- 1Department of Orthopedics, Southern Medical University Pingshan Hospital (Pingshan District Peoples’ Hospital of Shenzhen), Shenzhen, Guangdong, China
- 2Department of Sport Medicine, Huazhong University of Science and Technology Union Shenzhen Hospital (Nanshan Hospital), Shenzhen, China
Background: The current understanding of the mechanisms by which metal ion metabolism promotes the progression and drug resistance of osteosarcoma remains incomplete. This study aims to elucidate the key roles and mechanisms of genes involved in cuproptosis-related sphingolipid metabolism (cuproptosis-SPGs) in regulating the immune landscape, tumor metastasis, and drug resistance in osteosarcoma cells.
Methods: This study employed multi-omics approaches to assess the impact of cuproptosis-SPGs on the prognosis of osteosarcoma patients. Lasso regression analysis was utilized to construct a prognostic model, while multivariate regression analysis was applied to identify key core genes and generate risk coefficients for these genes, thereby calculating a risk score for each osteosarcoma patient. Patients were then stratified into high-risk and low-risk groups based on their risk scores. The ESTIMATE and CIBERSORT algorithms were used to analyze the level of immune cell infiltration within these risk groups to construct the immune landscape. Single-cell analysis was conducted to provide a more precise depiction of the expression patterns of cuproptosis-SPGs among immune cell subtypes. Finally, experiments on osteosarcoma cells were performed to validate the role of the cuproptosis-sphingolipid signaling network in regulating cell migration and apoptosis.
Results: In this study, seven cuproptosis-SPGs were identified and used to construct a prognostic model for osteosarcoma patients. In addition to predicting survival, the model also demonstrated reliability in forecasting the response to chemotherapy drugs. The results showed that a high cuproptosis-sphingolipid metabolism score was closely associated with reduced CD8 T cell infiltration and indicated poor prognosis in osteosarcoma patients. Cellular functional assays revealed that cuproptosis-SPGs regulated the LC3B/ERK signaling pathway, thereby triggering cell death and impairing migration capabilities in osteosarcoma cells.
Conclusion: The impact of cuproptosis-related sphingolipid metabolism on the survival and migration of osteosarcoma cells, as well as on CD8 T cell infiltration, highlights the potential of targeting copper ion metabolism as a promising strategy for osteosarcoma patients.
1 Introduction
Osteosarcoma, an intensely aggressive malignant tumor originating from bone, predominantly affects adolescents (1–3). Recent studies have revealed that disruptions in metabolic pathways and alterations in the immune microenvironment significantly contribute to the pathogenesis of osteosarcoma (4–7). In the tumor microenvironment, tumor cells reprogram both the immune and metabolic landscapes to augment their proliferative, survival, and metastatic potential (8–10).
Sphingolipids are critical components of the cellular membrane surface, essential for maintaining the structural integrity of sphingolipids (11, 12). Sphingolipids and their metabolic derivatives are involved in regulating processes including apoptosis and autophagy. Sphingolipids and their metabolic derivatives are involved in regulating processes including apoptosis and autophagy (13, 14). The metabolism of sphingolipids also plays a role in the interactions between tumor cells and the immune system, influencing tumor cell behavior and the functionality of immune responses (15–17). Regulation of these metabolic pathways may reveal new therapeutic strategies for osteosarcoma.
Copper is an essential trace element involved in the activity and function of multiple enzymes, significantly impacting cellular energy production, neural conduction, antioxidative defense, and the absorption and metabolism of iron (18–21). The relationship between copper ion metabolism and tumor development has become increasingly clear, particularly with the discovery of a new mode of cell death induced by copper-cuproptosis (22, 23). Cuproptosis involves copper directly binding to certain intracellular fatty acid synthases, leading to protein aggregation and cellular dysfunction (24). This mechanism may provide targets for developing new therapies against refractory tumors such as osteosarcoma.
Studies indicate that metal ions like copper and iron can affect sphingolipid metabolism. These metal ions are involved in regulating the activity of enzymes directly related to sphingolipid metabolic pathways, affecting cell signaling and the physical properties of membranes. Additionally, copper ions are hypothesized to exert an indirect influence on the synthesis and degradation pathways of sphingolipids. Recent discoveries have delineated cuproptosis as a distinct form of cell death, uniquely precipitated by copper exposure (25). This process is markedly different from other established cell death mechanisms. Experimental validations have demonstrated that copper can provoke apoptosis through activation of aSMase, leading to subsequent ceramide release (26, 27). Despite these advancements, the connection between sphingolipid metabolism and cuproptosis remains nascent. However, it is understood that any dysregulation in sphingolipid metabolism might influence various cell death pathways. Since sphingolipids are crucial components of the cell membrane and affect multiple cellular signaling pathways, their metabolic changes may influence the cellular stress responses induced by copper. Understanding how sphingolipids regulate the pathways of copper-induced cell death will provide crucial insights into the molecular mechanisms of cuproptosis and the development of new anti-tumor strategies.
In elucidating the mechanistic progression of tumor diseases, multi-omics plays a pivotal role (28–30). By integrating multi-omic datasets with single-cell analysis methods, we explored the value of cuproptosis-related sphingolipid metabolism (cuproptosis-SPGs) in predicting outcomes and treatment efficacy in osteosarcoma patients, and revealed the impact of cuproptosis-SPGs on immune cell infiltration in osteosarcoma tissues. These studies provide a theoretical basis for future osteosarcoma research focusing on copper ion metabolism therapies, highlighting the interactions between copper ions and sphingolipid metabolism, and how they jointly regulate tumor cell behavior and immune system functionality. In-depth research in this field is expected to drive the development of a new generation of anti-tumor therapies.
2 Materials and methods
2.1 Data collection
To conduct our study on sphingolipid metabolism, a total of 160 genes were retrieved from the InnateDB (https://www.innatedb.com/), which are known to be implicated in this metabolic pathway. Additionally, transcriptomic datasets along with comprehensive clinical profiles for osteosarcoma patients were procured from the TARGET initiative (https://ocg.cancer.gov/programs/target). In total, transcriptomic profiles were obtained for 88 individuals diagnosed with osteosarcoma. However, complete clinical records were available for only 85 of these patients. Consequently, transcriptomic data from the remaining three patients were excluded from the study, thereby enabling subsequent analyses to proceed with the cohort of 85 osteosarcoma patients. The U-2 OS cell line, characterized by its epithelial morphology, originates from a sarcoma of moderate differentiation. This line exhibits adherent growth characteristics and displays significant chromosomal alterations. The osteosarcoma cell line U2OS (KCB200962YJ) was procured from Kmcellbank, serving as a crucial biological model for our analyses.
2.2 Establishment of risk scoring model
To evaluate the prognostic influence of distinct cuproptosis-SPGs, univariate Cox regression analyses were undertaken, linking their differential expression with survival durations (31). Genes demonstrating a significant association with survival metrics were identified as prospective elements for subsequent LASSO regression (32, 33). Selection of the optimal penalty parameter (λ) for the model was achieved through 10-fold cross-validation, utilizing a criterion of minimality. Computation of the final risk score was based on normalized expression values and coefficients of the selected variables. The formula is presented as follows: B4GALNT1*0.0314 + SGMS2*0.1847 + ABCA2*0.3275 - B3GALT4*0.0206 -ST3GAL2*0.1409 - NPC2*0.1068 - APOE*0.0882.
Following the development of the model, individuals in the TARGET-OS cohort were stratified into high-risk and low-risk categories, centered on the median score from the training set. Differences in survival rates between these categories were assessed using Kaplan-Meier survival plots and log-rank tests, facilitated by the “survival” and “survivalminer” software packages. Additionally, the model’s predictive accuracy was evaluated over 1, 3, and 5 years, using receiver operating characteristic (ROC) analysis (34, 35), implemented via an R package.
2.3 Decision curve analysis
To assess the clinical relevance of the prognostic framework, decision curve analysis (DCA) curves were generated using the “ggDCA” package. This methodology facilitates the identification of patient risk and quantifies the net benefit of the predictive model across varying threshold probabilities.
2.4 Functional enrichment analysis
For functional enrichment assessment, the “clusterProfiler” R package was employed to analyze functional enrichment based on differentially expressed genes (DEGs) between high-risk and low-risk groups. Additionally, single-sample Gene Set Enrichment Analysis (ssGSEA) was performed for each individual in both cohorts (36). Pathways that were significantly enriched were depicted through heatmaps illustrating the average ssGSEA scores.
2.5 Evaluation of immune landscape
The algorithms CIBERSORT and ESTIMATE were employed to determine the relative abundance and infiltration levels of immune cell subtypes, respectively (37). Spearman’s method was utilized to assess the correlation between the Riskscore and the degree of immune infiltration as per ESTIMATE. Additionally, the immune scores for TIL subgroups were derived using ssGSEA. Furthermore, ssGSEA in the GSVA packages was applied to quantify immune features within each sample.
2.6 Single-cell analysis of cuproptosis-SPGs expression in osteosarcoma tissues
For the processing of single-cell RNA sequencing data, we employed the R package Seurat (38, 39), version 4.1.0, to facilitate the log-normalization and standardization of single-cell transcriptomic profiles (40). Rigorous quality control measures were implemented, evaluating cells based on gene expression within the count matrix and the proportion of mitochondrial gene counts. Cells expressing more than 1500 genes were excluded, along with those exhibiting over 5% mitochondrial gene count. Subsequently, data normalization across the library size was conducted using the “normalize_total” function from the Scanpy toolkit. This normalization facilitated the generation of a logarithmically transformed data matrix, which was utilized in subsequent analytical processes. Ultimately, eight distinct cell types were annotated.
2.7 Drug sensitivity analysis and cell cytotoxicity detection
To investigate variations in drug responsiveness between low-risk and high-risk cohorts, we utilized the R package “pRRophetic” (41). Subsequently, differentially expressed genes (DEGs) distinguishing these groups were integrated into the Connectivity Map to pinpoint therapeutic agents that could potentially benefit patients at higher risk. Following this, the U2OS cell line was treated with roscovitine to assess the impact of cuproptosis-SPGs on drug sensitivity via cytotoxic assays.
2.8 Migration ability impacted by cuproptosis-SPGs
The impact of cuproptosis-SPGs on the migratory abilities of U2OS cells was evaluated utilizing both wound-healing and transwell migration assays (42). In the wound-healing assay, siRNA was employed to disrupt U2OS cells over a period of 72 hours. Subsequently, a deliberate “wound” was created within the cell monolayer, and cell migration rates were assessed after 48 hours. Additionally, siRNA-treated U2OS cells were placed in the upper chamber of a transwell setup. The lower chamber was supplied with 15% fetal bovine serum to serve as a chemoattractant for the U2OS cells. The number of cells present in the lower chamber after 36 hours was quantified to gauge cellular migration capabilities (43).
2.9 Statistical analysis
In this study, the analytical procedures were performed using RStudio, release 4.1.3. To assess overall survival differences, we utilized the log-rank test along with Kaplan-Meier survival analysis. For processing Western blot data, software such as ImageJ and GraphPad Prism were applied. Pearson’s method of correlation analysis was employed to execute all correlation assessments. Evaluation of disparities between the groups was conducted through the unpaired, two-tailed Student’s t-test, with a significance threshold set at P<0.05.
