- 1Department of Emergency, The Fourth Hospital of Hebei Medical University, Shijiazhuang, Hebei, China
- 2Department of Emergency, Third Hospital of Hebei Medical University, Shijiazhuang, Hebei, China
Purpose: Sepsis is an unusual systemic reaction to what is sometimes an otherwise ordinary infection, and it probably represents a pattern of response by the immune system to injury. However, the relationship between biomarkers and sepsis remains unclear. This study aimed to find potential molecular biomarkers, which could do some help to patients with sepsis.
Methods: The sepsis dataset GSE28750, GSE57065 was downloaded from the GEO database, and ten patients with or without sepsis from our hospital were admitted for RNA-seq and the differentially expressed genes (DEGs) were screened. The Metascape database was used for functional enrichment analysis and was used to found the differential gene list. Protein-protein interaction network was used and further analyzed by using Cytoscape and STRING. Logistic regression and Correlation analysis were used to find the potential molecular biomarkers.
Results: Taking the intersection of the three datasets yielded 287 differential genes. The enrichment results included Neutrophil degranulation, leukocyte activation, immune effectors process, positive regulation of immune response, regulation of leukocyte activation. The top 10 key genes of PPI connectivity were screened using cytoHubba plugin, which were KLRK1, KLRB1, IL7R, GZMA, CD27, PRF1, CD8A, CD2, IL2RB, and GZMB. All of the hub genes are higher expressed in health group of different databases. Logistic regression showed that IL7R, GZMA and CD8A proteins were analyzed and all of them were statistically significant. Correlation analysis showed that there was a statistically significant correlation between IL7R, GZMA and CD8A.
Conclusion: KLRK1, KLRB1, IL7R, GZMA, CD27, PRF1, CD8A, CD2, IL2RB, GZMB are key genes in sepsis, which associated with the development of sepsis. However, IL7R, GZMA and CD8A may serve as the attractively potential molecular biomarkers for sepsis.
Introduction
Sepsis is characterized by a dysregulated host immune response to infection (1). Sepsis-induced immunosuppression is resulted from disruption of immune homeostasis. It is characterized by the release of anti-inflammatory cytokines, abnormal death of immune effector cells, hyperproliferation of immune suppressor cells, and expression of immune checkpoints (2).
With the efforts of critical care specialists around the world applying anti-infective combined with fluid resuscitation and organ function support, the mortality rate of septic patients is still as high as 20%, although it has been significantly reduced (3). The pathophysiologic mechanisms of sepsis are complex, and relevant indicators are not easily accessible, making early diagnosis difficult in the clinic. Therefore, understanding the pathophysiological mechanisms of sepsis pathogenesis and identifying potential diagnostic and therapeutic biomarkers are essential for improving clinical outcomes and reducing mortality in sepsis patients (4–6). However, the relationship between biomarkers and sepsis remains unclear.
Bioinformatics was used to analyze genomic and proteomic data, which may do some help to predicting novel genes of potential value accurately (7). In recent years, bioinformatics analysis has been widely used for the detection and analysis of differential gene expression in sepsis (8–11). Currently, there is little exploration of the role of inflammatory factors in sepsis through sequencing and big data analysis. Therefore, it is necessary to explore the role of inflammatory factors in sepsis through our clinical sample sequencing combined with database data. This study aimed to find potential molecular biomarkers, which could do some help to patients with sepsis.
Materials and methods
Subject recruitment and blood collection
In the present study, we analyzed 6 patients with sepsis who were admitted to the emergency intensive care unit of the Fourth Hospital of Hebei Medical University. The recruitment lasted from May 2023 to July 2023. Their blood samples were collected within 24 hours after the admission and were analyzed. Peripheral blood samples were also collected from the control group, they were 4 healthy individuals. Inclusion criteria were as followed: patients were diagnosed with sepsis and were admitted to emergency intensive care unit, sepsis diagnosed with sepsis 3.0 definition and diagnosis standard (infection + ΔSOFA scoring ≥2) jointly issued by the Society of Critical Care Medicine and European Society of Intensive Care Medicine, age ≥18 and ≤65 years, and subjects or legal representatives willing to participate in the study and to sign an informed consent form. Exclusion criteria were as followed: those with previous organ failure(Including heart valve disease, nephritis, nephrotic syndrome, etc), those with previous immune system diseases, those with previous blood system diseases, patients unwilling to participate. Our study plans were approved by the Ethics Committee of our hospital.
RNA-seq
In the first 24 hours after the patients’ admission, their blood samples were collected with the PAXgene Blood RNA Tube (PreAnalytix GmbH, Switzerland)and were stored in the refrigerator in our hospital until further analysis. After extraction of RNA samples, quality control detection was made with Bioanalyzer 2100 (Agilent, CA, USA), and mRNA was required to meet Concentration >50ng/μL, RIN value >7.0, total RNA >1μg to satisfy downstream experiments. After sample quality control was passed, a DNA database was built, and RNA-seq was made with Illumina NovaseqTM 6000.
Sepsis dataset
In the present study, the GSE28750, GSE57065 dataset was downloaded from the GEO online database (www.ncbi.nlm.nih.gov/geo). In our study, 10 peripheral blood samples of sepsis patients were selected from GSE28750 as the sepsis group, and 20 peripheral blood samples of healthy volunteers as the control group; 28 peripheral blood samples of sepsis patients were selected from GSE57065 as the sepsis group, and 25 peripheral blood samples of healthy volunteers as the control group.
Screening of differential genes
Differential expression genes (DEGs) between the healthy group and the sepsis group were screened using DESeq2. The R package limma (version 3.40.6) was used to explore the DEGs between sepsis and non-sepsis peripheral blood. The result of RNA-seq (sepsis patients VS. non-sepsis participants) dataset was analyzed using the lmFit method for multivariate regression. The Bayes method was used to calculate the moderated t-statistics, moderated f-statistics and log ratios of differential expression by empirical Bayesian adjustment. A multiplicity of differences |FC|≥1.5 and a q-value of <0.05 was used as the threshold criterion (no multiplicity of differences in the multiple group comparisons, and genes screened for q<0.05 were genes statistically different between the multiple groups). Volcano plots were used to find the DEGs.
Functional enrichment analysis
The Metascape database (https://metascape.org/gp/index.html#/main/step1) provides a list of annotations and resources and can visually export them. The Metascape database was used for functional enrichment analysis and was used to found the differential gene list.
Protein-protein interaction analysis
Protein-protein interaction (PPI) network is used to screen potential core genes. In our study, STRING (https://string-db.org/) online platform was used to screen core genes of DEGs. By using the multi-protein online tool in the STRING database, the PPI networks were predicted. The results were showed with Cytoscape 3.6.1The most critical modules were then found using the Complex Detection (MCODE) plugin.
Statistical analysis
The data were analyzed by using Graph PadPrism version 9.0 (GraphPad Software, Inc.). For comparisons between two groups, an unpaired, 2-tailed Student’s t-test was used. The data is presented as the mean ± standard deviation. Univariate and multivariate logistic regression analyses were performed to analyze the potential molecular biomarkers. P<0.05 was considered to indicate a statistically significant difference.
Results
Differentially expressed genes
The design concept of the article was represented by a flowchart (Figure 1). 244 differential genes were screened from GSE28750, including 152 expression up-regulated genes and 92 expression down-regulated genes, and 264 differential genes were screened from GSE57065, including 163 expression up-regulated genes and 103 expression down-regulated genes. A total of 6,171 differentially expressed genes were obtained between sepsis and normal control groups, including 4625 up-regulated and 1546 down-regulated expressed genes. Taking the intersection of the three datasets yielded 287 differential genes (Figure 2). Three volcano plots showed up- and down-regulated expression genes (Figure 3).
