- 1Department of Respiratory Medicine, Nanjing Chest Hospital, Affiliated Nanjing Brain Hospital, Nanjing Medical University, Nanjing, China
- 2Duke Cancer Institute, Duke University Medical Center, Durham, NC, United States
- 3Department of Population Health Sciences, Duke University School of Medicine, Durham, NC, United States
- 4Department of Respiratory Oncology, Guangxi Cancer Hospital, Guangxi Medical University Cancer Hospital, Nanning, China
- 5Department of Head and Neck Surgery, Guangxi Cancer Hospital, Guangxi Medical University Cancer Hospital, Nanning, China
- 6Department of Biostatistics and Bioinformatics, Duke University School of Medicine, Durham, NC, United States
- 7Departments of Environmental Health and Epidemiology, Harvard TH Chan School of Public Health, Boston, MA, United States
- 8Department of Medicine, Massachusetts General Hospital, Boston, MA, United States
- 9Department of Medicine, Duke University Medical Center, Durham, NC, United States
- 10Duke Global Health Institute, Duke University Medical Center, Durham, NC, United States
B cells are adaptive immune cells in the tumor microenvironment and play an important role in tumor development and metastasis. However, the roles of genetic variants of the immunity B cell-related genes in the survival of patients with non-small cell lung cancer (NSCLC) remain unknown. In the present study, we first evaluated associations between 10,776 single nucleotide polymorphisms (SNPs) in 220 immunity B cell-related genes and survival of NSCLC in a discovery dataset of 1,185 patients from the Prostate, Lung, Colorectal and Ovarian (PLCO) Cancer Screening Trial. We found that 369 SNPs were significantly associated with overall survival (OS) of NSCLC in multivariable Cox proportional hazards regression analysis (P ≤ 0.05, Bayesian false discovery probability ≤ 0.80), of which 18 SNPs were validated in another independent genotyping dataset of 984 patients from the Harvard Lung Cancer Susceptibility (HLCS) Study. We then performed linkage disequilibrium (LD) analysis, followed by stepwise analysis with a multivariable Cox regression model. Finally, two independent SNPs, inositol polyphosphate-5-phosphatase D (INPP5D) rs13385922 C>T and exosome component 3 (EXOSC3) rs3208406 A>G, remained significantly associated withNSCLC OS with a combined hazards ratio (HR) of 1.14 (95% confidence interval = 1.06-1.23, P = 2.41×10-4) and 1.20 (95% confidence interval = 1.14-1.28, P = 3.41×10-9), respectively. Furthermore, NSCLC patients with the combination of unfavorable genotypes for these two SNPs were associated with a poor OS (Ptrend = 0.0002) and disease-specific survival (DSS, Ptrend < 0.0001) in the PLCO dataset. Expression quantitative trait loci (eQTL) analysis suggested that the INPP5D rs6782875 T allele was significantly correlated with elevated INPP5D mRNA expression levels in normal lung tissues and whole blood samples, while the EXOSC3 rs3208406 G allele was significantly correlated with increased EXOSC3 mRNA expression levels in normal lung tissues. Our data indicated that genetic variants in these immunity B cell-related genes may predict NSCLC survival possibly by influencing the gene expression.
1 Introduction
Lung cancer is the leading cause of cancer-related deaths in the world. In 2023, there were nearly 238,340 new cases diagnosed with and 127,070 deaths from lung cancer in the United States (1). In 2022 the National Cancer Center of China reported that lung cancer was both the most common cancer and the leading cause of cancer deaths in China (2). Therefore, lung cancer remains a substantial economic burden for both patients and healthcare systems globally (3). Based on its histological types, lung cancer can be divided into small cell lung cancer and non-small cell lung cancer (NSCLC), with the latter accounting for approximately 85% of all lung cancer cases (4). In recent years, despite remarkable advancements in earlier detection and therapeutic strategies, such as targeted molecular therapy and immunotherapy, the 5-year survival rate of advanced lung cancer remains low at only about 21% (5). However, individual lung cancer patients may respond dramatically differently to the same treatment and present different survival rates, and genetic variation may be involved in cancer progression (6). As a result, identifying genetic variation such as single-nucleotide polymorphisms (SNPs) in key genes and pathways may provide some new insights into the strategy of treating lung cancer.
The tumor microenvironment (TME) is a complex ecosystem where cancer cells are surrounded by diverse immune cells, inflammatory cells, tumor-associated fibroblasts, and altered extracellular matrix (7). Accumulating evidence suggests that TME has an important role in tumor initiation, progression, and metastasis (8, 9). As the second in the number of adaptive immune cells in TME, B cells localize to tumor-associated tertiary lymphoid structures and then interact with peripheral T cells and antigen-presenting cells to play a critical role in both pro-tumorigenic and anti-tumorigenic immunity (10). B cells not only promote tumor growth by secreting suppressive cytokines like IL-10, promoting immune tolerance by PD-L1+ B cells and producing proinflammatory cytokines such as IL-1β, but also inhibit tumor growth by secreting tumor-specific antibodies, serving as antigen-presenting cells themselves, and directly killing tumor cells (10–12). B cells were reported to be the second most common immune cell type with elevated expression levels in NSCLC tissues (13). A single-cell RNA-seq analysis indicated that plasma-like B cells inhibited tumor cell growth in the early stage and promoted cell growth in the advanced NSCLC (14). Moreover, the high percentage of naive‐like B cells in tumor tissues of NSCLC patients was associated with a better prognosis (14, 15). However, the potential role played by genetic variants in immunity B cell-related genes in NSCLC progression has not been reported.
Genome-wide association studies (GWASs) have been used to dissect the genotype-phenotype associations, providing new insights into the understanding of associations between genetic variants and certain diseases (16). For example, Chen et al. reported that genetic variants in peroxisome-related genes predicted NSCLC survival by influencing gene regulation (17). Another study indicated that two genetic variants in the immune-activation pathway genes affected the prognosis of NSCLC patients by regulating corresponding gene expression (18). In the present study, we have hypothesized that genetic variants in immunity B cell-related genes are associated with NSCLC survival. To test this hypothesis, we performed a two-stage analysis, using available GWAS data to evaluate associations between genetic variants in immunity B cell-related genes and survival of NSCLC patients.
2 Materials and methods
2.1 Study populations
In the two-stage analysis, we first used the GWAS dataset of lung cancer patients of European ancestry from the Prostate, Lung, Colorectal, and Ovarian cancer screening trial (PLCO) Cancer Screening Trial as the discovery. The PLCO enrolled approximately 155,000 participants aged 55-74 from 10 competitively selected screening centers across the United States between 1993 and 2001. Among all the participants, we extracted 1185 NSCLC patients (487 women and 698 men) with detailed personal information such as age, sex, smoking status, treatment history, and follow-up time for further survival analysis. Genotyping data were extracted from whole blood DNA samples genotyped using Illumina HumanHap240Sv1.0 and HumanHap550v3.0 platforms (dbGaP accession numbers: phs000093.v2.P2 and phs000336.v1.p1) (19, 20). The PLCO trial was approved by the National Cancer Institute and the institutional review boards of each involved center.
Then, we used another genotyping dataset from the Harvard University Lung Cancer Susceptibility (HLCS) study to validate the findings of the PLCO dataset. HLCS study included 984 histologically confirmed Caucasian NSCLC patients from the Massachusetts General Hospital (MGH) (21). The genomic blood DNA samples were used for genotyping by the Illumina Humanhap610-Quad array, and the genotyping data were subsequently imputed with the software MaCH based on the 1000 Genomes Project.
The use of the PLCO trial and HLCS study for experimentation was approved by the Internal Review Board of Duke University School of Medicine (Project #Pro00054575) and the dbGaP database (Project #6404). The characteristics of the two datasets are shown in Supplementary Table S1.
