- 1MOE Medical Basic Research Innovation Center for Gut Microbiota and Chronic Diseases, Wuxi School of Medicine, Jiangnan University, Wuxi, China
- 2Macao Centre for Research and Development in Chinese Medicine, State Key Laboratory of Quality Research in Chinese Medicine, Institute of Chinese Medical Sciences, University of Macau, Macao, Macao SAR, China
Prostate adenocarcinoma (PRAD) is a prevalent global malignancy which depends more on lipid metabolism for tumor progression compared to other cancer types. Although Stearoyl-coenzyme A desaturase (SCD) is documented to regulate lipid metabolism in multiple cancers, landscape analysis of its implications in PRAD are still missing at present. Here, we conducted an analysis of diverse cancer datasets revealing elevated SCD expression in the PRAD cohort at both mRNA and protein levels. Interestingly, the elevated expression was associated with SCD promoter hypermethylation and genetic alterations, notably the L134V mutation. Integration of comprehensive tumor immunological and genomic data revealed a robust positive correlation between SCD expression levels and the abundance of CD8+ T cells and macrophages. Further analyses identified significant associations between SCD expression and various immune markers in tumor microenvironment. Single-cell transcriptomic profiling unveiled differential SCD expression patterns across distinct cell types within the prostate tumor microenvironment. The Gene Ontology and Kyoto Encyclopedia of Genes and Genome analyses showed that SCD enriched pathways were primarily related to lipid biosynthesis, cholesterol biosynthesis, endoplasmic reticulum membrane functions, and various metabolic pathways. Gene Set Enrichment Analysis highlighted the involvement of elevated SCD expression in crucial cellular processes, including the cell cycle and biosynthesis of cofactors pathways. In functional studies, SCD overexpression promoted the proliferation, metastasis and invasion of prostate cancer cells, whereas downregulation inhibits these processes. This study provides comprehensive insights into the multifaceted roles of SCD in PRAD pathogenesis, underscoring its potential as both a therapeutic target and prognostic biomarker.
1 Introduction
Prostate adenocarcinoma (PRAD) is the second most common cancer among men globally, and the fifth leading cause of cancer-related mortality (1). In China, the situation is also concerning with the rising incidence of prostate cancer (2). Although there have been advancements in surgical techniques, radiotherapy, and newer therapies such as focal ultrasound ablation, androgen deprivation therapy (ADT) remains the cornerstone of standard prostate cancer (PRAD) treatment (3). As aging progresses, PRAD can develop rapidly and advanced PRAD poses significant risks of metastasis, drug resistance, and transformation into castration-resistant prostate cancer. This underscores the paramount importance of timely intervention (4).
PRAD exhibits a unique ‘heterogeneity’ in its lipid metabolism. This characteristic is twofold: firstly, PRAD relies more on lipid oxidation as its primary energy source compared to other tumors, accompanied by a relatively low rate of glucose uptake (5). Secondly, as tumors progress malignantly, there is a notable increase in the activity of fatty acid (FA) synthesis in PRAD cells, highlighted by the elevated expression of key enzymes involved in fatty acid synthesis (6). Epidemiological studies have revealed that conditions such as obesity, metabolic syndrome, diabetes, and systemic metabolic disorders, as well as a high-fat diet, are associated with an elevated risk of PRAD and a decreased survival rate among PRAD patients (7). Consequently, targeting the key processes and enzymes involved in lipid metabolism emerges as a potential strategy for the treatment of malignant PRAD.
Stearoyl-coenzyme A desaturase (SCD, also known as SCD1 or FADS5) is a key rate-limiting enzyme localized in the endoplasmic reticulum (ER) membrane. It catalyzes the desaturation of fatty acids and plays a crucial role in the de novo lipogenesis (8). Notably, SCD facilitates the conversion of saturated fatty acids (SFA) to monounsaturated fatty acids (MUFA). Specifically, it converts palmitic acid (PA, C16:0) into palmitoleic acid (POA, C16:1), and stearic acid (SA, C18:0) into oleic acid (OA, C18:1) (9). The unsaturated lipids serve as lipid pools for β-oxidation and as components of the cell membrane, which are indispensable for tumor progression (10). This is demonstrated by the significant overexpression of SCD in various types of cancer (11–14). Therefore, inhibiting SCD functions has been proposed as a therapeutic strategy for some types of cancer (15–18). Despite reports suggesting SCD as a therapeutic target, a comprehensive understanding of its implications in PRAD has not yet been realized.
The tumor microenvironment (TME) consists of immune cells, stromal cells, and the extracellular matrix. Within this complex network, two categories of immune cells emerge as pivotal players: tumor-infiltrating lymphocytes (TILs) and macrophages. Among TILs, CD8+ T cells are central to orchestrating anti-tumor immunity by recognizing and eliminating tumor cells presenting tumor-associated antigens. However, their cytotoxic function is often suppressed by tumor-induced immune checkpoints, such as programmed death-1 (PD-1) and cytotoxic T-lymphocyte-associated protein 4 (CTLA-4) (19–22). Macrophages, another crucial component of the TME, exhibit plasticity by differentiating into either M1 (anti-tumorigenic) or M2 (tumor-promoting) phenotypes. M1 macrophages possess strong phagocytic and immunostimulatory properties that contribute to anti-tumor immunity, while M2 macrophages create an inflammatory and immunosuppressive environment that promotes tumor progression (23, 24). Therapeutic strategies that target immune checkpoints or modulate macrophage polarization to enhance anti-tumor immune responses can improve outcomes for cancer patients.
In this study, we employed bioinformatics approaches to analyze public datasets encompassing clinicopathological data, promoter methylation levels, genetic alterations, immune infiltrations, single-cell transcriptomic data, and pathway enrichment analysis. Additionally, we conducted functional experiments to validate our computational findings. Our findings demonstrated that SCD overexpression is correlated with PRAD progression, as well as the changes in the tumor immune microenvironment.
2 Materials and methods
2.1 UALCAN
The University of Alabama at Birmingham cancer data analysis portal (UALCAN, http://ualcan.path.uab.edu/) is a comprehensive web portal for analyzing cancer omics data (25). In this study, it was used to analyze the difference of SCD expression between tumor tissue and normal tissue, and the expression of SCD in PRAD was analyzed based on patients’ gender, age, race, tumor protein P53 (TP53) mutation status, lymph node metastasis status, or a molecular signature. The expression level of SCD was normalized as transcript per million reads. P < 0.05 was considered statistically significant. The abbreviations for the cancers were illustrated in Supplementary Table S1.
2.2 GEPIA
The Gene Expression Profiling Interactive Analysis (GEPIA) database (http://gepia.cancer-pku.cn/) consists of RNA sequencing expression data of 9,736 tumors and 8,587 normal samples derived from The Cancer Genome Atlas (TCGA) and the Genotype-Tissue Expression (GTEx) databases (26). It was used to analyze the differential mRNA expression of SCD between PRAD, colon adenocarcinoma (COAD), liver hepatocellular carcinoma (LIHC), or uterine corpus endometrial carcinoma (UCEC) tissues and their corresponding normal tissues.
2.3 The human protein atlas
The Human Protein Atlas (HPA, https://www.proteinatlas.org/) provides comprehensive data on human proteins in cells and tissues using various omics techniques (27). The database consists of 26,941 antibody proteome data for 17,165 unique proteins. PRAD and adjacent tissue sections were immunostained with SCD antibodies (Sigma-Aldrich, HPA012107, Rabbit).
2.4 Immunohistochemistry
The tissue microarray (TMA) containing PRAD, related urological tumors, and adjacent normal tissues was purchased from Outdo Biotech (Shanghai, China). The TMA (HUrSC060PT01) contained 60 tissue samples, including 36 tumor samples. SCD expression in the patient-derived normal and tumor samples was analyzed by immunohistochemistry (IHC). The IHC staining was performed using a SCD-specific antibody included in the IHCeasy SCD Ready-To-Use IHC kit (Proteintech; Wuhan, China). The experiments were carried out according to the manufacturer’s instructions. The IHC images were captured with the Pannoramic MIDI II Digital Slide Scanners (3DHISTECH Ltd., Budapest, Hungary). H-score was used to evaluate the IHC results, the principle of which was to use ImageJ software (Rawak Software Inc, Stuttgart, Germany) to analyze automatically the intensity and proportion of immunostaining. Grading scale was defined in a semiquantitative manner, as follows: 0, Negative; 1+, Low Positive; 2+, Positive; and 3+, High Positive. The formula was used to calculate H-score: H-score = (percentage of 1+ cells × 1) + (percentage of 2+ cells × 2) + (percentage of 3+ cells × 3).
2.5 cBioPortal
The cBio Cancer Genomics Portal (cBioPortal, http://www.cbioportal.org/) provides data for more than 5,000 tumor samples from 20 cancer studies (28). The alteration frequency of SCD mutations in the genomic profiles was used to calculate Pearson’s correlation coefficients across pan-cancer, along with the data on missense mutation sites in PRAD.
2.6 CancerSEA
CancerSEA (http://biocc.hrbmu.edu.cn/CancerSEA/) was created to decode Pearson correlations between 14 different functional states and relevant genes in human malignancies, containing gene sequencing profiles of 41,900 cancer single cells (29). In this study, we used CancerSEA to investigate the relationships between the SCD genes and the afore mentioned PRAD functional states at the single-cell level.
2.7 TIMER
Tumor immune estimation resource (TIMER, https://cistrome.shinyapps.io/timer/) is a database for comprehensive analysis of immune infiltrates across diverse cancer types (30), which consists of 10,897 samples from 32 cancer types. It enables the evaluation of the correlation between the transcriptional level of SCD and the abundances of six immune infiltrates (B cells, CD4+ T cells, CD8+ T cells, neutrophils, macrophages and dendritic cells), as well as correlations between transcriptional level of SCD and immune cell markers. The gene expression level was assessed using log2 TPM.
2.8 GeneMANIA
The GeneMANIA database (http://www.genemania.org/) is a web-based tool for generating hypotheses about gene function, analyzing gene lists, and prioritizing genes for functional testing (31). It was used to explore the 20 genes that are associated with SCD, which have physical interactions, co-expression, predicted gene-gene relationships, pathway relationships, co-localization, genetic interactions, and shared protein domains.
2.9 TISCH
Tumor immune single-cell hub (TISCH, http://tisch.comp-genomics.org/) is a single-cell RNA sequencing database focusing on the tumor microenvironment (TME) (32), which provides detailed cell-type annotation at the single-cell level, consisting of 6,297,320 cells and 190 datasets. It was used to analyze SCD expression in PRAD datasets, including PRAD_GSE137829, PRAD_GSE141445, PRAD_GSE150692 and PRAD_GSE172301.
2.10 Related-gene enrichment analysis
The Gene Ontology (GO, http://www.geneontology.org/) database and Kyoto Encyclopedia of Genes and Genome (KEGG, http://www.genome.jp/kegg/) database were employed for the enrichment analysis of SCD and its 20 related genes. They were performed with the Database for Annotation, Visualization and Integrated Discovery (DAVID, https://david.ncifcrf.gov/) (33), focusing on the key phenotypes and signal pathways.
