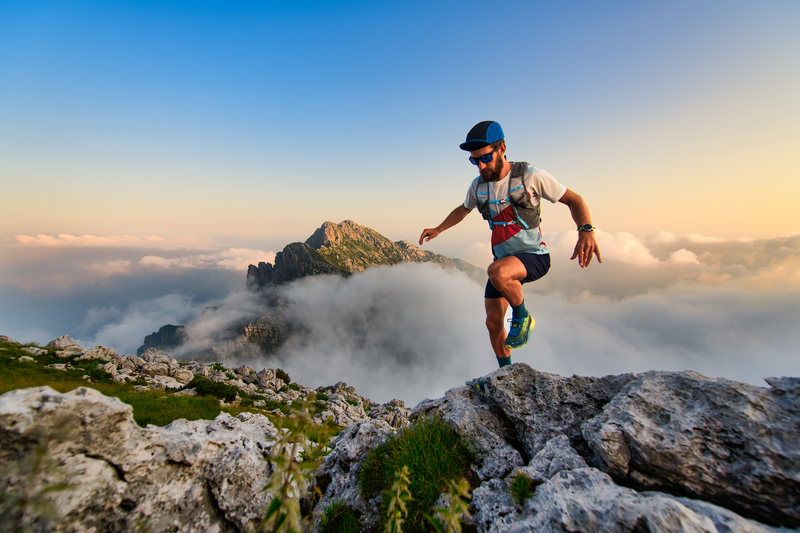
94% of researchers rate our articles as excellent or good
Learn more about the work of our research integrity team to safeguard the quality of each article we publish.
Find out more
BRIEF RESEARCH REPORT article
Front. Immunol. , 25 October 2024
Sec. Molecular Innate Immunity
Volume 15 - 2024 | https://doi.org/10.3389/fimmu.2024.1445638
Background: Primary human neutrophils play a pivotal role in innate immunity, mainly through the formation of neutrophil extracellular traps (NETs) in a process known as NETosis. This cell-death pathway is crucial for combating infections but is also implicated in many inflammatory diseases, such as sepsis, systemic lupus erythematosus, and rheumatoid arthritis.
Methods: The study presented here investigates chromatin dynamics during NET formation by stimulating primary human neutrophils with phorbol 12-myristate 13-acetate (PMA). We adapt the ATAC-Seq (assay for transposase-accessible chromatin using sequencing) method to isolated neutrophils and characterize a time-dependent chromatin response.
Results: We found that chromatin accessibility patterns are consistent across individual donors and most chromatin changes occur within 30 min, with many continuing across the 90 min assessed in this study. Regulatory regions gaining accessibility were associated with the activity of pathways that have been implicated in NOX-dependent NET formation.
Conclusions: Our findings increase the understanding of the chromatin changes underlying NET formation and also identify potential early-acting targets for modulating this process in inflammatory diseases.
Neutrophils are the most abundant white blood cell type in humans and play crucial roles in the innate immune system as one of the first lines of defense against infection (1). In response to pathogens, neutrophils undergo a process called NETosis, which culminates in the release of neutrophil extracellular traps (NETs) composed of chromatin fibers adorned with anti-microbial proteins and proteases that entrap and neutralize a variety of microbial invaders, including bacteria, viruses, and fungi (2–6). Although NET formation represents a crucial defense mechanism, dysregulation of this process contributes to the pathogenesis of diverse inflammatory conditions, including sepsis, systemic lupus erythematosus (SLE), and rheumatoid arthritis (RA) (6). This underscores the delicate balance required for effective immune responses, wherein the precise regulation of NET formation is vital in maintaining immune homeostasis and preventing inflammatory disease progression (7–9). In sepsis, excessive NET formation can cause tissue damage and organ dysfunction; in SLE, neutrophils and aberrant NET formation could be a cause of an increase in anti-dsDNA antibodies; and in RA, aberrant NET formation perpetuates chronic inflammation and joint tissue destruction (10–13). Thus, maintaining the delicate balance of regulating NET formation is crucial for effective immune responses and preventing inflammatory diseases.
Understanding the mechanisms underlying NET formation is critical for identifying biomarkers and developing therapeutic interventions. However, as neutrophils are inherently designed to sense environmental perturbations, they are highly sensitive to external stimuli. Additionally, they have short half-lives and limited survival both in vitro and in vivo, which complicates their study (14). Their viability is further compromised by freezing, making them challenging to work with experimentally. Although neutrophil-like cell lines such as differentiated HL-60 cells have been employed to investigate the regulation of NET formation, these models do not faithfully replicate the behavior of primary neutrophils (15). Moreover, the study of isolated neutrophils fails to capture the complexity of cell-cell interactions and indirect signaling among various immune cell types. Furthermore, findings based on studying NET formation in murine models do not always translate to human biology (16, 17). Investigating NET formation within the context of human primary neutrophils is crucial for translating laboratory-based findings into clinical advancements.
NET formation has been shown to occur via two distinct pathways: dependent or independent of NADPH oxidase (NOX) (1, 18). Phorbol 12-myristate 13-acetate (PMA) stimulation has been shown to be NOX dependent (19), whereas other activators such as calcium ionophore are NOX independent (20). Both the NOX-dependent and -independent NET formation pathways likely contribute to host defense against pathogens, and dysregulation of either pathway can exacerbate inflammatory conditions. For instance, excessive reactive oxygen species (ROS) production in the NOX pathway can lead to tissue damage and inflammation, and the aberrant activation of protein kinases in the non-oxidative pathway can contribute to autoimmune responses and tissue injury (21). Understanding the intricate regulation of these pathways is crucial for developing targeted therapeutic strategies to modulate NET formation and mitigate inflammatory diseases.
Chromatin decondensation studies have investigated the dynamics of chromatin modifications during NET formation, highlighting the importance of histone citrullination by PAD4 in chromatin decondensation and trap formation (22, 23). Other research has explored the impact of chromatin regulators on NET release (7). In this study, we aimed to probe the temporal dynamics of chromatin reorganization during PMA activation of NET formation (18). Leveraging the assay for transposase-accessible chromatin using sequencing (ATAC-Seq) (24) with the incorporation of fixation methods (25), we interrogated the landscape of chromatin accessibility at distinct timepoints following PMA stimulation. By outlining the spatial and temporal trends in chromatin remodeling, we sought to unravel the underlying regulatory networks orchestrating NET formation and identify candidate epigenetic modifiers that may provide answers to therapeutic intervention. We found that chromatin accessibility patterns are consistent across individual donors and that chromatin dynamics were organized across the genome. Finally, the chromatin accessibility patterns highlight key regulatory mechanisms activated during PMA-stimulated NET formation.
Recombinant Tn5 transposase protein (Active Motif #81284) lots were assembled with IDT custom oligos mosaic end (ME) ME_Rev, ME_A, and ME_B, and activity was tested as described previously (24) before initiating ATAC-seq experiments.