2.2 Gene selection and SNP imputation
We searched for the immunity B cell-related genes by using the keywords “B cell” and “immunity” from the Molecular Signature Database (http://www.gsea-msigdb.org/gsea/msigdb/human/search.jsp) (22). After excluding duplicated genes and genes on the X chromosome, 220 remaining genes were identified as candidate genes for further analyses (Supplementary Table S2). SNPs with ±2kb flanking regions of 220 immunity B cell-related genes were extracted from the PLCO trial and conducted using the Minimac4 based on the European data in the 1000 Genomes Project (phase 3). For the control quality, all the SNPs were extracted according to the following criteria: an imputation info score ≥ 0.3 (Supplementary Figure S1), a minor allele frequency (MAF) ≥ 5%, an individual call rate ≥ 95%, and the Hardy-Weinberg equilibrium P-value (HWE) ≥ 1 × 10−5. Finally, a total of 10,776 SNPs (1,196 genotyped and 9,580 imputed) were obtained from the PLCO dataset for further analysis.
2.3 Statistical methods
In the PLCO dataset, to estimate the associations between 10,776 candidate SNPs and NSCLC survival in an additive genetic model, we first performed single-locus Cox proportional hazards regression analysis using the R package GenABEL package (23). The Cox regression analysis was performed with adjustment for clinical variables (including age, sex, smoking status, histologic subtype, tumor stage, chemotherapy, radiotherapy, and surgery) and the top four of the 10 principal components (PCs) in the discovery dataset (Supplementary Table S3). To filter out potential false-positive results, we employed a multiple testing correction by Bayesian false discovery probability (BFDP) with the threshold of 0.8 as recommended (24). We used a prior probability of 0.10 to detect an upper boundary hazards ratio (HR) of 3.0 for an association with variant genotypes or minor alleles of the SNPs with P < 0.05.
The identified SNPs from the PLCO GWAS dataset were validated using the GWAS dataset of the HLCS study in a multivariable Cox regression model. To combine the results of the two GWAS datasets, the inverse variance weighted meta-analysis was performed, using Cochran’s Q-test and the heterogeneity statistic (I2) to assess inter-study and determine the appropriate model. If no heterogeneity (Q-test P > 0.10 and I2 < 50%), the meta-analysis was performed with a fixed-effects model, otherwise with the random-effects model.
Linkage disequilibrium (LD) analysis was performed with Haploview 4.1. Two online bioinformatics tools, RegulomeDB (http://www.regulomedb.org/) and HaploReg v4.2 (https://pubs.broadinstitute.org/mammals/haploreg/haploreg.php), were used to predict the potentially functional SNPs (25, 26). Subsequently, to identify the associations between independent SNPs and NSCLC survival, we employed the multivariable stepwise Cox regression model with adjustment for demographic and clinical variables, the top four PCs, and 54 previously published SNPs from the same PLCO GWAS dataset. We also generated the Manhattan plots with Haploview4.1 and regional association plots with Locus Zoom (http://http://locuszoom.sph.umich.edu) to visualize the selected SNPs (27).
Subsequently, we employed the combined unfavorable genotypes to evaluate the cumulative effects of the two identified SNPs and the Kaplan-Meier (KM) survival curves to assess the survival probability. We also performed stratified analysis and evaluated inter-study heterogeneity to assess the associations between subgroups and survival as well as the combined effect of unfavorable genotypes that might be influenced by clinical characteristics. To evaluate predictive accuracy of the clinical models with the addition of the genetic variables, we constructed time-dependent area under the curve (AUC) and the receiver operating characteristic (ROC) curves using R (version 3.6.3) package “time ROC” and “survival” (28).
To explore the genotype-phenotype correlation between two identified SNPs and mRNA expression levels of their corresponding genes, we performed expression quantitative trait loci (eQTL) analyses with a linear regression model using data from two sources: lymphoblastoid cell lines in 373 European descendants from the 1,000 Genomes Project (Phase 3) and the genotype-tissue expression (GTEx) project (including 515 normal lung tissues and 670 whole blood samples) (29). To compare the mRNA expression among tumor tissue of lung adenocarcinoma (LUAD), lung squamous cell carcinoma (LUSC) and adjacent normal tissue with paired and unpaired t-tests, we downloaded the raw expression data from the Cancer Genome Atlas (TCGA) database and performed the analyses on the online data platform UALCAN (https://ualcan.path.uab.edu/) (30), respectively. Finally, we assessed the correlations between the mRNA expression levels of two genes and NSCLC survival probability using the online survival analysis database Kaplan-Meier (http://kmplot.com/analysis/) (31). Unless specified otherwise, all statistical analyses were conducted with the SAS software 9.4 (SAS Institute, Cary, NC, USA).
3 Results
3.1 Associations between SNPs in the immunity B cell-related genes and the survival of NSCLC
The final analysis in the present study included 1185 NSCLC patients from the PLCO trial and 984 NSCLC patients from the HLCS study, and their clinical features are described in Supplementary Table S1. The study flow chart is depicted in Figure 1. After multiple testing corrections by BFDP (≤ 0.80), 369 SNPs out of the 10,776 SNPs in the immunity B cell-related genes were found to be statistically significantly associated with NSCLC overall survival (OS) (P ≤ 0.05); then, these SNPs were further validated in the HLCS dataset. As a result, 18 SNPs in three genes (i.e., inositol polyphosphate-5-phosphatase D, INPP5D; complement factor I, CFI; and exosome component 3, EXOSC3) remained significant. While EXOSC3 has only one SNP (i.e., rs3208406 in EXOSC3), further LD analyses of the remaining 17 SNPs with Haploview 4.1 software identified two SNPs (one in each of INPP5D and CFI) as the tagger SNPs (Supplementary Figure S2). The results of subsequent online functional prediction for these three SNPs are listed in Supplementary Table S4. EXOSC3 rs3208406 A>G and CFI rs6836770 G>A may have an effect on enhancer histone marks, and the allele change in these three SNPs may alter protein motifs.
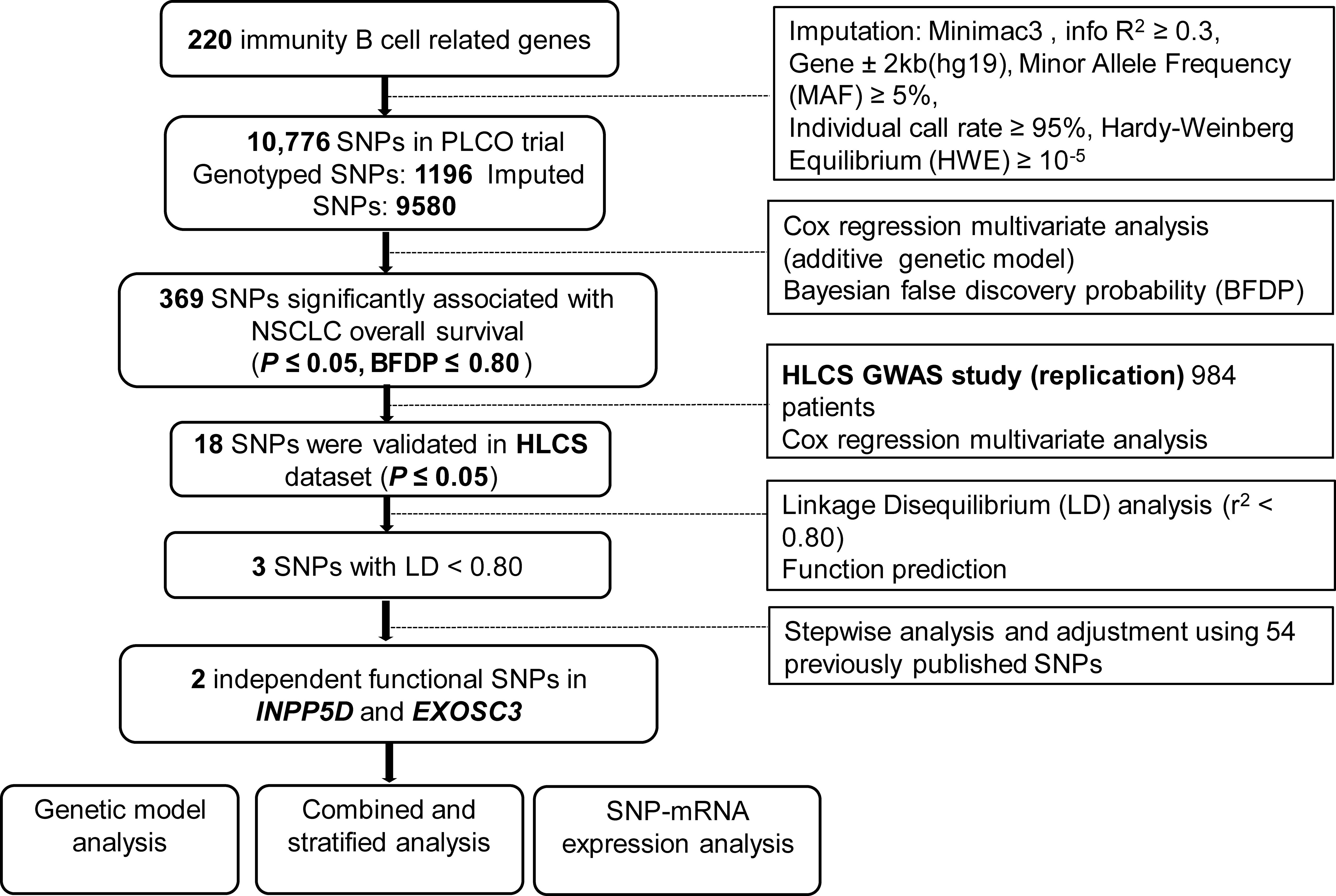
Figure 1 The flowchart of the present study. SNP, single-nucleotide polymorphism; PLCO, Prostate, Lung, Colorectal and Ovarian cancer screening trial; NSCLC, non-small cell lung cancer; HLCS, Harvard lung cancer susceptibility study; GWAS, Genome-Wide Association Study.
