advanced NSCLC patients with EGFR mutations: A meta-analysis. Pak J Pharm Sci. (2021) 34:1459–68.
22. Ma H, Tian X, Zeng X-T, Zhang Y, Wang Y, Wang F, et al. The efficacy of erlotinib versus conventional chemotherapy for advanced nonsmall-cell lung cancer: A PRISMA-compliant systematic review with meta-regression and meta-analysis. Med (Baltimore). (2016) 95:e2495. doi: 10.1097/MD.0000000000002495
23. Yang JCH, Sequist LV, Geater SL, Tsai C-M, Mok TSK, Schuler M, et al. Clinical activity of afatinib in patients with advanced non-small-cell lung cancer harbouring uncommon EGFR mutations: a combined post-hoc analysis of LUX-Lung 2, LUX-Lung 3, and LUX-Lung 6. Lancet Oncol. (2015) 16:830–8. doi: 10.1016/S1470-2045(15)00026-1
24. Zhou C, Tang K-J, Cho BC, Liu B, Paz-Ares L, Cheng S, et al. Amivantamab plus chemotherapy in NSCLC with EGFR exon 20 insertions. N Engl J Med. (2023) 389:2039–51. doi: 10.1056/NEJMoa2306441
25. Solomon BJ, Bauer TM, Mok TSK, Liu G, Mazieres J, de Marinis F, et al. Efficacy and safety of first-line lorlatinib versus crizotinib in patients with advanced, ALK-positive non-small-cell lung cancer: updated analysis of data from the phase 3, randomised, open-label CROWN study. Lancet Respir Med. (2023) 11:354–66. doi: 10.1016/S2213-2600(22)00437-4
26. Soria J-C, Tan DSW, Chiari R, Wu Y-L, Paz-Ares L, Wolf J, et al. First-line ceritinib versus platinum-based chemotherapy in advanced ALK-rearranged non-small-cell lung cancer (ASCEND-4): a randomised, open-label, phase 3 study. Lancet. (2017) 389:917–29. doi: 10.1016/S0140-6736(17)30123-X
27. Wu Y-L, Dziadziuszko R, Ahn JS, Barlesi F, Nishio M, Lee DH, et al. Alectinib in resected ALK-positive non-small-cell lung cancer. N Engl J Med. (2024) 390:1265–76. doi: 10.1056/NEJMoa2310532
28. Camidge DR, Kim HR, Ahn M-J, Yang JC-H, Han J-Y, Lee J-S, et al. Brigatinib versus crizotinib in ALK-positive non-small-cell lung cancer. N Engl J Med. (2018) 379:2027–39. doi: 10.1056/NEJMoa1810171
29. Solomon BJ, Bauer TM, Ignatius Ou S-H, Liu G, Hayashi H, Bearz A, et al. Post hoc analysis of lorlatinib intracranial efficacy and safety in patients with ALK-positive advanced non-small-cell lung cancer from the phase III CROWN study. J Clin Oncol. (2022) 40:3593–602. doi: 10.1200/JCO.21.02278
30. Drilon A, Rekhtman N, Arcila M, Wang L, Ni A, Albano M, et al. Cabozantinib in patients with advanced RET-rearranged non-small-cell lung cancer: an open-label, single-centre, phase 2, single-arm trial. Lancet Oncol. (2016) 17:1653–60. doi: 10.1016/S1470-2045(16)30562-9
31. Drilon A, Oxnard GR, Tan DSW, Loong HHF, Johnson M, Gainor J, et al. Efficacy of selpercatinib in RET fusion-positive non-small-cell lung cancer. N Engl J Med. (2020) 383:813–24. doi: 10.1056/NEJMoa2005653
32. Gainor JF, Curigliano G, Kim D-W, Lee DH, Besse B, Baik CS, et al. Pralsetinib for RET fusion-positive non-small-cell lung cancer (ARROW): a multi-cohort, open-label, phase 1/2 study. Lancet Oncol. (2021) 22:959–69. doi: 10.1016/S1470-2045(21)00247-3
33. Shaw AT, Ou S-HI, Bang Y-J, Camidge DR, Solomon BJ, Salgia R, et al. Crizotinib in ROS1-rearranged non-small-cell lung cancer. N Engl J Med. (2014) 371:1963–71. doi: 10.1056/NEJMoa1406766