Whole blood was obtained from healthy donors (Supplementary Table 1) in K2-EDTA tubes (BD #366643) (PrecisionMed, San Diego, CA, USA). The research was approved under WCG IRB Protocol number 20181025 and all human participants provided written informed consent. Each subject was self-declared healthy, between the ages of 18–50, with a BMI of <30, and not taking NSAIDs. Neutrophil isolation was initiated within 1 h of blood collection using an MACSxpress Whole Blood Neutrophil Isolation Kit for humans (Miltenyl Biotec #130-104-434). Briefly, a fraction of the prepared bead mixture was added to the blood sample, followed by incubation on a rotator for cell binding. Subsequent magnetic separation yielded isolated neutrophils, which were washed in 1× PBS and red blood cell lysis buffer to eliminate contaminants, resulting in >97% pure neutrophils (26) with >98% survivability as measured by Trypan Blue staining on a Countess 3 Cell Counter (Thermo Fisher Scientific). The resulting cell pellet was resuspended in prewarmed Roswell Park Memorial Institute (RPMI) at 37°C for induction or untreated conditions.
Isolated neutrophils were stimulated with 100 nM PMA and imaged using the S3 Live-Cell Analysis System (Sartorious) as described previously (26). For immunocytochemistry, acid-washed 12 mm coverslips (Fisher Scientific, 12-541-026) were placed in a 35 mm dish (Thermo Fisher Scientific, 171099) and coated with 70 µl of 1 mg/ml fibronectin (Sigma-Aldrich, F1141) at 37°C for 1 h. After aspirating fibronectin, the dish was seeded with 2 ml of human neutrophils (20,000 cells/ml). Neutrophils were stimulated with PMA (100 nM) or DMSO (0.1%) for 3 hours at 37°C. Cells were fixed with 4% paraformaldehyde for 10 min and permeabilized with 0.1% Triton X-100 in PBS for 5 min. Coverslips were blocked with 5% donkey serum in PBS + 0.1% Tween 20 overnight at 4°C. After PBS washes, primary antibodies (50 µl) (Supplementary Table 2) were added and incubated overnight at 4°C. Secondary antibodies (Supplementary Table 2) were added after washes and incubated at room temperature for 1 h. Coverslips were stained with DAPI and Cell Mask Orange (Thermo Fisher Scientific, C10045) in PBS for 10 min, washed, rinsed in DI water, and mounted with Fluoromount (Sigma-Aldrich, F4680). Imaging was performed at the Nikon Imaging Center at the University of California, San Diego, using an AXR confocal microscope.
Isolated neutrophils were resuspended in prewarmed (37°C) RPMI 1640 Medium (Gibco Cat #11875135) at a concentration of 1–2 × 106 cells/ml in a final volume of 10 ml and treated with either 100 nM of PMA (Sigma-Aldrich, Cat #P1585) or an equivalent volume of DMSO (ATCC Cat #4-X) for each timepoint. Subsequently, each tube was incubated at 37°C with 5% CO2, and 1 ml aliquots were collected prior to treatment and at 30, 60, and 90 min intervals in duplicate. Each aliquot was fixed as described below for further processing.
A 10× solution of formaldehyde (formaldehyde 11%, NaCl 1M, EDTA 0.1 mM, and HEPES 0.5 mM) was prepared, and a 1:10 (1% final) volume was added to isolated neutrophils for fixation. After incubation at room temperature for 10 min, fixation was quenched with a 1:20 volume of glycine (2.5 M), followed by incubation on ice for 5 min and then centrifugation at 300 × g. Cells were subsequently washed in ice-cold 1× PBS; cells were then pelleted again to retain only intact neutrophils and wash away any potential NET fragments or other DNA. The cells were counted and 125,000 cells per condition/timepoint/replicate were flash frozen in liquid nitrogen and stored at –80°C until further processing.
Unfixed ATAC-Seq was performed as previously reported without major changes (27). The fixed ATAC-Seq protocol was adapted from Buenrostro et al. utilizing information from Chen et al. to determine fixation steps (25, 27), in which each frozen cell pellet (125,000 cells) was resuspended in 50 µl of ATAC RSB Complete [10 mM Tris (pH 7.5), 3 mM MgCl2, 10 mM NaCl, 0.1% NP-40, 0.1% Tween 20, and 0.01% digitonin] and incubated on ice for 3 min. An aliquot of 1 m of cold ATAC RSB NULL [10 mM Tris (pH 7.5), 3 mM MgCl2, 10 mM NaCl, and 0.1% Tween 20] was added, and the mixture was inverted three times before pelleting nuclei at 500 × g for 10 min at 4°C. Supernatant was removed and the pellet was resuspended in Omni-ATAC Mix (28) {1×TD [10 mM Tris (pH 7.5), 5 mM MgCl2, 10% dimethylformamide], 1× PBS, 0.01% digitonin, and 0.01% Tween 20} and assembled Tn5 (3.26 µM) was added to a final volume of 50 µl and incubated at 37°C for 30 min in a thermal cycler and the reaction was stopped by incubating on ice for at least 5 min. ATAC-Seq reverse cross-link buffer [50 mM Tris (pH 8.0), 200 mM NaCl, 1 mM EDTA, and 1% SDS] and proteinase K (1.25 µl) (Invitrogen, #25530049) were subsequently added and incubated at 65°C overnight, and DNA was isolated using Zymo DNA purification (Zymo, #D4013) and eluted in 18.5 µl of pre-warmed 10 mM Tris (pH 8.0).
DNA libraries were generated using NEB Next Ultra II Q5 Master Mix (NEB# M0544L) and amplified by PCR as described previously (27). PCR amplicons were purified by size selection (0.5× followed by 1.2×) using AMPure XP Reagent (Beckman Coulter, #A63881), quantified using a Qubit Flex Fluorometer (Thermo Fisher Scientific, #Q3327), and analyzed using an Agilent TapeStation 4150 with Agilent High Sensitivity D1000 tapes (Agilent, #5067-5594). Libraries were quantified using a Roche KAPA Library Quantification Kit (Roche, #0796014000) and pooled for sequencing on an Illumina NextSeq2500 (Supplementary Table 3).
ATAC-Seq alignment and processing were conducted using an ATAC-Seq Nextflow pipeline (https://nf-co.re/atacseq/2.1.2) (29) with the nf-core framework (30); default settings were used unless otherwise specified. Samples were aligned to the hg38 reference genome with a fragment size parameter set to 200. Peak calling was performed using MACS2 (31), with narrow peaks identified at a false discovery rate (FDR) of 0.01 (macs_fdr=0.01). We followed the general ENCODE current standards for sequencing (32).
Consensus peaks (*.featureCounts.txt) and annotated peaks (*.annotatePeaks.txt) were generated using the nf-core pipeline and utilized for subsequent analysis. To normalize read counts across samples, scaling factors from bigwig normalization (*./bigwig/scale) (https://cran.r-project.org/web/packages/scales/index.html) were applied to balance the total number of reads across samples. For untreated samples, normalized peak counts were combined with annotated peaks to create a consensus peak dataset. UpSetR (https://cran.r-project.org/web/packages/UpSetR/index.html) plots were generated using the *.boolean.annotatePeaks.txt files. Additional metrics, including read counts, peak annotations, bigwigs, fraction of reads in peaks (FRiP) scores, insert sizes, and other alignment metrics, were generated using the nf-core ATAC-Seq pipeline.