3 Results
3.1 Cuproptosis-SPGs for predicting osteosarcoma survivals
We conducted univariate Cox regression analysis on 158 cuproptosis-SPGs, identifying 32 of them as prognostically significant genes in osteosarcoma. Of these, 12 were associated with lower survival rates (Figure 1A). Subsequent to this identification, we employed LASSO regression modeling based on the same set of 32 cuproptosis-SPGs. This process culminated in the selection of seven core cuproptosis-SPGs for the construction of a prognostic model for osteosarcoma patients (Figures 1B, C). Multivariable Cox regression analysis elucidated the risk coefficients for these seven SPGs, categorizing patients into high and low-risk groups (Figure 1D). Kaplan-Meier survival plots revealed that patients in the high-risk category exhibited significantly poorer prognoses compared to those scored as low-risk (Figure 1E). The predictive capacity of the cuproptosis-SPGs model was evaluated using ROC curves. The model demonstrated impressive predictive accuracy with an AUC of 0.874 at two years and 0.837 at five years (Figure 1F).
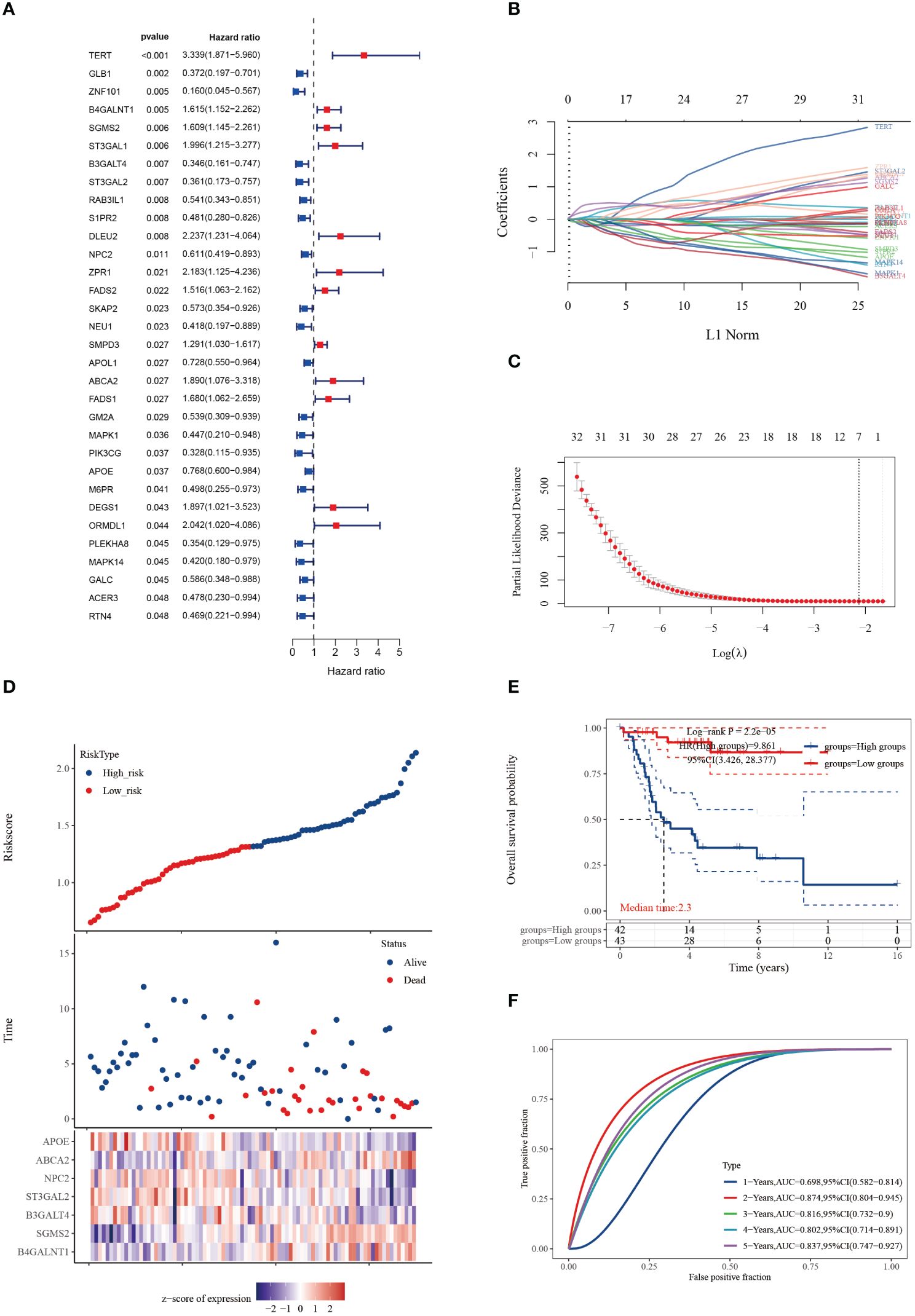
Figure 1 Cuproptosis-SPGs for predicting osteosarcoma survivals. (A) Differential gene analysis was employed to identify cuproptosis-SPGs correlated with osteosarcoma. (B) Coefficients for 32 cuproptosis-SPGs. (C) Employing the Lasso technique, a prognostic model based on 7 SRGs was formulated. (D) Expression levels of the foremost 7 cuproptosis-SPGs were graphically depicted to illustrate prognostic risk distribution. (E) KM plot was conducted to further explore the prognostic relevance of the 7 cuproptosis-SPGs across diverse osteosarcoma subtypes. (F) The prognostic model’s predictive efficacy was assessed via ROC analysis.
3.2 Cuproptosis-SPGs model provides clinical benefits for osteosarcoma patients
Following the construction of the prognostic model, we further assessed its overall accuracy and credibility using the C-index (Figure 2A). Calibration curves additionally demonstrated the predictive capacity of the model, showing good consistency with actual outcomes over 1, 3, and 5 years (Figure 2B). Moreover, osteosarcoma patients classified as high risk exhibited an increased cumulative hazard (Figure 2C), suggesting that Cuproptosis-SPGs may serve as a potential marker for the progression of osteosarcoma patients. Decision curve analysis indicated that the drug decisions based on nomograms provided greater benefits for osteosarcoma patients compared to other individual indicators (Figure 2D).
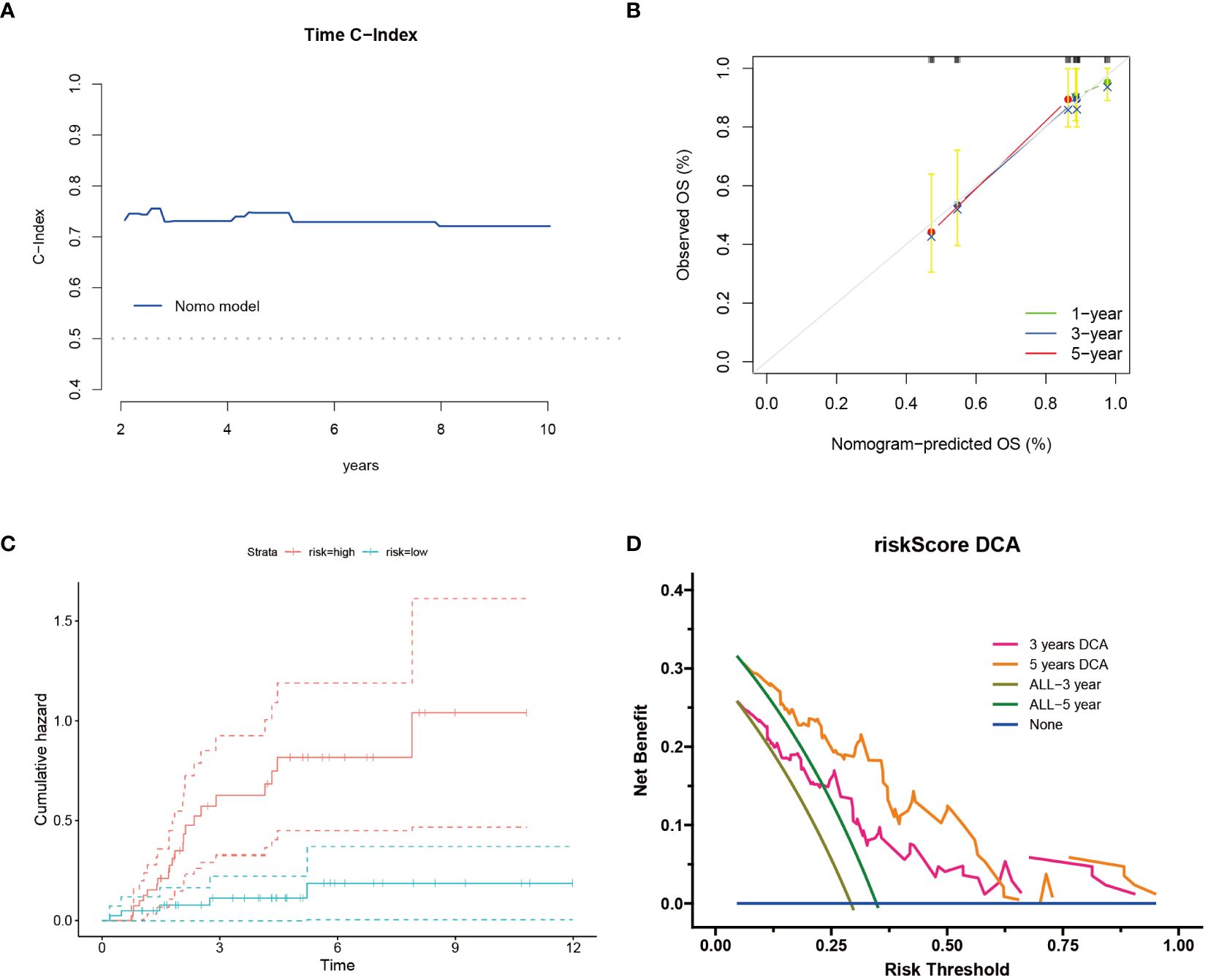
Figure 2 Cuproptosis-SPGs model provides clinical benefits for osteosarcoma patients. (A) Time C-Index plot showcases the model’s discriminative ability over time. (B) Calibration plot demonstrates the agreement between predicted and observed outcomes. (C) Cumulative risk curves illustrate the evolving risk estimates. (D) Decision curve analysis evaluates the net benefit of utilizing the model in clinical decision-making.
3.3 The classification of osteosarcoma groups based on Cuproptosis-SPGs reveals distinct immune networks
The tumor microenvironment plays a pivotal role in the development and progression of cancer. In this study, patients with osteosarcoma were stratified into high-risk and low-risk categories utilizing the Cuproptosis-SPGs model. Differential gene expression analysis was conducted for both groups, followed by functional enrichment studies. This approach identified 79 differentially expressed genes (DEGs), the majority of which were upregulated (Figure 3A). Functional enrichment of these DEGs highlighted extensive involvement in immune response processes and sphingolipid metabolism between the high and low-risk groups (Figures 3B, C). Among these, four Gene Ontology (GO) processes showed significant alterations (Figure 3D). The copper ion binding process and sphingomyelin metabolic process are linked through the extracellular structure organization, suggesting that the extracellular structure may act as a bridge in the sphingolipid-cuproptosis network (Figure 3E).
