- 1Department of Thoracic Medical Oncology, Fudan University Shanghai Cancer Center, and Shanghai Key Laboratory of Medical Epigenetics, International Co-laboratory of Medical Epigenetics and Metabolism, Institutes of Biomedical Sciences, Shanghai Medical College, Fudan University, Shanghai, China
- 2Department of Oncology, Shanghai Medical College, Fudan University, Shanghai, China
- 3Institute of Thoracic Oncology, Fudan University Shanghai Cancer Center, Shanghai, China
Introduction: The introduction of Immune Checkpoint Inhibitors (ICIs) has marked a paradigm shift in treating Lung Squamous Cell Carcinoma (LUSC), emphasizing the urgent need for precise molecular biomarkers to reliably forecast therapeutic efficacy. This study aims to identify potential biomarkers for immunochemotherapy efficacy by focusing on plasma extracellular vesicle (EV)-derived long RNAs (exLRs).
Methods: We enrolled 78 advanced LUSC patients undergoing first-line immunochemotherapy. Plasma samples were collected, and exLR sequencing was conducted to establish baseline profiles. A retrospective analysis was performed on 42 patients to identify differentially expressed exLRs. Further validation of the top differentially expressed exLRs was conducted using quantitative reverse transcription PCR (qRT-PCR). Univariate Cox analysis was applied to determine the prognostic significance of these exLRs. Based on these findings, we developed a predictive signature (p-Signature).
Results: In the retrospective analysis of 42 patients, we identified 460 differentially expressed exLRs, with pathways related to leukocyte migration notably enriched among non-responders. Univariate Cox analysis revealed 45 exLRs with prognostic significance. The top 6 protein-coding exLRs were validated using qRT-PCR, identifying CXCL8, SSH3, and SDHAF1 as differentially expressed between responders and non-responders. The p-Signature, comprising these three exLRs, demonstrated high accuracy in distinguishing responders from non-responders, with an Area Under the Curve (AUC) of 0.904 in the retrospective cohort and 0.812 in the prospective cohort.
Discussion: This study highlighted the potential of plasma exLR profiles in predicting LUSC treatment efficacy. Intriguingly, lower p-Signature scores were associated with increased abundance of activated CD4+ and CD8+ T cells, indicating a more robust immune environment. These findings suggest that the p-Signature could serve as a valuable tool in guiding personalized and effective therapeutic strategies for LUSC.
1 Introduction
Lung cancer is acknowledged as the leading cause of cancer-related deaths worldwide, consistently ranking as the most prevalent cancer (1). Within the spectrum of non-small cell lung cancer (NSCLC), lung squamous cell carcinoma (LUSC) represents approximately 20% to 30% of cases, making it the second most common subtype of NSCLC (2). The therapeutic options for advanced and metastatic LUSC have traditionally been constrained to chemotherapy, largely due to the lack of actionable genetic aberrations. The recent advent of immune checkpoint inhibitors (ICI), particularly those targeting the programmed cell death 1 (PD-1) pathway, has revolutionized LUSC treatment (3, 4). PD-1 inhibitors have shown notable clinical success, both as monotherapy in patients with a PD-1 tumor proportion score (TPS) ≥50% and as part of combination therapies with chemotherapy, irrespective of PD-1 expression levels (5–7).
The introduction of immunotherapy has opened new avenues of hope for lung cancer patients, yet identifying those who will most benefit remains a significant challenge. Currently, only about 20% of patients with advanced-stage NSCLC derive considerable benefits from immunotherapy (7). Biomarkers such as PD-L1 expression, tumor mutation burden (TMB), and microsatellite instability (MSI) or mismatch repair deficiency (dMMR) are associated with clinical efficacy (8–11). However, these markers are predominantly validated in the context of ICI monotherapy, and their predictive value in the setting of immunochemotherapy, especially when PD-L1 expression and TMB levels vary, is less clear (6). Furthermore, the utility of MSI/dMMR in lung cancer is somewhat limited due to its rare occurrence, less than 1% (12). Therefore, further research and exploration of better biomarkers are imperative to tailor immunochemotherapy.
Extracellular vesicles (EVs), secreted by a variety of cell types, contain a diverse array of proteins, nucleic acids, and lipids. These components provide insights into the physiological and pathological states of the source cells (13, 14). EV-based liquid biopsy has recently been recognized as a promising method for tumor profiling, prognostication, monitoring treatment efficacy, and identifying therapeutic targets (15, 16). Significantly, studies have underscored the role of EV-derived miRNA and PD-L1 in predicting the effectiveness of immunotherapy in advanced NSCLC (17, 18). In our previous work, we have demonstrated that EV-derived long RNA (exLRs), particularly CD160, shows potential as a prognostic biomarker for lung adenocarcinoma (LUAD) (19). However, the role of exLRs in the context of immunochemotherapy for LUSC has not been thoroughly investigated.
In this study, we employed an optimized exLR sequencing technique previously developed by our team (20) to conduct a comprehensive profiling of plasma exLRs in 78 advanced LUSC patients receiving first-line immunochemotherapy. Our analysis led to the identification of a LUSC-specific predictive signature, comprising three distinct exLRs. This signature has shown significant potential in accurately predicting the efficacy of immunochemotherapy in LUSC patients, marking a notable advancement in personalized cancer treatment.
2 Material and methods
2.1 Participants and blood samples
A total of 78 patients with locally advanced or metastatic LUSC and 51 healthy individuals were enrolled in this study at Fudan University Shanghai Cancer Center (FUSCC) between January 2020 and October 2022. Patient inclusion criteria include histologically confirmed diagnosis of inoperable stage III to IV LUSC, absence of targetable EGFR/ALK/ROS1 mutations, treatment-naïve status, and a baseline Eastern Cooperative Oncology Group (ECOG) performance status score of 0 or 1. The clinical stage was determined according to the eighth edition of the American Joint Committee on Cancer (AJCC) TNM staging system (21). Tumor PD-L1 expression levels of 47 LUSC patients were measured using the Dako IHC 22C3 pharmDx assay. All patients received ICIs (pembrolizumab or tislelizumab) in combination with chemotherapy (paclitaxel and carboplatin) every three weeks for four cycles, followed by ICIs maintenance every three weeks until disease progression or unacceptable toxicity.
2.2 Efficacy assessment
The tumor response was assessed every two treatment cycles using the Response Evaluation Criteria in Solid Tumors (RECIST, version 1.1) (22). Patients without disease progression or death by the cutoff date, or those who were lost to follow-up before progression or death at their last contact were censored. We utilized progression-free-survival (PFS), overall survival (OS), objective response rate (ORR) to access immunochemotherapy efficacy. PFS was defined as the time from the start of immunochemotherapy until either disease progression or death from any cause. OS was defined as the time from the initiation of immunochemotherapy until death from any cause. The ORR was calculated as the proportion of patients who achieve a complete response (CR) or partial response (PR) as the best overall response. Responders were defined as patient who achieved CR or PR as the best overall response during immunochemotherapy with PFS≥6 months. Non-responders were defined as patients who achieved stable disease (SD) or progressed disease (PD) as the best overall response, or patients with PFS<6 months.
2.3 Isolation of EVs and EV-derived long RNA
The EVs were purified from a 1 mL plasma sample using the exoRNeasy Serum/Plasma Kit (Qiagen, Cat. No. 77144, Hilden, Germany). Briefly, thawed plasma was mixed with binding buffer and added to the exoEasy membrane affinity spin column to bind the vesicles to the membrane. For transmission electron microscopy (TEM), size distribution measurement, and Western blotting, the EVs were eluted with 400 μL of XE elution buffer (Qiagen, Cat. No. 76214). Ultrafiltration was performed at 12,000 rpm for 17 minutes to reduce the eluate volume to 50 µL, followed by buffer exchange with phosphate-buffered saline (PBS) using an Amicon Ultra-0.5 Centrifugal Filter with a 10 kDa molecular weight cutoff (Merck Millipore, Germany). For EV RNA isolation, the EVs were lysed on the column using QIAzol (Qiagen), and total RNA was subsequently eluted with 18 µL of RNase-free water. See the Supplementary Methods for details.
2.4 RNA-seq analysis
Extracellular vesicle-derived long RNA isolated from 1mL of plasma was treated with DNase I (NEB, Ipswich, Massachusetts, USA) to remove DNA. Strand-specific RNA-seq libraries were prepared using the SMARTer Stranded Total RNA-seq Kit (Clontech, Palo Alto, California, USA). Library quality was analyzed using a Qubit fluorometer (Thermo Fisher Scientific, Waltham, Massachusetts, USA) and Qsep100 (BiOptic, New Taipei City, Taiwan). ExLR-seq was performed on an Illumina sequencing platform (San Diego, California, USA) with 150 bp paired-end run metrics. The raw sequencing reads were filtered by FastQC (version 0.11.8) and aligned to the Gencode human genome (GRCh38) using the read aligner STAR (version 2.7.1a). Gene expression levels were then quantified by featureCounts (Version 1.6.3) and transformed into transcripts per kilobase million (TPM). Annotation information of mRNA, lncRNA and pseudogene genes were retrieved from the GENCODE database (Human, version 29).