2.11 GSEA analysis
The Gene Set Enrichment Analysis (GSEA) analysis was implemented to further investigate the potential mechanisms of SCD behind the initiation and progression of PRAD (34). Samples in the TCGA-PRAD dataset were classified into two groups (high-expression group and low-expression group) based on the average expression of SCD. A false discovery rate (FDR) < 0.05 and P < 0.05 were considered as criteria for significantly enriched gene sets.
2.12 Cell culture
Two PRAD cell lines, PC3 and LNCaP cells, were obtained from the National Collection of Authenticated Cell Cultures (Shanghai, China). Both of the cells were cultured in 1640 (Sigma, St. Louis, MO, USA) with 10% fetal bovine serum (HyClone, Logan, Utah, USA), 100 U/ml penicillin and 100 mg/ml streptomycin. Cells were maintained at 37°C in a humidified environment in an incubator with 5% CO2.
2.13 Small interfering RNA
Small interfering (si) RNAs used in this study were purchased from Geenchem (Shanghai, China). The siRNA sequences were as follows: negative control (NC) (Forward: 5’-UUCUCCGAACGUGUCACGUdTdT-3’; Reverse: 5’-ACGUGACACGUUCGGAGAAdTdT-3’), and si-SCD (Forward: 5’-GCACAUCAACUUCACCACATT-3’; Reverse: 5’-UGUGGUGAAGUUGAUGUGCTT-3’). Lipofectamine 8000 from Beyotime (Shanghai, China) was used to transfect siRNA into PC3 and LNCaP cells according to the manufacturer’s instructions.
2.14 Lentiviral overexpression
Lentivirus transduction was applied to establish SCD overexpression cell lines. SCD-inserted lentiviral vector was obtained from Hanbio Biotech (Shanghai, China), and SCD was transduced into PC3 and LNCaP cells according to the manufacturer’s instructions. In short, the cells were seeded in a 12-well plate, incubated overnight, and then infected for 24 hours. The SCD-overexpression vector carries the green fluorescent protein (GFP) reporter gene, flag-tagged SCD gene and the puromycin resistance gene. Transduction efficiency was analyzed with a fluorescence microscope. SCD overexpression in PC3 and LNCaP cell lines was confirmed by Western blot with a SCD-specific antibody.
2.15 Western blot
PC3 and LNCaP cells with siRNA or lentivirus transfection were lysed with a cell lysis buffer containing protease inhibitors (Beyotime, Shanghai, China). Protein concentrations were determined by using the BCA Protein Assay Kit (BIO - RAD, California, USA). Protein samples were loaded for electrophoresis on SDS–PAGE and transferred to PVDF membranes (BIO - RAD, California, USA). After blocking with 5% non - fat milk, the membranes were treated with primary antibodies (1:1000 dilution) and then secondary antibodies (1:3000 dilution). All antibodies were purchased from Cell Signaling Technology (Beverly, MA, USA). Finally, protein membrane was treated with an enhanced chemiluminescence detection buffer, and protein bands were captured with the ChemiDOC MP (BIO - RAD, California, USA).
2.16 Cell viability
Cell proliferation was analyzed by 3 - (4,5 - dimetrylthiazol) - 2,5 - diphenyltetrazolium bromide (MTT) assay. Exponentially growing cells with siRNA or lentivirus transfection were seeded into 96 - well plates. MF438 was purchased from MedChem Express (New Jersey, USA). MTT was added to each well and the plates were incubated for an additional 4h. The formazan crystal was dissolved by adding 100 μl DMSO. The light absorbance value was measured at 490 nm using a BioTek Synergy H4 all-in-one microplate reader (Vermont, USA).
2.17 Migration assay
PC3 and LNCaP cells with siRNA or lentivirus transfection were inoculated in a 6-well plate and cultured overnight. When the cells fused to a monolayer, a sterile suction head with the same width was used to make a “+” scratch. The cells were washed with PBS and incubated for certain time periods. The plate wells were captured with a microscope and the scratch widths were analyzed with the ImageJ software at different time points.
2.18 Invasion assay
24-well Transwell chambers with 8-µm membrane filters (Corning Incorporated) were precoated with Matrigel (Yeasen Biotech Co, Ltd, Shanghai, China). The cells were seeded into the upper chamber in serum-free medium, whereas the lower chamber was filled with complete medium containing 10% FBS. Following incubation for 24 h at 37˚C, cells on the lower surface were fixed with 4% paraformaldehyde for 10 min and stained with 0.1% crystal violet for 10 min at room temperature. The stained cells were photographed and quantified.
2.19 Statistical analysis
All in vitro experiments were repeated at least three times, and the results were presented as the mean ± standard deviation (SD). Student’s t-test was used to analyze the significance of the differences. Data were analyzed with GraphPad Prism 7.0 (GraphPad Software, San Diego, CA, USA). P < 0.05 was considered statistically significant.
3 Results
3.1 Pan-cancer analysis of SCD expression at the mRNA and protein level
Pan-cancer analysis of SCD transcriptional expression was conducted using the UALCAN platform based on TCGA data. It was revealed that SCD expression was upregulated in 24 types of tumors compared to adjacent normal tissues, including PRAD and several other cancer types (Figure 1A). Re-examination using an alternative online tool, GEPIA, confirmed these findings, indicating an upregulation of SCD expression in PRAD and other cancers (Figure 1B). Compared to other tumors, PRAD relies more heavily on lipid oxidation as its primary energy source. Increasing evidence suggests that the lipid synthesis pathway in PRAD is highly activated with the upregulated expression of SCD. Therefore, we focused on PRAD to analyze the role of SCD in cancer pathogenesis.
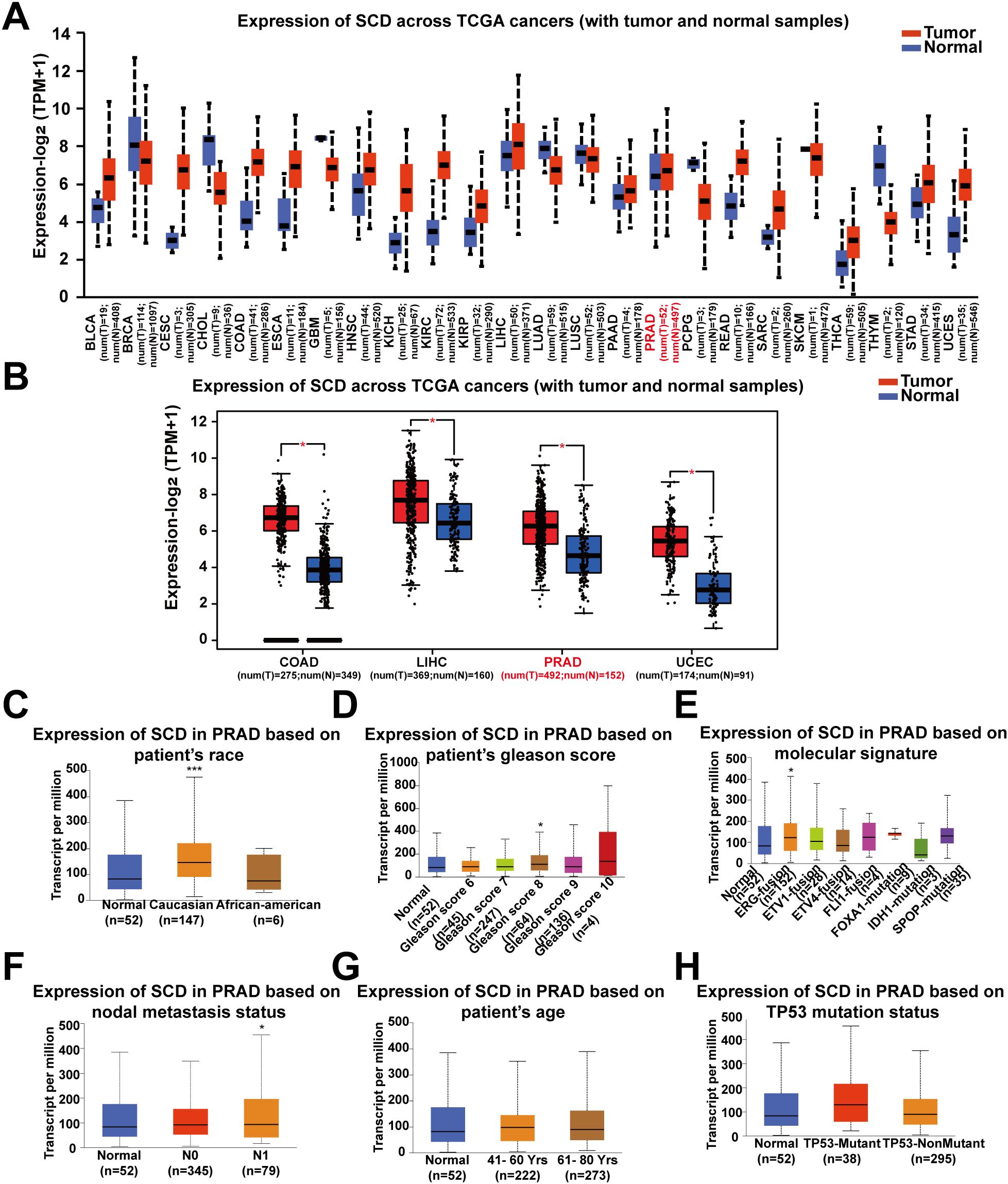
Figure 1. Pan-cancer analyses of the upregulated SCD expression at the mRNA level. (A) SCD expression in the UALCAN database. The mRNA expression between tumors and adjacent normal tissues in 24 types of cancers was analyzed. (B) SCD expression in the GEPIA database. The mRNA expression between COAD, LIHC, PRAD and UCEC cancers and normal tissues was analyzed and matched TCGA normal and GTEx data. Four-way analysis of variance (ANOVA), P< 0.01 and |log2FC| > 1 are considered differentially expressed genes. SCD mRNA expression in PRAD subtypes with different clinicopathological characteristics, including patients’ race (C), Gleason score (D), molecular signature (E), nodal metastasis status (F), age (G) and TP53 mutation status (H) using the UALCAN database. Multiple groups were compared using one-way ANOVA followed by Bonferroni’s multiple comparisons for every two groups. *P <0.05; ***P <0.001.
We next determined the correlation of SCD transcriptional expression levels with various clinicopathological characteristics in PRAD samples using UALCAN analysis. The results demonstrated elevated SCD expression in specific subgroups of PRAD patients, including those of Caucasian race (Figure 1C), those with a Gleason score of 8 (Figure 1D), those displaying the ERG-fusion molecular signature (Figure 1E), and those with N1 nodal metastasis status (Figure 1F). In terms of patient’s age and TP53 mutation status, there was also a trend indicating that higher SCD expression was associated with older age (Figure 1G) and TP53 mutation (Figure 1H). These findings suggest that SCD holds potential as a diagnostic tumor marker for PRAD and highlight the importance of considering different clinical parameters for a comprehensive understanding of its role in PRAD.