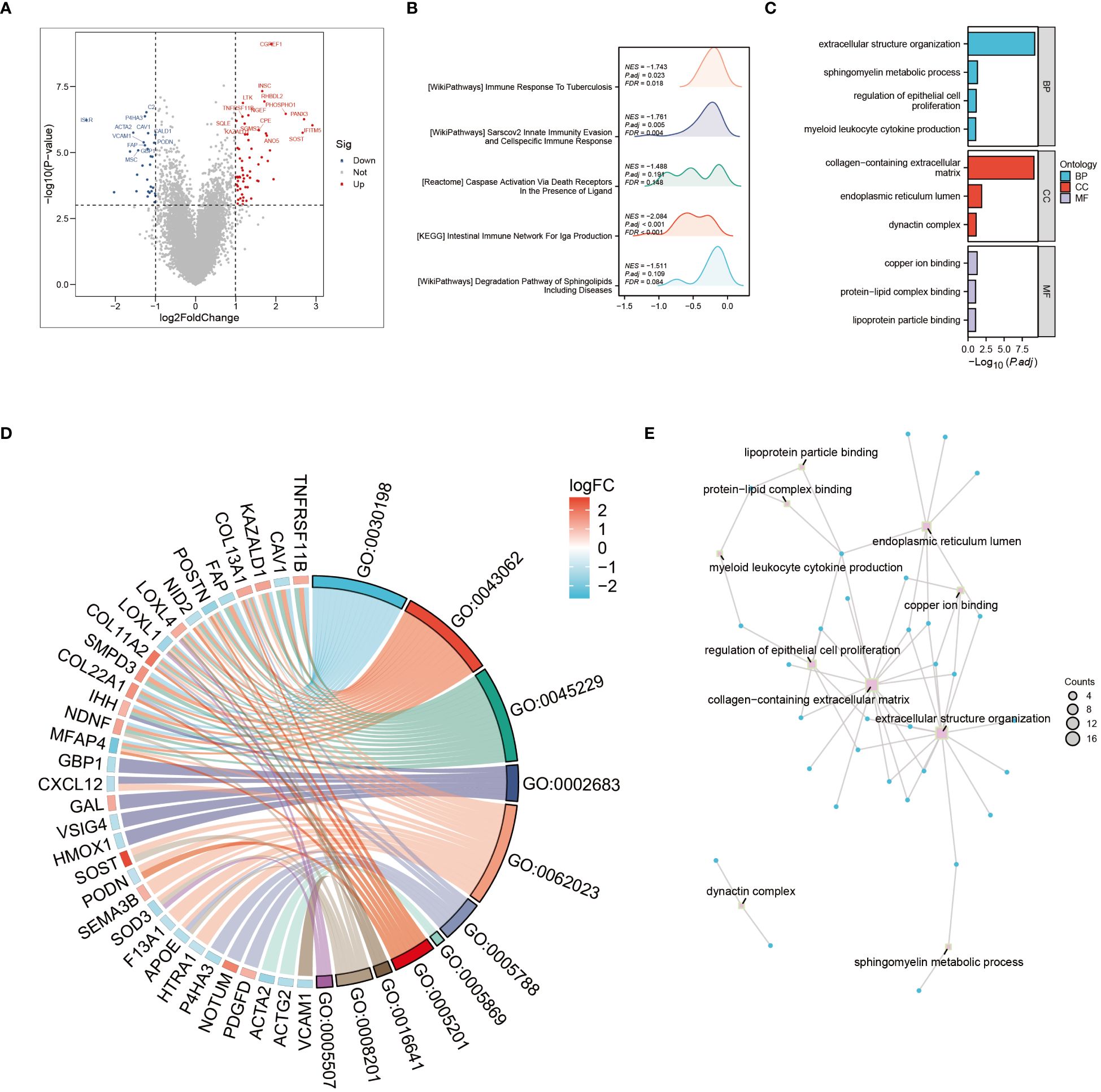
Figure 3 Go and KEGG enrichment analysis. (A) Volcano plot illustrating differential genes in high- and low-risk osteosarcoma patients. (B) Mountain plot depicting enrichment of differential genes in immune pathways. (C) Enrichment of differential genes in Gene Ontology (GO) terms related to Biological Processes (BP), Cellular Components (CC), and Molecular Functions (MF). (D) Pathways enriched in GO analysis and corresponding differential genes. (E) Interconnections between enriched pathways.
3.4 Interactions between cuproptosis and sphingolipid metabolic genes
To elucidate the interplay between cuproptosis metabolism and sphingolipid metabolism, correlations between genes involved in cuproptosis and sphingolipid pathways were investigated. As depicted in Figure 4A, a notable, albeit negative, correlation persists between these gene sets. Noteworthy, the correlation between FDX1 and B4GALNT1 was found to be the most significant (P < 0.01) (Figure 4B). Additionally, the heatmap in Figure 4C illustrates the correlation levels among cuproptosis genes, with DAL and DLD exhibiting the highest correlation coefficient (R = 0.52).
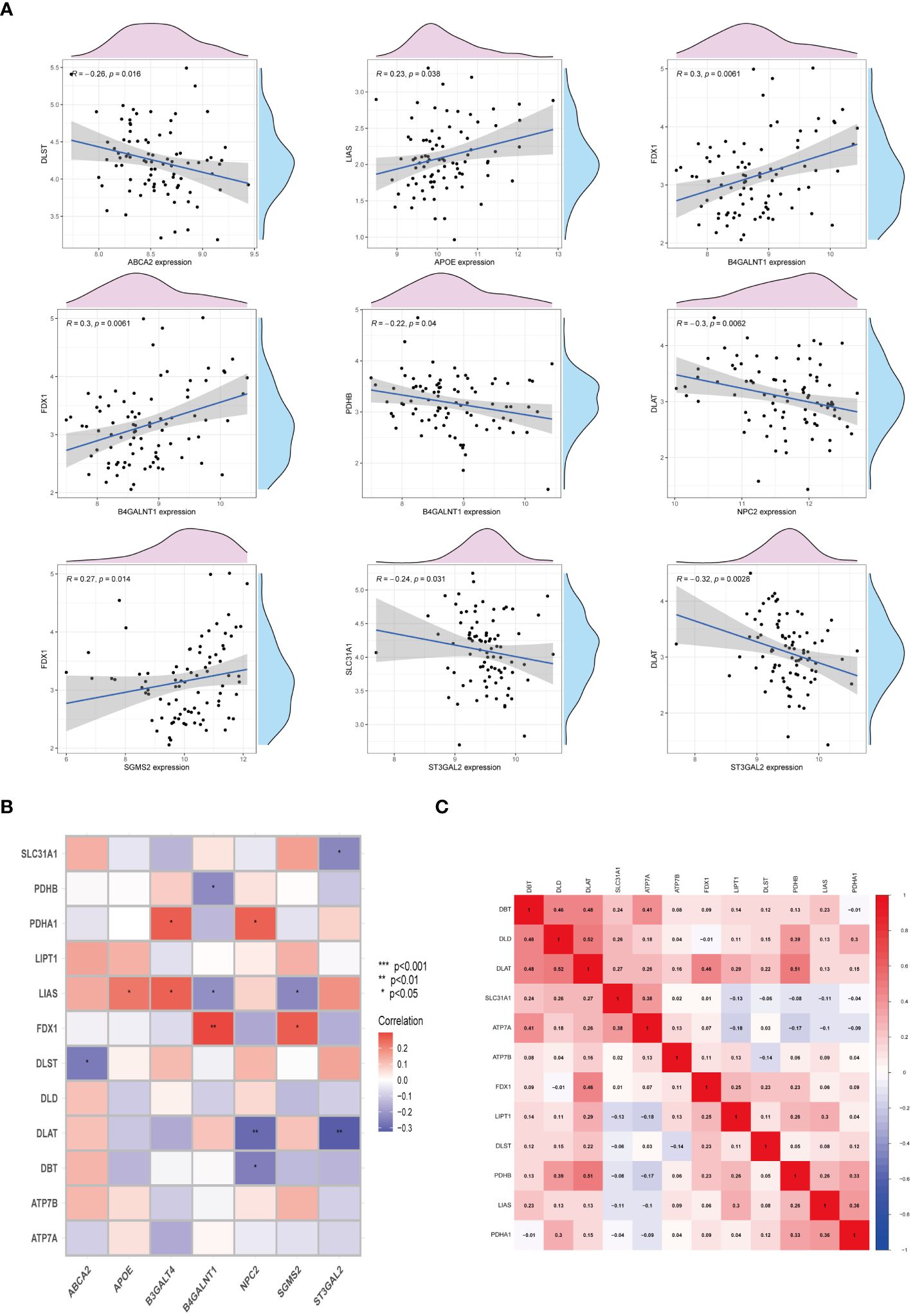
Figure 4 Interactions between cuproptosis and sphingolipid metabolic genes. (A, B) Relationship of cuproptosis-SPGs and cuproptosis genes. (C) Heatmap exhibits the association among cuproptosis-SPGs. (*P < 0.05, **P < 0.01).
3.5 Single-cell analysis exhibits Cuproptosis-SPGs expression levels in tumor microenvironment
Examination at the level of individual cells has unveiled a significant presence of T cell and monocyte/macrophage subsets within the immune context of osteosarcoma (Figures 5A, B). By employing UMAP analysis, we identified 28 unique clusters across eight cellular subtypes, revealing diverse subpopulations of cells (Figures 5C, D). Employing heatmap visualization methods, distinctive patterns of interaction between osteoblasts and fibroblasts were emphasized (Figure 5E). To delve into the expression patterns of SRGs within the tumor microenvironment in osteosarcoma, we explored the expression levels of the top six ranked SRGs in each cell subtype (Figures 5F, G). A pivotal finding was the notable decrease in peptide antigen binding pathways (Figures 6A, C), coupled with a notable increase in ATP synthase activity in T cells (Figures 6B, D). Analysis of SRGs showed a consistent rise in APOE expression among different cell types (Figure 7A). Given the variety of T cell subsets discovered in osteosarcoma, additional studies were initiated to explore the interactions between these lymphocytes and the tumor cells (Figures 7B–D). Investigative efforts into the transcription factors in tumor cells highlighted potential pathways influencing differential expression (Figure 7E).