2.5 RT-qPCR analysis
Total EV RNA isolated from 1mL of plasma were treated with DNase I (NEB, Ipswich, Massachusetts, USA) to remove DNA. Then the cDNA was synthesized using Evo M-MLV RT Master Mix (Accurate Biology, Hunan, China). Gene expression levels were measured by qRT-PCR using SYBR Premix Ex Taq (Accurate Biology, Hunan, China). The relative expression levels of exLRs were calculated using the comparative Ct method normalized to Actin. The primers for Quantitative PCR (qPCR) are listed in Supplementary Table 1.
2.6 Data and statistical analyses
The raw read counts from RNA-seq were transformed into TPM values to standardize the expression levels of exLR genes across samples. Genes with low overall frequencies (expressed in less than 30% of all analyzed samples) were removed, and the remaining exLRs were utilized for subsequent analysis. To assess the differences in exLRs expression between responders and non-responders, the limma R package was employed, utilizing fold change (FC) and comparing them with the Mann-Whitney U-test. ExLRs with FC >1.5 and false discovery rate (FDR) <0.05 were considered as statistically significant differentially expressed genes (DEGs). Gene Ontology (GO) biological process (BP) enrichment analysis and Kyoto Encyclopedia of Genes and Genomes (KEGG) pathway analysis were conducted on the DEGs to determine the pathway enrichment and significant molecular mechanisms of the different groups. The FDR was controlled using Benjamini-Hochberg adjustment.
To identify the exLRs for predicting the efficacy of LUSC immunochemotherapy, we employed a multistep approach. First, we identified the exLRs that were differentially expressed between the responders and the non-responders, considering a FDR <0.05 and FC >1.5. Next, we analyzed the exLRs associated with PFS using a Cox regression model, considering a significance level of p < 0.05. The genes that showed a significant correlation with PFS were considered as survival-related genes. Subsequently, six significantly differentially expressed (FC >2) protein coding exLRs were selected to detected by real-time quantitative PCR (qRT-PCR) in both retrospective cohort and prospective cohort. Finally, three differential expression exLRs were used for the construction of a least absolute shrinkage and selection operator (LASSO)-COX regression model for efficacy prediction. We utilized the R software package glmnet to integrate progression-free survival time, survival status, and gene expression data, and performed regression analysis using the LASSO-COX method. Furthermore, we used fivefold cross-validation and the Akaike information criterion (AIC) to estimate the expected generalization error and the select optimal value of the ‘1-se’ lambda parameter to obtain the optimal model.
3 Results
3.1 Patient characteristics
In this study, a total of 78 patients diagnosed with LUSC and 51 healthy individuals were included (Supplementary Table 2). Within the LUSC patient group (Table 1), males were predominantly represented, with a significant proportion being current or former smokers. The majority of patients in both the retrospective (N=42) and prospective (N=36) cohorts were diagnosed with stage IV cancer, exhibiting common metastatic sites primarily in the chest region. The PD-L1 status was unknown for 28.58% of the retrospective cohort and 74.99% of the prospective cohorts. In the retrospective cohort 37 (88.10%) patients received pembrolizumab, while 5 (11.90%) patients received tislelizumab. In the prospective cohort 13 (36.11%) patients received pembrolizumab, and the remaining 23 (63.89%) received tislelizumab. There were no significant differences between the two cohorts regarding age, gender, stage, metastatic sites, and response to immunochemotherapy. As of the data cutoff in June 2023, the median follow-up duration was 26.5 months for the retrospective cohort and 11 months for the prospective cohort. The ORR was 66.67% in both cohorts. Specifically, 26 patients (61.90%) in the retrospective cohort and 22 patients (61.11%) in the prospective cohort were classified as responders to immunochemotherapy.
3.2 EV isolation and exLR-seq
The baseline plasma EVs were isolated from 78 LUSC patients and 51 healthy donors, and the exLR-seq technique was applied to each plasma sample (Figure 1A). Under transmission electron microscopy, the isolated vesicles exhibited a rounded, cup-shaped morphology with a membrane enclosure (Figure 1B). Through flow cytometry analysis, a diverse population of spherical nanoparticles was identified (Figure 1C). Western blot analysis displayed enriched expression of EV markers, TSG101 and CD63, within the isolated vesicles, contrasting with PBMCs (Figure 1D). These findings suggest that the isolated EVs may predominantly consist of exosomes.
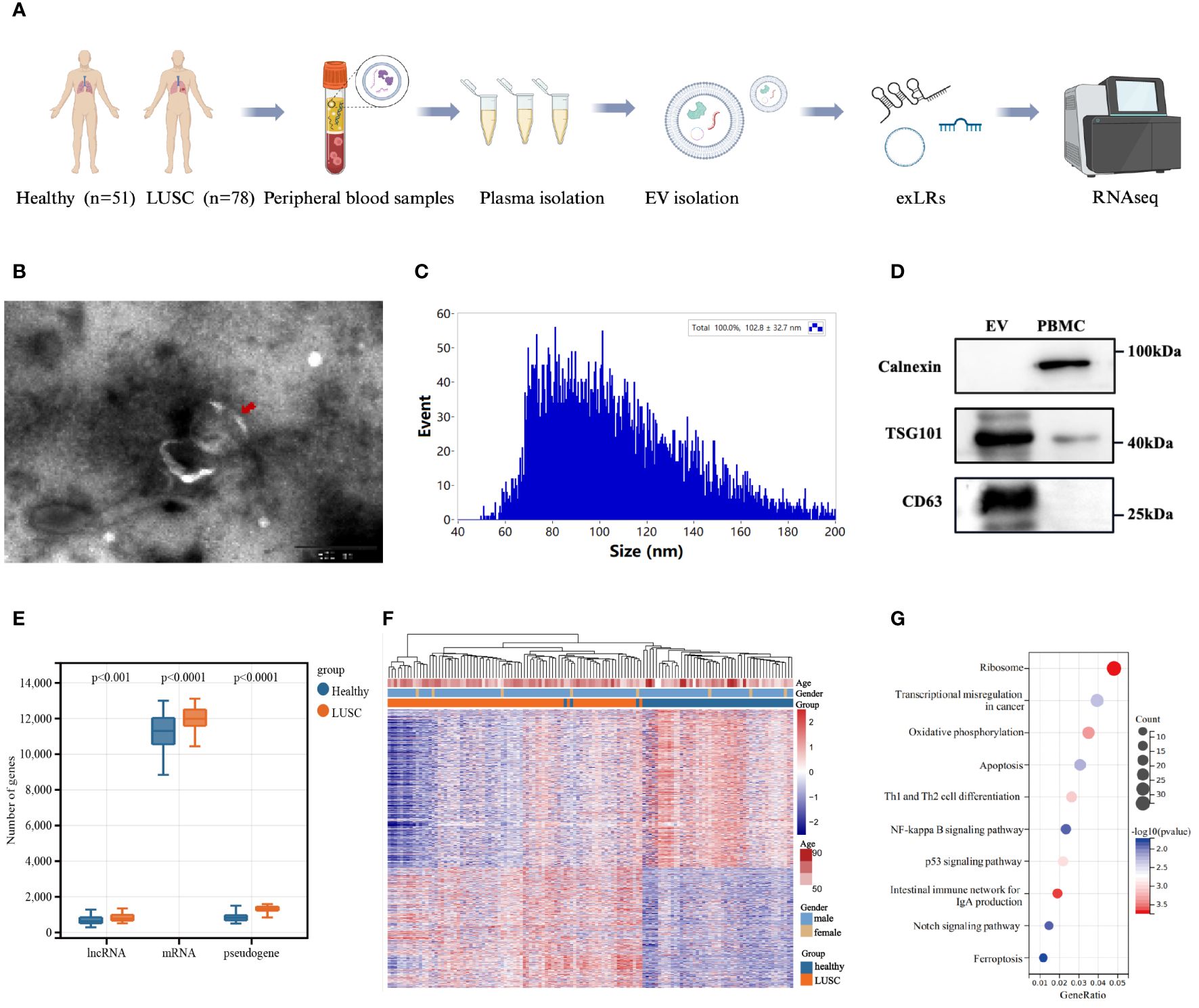
Figure 1 Extracellular vesicle-derived long RNA (exLR) profiles in lung squamous carcinoma (LUSC) patients and healthy individuals. (A) Workflow of extracellular vesicle (EV) extraction and exLR-seq. (B) Transmission electron microscopy image of isolated vesicles. (C) Particle size analysis of isolated vesicles. (D) Western blots of EV markers TSG101 and CD63 expression in isolated vesicles and peripheral blood mononuclear cell (PBMC). (E) Distribution of different exLRs per sample between LUSC patients and healthy individuals. (F) Heatmap of unsupervised hierarchical clustering of the differentially expressed exLRs between LUSC patients and healthy controls. Differential expression was defined by fold change >1.5 and adjusted p < 0.05. (G) KEGG pathway enrichment analysis for the differentially expressed exLRs.