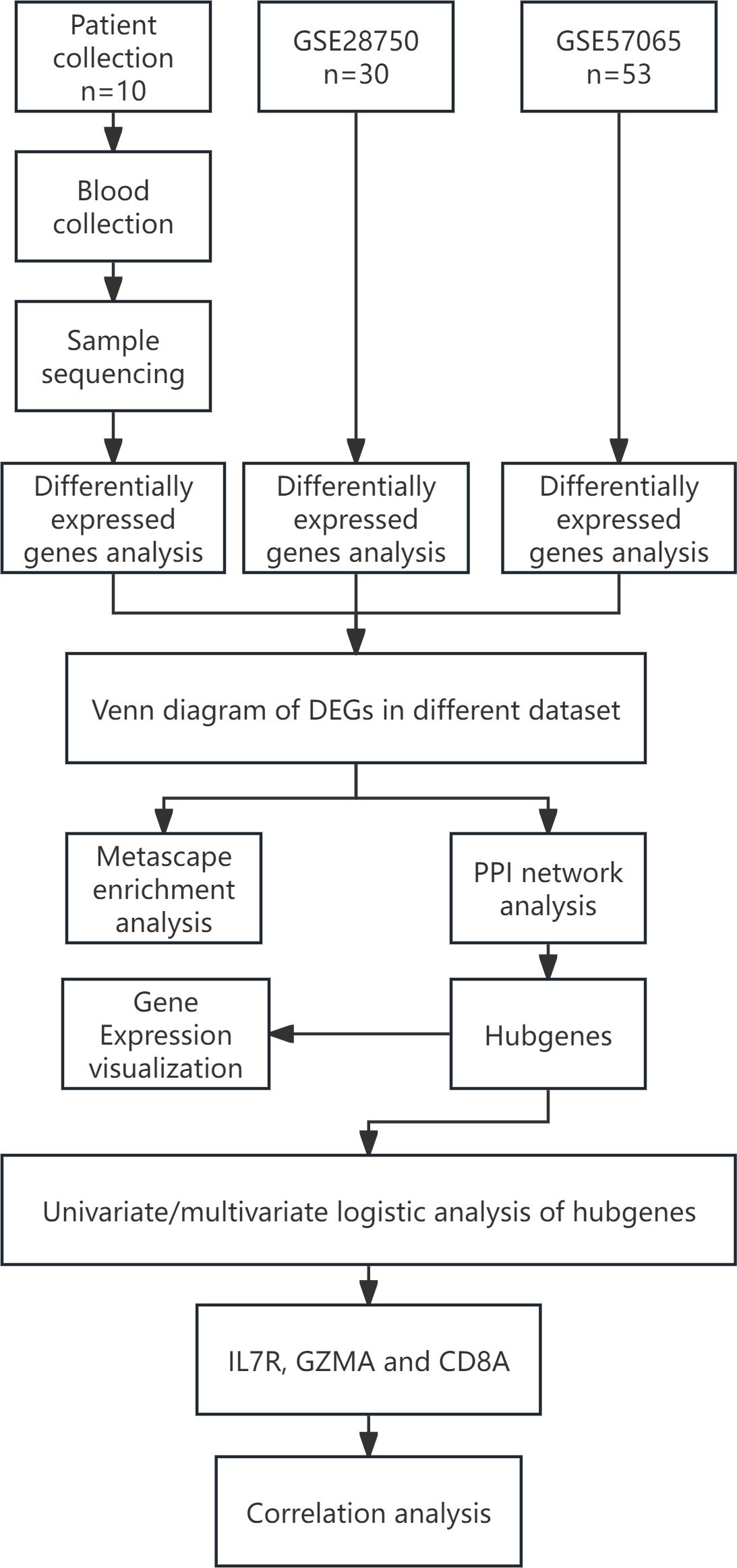
Figure 1. Flow chart of the statistical analysis. DEGs, Differentially Expressed Genes; PPI, protein-protein interaction.st.
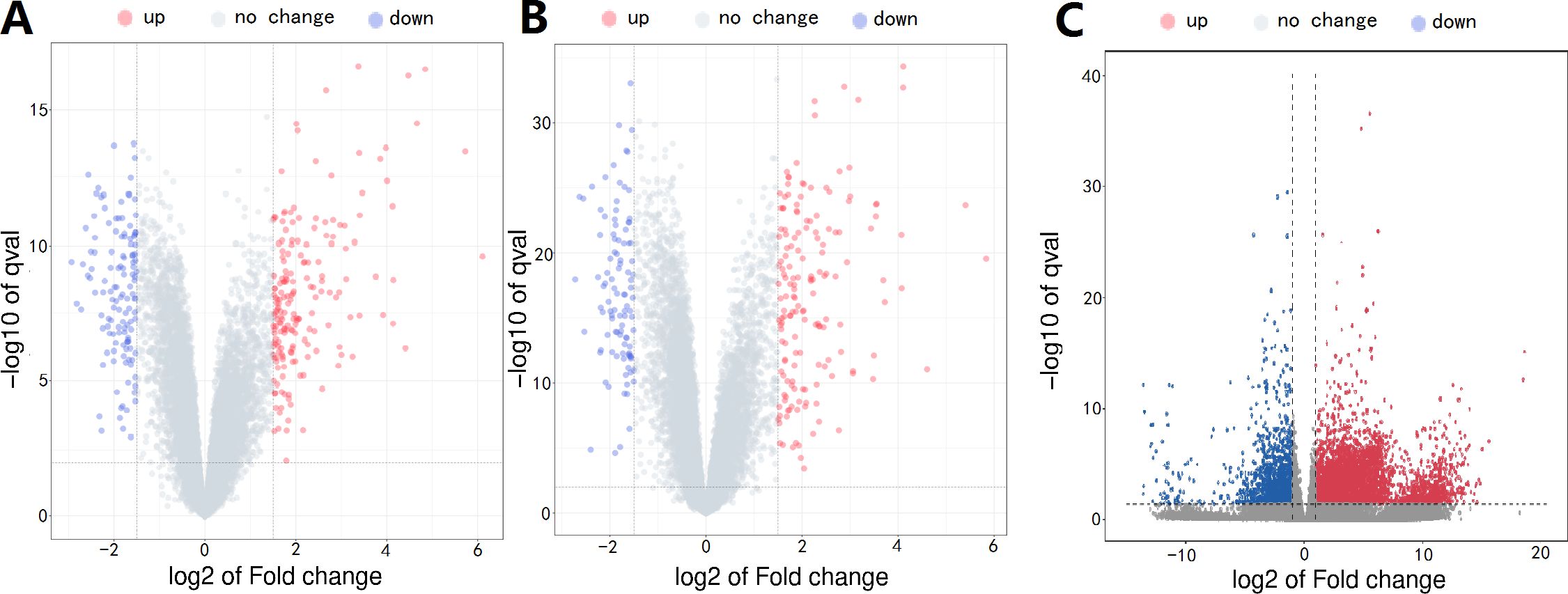
Figure 3. (A) GSE28750 microarray, (B) GSE57065 microarray, (C) RNA-seq, Red, relatively high expression; Blue, relatively low expression.