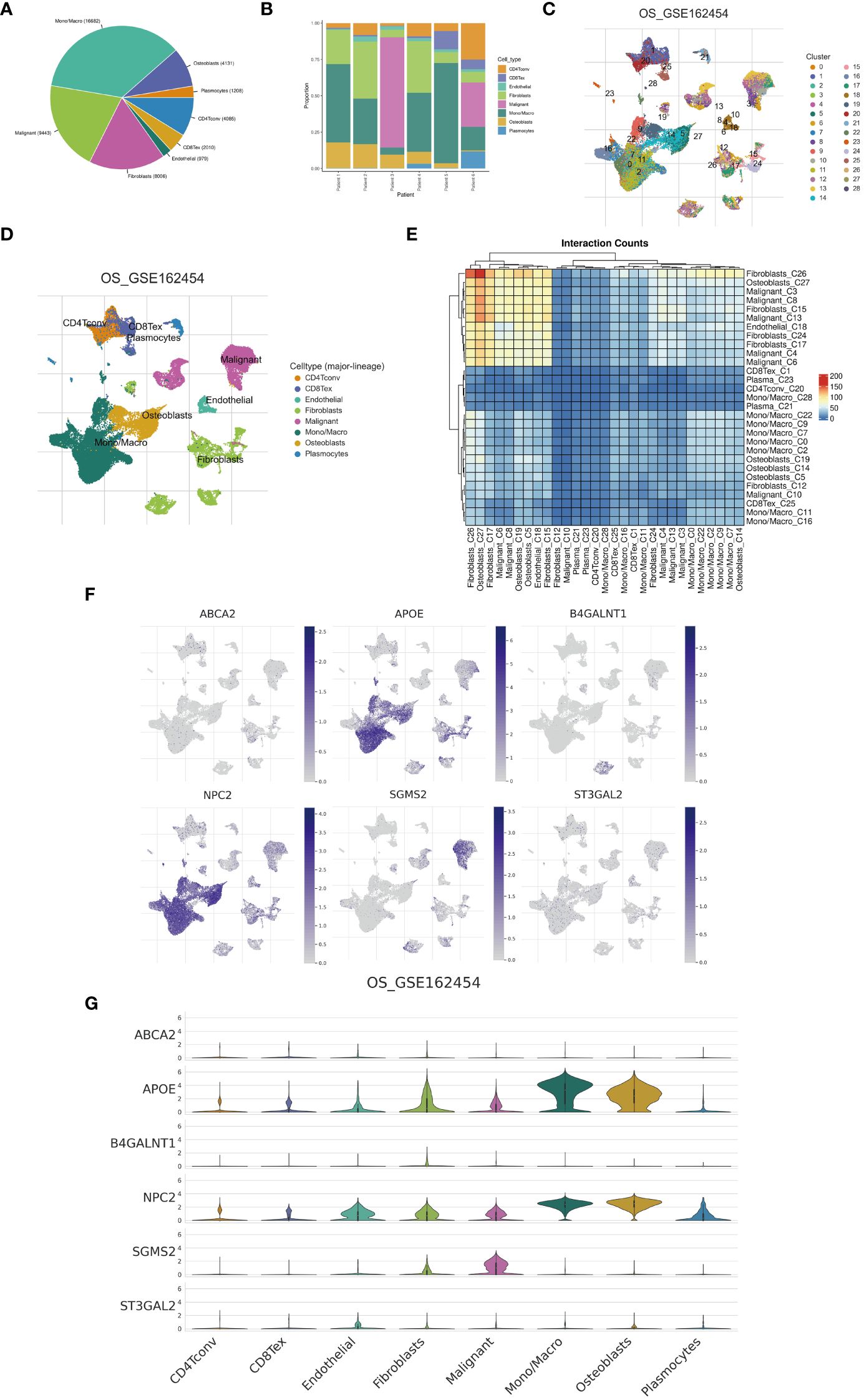
Figure 5 Single-cell analysis. (A) A pie chart illustrates the proportional representation of all cellular subpopulations. (B) Bar graphs depict the distribution of cellular subpopulations for each osteosarcoma patient. (C, D) UMAP visualization demonstrates the clustering of cellular subpopulations. (E) A heatmap displays the interaction counts among cellular subpopulations. (F, G) Distribution patterns of cuproptosis-SPGs between different cells are depicted.
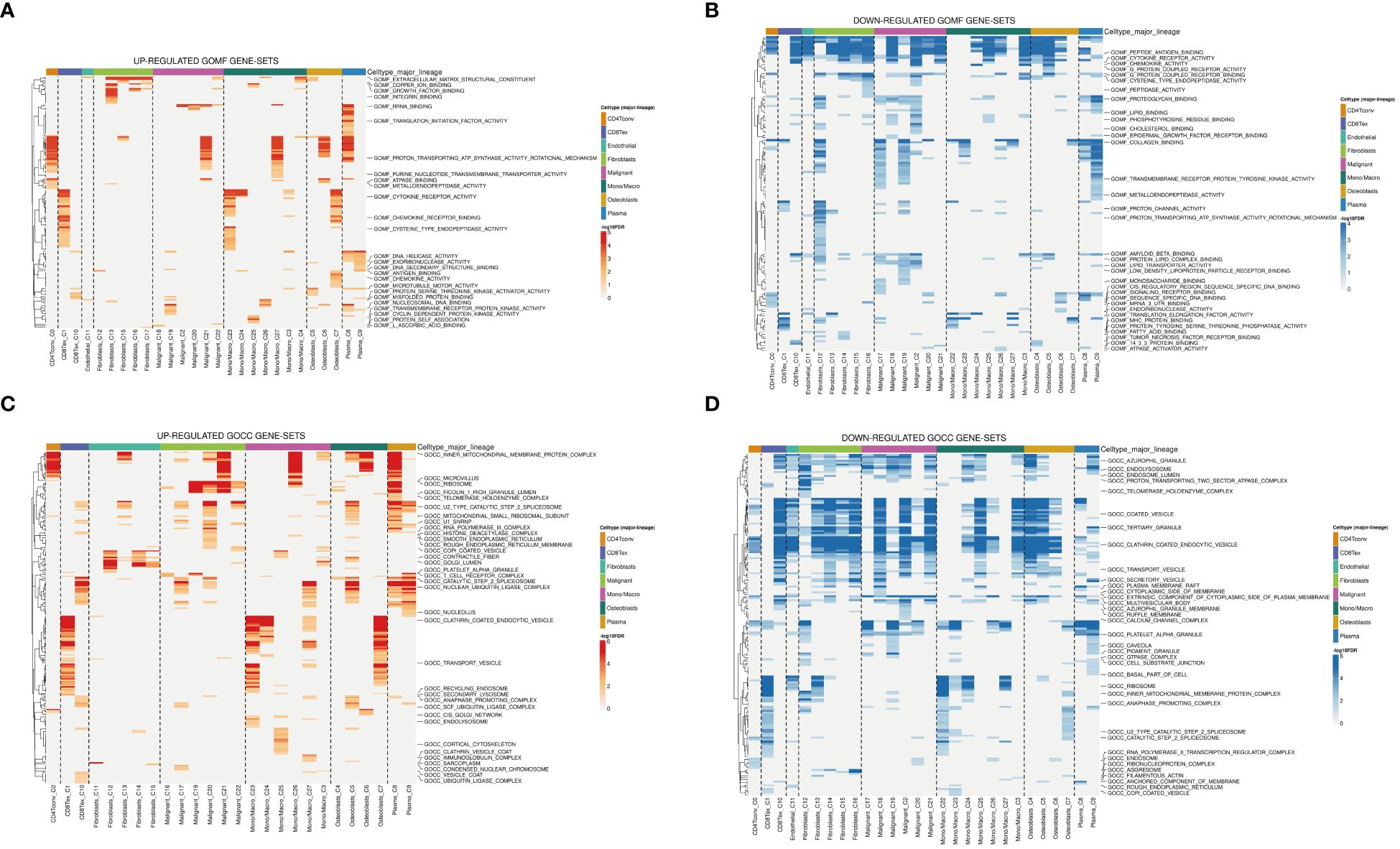
Figure 6 Analysis of GO terms in osteosarcoma patients. (A, B) Upregulated and downregulated gene sets in GOMF. (C, D) Upregulated and downregulated gene sets in GOCC.
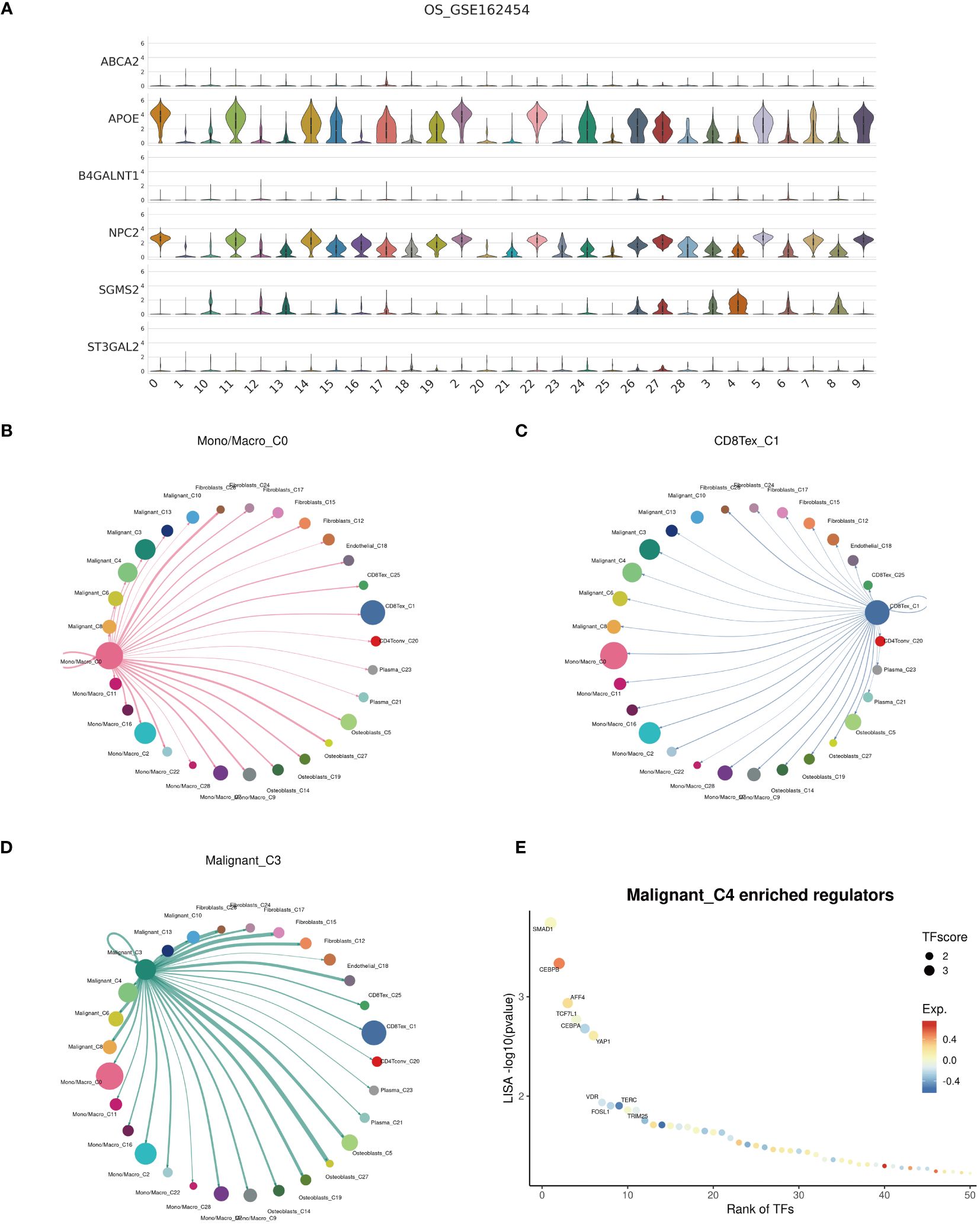
Figure 7 Cell communication. (A) Expression profiles of cuproptosis-SPGs within cellular subgroups clusters. (B–D) Interactions among Mono/Macro, T cells, and malignant cells within the osteosarcoma cellular subgroups. (E) Transcription factor regulation of osteosarcoma cells.
3.6 Alterations in CD8 T Cell infiltration mediated by Cuproptosis-SPGs
In patients with osteosarcoma, there exists a stark contrast in the levels of Cuproptosis-SPGs between those at high risk and those at low risk (Figure 8A). An estimation of cellular proportions indicates a diminished presence of CD8+ T cells in the high-risk group (Figure 8B). Analyses of the tumor immune microenvironment (TME) reveal significant variances not only in CD8 T cells but also in NK cells and Tregs across different risk categories of osteosarcoma patients (Figures 8C, D). These differences were corroborated by an analysis linking risk scores with immune cell correlations (Figure 9A). Given that risk scores are derived from Cuproptosis-SPGs, further investigation into the association between Cuproptosis-SPGs and the infiltration levels of CD8 T cells, NK cells, and Tregs was conducted. The results demonstrate a significant negative correlation between ABCA2 and the infiltration of CD8 T cells (Figure 9B), suggesting that ABCA2 may be a critical Cuproptosis-SPG gene influencing anti-tumor immunity.