Roughly 15,000 annotated genes, encompassing mRNAs, lncRNAs and pseudogenes, were consistently identified in both plasma samples from healthy donors and LUSC patients. Within the detected exLRs, a majority constituted mRNAs. Notably, the quantities of enriched mRNAs, lncRNAs, and pseudogenes were higher in LUSC patients than in healthy donors (Figure 1E). We identified 1,134 upregulated and 1,486 downregulated exLRs in LUSC patients compared with healthy donors (FDR <0.05, FC >1.5). Unsupervised hierarchical clustering revealed a clear separation of LUSC and healthy samples (Figure 1F). Furthermore, the KEGG pathway analysis illuminated the significance of differentially expressed exLRs enriched in cancer-related pathway, such as transcriptional misregulation in cancer, the NF-kappa B signaling pathway, and the p53 signaling pathway (Figure 1G).
3.3 Analysis of baseline exLRs among immunochemotherapy responders and non-responders within the retrospective LUSC patient cohort
Firstly, we conducted a comparative analysis of baseline exLR expression profiles between immunochemotherapy responders (n=26) and non-responders (n=16) within the retrospective LUSC patient cohort to identify exLR-based biomarkers associated with treatment outcome. A total of 460 exLRs exhibited statistical significance (P<0.05, FC>1.5) (Figure 2A). Notably, unsupervised hierarchical clustering unveiled a distinct separation between responders and non-responders, underscoring the potential of exLRs as prognostic biomarkers (Figure 2B). Moreover, GO-BP enrichment analysis indicated the DEGs were significantly enriched in inflammation-related pathways, especially those linked to leukocyte migration and chemotaxis (Figure 2C). These findings suggested a pivotal role of distinct inflammatory statuses in shaping responses to immunochemotherapy among LUSC patients. To further elucidate prognostic-associated exLRs, we performed univariant Cox regression analysis to explore the correlation between baseline exLR expression levels and patients’ PFS data. Through this analysis, we successfully identified 45 exLRs with prognostic significance (Supplementary Figure S1A). Considering that tumor PD-L1 expression level, peripheral platelet to lymphocyte ratio (PLR), lymphocyte to monocyte ratio (LMR), neutrophil to lymphocyte ratio (NLR) and serum LDH level have been reported to correlate with ICI therapy efficacy (23–25), we proceeded to verify PD-L1 status in 30 LUSC patients, along with baseline PLR, LMR, NLR and serum LDH level in the entire cohort of 42 LUSC patients. Our results demonstrated that PD-L1 status, PLR, LMR, NLR and serum LDH level did not significantly differ between responders and non-responders (Figure 2D, Supplementary Figures S1B, C).
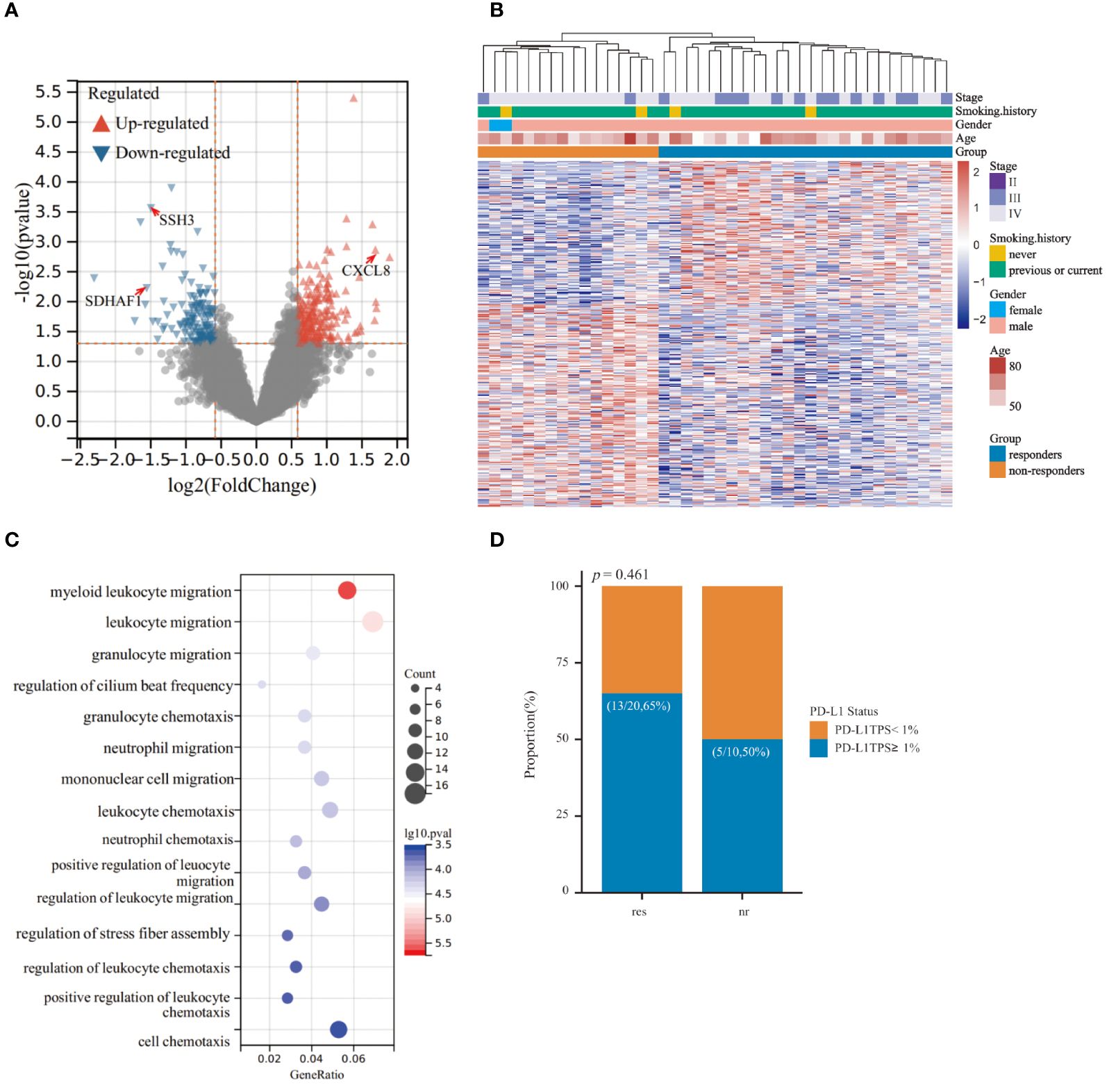
Figure 2 Comparison of responders and non-responders in the retrospective cohort of lung squamous cell carcinoma (LUSC) patients. (A) Volcano plot showing the 360 differential expressed EV-derived long RNAs (exLRs) between responders and non-responders within the retrospective cohort. Differential expression was defined by fold change >1.5 and adjusted p < 0.05. (B) Heatmap of unsupervised hierarchical clustering of the differentially expressed exLRs. (C) Top 15 significantly enriched GO-BP pathways analyzed from differentially expressed exLRs. (D) Histogram illustrating the distribution of PD-L1 status between responders and non-responders.
3.4 Establishment of exLRs-based predictive signature for immunochemotherapy outcomes within LUSC patients
The workflow for identifying a predictive signature for immunochemotherapy outcomes in LUSC patients was depicted in Figure 3A. Initially, we selected 6 significantly differentially expressed (FC>2) protein coding exLRs (CXCL8, CXCL10, RNF25, ESM1, SSH3, SDHAF1) from 45 prognostic-related exLRs as candidate biomarkers. Subsequently, we performed ROC analyses to evaluate their predictive potential. SSH3 exhibited the highest areas under the curve (AUC) of 0.806, while the other exLRs achieved AUC values ranging from 0.661 to 0.752 (Supplementary Figure S2A). To validate the predictive value of these exLRs in the retrospective cohort, we further enrolled 36 LUSC patients including 22 responders and 14 non-responders. The expression levels of these 6 candidate biomarkers were detected by qRT-PCR. The results indicated that the expression of 3 candidate exLRs (CXCL8, SDHAF1, SSH3) displayed significant differences and consistent expression trends with RNA-seq data between responders and non-responders (Figures 3B–D, Supplementary Figures S2B–D).