Metascape enrichment analysis
Metascape enrichment shows GO enrichment terms (Figure 4A) and enrichment networks colored with enrichment terms and p-values (Figures 4B, C, 5). Figure 4A is a bar graph of enriched terms across input gene lists, colored by p-values. The enrichment results included Neutrophil degranulation, leukocyte activation, immune effector process, positive regulation of immune response, regulation of leukocyte activation. Figure 4B shows the network of enriched terms. The enriched results were colored with cluster ID, where nodes sharing the same cluster ID are typically close to each other. The Figure 4C shows the enrichment result items colored with P-values. Items with more genes in them tend to have more significant P-values. As shown in the Figure 5, the significant models of genes were shown in Figure 5A, which was analyzed by MCODE. The significant models of genes were also shown in the whole Protein-protein interaction network.
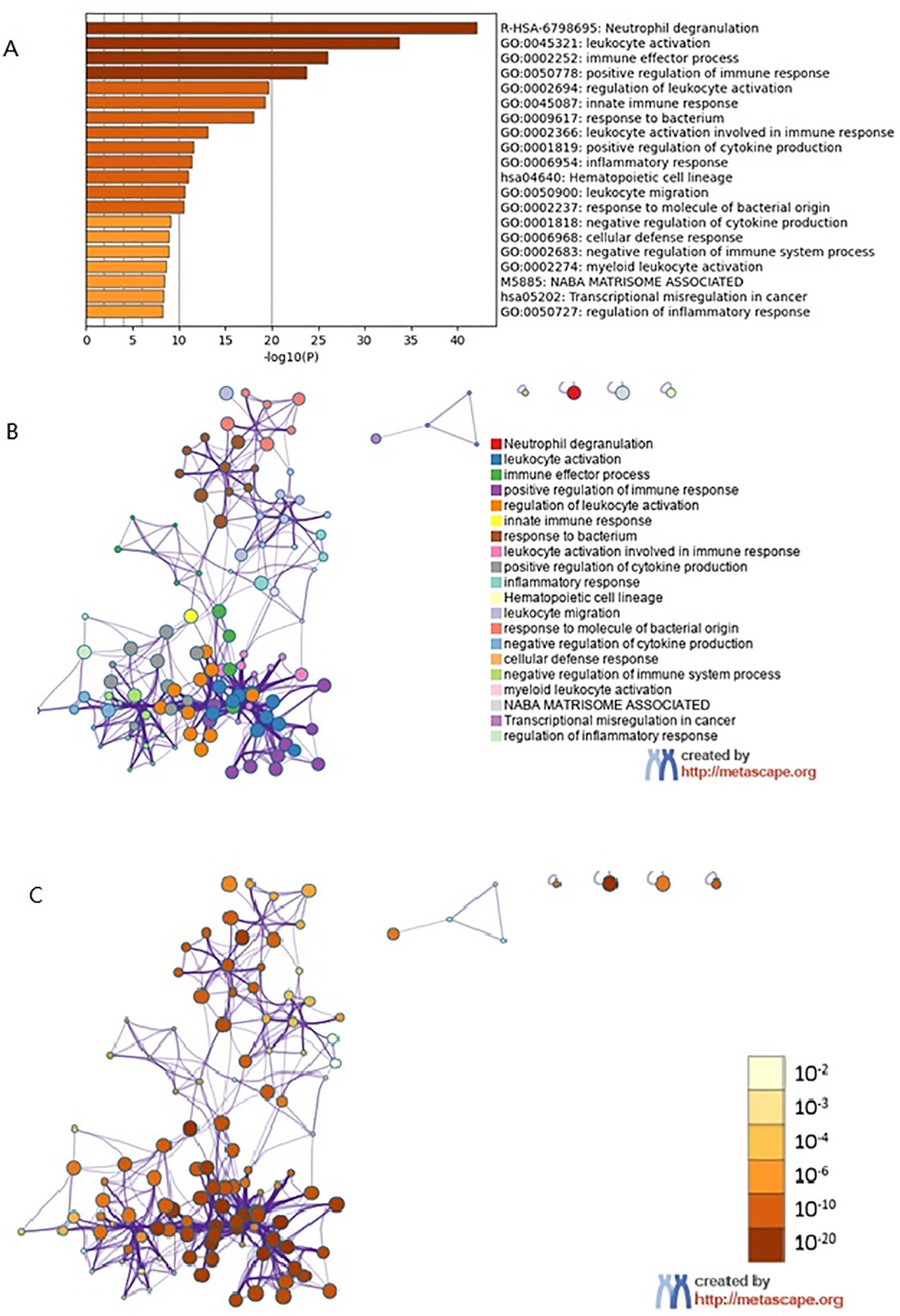
Figure 4. Metascape enrichment analysis. (A) GO enrichment terms. (B) Enrichment network colored by enrichment terms. (C) Enrichment network colored by p-value.
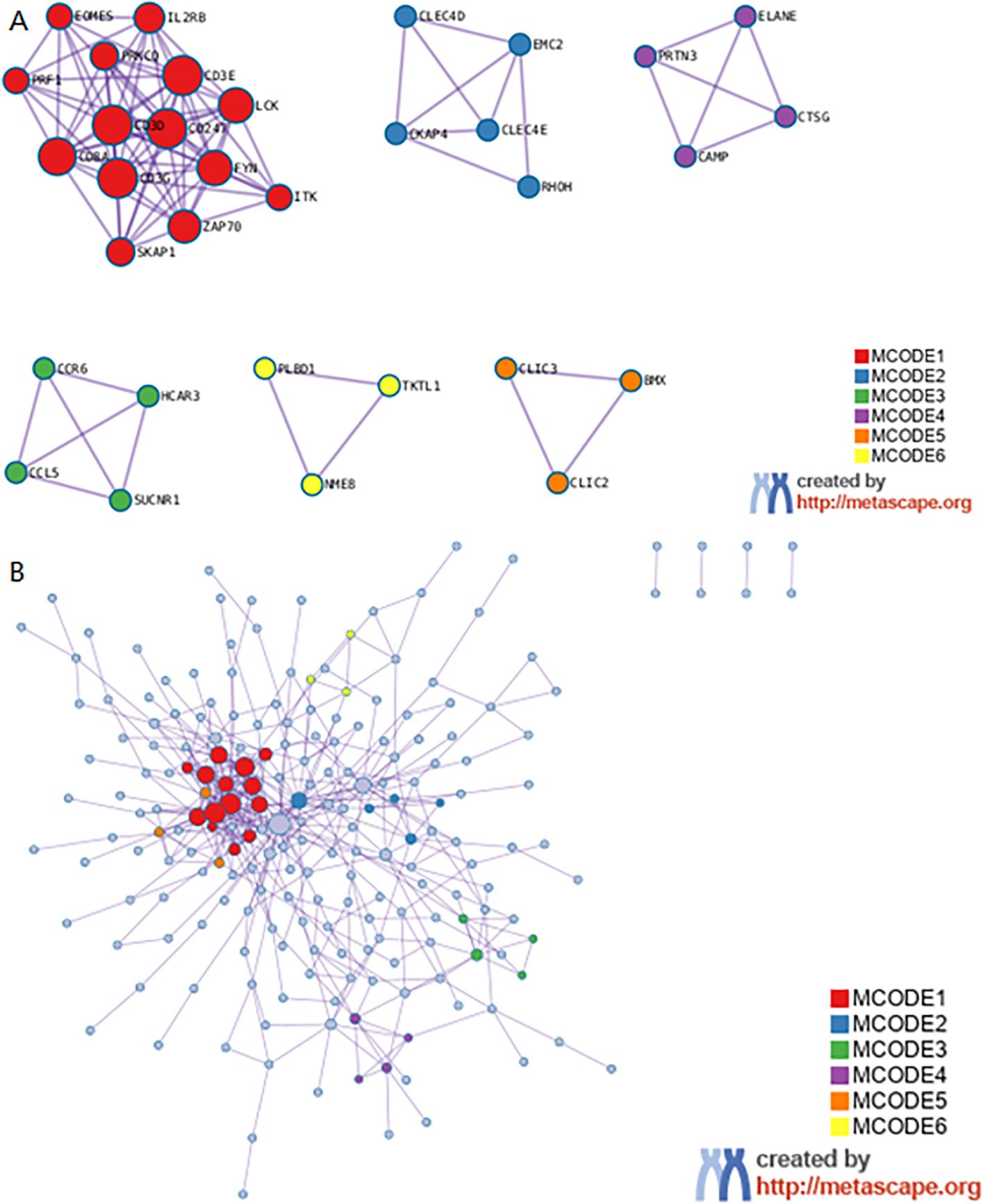
Figure 5. Metascape enrichment analysis. (A) The significant model. (B) The protein-protein interaction network.