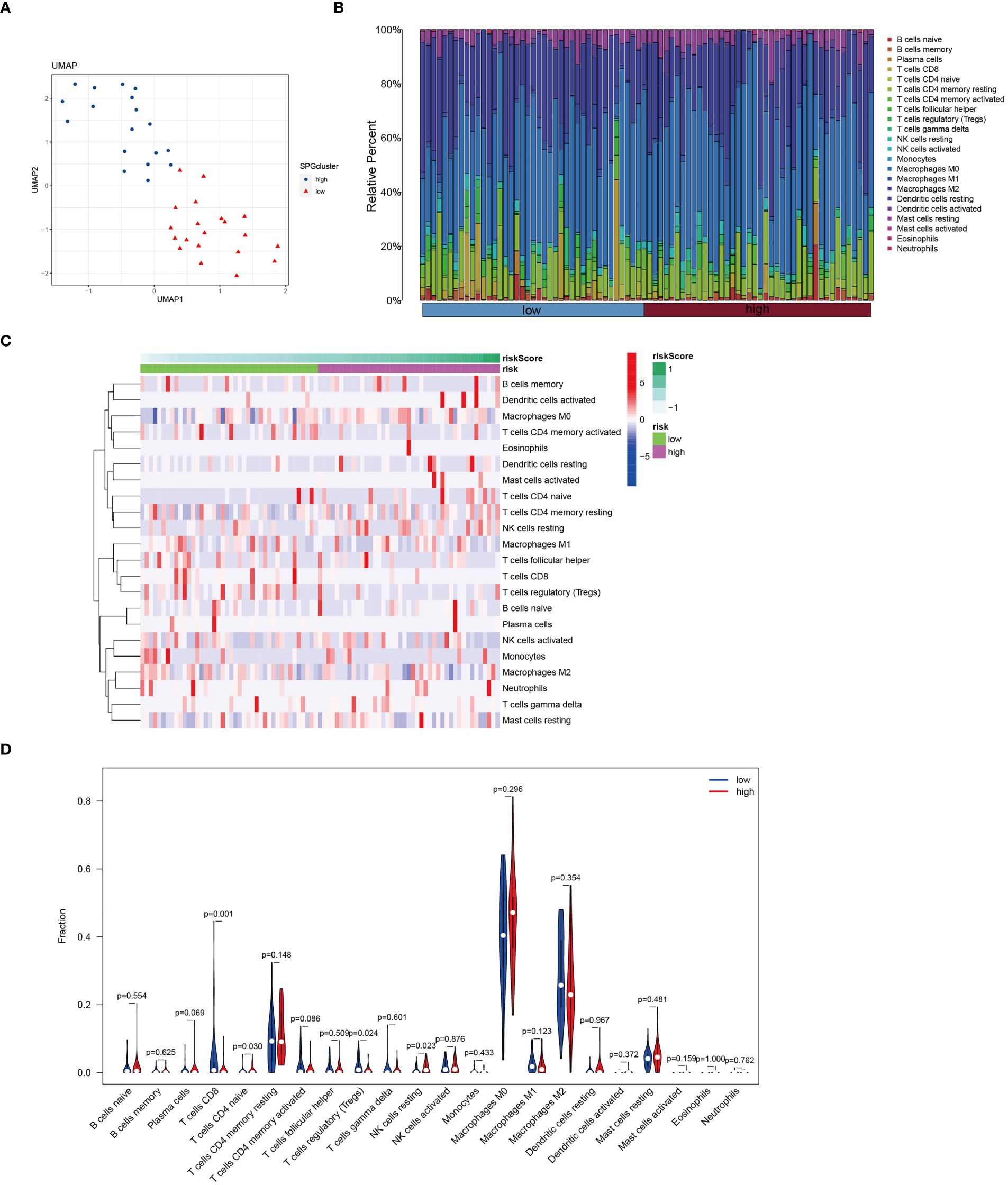
Figure 8 Immune features of cuproptosis-SPGs classified osteosarcoma subtypes. (A) UMAP visualization showcases the expression levels of cuproptosis-SPGs within immune cells. (B) CIBERSORT reveals the proportions of immune cell components in osteosarcoma patients. (C) The level of immune cell infiltration for each osteosarcoma patient is illustrated. (D) Variations in immune cell infiltration between high-risk and low-risk osteosarcoma patients are delineated.
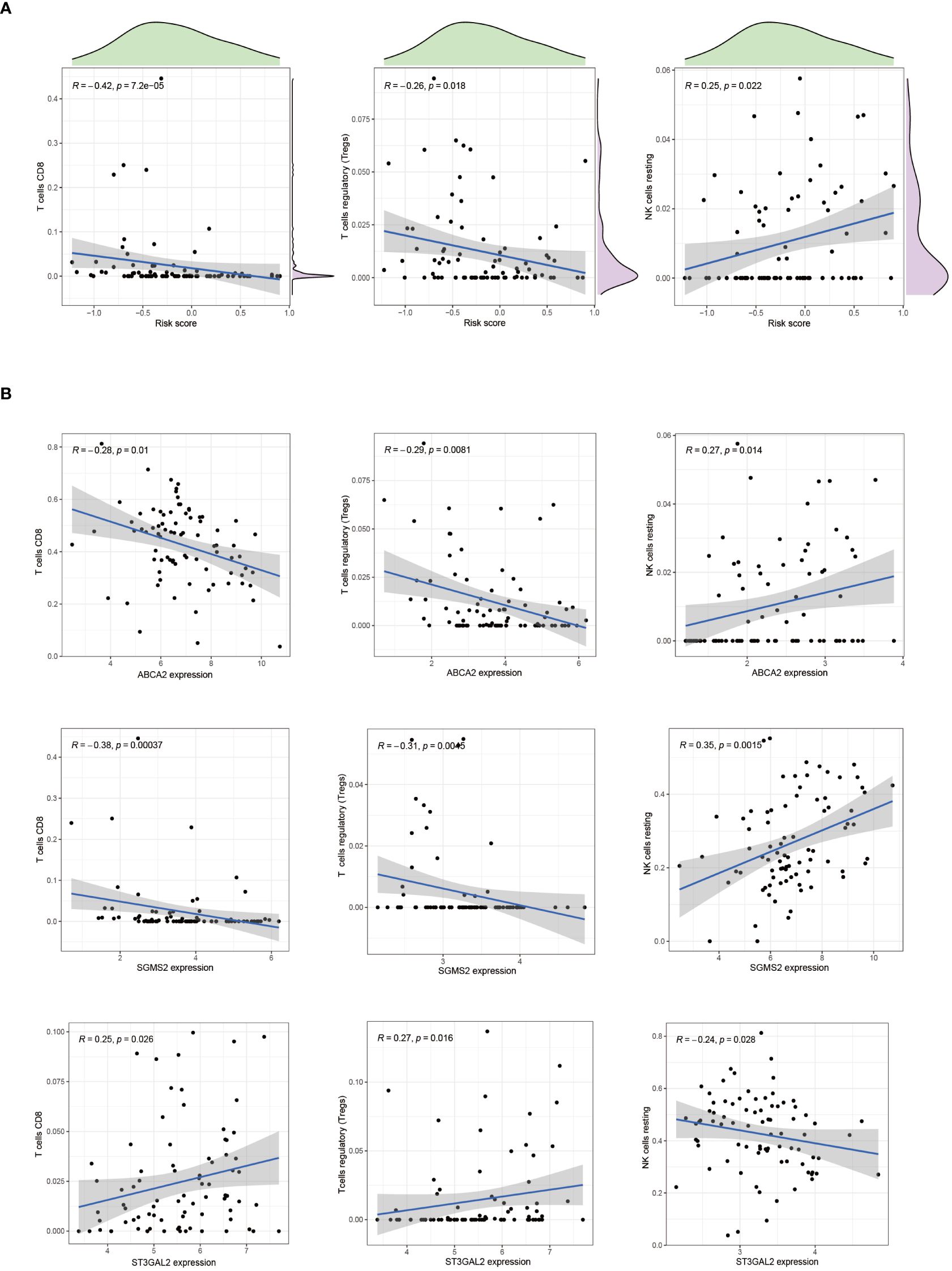
Figure 9 The association between immune cells and riskscore. (A) Correlation exists between CD8 T cells, Treg cells, NK cells, and riskscore. (B) The correlation between CD8 T cells, Treg cells, NK cells, and cuproptosis-SPGs.
3.7 Drug response differences and validations
The emergence of chemoresistance presents a significant challenge in the treatment of tumors. Our predictive analyses suggest that patients with high-risk osteosarcoma exhibit enhanced sensitivity to roscovitine, metformin, and bortezomib compared to those with low-risk osteosarcoma (Figure 10). Given the substantial weight of ABCA2 in the risk assessment, further investigations were conducted to explore its role in mediating drug sensitivity in osteosarcoma. After transfection with siRNA, osteosarcoma U2OS cells were exposed to varying concentrations of roscovitine for 48 hours. Cytotoxicity assays revealed that, relative to controls, cells with ABCA2 knockdown demonstrated reduced cytotoxic response to roscovitine (Figure 11A), with more pronounced differences at concentrations between 100–200 µM (Figure 11B). These findings align with the predictions from the drug sensitivity model, indicating that osteosarcoma patients with elevated ABCA2 expression are more susceptible to roscovitine. Future research should elucidate the mechanisms by which ABCA2 influences drug sensitivity through its interactions with pathways such as cuproptosis and sphingolipid metabolism.