Furthermore, the translational expression level of SCD in pan-cancer was investigated on the HPA platform. The protein expression level of SCD in colorectal cancer, breast cancer, prostate cancer was higher than those in the adjacent normal tissues (Supplementary Figures S1A, B). Quantification of the online data revealed that over 50% of cancer cases exhibited medium to high expression levels of SCD (Supplementary Figure S1C). To validate the online data, SCD expression in PRAD and related urological tumors was analyzed using a tissue microarray (TMA) by IHC. In patient-derived tissues, SCD expression was upregulated in many urological cancers, including PRAD and renal cancer, compared to adjacent normal tissues (Figures 2A, B; Supplementary Figure S2). It is worth noting that SCD expression increased as the Gleason scores of PRAD increased (Figure 2A). Quantification of our IHC data confirmed that the protein expression level of SCD was upregulated in PRAD (Figure 2C).
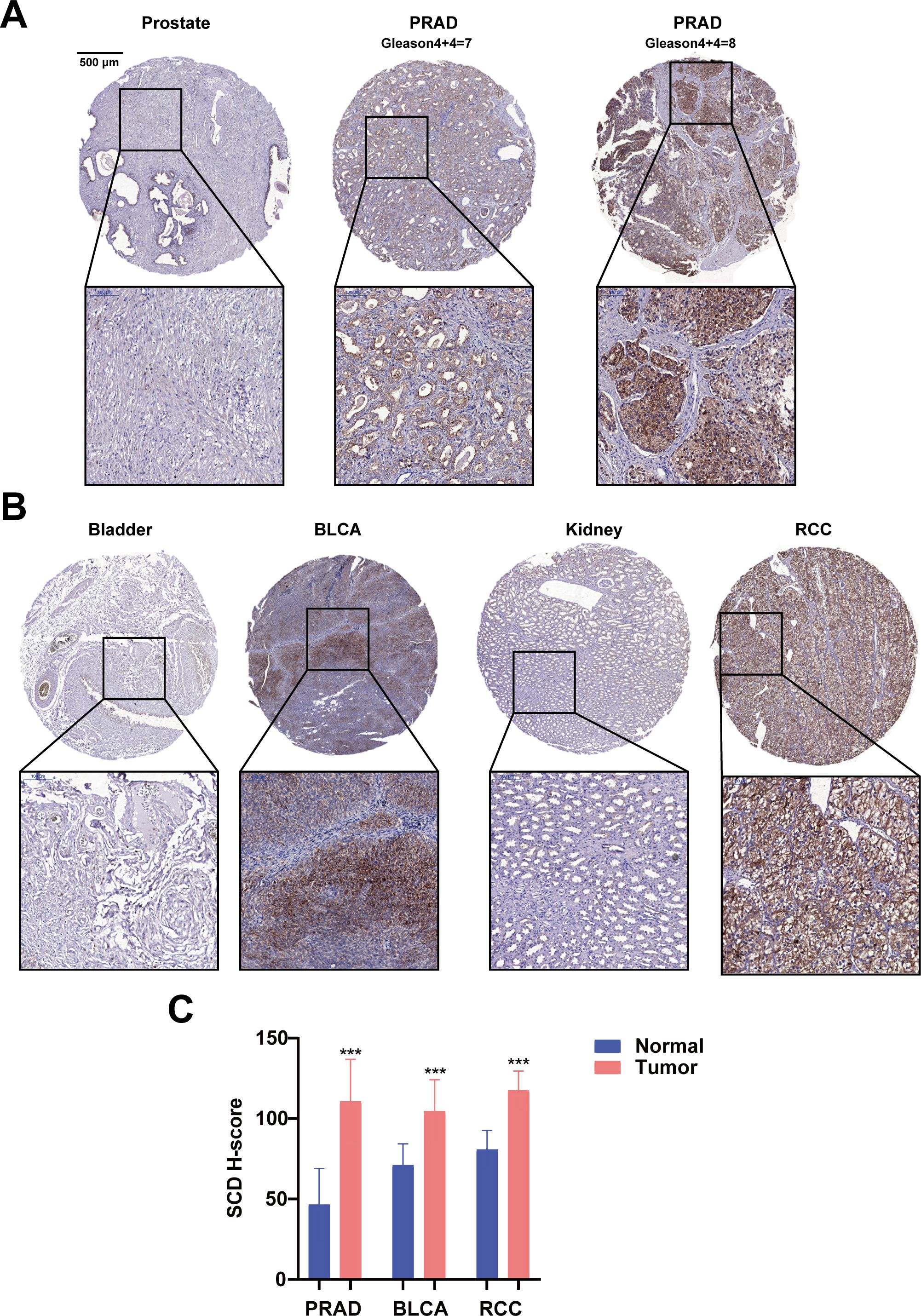
Figure 2. Immunohistochemical analyses of SCD protein expression in PRAD and related urological cancers. (A) SCD expression in normal prostate and PRAD. Gleason scores of PRAD were indicated. (B) SCD expression in related urological cancers. Bladder urothelial carcinoma (BLCA) and Renal cell carcinoma (RCC) were compared with the respective adjacent normal tissues. (C) Quantification of SCD IHC staining intensities. The protein levels of SCD were analyzed with the IHC intensities based on the histoscore algorithm (H-score). ***P < 0.001.
3.2 DNA methylation and genetic alterations related with clinicopathological characteristics
Abnormal DNA methylation is closely related to the occurrence and development of cancer. Therefore, we performed UALCAN analysis using TCGA-PRAD samples to compare the promoter methylation levels of SCD. The results suggested that promoter methylation levels of SCD were elevated in patients aged 41-80 years, especially 61-80 years (Figure 3A), in Caucasian and African-American races (Figure 3B), in those with N0 nodal metastasis status (Figure 3C) and in patients with non-mutant TP53 (Figure 3D). Despite the traditional perception of DNA methylation as a transcriptional silencing mechanism, emerging research indicates that high promoter methylation levels in malignant tumors are associated with elevated gene expression (35, 36). The most frequently postulated mechanism posits that the hypermethylation of promoter DNA obstructs the binding of known inhibitory transcription factors, ultimately fostering active gene transcription (37).
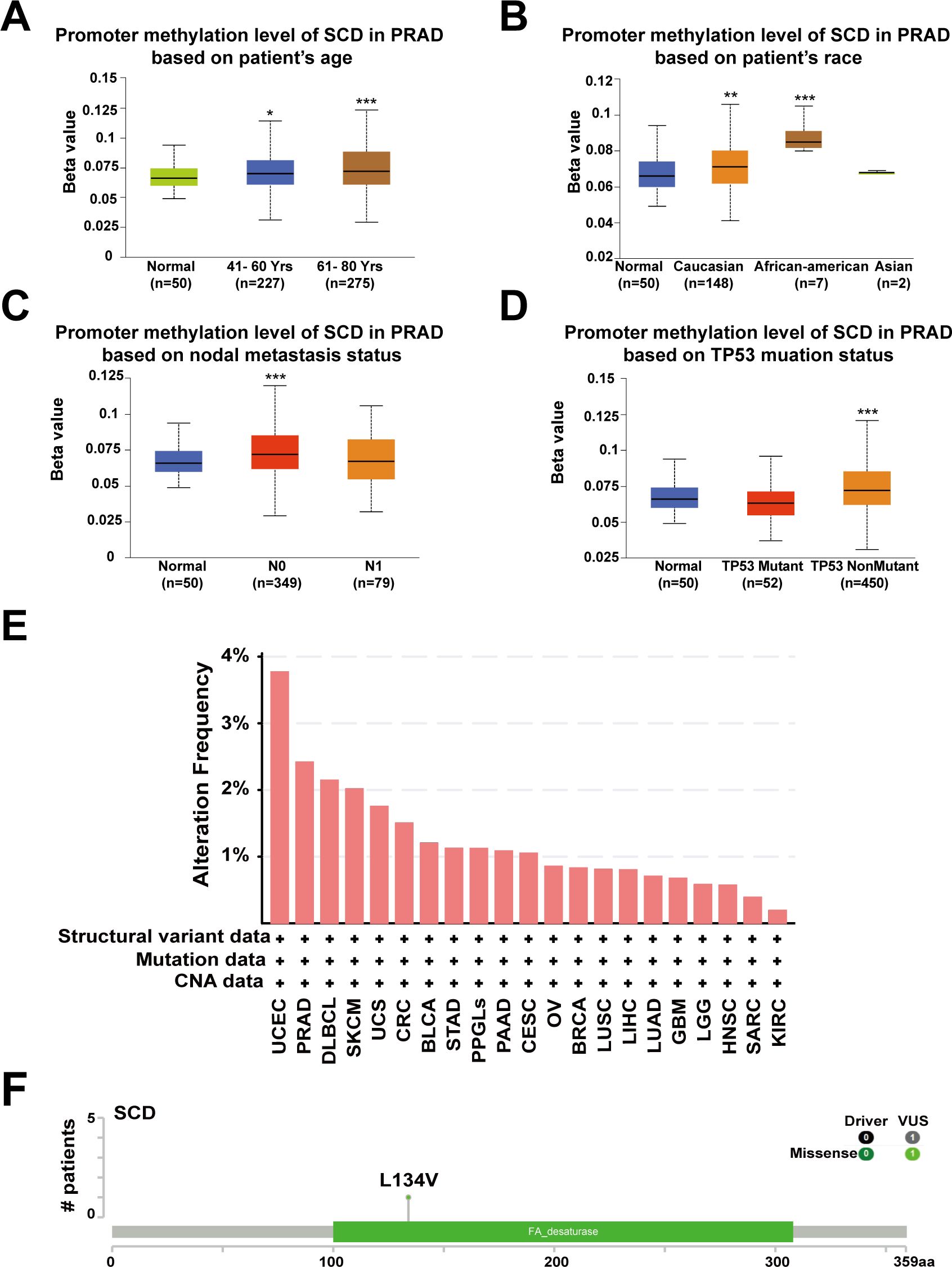
Figure 3. Promoter methylation and genetic alterations of the SCD gene. Box plots evaluating SCD promoter methylation levels in PRAD among different groups of patients based on clinical parameters including patients’ age (A), race (B), nodal metastasis status (C), TP53 mutation status (D) using the UALCAN database. Multiple groups were compared using one-way ANOVA followed by Bonferroni’s multiple comparisons for every two groups. (E) Alterations summary of SCD in different tumors. (F) The most common SCD genetic mutation in PRAD analyzed by the cBioPortal database. *P <0.05; **P <0.01; ***P <0.001.
Furthermore, we investigated the genetic alteration characteristics of SCD in the TCGA cohort through the cBioPortal network. According to the results, the frequency of SCD alteration in PRAD patients was 2.43% in 494 cases (Figure 3E). Particularly, mutations in the FA desaturase domain containing the SCD active site, with L134V (Leucine converts to Valine) being the most common locus, were observed in PRAD (Figure 3F). Both the original (Leu) and the substituted (Val) residues are non-polar with hydrophobic side chains and have only a slight difference. Therefore, we speculate that the L134V mutation may increase PRAD risk by enhancing SCD function. However, further clinical patient data is required to confirm this relationship. These findings revealed that the abnormal expression of SCD in specific cancers may stem from genetic alterations and promoter hypermethylation, providing a deeper understanding of PRAD tumorigenesis and guiding potential treatment strategy.