3.2 Identification the effect of independent SNPs on NSCLC OS in the PLCO trial
Because the HLCS replication dataset did not have the same genotyping data as the PLCO did, to identify the effect of independent SNPs on NSCLC OS, we first used the PLCO genotyping dataset to perform the stepwise multivariable Cox regression analysis. Next, we put the remaining significant SNPs into a post-stepwise multivariable model including 54 previously reported SNPs in the PLCO GWAS dataset. Finally, two SNPs (INPP5D rs13385922 C>T and EXOSC3 rs3208406 A>G) remained significantly associated with NSCLC OS (P = 0.003 and 0.002, respectively) (Table 1). Moreover, the meta-analysis of the PLCO trial and HLCS study revealed that there was no interstudy heterogeneity across these two datasets, and the combined results are listed in Table 2. We also depicted Manhattan plots (Supplementary Figure S3) and regional association plots (Supplementary Figure S4) for these two significant SNPs.
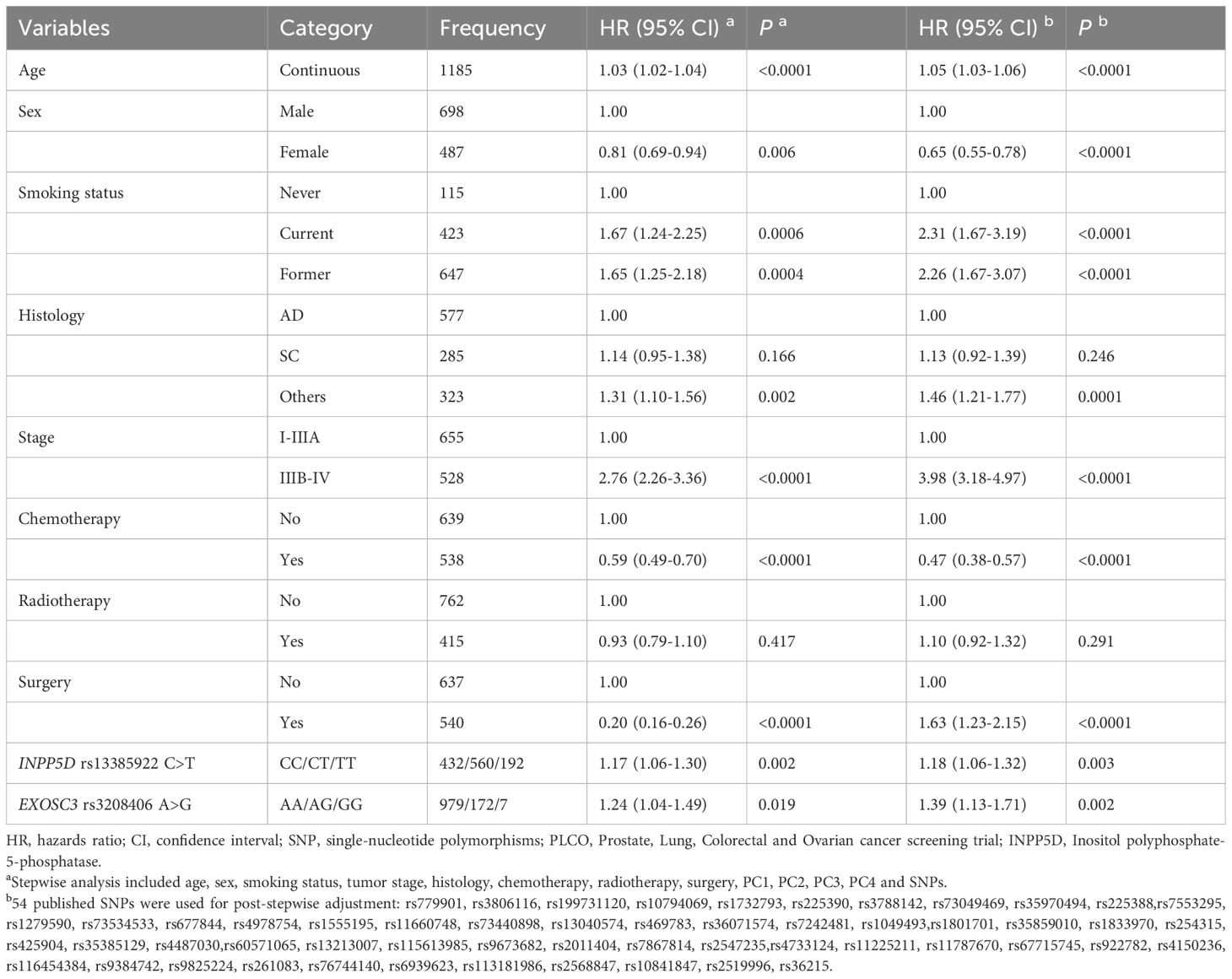
Table 1 Two independent SNPs in multivariable Cox proportional hazards regression analysis with adjustment for other covariates and 54 previously published SNPs in the PLCO dataset.
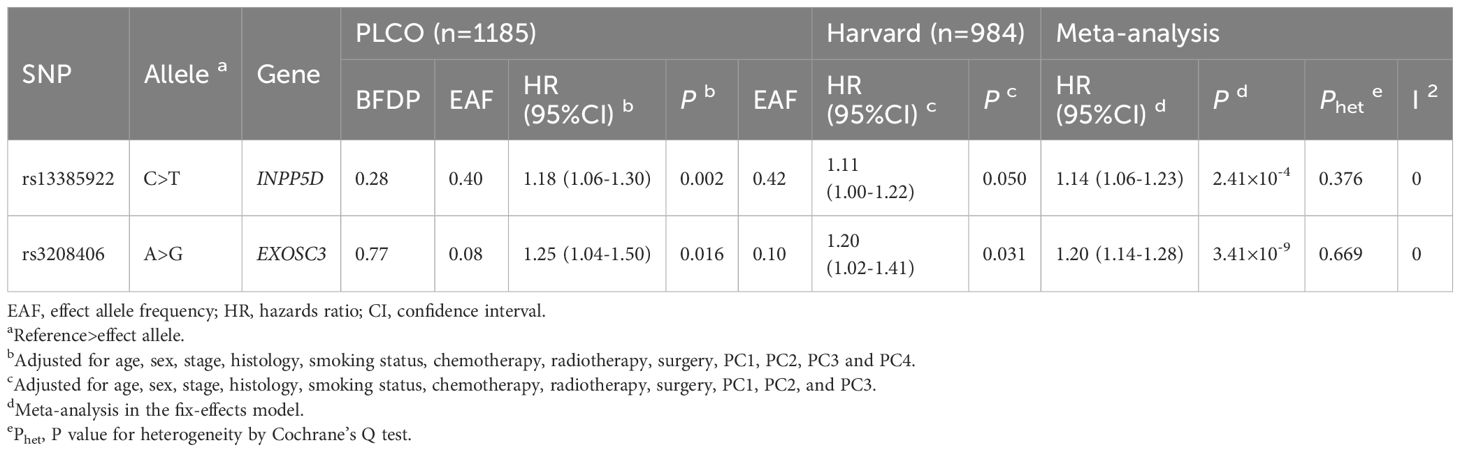
Table 2 Associations of two independent SNPs with overall survival in both discovery and validation datasets from two previously published NSCLC GWASs.