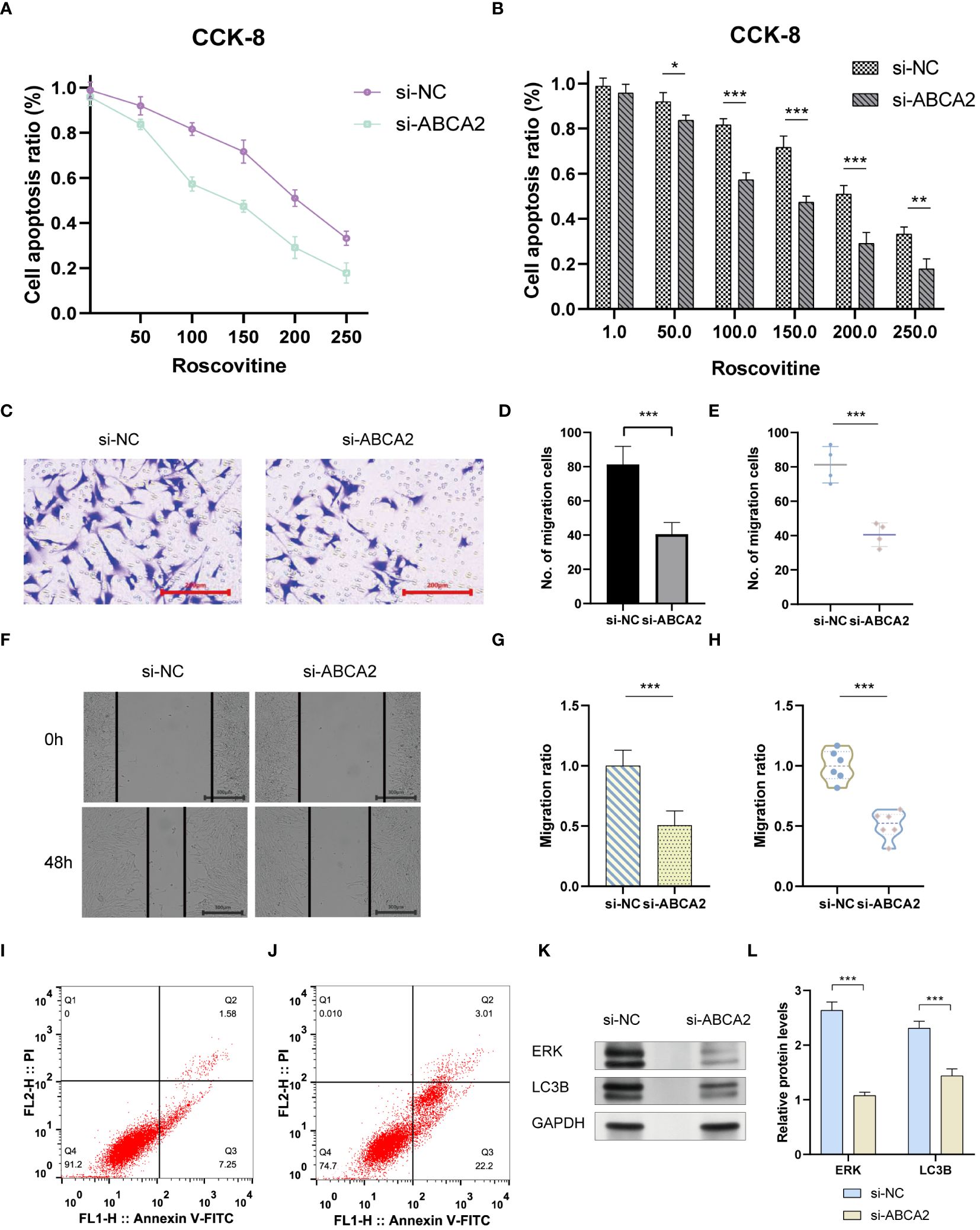
Figure 11 The impact of ABCA2 on tumor migration ability. (A) CCK-8 cell viability examination. (B) Bar graph showing the synergistic effect of ABCA2 inhibition at different concentrations of Roscovitine. (C) Transwell assay demonstrating osteosarcoma cells crossing through chambers after interference with ABCA2. (D, E) Bar graph and scatter plot illustrating the influence of ABCA2 on osteosarcoma cell migration capability. (F) Wound-healing assay showing migrated osteosarcoma cells after interference with ABCA2. (G, H) Bar graph and scatter plot demonstrating the inhibition of osteosarcoma cell migration capability by ABCA2. (I, J) Flow cytometry tests. (K, L) Western blot shows the influence of inhibition of ABCA2. (*P < 0.05, **P< 0.01, ***P< 0.001).
3.8 Cuproptosis-SPG regulates osteosarcoma cells migration ability
Following the validation of the drug sensitivity model using siRNA, the precision of the Cuproptosis-SPGs prognostic model was assessed. It was observed that 48 hours post siRNA treatment, the number of U2OS cells traversing the compartments was diminished (Figure 11C) and exhibited statistically significant differences (Figures 11D, E). Consistent with the transwell assay results, wound healing experiments indicated that inhibition of ABCA2 curtailed the migration distance of U2OS cells (Figure 11F), thus underscoring the role of ABCA2 in facilitating U2OS cell migration (Figures 11G, H).
3.9 Cuproptosis-SPG regulation of autophagy and MAPK proteins affects apoptosis in U2OS cells
Tumor cells evade apoptosis by remodeling their metabolism. We assessed the impact of the Cuproptosis-SPG gene ABCA2 on apoptosis in osteosarcoma cells using flow cytometry. It was observed that U2OS cells with disrupted ABCA2 were more susceptible to apoptosis, exhibiting an increased apoptotic rate of 16.39% compared to the control group (Figures 11I, J). Interestingly, after ABCA2 knockdown, autophagy levels in U2OS cells were also suppressed, and this was associated with hindered expression of ERK proteins (Figures 11K, L). These findings suggest that Cuproptosis-SPG may regulate the apoptotic process in osteosarcoma cells through both the autophagy and the MAPK pathways.
3.10 Cuproptosis-SPG expression patterns in pan-cancer
We further tried to explore the potential utility of Cuproptosis-SPG across additional tumor contexts. Our investigations incorporated an expression analysis of ABCA2 in normal tissues (Supplementary Figure S1A). The findings indicate a predominant expression of ABCA2 in immune cells, such as Gdh T cells (Supplementary Figure S1B). Notably, within a pan-cancer analysis, ABCA2 exhibited a significantly elevated expression in CHOL compared to normal tissues (Supplementary Figure S1C). Despite this, the correlation between ABCA2 expression levels and prognosis in CHOL was minimal (Supplementary Figure S1D).
4 Discussion
Osteosarcoma is characterized by complex epigenetic features and a high propensity for metastasis, frequently occurring in adolescents (44). Currently, chemotherapy and surgical interventions are effective predominantly in early-stage osteosarcoma patients (45). Those diagnosed with metastatic or recurrent osteosarcoma continue to face grim outcomes, with less than 30% achieving long-term survival (46). Clinical advancements for patients with advanced-stage osteosarcoma remain limited. Immunotherapy, as an emerging therapeutic approach, has yet to be extensively explored in osteosarcoma (47, 48).
In this study, we focused on the prognostic capabilities of copper metabolism and sphingolipid metabolism networks in osteosarcoma patients, elucidating the potential mechanisms through which cuproptosis-related sphingolipid genes (cuproptosis-SPGs) impact patient outcomes. We initially identified seven cuproptosis-SPGs and developed a prognostic model demonstrating robust predictive performance. Indeed, effective prediction models are crucial for the treatment of osteosarcoma patients, given the significant variability in pathological patterns among patients, which markedly influences treatment outcomes (49–51). Previous studies have shown that single genes or proteins can exhibit strong prognostic abilities (52–54). Metabolic alterations are key factors in cancer development and progression (55, 56), with sphingolipid metabolism playing a broad role in disease onset and serving a critical function in cancer progression and prognosis. It has been demonstrated that sphingolipid metabolism can modulate iron metabolism (57), such as ferroptosis, thereby inducing autophagy-related changes that lead to drug resistance (58). Additionally, sphingolipid metabolism regulates T lymphocyte calcium channels, contributing to tumor immune evasion (13, 59). Studies have explained the network between copper and aSMase, indicating the potential regulation mechanism in diseases’ development. However, no studies have yet disclosed the link between sphingolipid and copper metabolism in osteosarcoma. In our research, we identified seven cuproptosis-related sphingolipid metabolism genes (cuproptosis-SPGs) and clarified the connection between cuproptosis and sphingolipids (Figure 4). Extracellular structure organization may be a crucial hub in the cuproptosis-SPGs process (Figure 3E). The extracellular matrix (ECM) and other components provide structural and biochemical support to surrounding cells, facilitate intercellular communication, and influence cellular functions such as differentiation, migration, and adhesion. Sphingolipids are involved in the formation of focal adhesions (FAs), affecting the migratory capacity of cancer cells (60, 61). Our study found that knockdown of the cuproptosis-SPG gene ABCA2 significantly inhibited the migration ability of osteosarcoma cells (Figures 11C, F), suggesting that the sphingolipid-cuproptosis metabolic network jointly influences the formation of focal adhesions, thereby regulating cancer cell migration.
Drug resistance remains one of the greatest obstacles to successful cancer therapy (62, 63). Due to tumor heterogeneity, the molecular expression patterns vary among cancer patients (64–66), leading to significant differences in responses to anti-cancer treatments (67, 68). Based on cuproptosis-SPGs, we stratified osteosarcoma patients into high-risk and low-risk groups. The study demonstrated that the sphingolipid-cuproptosis metabolic network affects osteosarcoma patients’ drug sensitivity (Figure 10). Research by Vu NT et al. found that sphingolipids can regulate mitochondrial functions to drive ferroptosis in tumors, thereby altering tumor cell sensitivity to cisplatin (58). Our research is the first to reveal the significant role of the sphingolipid-cuproptosis metabolic network in osteosarcoma drug sensitivity and preliminarily identified autophagy and MAPK as key downstream regulatory pathways (Figure 11K).
Osteosarcoma patients exhibit unique immune infiltration characteristics associated with poor prognosis (69). Stratification of cancer patients based on multi-omics data facilitates the guidance of precision treatment strategies (70–72). Our study, using seven core cuproptosis-SPGs, developed a prognostic model that demonstrated excellent predictive performance (Figure 1F), offering clinical benefits to stratified osteosarcoma patients. Interestingly, different stratifications of osteosarcoma patients showed distinct immune infiltration. As delineated by the single-cell analysis, monocytes/macrophages represent the predominant cell type within the osteosarcoma tissue matrix, followed by CD8+ T cells (Figure 5A). These observations suggest that cuproptosis-SPGs may predominantly influence the infiltration levels of these two cell types. Subsequent multi-omic analyses revealed that within the macrophage population, the M0, M1, and M2 phenotypes indeed constituted the majority (Figure 8B). However, no significant differences were observed in the macrophage infiltration levels between the high-risk and low-risk groups, indicating that macrophage seems to influence limited in the progression of osteosarcoma. Notably, CD8 T cells, crucial for effective recognition and killing of tumor cells during the immune response (73, 74), were observed to have lower infiltration levels in high-risk osteosarcoma patients compared to those at low risk (Figure 8D). This suggests that the sphingolipid-cuproptosis metabolic network participates in the recruitment of CD8 T cells. Therefore, targeting cuproptosis-SPGs may enhance the efficacy of immunotherapies such as CAR-T in combating osteosarcoma.
Although our study has revealed the impact of cuproptosis-SPGs on the immune microenvironment and drug sensitivity in osteosarcoma, and has preliminarily identified potential regulatory mechanisms, there are several limitations to be acknowledged. Firstly, more osteosarcoma samples need to be collected to adjust the reliability and applicability of the model. Secondly, despite single-cell analysis revealing the immune infiltration landscape, these conclusions still require further validation in in vivo experiments. Similarly, experiments on drug sensitivity also need further validation in in vivo experiments. In future studies, we will delve deeper into in vivo mechanistic research to elucidate the significant role of cuproptosis-SPGs in the progression of osteosarcoma.
5 Conclusion
In summary, by integrating multi-omics datasets and single-cell analysis methods, our study explored the interplay between cuproptosis and sphingolipid metabolism and identified seven cuproptosis-related sphingolipid metabolism genes (cuproptosis-SPGs), used to construct a prognostic model for osteosarcoma patients. Our results delineate the value of this model in predicting patient outcomes and treatment efficacy, elucidate the regulation of immune cell infiltration by cuproptosis-SPGs, and validate these effects through cellular experiments. This study provides a theoretical foundation for future osteosarcoma studies focusing on copper ion metabolism therapies, highlighting the interactions.
Data availability statement
The original contributions presented in the study are included in the article/Supplementary Material. Further inquiries can be directed to the corresponding authors.
Ethics statement
Ethical approval was not required for the studies on humans in accordance with the local legislation and institutional requirements because only commercially available established cell lines were used.
Author contributions
QL: Data curation, Formal analysis, Investigation, Software, Visualization, Writing – original draft. JF: Data curation, Formal analysis, Visualization, Writing – original draft. KL: Investigation, Resources, Writing – original draft. PL: Conceptualization, Funding acquisition, Supervision, Validation, Writing – review & editing. XW: Conceptualization, Investigation, Methodology, Project administration, Supervision, Validation, Writing – review & editing.