Protein-protein interaction network analysis and identification of hub genes
The Search Tool for Retrieval of 31 Interacting EP-DEG online database was used to create protein network interaction maps. By using the multi-protein online tool in the STRING database, the PPI networks were predicted. The results were showed with Cytoscape 3.6.1. The most critical modules were then found using the Complex Detection (MCODE) plugin. The PPI network obtained from the visual analysis of Cytoscape software included 281 nodes and 1639 edges, Figure 6A; the most significant modules were found using the MCODE plug-in, which contained a total of 34 genes, see Figure 6B. The top 10 key genes of PPI connectivity were screened using cytoHubba plugin, which were KLRK1, KLRB1, IL7R, GZMA, CD27, PRF1, CD8A, CD2, IL2RB, GZMB (Figure 6C).
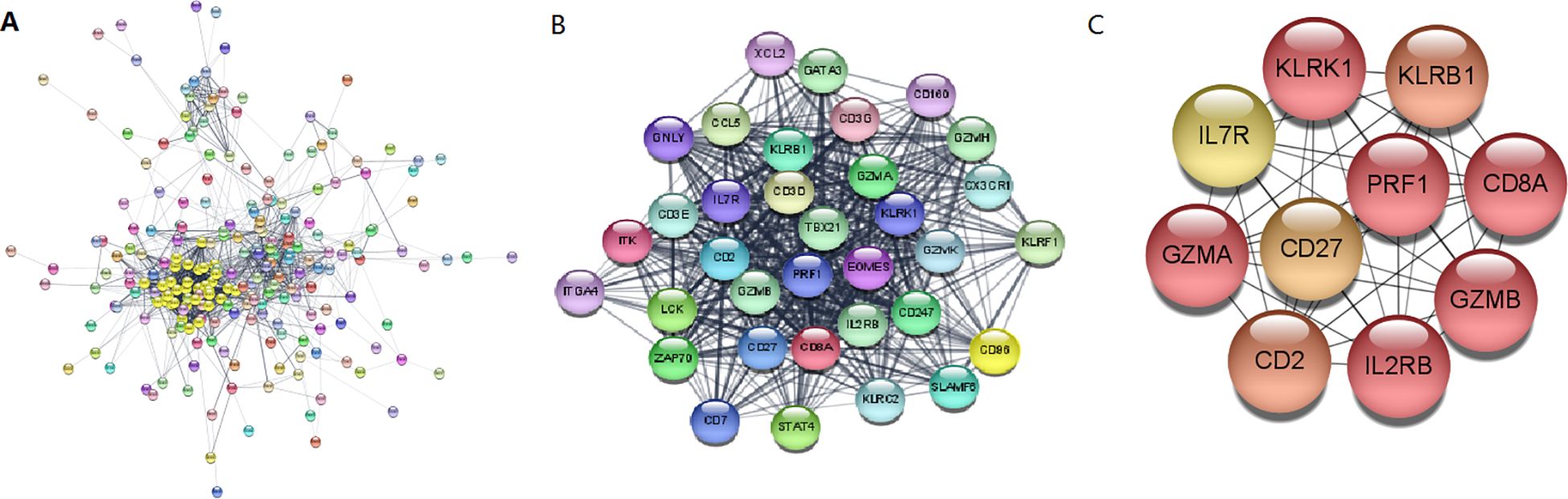
Figure 6. Construction and analysis of the PPI networks. (A) PPI network of DEGs. (B) The interaction of core genes. (C) The top 10 hub genes. hub genes: KLRK1, KLRB1, IL7R, GZMA, CD27, PRF1, CD8A, CD2, IL2RB, GZMB. PPI, protein-protein interaction; DEG, differentially expressed gene.
Expression of key genes in sepsis and healthy groups
All of the hub genes (IL7R, KLRK1, KLRB1, GZMA, CD27, PRF1, CD8A, CD2, IL2RB, GZMB) are higher expressed in health group of different databases (Figures 7A–C).
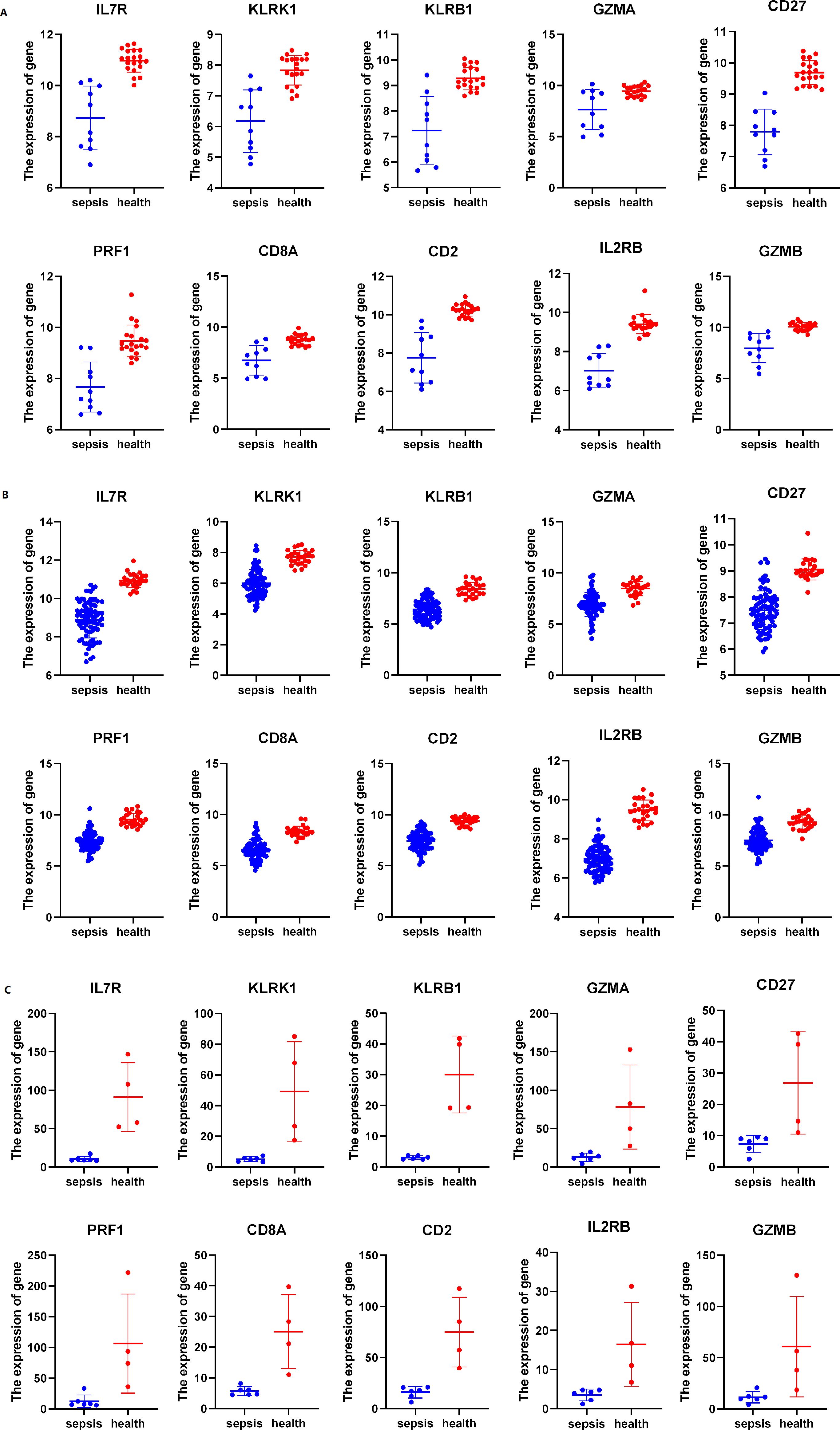
Figure 7. The expression of hub genes in different dataset (A) The expression of genes in GSE28750, (B) The expression of genes in GSE57065, (C) RNA seq of our dataset. All data was shown by mean ± SD.