Treated samples were analyzed similarly to untreated samples with some modifications. Samples were normalized in the same manner as above and then divided into six groups based on the time course (T30, T60, and T90 min) and treatment (DMSO vs. PMA). To retain the most relevant peaks, we filtered the peaks using the *.boolean.annotatePeaks.txt files. Peaks were retained if at least two-thirds of the donors had a peak within that interval. The peaks were then concatenated, retaining only the unique intervals. The normalized counts for these consensus peaks were analyzed using DESeq2 (33). The DESeqDataSetFromMatrix() function was used to create the dataset, followed by the assay() function for data extraction. Principal component analysis (PCA) scores were calculated using prcomp, and PCA contributions were calculated with the summary() command. Pairwise comparisons were performed with DESeq(), and results were summarized using the results() function. Significant regions (padj < 0.01) were z-score normalized based on the normalized peak counts, and heatmaps were generated using pheatmap (https://cran.r-project.org/web/packages/pheatmap/index.html) with row clustering. Volcano plots were created from the results() function from DESeq2, with significant regions defined by -log10(padj) >20. For all-vs-all comparisons, significant regions from each grouping (treatment and timepoint) with padj <0.01 were included. Pheatmap was then used with both row and column clustering. To analyze overlaps between DMSO Early vs. PMA Early, Mid, and Late, an inner_join (tidyverse) (34) was used, followed by eulerr version 7.0.2 (https://CRAN.R-project.org/package=eulerr) for venn diagram visualization in a custom R script.
To determine whether there was any enrichment of motifs in the gained or lost peaks after PMA stimulation, HOMER (35) analysis was completed on the top 1,000 significant regions based on Wald scoring for all PMA or DMSO treatments (Early, Mid, and Late) using findMotifsGenome.pl with hg38 and standard settings.
To gain a deeper understanding of the chromatin structure of neutrophils, we performed ATAC-Seq with formaldehyde fixation of unstimulated (n=6), PMA-, or DMSO-stimulated (n=3) primary human neutrophils (Figure 1A), and standard (unfixed) ATAC-Seq in one donor (Figure 1B – D32). To assess the open chromatin regions across donors, we looked at genes associated with the activating H3K4me3 modification across various stages of neutrophil development (36) (Figure 1B). We found the expected overlap between identified H3K4me3 regions and ATAC-Seq peaks in our samples and what has been reported for polymorphonuclear neutrophils (PMN) (37), indicating that there is stable organization of accessible chromatin in neutrophils, across donors. Fixed ATAC-Seq samples from donors (D35, D39, D47, D58, D71, and D73) had a higher signal overall than the unfixed sample (D32), as highlighted in the CLEC7A and HCAR loci (Figure 1B). There was also signal in those loci in D32 but the signal to noise was much lower. D32 also had signal present in regions such as AZU1, MPO, and CTSG, which are more associated with promyelocytes and were not represented in the fixed samples (Figure 1B).
Figure 1. Untreated primary human neutrophil ATAC-Seq shows consistent peaks. (A) Experimental schematic. Fresh whole blood was collected, and neutrophils were isolated using an MACSxpress Whole Blood Neutrophil Isolation Kit for humans (Miltenyi Biotec #130-104-434). Neutrophils were then stimulated with phorbol 12-myristate 13-acetate (PMA) or dimethyl sulfoxide (DMSO) for a given time, fixed with formaldehyde, and then used for ATAC-Seq. (B) Merged replicate tracks visualized using the IGV genome browser with untreated healthy donors (n=7), standard ATAC-Seq (D32, top), and fixed samples (D35, D39, D47, D58, D71, and D72) are shown below. The top bars (gray) indicate the stage of neutrophil development at which H3K4me3 signal was present [promyelocytes, band neutrophils (band), segmented, or polymorphonuclear neutrophils (PMNs)]. Housekeeping genes (GAPDH and ACTB) are shown, followed by several other neutrophil genes (AZU1, MPO, CTSG, CEACAM6, C3AR1, CLEC7A, and HCAR1, HCAR2, and HCAR3). (C) Peak annotations generated on called MACS2 peaks (q <0.01) for the fixed donors shown as a percentage. TSS, transcription start site. TTS, transcription termination site. (D) UpSetR plot for the fixed donors showing the intersections of untreated neutrophils. (E) Total number of consensus peaks (y-axis) based on the overlap for the indicated number of donors (x-axis) for the fixed samples (n=6).
We identified peaks using MACS2 (q <0.01) and found that accessibility regions were present across a variety of genomic features with some variability across samples (Figure 1C), which potentially was driven by donor-to-donor differences. We found the peaks were reproducible across the six healthy donors indicated by a high number of consensus peaks. Donors D39, D47, and D58 had a high number of unique peaks (Figure 1D), which may be due to the variable sequencing depth among samples (Supplementary Figure 1A). There was an overall trend of increased FRiP as the number of peaks increased with some variability, which is not unusual when using primary cells (Supplementary Figure 1B). The number of peaks shared across all six fixed donors was 6,930 (Figure 1E). The unfixed sample, D32, had significantly more peaks than the fixed samples (>100,000) and most of the peaks did not correlate with the fixed samples. Of the approximate 7,000 peaks found in all fixed samples, over 81% were also shared with the unfixed donor (Supplementary Figure 1C). To generate a consensus neutrophil peak set across the donors, we set the threshold of the peak being present in at least half of the fixed samples for the peak to be included as a consensus peak. We found that approximately 23,000 peaks were present using this threshold (Figure 1E). These findings suggest a well-structured and regulated chromatin landscape of primary human neutrophils.
To assess chromatin changes associated with PMA-induced NET formation, we treated neutrophils from three donors with PMA or DMSO, collected samples at 30, 60, and 90 min, and subsequently ran ATAC-Seq (Supplementary Figure 2A). These timepoints preceded NET release; therefore, to confirm that these conditions resulted in NET formation we performed live-cell imaging on parallel samples using an extended time course and Cytotox dye intercalation and showed that DNA release began at 2 h (Supplementary Figure 3A) and that NETs contained markers associated with NET formation, such as H3.1 (Supplementary Figure 3B), H3R8cit (Supplementary Figure 3C), myeloperoxidase (MPO) (Supplementary Figure 3D), neutrophil elastase (NE) (Supplementary Figure 3E), and nucleosome (Supplementary Figure 3F).