Funding
The author(s) declare financial support was received for the research, authorship, and/or publication of this article. This study was supported by the project of Shenzhen Nanshan District Science and Technology (NS2022017); the project of Huazhong University of Science and Technology Union Shenzhen Hospital (YN2021024); the project of Shenzhen Nanshan District Science and Technology (NS2024016); Medical Scientific Research Foundation of Guangdong Province (A2024380).
Acknowledgments
We acknowledge the contributions from the TARGET (https://ocg.cancer.gov/programs/target) and InnateDB (https://www.innatedb.com/). We also appreciate the contributors of all R packages used in this study.
Conflict of interest
The authors declare that the research was conducted in the absence of any commercial or financial relationships that could be construed as a potential conflict of interest.
Publisher’s note
All claims expressed in this article are solely those of the authors and do not necessarily represent those of their affiliated organizations, or those of the publisher, the editors and the reviewers. Any product that may be evaluated in this article, or claim that may be made by its manufacturer, is not guaranteed or endorsed by the publisher.
Supplementary material
The Supplementary Material for this article can be found online at: https://www.frontiersin.org/articles/10.3389/fimmu.2024.1424806/full#supplementary-material
References
1. Arnett AB, Heczey A. GD2-CAR CAR T cells in patients with osteosarcoma and neuroblastoma-it’s not only the T cells that matter. Cancer Cell. (2024) 42:8–10. doi: 10.1016/j.ccell.2023.11.012
2. Keegan THM, Abrahao R, Alvarez EM. Survival trends among adolescents and young adults diagnosed with cancer in the United States: comparisons with children and older adults. J Clin Oncol. (2024) 42:630–41. doi: 10.1200/JCO.23.01367
3. Flores-Toro JA, Jagu S, Armstrong GT, Arons DF, Aune GJ, Chanock SJ, et al. The childhood cancer data initiative: using the power of data to learn from and improve outcomes for every child and young adult with pediatric cancer. J Clin Oncol. (2023) 41:4045–53. doi: 10.1200/JCO.22.02208
4. Dyson KA, Stover BD, Grippin A, Mendez-Gomez HR, Lagmay J, Mitchell DA, et al. Emerging trends in immunotherapy for pediatric sarcomas. J Hematol Oncol. (2019) 12:78. doi: 10.1186/s13045-019-0756-z
5. Wu C, Tan J, Shen H, Deng C, Kleber C, Osterhoff G, et al. Exploring the relationship between metabolism and immune microenvironment in osteosarcoma based on metabolic pathways. J BioMed Sci. (2024) 31:4. doi: 10.1186/s12929-024-00999-7
6. Huang L, Sun F, Liu Z, Jin W, Zhang Y, Chen J, et al. Probing the potential of defense response-associated genes for predicting the progression, prognosis, and immune microenvironment of osteosarcoma. Cancers (Basel). (2023) 15:2405. doi: 10.3390/cancers15082405
7. Zhong Y, Zhang Y, Wei S, Chen J, Zhong C, Cai W, et al. Dissecting the effect of sphingolipid metabolism gene in progression and microenvironment of osteosarcoma to develop a prognostic signature. Front Endocrinol (Lausanne). (2022) 13:1030655. doi: 10.3389/fendo.2022.1030655
8. Zhang Y, Gong R, Liu Y, Sun X, Liang J, Zhou Y, et al. Ailanthone inhibits proliferation, migration and invasion of osteosarcoma cells by downregulating the serine biosynthetic pathway. Front Oncol. (2022) 12:842406. doi: 10.3389/fonc.2022.842406
9. Cortini M, Armirotti A, Columbaro M, Longo DL, Di Pompo G, Cannas E, et al. Exploring metabolic adaptations to the acidic microenvironment of osteosarcoma cells unveils sphingosine 1-Phosphate as a valuable therapeutic target. Cancers (Basel). (2021) 13:311. doi: 10.3390/cancers13020311
10. Yang H, Li Z, Zhu S, Wang W, Zhang J, Zhao D, et al. Molecular mechanisms of pancreatic cancer liver metastasis: the role of PAK2. Front Immunol. (2024) 15:1347683. doi: 10.3389/fimmu.2024.1347683
11. Ogretmen B. Sphingolipid metabolism in cancer signalling and therapy. Nat Rev Cancer. (2018) 18:33–50. doi: 10.1038/nrc.2017.96
12. Hannun YA, Obeid LM. Sphingolipids and their metabolism in physiology and disease. Nat Rev Mol Cell Biol. (2018) 19:175–91. doi: 10.1038/nrm.2017.107
13. Xiao J, Lin H, Liu B, Xia Z, Zhang J, Jin J. Decreased S1P and SPHK2 are involved in pancreatic acinar cell injury. biomark Med. (2019) 13:627–37. doi: 10.2217/bmm-2018-0404
14. Janneh AH, Atkinson C, Tomlinson S, Ogretmen B. Sphingolipid metabolism and complement signaling in cancer progression. Trends Cancer. (2023) 9:782–7. doi: 10.1016/j.trecan.2023.07.001
15. Gope A, Mauro C. When ceramides meet immune senescence, a GIMAP5 connection. Nat Immunol. (2024) 25:196–7. doi: 10.1038/s41590-023-01736-2
16. Luthers CR, Dunn TM, Snow AL. ORMDL3 and asthma: linking sphingolipid regulation to altered T cell function. Front Immunol. (2020) 11:597945. doi: 10.3389/fimmu.2020.597945
17. Hose M, Gunther A, Naser E, Schumacher F, Schonberger T, Falkenstein J, et al. Cell-intrinsic ceramides determine T cell function during melanoma progression. Elife. (2022) 11:e83073. doi: 10.7554/eLife.83073
18. Yi WJ, Yuan Y, Bao Q, Zhao Z, Ding HS, Song J. Analyzing immune cell infiltration and copper metabolism in diabetic foot ulcers. J Inflammation Res. (2024) 17:3143–57. doi: 10.2147/JIR.S452609
19. Zhao Y, Zhao B, Zhu S. Disulfiram/copper activates ER stress to promote immunogenic cell death of oral squamous cell carcinoma. Cell Biochem Biophys. (2024). doi: 10.1007/s12013-024-01283-z
20. Guo W, Jia L, Xie L, Kiang JG, Wang Y, Sun F, et al. Turning anecdotal irradiation-induced anticancer immune responses into reproducible in situ cancer vaccines via disulfiram/copper-mediated enhanced immunogenic cell death of breast cancer cells. Cell Death Dis. (2024) 15:298. doi: 10.1038/s41419-024-06644-3
21. Lu J, Liu X, Li X, Li H, Shi L, Xia X, et al. Copper regulates the host innate immune response against bacterial infection via activation of ALPK1 kinase. Proc Natl Acad Sci U.S.A. (2024) 121:e2311630121. doi: 10.1073/pnas.2311630121
22. Wei M, Lu L, Luo Z, Ma J, Wang J. Prognostic analysis of hepatocellular carcinoma based on cuproptosis -associated lncRNAs. BMC Gastroenterol. (2024) 24:142. doi: 10.1186/s12876-024-03219-6
23. Zhong Y, Zeng W, Chen Y, Zhu X. The effect of lipid metabolism on cuproptosis-inducing cancer therapy. BioMed Pharmacother. (2024) 172:116247. doi: 10.1016/j.biopha.2024.116247
24. Mo JQ, Zhang SY, Li Q, Chen MX, Zheng YQ, Xie X, et al. Immunomodulation of cuproptosis and ferroptosis in liver cancer. Cancer Cell Int. (2024) 24:22. doi: 10.1186/s12935-023-03207-y
25. Jawed R, Bhatti H. Cuproptosis in lung cancer: therapeutic options and prognostic models. Apoptosis. (2024). doi: 10.1007/s10495-024-01978-x
26. Gaetke LM, Chow-Johnson HS, Chow CK. Copper: toxicological relevance and mechanisms. Arch Toxicol. (2014) 88:1929–38. doi: 10.1007/s00204-014-1355-y
27. Rana SV. Metals and apoptosis: recent developments. J Trace Elem Med Biol. (2008) 22:262–84. doi: 10.1016/j.jtemb.2008.08.002
28. Zhu C, Sun Z, Wang J, Meng X, Ma Z, Guo R, et al. et al: Exploring oncogenes for renal clear cell carcinoma based on G protein-coupled receptor-associated genes. Discovery Oncol. (2023) 14:182. doi: 10.1007/s12672-023-00795-z
29. Wu Z, Zeng J, Wu M, Liang Q, Li B, Hou G, et al. Identification and validation of the pyroptosis-related long noncoding rna signature to predict the prognosis of patients with bladder cancer. Med (Baltimore). (2023) 102:e33075. doi: 10.1097/MD.0000000000033075
30. Li C, Liu T, Liu Y, Zhang J, Zuo D. Prognostic value of tumour microenvironment-related genes by TCGA database in rectal cancer. J Cell Mol Med. (2021) 25:5811–22. doi: 10.1111/jcmm.16547
31. Liu T, Li C, Zhang J, Hu H, Li C. Unveiling efferocytosis-related signatures through the integration of single-cell analysis and machine learning: a predictive framework for prognosis and immunotherapy response in hepatocellular carcinoma. Front Immunol. (2023) 14:1237350. doi: 10.3389/fimmu.2023.1237350
32. Guan H, Chen X, Liu J, Sun J, Guo H, Jiang Y, et al. Molecular characteristics and therapeutic implications of Toll-like receptor signaling pathway in melanoma. Sci Rep. (2023) 13:13788. doi: 10.1038/s41598-023-38850-y
33. Zhang S, Jiang C, Jiang L, Chen H, Huang J, Gao X, et al. Construction of a diagnostic model for hepatitis B-related hepatocellular carcinoma using machine learning and artificial neural networks and revealing the correlation by immunoassay. Tumour Virus Res. (2023) 16:200271. doi: 10.1016/j.tvr.2023.200271
34. Pan C, Deng D, Wei T, Wu Z, Zhang B, Yuan Q, et al. Metabolomics study identified bile acids as potential biomarkers for gastric cancer: A case control study. Front Endocrinol (Lausanne). (2022) 13:1039786. doi: 10.3389/fendo.2022.1039786
35. Zeng J, Wu Z, Luo M, Xu X, Bai W, Xie G, et al. Development and validation of an endoplasmic reticulum stress long non-coding RNA signature for the prognosis and immune landscape prediction of patients with lung adenocarcinoma. Front Genet. (2023) 14:1024444. doi: 10.3389/fgene.2023.1024444
36. Chi H, Gao X, Xia Z, Yu W, Yin X, Pan Y, et al. et al: FAM family gene prediction model reveals heterogeneity, stemness and immune microenvironment of UCEC. Front Mol Biosci. (2023) 10:1200335. doi: 10.3389/fmolb.2023.1200335
37. Li C, Wirth U, Schardey J, Ehrlich-Treuenstatt VV, Bazhin AV, Werner J, et al. An immune-related gene prognostic index for predicting prognosis in patients with colorectal cancer. Front Immunol. (2023) 14:1156488. doi: 10.3389/fimmu.2023.1156488
38. Wang J, Zuo Z, Yu Z, Chen Z, Meng X, Ma Z, et al. et al: Single-cell transcriptome analysis revealing the intratumoral heterogeneity of ccRCC and validation of MT2A in pathogenesis. Funct Integr Genomics. (2023) 23:300. doi: 10.1007/s10142-023-01225-7