As shown in Table 3, both the INPP5D rs13385922 T allele and EXOSC3 rs3208406 G allele were significantly associated with OS (Ptrend = 0.003 and 0.023, respectively) and disease-specific survival (DSS) (Ptrend = 0.0005 and 0.003, respectively). Compared with those having the reference genotype in a dominant genetic model, NSCLC patients had a significantly poor survival associated with INPP5D rs13385922 CT+TT (OS: HR = 1.22, 95% CI = 1.06-1.42, and P = 0.008; DSS: HR = 1.29, 95% CI = 1.10-1.51, and P = 0.002), and with EXOSC3 rs3208406 AG+GG (OS: HR = 1.27, 95% CI = 1.05-1.55, and P = 0.015; DSS: HR = 1.37, 95% CI = 1.12-1.67, and P = 0.002). Additionally, we also depicted Kaplan-Meier survival curves for these results (Supplementary Figure S5).
3.3 Combined effect of two independent SNPs on NSCLC survival in the PLCO dataset
To assess the combined effect of these two independent SNPs on NSCLC survival, we combined unfavorable genotypes (INPP5D rs13385922 CT+TT and EXOSC3 rs3208406 AG+GG) into a genetic score and divided all NSCLC patients into three groups (i.e., 0, 1, and 2) according to the number of unfavorable genotypes (NUGs). As shown in Table 3, an elevated NUG score was associated with a poor survival for both OS (Ptrend < 0.0002) and DSS (Ptrend < 0.0001). Furthermore, we dichotomized the NUGs and divided all NSCLC patients into low-unfavorable-genotypes group (0 NUGs) and high-unfavorable-genotypes group (1-2 NUGs). Compared with the 0 NUGs group, the 1-2 NUGs group had a significantly poorer survival for OS (HR = 1.34, 95% CI = 1.14-1.58, and P = 0.0003) and DSS (HR = 1.46, 95% CI = 1.23-1.73, and Ptrend < 0.0001). We also depicted these results with Kaplan-Meier survival curves from a log-rank perspective (Figures 2A–D).
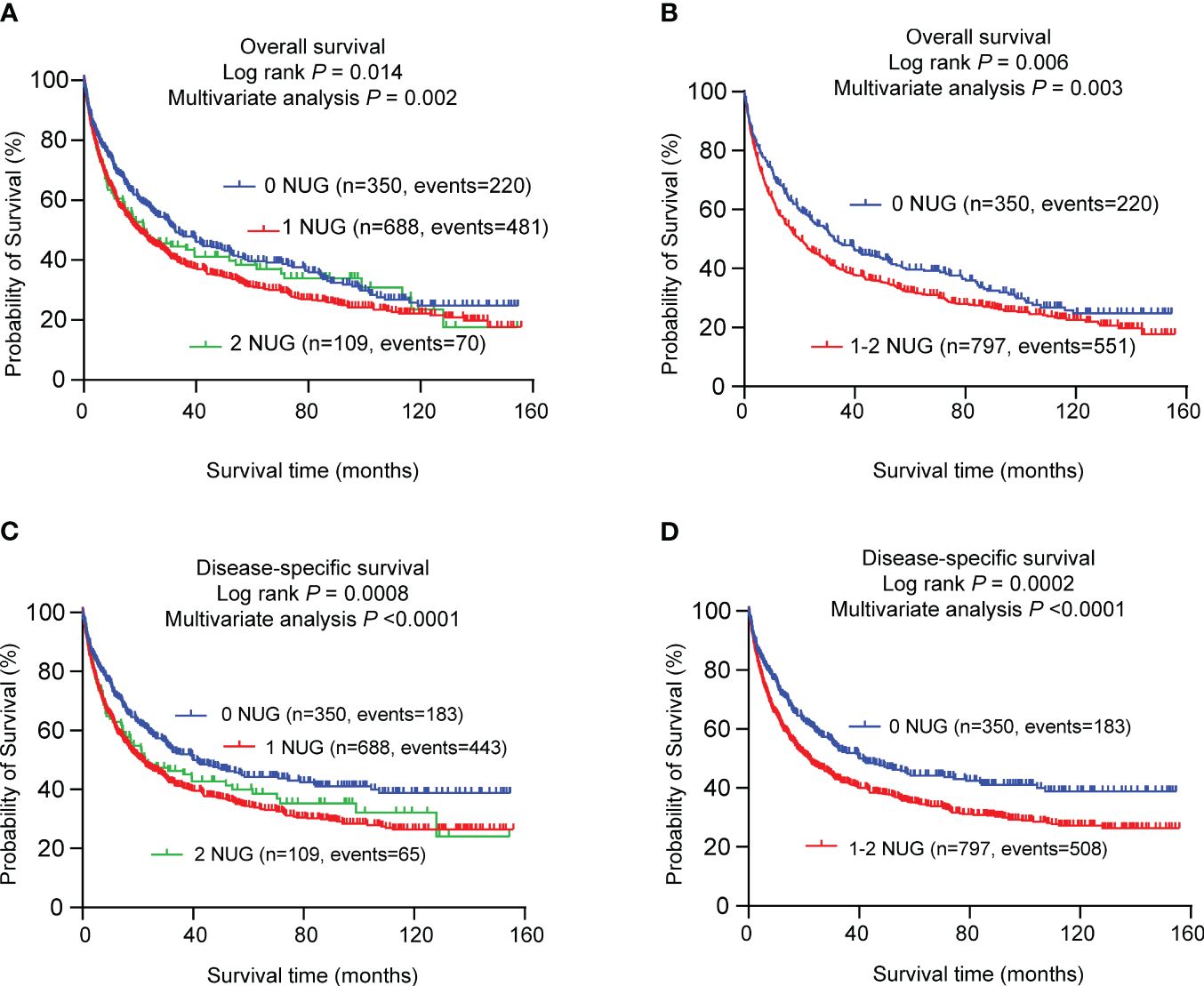
Figure 2 Prediction of survival with the combined unfavorable genotypes. Kaplan–Meier survival curves in the PLCO dataset for (A) OS with the combined unfavorable genotypes; (B) OS with the dichotomized groups of the NUGs; (C) DSS with the combined unfavorable genotypes; (D) DSS with dichotomized groups of the NUGs. #Unfavorable genotypes were INPP5D rs13385922 CT+TT and EXOSC3 rs3208406 AG+GG. SNPs, single nucleotide polymorphism; NUG, number of unfavorable genotypes; PLCO, The Prostate, Lung, Colorectal and Ovarian Cancer Screening Trial.
3.4 Stratified analysis for associations between NUGs and NSCLC survival
To assess the possible modification effect of NUGs on NSCLC survival by age, sex, smoking status, histology, tumor stage, chemotherapy, radiotherapy, and surgery, we further conducted stratified analysis in the PLCO trial. As shown in Supplementary Table S5, For the effects of both 0 and 1-2 NUG groups on NSCLC OS and DSS, no significant interactions were found between NUGs and age, sex, smoking status, histology, tumor stage, chemotherapy, radiotherapy, and surgery (all Pinter > 0.05).
3.5 Time-dependent AUC and ROC curves to predict NSCLC survival
To further evaluate the predictive role in survival of the two SNPs for OS and DSS, we performed the time-dependent AUC and ROC curves at the 12th,36th, and 60th month in the PLCO trial. Compared with the predictive model for clinical covariates including age, sex, smoking status, histology, tumor stage, chemotherapy, radiotherapy, surgery, and the four PCs, the predictive values before and after adding the two independent SNPs to the model were different. Time-dependent AUC for OS and DSS were shown in Supplementary Figures S6A, B. With the addition of the two SNPs, the AUC increased from 87.38% to 88.08% for OS (P = 0.020) and from 87.48% to 88.20% for DSS (P = 0.039) at 12th month (Supplementary Figures S6C, D). However, the predictive performance of AUC curves at the 36th and 60th month for both OS and DSS was not significantly improved (all P > 0.05, Supplementary Figures S6E–H).