Univariate and multivariate logistic regression analyses of hubgenes
In this study we applied logistic regression for analysis and the results showed that IL7R, GZMA and CD8A proteins were analyzed and all of them were statistically significant, so we analyzed these 3 proteins for subsequent experiments (Tables 1, 2). Correlation analysis showed that there was a correlation between the two IL7R, GZMA and CD8A proteins and the correlation was statistically significant (Figure 8).
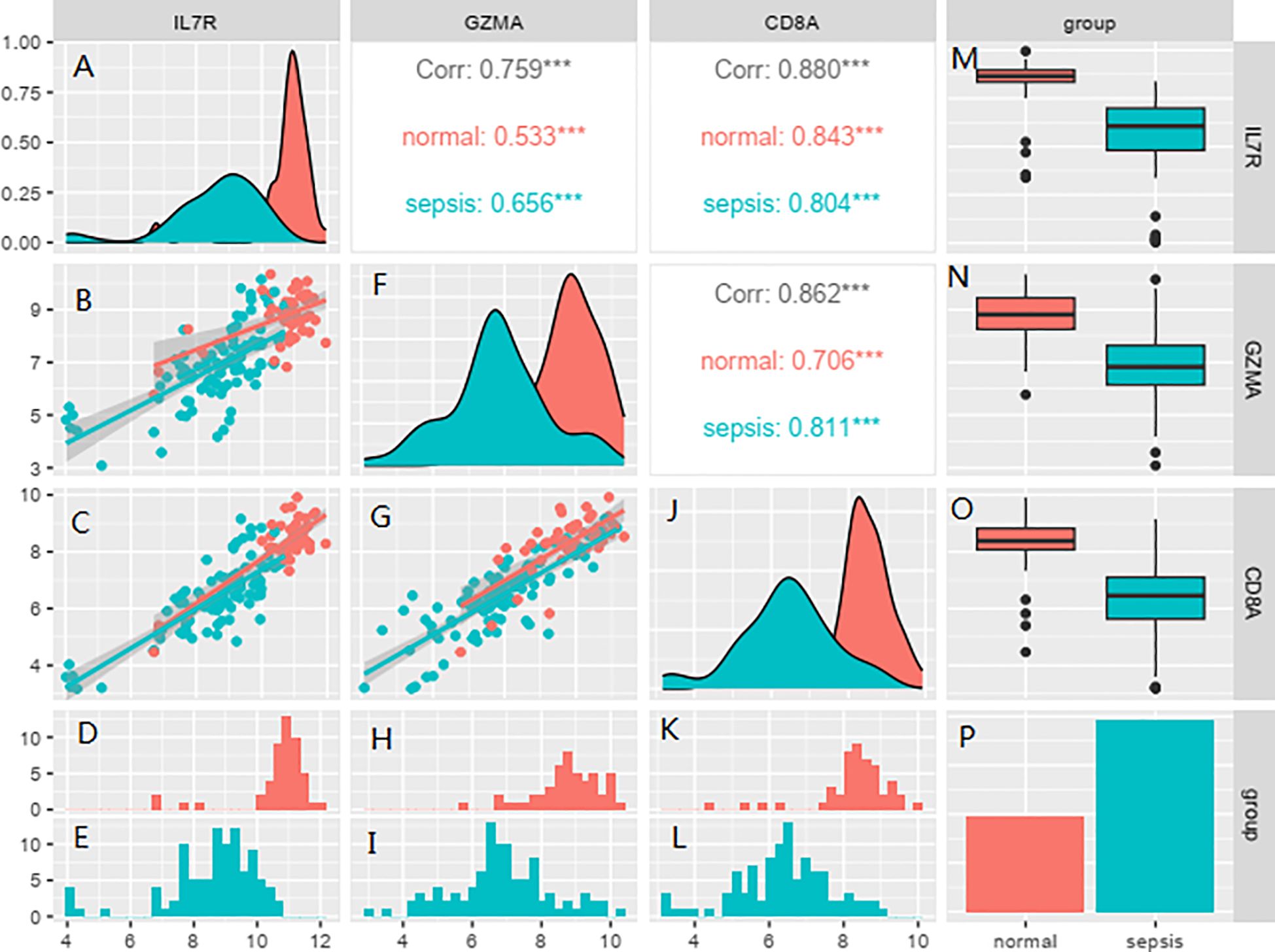
Figure 8. Correlation analysis showed that there was a correlation between the IL7R, GZMA and CD8A. Red represents normal subjects, and blue represents sepsis patients. (A, F, J) are the abundance maps of gene expression in different groups, (B, C, G) are the scatter plots of correlation analysis of corresponding gene expression in different groups, (D, H, K) are the gene expression levels of each patient in the normal group, (E, I, L) are the expression levels of corresponding genes in each patient in the sepsis group, (M, N, O) are the line box plots of corresponding gene expression in different groups, presented in the form of mean ± standard deviation. (P) shows the sepsis group and the healthy group.
Discussion
Sepsis is described as the syndrome consisting of complex biochemical and pathophysiological dysregulation (12). Dysregulation of the body’s inflammatory response followed by the promotion of an inflammatory cascade is an important basis for the pathophysiologic changes in sepsis (13). Multiple pathophysiologic processes are involved in septic organ damage, and immunoregulatory imbalance is one of the important mechanisms (14). At the cellular and molecular level, the mechanisms include dysregulation of the inflammatory response, immunosuppression, coagulation disorders, apoptosis of immune cells, endoplasmic reticulum stress, and other pathophysiological processes (15).
Mechanisms of sepsis have been reported by investigators, but few of them have addressed its molecular markers; in fact, molecular biomarkers have great potential for the diagnosis, monitoring and prognosis of sepsis. Using the sequencing technology, the use of RNA-seq in subbiology has become very common, and its role has expanded the understanding of tiny molecules (16, 17).