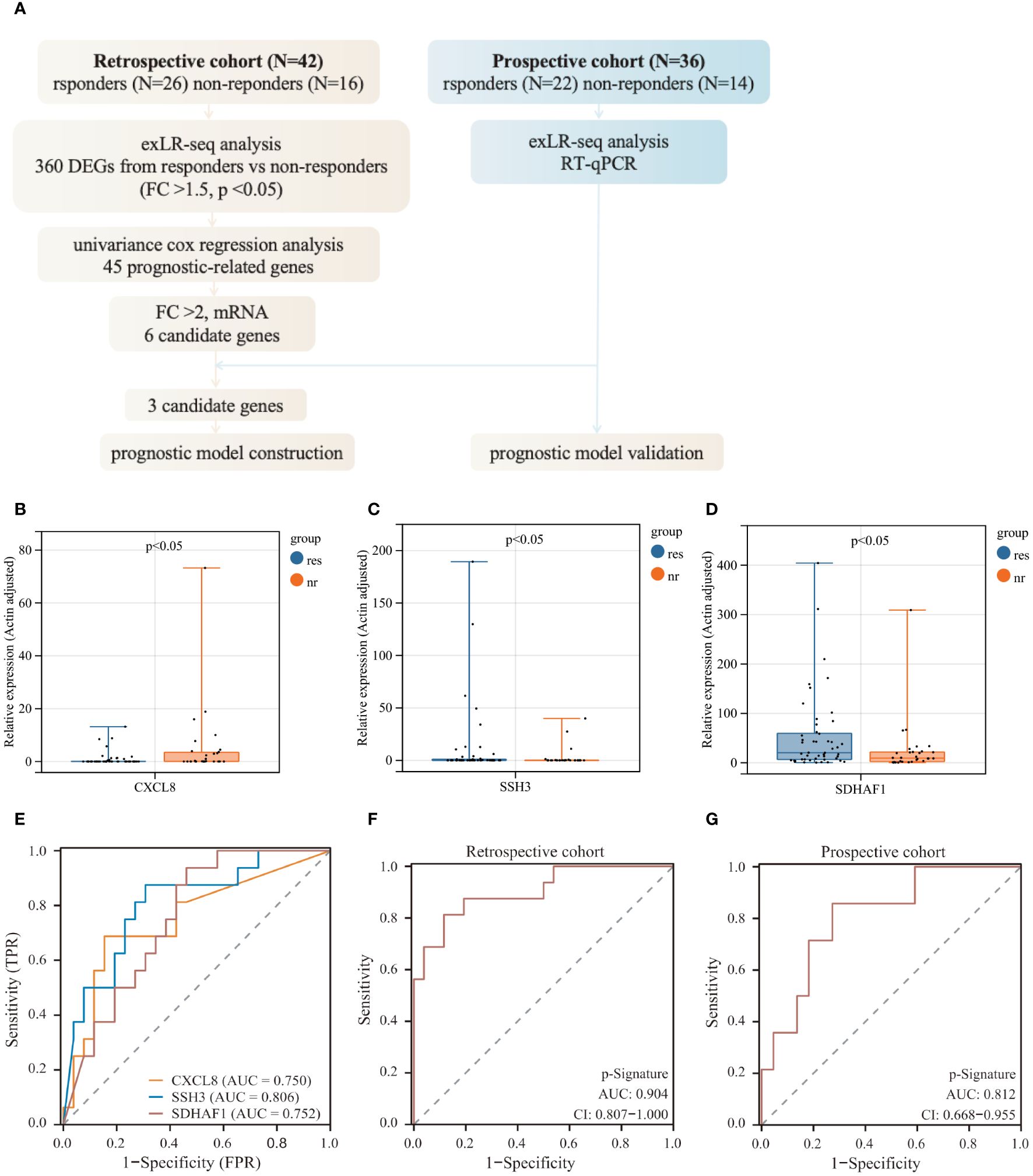
Figure 3 Predictive model construction for immunochemotherapy efficacy. (A) Workflow of prognostic model construction. (B–D) Relative RNA expression levels of 3 exLRs that exhibit differential expression and consistent expression trends between responders and non-responders. (E) ROC analysis of 3 candidate exLRs. (F, G) ROC analysis of the p-signature in the retrospective cohort (F) and the prospective cohort (G).
In an effort to develop a more effective predictive model based on exLRs, we tried to construct a multi-biomarker predictive model based on the three identified exLRs. Employing the LASSO-COX regression model, we established a prognostic model and generated an exLR based p-signature for LUSC patients who received immunochemotherapy (Supplementary Figures S2E, F). The exLR based p-Signature, comprising the three exLRs, successfully distinguished the responders from non-responders with an impressive AUC of 0.904 (95% confidence interval (CI): 0.807-1.000) in the retrospective cohort, demonstrating significantly higher accuracy than the exLR alone (Figures 3E, F). Subsequently, we applied the p-signature to the prospective cohort, where it detected responders with an AUC of 0.812 (95% CI: 0.668-0.955) (Figure 3G).
3.5 Performance of the exLR-derived p-signature in predicting immunochemotherapy outcomes in LUSC patients
Higher levels of p-Signature were observed in non-responders than those in responders (p<0.0001) (Figure 4A). The p-Signature aligns consistently with the best efficacy status assessed by imaging, with highest p-Signature levels in the PD groups and lowest levels in the PR group (Figure 4B). Furthermore, we conducted tumor PD-L1 assessment in 47 patients. The ROC analysis demonstrated that the AUC of tissue PD-L1 levels in distinguishing responders from non-responders is 0.577 (95% CI: 0.409-0.744) (Figure 4C). However, this accuracy is notably lower than using the p-Signature alone (AUC: 0.855, 95% CI: 0.733-0.977) (Figure 4D). When combining the PD-L1 status with the p-Signature, the AUC was 0.861 (95% CI: 0.737-0.985) (Figure 4E), which was similar to the AUC achieved with the p-Signature alone. This finding suggested that tumor PD-L1 level may not be an optimal predictive biomarker for immunochemotherapy. Then, we divided the patients into high and low-score group based on the median p-Signature value. Patients in the high-score group experienced significantly poorer PFS (p<0.001) (Figure 4F) and OS (p=0.025) (Figure 4G) than those in the low-score group, median progression-free survival (mPFS) was 868 days for low-score group vs. 372 days for high-score group, median overall survival (mOS) was 878 days for low-score group vs. 579 days for high-score group. Univariate analysis demonstrated that PD-L1 status and p-Signature were significantly associated with shorter PFS and OS(p<0.1), with the p-Signature acting as a promising predictor independent of other clinicopathological variables (Table 2). Collectively, our results suggested that the EV based p-Signature serves as valuable predictive factor, enhancing the predictive performance for clinical outcomes in LUSC patients receiving immunochemotherapy.
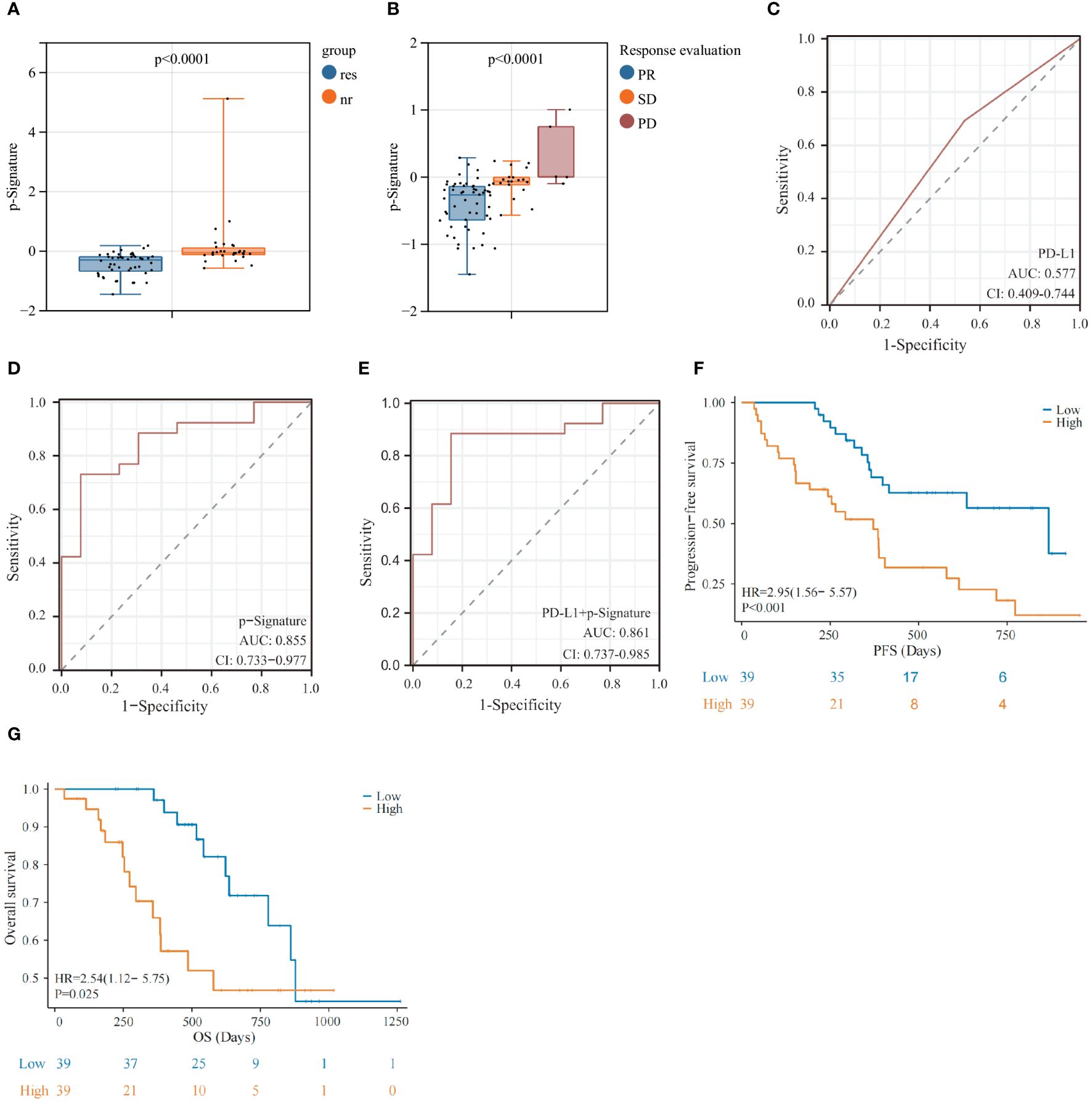
Figure 4 Prognostic predictive performance of the exLR-based p-Signature. (A) p-Signature between responders and non-responders in LUSC patients. (B) The patients with different best immunochemotherapy treatment response (partial response [PR], stable disease [SD] and progressive disease [PD]) demonstrated significant differences in p-Signature levels. (C–E) The ROC curve of PD-L1 (C), p-Signature (D) and combined PD-L1 levels and p-Signature (E) in patients who received tissue PD-L1 detection. (F, G) Kaplan-Meier survival analysis (log-rank test) of progression-free survival (PFS) (F) and overall survival (OS) (G) among LUSC patients with different p-Signature levels.