3.3 Immune cell infiltration and TME correlated with SCD expression
The association between SCD expression and the infiltration of immune cells was evaluated across TCGA-PRAD using the TIMER tool. The correlation between SCD expression and markers of various immune cells, including B cells, T cells, monocytes, macrophages, neutrophils, natural killer cells, and dendritic cells, was analyzed (Supplementary Table S2). While some immune cells, such as CD8+ T cells and macrophages, were positively associated with SCD expression, a greater number of immune cells showed a negative correlation (Figure 4A; Supplementary Table S2). Additionally, the correlation between SCD expression and immune checkpoints was assessed (Table 1). Notably, the activity of immunosuppressive molecules like LAG3, LGALS9, PD-L1(PDCD1), and CTLA4 was negatively correlated with SCD expression, whereas immune-activating molecules such as TNFRSF18, TNFSF4, and CD47 were positively correlated. This suggests that the promotive effects of SCD expression on PRAD progression may lead to the activation of immune cells in the tumor microenvironment.
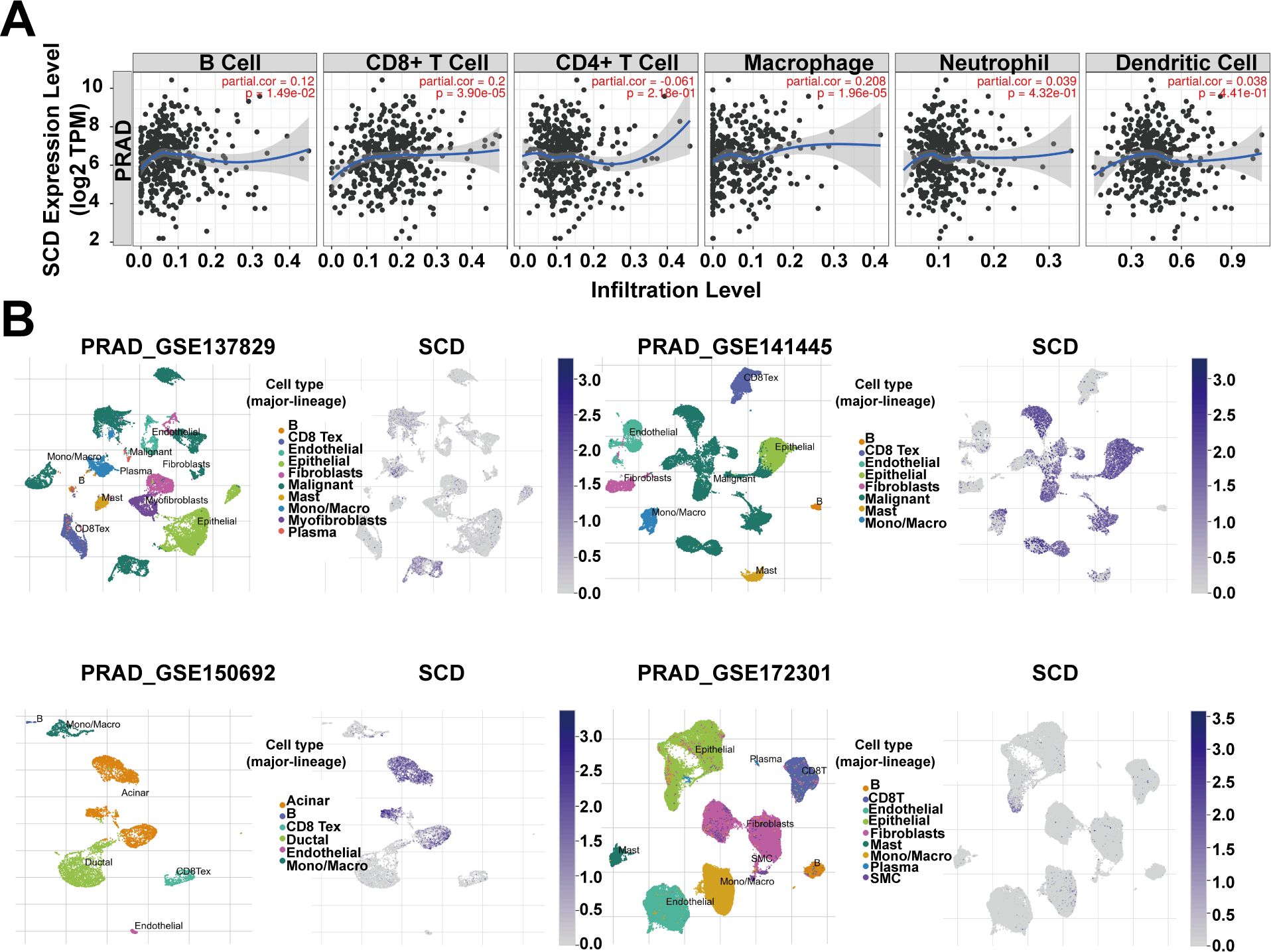
Figure 4. SCD expression in tumor microenvironment. (A) Relationship between the SCD expression and immune cell infiltration in PRAD using the TIMER tool. Spearman’s correlation was used to perform this association analysis. (B) SCD expression at single-cell resolution in different PRAD datasets from TISCH database.
In addition, four datasets (PRAD_GSE137829, PRAD_GSE141445, PRAD_GSE150692, and PRAD_GSE172301) from the TISCH database were utilized to explore SCD expression in the TME. The results revealed that malignant cells, mono/macro cells, epithelial cells, and acinar cells exhibited higher levels of SCD expression compared to other cell types (Figure 4B). These findings suggest that SCD is highly expressed not only in malignant cells but also in immune and stromal cells. In summary, SCD shows a broad expression pattern across various cell types within the TME, highlighting its significant immunomodulatory potential.
3.4 Gene association network analysis unveiling potential SCD roles in PRAD
In order to explore the biological functions of SCD, gene association analysis was conducted with the GeneMANIA and 20 SCD-related genes were identified (Figure 5A). The related genes were mainly involved in regulating sterol biosynthesis, steroid biosynthesis, and lipid biosynthesis processes according to physical interactions, co-expression, prediction and co-localization (Supplementary Table S3). Similar correlations were shown in Figure 5B from TIMER database at the pan-cancer level. The top six genes in the relevance ranking were identified, including isopentenyl-diphosphate delta-isomerase 1 (IDI1), 3-hydroxy-3-methylglutaryl-coenzyme A synthase 1 (HMGCS1), farnesyl diphosphate synthase (FDPS), farnesyl-diphosphate farnesyltransferase 1 (FDFT1), 7-dehydrocholesterol reductase (DHCR7), squalene epoxidase (SQLE). Scatter plots showing the expression of these genes, along with SCD, are presented in Figure 5C.
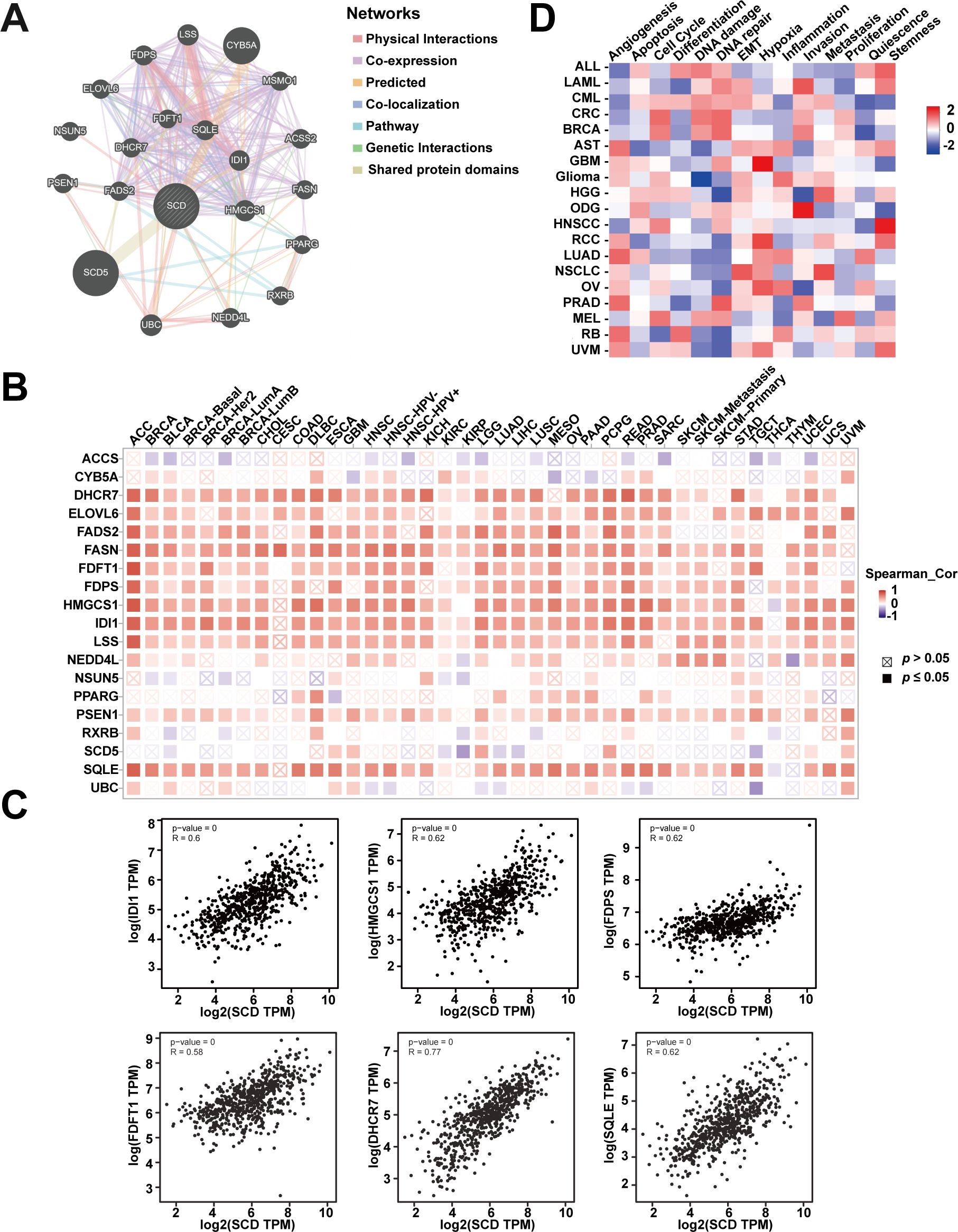
Figure 5. Analyses of SCD-related genes. (A) SCD-related gene network analyzed in the GeneMANIA database. (B) The expression of SCD-related genes in different cancers. (C) Scatter plots of correlation analyses of the expression of SCD and SCD-related genes. Spearman’s correlation was used to perform this association analysis. (D) Correlations between SCD and 14 functional states in different cancers.
In addition, single-cell transcriptome sequencing is a key technique for analyzing the potential functions of candidate molecules at the single-cell level. Using the CancerSEA, we investigated the potential functions of SCD at single cell levels in various cancers. The results highlighted a noteworthy correlation between SCD expression and inflammation in pan-cancer (Figure 5D). Moreover, SCD was also positively correlated with epithelial-mesenchymal transition (EMT) and hypoxia, suggesting that SCD is a key factor in promoting cancer malignancy.