Taking the intersection of the three datasets, we find 287 DEGs. Moreover, The enrichment results included Neutrophil degranulation, leukocyte activation, immune effector process, positive regulation of immune response, regulation of leukocyte activation. It has been shown that DEGs are associated with the process of apoptosis and immune system, further, studies have confirmed that apoptosis of immune cells promotes the process of organ dysfunction along with immune failure in patients with sepsis (18). Innate immune cells recognize pathogens and release pro-inflammatory cytokines such as TNF-α, IL-1, IL-6, which play an immune defense role; at the same time, they also negatively elevate anti-inflammatory cytokines such as TGF-β, IL-4, and IL-10 (19–21). It is now believed that sepsis is caused by excessive systemic inflammation leading to immune dysfunction, and that immune cells such as neutrophils, macrophages, and T-lymphocytes are involved in the regulation of the inflammatory response (22). By using cytoHubba plugin, the top 10 genes KLRK1, KLRB1, IL7R, GZMA, CD27, PRF1, CD8A, CD2, IL2RB, and GZMB were found and more of these hub-genes have high relationship with immune system. Therefore, keeping an eye on the expression of these genes in the blood can help clinicians make clinical decisions that will benefit their patients. After initially analyzing the more important 10 hub-genes, we further performed Univariate and multivariate logistic regression based on the patients’ clinical information, and found that three genes, IL7R, GZMA and CD8A, were statistically significant in the multivariate logistic regression, which implies that these three genes are independent risk factors for determining sepsis. This provides clinicians with a basis for decision-making in the diagnosis, treatment, and prediction of clinical prognosis for patients with sepsis.
Imbalance of the immune response is one of the main mechanisms of sepsis, and immune dysfunction in sepsis is closely related to T lymphocytes (23). CD4, CD8A, CD28, CD2, CD3E as T cell surface active molecules play important roles in the immune response process; among them, the roles of CD4 and CD8 T lymphocytes in sepsis have been widely recognized (24). Several previous studies have demonstrated the CD28, CD3, CD4 molecules in sepsis or septic shock (25, 26). Study showed some hub genes (CD2, CD27, GZMA, KLRB1, and PRF1) have been screened out as sepsis biomarkers and all of them were down regulated genes in sepsis, which consistent with our findings (27).
The protein encoded by CD2 is a surface antigen found on all peripheral blood T cells. A study also found that CD2 is identified as the down regulated crucial gene set in sepsis (28). The protein encoded by CD27 is a member of the tumor necrosis factor receptor superfamily and is also necessary for the production and long-term maintenance of T cell immunity. CD27 may help identify preterm infants with sepsis and may also help clinicians identify children at high risk (29). CD8A encodes the cd8α chain of the dimeric CD8 protein. CD8A is primarily involved in cell-mediated immune defense and T cell development (30, 31). CD8 deficiency increases susceptibility to infection (32). Harland et al (24) revealed that cpG methylation of the cd8a locus has a potential role in the downregulation of CD8.
IL7, an important member of the chemokine family, mediates the immune response by binding to the receptor IL7R to promote lymphocyte growth, macrophage activation, and cytokine and inflammatory factor secretion (33). Some studies had shown that the level of IL7R in sepsis was low expressed, which was consistent with our findings (34). Animal experiments have shown that activation of the IL-7/IL-7R signaling pathway improves survival in septic mice (35). Some clinical studies have also suggested that the IL-7/IL-7R signaling pathway is associated with improved lymphocyte function in sepsis patients (36). IL2RB is a subunit of IL2R and is closely related to humoral immunity as it triggers IL2R through IL2 binding, leading to proliferation and differentiation of a large number of immune cells, including T cells, B cells and macrophages. IL2RB is recognized as an indicator of sequential organ failure and is negatively correlated with sepsis mortality (37). Another study showed that targeting IL2RB can do some help to reduce acute lung injury caused by sepsis (38).
PRF1 is a perforin protein secreted by natural killer cells, cytotoxic T-lymphocytes and T-cells, which plays an important role in immunoregulation and immunosurveillance, and has been widely studied in tumors and immune diseases (39). Some studies have found that PRF1 gene expression is reduced in T cells and natural killer cells in patients with sepsis, but the exact mechanism of action still needs to be further clarified (40). GZMA is a serine protease specific for T cells and NK cells. It may be a common component for cytotoxic T lymphocytes and NK cells to cleave target cells. Study showed that GZMA was a key gene of the inflammatory response during abdominal sepsis (41). A study also showed the inhibition of GZMA can reduce inflammation and improve survival during Escherichia coli sepsis (42). GZMB is a member of grazymes family that was considered to exert cytotoxic effects against pathogen invasion (43). Some studies reported that GZMB is involved in the coagulation cascade, regulating the function of platelets and endothelial barrier permeability in sepsis (44). Study showed GZMB have potential diagnostic value in sepsis diagnosis (45). KLRK1 is known as homo sapiens killer cell lectin-like receptor subfamily K, member 1. Viral or bacterial infection can lead to the induction of KLRK1 ligands on cells, which can activate the immune system to recognize and eliminate them (46). KLRB1, known as killer cell lectin like receptor B1, is a gene encoding CD161. CD161 is expressed on immune cells (47). Study showed that KLRB1 was identified as the downregulated crucial gene set in sepsis (28).
The stability of mRNA is influenced by temperature, mRNA length, concentration, pH value, and buffer type, which in turn affects protein expression (48). A study shows that attenuating ribosome load improves protein output from mRNA by limiting translation-dependent mRNA decay (49). Another study states that, cytidine-containing tails robustly enhance and prolong protein production of synthetic mRNA in cell and in vivo (50). Although the half-life of mRNA has a significant impact on protein formation, it does not affect the conclusion of the data analysis in this article that IL7R, GZMA and CD8A may serve as the attractively potential molecular biomarkers for sepsis.
Conclusion
Based on the discussion and analysis, it is reasonable to assume that IL7R, GZMA and CD8A possess significant potential value in the diagnosis and prediction of sepsis. We have found that the immune system plays an important role in the development of sepsis, so intervening or modulating the balance of the immune system in the body may benefit sepsis patients in clinical diagnosis and treatment. Our study also had the limitation of a small sample size, so we used correlation analysis to identify key genes for further clinical trial validation.
Data availability statement
The datasets presented in this study can be found in online repositories. The names of the repository/repositories and accession number(s) can be found in the article/supplementary material.
Ethics statement
The studies involving humans were approved by The Fourth Hospital of Hebei Medical University. The studies were conducted in accordance with the local legislation and institutional requirements. The participants provided their written informed consent to participate in this study. The animal studies were approved by The Fourth Hospital of Hebei Medical University. The studies were conducted in accordance with the local legislation and institutional requirements. Written informed consent was obtained from the owners for the participation of their animals in this study.
Author contributions
JL: Writing – original draft, Conceptualization, Data curation, Formal analysis, Funding acquisition, Investigation, Methodology, Project administration, Resources, Software, Supervision, Validation, Visualization, Writing – review & editing. BY: Writing – review & editing, Formal analysis. SD: Writing – review & editing, Data curation, Investigation. LW: Writing – review & editing. JS: Writing – review & editing.
Funding
The author(s) declare that no financial support was received for the research, authorship, and/or publication of this article.
Acknowledgments
The authors would like to thank all the staff of the participating departments.
Conflict of interest
The authors declare that the research was conducted in the absence of any commercial or financial relationships that could be construed as a potential conflict of interest.
Publisher’s note
All claims expressed in this article are solely those of the authors and do not necessarily represent those of their affiliated organizations, or those of the publisher, the editors and the reviewers. Any product that may be evaluated in this article, or claim that may be made by its manufacturer, is not guaranteed or endorsed by the publisher.