3.6 The result of eQTL analysis
To explore the genotype-phenotype correlation, we first performed the eQTL analysis using genomic data of lymphoblastoid cell lines from the 373 European descendants in the 1000 Genomes Project. The results suggested that the INPP5D rs13385922 T allele was not associated with expression levels of INPP5D mRNA in the additive (P = 0.405, Supplementary Figure S7A), dominant model (P = 0.430, Supplementary Figure S7B), and recessive model (P = 0.586, Supplementary Figure S7C). We also employed an eQTL analysis using GTEx project data. The results suggested that the INPP5D rs13385922 T allele was significantly associated with high mRNA expression levels of INPP5D in both normal lung tissues (P = 0.001, Figure 3A) and whole blood samples (P = 1.67e-7, Figure 3B). Moreover, the EXOSC3 rs3208406 G allele was associated with high mRNA expression levels of EXOSC3 in the recessive model (P = 2e-05, Figure 3C), but not in the additive (P = 0.117, Supplementary Figure S7D) and dominant model (P = 0.557, Supplementary Figure S7E). In the GTEx project, the EXOSC3 rs3208406 G allele was associated with mRNA expression levels of EXOSC3 whole blood samples (P = 0.018, Figure 3D), but not in normal lung tissues (P = 0.105, Supplementary Figure S7F).
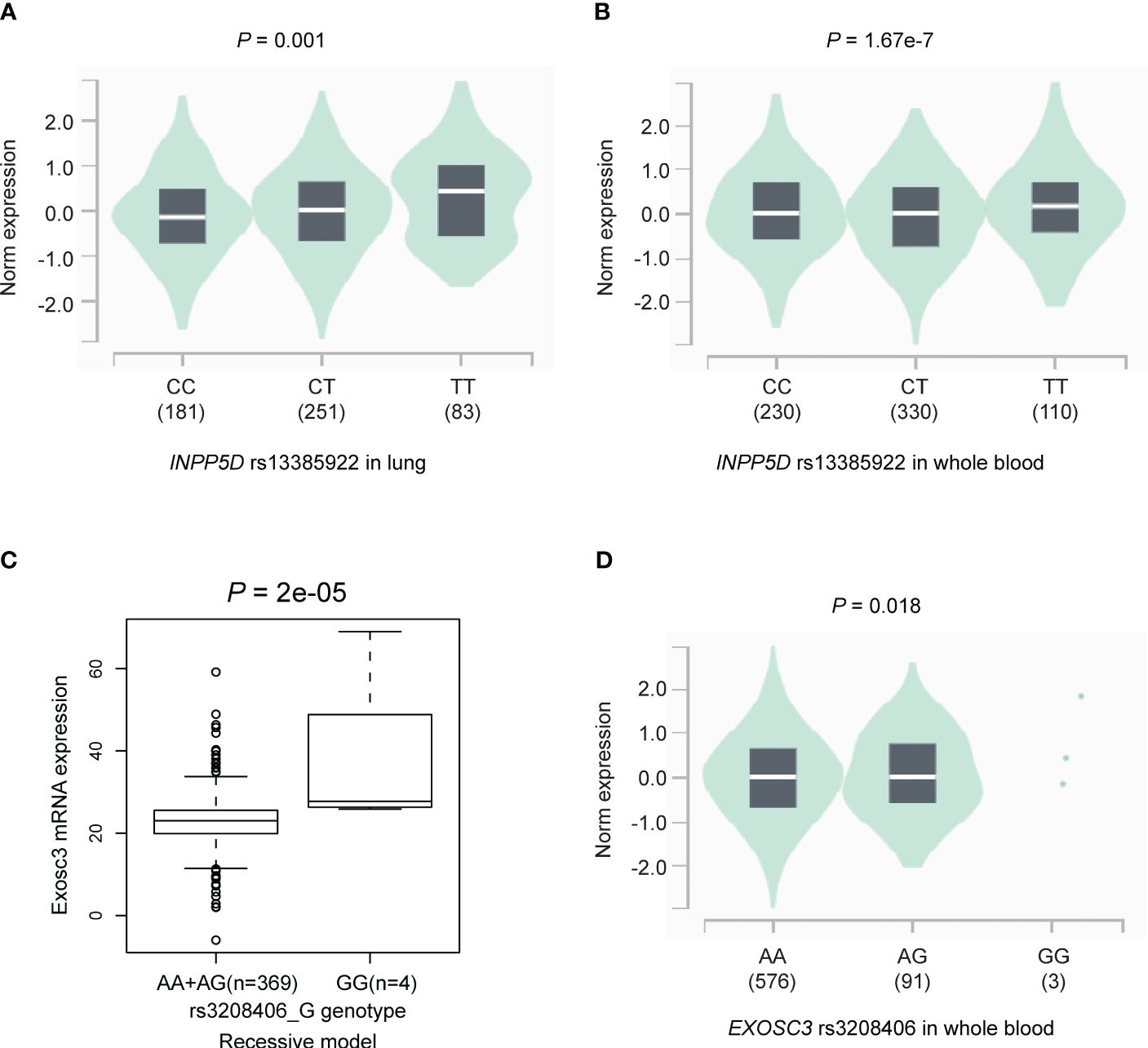
Figure 3 The results of the eQTL analysis. The INPP5D rs13385922 T allele was significantly associated with high mRNA expression levels of INPP5D in (A) normal lung tissues and (B) whole blood samples in the GTEx project; the EXOSC3 rs3208406 G allele was associated with high mRNA expression levels of EXOSC3 in (C) recessive model in the lymphoblastoid cell lines from the 1000 Genomes Project and (D) whole blood samples in the GTEx project. eQTL, expression quantitative trait loci.
3.7 The analysis of mRNA expression and survival in NSCLC
To explore potential mechanisms of INPP5D and EXOSC3 on NSCLC survival, we first evaluated the mRNA expression levels of these two genes using paired t-tests with data from the TCGA database and unpaired tests with the online UALCAN portal. Then, we used Kaplan-Meier Plotter web tool to estimate the associations between their mRNA expression levels and NSCLC survival. Compared with adjacent paired normal tissues, INPP5D mRNA expression was significantly down-regulated in tissues from the combined LUSC and LUAD (P = 0.0007) (Figure 4A), and LUSC (P < 0.0001), but not from LUAD (P = 0.565) (Supplementary Figures S8A, B). Similar results were also observed for LUSC (P < 0.0001) and LUAD (P = 0.888) in the UALCAN database using unpaired tests (Supplementary Figures S8C, D). Furthermore, mRNA expression levels of INPP5D were not associated with OS of NSCLC (HR = 0.93, 95% CI: 0.83-1.05, log-rank P = 0.23) (Figure 4B). EXOSC3 mRNA expression levels were significantly up-regulated in tissues from the combined LUSC + LUAD (Figure 4C), LUSC, and LUAD (Supplementary Figures S8E, F) (all P < 0.0001) than those in adjacent normal tissues using paired t-test. Similar results were also observed for LUSC and LUAD (all P < 0.0001) in the UALCAN database using unpaired tests (Supplementary Figures S8G, H). Moreover, high mRNA expression levels of EXOSC3 were associated with a poor NSCLC OS (HR = 1.69, 95% CI: 1.45-1.96, log-rank P = 4e-12) (Figure 4D).