3.5 Functional enrichment analysis of SCD relationships with cancer cell fate
To further explore the functions of SCD based on the 20 identified SCD-related genes, we performed GO and KEGG analyses, which are essential tools in functional genomics. GO focuses on classifying and describing gene functions across three main categories—molecular function, biological process, and cellular component. KEGG analysis is primarily used to explore how genes interact within biological pathways, such as those involved in metabolic processes, signal transduction, and disease mechanisms. Our GO analysis revealed that SCD was mainly enriched in biological processes, including lipid biosynthesis and metabolism, cholesterol biosynthesis, steroid biosynthesis, and endoplasmic reticulum membrane functions (Figure 6A). Our KEGG analysis revealed major enrichment in metabolic processes, including lipid biosynthesis, cholesterol biosynthesis, endoplasmic reticulum membrane functions (Figure 6B).
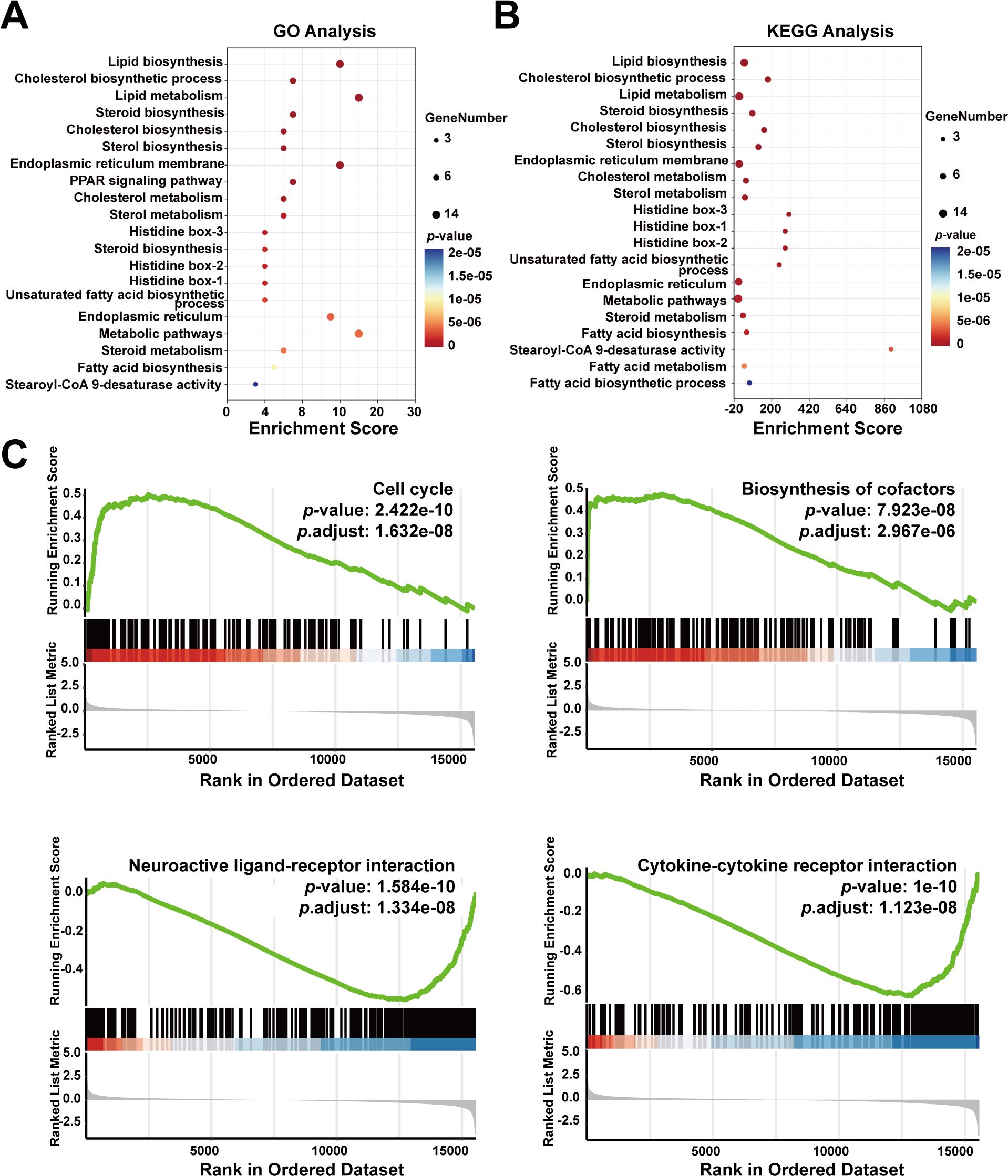
Figure 6. Enrichment analysis of SCD-related genes in cancer functional states. (A) GO enrichment analysis of SCD-related genes. (B) KEGG enrichment analysis of SCD-related genes. The size of the bubble represented the number of enriched genes, and the color correlated with the p-value. (C) Functional enrichment analyses of SCD in PRAD by GSEA.
To explore SCD roles in cellular events, the GSEA tool was used, which revealed a positive correlation between high SCD expression and the cell cycle (P = 1.632e-08) as well as biosynthesis of cofactors (P = 2.967e-06) (Figure 6C). Conversely, SCD expression was negatively correlated with neuroactive ligand-receptor interaction (P = 1.334e-08) and cytokine-cytokine receptor interaction (P = 1.123e-08) (Figure 6C). This suggested potential alterations in the immune microenvironment that may facilitate immune escape and drug resistance.
3.6 SCD regulation of PRAD progression in functional assays
The bioinformatic analyses suggested SCD plays important roles in the pathogenesis and progression of PRAD. To validate SCD roles directly, functional studies with SCD overexpression and knockdown were conducted in PRAD cell lines. Two PRAD cell lines, PC3 and LNCaP, were treated with siRNA and/or lentivirus to knock down or overexpress SCD (Figure 7A). In MTT assays, siRNA knockdown of SCD led to a significant decrease in cell viability, while its overexpression through lentivirus transfection resulted in increased viability in both of the cell lines (Figure 7B). Additionally, treatment of both cell lines with the SCD inhibitor MF438 resulted in a concentration-dependent inhibition of cell viability (Figure 7C). These findings demonstrate the pivotal role of SCD protein in promoting PRAD cell proliferation.
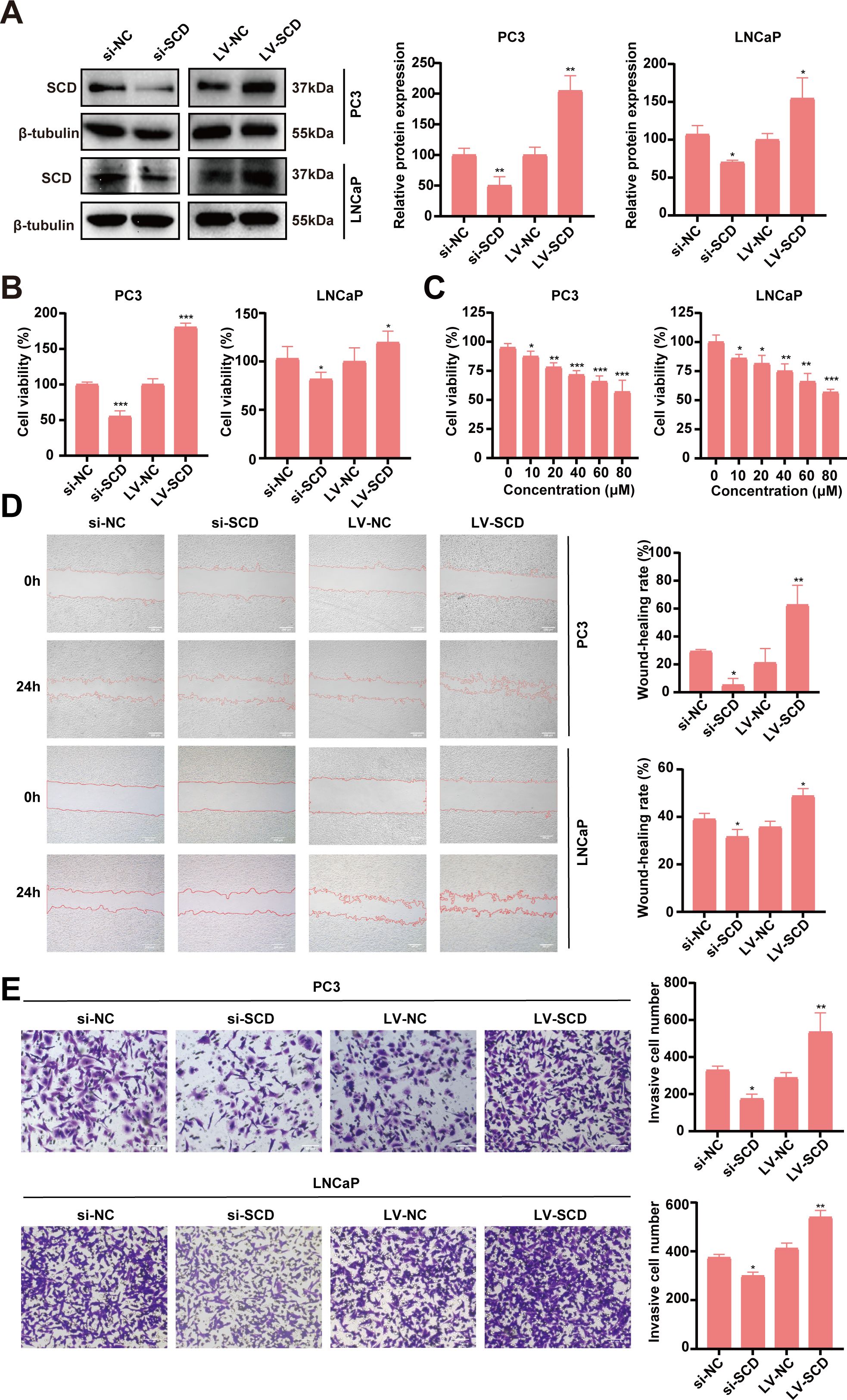
Figure 7. Validation of SCD roles in PRAD in vitro. (A) SCD knockdown or overexpression in PC3 cells or LNCaP cells was conducted with siRNA (si) or SCD-loaded lentivirus (LV-SCD). SCD expression was analyzed by Western blot, with β-tubulin as a control. Quantification of protein levels were shown in the right. (B) The viability of PRAD cells was affected by SCD knockdown or overexpression in MTT assays. (C) SCD inhibitor, MF438, dose-dependently suppressed the viability of PRAD cells. SCD expression levels affected the migration (D) and the invasion (E) of PRAD cells. Representative images from three independent experiments are shown. Quantification of migration and invasion were conducted with the ImageJ software. Data are presented as the mean ± SD (n = 3). Student’s t-test was used to analyze the significance of the differences. *P <0.05; **P <0.01; ***P <0.001.
The impact of SCD on PRAD cell migration and invasion was further investigated using PC3 and LNCaP cell lines. In the scratch assays for analyzing cell migration, SCD knockdown significantly reduced the migration capacity of both PRAD cell lines compared to the negative control (si-NC) group, as shown in Figure 7D. In contrast, overexpression of SCD substantially enhanced migration ability relative to the control group (Figure 7D). Similarly, in the transwell invasion assays, SCD knockdown markedly decreased the invasion ability of both PRAD cell lines compared to the si-NC group (Figure 7E). Conversely, overexpression of SCD significantly boosted invasion capability compared to the control group (Figure 7E). These findings collectively suggest that SCD plays a crucial role in regulating PRAD aggressiveness, underscoring its potential as a therapeutic target for PRAD treatment.