34. Desai AV, Robinson GW, Gauvain K, Basu EM, Macy ME, Maese L, et al. Entrectinib in children and young adults with solid or primary CNS tumors harboring NTRK, ROS1, or ALK aberrations (STARTRK-NG). Neuro Oncol. (2022) 24:1776–89. doi: 10.1093/neuonc/noac087
35. Shaw AT, Solomon BJ, Chiari R, Riely GJ, Besse B, Soo RA, et al. Lorlatinib in advanced ROS1-positive non-small-cell lung cancer: a multicentre, open-label, single-arm, phase 1-2 trial. Lancet Oncol. (2019) 20:1691–701. doi: 10.1016/S1470-2045(19)30655-2
36. Engstrom LD, Aranda R, Lee M, Tovar EA, Essenburg CJ, Madaj Z, et al. Glesatinib exhibits antitumor activity in lung cancer models and patients harboring MET exon 14 mutations and overcomes mutation-mediated resistance to type I MET inhibitors in nonclinical models. Clin Cancer Res. (2017) 23:6661–72. doi: 10.1158/1078-0432.CCR-17-1192
37. Planchard D, Kim TM, Mazieres J, Quoix E, Riely G, Barlesi F, et al. Dabrafenib in patients with BRAF(V600E)-positive advanced non-small-cell lung cancer: a single-arm, multicentre, open-label, phase 2 trial. Lancet Oncol. (2016) 17:642–50. doi: 10.1016/S1470-2045(16)00077-2
38. Bouffet E, Geoerger B, Moertel C, Whitlock JA, Aerts I, Hargrave D, et al. Efficacy and safety of trametinib monotherapy or in combination with dabrafenib in pediatric BRAF V600-mutant low-grade glioma. J Clin Oncol. (2023) 41:664–74. doi: 10.1200/JCO.22.01000
39. Hyman DM, Puzanov I, Subbiah V, Faris JE, Chau I, Blay J-Y, et al. Vemurafenib in multiple nonmelanoma cancers with BRAF V600 mutations. N Engl J Med. (2015) 373:726–36. doi: 10.1056/NEJMoa1502309
40. Skoulidis F, Goldberg ME, Greenawalt DM, Hellmann MD, Awad MM, Gainor JF, et al. STK11/LKB1 mutations and PD-1 inhibitor resistance in KRAS-mutant lung adenocarcinoma. Cancer Discov. (2018) 8:822–35. doi: 10.1158/2159-8290.CD-18-0099
41. Canon J, Rex K, Saiki AY, Mohr C, Cooke K, Bagal D, et al. The clinical KRAS(G12C) inhibitor AMG 510 drives anti-tumour immunity. Nature. (2019) 575:217–23. doi: 10.1038/s41586-019-1694-1
42. Patel JD, Socinski MA, Garon EB, Reynolds CH, Spigel DR, Olsen MR, et al. PointBreak: a randomized phase III study of pemetrexed plus carboplatin and bevacizumab followed by maintenance pemetrexed and bevacizumab versus paclitaxel plus carboplatin and bevacizumab followed by maintenance bevacizumab in patients with stage IIIB or IV nonsquamous non-small-cell lung cancer. J Clin Oncol. (2013) 31:4349–57. doi: 10.1200/JCO.2012.47.9626
43. Garon EB, Ciuleanu T-E, Arrieta O, Prabhash K, Syrigos KN, Goksel T, et al. Ramucirumab plus docetaxel versus placebo plus docetaxel for second-line treatment of stage IV non-small-cell lung cancer after disease progression on platinum-based therapy (REVEL): a multicentre, double-blind, randomised phase 3 trial. Lancet. (2014) 384:665–73. doi: 10.1016/S0140-6736(14)60845-X
44. Raja R, Kuziora M, Brohawn PZ, Higgs BW, Gupta A, Dennis PA, et al. Early reduction in ctDNA predicts survival in patients with lung and bladder cancer treated with durvalumab. Clin Cancer Res. (2018) 24:6212–22. doi: 10.1158/1078-0432.CCR-18-0386
45. Zhang P, Wu X, Wang D, Zhang M, Zhang B, Zhang Z. Unraveling the role of low-density lipoprotein-related genes in lung adenocarcinoma: Insights into tumor microenvironment and clinical prognosis. Environ Toxicol. (2024) 24:6212–22. doi: 10.1002/tox.24230