There was a reproducible chromatin response to PMA stimulation but not with a DMSO vehicle control compared with the untreated samples (Figure 2A). We found consistent chromatin accessibility in the housekeeping genes TBP, RPL32 (Figure 2A), GAPDH, and ACTB (data not shown) throughout the untreated, DMSO, and PMA treatments. Interestingly, peaks were both gained or lost upon PMA stimulation. A gain of peaks generally appeared within 30 min (Early) of stimulation, as highlighted at the RAD9a and KCANB2 loci (Figure 2A). Loss of peaks started to appear at the Early timepoint but were not fully resolved until 60–90 min (Mid-Late). The HCK and TNFAIP6 loci are two examples that showed this reduction of ATAC-Seq signal over the time course reflective the general genome-wide trend (Figure 2A).
Figure 2. PMA generates unique chromatin dynamics compared with DMSO or untreated neutrophils. (A) Merged replicate tracks visualized using the IGV genome browser with untreated healthy donors (n=6, green tracks) and phorbol 12-myristate 13-acetate (PMA)- or dimethyl sulfoxide (DMSO)-treated donors (n=3) at Early (T30 minutes – yellow tracks), Mid (T60 minutes – blue tracks), and Late (T90 minutes – purple tracks). The treated donors D47 (blue), D74 (red), and D75 (gold) are shown at known housekeeping genes: TBP and RPL32. Examples of gained peaks are shown at the RAD9a and KCANB2 loci. Decreased peak examples are shown at the HCK and TNFAIP6 loci. (B) Volcano plot comparing untreated samples with Late DMSO. DESeq2 was used for a pairwise comparison and then plotted. The -log10(p.adj) value is graphed on the y-axis and significant values are plotted in red [-log10(p.adj) <10]. Log2(fold change) is indicated on the x-axis. (C) Similar to Figure 2B but comparing untreated samples vs. Late PMA. (D) Sequencing fragment distribution for the DMSO controls (left) and PMA samples (right) plotted as base pairs (bp) on the x-axis from 0–1,000, grouped into 20 bp windows. The percentage of total reads over 0–1,000 is plotted on the y-axis. Donors are indicated by color (D47, blue; D74, red; D75, gold). Timepoints (Early, Mid, and Late) are graphed per donor.
We found that DMSO-treated samples had a low number of differential accessible regions (DARs) at the most extreme pairwise comparison (DESeq2) of untreated versus Late DMSO (90 min incubation) (Figure 2B). However, there were many DARs between the untreated and the Late PMA treatment across the 3 donors (Figure 2C). Volcano plots showed a much greater response overall in the PMA comparison in both the p-values present and the fold change. As a result, we used Early-DMSO-treated samples as the analysis control.
Interestingly, we observed a shift toward mono- and di-nucleosome fragments after PMA treatment at all timepoints (Figure 2D), indicating a dynamic chromatin response. Despite the nucleosome pattern, PMA stimulation did not have an impact on peak localization (Supplementary Figure 2B) as there was a larger impact from donors than from the stimulation condition or timepoint. The number of unique peaks varied throughout the time course for all donors (Supplementary Figure 2C). To minimize the impact of peak number at a specific timepoint and treatment, a peak was only retained if it was present in two of the three donors and was used in subsequent analyses.
To determine whether PMA stimulation responses were unique compared with DMSO, we performed unsupervised clustering PCA comparing PMA with DMSO using unmerged replicates (Figure 3A). The first principal component (PC1) accounted for 65% of the proportion of variance between PMA treatment and DMSO vehicle controls, and PC2 accounted for 10.4% of the variance and separated each group based on time (Early, Mid, and Late). Importantly, we did not observe any obvious donor batch effects (Figure 3A).
Figure 3. PMA stimulation causes a genome-wide alteration of chromatin accessibility across donors and time. (A) Principal component analysis (PCA) based on unbiased clustering of dimethyl sulfoxide (DMSO) vs. phorbol 12-myristate 13-acetate (PMA) using the unmerged data. Timepoints are indicated by color (Early, gold; Mid, blue; Late, purple). Treatment is indicated by squares (DMSO) or circles (PMA). PC1 (x-axis) represented 65% of the total variance and PC2 (y-axis) represented 10.4% of variance. Donors are indicated by the number adjacent to the data point. (B) Merged replicate tracks visualized with the IGV genome browser with Early DMSO (black), Early PMA (gold), Mid PMA (blue), and Late PMA (purple) at various gene loci. Donors are indicated by color (D47, blue; D74, red; D75, gold). Significant differential accessible regions based on Early DMSO vs. each timepoint [DESeq2 p.adj >0.01 and log2(fold change) less than −1.5 or greater than 1.5] are shown below the genes, where gained peaks are shown in red and loss of peaks are shown in blue. The bimodal response is shown at ACTG1 and RAB7A, increased accessibility at PRKCB, RPLP2, and PNPLA2, and decreased accessibility at CYTIP, RGS2, and ALOX5. (C) Heatmap showing the number of differential accessible regions (DARs) between all pairwise comparisons (each timepoint for each treatment condition) [DESeq2 p.adj >0.01 and log2(fold change) less than −1.5 or greater than 1.5]. (D) Venn diagram of the overlaps between Early DMSO and the PMA treatments (Early, gold; Mid, blue; Late, purple). The total number of DARs in each condition is indicated. (E) Z-score normalized heatmap representation of the Early DMSO vs. PMA treatments (Early, left; Mid, middle; Late, right). Donors are indicated by color (D47, blue; D74, red; D75, gold). Timepoints (Early, Mid, and Late) are graphed per donor. Treatment is indicated by DMSO (blue) and PMA (red). The top 1,000 DARs were selected based on the Wald score. Columns are organized by treatment, timepoint, and donor across all conditions, and rows are hierarchically clustered.
There are several examples of chromatin accessibility changes in inflammatory genes or genes known to be important in neutrophil functions (Figure 3B), with a few different modes of chromatin accessible changes observed. Regions, such as ACTG1 and RAB7a, were more accessible in the Early and into the Mid stage, followed by less accessible at the Late timepoint. We found some instances of increased accessibility at intragenic (PRKCB) or transcription start sites (RPLP2 and PNPLA2). Additionally, there were regions that had a reduction in peaks (CYTIP, RGS2, and ALOX5) (Figure 3B). These represent the dynamic and structured chromatin response seen genome-wide during PMA activation across donors.
We next performed pairwise comparisons (DESeq2) between every grouping (each timepoint and treatment) and calculated the total number of differentially accessible regions (DARs) (q <0.01). The DARs highlighted large differences between the PMA and DMSO groupings but very minor differences within treatment groups (Figure 3C). Owing to limited variability in the DMSO treatment group, future comparisons used only the Early DMSO as the control. Overlapping DARs between the PMA groups (Early, Mid, and Late) were identified using a q-value <0.01 and a log2 fold change greater than 1.5 or less than −1.5 (Figure 3D). Almost 40% (1,121/2,922) of the DARs were sustained in at least two of the timepoints, with the majority of regions being found in the Early or Mid timepoints. These data suggest an initial response at 30 min, followed by a secondary response at 60 min. After 60 min, there were less than 5% (117/2,922) unique changes (Figure 3D). Next, pairwise comparisons of the Early DMSO vs. each PMA treatment time were made. The top 1,000 DARs were z-score normalized and plotted as a heatmap (Figure 3E). The results suggest the stability of the DARs over time and across donors over each comparison.