4 Discussion
In this study, the impact of SCD gene expression and the functional roles of the SCD protein in prostate adenocarcinoma (PRAD) were comprehensively analyzed using bioinformatic tools and functional experiments. Our findings revealed that the SCD gene is highly expressed in PRAD patients, and the SCD protein promotes the proliferation, invasion, and migration of PRAD tumor cells. Our research aligns with other studies that have identified a significant correlation between high SCD expression and an unfavorable prognosis in various tumor types (38–40). SCD has been extensively studied for its role in promoting tumor initiation, progression, metastasis, and stemness (41). Collectively, these findings suggest that SCD could serve as both a biomarker and a therapeutic target for PRAD patients.
We have revealed that SCD is notably overexpressed in Caucasian patients, as well as in patients with Gleason score 8, stage N1 lymph node metastasis, or ERG fusion. Racial disparities in prostate cancer incidence are largely rooted in inherent genetic factors that are non-modifiable, underscoring the necessity of targeted screening in specific populations for early detection (42). Early detection facilitates prompt treatment, which can begin even during adolescence, and is crucial for improving outcomes (43, 44). The Gleason scoring system is a widely accepted method for evaluating the malignancy of prostate cancer, based on the differentiation degree of cancer cells and pathophysiological changes in prostate tissues. A score of 8 indicates poor differentiation and categorizes the cancer as high-risk (45). Lymph node metastasis, indicated by stage N1, signifies the progression of cancer cells through lymphatic vessels, leading to tumor spread and often necessitating immediate intervention through surgery, chemotherapy, radiotherapy, or targeted therapy to manage symptoms such as swelling, skin depression, and local redness (46). The most common molecular alteration in prostate cancer is the fusion of ERG and TMPRSS2, a widespread event associated with poor prognosis (47, 48). In summary, effective management of prostate cancer requires a holistic approach that considers individual patient characteristics and disease progression, with early detection, accurate diagnosis, and personalized treatment plans being essential for enhancing prognosis.
Interestingly, the elevated SCD expression in PRAD was accompanied by promoter hypermethylation and genetic mutations. Although DNA methylation represses gene transcription in many other circumstances, promoter hypermethylation is usually associated with enhanced gene expression in malignant tumors (35, 36, 49). This is explained by that promoter hypermethylation obstructs the binding of known inhibitory transcription factors, ultimately fostering gene transcription (37). Gene mutations and methylation play crucial roles in tumor development (50, 51). Specifically, gene mutations alter the function of critical genes, driving tumor progression. Meanwhile, methylation regulates gene expression and cellular function, thereby influencing tumor development. These two mechanisms are intricately intertwined and work synergistically to promote tumor formation and advancement. An in-depth study of these two mechanisms is crucial for the advancement of novel tumor diagnostic methodologies and therapeutic strategies (51). Among various cancer types, the mutation frequency of SCD in prostate cancer patients stands at 2.43%, making it the second highest. This provides a clue for us to find SCD mutations, that is, L134V was observed as the most common locus in PRAD. It has been documented that the substitution of leucine (Leu) for valine (Val) within the amino acid sequence of a protein leads to significant alterations in its three-dimensional structure. These modifications can have profound effects on the protein’s activity, stability, and its ability to interact with other molecules, ultimately establishing a correlation with a wide spectrum of diseases (52, 53). It’s reported that mutations in the type II steroid 5 alpha-reductase (SRD5A2) gene, which replaces valine with leucine at codon 89, reduced the risk of prostate cancer in Italian patients (54).
This study showed that SCD expression exhibits a negative correlation with the expression of various immune cell markers, suggesting a reduction in immune cell infiltration in PRAD. This reduction likely contributes to a tumor-friendly immune microenvironment, facilitating PRAD progression. However, SCD expression was positively associated with the infiltration of CD8+ T cells and macrophages in the TCGA-PRAD datasets. CD8+ T cells a are known to enhance the clearance of tumor cells, and M1 macrophages secrete pro-inflammatory factors and cytotoxic molecules, effectively targeting and eliminating tumor cells. Moreover, SCD expression was negatively correlated with immune checkpoints such as PD-L1 and CTLA4. Therefore, it should not be concluded that SCD expression enhances the anti-tumor effects of immune cells. Instead, the enhancement of certain immune responses may be accompanied by PRAD progression, which is the consequence of SCD expression. Currently, numerous emerging technologies and materials have shown promising outcomes by specifically targeting the TME in immunotherapy. These include biological materials such as lipid nanoparticles, organic biomaterials, and metal oxide nanomaterials, which can reprogram the TME, thereby enhancing the efficacy of immunotherapy and strengthening the anti-tumor effect (55, 56). Additionally, chimeric antigen receptor (CAR) T cell therapy, a novel precision-targeted approach, has demonstrated remarkable success in treating hematological malignancies (57). Immune checkpoint inhibitors (ICI), another crucial therapeutic modality, have the potential to cure cancer by modulating the immune system and reducing drug resistance (58). Given these advancements, SCD presents considerable therapeutic potential within the realm of immunotherapy. Its application could be further explored and optimized through combination therapies or the innovative use of biological materials in future research and development.
Lipid metabolism plays a critical role in tumor development. Several drugs targeting lipid metabolism have been developed for the treatment of prostate cancer, including statins, proprotein convertase subtilisin/kexin type 9 (PCSK9) inhibitors, and sterol regulatory element-binding protein (SREBP) inhibitors (59–61). Lipids not only serves as a source of energy but also maintains redox homeostasis for cancer cells. Cancer cells have a tendency to synthesize lipids de novo and the desaturation process of fatty acids is of particular significance in lipid synthesis. Our study has revealed a strong correlation between the expression of SCD and the expression of IDI1, HMGCS1, FDPS, FDFT1, DHCR7, and SQLE genes in the pathogenesis of PRAD. These genes play a pivotal role in the biosynthesis of bioactive substances, notably cholesterol, and are extensively implicated in tumor lipid metabolism. They are consistently overexpressed in a wide range of cancers, indicating a potential synergistic contribution to the promotion of tumor initiation and development, particularly in malignancies involving cholesterol metabolism (62–69). The specific mechanism by which targeting lipid desaturation may combat cancer includes reducing membrane fluidity to inhibit metastasis, increasing the lipid toxicity of saturated fatty acids, and decreasing the polarization of tumor stem cells and the secretion of factors such as interleukin-6 and nitric oxide by mesenchymal stem cells (70–72). Further research is required to fully elucidate the roles of these lipid metabolism-associated genes in cancer pathogenesis and to explore their potential as therapeutic targets.
The enrichment analyses further investigate the biological functions and pathways that SCD may be implicated in PRAD. Interestingly, we observed a negative correlation between SCD expression and cytokine-cytokine receptor interaction, a process closely linked to immune activity. Signaling between cytokines and cytokine receptors is essential for the production, survival, and homeostasis of immune cells, and for generating immune responses in response to external stimuli (73). Cytokines play a pivotal role in the pathogenesis and treatment of cancer (74). A reduction in cytokine-cytokine receptor interaction may alter the TME, potentially enabling tumors to evade immune surveillance and develop treatment resistance. Combined with bioinformatic analyses, our functional studies clearly indicate that targeting SCD is a promising strategy for combating PRAD, as has been extensively reported in cases of other cancers (75–77). For example, T-3764518, a novel oral small molecule inhibitor of SCD, has demonstrated efficacy in inhibiting the growth of HCT-116 cell xenografts in mouse models of colorectal cancer (78). The combination of SCD inhibitor A939572 with Tisirolimus synergistically inhibits the growth of clear cell renal cell carcinoma both in vitro and in vivo (79). In addition, SCD has potential as a prognostic marker in various cancers and may aid in overcoming drug resistance (80, 81). Therefore, SCD emerges as a potential target for cancer therapy, exhibiting robust anti-cancer effects in both in vivo and in vitro models, and showing promise in the realm of overcoming drug resistance.
In conclusion, this study systematically explored the roles of SCD in PRAD through bioinformatic and experimental analyses. The gene and protein expression of SCD were significantly upregulated in PRAD. Importantly, this upregulation was associated with specific clinicopathological features and the TME, highlighting its potential as a biomarker and therapeutic target for PRAD. These findings are significant for understanding PRAD pathogenesis and provide insights for targeted interventions in this aggressive cancer.
Data availability statement
The original contributions presented in the study are included in the article/Supplementary Material. Further inquiries can be directed to the corresponding author.
Author contributions
JW: Writing – original draft, Software, Formal analysis. LY: Writing – original draft, Investigation, Data curation. HX: Writing – original draft, Validation. D-RZ: Writing – original draft, Methodology. Y-XW: Writing – original draft, Methodology. H-LC: Writing – original draft, Software. Z-FZ: Writing – original draft, Funding acquisition. G-SW: Writing – original draft, Resources, Project administration, Conceptualization. Y-JG: Writing – review & editing, Supervision, Conceptualization.
Funding
The author(s) declare financial support was received for the research, authorship, and/or publication of this article. This work was supported by the National Science Foundation for Young Scientists of China (No. 82202910, 81603158), the Wuxi Science and Technology Development Fund Project (No. N20202012), the Fundamental Research Funds for the Central Universities (No. JUSRP123072), Macao Science and Technology Development Fund (001/2023/ALC and 0006/2020/AKP).
Conflict of interest
The authors declare that the research was conducted in the absence of any commercial or financial relationships that could be construed as a potential conflict of interest.
Publisher’s note
All claims expressed in this article are solely those of the authors and do not necessarily represent those of their affiliated organizations, or those of the publisher, the editors and the reviewers. Any product that may be evaluated in this article, or claim that may be made by its manufacturer, is not guaranteed or endorsed by the publisher.