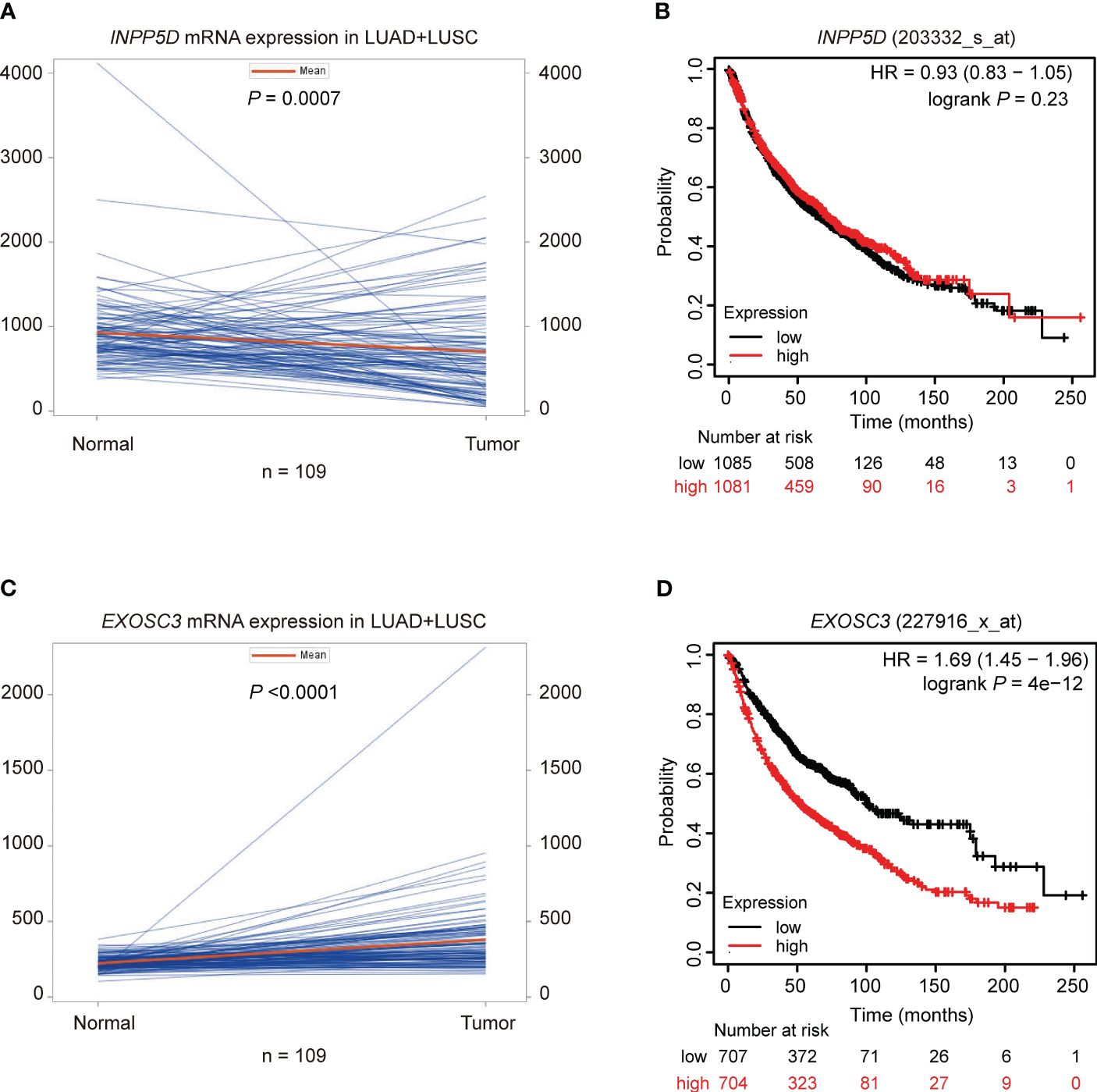
Figure 4 Paired mRNA expression and survival analyses in NSCLC. Paired t-test suggested that the mRNA expression of (A) INPP5D was down-regulated and (C) EXOSC3 was up-regulated in NSCLC; survival analysis suggested that (B) mRNA expression levels of INPP5D were not associated with NSCLC survival; (D) high mRNA expression levels of EXOSC3 were associated with a poor NSCLC survival.
4 Discussion
In the present study, we evaluated the associations between 10,776 genetic variants in the 220 immunity B cell-related genes and NSCLC survival using available GWAS genotyping data from both PLCO trial and HLCS study. Our results indicated that the INPP5D rs13385922 C>T and EXOSC3 rs3208406 A>G were significantly associated with a poor survival in the United States Caucasian populations. Our findings also suggested that the INPP5D rs13385922 variant T allele and EXOSC3 rs3208406 variant G allele were associated with up-regulated mRNA expression levels of INPP5D and EXOSC3 in the lymphoblastoid cell lines from the 1000 genomes project and the GTEx project, implying that these alleles may modulate mRNA expression and influence NSCLC survival. Our findings provided additional support for associations between genetic variants in the immunity B cell-related genes and survival of NSCLC patients.
B cells are the second most numerous adaptive immune cells in TME and may mediate both pro- and antitumorigenic effects in tumor development (32, 33). Within the tertiary lymphoid structures, B cells perform the function of antigen presentation and antibody production to focus immune responses, and the expression of B cells is associated with clinical outcomes in multiple cancer types (34, 35). However, up to now no studies have reported the association between functional genetic variants of immunity B cell-related genes and NSCLC survival. To the best of our knowledge, this is the first study to explore the role of genetic variants of immunity B cell-related genes in predicting NSCLC survival. As a result, we identified two SNPs (i.e., INPP5D rs13385922 C>T and EXOSC3 rs3208406 A>G) from immunity B cell-related genes that predicted the prognosis of NSCLC patients.
INPP5D, inositol polyphosphate-5-phosphatase D (all known as SHIP1), is located on chromosome 2 and composed of 1189 amino acids. INPP5D is a member of the inositol polyphosphate-5-phosphatase (INPP5) family and plays an important role in the immune system (36). A previous study reported that INPP5D can skew macrophage progenitors toward M1 macrophages and naive T cells to T helper 1 and T helper 17 cells; as a result, INPP5D is intricately linked to the activation of the immune system and plays a key role in the solid tumor eradication (37). Pulsatile INPP5D inhibition contributed to the enhancement of T and NK cell function and improved antitumor immunity and survival in mouse models of lymphoma and colon cancer (38). INPP5D has been shown to suppress the activity of PI3K/AKT/mTOR signaling pathway via reducing PI(3,4,5)P3 levels at the plasma membrane and promote cancer cell survival (39). In NSCLC, the expression of INPP5D was down-regulated in both tumor tissues and cell lines, and the overexpression of INPP5D suppressed cell growth, migration, and invasion by inactivating PI3K/AKT pathway (40). However, no report has investigated the role of the genetic variants of INPP5D in NSCLC survival. In the present study, for the first time, we found that the genetic variants of INPP5D were significantly associated with OS and DSS in NSCLC patients. The INPP5D rs13385922 T allele showed a significant unfavorable effect on NSCLC survival and an association with increased mRNA expression levels of INPP5D. However, the mRNA expression of INPP5D was not associated with NSCLC survival. We also found that the mRNA expression levels of INPP5D were down-regulated in LUSC but not in LUAD, suggesting tumor specificity between LUSC and LUAD. Moreover, the mRNA levels of INPP5D may also be possibly modulated by other factors, such as the regulation of RNA transcription and degradation. Nevertheless, the down-regulation of the INPP5D mRNA expression in NSCLC identified in the present study is in line with a previous study (40). Taken together, we concluded that INPP5D might be a potential tumor suppressor gene in NSCLC.
EXOSC3 (also known as exosome component 3) is located on chromosome 9 and composed of 275 amino acids. EXOSC3 is one of the constituent elements of RNA exosomes, and mutations in EXOSC3 have been linked to pontocerebellar hypoplasia and spinal motor neuron degeneration (41). One study showed that exosc3-deficient B cells were impaired in the ability to undergo normal levels of somatic hypermutation and class switch recombination (42). Few studies have investigated the role of EXOSC3 in cancer. A previous study showed that the protein expression of EXOSC3 was significantly up-regulated in pancreatic cancer tissue using protein-deep sequencing (43), but there were no published studies that explored the associations between genetic variants of EXOSC3 and NSCLC survival. In the present study, our results suggest that the EXOSC3 rs3208406 G allele may predict a reduced risk of survival for NSCLC patients and up-regulated the mRNA expression levels of EXOSC3 in the 1000 Genomes Project and whole blood samples. Our findings on this SNP-mRNA correlation suggested that EXOSC3 rs3208406 G may influence the prognosis of NSLCLC via modulating the mRNA expression of EXOSC3. However, functional experiments should be designed to explore the potential molecular mechanisms underlying the observed SNP-mRNA associations.