46. Wu F, Fan J, He Y, Xiong A, Yu J, Li Y, et al. Single-cell profiling of tumor heterogeneity and the microenvironment in advanced non-small cell lung cancer. Nat Commun. (2021) 12:2540. doi: 10.1038/s41467-021-22801-0
47. Zhao Y, Zhang Q, Tu K, Chen Y, Peng Y, Ni Y, et al. Single-cell transcriptomics of immune cells reveal diversity and exhaustion signatures in non-small-cell lung cancer. Front Immunol. (2022) 13:854724. doi: 10.3389/fimmu.2022.854724
48. Larroquette M, Guegan J-P, Besse B, Cousin S, Brunet M, Le Moulec S, et al. Spatial transcriptomics of macrophage infiltration in non-small cell lung cancer reveals determinants of sensitivity and resistance to anti-PD1/PD-L1 antibodies. J Immunother Cancer. (2022) 10:e003890. doi: 10.1136/jitc-2021-003890
49. Ma L, Chen C, Zhao C, Li T, Ma L, Jiang J, et al. Targeting carnitine palmitoyl transferase 1A (CPT1A) induces ferroptosis and synergizes with immunotherapy in lung cancer. Signal Transduct Target Ther. (2024) 9:64. doi: 10.1038/s41392-024-01772-w
50. Thaiparambil J, Dong J, Grimm SL, Perera D, Ambati CSR, Putluri V, et al. Integrative metabolomics and transcriptomics analysis reveals novel therapeutic vulnerabilities in lung cancer. Cancer Med. (2023) 12:584–96. doi: 10.1002/cam4.v12.1
51. Ye Q, Hickey J, Summers K, Falatovich B, Gencheva M, Eubank TD, et al. Multi-omics immune interaction networks in lung cancer tumorigenesis, proliferation, and survival. Int J Mol Sci. (2022) 23:14978. doi: 10.3390/ijms232314978
52. Chen F, Chandrashekar DS, Varambally S, Creighton C.A.-O.X. Pan-cancer molecular subtypes revealed by mass-spectrometry-based proteomic characterization of more than 500 human cancers. Nat Commun. (2019) 10(1):5679. doi: 10.1038/s41467-019-13528-0
53. Hanash S, Taguchi A. Application of proteomics to cancer early detection. Cancer J. (2011) 17(6):423–8. doi: 10.1097/PPO.0b013e3182383cab
54. Moonmuang SA-O, Tantraworasin AA-O, Orrapin S, Udomruk S, Chewaskulyong B, Pruksakorn D, et al. The role of proteomics and phosphoproteomics in the discovery of therapeutic targets and biomarkers in acquired EGFR-TKI-resistant non-small cell lung cancer. Int J Mol Sci. (2023) 24(5):4827. doi: 10.3390/ijms24054827
55. Zhang P, Zhang H, Tang J, Ren Q, Zhang J, Chi H, et al. The integrated single-cell analysis developed an immunogenic cell death signature to predict lung adenocarcinoma prognosis and immunotherapy. Aging (Albany NY). (2023) 15:10305–29. doi: 10.18632/aging.205077
56. Tyers M, Mann M. From genomics to proteomics. Nature. (2003) 422(6928):193–7. doi: 10.1038/nature01510
57. Zhou L, Beuerman RW. The power of tears: how tear proteomics research could revolutionize the clinic. Expert Rev Proteomics. (2017) 14(3):189–91. doi: 10.1080/14789450.2017.1285703
58. Geyer PA-O, Voytik E, Treit PV, Doll S, Kleinhempel A, Niu L, et al. Plasma Proteome Profiling to detect and avoid sample-related biases in biomarker studies. EMBO Mol Med. (2019) 11(11):e10427. doi: 10.15252/emmm.201910427
59. Youssef O, Sarhadi VK, Armengol G, Piirilä P, Knuuttila A, Knuutila S. Exhaled breath condensate as a source of biomarkers for lung carcinomas. A focus on genetic and epigenetic markers-A mini-review. Genes Chromosomes Cancer. (2016) 55(12):905–14. doi: 10.1002/gcc.22399