Supplementary material
The Supplementary Material for this article can be found online at: https://www.frontiersin.org/articles/10.3389/fimmu.2024.1460915/full#supplementary-material
References
1. Sung H, Ferlay J, Siegel RL, Laversanne M, Soerjomataram I, Jemal A, et al. Global cancer statistics 2020: GLOBOCAN estimates of incidence and mortality worldwide for 36 cancers in 185 countries. CA Cancer J Clin. (2021) 71:209–49. doi: 10.3322/caac.21660
2. Health Commission Of The People’s Republic Of China N. National guidelines for diagnosis and treatment of prostate cancer 2022 in China (English version). Chin J Cancer Res. (2022) 34:270–88. doi: 10.21147/j.issn.1000-9604.2022.03.07
3. Feng D, Li D, Xiao Y, Wu R, Wang J, Zhang C. Focal ablation therapy presents promising results for selectively localized prostate cancer patients. Chin J Cancer Res. (2023) 35:424–30. doi: 10.21147/j.issn.1000-9604.2023.04.08
4. Shen W, He J, Hou T, Si J, Chen S. Common pathogenetic mechanisms underlying aging and tumor and means of interventions. Aging Dis. (2022) 13:1063–91. doi: 10.14336/AD.2021.1208
5. Bader DA, McGuire SE. Tumour metabolism and its unique properties in prostate adenocarcinoma. Nat Rev Urol. (2020) 17:214–31. doi: 10.1038/s41585-020-0288-x
6. Pardo JC, Ruiz de Porras V, Gil J, Font A, Puig-Domingo M, Jordà M. Lipid metabolism and epigenetics crosstalk in prostate cancer. Nutrients. (2022) 14:851. doi: 10.3390/nu14040851
7. Zadra G, Photopoulos C, Loda M. The fat side of prostate cancer. Biochim Biophys Acta. (2013) 1831:1518–32. doi: 10.1016/j.bbalip.2013.03.010
8. Bai Y, McCoy JG, Levin EJ, Sobrado P, Rajashankar KR, Fox BG, et al. X-ray structure of a mammalian stearoyl-CoA desaturase. Nature. (2015) 524:252–6. doi: 10.1038/nature14549
9. Enoch HG, Catalá A, Strittmatter P. Mechanism of rat liver microsomal stearyl-CoA desaturase. Studies of the substrate specificity, enzyme-substrate interactions, and the function of lipid. J Biol Chem. (1976) 251:5095–103. doi: 10.1016/S0021-9258(17)33223-4
10. Zhao G, Tan Y, Cardenas H, Vayngart D, Wang Y, Huang H, et al. Ovarian cancer cell fate regulation by the dynamics between saturated and unsaturated fatty acids. Proc Natl Acad Sci USA. (2022) 119:e2203480119. doi: 10.1073/pnas.2203480119
11. Tesfay L, Paul BT, Konstorum A, Deng Z, Cox AO, Lee J, et al. Stearoyl-coA desaturase 1 protects ovarian cancer cells from ferroptotic cell death. Cancer Res. (2019) 79:5355–66. doi: 10.1158/0008-5472.CAN-19-0369
12. Peck B, Schug ZT, Zhang Q, Dankworth B, Jones DT, Smethurst E, et al. Inhibition of fatty acid desaturation is detrimental to cancer cell survival in metabolically compromised environments. Cancer Metab. (2016) 4:6. doi: 10.1186/s40170-016-0146-8
13. Kim SJ, Choi H, Park SS, Chang C, Kim E. Stearoyl CoA desaturase (SCD) facilitates proliferation of prostate cancer cells through enhancement of androgen receptor transactivation. Mol Cells. (2011) 31:371–7. doi: 10.1007/s10059-011-0043-5
14. Noto A, Raffa S, De Vitis C, Roscilli G, Malpicci D, Coluccia P, et al. Stearoyl-CoA desaturase-1 is a key factor for lung cancer-initiating cells. Cell Death Dis. (2013) 4:e947. doi: 10.1038/cddis.2013.444
15. Wang YX, Jin YY, Wang J, Zhao ZC, Xue KW, Xiong H, et al. Icaritin derivative IC2 induces cytoprotective autophagy of breast cancer cells via SCD1 inhibition. Molecules. (2023) 28:1109. doi: 10.3390/molecules28031109
16. Lee J, Jang S, Im J, Han Y, Kim S, Jo H, et al. Stearoyl-CoA desaturase 1 inhibition induces ER stress-mediated apoptosis in ovarian cancer cells. J Ovarian Res. (2024) 17:73. doi: 10.1186/s13048-024-01389-1
17. Yang C, Jin YY, Mei J, Hu D, Jiao X, Che HL, et al. Identification of icaritin derivative IC2 as an SCD-1 inhibitor with anti-breast cancer properties through induction of cell apoptosis. Cancer Cell Int. (2022) 22:202. doi: 10.1186/s12935-022-02621-y
18. Xiao H, Du X, Tao Z, Jing N, Bao S, Gao WQ, et al. Taurine inhibits ferroptosis mediated by the crosstalk between tumor cells and tumor-associated macrophages in prostate cancer. Adv Sci (Weinh). (2024) 11:e2303894. doi: 10.1002/advs.202303894
19. Chen DS, Mellman I. Elements of cancer immunity and the cancer-immune set point. Nature. (2017) 541:321–30. doi: 10.1038/nature21349
20. Li Y, Li F, Xu L, Shi X, Xue H, Liu J, et al. Single cell analyses reveal the PD-1 blockade response-related immune features in hepatocellular carcinoma. Theranostics. (2024) 14:3526–47. doi: 10.7150/thno.95971
21. Sudholz H, Delconte RB, Huntington ND. Interleukin-15 cytokine checkpoints in natural killer cell anti-tumor immunity. Curr Opin Immunol. (2023) 84:102364. doi: 10.1016/j.coi.2023.102364
22. Li Z, Duan D, Li L, Peng D, Ming Y, Ni R, et al. Tumor-associated macrophages in anti-PD-1/PD-L1 immunotherapy for hepatocellular carcinoma: recent research progress. Front Pharmacol. (2024) 15:1382256. doi: 10.3389/fphar.2024.1382256
23. Luo Y, Zhou H, Krueger J, Kaplan C, Lee SH, Dolman C, et al. Targeting tumor-associated macrophages as a novel strategy against breast cancer. J Clin Invest. (2006) 116:2132–41. doi: 10.1172/JCI27648
24. Lin C, Chu Y, Zheng Y, Gu S, Hu Y, He J, et al. Macrophages: plastic participants in the diagnosis and treatment of head and neck squamous cell carcinoma. Front Immunol. (2024) 15:1337129. doi: 10.3389/fimmu.2024.1337129
25. Chandrashekar DS, Karthikeyan SK, Korla PK, Patel H, Shovon AR, Athar M, et al. UALCAN: An update to the integrated cancer data analysis platform. Neoplasia. (2022) 25:18–27. doi: 10.1016/j.neo.2022.01.001
26. Tang Z, Kang B, Li C, Chen T, Zhang Z. GEPIA2: an enhanced web server for large-scale expression profiling and interactive analysis. Nucleic Acids Res. (2019) 47:W556–w60. doi: 10.1093/nar/gkz430
27. Uhlén M, Fagerberg L, Hallström BM, Lindskog C, Oksvold P, Mardinoglu A, et al. Proteomics. Tissue-based map of the human proteome. Science. (2015) 347:1260419. doi: 10.1126/science.1260419
28. Gao J, Aksoy BA, Dogrusoz U, Dresdner G, Gross B, Sumer SO, et al. Integrative analysis of complex cancer genomics and clinical profiles using the cBioPortal. Sci Signal. (2013) 6:pl1. doi: 10.1126/scisignal.2004088
29. Yuan H, Yan M, Zhang G, Liu W, Deng C, Liao G, et al. CancerSEA: a cancer single-cell state atlas. Nucleic Acids Res. (2019) 47:D900–d8. doi: 10.1093/nar/gky939
30. Li T, Fan J, Wang B, Traugh N, Chen Q, Liu JS, et al. TIMER: A web server for comprehensive analysis of tumor-infiltrating immune cells. Cancer Res. (2017) 77:e108–e10. doi: 10.1158/0008-5472.CAN-17-0307
31. Warde-Farley D, Donaldson SL, Comes O, Zuberi K, Badrawi R, Chao P, et al. The GeneMANIA prediction server: biological network integration for gene prioritization and predicting gene function. Nucleic Acids Res. (2010) 38:W214–20. doi: 10.1093/nar/gkq537
32. Sun D, Wang J, Han Y, Dong X, Ge J, Zheng R, et al. TISCH: a comprehensive web resource enabling interactive single-cell transcriptome visualization of tumor microenvironment. Nucleic Acids Res. (2021) 49:D1420–d30. doi: 10.1093/nar/gkaa1020
33. Dennis G Jr., Sherman BT, Hosack DA, Yang J, Gao W, Lane HC, et al. DAVID: database for annotation, visualization, and integrated discovery. Genome Biol. (2003) 4:P3. doi: 10.1186/gb-2003-4-5-p3
34. Subramanian A, Tamayo P, Mootha VK, Mukherjee S, Ebert BL, Gillette MA, et al. Gene set enrichment analysis: a knowledge-based approach for interpreting genome-wide expression profiles. Proc Natl Acad Sci USA. (2005) 102:15545–50. doi: 10.1073/pnas.0506580102
35. De Larco JE, Wuertz BR, Yee D, Rickert BL, Furcht LT. Atypical methylation of the interleukin-8 gene correlates strongly with the metastatic potential of breast carcinoma cells. Proc Natl Acad Sci USA. (2003) 100:13988–93. doi: 10.1073/pnas.2335921100
36. Bert SA, Robinson MD, Strbenac D, Statham AL, Song JZ, Hulf T, et al. Regional activation of the cancer genome by long-range epigenetic remodeling. Cancer Cell. (2013) 23:9–22. doi: 10.1016/j.ccr.2012.11.006
37. Smith J, Sen S, Weeks RJ, Eccles MR, Chatterjee A. Promoter DNA hypermethylation and paradoxical gene activation. Trends Cancer. (2020) 6:392–406. doi: 10.1016/j.trecan.2020.02.007
38. Huang J, Fan XX, He J, Pan H, Li RZ, Huang L, et al. SCD1 is associated with tumor promotion, late stage and poor survival in lung adenocarcinoma. Oncotarget. (2016) 7:39970–9. doi: 10.18632/oncotarget.v7i26
39. Han C, Hu C, Liu T, Sun Y, Hu F, He Y, et al. IGF2BP3 enhances lipid metabolism in cervical cancer by upregulating the expression of SCD. Cell Death Dis. (2024) 15:138. doi: 10.1038/s41419-024-06520-0
40. Wang J, Zhang Q, Zhou D, Wang Y, Che H, Ge Y, et al. Systematic analysis of fatty acid desaturases in breast invasive carcinoma: The prognosis, gene mutation, and tumor immune microenvironment. Med (Baltimore). (2024) 103:e38597. doi: 10.1097/MD.0000000000038597
41. Luo Y, Huang S, Wei J, Zhou H, Wang W, Yang J, et al. Long noncoding RNA LINC01606 protects colon cancer cells from ferroptotic cell death and promotes stemness by SCD1-Wnt/β-catenin-TFE3 feedback loop signalling. Clin Transl Med. (2022) 12:e752. doi: 10.1002/ctm2.752
42. Vickers AJ, Mahal B, Ogunwobi OO. Racism does not cause prostate cancer, it causes prostate cancer death. J Clin Oncol. (2023) 41:2151–4. doi: 10.1200/JCO.22.02203
43. Hur J, Giovannucci E. Racial differences in prostate cancer: does timing of puberty play a role? Br J Cancer. (2020) 123:349–54. doi: 10.1038/s41416-020-0897-4