The observed SNP-survival associations in the present study suggested that genetic variants in the immunity B cell-related genes might be potential therapy targets for NSCLC. However, several methodological weaknesses in this study should be acknowledged. First, due to NSCLC patients in the two GWAS datasets were only limited to Caucasian descents, our findings may not be generalizable to other populations with different ethnicities. To address this gap, we will design replication study with other larger and independent populations from different races or geographic regions. Second, because the lack of detailed genotype data and clinical outcomes information in the HLCS study, we conducted the combined and stratified analyses with data only from the PLCO trial, and the results should be interpreted cautiously. Third, no further clinical information on nutrition status and details of treatment were available for further analysis. Moreover, although the two identified SNPs were identified to be associated with NSCLC survival, the potential mechanisms are not clear. Further experiments should be undertaken both in vivo and in vitro to better understand the mechanisms underlying the observed associations between two identified SNPs and NSCLC survival.
Data availability statement
Publicly available datasets were analyzed in this study. This data can be found here: dbGaP accession numbers: phs000093.v2.P2 and phs000336.v1.p1.
Ethics statement
The studies involving humans were approved by The PLCO trial was approved by the National Cancer Institute and the institutional review boards of each involved center. The use of the PLCO trial and HLCS study for experimentation was approved by the Internal Review Board of Duke University School of Medicine (Project #Pro00054575) and the dbGaP database (Project #6404). The studies were conducted in accordance with the local legislation and institutional requirements. Written informed consent for participation was not required from the participants or the participants’ legal guardians/next of kin in accordance with the national legislation and institutional requirements.
Author contributions
GL: Conceptualization, Formal analysis, Funding acquisition, Methodology, Software, Writing – original draft. HL: Conceptualization, Data curation, Validation, Writing – original draft. HW: Formal analysis, Writing – original draft. XT: Formal analysis, Writing – original draft. SL: Methodology, Writing – review & editing. MD: Data curation, Formal analysis, Validation, Writing – original draft. DC: Funding acquisition, Methodology, Writing – review & editing. QW: Conceptualization, Funding acquisition, Methodology, Writing – review & editing.
Funding
The author(s) declare financial support was received for the research, authorship, and/or publication of this article. QW was supported by NIH grants 2R01 ES011740 and 1R01CA 131274 and also partly supported by the Duke Cancer Institute as part of the P30 Cancer Center Support Grant (Grant ID: NIH/NCI CA014236). The Harvard Lung Cancer Susceptibility Study was supported by NIH grants U01CA209414, CA092824, CA074386 and CA090578 to DC. GL was supported by Jiangsu Health International Exchange Program.
Acknowledgments
The authors thank all the participants of the PLCO Cancer Screening Trial. The authors also thank the National Cancer Institute for granting access to the data collected during the PLCO trial. It is important to note that the statements provided in this document solely reflect the perspectives of the authors and do not imply concurrence or endorsement by the National Cancer Institute. The authors would also like to acknowledge the dbGaP repository for providing valuable cancer genotyping datasets. The accession numbers for the lung cancer datasets are phs000336.v1.p1 and phs000093.v2.p2. A list of contributing investigators and funding agencies for those studies can be found in the Supplementary Data.
Conflict of interest
The authors declare that the research was conducted in the absence of any commercial or financial relationships that could be construed as a potential conflict of interest.
Publisher’s note
All claims expressed in this article are solely those of the authors and do not necessarily represent those of their affiliated organizations, or those of the publisher, the editors and the reviewers. Any product that may be evaluated in this article, or claim that may be made by its manufacturer, is not guaranteed or endorsed by the publisher.
Supplementary material
The Supplementary Material for this article can be found online at: https://www.frontiersin.org/articles/10.3389/fimmu.2024.1440454/full#supplementary-material
Abbreviations
AUC, receiver operating characteristic curve; BFDP, Bayesian false discovery probability; CI, confidence interval; DSS, disease special survival; eQTL, expression quantitative trait loci; EXOSC3, exosome component 3; GWAS, Genome-Wide Association Study; HWE, Hardy-Weinberg equilibrium; HLCS, Harvard Lung Cancer Susceptibility; HR, hazards ratio; INPP5D, inositol polyphosphate-5-phosphatase D; LD, linkage disequilibrium; LUAD, lung adenocarcinoma; LUSC, lung squamous cell carcinoma; MAF, minor allelic frequency; NSCLC, non-small cell lung cancer; PLCO, the Prostate, Lung, Colorectal and Ovarian Cancer Screening Trial; SNPs, single nucleotide polymorphisms; OS, overall survival; ROC, receiver operating characteristic; TCGA, the Cancer Genome Atlas.
References
1. Siegel RL, Miller KD, Wagle NS, Jemal A. Cancer statistics, 2023. CA Cancer J Clin. (2023) 73:17–48. doi: 10.3322/caac.21763
2. Han B, Zheng R, Zeng H, Wang S, Sun K, Chen R, et al. Cancer incidence and mortality in China, 2022. Zhonghua Zhong Liu Za Zhi. (2024) 46:221–31. doi: 10.3760/cma.j.cn112152-20240119-00035
3. Carbone DP, Gandara DR, Antonia SJ, Zielinski C, Paz-Ares L. Non-small-cell lung cancer: role of the immune system and potential for immunotherapy. J Thorac Oncol. (2015) 10:974–84. doi: 10.1097/JTO.0000000000000551
4. Liu X, Li R, Chen X, Yao J, Wang Q, Zhang J, et al. SYT7 is a key player in increasing exosome secretion and promoting angiogenesis in non-small-cell lung cancer. Cancer Lett. (2023) 577:216400. doi: 10.1016/j.canlet.2023.216400
5. Howlader N, Forjaz G, Mooradian MJ, Meza R, Kong CY, Cronin KA, et al. The effect of advances in lung-cancer treatment on population mortality. N Engl J Med. (2020) 383:640–9. doi: 10.1056/NEJMoa1916623
6. Wang S, Wang J, Bai Y, Wang Q, Liu L, Zhang K, et al. The genetic variations in DNA repair genes ERCC2 and XRCC1 were associated with the overall survival of advanced non-small-cell lung cancer patients. Cancer Med. (2016) 5:2332–42. doi: 10.1002/cam4.822
7. Yang E, Wang X, Gong Z, Yu M, Wu H, Zhang D. Exosome-mediated metabolic reprogramming: the emerging role in tumor microenvironment remodeling and its influence on cancer progression. Signal Transduct Target Ther. (2020) 5:242. doi: 10.1038/s41392-020-00359-5
8. de Visser KE, Joyce JA. The evolving tumor microenvironment: From cancer initiation to metastatic outgrowth. Cancer Cell. (2023) 41:374–403. doi: 10.1016/j.ccell.2023.02.016
9. Hinshaw DC, Shevde LA. The tumor microenvironment innately modulates cancer progression. Cancer Res. (2019) 79:4557–66. doi: 10.1158/0008-5472.CAN-18-3962
10. Downs-Canner SM, Meier J, Vincent BG, Serody JS. B cell function in the tumor microenvironment. Annu Rev Immunol. (2022) 40:169–93. doi: 10.1146/annurev-immunol-101220-015603
11. Anderson KG, Stromnes IM, Greenberg PD. Obstacles posed by the tumor microenvironment to T cell activity: A case for synergistic therapies. Cancer Cell. (2017) 31:311–25. doi: 10.1016/j.ccell.2017.02.008