3.6 Potential mechanisms of p-signature in predicting LUSC patient’s prognosis
To gain a deeper insight into the mechanism of the p-Signature in efficacy prediction, we utilized single sample gene set enrichment analysis (ssGSEA) to investigate the relative abundance of immune cell types in LUSC patients. The results revealed that the low-score group exhibited higher enrichment scores (ES) in activated CD4+ (Figure 5A) and CD8+ T cells (Figure 5B). To further explore the specificity of the p-Signature to LUSC patients, we applied the p-Signature to 74 LUAD patients. However, the p-Signature showed no difference between responders and non-responders in the LUAD patients (Figure 5C). The ROC analysis demonstrated that the AUC of the p-Signature in distinguishing responders from non-responders in LUAD is 0.610 (95% CI: 0.480-0.740) (Figure 5D). We also performed ssGSEA analysis in LUAD patients, and unsupervised hierarchical clustering unveiled a distinct separation of immune cell types between LUSC and LUAD patients (Figure 5E).
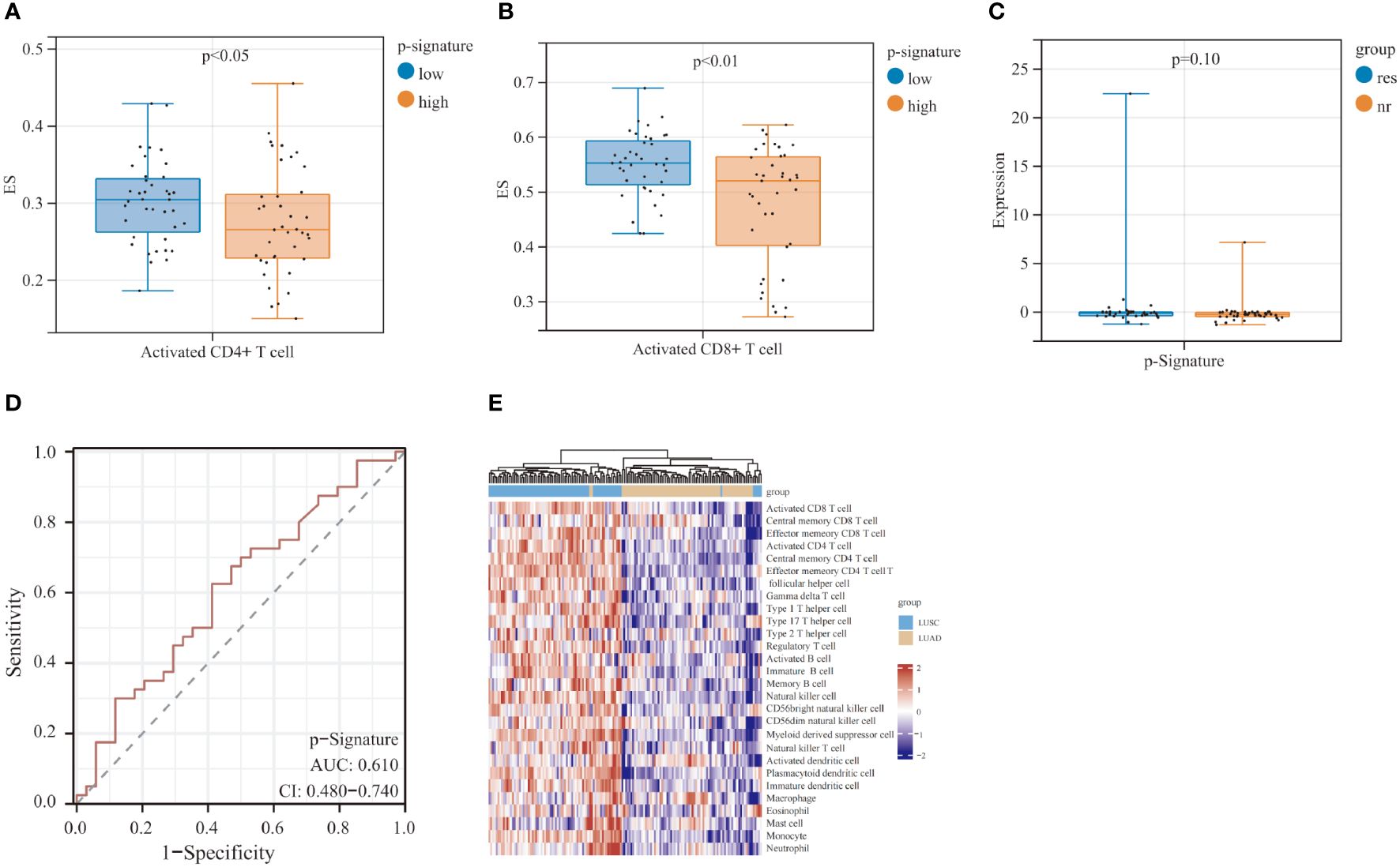
Figure 5 Prognostic predictive performance of p-Signature in the lung adenocarcinoma (LUAD) patients and the potential mechanism of p-Signature in predicting LUSC patient prognosis. (A, B) Activated CD4+ T cell (A) and activated CD8+ T cell (B) show significant difference between high and low p-Signature groups. (C) p-Signature between responders and non-responders in LUAD patients. (D) The ROC curve of p-Signature in responders and non-responders within the LUAD patients. (E) Heatmap of hierarchical clustering of cell types differentially observed in LUSC and LUAD patients.
3.7 High level EV-derived CXCL8 correlates with reduced clinical benefit of immunochemotherapy
EV-derived CXCL8 exhibited significant differences between responders and non-responders in patients with LUSC (Figure 6A). Moreover, we observed a notable extension in PFS (p=0.018) and OS (p=0.019) in the lower level EV-derived CXCL8 group (Figures 6B, C), mPFS was 773 days for lower level EV-derived CXCL8 group vs. 385 days for higher level group, mOS was 636 days for higher level EV-derived CXCL8 group, whereas lower level EV-derived CXCL8 group has not reached its mOS. Additionally, tissue PD-L1 TPS<1% group had a high level of EV-derived CXCL8 (Figure 6D). To further understand the role of CXCL8, we explored tissue-derived CXCL8 expression in the Cancer Genome Atlas (TCGA) - LUSC database. There was no significant difference between LUSC and noncancerous tissues in CXCL8 expression, and no survival benefit was observed (Supplementary Figures S3A, B). Furthermore, using ssGSEA, we discovered that the level of EV-derived CXCL8 has a positive correlation with myeloid-derived suppressor cells (MDSC) and neutrophils (Figures 6E, F).