To obtain the highest significant DARs based on their Wald test score, the top 15% (500) upregulated in Early DMSO and the top 500 DARs for all PMA timepoints were selected (Supplementary Table 4). The DARs were then z-score normalized and ordered based on treatment and time (Figure 4A). There was some variability in the PMA DARs, with the largest differences being between Early PMA and Mid PMA. The data were further reduced to the top 1.5% DARs (50) in DMSO or PMA to highlight the largest differences between the treatments and timepoints. There were numerous DARs that appeared Early and then were lost as well as the opposite of a lack of signal increasing into the Mid timepoint (Figure 4A – right). These data also support the similarities between the Mid and Late PMA treatments.
Figure 4. PMA-induced NET formation leads to increased accessibility in RAS-associated transcription factors. (A) Z-score normalized heatmap representation of the top 500 (left panel) differential accessible regions (DARs) for each Early dimethyl sulfoxide (DMSO) and phorbol 12-myristate 13-acetate (PMA) based on the Wald score [DESeq2 p.adj >0.01 and log2(fold change) less than −1.5 or greater than 1.5]. The top 100 DARs (right panel) were then filtered by the Wald score (50 upregulated in DMSO and 50 upregulated in PMA). Donors are indicated by color (D47, blue; D74, red; D75, gold). Timepoints (Early, Mid, and Late) are graphed per donor. Treatment is indicated by DMSO (blue) and PMA (red). Columns are organized by treatment, timepoint, and then donor across all conditions, and rows are hierarchically clustered. (B) HOMER analysis from the top 1,000 upregulated DARs in PMA samples [DESeq2 p.adj > 0.01 and log2(fold change) >1.5]. (C) Similar to Figure 4B but upregulated in DMSO samples [log2(fold change) <1.5]. (D) A potential mechanism for transcription factor (TF) activation through PMA-activated NET formation. Briefly, PMA activates PKC, which causes activation of NADPH and NOX through RhoG to generate an increase in intracellular reactive oxygen species (ROS). ROS then activate the RAS/RAF pathway and lead to the downstream activation of TFs such as AP-1, JUN/FOS, FRA1, FRA2, and ATF3. There is no increased calcium level caused by PKC activation; therefore, PAD4, NFAT, and NF-κB are not activated.
The top 1,000 DARs from DMSO or PMA were then used for HOMER analysis (Figures 4B, C). In the PMA DARs, TFs previously associated with NET formation or inflammation, such as FRA1 (38), FRA2 (18), JUN/FOS (18), ATF3 (39), and AP-1 (18), were among the more prevalent (Figures 4B, D). CTCF was also present in 4.4% of the target sequences of the PMA DARs, which indicated a potential role of CTCF in the regulation of the chromatin response; however, CTCF is often overrepresented in HOMER analysis (40), therefore future studies should probe the potential impact of CTCF in chromatin regulation during NET formation. In DMSO DARs, there was not a strong correlation to NET-formation-associated TF binding sites or many direct pathways (Figure 4C). However, one of the top HOMER results was from the ELF family, which has been associated with autoimmune disease, and the reduction in ELF4 in macrophages has been correlated to increased neutrophilic infiltration (41, 42).
Overall, TF binding sites associated with PAD4, such as NF-AT and NF-κb, were not well represented in upregulated or downregulated DARs (Figures 4B, C), which could be modulated by a lack of intracellular calcium or other mechanistic reasons (Figure 4D). The representation of RAS-associated TFs seen in Figure 4B support previous findings of RAS activation through PMA stimulation through NOX dependency (18, 43). These results highlight the importance of these TFs and their potential roles in chromatin regulation during NET formation (41).
NET formation is a key component of the innate immune response, playing a crucial role in trapping and neutralizing pathogens. However, this process can become dysregulated and lead to sepsis and immunothrombotic diseases (44, 45). The transition from a beneficial to a pathogenic response is not well understood but may be driven by an excess of pro-inflammatory cytokines, inefficiencies in inhibitory molecules such as IL-10 (46), or repressive mechanisms. Additionally, the release of NET components, particularly chromatin, can contribute to cytotoxicity, exacerbating the pathological state. Therapeutics targeting NET formation have been challenging and not widely approved in clinical settings (47), indicating that a deeper understanding of the underlying mechanisms is likely needed to develop effective treatments. The molecular processes occurring during NET formation might vary significantly under (patho)physiological conditions and thus deciphering the molecular choreography underlying NET formation could provide important insights into these mechanisms governing both host defense and inflammation-associated pathology. Changes in chromatin architecture are an important early step in altering gene expression and initiating cellular regulatory programs, likely dictating the fate of neutrophils and shaping the ensuing immune response.
We used PMA to study chromatin accessibility during NET formation due to its robust and consistent induction of NETs. Unlike lipopolysaccharide (LPS) or other more physiologically relevant stimuli, PMA directly activates protein kinase C (PKC), leading to a highly reproducible and synchronous activation of downstream signaling pathways, including the RAS/RAF/MEK/ERK cascade, which are crucial for some types of NET formation (6). This consistency is important in generating uniformity in cell activation to ensure the observed changes in chromatin accessibility were due to the induced biological process rather than variability in stimulus response. PMA-induced NET formation involves the production of ROS and other signaling molecules, providing a well-characterized model to dissect the molecular mechanisms underlying chromatin remodeling. Furthermore, as little is known about how chromatin structure is changed during the early stages of NET formation, we chose to perform a focused characterization of chromatin changes, which can be utilized to interpret the bulk of the existing literature using PMA induction. Future studies will investigate whether the chromatin accessibility changes described here are specific to PMA-induced NET formation or are common to other NET inducing stimuli.
We have previously shown NET formation in isolated neutrophils with 100 nM PMA and have developed ATAC-Seq using the same stimulation conditions with an added formaldehyde fixation at specific timepoints to maintain chromatin structure within the nucleus and avoid NET release (Supplementary Figure 3F) (26). Although 100 nM PMA is a high concentration in isolated conditions, we wanted to ensure a rapid response of the entire population of cells to ensure uniform results during ATAC-Seq. Overall, we noticed an increase in noise and a lack of overlap of peaks being called between the standard and fixed ATAC-Seq samples (Supplementary Figures 1B, C). We have found that accessible chromatin regions in fixed samples were consistent across multiple donors, similar to other primary immune cell types (28, 48). We observed some donor-to-donor variability, but we generated a set of over 22,000 consensus peaks that were shared among 50% of the donors tested.