12. Sarvaria A, Madrigal JA, Saudemont A. B cell regulation in cancer and anti-tumor immunity. Cell Mol Immunol. (2017) 14:662–74. doi: 10.1038/cmi.2017.35
13. Stankovic B, Bjørhovde HAK, Skarshaug R, Aamodt H, Frafjord A, Müller E, et al. Immune cell composition in human non-small cell lung cancer. Front Immunol. (2018) 9:3101. doi: 10.3389/fimmu.2018.03101
14. Chen J, Tan Y, Sun F, Hou L, Zhang C, Ge T, et al. Single-cell transcriptome and antigen-immunoglobin analysis reveals the diversity of B cells in non-small cell lung cancer. Genome Biol. (2020) 21:152. doi: 10.1186/s13059-020-02064-6
15. Diao XY, Wang F. Single-cell transcriptome analysis of tumor-infiltrating B cells reveals their clinical implications in non-small cell lung cancer. Thorac cancer. (2021) 12:5–7. doi: 10.1111/1759-7714.13719
16. Cusanovich DA, Caliskan M, Billstrand C, Michelini K, Chavarria C, De Leon S, et al. Integrated analyses of gene expression and genetic association studies in a founder population. Hum Mol Genet. (2016) 25:2104–12. doi: 10.1093/hmg/ddw061
17. Chen AS, Liu H, Wu Y, Luo S, Patz EF Jr, Glass C, et al. Genetic variants in DDO and PEX5L in peroxisome-related pathways predict non-small cell lung cancer survival. Mol Carcinog. (2022) 61:619–28. doi: 10.1002/mc.23400
18. Bai Y, Zheng J, Cheng L, Liu Q, Zhao G, Li J, et al. Potentially functional genetic variants of VAV2 and PSMA4 in the immune-activation pathway and non-small cell lung cancer survival. J Gene Med. (2022) 24:e3447. doi: 10.1002/jgm.3447
19. Tryka KA, Hao L, Sturcke A, Jin Y, Wang ZY, Ziyabari L, et al. NCBI's database of genotypes and phenotypes: dbGaP. Nucleic Acids Res. (2014) 42:D975–979. doi: 10.1093/nar/gkt1211
20. Wong KM, Langlais K, Tobias GS, Fletcher-Hoppe C, Krasnewich D, Leeds HS, et al. The dbGaP data browser: a new tool for browsing dbGaP controlled-access genomic data. Nucleic Acids Res. (2017) 45:D819–d826. doi: 10.1093/nar/gkw1139
21. Zhai R, Yu X, Wei Y, Su L, Christiani DC. Smoking and smoking cessation in relation to the development of co-existing non-small cell lung cancer with chronic obstructive pulmonary disease. Int J Cancer. (2014) 134:961–70. doi: 10.1002/ijc.28414
22. Liberzon A, Birger C, Thorvaldsdóttir H, Ghandi M, Mesirov JP, Tamayo P. The Molecular Signatures Database (MSigDB) hallmark gene set collection. Cell Syst. (2015) 1:417–25. doi: 10.1016/j.cels.2015.12.004
23. Karssen LC, van Duijn CM, Aulchenko YS. The GenABEL Project for statistical genomics. F1000Res. (2016) 2016:5:914. doi: 10.12688/f1000research.8733.1
24. Park JH, Geum DI, Eisenhut M, van der Vliet HJ, Shin JI. Beaysian statistical methods in genetic association studies: Empirical examination of statistically non-significant Genome Wide Association Study (GWAS) meta-analyses in cancers: A systematic review. Gene. (2019) 685:170–8. doi: 10.1016/j.gene.2018.10.057
25. Dong S, Zhao N, Spragins E, Kagda MS, Li M, Assis P, et al. Annotating and prioritizing human non-coding variants with RegulomeDB v.2. Nat Genet. (2023) 55:724–6. doi: 10.1038/s41588-023-01365-3
26. Ward LD, Kellis M. HaploReg v4: systematic mining of putative causal variants, cell types, regulators and target genes for human complex traits and disease. Nucleic Acids Res. (2016) 44:D877–881. doi: 10.1093/nar/gkv1340
27. Boughton AP, Welch RP, Flickinger M, VandeHaar P, Taliun D, Abecasis GR, et al. LocusZoom.js: interactive and embeddable visualization of genetic association study results. Bioinformatics. (2021) 37:3017–8. doi: 10.1093/bioinformatics/btab186
28. Chambless LE, Diao G. Estimation of time-dependent area under the ROC curve for long-term risk prediction. Stat Med. (2006) 25:3474–86. doi: 10.1002/sim.2299
29. Human genomics. The Genotype-Tissue Expression (GTEx) pilot analysis: multitissue gene regulation in humans. Science. (2015) 348:648–60. doi: 10.1126/science.1262110
30. Chandrashekar DS, Karthikeyan SK, Korla PK, Patel H, Shovon AR, Athar M, et al. UALCAN: An update to the integrated cancer data analysis platform. Neoplasia. (2022) 25:18–27. doi: 10.1016/j.neo.2022.01.001
31. Győrffy B. Transcriptome-level discovery of survival-associated biomarkers and therapy targets in non-small-cell lung cancer. Br J Pharmacol. (2024) 181:362–74. doi: 10.1111/bph.16257
32. Wang SS, Liu W, Ly D, Xu H, Qu L, Zhang L. Tumor-infiltrating B cells: their role and application in anti-tumor immunity in lung cancer. Cell Mol Immunol. (2019) 16:6–18. doi: 10.1038/s41423-018-0027-x
33. Fridman WH, Petitprez F, Meylan M, Chen TW, Sun CM, Roumenina LT, et al. B cells and cancer: To B or not to B? J Exp Med. (2021) 218. doi: 10.1084/jem.20200851
34. Zheng M, Li YM, Liu ZY, Zhang X, Zhou Y, Jiang JL, et al. Prognostic landscape of tumor-infiltrating T and B cells in human cancer. Front Immunol. (2021) 12:731329. doi: 10.3389/fimmu.2021.731329
35. Laumont CM, Nelson BH. B cells in the tumor microenvironment: Multi-faceted organizers, regulators, and effectors of anti-tumor immunity. Cancer Cell. (2023) 41:466–89. doi: 10.1016/j.ccell.2023.02.017
36. Kerr WG. Inhibitor and activator: dual functions for SHIP in immunity and cancer. Ann N Y Acad Sci. (2011) 1217:1–17. doi: 10.1111/j.1749-6632.2010.05869.x
37. Hamilton MJ, Ho VW, Kuroda E, Ruschmann J, Antignano F, Lam V, et al. Role of SHIP in cancer. Exp Hematol. (2011) 39:2–13. doi: 10.1016/j.exphem.2010.11.002
38. Gumbleton M, Sudan R, Fernandes S, Engelman RW, Russo CM, Chisholm JD, et al. Dual enhancement of T and NK cell function by pulsatile inhibition of SHIP1 improves antitumor immunity and survival. Sci Signal. (2017) 10. doi: 10.1126/scisignal.aam5353
39. Fernandes S, Iyer S, Kerr WG. Role of SHIP1 in cancer and mucosal inflammation. Ann N Y Acad Sci. (2013) 1280:6–10. doi: 10.1111/nyas.12038
40. Fu Q, Huang Y, Ge C, Li Z, Tian H, Li Q, et al. SHIP1 inhibits cell growth, migration, and invasion in non−small cell lung cancer through the PI3K/AKT pathway. Oncol Rep. (2019) 41:2337–50. doi: 10.3892/or.2019.6990
41. Wan J, Yourshaw M, Mamsa H, Rudnik-Schöneborn S, Menezes MP, Hong JE, et al. Mutations in the RNA exosome component gene EXOSC3 cause pontocerebellar hypoplasia and spinal motor neuron degeneration. Nat Genet. (2012) 44:704–8. doi: 10.1038/ng.2254
42. Pefanis E, Wang J, Rothschild G, Lim J, Chao J, Rabadan R, et al. Noncoding RNA transcription targets AID to divergently transcribed loci in B cells. Nature. (2014) 514:389–93. doi: 10.1038/nature13580
Keywords: non-small cell lung cancer, immunity B cell, single-nucleotide polymorphism, overall survival, tumor microenvironment
Citation: Lu G, Liu H, Wang H, Tang X, Luo S, Du M, Christiani DC and Wei Q (2024) Potentially functional variants of INPP5D and EXOSC3 in immunity B cell-related genes are associated with non-small cell lung cancer survival. Front. Immunol. 15:1440454. doi: 10.3389/fimmu.2024.1440454
Received: 29 May 2024; Accepted: 22 July 2024;
Published: 08 August 2024.
Edited by:
Jiaheng Xie, Central South University, ChinaReviewed by:
Huaping Xiao, Mayo Clinic, United StatesXuemei Zhang, North China University of Science and Technology, China
Copyright © 2024 Lu, Liu, Wang, Tang, Luo, Du, Christiani and Wei. This is an open-access article distributed under the terms of the Creative Commons Attribution License (CC BY). The use, distribution or reproduction in other forums is permitted, provided the original author(s) and the copyright owner(s) are credited and that the original publication in this journal is cited, in accordance with accepted academic practice. No use, distribution or reproduction is permitted which does not comply with these terms.
*Correspondence: Guojun Lu, Z3VvanVubHVAbmptdS5lZHUuY24=; Qingyi Wei, cWluZ3lpLndlaUBkdWtlLmVkdQ==