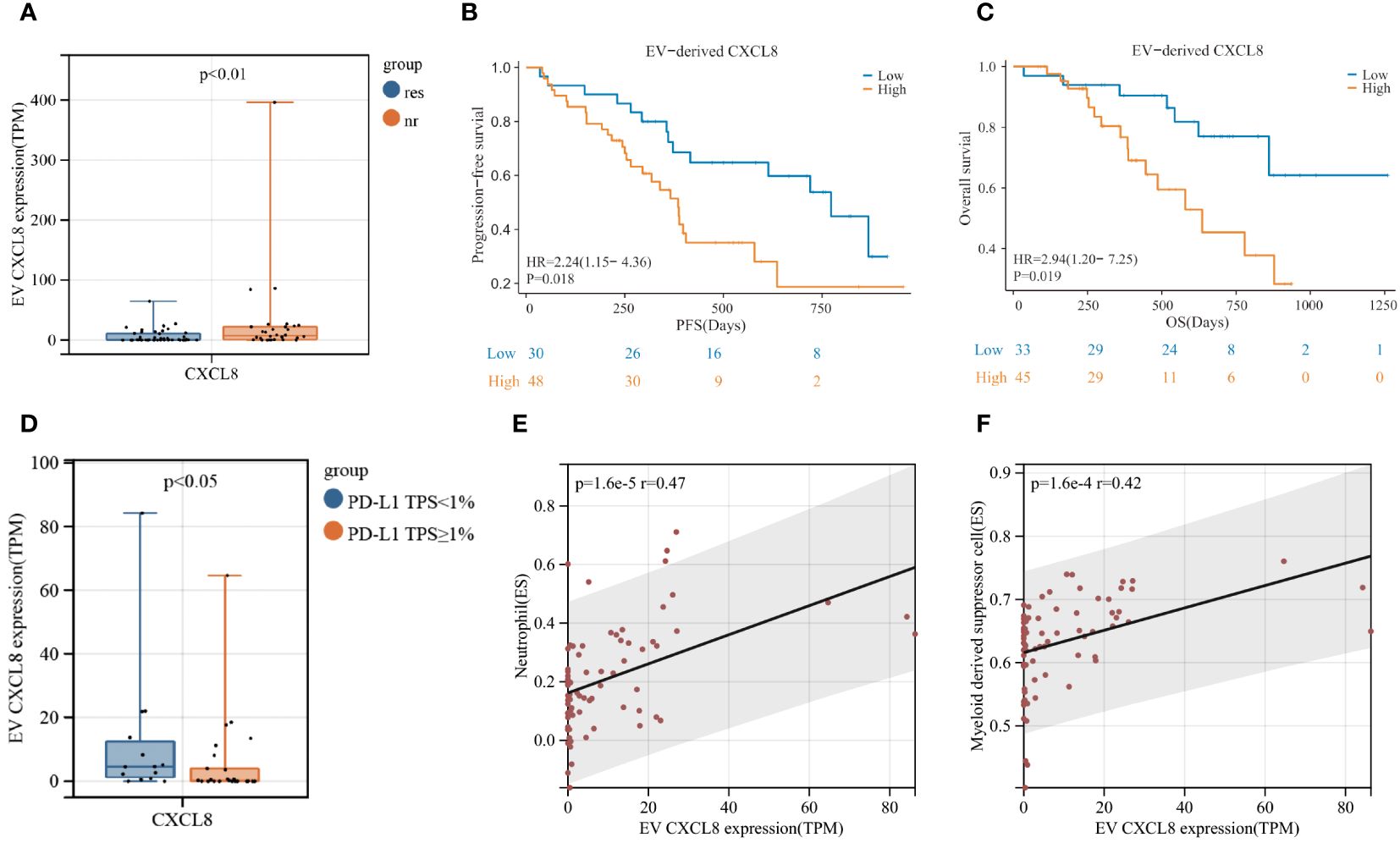
Figure 6 Elevated levels of EV-derived CXCL8 are associated with poor clinical efficacy of immunochemotherapy in LUSC patients. (A) Comparison of EV-derived CXCL8 expression levels between responders and non-responders. (B, C) Kaplan-Meier survival analysis (log-rank test) illustrating the impact of EV-derived CXCL8 expression on progression-free survival (PFS) (B) and overall survival (OS) (C) in LUSC patients. (D) Comparison of EV-derived CXCL8 expression levels between the PD-L1 TPS<1% group and PD-L1 TPS≥1% group. (E, F) Examination of the correlation between EV-derived CXCL8 levels and myeloid-derived suppressor cells (MDSC) (E) and neutrophils in LUSC patients.
4 Discussion
Our research contributes notably to the ongoing efforts to identify dependable biomarkers for immunotherapy-based treatments. To our knowledge, this study is pioneering in its analysis of the plasma exLRs transcriptome in advanced LUSC patients. Moreover, it’s the first to establish a predictive p-Signature that accurately forecasts the efficacy of first-line immunochemotherapy. This innovative approach, rooted in liquid biopsy techniques, offers a fresh and valuable perspective for pinpointing patients who are most likely to benefit from immunochemotherapy. By doing so, our study not only advances the field of precision medicine in oncology but also opens new pathways for personalized treatment strategies in lung cancer care.
In the quest for practical predictive biomarkers, new technologies that are easily implementable and capable of identifying novel biomarkers are in high demand. While PD-L1 expression level is the most widely used marker for immunotherapy efficacy prediction (26), recent evidence from the KEYNOTE 407 and RATIONALE 307 clinical trials demonstrated that advanced squamous lung cancer patients can benefit from Pembrolizumab plus chemotherapy regardless of their tissue PD-L1 status (5, 27). Furthermore, a retrospective analyze has shown that there was no predictive value of PD-L1 expression in squamous cell carcinoma in contrast to adenocarcinoma (6), suggesting that PD-L1 may not be an ideal biomarker for LUSC patients who received immunochemotherapy. Therefore, finding accessible biomarkers for patients with advanced squamous lung cancer is crucial. Liquid biopsy has emerged as a revolutionary strategy for cancer diagnosis and prognosis prediction (28, 29), given its noninvasive nature, ease of sample collection, ability to reflect the overall tumor status, and real-time monitoring capabilities. Previous studies have shown that plasma exLRs hold promise as biomarkers (30–33), and our previous research has indicated that CD160 has potential as a prognostic biomarker for LUAD patients receiving immunochemotherapy (19), albeit with limited success in LUSC prognosis prediction. Consequently, leveraging the availability of plasma samples and their capacity to reflect the systemic microenvironment, our study establishes a predictive signature based on plasma exLRs transcriptomics for patients with advanced/metastatic squamous lung cancer undergoing immunochemotherapy.
In our study, we first compared the plasma exLRs expression profiles of 78 patients with squamous lung cancer and 51 healthy subjects, revealing significant differences that suggest the potential utility of plasma exLRs as a biomarker. Subsequently, we evaluated the exLR profiles of responders and non-responders in 78 LUSC patients, and three exLRs were included in the construction of predictive signature of LUSC patients receiving immunochemotherapy. This signature efficiently distinguished responders from non-responders (respective cohort AUC = 0.904, prospective cohort AUC = 0.812). One of the key biomarkers identified, C-X-C motif chemokine ligand 8 (CXCL8/IL-8), is an angiogenic polypeptide expressed in multiple cancers (34). Previous studies have demonstrated that a decrease in serum IL-8 level is associated with decreased immunotherapy efficacy and longer OS in several advanced cancers (35–37). Moreover, a high level of CXCL8 is associated with decreased CD8+ T cell and neutrophil infiltration and contributes to immune evasion by regulating PD-L1 expression on macrophages (36, 38). Our study highlighted that EV-derived CXCL8 was correlated with a poor response to immunochemotherapy in LUSC patients, with high levels EV-derived CXCL8 being positively associated with MDSC and neutrophil infiltration. Additionally, the PD-L1 TPS<1% group exhibited high levels of EV-derived CXCL8. These findings underscore the potential of EV-derived CXCL8 as a biomarker for immunochemotherapy. The other two exLRs, succinate dehydrogenase complex assembly factor 1 (SDHAF1) and slingshot protein phosphatase 3 (SSH3), have not been extensively studied in the context of immunochemotherapy. SDHAF1 plays a crucial role in linking the citric acid cycle and electron transport chain (39, 40), while SSH3 has been identified as a potential antigen for developing mRNA-based vaccines against balder urothelial carcinoma (BLCA) and has been associated with colorectal cancer cell invasion and metastasis (41, 42). Our results reveal a positive correlation between these two exLRs and the response in LUSC patients who received immunochemotherapy, suggesting their potential roles that warrant further exploration. Overall, while our findings provide promising insights into the potential of these exLRs as biomarkers for immunochemotherapy response in LUSC patients, further studies are necessary to investigate their impact at the protein level and to elucidate the underlying mechanisms.
ExLRs are found in various bodily fluids due to active cellular secretion, and they can reflect the phenotype and functional states of their parent cells (43). Despite the strong predictive power of our p-Signature, ssGSEA analysis showed that a negative correlation between p-Signature and activated CD4+ and CD8+ T cells, suggesting that higher level p-Signature score may indicate an immune-evasion tumor immune microenvironment. Additionally, we observed that LUAD patients exhibited a significantly different TME compared to LUSC patients, and p-Signature was less effective in predicting prognosis among LUAD patients, highlighting its specificity for LUSC patients. However, the differences in TME between LUSC and LUAD require further investigation.