PMA is a strong inducer of NET formation through the activation of PKC, which leads to increased intracellular ROS through NADPH oxidase (NOX) (18, 19). We used our ATAC-Seq method to study PMA-induced neutrophils, first showing that DMSO-treated neutrophils were similar to untreated samples for up to 90 min post-treatment (Figure 2B). When neutrophils were treated with PMA, there was a consistent response across donors at multiple timepoints, suggesting an ordered and mechanistic regulatory program. A unique change in fragment profile could be seen as early as 30 min (Figure 2D), suggesting that chromatin re-organization happens before NET production, which can take several hours following PMA stimulation (26).
In concert with this early fragmentation change, we identified approximately 1,500 DARs that occurred between PMA- and DMSO-treated cells at 30 min, and the majority of these remained so up to 90 min. Globally, PMA-treated samples clustered away from DMSO-treated samples in an unsupervised clustering analysis (PCA), with sub-clustering by timepoint among the PMA-treated samples and limited donor effects. Protein Kinase C - β (PRKCB) has previously been shown to be upregulated during PMA stimulation (19, 49) and showed a stable increase in chromatin accessibility. More complex chromatin accessibility changes across the time course were evident at loci such as ACTG1, which has been shown to have an initial burst of transcription at 30 min followed by a reduction at 60 min in PMA-induced neutrophils (6), which corresponded to more accessible chromatin at 30 min followed by a reduction in accessibility by 60 min (Figure 3B).
Interestingly, we observed a gain of accessibility at DARs containing binding sites for the transcription factors JUN/FOS, FRA1, FRA2, ATF3, and AP-1 (18, 38, 39, 49–51) (Figure 4B). Upon PMA stimulation, an increase of ROS intracellularly can lead to various effects including the activation of the RAS pathway and activation of these same TFs, but not other TFs such as PAD4, NF-AT, or NF-kB (18, 19, 38, 52). There was not a strong signal for TFs associated with reduced chromatin accessibility, with the most significant hits occurring in the ELF TF family, which is known to be involved with many processes, including cell proliferation (53). A potential reason for a weaker signal in TFs associated with the loss of accessibility could be that the decondensation of the chromatin is targeted through a variety of TFs or another mechanism. The transcription factor motif findings support previous data that PMA-stimulated neutrophils act via a NOX-dependent mechanism, which activates the RAS pathway (Figure 4D), as opposed to when NET formation is activated through the NOX-independent pathway, which leads to the activation of PAD4 and subsequently NFAT and NF-kB (8, 19, 49). Importantly, we did not identify NFAT or NF-kB binding sites in our HOMER analysis of the top 1,000 DARs (Figures 4B, C).
Our findings deepen our comprehension of the epigenetic blueprint governing PMA-stimulated NET formation, and understanding the changes in chromatin accessibility may hold significant clinical implications, potentially leading to more targeted therapies. Such organized changes could reveal consistent epigenetic modifications and transcriptional programs essential for NET formation and immune response, highlighting potential therapeutic targets for diseases characterized by aberrant NET formation. The targets could lead to the development of drugs aimed at specific genes involved in pathological NET formation or the modulation of feedback loops. Additionally, understanding how PMA-induced chromatin changes compare with those triggered by more physiologically relevant stimuli, such as LPS or naturally occurring cytokines, could provide insights into the broader landscape of neutrophil epigenetics and the molecular mechanisms underlying NET formation. This knowledge can further elucidate how various stimuli influence physiological epigenetic processes, such as differentiation, activation, and response to pathogens, to offer a comprehensive view of neutrophil function in health and disease.
The original contributions presented in the study are publicly available. This data can be found here: https://www.ncbi.nlm.nih.gov/bioproject/?term=PRJNA1120432.
The studies involving humans were approved by WCG IRB Protocol number 20181025. The studies were conducted in accordance with the local legislation and institutional requirements. The participants provided their written informed consent to participate in this study.
BA: Formal analysis, Investigation, Methodology, Writing – original draft, Writing – review & editing, Data curation. BB: Conceptualization, Formal analysis, Methodology, Writing – review & editing. TK: Conceptualization, Formal analysis, Methodology, Validation, Writing – original draft, Writing – review & editing, Data curation. JC: Conceptualization, Formal analysis, Methodology, Validation, Writing – original draft, Writing – review & editing, Data curation, Supervision.
The author(s) declare financial support was received for the research, authorship, and/or publication of this article. This work was funded by VolitionRx. The funder was not involved in the study design, data collection, analysis, and interpretation of data, as well as in the writing of this article and the decision to submit it for publication.s
We would like to thank Finley Serneo for his contribution to the manuscript with his work on NET Imaging and Immunocytochemistry. We would also like to thank Sarah Erdman, Akanksha Singh-Taylor, and Kieran Zukas, as well as the broader scientific team at Volition, for their helpful review of the data and discussion. ChatGPT version 4o was used to support the writing of R codes and minor edits throughout the manuscript. This manuscript was previously published as a preprint on bioRxiv (54).
All authors are employees or contractors for VolitionRx. BA, JC, BB, and TK hold stock in VolitionRx.
All claims expressed in this article are solely those of the authors and do not necessarily represent those of their affiliated organizations, or those of the publisher, the editors and the reviewers. Any product that may be evaluated in this article, or claim that may be made by its manufacturer, is not guaranteed or endorsed by the publisher.