Abbreviations
GEO, Gene Expression Omnibus; DEGs, Differentially Expressed Genes; PPI, protein-protein interaction; SIRS, systemic inflammatory response syndrome; SOFA, Sequential Organ Failure Assessment; GO, gene ontology.
References
1. Cajander S, Kox M, Scicluna BP, Weigand MA, Mora RA, Flohé SB, et al. Profiling the dysregulated immune response in sepsis: overcoming challenges to achieve the goal of precision medicine. Lancet Respir Med. (2024) 12:305–22. doi: 10.1016/S2213-2600(23)00330-2
2. Liu D, Huang SY, Sun JH, Zhang HC, Cai QL, Gao C, et al. Sepsis-induced immunosuppression: mechanisms, diagnosis and current treatment options. Mil Med Res. (2022) 9:56. doi: 10.1186/s40779-022-00422-y
3. Seymour CW, Kennedy JN, Wang S, Chang CH, Elliott CF, Xu Z, et al. Derivation, validation, and potential treatment implications of novel clinical phenotypes for sepsis. JAMA. (2019) 321:2003–17. doi: 10.1001/jama.2019.5791
4. Delano MJ, Ward PA. Sepsis-induced immune dysfunction: can immune therapies reduce mortality. J Clin Invest. (2016) 126:23–31. doi: 10.1172/JCI82224
5. Kumar S, Tripathy S, Jyoti A, Singh SG. Recent advances in biosensors for diagnosis and detection of sepsis: A comprehensive review. Biosens Bioelectron. (2019) 124-125:205–15. doi: 10.1016/j.bios.2018.10.034
6. Póvoa P, Coelho L, Dal-Pizzol F, Ferrer R, Huttner A, Conway Morris A, et al. How to use biomarkers of infection or sepsis at the bedside: guide to clinicians. Intensive Care Med. (2023) 49:142–53. doi: 10.1007/s00134-022-06956-y
7. Ayyildiz D, Piazza S. Introduction to bioinformatics. Methods Mol Biol. (2019) 1986:1–15. doi: 10.1007/978-1-4939-9442-7_1
8. Gong T, Liu Y, Tian Z, Zhang M, Gao H, Peng Z, et al. Identification of immune-related endoplasmic reticulum stress genes in sepsis using bioinformatics and machine learning. Front Immunol. (2022) 13:995974. doi: 10.3389/fimmu.2022.995974
9. Zhang WY, Chen ZH, An XX, Li H, Zhang HL, Wu SJ, et al. Analysis and validation of diagnostic biomarkers and immune cell infiltration characteristics in pediatric sepsis by integrating bioinformatics and machine learning. World J Pediatr. (2023) 19:1094–103. doi: 10.1007/s12519-023-00717-7
10. Wang Q, Wang C, Zhang W, Tao Y, Guo J, Liu Y, et al. Identification of biomarkers related to sepsis diagnosis based on bioinformatics and machine learning and experimental verification. Front Immunol. (2023) 14:1087691. doi: 10.3389/fimmu.2023.1087691
11. Chen P, Chen J, Ye J, Yang L. Identification of an immune-related gene diagnostic model and potential drugs in sepsis using bioinformatics and pharmacogenomics approaches. Infect Drug Resist. (2023) 16:5665–80. doi: 10.2147/IDR.S418176
12. Singer M, Deutschman CS, Seymour CW, Shankar-Hari M, Annane D, Bauer M, et al. The third international consensus definitions for sepsis and septic shock (Sepsis-3). JAMA. (2016) 315:801–10. doi: 10.1001/jama.2016.0287
13. Sygitowicz G, Sitkiewicz D. Molecular mechanisms of organ damage in sepsis: an overview. Braz J Infect Dis. (2020) 24:552–60. doi: 10.1016/j.bjid.2020.09.004
14. Zhang W, Jiang H, Wu G, Huang P, Wang H, An H, et al. The pathogenesis and potential therapeutic targets in sepsis. MedComm (2020). (2023) 4:e418. doi: 10.1002/mco2.418
15. Schulte W, Bernhagen J, Bucala R. Cytokines in sepsis: potent immunoregulators and potential therapeutic targets–an updated view. Mediators Inflammation. (2013) 2013:165974. doi: 10.1155/2013/165974
16. Byron SA, Van Keuren-Jensen KR, Engelthaler DM, Carpten JD, Craig DW. Translating RNA sequencing into clinical diagnostics: opportunities and challenges. Nat Rev Genet. (2016) 17:257–71. doi: 10.1038/nrg.2016.10
17. Stark R, Grzelak M, Hadfield J. RNA sequencing: the teenage years. Nat Rev Genet. (2019) 20:631–56. doi: 10.1038/s41576-019-0150-2
18. Perl M, Chung CS, Swan R, Ayala A. Role of programmed cell death in the immunopathogenesis of sepsis. Drug Discovery Today Dis Mech. (2007) 4:223–30. doi: 10.1016/j.ddmec.2008.02.010
19. Mehra S, Tiwari AK, Aggarwal G, Mehta SP, Chauhan R, Rajvanshi C, et al. Diagnostic value of different interleukins and procalcitonin in critically ill patients admitted with suspected sepsis. Indian J Pathol Microbiol. (2022) 65:111–6. doi: 10.4103/IJPM.IJPM_647_20
20. Zeng G, Chen D, Zhou R, Zhao X, Ye C, Tao H, et al. Combination of C-reactive protein, procalcitonin, IL-6, IL-8, and IL-10 for early diagnosis of hyperinflammatory state and organ dysfunction in pediatric sepsis. J Clin Lab Anal. (2022) 36:e24505. doi: 10.1002/jcla.24505
21. Hu S, Yang J, Yu C, Feng Z, Zhang J, Yin W, et al. IL-6, IL-1β, and IL-10 levels in peripheral blood as indicators for early identification of gram-positive and gram-negative sepsis. Xi Bao Yu Fen Zi Mian Yi Xue Za Zhi. (2021) 37:532–7.
22. Lelubre C, Vincent JL. Mechanisms and treatment of organ failure in sepsis. Nat Rev Nephrol. (2018) 14:417–27. doi: 10.1038/s41581-018-0005-7
23. Martin MD, Badovinac VP, Griffith TS. CD4 T cell responses and the sepsis-induced immunoparalysis state. Front Immunol. (2020) 11:1364. doi: 10.3389/fimmu.2020.01364
24. Harland KL, Day EB, Apte SH, Russ BE, Doherty PC, Turner SJ, et al. Epigenetic plasticity of Cd8a locus during CD8(+) T-cell development and effector differentiation and reprogramming. Nat Commun. (2014) 5:3547. doi: 10.1038/ncomms4547
25. Feuerecker M, Sudhoff L, Crucian B, Pagel JI, Sams C, Strewe C, et al. Early immune anergy towards recall antigens and mitogens in patients at onset of septic shock. Sci Rep. (2018) 8:1754. doi: 10.1038/s41598-018-19976-w
26. de Pablo R, Monserrat J, Prieto A, Alvarez-Mon M. Role of circulating lymphocytes in patients with sepsis. BioMed Res Int. (2014) 2014:671087. doi: 10.1155/2014/671087
27. Liang G, Li J, Pu S, He Z. Screening of sepsis biomarkers based on bioinformatics data analysis. J Healthc Eng. (2022) 2022:6788569. doi: 10.1155/2022/6788569
28. Lu J, Li Q, Wu Z, Zhong Z, Ji P, Li H, et al. Two gene set variation indexes as potential diagnostic tool for sepsis. Am J Transl Res. (2020) 12:2749–59.