60. Kumar R, George B, Campbell MR, Verma N, Paul AM, Melo-Alvim C, et al. HER family in cancer progression: From discovery to 2020 and beyond. Adv Cancer Res. (2020) 147:109–60. doi: 10.1016/bs.acr.2020.04.001
61. Holbro T, Hynes NE. ErbB receptors: directing key signaling networks throughout life. Annu Rev Pharmacol Toxicol. (2004) 44:195–217. doi: 10.1146/annurev.pharmtox.44.101802.121440
62. Sharma SV, Bell DW, Settleman J, Settleman DA, Haber DA. Epidermal growth factor receptor mutations in lung cancer. Nat Rev Cancer. (2007) 7(3):169–81. doi: 10.1038/nrc2088
63. Bagchi S, Yuan R, Engleman EG. Immune checkpoint inhibitors for the treatment of cancer: clinical impact and mechanisms of response and resistance. Annu Rev Pathol. (2021) 16:223–49. doi: 10.1146/annurev-pathol-042020-042741
64. Powles T, Durán I, van der Heijden MS, Loriot Y, Vogelzang NJ, De Giorgi U, et al. Atezolizumab versus chemotherapy in patients with platinum-treated locally advanced or metastatic urothelial carcinoma (IMvigor211): a multicentre, open-label, phase 3 randomised controlled trial. Lancet. (2018) 391(10122):748–57. doi: 10.1016/S0140-6736(17)33297-X
65. Huang L, Jiang S, Shi YA-O. Tyrosine kinase inhibitors for solid tumors in the past 20 years (2001-2020). J Hematol Oncol. (2020) 13(1):143. doi: 10.1186/s13045-020-00977-0
66. Seegobin K, Majeed U, Wiest N, Manochakian R, Lou Y, Zhao Y. Immunotherapy in non-small cell lung cancer with actionable mutations other than EGFR. Front Oncol. (2021) 11:750657. doi: 10.3389/fonc.2021.750657
67. Antonia SJ, López-Martin JA, Bendell J, Ott PA, Taylor M, Eder JP, et al. Nivolumab alone and nivolumab plus ipilimumab in recurrent small-cell lung cancer (CheckMate 032): a multicentre, open-label, phase 1/2 trial. Lancet Oncol. (2016) 17(7):883–95. doi: 10.1016/S1470-2045(16)30098-5
68. Patnaik A, Kang SP, Rasco D, Papadopoulos KP, Elassaiss-Schaap J, Beeram M, et al. Phase I study of pembrolizumab (MK-3475; anti-PD-1 monoclonal antibody) in patients with advanced solid tumors. Clin Cancer Res. (2015) 21(19):4286–93. doi: 10.1158/1078-0432.CCR-14-2607
69. Kelly RJ, Giaccone G. Lung cancer vaccines. Cancer J. (2011) 17(5):302–8. doi: 10.1097/PPO.0b013e318233e6b4
70. Wang J, Zou ZH, Xia HL, He JX, Zhong NS, Tao AL. Strengths and weaknesses of immunotherapy for advanced non-small-cell lung cancer: a meta-analysis of 12 randomized controlled trials. PLOS ONE. (2012) 7(3):e32695. doi: 10.1371/journal.pone.0032695
71. Chiu M, Armstrong EJL, Jennings V, Foo S, Crespo-Rodriguez E, Bozhanova G, et al. Combination therapy with oncolytic viruses and immune checkpoint inhibitors. Expert Opin Biol Ther. (2020) 20(6):635–52. doi: 10.1080/14712598.2020.1729351
72. Moreno P, Jiménez-Jiménez C, Garrido-Rodríguez M, Calderón-Santiago M, Molina S, Lara-Chica M, et al. Metabolomic profiling of human lung tumor tissues - nucleotide metabolism as a candidate for therapeutic interventions and biomarkers. Mol Oncol. (2018) 12(10):1778–96. doi: 10.1002/1878-0261.12369
73. Tonorezos ES, Jones LW. Energy balance and metabolism after cancer treatment. Semin Oncol. (2013) 40(6):745–56. doi: 10.1053/j.seminoncol.2013.09.011