While our study has yielded valuable insights into the use of exLRs as biomarkers in LUSC treatment, we recognize certain limitations. Firstly, the patient cohort was relatively small and sourced exclusively from a single center, the FUSCC. Future studies should aim to include a more diverse and larger patient population from multiple centers, which would enhance the generalizability and robustness of our predictive model. Additionally, our study utilized an affinity-based method for EV isolation, which presents a limitation due to potential biases and variability affecting EV purity. To improve the accuracy and reliability of EV characterization, future studies should consider adopting methods such as size-exclusion chromatography or ultracentrifugation, as recommended by the Minimum Information for Studies of Extracellular Vesicles (MISEV) 2023 guidelines (44). These approaches are expected to provide a more representative EV population and further validate the predictive signature identified in this study. Furthermore, the specific roles of the three identified exLRs in modifying the TME warrant more detailed investigation. This is particularly true for EV-derived CXCL8, whose impact on the TME could provide deeper understanding and potentially influence future therapeutic strategies. Such expanded research efforts would be crucial in further validating and refining our findings.
In conclusion, our study marks a significant progression in identifying reliable biomarkers for immunochemotherapy in advanced LUSC. By analyzing the EV transcriptomic profiles of 78 advanced LUSC patients across both retrospective and prospective cohorts, we have successfully identified a LUSC-specific predictive signature (p-Signature) based on three exLRs. This p-Signature shows considerable potential in enhancing the precision of immunochemotherapy for lung cancer, potentially guiding more targeted and effective treatment strategies. Our findings represent a meaningful step forward in the pursuit of personalized medicine in the field of oncology.
Data availability statement
The datasets presented in this study can be found in The Genome Sequence Archive, and accession number for RNA-seq data is PRJNA1132376.
Ethics statement
The studies involving humans were approved by Fudan University Shanghai Cancer Center Medical Ethics Committee. The studies were conducted in accordance with the local legislation and institutional requirements. The participants provided their written informed consent to participate in this study.
Author contributions
JW: Conceptualization, Funding acquisition, Investigation, Project administration, Supervision, Validation, Writing – review & editing. ZH: Conceptualization, Investigation, Project administration, Supervision, Writing – review & editing. XZ: Conceptualization, Data curation, Formal analysis, Methodology, Software, Validation, Visualization, Writing – original draft. JL: Data curation, Investigation, Methodology, Visualization, Writing – original draft. WY: Data curation, Formal analysis, Writing – review & editing. QL: Methodology, Resources, Software, Writing – review & editing. ZW: Conceptualization, Investigation, Writing – review & editing. HY: Data curation, Resources, Writing – review & editing. XW: Data curation, Resources, Writing – review & editing. HW: Data curation, Resources, Writing – review & editing. SS: Data curation, Resources, Writing – review & editing. XMZ: Data curation, Resources, Writing – review & editing.
Funding
The author(s) declare financial support was received for the research, authorship, and/or publication of this article. This work was supported by Shanghai Municipal Health Commission (grant number: 2020CXJQ02), Shanghai Anti-Cancer Association (SACA-AX202210) and Shanghai Municipal Science and Technology Commission (grant number: 22Y31920405).
Acknowledgments
The authors thank all the patients and healthy volunteers who participated in this study.
Conflict of interest
The authors declare that the research was conducted in the absence of any commercial or financial relationships that could be construed as a potential conflict of interest.
Publisher’s note
All claims expressed in this article are solely those of the authors and do not necessarily represent those of their affiliated organizations, or those of the publisher, the editors and the reviewers. Any product that may be evaluated in this article, or claim that may be made by its manufacturer, is not guaranteed or endorsed by the publisher.
Supplementary material
The Supplementary Material for this article can be found online at: https://www.frontiersin.org/articles/10.3389/fimmu.2024.1421604/full#supplementary-material
Glossary
References
1. Siegel RL, Miller KD, Wagle NS, Jemal A. Cancer statistics, 2023. Ca-a Cancer J Clin. (2023) 73:17–48. doi: 10.3322/caac.21763
2. Shukla AA, Podder S, Chaudry SR, Benn BS, Kurman JS. Non-small cell lung cancer: epidemiology, screening, diagnosis, and treatment. Aims Med Sci. (2022) 9:348–61. doi: 10.3934/medsci.2022016
3. Doroshow DB, Sanmamed MF, Hastings K, Politi K, Rimm DL, Chen L, et al. Immunotherapy in non-small cell lung cancer: Facts and hopes. Clin Cancer Res. (2019) 25:4592–602. doi: 10.1158/1078-0432.CCR-18-1538
4. Socinski MA, Obasaju C, Gandara D, Hirsch FR, Bonomi P, Bunn PA Jr, et al. Current and emergent therapy options for advanced squamous cell lung cancer. J Thorac Oncol. (2018) 13:165–83. doi: 10.1016/j.jtho.2017.11.111
5. Novello S, Kowalski DM, Luft A, Gumus M, Vicente D, Mazieres J, et al. Pembrolizumab plus chemotherapy in squamous non small-cell lung cancer: 5-year update of the phase III KEYNOTE-407 study. J Clin Oncol. (2023) 41:1999–2006. doi: 10.1200/JCO.22.01990
6. Paz-Ares L, Vicente D, Tafreshi A, Robinson A, Parra HS, Mazieres J, et al. A randomized, placebo-controlled trial of pembrolizumab plus chemotherapy in patients with metastatic squamous NSCLC: Protocol-specified final analysis of KEYNOTE-407. J Thorac Oncol. (2020) 15:1657–69. doi: 10.1016/j.jtho.2020.06.015
7. Kim TK, Vandsemb EN, Herbst RS, Chen L. Adaptive immune resistance at the tumour site: mechanisms and therapeutic opportunities. Nat Rev Drug Discovery. (2022) 21:529–40. doi: 10.1038/s41573-022-00493-5
8. Herbst RS, Baas P, Kim D-W, Felip E, Perez-Gracia JL, Han J-Y, et al. Pembrolizumab versus docetaxel for previously treated, PD-L1-positive, advanced non-small-cell lung cancer (KEYNOTE-010): a randomised controlled trial. Lancet. (2016) 387:1540–50. doi: 10.1016/S0140-6736(15)01281-7
9. Ren D, Hua Y, Yu B, Ye X, He Z, Li C, et al. Predictive biomarkers and mechanisms underlying resistance to PD1/PD-L1 blockade cancer immunotherapy (vol 19, 19, 2020). Mol Cancer. (2020) 19(1).
10. Chan TA, Yarchoan M, Jaffee E, Swanton C, Quezada SA, Stenzinger A, et al. Development of tumor mutation burden as an immunotherapy biomarker: utility for the oncology clinic. Ann Oncol. (2019) 30:44–56. doi: 10.1093/annonc/mdy495
11. Subbiah V, Solit DB, Chan TA, Kurzrock R. The FDA approval of pembrolizumab for adult and pediatric patients with tumor mutational burden (TMB) ≥10: a decision centered on empowering patients and their physicians. Ann Oncol. (2020) 31:1115–8. doi: 10.1016/j.annonc.2020.07.002
12. Duchemann B, Remon J, Naigeon M, Cassard L, Jouniaux JM, Boselli L, et al. Current and future biomarkers for outcomes with immunotherapy in non-small cell lung cancer. Trans Lung Cancer Res. (2021) 10:2937–54. doi: 10.21037/tlcr
13. Raposo G, Stoorvogel W. Extracellular vesicles: Exosomes, microvesicles, and friends. J Cell Biol. (2013) 200:373–83. doi: 10.1083/jcb.201211138
14. Yanez-Mo M, Siljander PRM, Andreu Z, Zavec AB, Borras FE, Buzas EI, et al. Biological properties of extracellular vesicles and their physiological functions. J extracellular vesicles. (2015) 4:27066–6. doi: 10.3402/jev.v4.27066
15. Zhou E, Li Y, Wu F, Guo M, Xu J, Wang S, et al. Circulating extracellular vesicles are effective biomarkers for predicting response to cancer therapy. Ebiomedicine. (2021) 67:103365. doi: 10.1016/j.ebiom.2021.103365
16. Minciacchi VR, Freeman MR, Di Vizio D. Extracellular vesicles in cancer: exosomes, microvesicles and the emerging role of large oncosomes. Semin Cell Dev Biol. (2015) 40:41–51. doi: 10.1016/j.semcdb.2015.02.010
17. Correction: Correlation of plasma exosomal microRNAs with the efficacy of immunotherapy in EGFR / ALK wild-type advanced non-small cell lung cancer. J immunotherapy Cancer. (2020) 8.