The Supplementary Material for this article can be found online at: https://www.frontiersin.org/articles/10.3389/fimmu.2024.1445638/full#supplementary-material
1. Schoen J, Euler M, Schauer C, Schett G, Herrmann M, Knopf J, et al. Neutrophils’ Extracellular trap mechanisms: from physiology to pathology. Int J Mol Sci. (2022) 23(21):12855. doi: 10.3390/ijms232112855
2. Kanthack AA, Hardy WB. The morphology and distribution of the wandering cells of mammalia. J Physiol. (1894) 17:80.1–119. doi: 10.1113/jphysiol.1894.sp000520
3. Hardy SJ, Robinson BS, Poulos A, Harvey DP, Ferrante A, Murray AW. The neutrophil respiratory burst. Responses to fatty acids, N-formylmethionylleucylphenylalanine and phorbol ester suggest divergent signalling mechanisms. Eur J Biochem. (1991) 198:801–6. doi: 10.1111/j.1432-1033.1991.tb16084.x
4. Brinkmann V, Reichard U, Goosmann C, Fauler B, Uhlemann Y, Weiss DS, et al. Neutrophil extracellular traps kill bacteria. Science. (2004) 303:1532–5. doi: 10.1126/science.1092385
5. Dejas L, Santoni K, Meunier E, Lamkanfi M. Regulated cell death in neutrophils: From apoptosis to NETosis and pyroptosis. Semin Immunol. (2023) 70:101849. doi: 10.1016/j.smim.2023.101849
6. Boeltz S, Amini P, Anders HJ, Andrade F, Bilyy R, Chatfield S, et al. To NET or not to NET:current opinions and state of the science regarding the formation of neutrophil extracellular traps. Cell Death Differ. (2019) 26:395–408. doi: 10.1038/s41418-018-0261-x
7. Poli V, Zanoni I. Neutrophil intrinsic and extrinsic regulation of NETosis in health and disease. Trends Microbiol. (2023) 31:280–93. doi: 10.1016/j.tim.2022.10.002
8. Huang J, Hong W, Wan M, Zheng L. Molecular mechanisms and therapeutic target of NETosis in diseases. MedComm (2020). (2022) 3:e162. doi: 10.1002/mco2.v3.3
9. Mistry P, Nakabo S, O’Neil L, Goel RR, Jiang K, Carmona-Rivera C, et al. Transcriptomic, epigenetic, and functional analyses implicate neutrophil diversity in the pathogenesis of systemic lupus erythematosus. Proc Natl Acad Sci U S A. (2019) 116:25222–8. doi: 10.1073/pnas.1908576116
10. Kumar S, Payal N, Srivastava VK, Kaushik S, Saxena J, Jyoti A. Neutrophil extracellular traps and organ dysfunction in sepsis. Clin Chim Acta. (2021) 523:152–62. doi: 10.1016/j.cca.2021.09.012
11. Kaplan MJ. Neutrophils in the pathogenesis and manifestations of SLE. Nat Rev Rheumatol. (2011) 7:691–9. doi: 10.1038/nrrheum.2011.132
12. Han BK, Wysham KD, Cain KC, Tyden H, Bengtsson AA, Lood C. Neutrophil and lymphocyte counts are associated with different immunopathological mechanisms in systemic lupus erythematosus. Lupus Sci Med. (2020) 7. doi: 10.1136/lupus-2020-000382
13. Song W, Ye J, Pan N, Tan C, Herrmann M. Neutrophil extracellular traps tied to rheumatoid arthritis: points to ponder. Front Immunol. (2020) 11:578129. doi: 10.3389/fimmu.2020.578129
14. Thiam HR, Wong SL, Wagner DD, Waterman CM. Cellular mechanisms of NETosis. Annu Rev Cell Dev Biol. (2020) 36:191–218. doi: 10.1146/annurev-cellbio-020520-111016
15. Babatunde KA, Wang X, Hopke A, Lannes N, Mantel PY, Irimia D. Chemotaxis and swarming in differentiated HL-60 neutrophil-like cells. Sci Rep. (2021) 11:778. doi: 10.1038/s41598-020-78854-6
16. Seok J, Warren HS, Cuenca AG, Mindrinos MN, Baker HV, Xu W, et al. Genomic responses in mouse models poorly mimic human inflammatory diseases. Proc Natl Acad Sci U S A. (2013) 110:3507–12. doi: 10.1073/pnas.1222878110
17. Nauseef WM. Human neutrophils ≠ murine neutrophils: Does it matter? Immunol Rev. (2023) 314:442–56. doi: 10.1111/imr.13154
18. Khan MA, Palaniyar N. Transcriptional firing helps to drive NETosis. Sci Rep. (2017) 7:41749. doi: 10.1038/srep41749
19. Damascena HL, Silveira WAA, Castro MS, Fontes W. Neutrophil activated by the famous and potent PMA (Phorbol myristate acetate). Cells. (2022) 11(18):2889. doi: 10.3390/cells11182889
20. Parker H, Dragunow M, Hampton MB, Kettle AJ, Winterbourn CC. Requirements for NADPH oxidase and myeloperoxidase in neutrophil extracellular trap formation differ depending on the stimulus. J Leukoc Biol. (2012) 92:841–9. doi: 10.1189/jlb.1211601
21. Nguyen GT, Green ER, Mecsas J. Neutrophils to the ROScue: mechanisms of NADPH oxidase activation and bacterial resistance. Front Cell Infect Microbiol. (2017) 7:373. doi: 10.3389/fcimb.2017.00373
22. Leshner M, Wang S, Lewis C, Zheng H, Chen XA, Santy L, et al. PAD4 mediated histone hypercitrullination induces heterochromatin decondensation and chromatin unfolding to form neutrophil extracellular trap-like structures. Front Immunol. (2012) 3:307. doi: 10.3389/fimmu.2012.00307
23. Rohrbach AS, Slade DJ, Thompson PR, Mowen KA. Activation of PAD4 in NET formation. Front Immunol. (2012) 3:360. doi: 10.3389/fimmu.2012.00360
24. Buenrostro JD, Giresi PG, Zaba LC, Chang HY, Greenleaf WJ. Transposition of native chromatin for fast and sensitive epigenomic profiling of open chromatin, DNA-binding proteins and nucleosome position. Nat Methods. (2013) 10:1213–8. doi: 10.1038/nmeth.2688
25. Chen X, Shen Y, Draper W, Buenrostro JD, Litzenburger U, Cho SW, et al. ATAC-see reveals the accessible genome by transposase-mediated imaging and sequencing. Nat Methods. (2016) 13:1013–20. doi: 10.1038/nmeth.4031
26. Zukas K, Cayford J, Serneo F, Atteberry B, Retter A, Eccleston M, et al. Rapid high-throughput method for investigating physiological regulation of neutrophil extracellular trap formation. J Thromb Haemost. (2024) 22(9):2543–54. doi: 10.1016/j.jtha.2024.05.028
27. Buenrostro JD, Wu B, Chang HY, Greenleaf WJ. ATAC-seq: A method for assaying chromatin accessibility genome-wide. Curr Protoc Mol Biol. (2015) 109:21.9.1–.9.9. doi: 10.1002/0471142727.2015.109.issue-1
28. Grandi FC, Modi H, Kampman L, Corces MR. Chromatin accessibility profiling by ATAC-seq. Nat Protoc. (2022) 17:1518–52. doi: 10.1038/s41596-022-00692-9
29. Patel HE-C, J., Langer B, Ewels P, nf-core bot, Garcia MU, Syme R, Peltzer A, et al. An O. nf-core/atacseq: [2.1.2] - 2022-08-07 (2.1.2). Zenodo (2023). doi: 10.5281/zenodo.2634132
30. Ewels PA, Peltzer A, Fillinger S, Patel H, Alneberg J, Wilm A, et al. The nf-core framework for community-curated bioinformatics pipelines. Nat Biotechnol. (2020) 38:276–8. doi: 10.1038/s41587-020-0439-x
31. Zhang Y, Liu T, Meyer CA, Eeckhoute J, Johnson DS, Bernstein BE, et al. Model-based analysis of ChIP-Seq (MACS). Genome Biol. (2008) 9:R137. doi: 10.1186/gb-2008-9-9-r137