74. Aoyagi T, Terracina KP, Raza A, Matsubara H, Takabe K. Cancer cachexia, mechanism and treatment. World J Gastrointest Oncol. (2015) 7(4):17–29. doi: 10.4251/wjgo.v7.i4.17
75. Nomura M, Morita M, Tanuma N. A metabolic vulnerability of small-cell lung cancer. Oncotarget. (2018) 9(64):32278–9. doi: 10.18632/oncotarget.25964
76. Carpentier JA-O, Pavlyk I, Mukherjee U, Hall PE, Szlosarek PW. Arginine deprivation in SCLC: mechanisms and perspectives for therapy. Lung Cancer: Targets Ther. (2022) 13:53–66. doi: 10.2147/LCTT.S335117
77. Hall PE, Ready N, Johnston A, Bomalaski JS, Venhaus RR, Sheaff M, et al. Phase II study of arginine deprivation therapy with pegargiminase in patients with relapsed sensitive or refractory small-cell lung cancer. Clin Lung Cancer. (2020) 21(6):527–33. doi: 10.1016/j.cllc.2020.07.012
78. Szlosarek PW, Grimshaw MJ, Wilbanks GD, Hagemann T, Wilson JL, Burke F, et al. Aberrant regulation of argininosuccinate synthetase by TNF-alpha in human epithelial ovarian cancer. Int J Cancer. (2007) 121(1):6–11. doi: 10.1002/ijc.22666
79. Long Y, Tsai WB, Wangpaichitr M, Tsukamoto T, Savaraj N, Feun LG, et al. Arginine deiminase resistance in melanoma cells is associated with metabolic reprogramming, glucose dependence, and glutamine addiction. Mol Cancer Ther. (2013) 12(11):2581–90. doi: 10.1158/1535-7163.MCT-13-0302
80. Saxton RA, Sabatini DM. mTOR signaling in growth, metabolism, and disease. Cell. (2017) 169(2):361–71. doi: 10.1016/j.cell.2017.02.004
81. Stine ZE, Walton ZE, Altman BJ, Hsieh AL, Dang CV. MYC, metabolism, and cancer. Cancer Discov. (2015) 5(10):1024–39. doi: 10.1158/2159-8290.CD-15-0507
82. Krencz I, Sebestyen A, Khoor A. mTOR in lung neoplasms. Pathol Oncol Res. (2020) 26(1):35–48. doi: 10.1007/s12253-020-00796-1
83. Zhang PA-OX, Pei S, Zhou G, Zhang M, Zhang L, Zhang ZA-O. Purine metabolism in lung adenocarcinoma: A single-cell analysis revealing prognostic and immunotherapeutic insights. J Cell Mol Med. (2024) 28(8):e18284. doi: 10.1111/jcmm.18284
84. Ma QA-O, Wang J, Ren Y, Meng F, Zeng L. Pathological mechanistic studies of osimertinib resistance in non-small-cell lung cancer cells using an integrative metabolomics-proteomics analysis. J Oncol. (2020) 2020:6249829. doi: 10.1155/2020/6249829
85. Pan X, Chen W, Nie M, Liu Y, Xiao Z, Zhang Y, et al. A serum metabolomic study reveals changes in metabolites during the treatment of lung cancer-bearing mice with anlotinib. Cancer Manag Res. (2021) 13:6055–63. doi: 10.2147/CMAR.S300897
86. Zhang L, Cui Y, Mei J, Zhang Z, Zhang P.A.-O.X. Exploring cellular diversity in lung adenocarcinoma epithelium: Advancing prognostic methods and immunotherapeutic strategies. Cell Prolif. (2024) 30:e13703. doi: 10.1111/cpr.13703
87. Wang H, Yang X, Sun Y, Li Y, Dong Y, Shan D, et al. Synergistic effect of metformin and EGFR-TKI in the treatment of non-small cell lung cancer. Transl Cancer Res. (2020) 9(1):372–81. doi: 10.21037/tcr.2019.11.09
88. Reck M, Carbone DP, Garassino M, Barlesi F. Targeting KRAS in non-small-cell lung cancer: recent progress and new approaches. Ann Oncol. (2021) 32(9):1101–10. doi: 10.1016/j.annonc.2021.06.001