44. Stopsack KH, Mucci LA, Giovannucci EL. Aggressive prostate cancer is preventable and so are racial disparities. J Clin Oncol. (2023) 41:4597–8. doi: 10.1200/JCO.23.00507
45. Gleason DF, Mellinger GT. Prediction of prognosis for prostatic adenocarcinoma by combined histological grading and clinical staging. J Urol. (1974) 111:58–64. doi: 10.1016/S0022-5347(17)59889-4
46. Pollack A, Horwitz EM, Movsas B. Treatment of prostate cancer with regional lymph node (N1) metastasis. Semin Radiat Oncol. (2003) 13:121–9. doi: 10.1016/S1053-4296(03)70005-8
47. Tomlins SA, Rhodes DR, Perner S, Dhanasekaran SM, Mehra R, Sun XW, et al. Recurrent fusion of TMPRSS2 and ETS transcription factor genes in prostate cancer. Science. (2005) 310:644–8. doi: 10.1126/science.1117679
48. Abeshouse A, Ahn J, Akbani R, Ally A, Amin S, Andry CD, et al. The molecular taxonomy of primary prostate cancer. Cell. (2015) 163:1011–25. doi: 10.1016/j.cell.2015.10.025
49. Unoki M, Nakamura Y. Methylation at CpG islands in intron 1 of EGR2 confers enhancer-like activity. FEBS Lett. (2003) 554:67–72. doi: 10.1016/S0014-5793(03)01092-5
50. Chen YC, Gotea V, Margolin G, Elnitski L. Significant associations between driver gene mutations and DNA methylation alterations across many cancer types. PloS Comput Biol. (2017) 13:e1005840. doi: 10.1371/journal.pcbi.1005840
51. Baylin SB, Jones PA. Epigenetic determinants of cancer. Cold Spring Harbor Perspect Biol. (2016) 8:a019505. doi: 10.1101/cshperspect.a019505
52. Li X, Yang Q, Shi X, Chen W, Pu N, Li W, et al. Compound but non-linked heterozygous p.W14X and p.L279V LPL gene mutations in a Chinese patient with long-term severe hypertriglyceridemia and recurrent acute pancreatitis. Lipids Health Dis. (2018) 17:144. doi: 10.1186/s12944-018-0789-2
53. Wu FF, Takahashi MP, Pegoraro E, Angelini C, Colleselli P, Cannon SC, et al. A new mutation in a family with cold-aggravated myotonia disrupts Na(+) channel inactivation. Neurology. (2001) 56:878–84. doi: 10.1212/WNL.56.7.878
54. Margiotti K, Sangiuolo F, De Luca A, Froio F, Pearce CL, Ricci-Barbini V, et al. Evidence for an association between the SRD5A2 (type II steroid 5 alpha-reductase) locus and prostate cancer in Italian patients. Dis Markers. (2000) 16:147–50. doi: 10.1155/2000/683607
55. Kim M, Lee NK, Wang CJ, Lim J, Byun MJ, Kim TH, et al. Reprogramming the tumor microenvironment with biotechnology. Biomater Res. (2023) 27:5. doi: 10.1186/s40824-023-00343-4
56. Xiao M, Tang Q, Zeng S, Yang Q, Yang X, Tong X, et al. Emerging biomaterials for tumor immunotherapy. Biomater Res. (2023) 27:47. doi: 10.1186/s40824-023-00369-8
57. Tang L, Huang ZP, Mei H, Hu Y. Insights gained from single-cell analysis of chimeric antigen receptor T-cell immunotherapy in cancer. Mil Med Res. (2023) 10:52. doi: 10.1186/s40779-023-00486-4
58. Cerella C, Dicato M, Diederich M. Enhancing personalized immune checkpoint therapy by immune archetyping and pharmacological targeting. Pharmacol Res. (2023) 196:106914. doi: 10.1016/j.phrs.2023.106914
59. Agalliu I, Salinas CA, Hansten PD, Ostrander EA, Stanford JL. Statin use and risk of prostate cancer: results from a population-based epidemiologic study. Am J Epidemiol. (2008) 168:250–60. doi: 10.1093/aje/kwn141
60. Bhattacharya A, Chowdhury A, Chaudhury K, Shukla PC. Proprotein convertase subtilisin/kexin type 9 (PCSK9): A potential multifaceted player in cancer. Biochim Biophys Acta Rev Cancer. (2021) 1876:188581. doi: 10.1016/j.bbcan.2021.188581
61. Chen M, Zhang J, Sampieri K, Clohessy JG, Mendez L, Gonzalez-Billalabeitia E, et al. An aberrant SREBP-dependent lipogenic program promotes metastatic prostate cancer. Nat Genet. (2018) 50:206–18. doi: 10.1038/s41588-017-0027-2
62. Li Y, Ran Q, Duan Q, Jin J, Wang Y, Yu L, et al. 7-Dehydrocholesterol dictates ferroptosis sensitivity. Nature. (2024) 626:411–8. doi: 10.1038/s41586-023-06983-9
63. Fu L, Ding H, Bai Y, Cheng L, Hu S, Guo Q. IDI1 inhibits the cGAS-Sting signaling pathway in hepatocellular carcinoma. Heliyon. (2024) 10:e27205. doi: 10.1016/j.heliyon.2024.e27205
64. Liu D, Wong CC, Fu L, Chen H, Zhao L, Li C, et al. Squalene epoxidase drives NAFLD-induced hepatocellular carcinoma and is a pharmaceutical target. Sci Trans Med. (2018) 10:eaap9840. doi: 10.1126/scitranslmed.aap9840
65. Saito Y, Yin D, Kubota N, Wang X, Filliol A, Remotti H, et al. A therapeutically targetable TAZ-TEAD2 pathway drives the growth of hepatocellular carcinoma via ANLN and KIF23. Gastroenterology. (2023) 164:1279–92. doi: 10.1053/j.gastro.2023.02.043
66. Xu H, Zhou S, Tang Q, Xia H, Bi F. Cholesterol metabolism: New functions and therapeutic approaches in cancer. Biochim Biophys Acta Rev Cancer. (2020) 1874:188394. doi: 10.1016/j.bbcan.2020.188394
67. Yan Y, Mao X, Zhang Q, Ye Y, Dai Y, Bao M, et al. Molecular mechanisms, immune cell infiltration, and potential drugs for prostate cancer. Cancer biomark. (2021) 31:87–96. doi: 10.3233/CBM-200939
68. Zeng Y, Luo Y, Zhao K, Liu S, Wu K, Wu Y, et al. m6A-mediated induction of 7-dehydrocholesterol reductase stimulates cholesterol synthesis and cAMP signaling to promote bladder cancer metastasis. Cancer Res. (2024). doi: 10.1158/0008-5472.CAN-23-3703
69. Gui CP, Li JY, Fu LM, Luo CG, Zhang C, Tang YM, et al. Identification of mRNA vaccines and conserved ferroptosis related immune landscape for individual precision treatment in bladder cancer. J Big Data. (2022) 9:88. doi: 10.1186/s40537-022-00641-z
70. Zhao W, Prijic S, Urban BC, Tisza MJ, Zuo Y, Li L, et al. Candidate antimetastasis drugs suppress the metastatic capacity of breast cancer cells by reducing membrane fluidity. Cancer Res. (2016) 76:2037–49. doi: 10.1158/0008-5472.CAN-15-1970
71. Lin L, Ding Y, Wang Y, Wang Z, Yin X, Yan G, et al. Functional lipidomics: Palmitic acid impairs hepatocellular carcinoma development by modulating membrane fluidity and glucose metabolism. Hepatology. (2017) 66:432–48. doi: 10.1002/hep.29033
72. Goehring NW, Grill SW. Cell polarity: mechanochemical patterning. Trends Cell Biol. (2013) 23:72–80. doi: 10.1016/j.tcb.2012.10.009
73. Alexander WS, Hilton DJ. The role of suppressors of cytokine signaling (SOCS) proteins in regulation of the immune response. Annu Rev Immunol. (2004) 22:503–29. doi: 10.1146/annurev.immunol.22.091003.090312
74. Propper DJ, Balkwill FR. Harnessing cytokines and chemokines for cancer therapy. Nat Rev Clin Oncol. (2022) 19:237–53. doi: 10.1038/s41571-021-00588-9
75. Roongta UV, Pabalan JG, Wang X, Ryseck RP, Fargnoli J, Henley BJ, et al. Cancer cell dependence on unsaturated fatty acids implicates stearoyl-CoA desaturase as a target for cancer therapy. Mol Cancer Res. (2011) 9:1551–61. doi: 10.1158/1541-7786.MCR-11-0126
76. Rudalska R, Harbig J, Snaebjornsson MT, Klotz S, Zwirner S, Taranets L, et al. LXRα activation and Raf inhibition trigger lethal lipotoxicity in liver cancer. Nat Cancer. (2021) 2:201–17. doi: 10.1038/s43018-020-00168-3
77. Imamura K, Tomita N, Kawakita Y, Ito Y, Ono K, Nii N, et al. Discovery of novel and potent stearoyl coenzyme A desaturase 1 (SCD1) inhibitors as anticancer agents. Bioorg Med Chem. (2017) 25:3768–79. doi: 10.1016/j.bmc.2017.05.016
78. Nishizawa S, Sumi H, Satoh Y, Yamamoto Y, Kitazawa S, Honda K, et al. In vitro and in vivo antitumor activities of T-3764518, a novel and orally available small molecule stearoyl-CoA desaturase 1 inhibitor. Eur J Pharmacol. (2017) 807:21–31. doi: 10.1016/j.ejphar.2017.03.064
79. von Roemeling CA, Marlow LA, Wei JJ, Cooper SJ, Caulfield TR, Wu K, et al. Stearoyl-CoA desaturase 1 is a novel molecular therapeutic target for clear cell renal cell carcinoma. Clin Cancer Res. (2013) 19:2368–80. doi: 10.1158/1078-0432.CCR-12-3249
80. Guo Z, Huo X, Li X, Jiang C, Xue L. Advances in regulation and function of stearoyl-CoA desaturase 1 in cancer, from bench to bed. Sci China Life Sci. (2023) 66:2773–85. doi: 10.1007/s11427-023-2352-9
Keywords: stearoyl-coenzyme A desaturase, prostate adenocarcinoma, gene expression, immune microenvironment, functional enrichment analysis, tumor progression
Citation: Wang J, Ying L, Xiong H, Zhou D-R, Wang Y-X, Che H-L, Zhong Z-F, Wu G-S and Ge Y-J (2024) Comprehensive analysis of stearoyl-coenzyme A desaturase in prostate adenocarcinoma: insights into gene expression, immune microenvironment and tumor progression. Front. Immunol. 15:1460915. doi: 10.3389/fimmu.2024.1460915
Received: 07 July 2024; Accepted: 28 August 2024;
Published: 16 September 2024.
Edited by:
Guan-Jun Yang, Ningbo University, ChinaReviewed by:
Zhaotong Cong, Chengdu University of Traditional Chinese Medicine, ChinaDechao Feng, University College London, United Kingdom
Hongwei Gao, Guangxi University of Chinese Medicine, China
Copyright © 2024 Wang, Ying, Xiong, Zhou, Wang, Che, Zhong, Wu and Ge. This is an open-access article distributed under the terms of the Creative Commons Attribution License (CC BY). The use, distribution or reproduction in other forums is permitted, provided the original author(s) and the copyright owner(s) are credited and that the original publication in this journal is cited, in accordance with accepted academic practice. No use, distribution or reproduction is permitted which does not comply with these terms.
*Correspondence: Yun-Jun Ge, 8202209002@jiangnan.edu.cn
†These authors have contributed equally to this work and share first authorship