32. Landt SG, Marinov GK, Kundaje A, Kheradpour P, Pauli F, Batzoglou S, et al. ChIP-seq guidelines and practices of the ENCODE and modENCODE consortia. Genome Res. (2012) 22:1813–31. doi: 10.1101/gr.136184.111
33. Love MI, Huber W, Anders S. Moderated estimation of fold change and dispersion for RNA-seq data with DESeq2. Genome Biol. (2014) 15:550. doi: 10.1186/s13059-014-0550-8
34. Wickham H, Averick M, Bryan J, Chang W, McGowan LDA, François R, et al. Welcome to the Tidyverse. J Open Source Softw. (2019) 4:1686. doi: 10.21105/joss.01686
35. Heinz S, Benner C, Spann N, Bertolino E, Lin YC, Laslo P, et al. Simple combinations of lineage-determining transcription factors prime cis-regulatory elements required for macrophage and B cell identities. Mol Cell. (2010) 38:576–89. doi: 10.1016/j.molcel.2010.05.004
36. Fridlich O, Peretz A, Fox-Fisher I, Pyanzin S, Dadon Z, Shcolnik E, et al. Elevated cfDNA after exercise is derived primarily from mature polymorphonuclear neutrophils, with a minor contribution of cardiomyocytes. Cell Rep Med. (2023) 4:101074. doi: 10.1016/j.xcrm.2023.101074
37. Bermick JR, Issuree P, denDekker A, Gallagher KA, Santillan D, Kunkel S, et al. Differences in H3K4me3 and chromatin accessibility contribute to altered T-cell receptor signaling in neonatal naïve CD4 T cells. Immunol Cell Biol. (2022) 100:562–79. doi: 10.1111/imcb.v100.7
38. Bhosale PB, Kim HH, Abusaliya A, Vetrivel P, Ha SE, Park MY, et al. Structural and functional properties of activator protein-1 in cancer and inflammation. Evid Based Complement Alternat Med. (2022) 2022:9797929. doi: 10.1155/2022/9797929
39. Liu S, Li Z, Lan S, Hao H, Baz AA, Yan X, et al. The dual roles of activating transcription factor 3 (ATF3) in inflammation, apoptosis, ferroptosis, and pathogen infection responses. Int J Mol Sci. (2024) 25(2):824. doi: 10.3390/ijms25020824
40. Khoury A, Achinger-Kawecka J, Bert SA, Smith GC, French HJ, Luu PL, et al. Constitutively bound CTCF sites maintain 3D chromatin architecture and long-range epigenetically regulated domains. Nat Commun. (2020) 11:54. doi: 10.1038/s41467-019-13753-7
41. Tyler PM, Bucklin ML, Zhao M, Maher TJ, Rice AJ, Ji W, et al. Human autoinflammatory disease reveals ELF4 as a transcriptional regulator of inflammation. Nat Immunol. (2021) 22:1118–26. doi: 10.1038/s41590-021-00984-4
42. Cao M, Chen P, Peng B, Cheng Y, Xie J, Hou Z, et al. The transcription factor ELF4 alleviates inflammatory bowel disease by activating IL1RN transcription, suppressing inflammatory TH17 cell activity, and inducing macrophage M2 polarization. Front Immunol. (2023) 14:1270411. doi: 10.3389/fimmu.2023.1270411
43. Jin Y, Dixon B, Jones L, Gorbet M. The differential reactive oxygen species production of tear neutrophils in response to various stimuli in vitro. Int J Mol Sci. (2021) 22(23):12899. doi: 10.3390/ijms222312899
44. Nakamori Y, Park EJ, Shimaoka M. Immune deregulation in sepsis and septic shock: reversing immune paralysis by targeting PD-1/PD-L1 pathway. Front Immunol. (2020) 11:624279. doi: 10.3389/fimmu.2020.624279
45. Klavina PA, Leon G, Curtis AM, Preston RJS. Dysregulated haemostasis in thrombo-inflammatory disease. Clin Sci (Lond). (2022) 136:1809–29. doi: 10.1042/CS20220208
46. Saitoh T, Komano J, Saitoh Y, Misawa T, Takahama M, Kozaki T, et al. Neutrophil extracellular traps mediate a host defense response to human immunodeficiency virus-1. Cell Host Microbe. (2012) 12:109–16. doi: 10.1016/j.chom.2012.05.015
47. Chamardani TM, Amiritavassoli S. Inhibition of NETosis for treatment purposes: friend or foe? Mol Cell Biochem. (2022) 477:673–88. doi: 10.1007/s11010-021-04315-x
48. Benaglio P, Newsome J, Han JY, Chiou J, Aylward A, Corban S, et al. Mapping genetic effects on cell type-specific chromatin accessibility and annotating complex immune trait variants using single nucleus ATAC-seq in peripheral blood. PloS Genet. (2023) 19:e1010759. doi: 10.1371/journal.pgen.1010759
49. Chen T, Li Y, Sun R, Hu H, Liu Y, Herrmann M, et al. Receptor-mediated NETosis on neutrophils. Front Immunol. (2021) 12:775267. doi: 10.3389/fimmu.2021.775267
50. Whitmarsh AJ, Davis RJ. Transcription factor AP-1 regulation by mitogen-activated protein kinase signal transduction pathways. J Mol Med (Berl). (1996) 74:589–607. doi: 10.1007/s001090050063
51. Liang G, Wolfgang CD, Chen BP, Chen TH, Hai T. ATF3 gene. Genomic organization, promoter, and regulation. J Biol Chem. (1996) 271:1695–701. doi: 10.1074/jbc.271.3.1695
52. Son Y, Cheong YK, Kim NH, Chung HT, Kang DG, Pae HO. Mitogen-activated protein kinases and reactive oxygen species: how can ROS activate MAPK pathways? J Signal Transduct. (2011) 2011:792639. doi: 10.1155/2011/792639
53. Hsu T, Trojanowska M, Watson DK. Ets proteins in biological control and cancer. J Cell Biochem. (2004) 91:896–903. doi: 10.1002/jcb.20012
Keywords: NETosis, neutrophil, ATAC-seq, chromatin, PMA, sepsis, innate immune system, NET formation
Citation: Atteberry B, Berman BP, Kelly TK and Cayford J (2024) Understanding the complex chromatin dynamics in primary human neutrophils during PMA-induced NET formation. Front. Immunol. 15:1445638. doi: 10.3389/fimmu.2024.1445638
Received: 07 June 2024; Accepted: 26 September 2024;
Published: 25 October 2024.
Edited by:
Johan Van Der Vlag, Radboud University, NetherlandsReviewed by:
Elmar Pieterse, Radboud University Medical Centre, NetherlandsCopyright © 2024 Atteberry, Berman, Kelly and Cayford. This is an open-access article distributed under the terms of the Creative Commons Attribution License (CC BY). The use, distribution or reproduction in other forums is permitted, provided the original author(s) and the copyright owner(s) are credited and that the original publication in this journal is cited, in accordance with accepted academic practice. No use, distribution or reproduction is permitted which does not comply with these terms.
*Correspondence: Justin Cayford, ai5jYXlmb3JkQHZvbGl0aW9uLmNvbQ==
Disclaimer: All claims expressed in this article are solely those of the authors and do not necessarily represent those of their affiliated organizations, or those of the publisher, the editors and the reviewers. Any product that may be evaluated in this article or claim that may be made by its manufacturer is not guaranteed or endorsed by the publisher.
Research integrity at Frontiers
Learn more about the work of our research integrity team to safeguard the quality of each article we publish.