89. Cao WA-O, Tang Q, Zeng J, Jin X, Zu L, Xu S. A review of biomarkers and their clinical impact in resected early-stage non-small-cell lung cancer. Cancers. (2023) 15(18):4561. doi: 10.3390/cancers15184561
90. Nijhawan HP, Prabhakar B, Misra A, Yadav KS. Fragmented antibodies in non-small cell lung cancer: A novel nano-engineered delivery system for detection and treatment of cancer. Drug Discov Today. (2023) 28(11):103701. doi: 10.1016/j.drudis.2023.103701
91. Oxnard GR, Arcila ME, Sima CS, Riely GJ, Chmielecki J, Kris MG, et al. Acquired resistance to EGFR tyrosine kinase inhibitors in EGFR-mutant lung cancer: distinct natural history of patients with tumors harboring the T790M mutation. Clin Cancer Res. (2011) 17(6):1616-. doi: 10.1158/1078-0432.CCR-10-2692
92. Wang ZF, Ren SX, Li W, Gao GH. Frequency of the acquired resistant mutation T790 M in non-small cell lung cancer patients with active exon 19Del and exon 21 L858R: a systematic review and meta-analysis. BMC Cancer. (2018) 18(1):148. doi: 10.1186/s12885-018-4075-5
93. Yu HA, Arcila ME, Rekhtman N, Sima CS, Zakowski MF, Pao W, et al. Analysis of tumor specimens at the time of acquired resistance to EGFR-TKI therapy in 155 patients with EGFR-mutant lung cancers. Clin Cancer Res. (2013) 19(8):2240–7. doi: 10.1158/1078-0432.CCR-12-2246
94. Rangachari D, To C, Shpilsky JE, VanderLaan PA, Kobayashi SS, Mushajiang M, et al. EGFR-Mutated Lung Cancers Resistant to Osimertinib through EGFR C797S Respond to First-Generation Reversible EGFR Inhibitors but Eventually Acquire EGFR T790M/C797S in Preclinical Models and Clinical Samples. J Thoracic Oncol. (2019) 14(11):1995–2002. doi: 10.1016/j.jtho.2019.07.016
95. Tripathi SK, Biswal BK. Allosteric mutant-selective fourth-generation EGFR inhibitors as an efficient combination therapeutic in the treatment of non-small cell lung carcinoma. Drug Discov Today. (2021) 26(6):1466–72. doi: 10.1016/j.drudis.2021.02.005
Keywords: lung cancer, immunotherapy, precision medicine, multi-omics, individualised therapy, immune checkpoints
Citation: Yan Y, Shen S, Li J, Su L, Wang B, Zhang J, Lu J, Luo H, Han P, Xu K, Shen X and Huang S (2024) Cross-omics strategies and personalised options for lung cancer immunotherapy. Front. Immunol. 15:1471409. doi: 10.3389/fimmu.2024.1471409
Received: 27 July 2024; Accepted: 30 August 2024;
Published: 25 September 2024.
Edited by:
Wenyi Jin, City University of Hong Kong, Hong Kong SAR, ChinaReviewed by:
Qianhe Ren, Jiangsu University, ChinaCopyright © 2024 Yan, Shen, Li, Su, Wang, Zhang, Lu, Luo, Han, Xu, Shen and Huang. This is an open-access article distributed under the terms of the Creative Commons Attribution License (CC BY). The use, distribution or reproduction in other forums is permitted, provided the original author(s) and the copyright owner(s) are credited and that the original publication in this journal is cited, in accordance with accepted academic practice. No use, distribution or reproduction is permitted which does not comply with these terms.
*Correspondence: Ke Xu, Y3FnaHh1a2VAY3F1LmVkdS5jbg==; Xiang Shen, c2hlbnhpYW5nZG9jdG9yQDE2My5jb20=; Shangke Huang, aHVhbmdzaGFuZ2tlMDAxQHN3bXUuZWR1LmNu
†These authors have contributed equally to this work and share first authorship