39. Liu Y, Wu Z, Feng Y, Gao J, Wang B, Lian C, et al. Integration analysis of single-cell and spatial transcriptomics reveal the cellular heterogeneity landscape in glioblastoma and establish a polygenic risk model. Front Oncol. (2023) 13:1109037. doi: 10.3389/fonc.2023.1109037
40. Zhang B, Chen X, Wang Z, Guo F, Zhang X, Huang B, et al. Identifying endoplasmic reticulum stress-related molecular subtypes and prognostic model for predicting the immune landscape and therapy response in pancreatic cancer. Aging (Albany NY). (2023) 15:10549–79. doi: 10.18632/aging.v15i19
41. Chi H, Yang J, Peng G, Zhang J, Song G, Xie X, et al. Circadian rhythm-related genes index: A predictor for HNSCC prognosis, immunotherapy efficacy, and chemosensitivity. Front Immunol. (2023) 14:1091218. doi: 10.3389/fimmu.2023.1091218
42. Wang J, Zuo Z, Yu Z, Chen Z, Tran LJ, Zhang J, et al. Collaborating single-cell and bulk RNA sequencing for comprehensive characterization of the intratumor heterogeneity and prognostic model development for bladder cancer. Aging (Albany NY). (2023) 15:12104–19. doi: 10.18632/aging.v15i21
43. Zhang J, Xiao J, Wang Y, Zheng X, Cui J, Wang C. A universal co-expression gene network and prognostic model for hepatic-biliary-pancreatic cancers identified by integrative analyses. FEBS Open Bio. (2022) 12:2006–24. doi: 10.1002/2211-5463.13478
44. Ferri A, Bianchi B, Ferrari S. Mandibular osteosarcoma: diagnosis and treatment. Curr Opin Otolaryngol Head Neck Surg. (2022) 30:154–60. doi: 10.1097/MOO.0000000000000793
45. Meltzer PS, Helman LJ. New horizons in the treatment of osteosarcoma. N Engl J Med. (2021) 385:2066–76. doi: 10.1056/NEJMra2103423
46. Park JA, Cheung NV. Promise and challenges of T cell immunotherapy for osteosarcoma. Int J Mol Sci. (2023) 24:12520. doi: 10.3390/ijms241512520
47. Zhao X, Wu Q, Gong X, Liu J, Ma Y. Osteosarcoma: a review of current and future therapeutic approaches. BioMed Eng Online. (2021) 20:24. doi: 10.1186/s12938-021-00860-0
48. Wen Y, Tang F, Tu C, Hornicek F, Duan Z, Min L. Immune checkpoints in osteosarcoma: Recent advances and therapeutic potential. Cancer Lett. (2022) 547:215887. doi: 10.1016/j.canlet.2022.215887
49. Suehara Y, Alex D, Bowman A, Middha S, Zehir A, Chakravarty D, et al. Clinical genomic sequencing of pediatric and adult osteosarcoma reveals distinct molecular subsets with potentially targetable alterations. Clin Cancer Res. (2019) 25:6346–56. doi: 10.1158/1078-0432.CCR-18-4032
50. Wang J, Zhanghuang C, Tan X, Mi T, Liu J, Jin L, et al. A nomogram for predicting cancer-specific survival of osteosarcoma and ewing’s sarcoma in children: A SEER database analysis. Front Public Health. (2022) 10:837506. doi: 10.3389/fpubh.2022.837506
51. Sun Z, Wang J, Zhang Q, Meng X, Ma Z, Niu J, et al. Coordinating single-cell and bulk RNA-seq in deciphering the intratumoral immune landscape and prognostic stratification of prostate cancer patients. Environ Toxicol. (2024) 39:657–68. doi: 10.1002/tox.23928
52. Zhou X, Jing J, Peng J, Mao W, Zheng Y, Wang D, et al. Expression and clinical significance of galectin-3 in osteosarcoma. Gene. (2014) 546:403–7. doi: 10.1016/j.gene.2014.04.066
53. Fujiwara T, Katsuda T, Hagiwara K, Kosaka N, Yoshioka Y, Takahashi RU, et al. Clinical relevance and therapeutic significance of microRNA-133a expression profiles and functions in Malignant osteosarcoma-initiating cells. Stem Cells. (2014) 32:959–73. doi: 10.1002/stem.1618
54. Ma B, Qin L, Sun Z, Wang J, Tran LJ, Zhang J, et al. The single-cell evolution trajectory presented different hypoxia heterogeneity to reveal the carcinogenesis of genes in clear cell renal cell carcinoma: Based on multiple omics and real experimental verification. Environ Toxicol. (2024) 39:869–81. doi: 10.1002/tox.24009
55. Zhang H, Zhai X, Liu Y, Xia Z, Xia T, Du G, et al. NOP2-mediated m5C Modification of c-Myc in an EIF3A-Dependent Manner to Reprogram Glucose Metabolism and Promote Hepatocellular Carcinoma Progression. Res (Wash D C). (2023) 6:0184. doi: 10.34133/research.0184
56. Zhang P, Pei S, Wu L, Xia Z, Wang Q, Huang X, et al. Integrating multiple machine learning methods to construct glutamine metabolism-related signatures in lung adenocarcinoma. Front Endocrinol (Lausanne). (2023) 14:1196372. doi: 10.3389/fendo.2023.1196372
57. Rockfield S, Chhabra R, Robertson M, Rehman N, Bisht R, Nanjundan M. Links between iron and lipids: implications in some major human diseases. Pharm (Basel). (2018) 11:113. doi: 10.3390/ph11040113
58. Vu NT, Kim M, Stephenson DJ, MacKnight HP, Chalfant CE. Ceramide kinase inhibition drives ferroptosis and sensitivity to cisplatin in mutant KRAS lung cancer by dysregulating VDAC-mediated mitochondria function. Mol Cancer Res. (2022) 20:1429–42. doi: 10.1158/1541-7786.MCR-22-0085
59. Lepple-Wienhues A, Belka C, Laun T, Jekle A, Walter B, Wieland U, et al. Stimulation of CD95 (Fas) blocks T lymphocyte calcium channels through sphingomyelinase and sphingolipids. Proc Natl Acad Sci U.S.A. (1999) 96:13795–800. doi: 10.1073/pnas.96.24.13795
60. Kajiwara K, Yamada T, Bamba T, Fukusaki E, Imamoto F, Okada M, et al. c-Src-induced activation of ceramide metabolism impairs membrane microdomains and promotes Malignant progression by facilitating the translocation of c-Src to focal adhesions. Biochem J. (2014) 458:81–93. doi: 10.1042/BJ20130527
61. Ohkawa Y, Miyazaki S, Hamamura K, Kambe M, Miyata M, Tajima O, et al. Ganglioside GD3 enhances adhesion signals and augments Malignant properties of melanoma cells by recruiting integrins to glycolipid-enriched microdomains. J Biol Chem. (2010) 285:27213–23. doi: 10.1074/jbc.M109.087791
62. Wang X, Zhou T, Yang X, Cao X, Jin G, Zhang P, et al. DDRGK1 enhances osteosarcoma chemoresistance via inhibiting KEAP1-mediated NRF2 ubiquitination. Adv Sci (Weinh). (2023) 10:e2204438. doi: 10.1002/advs.202204438
63. Chen Y, Li Y, Li C, Zhang D, Liu Y, Zhang J, et al. The current perspective and opportunities of small nucleic acid-based therapeutics. Drug Dev Res. (2024) 85:e22164. doi: 10.1002/ddr.22164
64. Jiang S, Yang X, Lin Y, Liu Y, Tran LJ, Zhang J, et al. Unveiling Anoikis-related genes: A breakthrough in the prognosis of bladder cancer. J Gene Med. (2024) 26:e3651. doi: 10.1002/jgm.3651
65. Sun Z, Wang J, Fan Z, Yang Y, Meng X, Ma Z, et al. et al: Investigating the prognostic role of lncRNAs associated with disulfidptosis-related genes in clear cell renal cell carcinoma. J Gene Med. (2024) 26:e3608. doi: 10.1002/jgm.3608
66. Ling B, Wei P, Xiao J, Cen B, Wei H, Feng X, et al. Nucleolar and spindle−associated protein 1 promotes non−small cell lung cancer progression and serves as an effector of myocyte enhancer factor 2D. Oncol Rep. (2021) 45:1044–58. doi: 10.3892/or.2020.7918
67. Chi H, Jiang P, Xu K, Zhao Y, Song B, Peng G, et al. A novel anoikis-related gene signature predicts prognosis in patients with head and neck squamous cell carcinoma and reveals immune infiltration. Front Genet. (2022) 13:984273. doi: 10.3389/fgene.2022.984273
68. Ren Q, Zhang P, Lin H, Feng Y, Chi H, Zhang X, et al. A novel signature predicts prognosis and immunotherapy in lung adenocarcinoma based on cancer-associated fibroblasts. Front Immunol. (2023) 14:1201573. doi: 10.3389/fimmu.2023.1201573
69. Cillo AR, Mukherjee E, Bailey NG, Onkar S, Daley J, Salgado C, et al. Ewing sarcoma and osteosarcoma have distinct immune signatures and intercellular communication networks. Clin Cancer Res. (2022) 28:4968–82. doi: 10.1158/1078-0432.CCR-22-1471
70. Jiang Y, Wang J, Sun M, Zuo D, Wang H, Shen J, et al. Multi-omics analysis identifies osteosarcoma subtypes with distinct prognosis indicating stratified treatment. Nat Commun. (2022) 13:7207. doi: 10.1038/s41467-022-34689-5
71. Zhang B, Liu J, Li H, Huang B, Zhang B, Song B, et al. Integrated multi-omics identified the novel intratumor microbiome-derived subtypes and signature to predict the outcome, tumor microenvironment heterogeneity, and immunotherapy response for pancreatic cancer patients. Front Pharmacol. (2023) 14:1244752. doi: 10.3389/fphar.2023.1244752
72. Wang J, Zheng T, Liao Y, Geng S, Li J, Zhang Z, et al. Machine learning prediction model for post- hepatectomy liver failure in hepatocellular carcinoma: A multicenter study. Front Oncol. (2022) 12:986867. doi: 10.3389/fonc.2022.986867
73. Wang S, Li H, Chen T, Zhou H, Zhang W, Lin N, et al. Human gammadelta T cells induce CD8(+) T cell antitumor responses via antigen-presenting effect through HSP90-MyD88-mediated activation of JNK. Cancer Immunol Immunother. (2023) 72:1803–21. doi: 10.1007/s00262-023-03375-w
Keywords: cuproptosis, sphingolipid, multi-omics, immune, metabolism, osteosarcoma
Citation: Li Q, Fang J, Liu K, Luo P and Wang X (2024) Multi-omic validation of the cuproptosis-sphingolipid metabolism network: modulating the immune landscape in osteosarcoma. Front. Immunol. 15:1424806. doi: 10.3389/fimmu.2024.1424806
Received: 28 April 2024; Accepted: 06 June 2024;
Published: 25 June 2024.
Edited by:
Gang Tian, Sichuan Province Engineering Technology Research Center of Molecular Diagnosis of Clinical Diseases, ChinaReviewed by:
Chao Li, LMU Munich University Hospital, GermanyJing Zhang, University of South Dakota, United States
Copyright © 2024 Li, Fang, Liu, Luo and Wang. This is an open-access article distributed under the terms of the Creative Commons Attribution License (CC BY). The use, distribution or reproduction in other forums is permitted, provided the original author(s) and the copyright owner(s) are credited and that the original publication in this journal is cited, in accordance with accepted academic practice. No use, distribution or reproduction is permitted which does not comply with these terms.
*Correspondence: Xiuzhuo Wang, wxz0390@163.com; Peng Luo, gaidihu0308@sina.com
†These authors have contributed equally to this work