29. Vázquez Rodríguez S, Arriaga Pizano LA, Laresgoiti Servitje E, Mancilla Ramirez J, Peralta Méndez OL, Villalobos Alcazar G, et al. Multiparameter flow cytometry analysis of leukocyte markers for diagnosis in preterm neonatal sepsis. J Matern Fetal Neonatal Med. (2021) 34:2323–33. doi: 10.1080/14767058.2019.1666100
30. Tregaskes CA, Kong FK, Paramithiotis E, Chen CL, Ratcliffe MJ, Davison TF, et al. Identification and analysis of the expression of CD8 alpha beta and CD8 alpha alpha isoforms in chickens reveals a major TCR-gamma delta CD8 alpha beta subset of intestinal intraepithelial lymphocytes. J Immunol. (1995) 154:4485–94. doi: 10.4049/jimmunol.154.9.4485
31. Xu Q, Chen Y, Zhao WM, Huang ZY, Zhang Y, Li X, et al. DNA methylation and regulation of the CD8A after duck hepatitis virus type 1 infection. PloS One. (2014) 9:e88023. doi: 10.1371/journal.pone.0088023
32. Dumontet E, Osman J, Guillemont-Lambert N, Cros G, Moshous D, Picard C. Recurrent respiratory infections revealing CD8α Deficiency. J Clin Immunol. (2015) 35:692–5. doi: 10.1007/s10875-015-0213-x
33. Barata JT, Durum SK, Seddon B. Flip the coin: IL-7 and IL-7R in health and disease. Nat Immunol. (2019) 20:1584–93. doi: 10.1038/s41590-019-0479-x
34. Möhnle P, Hirschberger S, Hinske LC, Briegel J, Hübner M, Weis S, et al. MicroRNAs 143 and 150 in whole blood enable detection of T-cell immunoparalysis in sepsis. Mol Med. (2018) 24:54. doi: 10.1186/s10020-018-0056-z
35. Kulkarni U, Herrmenau C, Win SJ, Bauer M, Kamradt T. IL-7 treatment augments and prolongs sepsis-induced expansion of IL-10-producing B lymphocytes and myeloid-derived suppressor cells. PloS One. (2018) 13:e0192304. doi: 10.1371/journal.pone.0192304
36. Thampy LK, Remy KE, Walton AH, Hong Z, Liu K, Liu R, et al. Restoration of T Cell function in multi-drug resistant bacterial sepsis after interleukin-7, anti-PD-L1, and OX-40 administration. PloS One. (2018) 13:e0199497. doi: 10.1371/journal.pone.0199497
37. Almansa R, Heredia-Rodríguez M, Gomez-Sanchez E, Andaluz-Ojeda D, Iglesias V, Rico L, et al. Transcriptomic correlates of organ failure extent in sepsis. J Infect. (2015) 70:445–56. doi: 10.1016/j.jinf.2014.12.010
38. Lou W, Yan J, Wang W. Downregulation of miR-497-5p improves sepsis-induced acute lung injury by targeting IL2RB. BioMed Res Int. (2021) 2021:6624702. doi: 10.1155/2021/6624702
39. Willenbring RC, Johnson AJ. Finding a balance between protection and pathology: the dual role of perforin in human disease. Int J Mol Sci. (2017) 18:1608. doi: 10.3390/ijms18081608
40. Hinrichs C, Kotsch K, Buchwald S, Habicher M, Saak N, Gerlach H, et al. Perioperative gene expression analysis for prediction of postoperative sepsis. Clin Chem. (2010) 56:613–22. doi: 10.1373/clinchem.2009.133876
41. Garzón-Tituaña M, Sierra-Monzón JL, Comas L, Santiago L, Khaliulina-Ushakova T, Uranga-Murillo I, et al. Granzyme A inhibition reduces inflammation and increases survival during abdominal sepsis. Theranostics. (2021) 11:3781–95. doi: 10.7150/thno.49288
42. Uranga-Murillo I, Tapia E, Garzón-Tituaña M, Ramirez-Labrada A, Santiago L, Pesini C, et al. Biological relevance of Granzymes A and K during E. coli sepsis. Theranostics. (2021) 11:9873–83. doi: 10.7150/thno.59418
43. Kurschus FC, Jenne DE. Delivery and therapeutic potential of human granzyme B. Immunol Rev. (2010) 235:159–71. doi: 10.1111/j.0105-2896.2010.00894.x
44. Garzón-Tituaña M, Arias MA, Sierra-Monzón JL, Morte-Romea E, Santiago L, Ramirez-Labrada A, et al. The multifaceted function of granzymes in sepsis: some facts and a lot to discover. Front Immunol. (2020) 11:1054. doi: 10.3389/fimmu.2020.01054
45. Liang S, Xing M, Chen X, Peng J, Song Z, Zou W. Predicting the prognosis in patients with sepsis by a pyroptosis-related gene signature. Front Immunol. (2022) 13:1110602. doi: 10.3389/fimmu.2022.1110602
46. Stern-Ginossar N, Mandelboim O. An integrated view of the regulation of NKG2D ligands. Immunology. (2009) 128:1–6. doi: 10.1111/j.1365-2567.2009.03147.x
47. Cheng X, Cao Y, Wang X, Cheng L, Liu Y, Lei J, et al. Systematic pan-cancer analysis of KLRB1 with prognostic value and immunological activity across human tumors. J Immunol Res. (2022) 2022:5254911. doi: 10.1155/2022/5254911
48. Chheda U, Pradeepan S, Esposito E, Strezsak S, Fernandez-Delgado O, Kranz J. Factors affecting stability of RNA - temperature, length, concentration, pH, and buffering species. J Pharm Sci. (2024) 113:377–85. doi: 10.1016/j.xphs.2023.11.023
49. Bicknell AA, Reid DW, Licata MC, Jones AK, Cheng YM, Li M, et al. Attenuating ribosome load improves protein output from mRNA by limiting translation-dependent mRNA decay. Cell Rep. (2024) 43:114098. doi: 10.1016/j.celrep.2024.114098
Keywords: bioinformatics analysis, CD8A, emergency, GZMA, IL7R, sepsis
Citation: Li J, Wang L, Yu B, Su J and Dong S (2024) IL7R, GZMA and CD8A serve as potential molecular biomarkers for sepsis based on bioinformatics analysis. Front. Immunol. 15:1445858. doi: 10.3389/fimmu.2024.1445858
Received: 14 June 2024; Accepted: 30 October 2024;
Published: 25 November 2024.
Edited by:
Andrzej Lange, Polish Academy of Sciences, PolandReviewed by:
Georgia Damoraki, National and Kapodistrian University of Athens, GreeceYang Yang, Affiliated Hospital of Nantong University, China
Sandra Ampuero, University of Chile, Chile
Copyright © 2024 Li, Wang, Yu, Su and Dong. This is an open-access article distributed under the terms of the Creative Commons Attribution License (CC BY). The use, distribution or reproduction in other forums is permitted, provided the original author(s) and the copyright owner(s) are credited and that the original publication in this journal is cited, in accordance with accepted academic practice. No use, distribution or reproduction is permitted which does not comply with these terms.
*Correspondence: Jin Li, bGlqaW45MHhpYXpoaUAxNjMuY29t; NDkwMDE5ODFAaGVibXUuZWR1LmNu