18. de Miguel-Perez D, Russo A, Arrieta O, Ak M, Barron F, Gunasekaran M, et al. Extracellular vesicle PD-L1 dynamics predict durable response to immune-checkpoint inhibitors and survival in patients with non-small cell lung cancer. J Exp Clin Cancer Res. (2022) 41:186. doi: 10.1186/s13046-022-02379-1
19. Liao J, Lai H, Liu C, Zhang X, Ou Q, Li Q, et al. Plasma extracellular vesicle transcriptomics identifies CD160 for predicting immunochemotherapy efficacy in lung cancer. Cancer Sci. (2023) 114:2774–86. doi: 10.1111/cas.15804
20. Li Y, Zhao J, Yu S, Wang Z, He X, Su Y, et al. Extracellular Vesicles Long RNA Sequencing Reveals Abundant mRNA, circRNA, and lncRNA in Human Blood as Potential Biomarkers for Cancer Diagnosis. Clin Chem. (2019) 65:798–808. doi: 10.1373/clinchem.2018.301291
21. Amin MB, Greene FL, Edge SB, Compton CC, Gershenwald JE, Brookland RK, et al. The Eighth Edition AJCC Cancer Staging Manual: Continuing to build a bridge from a population-based to a more "personalized" approach to cancer staging. Ca-a Cancer J Clin. (2017) 67:93–9. doi: 10.3322/caac.21388
22. Eisenhauer E, Therasse P, Bogaerls J, Schwartz L, Sargent D, Ford R, et al. New response evaluation criteria in solid tumors: revised RECIST guideline version 1. 1. Ejc Suppl. (2008) 6:13–3. doi: 10.1016/S1359-6349(08)71964-5
23. Mandaliya H, Jones M, Oldmeadow C, Nordman IIC. Prognostic biomarkers in stage IV non-small cell lung cancer (NSCLC): neutrophil to lymphocyte ratio (NLR), lymphocyte to monocyte ratio (LMR), platelet to lymphocyte ratio (PLR) and advanced lung cancer inflammation index (ALI). Trans Lung Cancer Res. (2019) 8:886–94. doi: 10.21037/tlcr
24. Peng L, Wang Y, Liu F, Qiu X, Zhang X, Fang C, et al. Peripheral blood markers predictive of outcome and immune-related adverse events in advanced non-small cell lung cancer treated with PD-1 inhibitors. Cancer Immunol Immunotherapy. (2020) 69:1813–22. doi: 10.1007/s00262-020-02585-w
25. Platini H, Ferdinand E, Kohar K, Prayogo SA, Amirah S, Komariah M, et al. Neutrophil-to-lymphocyte ratio and platelet-to-lymphocyte ratio as prognostic markers for advanced non-small-cell lung cancer treated with immunotherapy: A systematic review and meta-analysis. Medicina-Lithuania. (2022) 58:1069. doi: 10.3390/medicina58081069
26. Doroshow DB, Wei W, Gupta S, Zugazagoitia J, Robbins C, Adamson B, et al. Programmed death-ligand 1 tumor proportion score and overall survival from first-line pembrolizumab in patients with nonsquamous versus squamous NSCLC. J Thorac Oncol. (2021) 16:2139–43. doi: 10.1016/j.jtho.2021.07.032
27. Wang J, Lu S, Yu X, Hu Y, Yang N. Tislelizumab plus chemotherapy vs chemotherapy alone as first-line treatment for advanced squamous non–small-cell lung cancer: A phase 3 randomized clinical trial. JAMA Oncol. (2021) 7:709–17. doi: 10.1001/jamaoncol.2021.0366
28. Li W, Liu J-B, Hou L-K, Yu F, Zhang J, Wu W, et al. Liquid biopsy in lung cancer: significance in diagnostics, prediction, and treatment monitoring. Mol Cancer. (2022) 21:25. doi: 10.1186/s12943-021-01462-z
29. Nikanjam M, Kato S, Kurzrock R. Liquid biopsy: current technology and clinical applications. J Hematol Oncol. (2022) 15. doi: 10.1186/s13045-022-01351-y
30. Su Y, Li Y, Guo R, Zhao J, Chi W, Lai H, et al. Plasma extracellular vesicle long RNA profiles in the diagnosis and prediction of treatment response for breast cancer (vol 7, 154, 2021). NPJ Breast Cancer. (2022) 8:34. doi: 10.1038/s41523-021-00356-z
31. Yu S, Li Y, Liao Z, Wang Z, Wang Z, Li Y, et al. Plasma extracellular vesicle long RNA profiling identifies a diagnostic signature for the detection of pancreatic ductal adenocarcinoma. Gut. (2020) 69:540–50. doi: 10.1136/gutjnl-2019-318860
32. Guo W, Zhou B, Zhao L, Huai Q, Tan F, Xue Q, et al. Plasma extracellular vesicle long RNAs predict response to neoadjuvant immunotherapy and survival in patients with non-small cell lung cancer. Pharmacol Res. (2023) 196:106921. doi: 10.1016/j.phrs.2023.106921
33. Liu C, Chen J, Liao J, Li Y, Yu H, Zhao X, et al. Plasma extracellular vesicle long RNA in diagnosis and prediction in small cell lung cancer. Cancers. (2022) 14:5493. doi: 10.3390/cancers14225493
35. Sanmamed MF, Perez-Gracia JL, Schalper KA, Fusco JP, Gonzalez A, Rodriguez-Ruiz ME, et al. Changes in serum interleukin-8 (IL-8) levels reflect and predict response to anti-PD-1 treatment in melanoma and non-small-cell lung cancer patients. Ann Oncol. (2017) 28:1988–95. doi: 10.1093/annonc/mdx190
36. Schalper KA, Carleton M, Zhou M, Chen T, Feng Y, Huang S-P, et al. Elevated serum interleukin-8 is associated with enhanced intratumor neutrophils and reduced clinical benefit of immune-checkpoint inhibitors. Nat Med. (2020) 26:688–92. doi: 10.1038/s41591-020-0856-x
37. Yuen KC, Liu L-F, Gupta V, Madireddi S, Keerthivasan S, Li C, et al. High systemic and tumor-associated IL-8 correlates with reduced clinical benefit of PD-L1 blockade. Nat Med. (2020) 26:693–8. doi: 10.1038/s41591-020-0860-1
38. Lin C, He H, Liu H, Li R, Chen Y, Qi Y, et al. Tumour-associated macrophages-derived CXCL8 determines immune evasion through autonomous PD-L1 expression in gastric cancer. Gut. (2019) 68:1764–73. doi: 10.1136/gutjnl-2018-316324
39. Na U, Yu W, Cox J, Bricker DK, Brockmann K, Rutter J, et al. The LYR factors SDHAF1 and SDHAF3 mediate maturation of the iron-sulfur subunit of succinate dehydrogenase. Cell Metab. (2014) 20:253–66. doi: 10.1016/j.cmet.2014.05.014
40. Roma A, Tcheng M, Ahmed N, Walker S, Jayanth P, Minden MD, et al. Glutamine metabolism mediates sensitivity to respiratory complex II inhibition in acute myeloid leukemia. Mol Cancer Res. (2022) 20:1659–73. doi: 10.1158/1541-7786.MCR-21-1032
41. Sun Z, Jing C, Zhan H, Guo X, Suo N, Kong F, et al. Identification of tumor antigens and immune landscapes for bladder urothelial carcinoma mRNA vaccine. Front Immunol. (2023) 14. doi: 10.3389/fimmu.2023.1097472
42. Hu Y-H, Lu Y-X, Zhang Z-Y, Zhang J-M, Zhang W-J, Zheng L, et al. SSH3 facilitates colorectal cancer cell invasion and metastasis by affecting signaling cascades involving LIMK1/Rac1. Am J Cancer Res. (2019) 9:1061–73.
43. Zhou R, Chen KK, Zhang J, Xiao B, Huang Z, Ju C, et al. The decade of exosomal long RNA species: an emerging cancer antagonist. Mol Cancer. (2018) 17:75. doi: 10.1186/s12943-018-0823-z
Keywords: extracellular vesicles, lung squamous cell carcinoma, immunochemotherapy, predictive signature, RNA sequencing
Citation: Zhang X, Liao J, Yang W, Li Q, Wang Z, Yu H, Wu X, Wang H, Sun S, Zhao X, Hu Z and Wang J (2024) Plasma extracellular vesicle long RNA profiling identifies a predictive signature for immunochemotherapy efficacy in lung squamous cell carcinoma. Front. Immunol. 15:1421604. doi: 10.3389/fimmu.2024.1421604
Received: 22 April 2024; Accepted: 16 July 2024;
Published: 05 August 2024.
Edited by:
Shi Hu, Second Military Medical University, ChinaReviewed by:
Xu Haipeng, Fujian Provincial Cancer Hospital, ChinaShuxiang Ma, Affiliated Cancer Hospital of Zhengzhou University, China
Copyright © 2024 Zhang, Liao, Yang, Li, Wang, Yu, Wu, Wang, Sun, Zhao, Hu and Wang. This is an open-access article distributed under the terms of the Creative Commons Attribution License (CC BY). The use, distribution or reproduction in other forums is permitted, provided the original author(s) and the copyright owner(s) are credited and that the original publication in this journal is cited, in accordance with accepted academic practice. No use, distribution or reproduction is permitted which does not comply with these terms.
*Correspondence: Jialei Wang, d2FuZ2ppYWxlaUBzaGNhLm9yZy5jbg==; Zhihuang Hu, emhpaHVhbmdodUBob3RtYWlsLmNvbQ==
†These authors have contributed equally to this work and share first authorship