- 1Department of Hematology, Guangdong Provincial People’s Hospital (Guangdong Academy of Medical Sciences), Southern Medical University, Guangzhou, China
- 2Department of Laboratory Medicine, Guangdong Engineering and Technology Research Center for Rapid Diagnostic Biosensors, Nanfang Hospital, Southern Medical University, Guangzhou, China
Introduction: Exploring monocytes’ roles within the tumor microenvironment is crucial for crafting targeted cancer treatments.
Methods: This study unveils a novel methodology utilizing four 20-color flow cytometry panels for comprehensive peripheral immune system phenotyping, specifically targeting classical, intermediate, and non-classical monocyte subsets.
Results: By applying advanced dimensionality reduction techniques like t-distributed stochastic neighbor embedding (tSNE) and FlowSom analysis, we performed an extensive profiling of monocytes, assessing 50 unique cell surface markers related to a wide range of immunological functions, including activation, differentiation, and immune checkpoint regulation.
Discussion: This in-depth approach significantly refines the identification of monocyte subsets, directly supporting the development of personalized immunotherapies and enhancing diagnostic precision. Our pioneering panel for monocyte phenotyping marks a substantial leap in understanding monocyte biology, with profound implications for the accuracy of disease diagnostics and the success of checkpoint-inhibitor therapies. Key findings include revealing distinct marker expression patterns linked to tumor progression and providing new avenues for targeted therapeutic interventions.
1 Introduction
Monocytes represent a heterogeneous cell population of circulating leukocytes characterized by variations in phenotype and function (1). They play a pivotal role in coordinating both innate and adaptive immune responses to pathogens and endogenous stimuli (2, 3). Monocytes execute a spectrum of functions including phagocytosis, release of reactive oxygen species, secretion of cytokines and chemokines, recruitment of neutrophils, antigen presentation, and modulation of lymphocyte activity. Monocytes can be divided into three subsets based on the presence of the cell surface lipopolysaccharide (LPS) co-receptor CD14, and Fc-Gamma Receptor III (CD16). CD14++ CD16− classical monocytes account for ~85% of circulating monocytes, intermediate cells (CD14++ CD16+) for ~5% and non-classical cells (CD14- CD16++) for ~10% of circulating monocytes (4, 5). In the context of inflammation and tumor microenvironment (TME), there is a notable increase in monocyte populations (6). For instance, the ratio of classical monocytes is a sensitive and specific marker for Chronic Myelomonocytic Leukemia (CMML), differentiating it from Myelodysplastic Syndrome (MDS) (7), yet it does not distinguish CMML from atypical chronic myeloid leukemia (aCML) (8). This highlights the limitations of relying solely on CD14/CD16 and the necessity for exploring additional markers and reclassifying monocyte subgroups for more precise diagnostics
Based on their ability to regulate immune responses, Mesenchymal stromal cells (MSCs) are considered to be potential candidates for managing immune-mediated diseases in the context of immune therapy (9). Monocytes engage in extensive interactions with MSCs and other immune cells, facilitated by immune-related antigens, surface molecules, and regulatory factors (9, 10). These interactions are part of the complex communication network within the TME that influences immune responses and cancer progression and may offer potential targets for therapeutic interventions. These molecules, including costimulatory markers such as CD86, CD80, CD163, CD73, and HLA-DR (11–13), along with co-inhibitory receptors (e.g., PD-1, LAG-3), and cytokine/chemokine receptors (e.g., CX3CR1, CXCR5).
The costimulatory markers are integral to T cell biology, influencing the functional outcome of T cell receptor (TCR) signaling and dictating T cell function and fate (14). Investigating the co-stimulatory signals on monocytes enhances our understanding of their impact on the activation, proliferation, and function of immune cells, such as T cells and MSCs, mediated by monocytes. Delving into these signals involving monocytes uncovers the underlying mechanisms of immune responses, thus revealing potential mechanisms underlying disease progression and informing the development of effective strategies for disease prevention, diagnosis, and immunotherapy treatment. Moreover, costimulatory signals play a critical role in regulating the establishment and maintenance of immune tolerance (15, 16). Understanding their intricate involvement in immune tolerance mechanisms not only enhances our understanding of the mechanisms of a wide range of diseases, including autoimmune diseases, infectious diseases, tumors, and various other illnesses but also propels the development of innovative approaches to research on immune tolerance (14). The significance of thoroughly studied co-stimulatory signals and activation markers on monocytes, as essential components of antigen presentation cells, cannot be overstated.
In recent years, checkpoint inhibitors gained increasing recognition as promising therapeutic targets. Understanding checkpoints on monocytes can provide valuable insights into the mechanisms of interaction between monocytes and MSCs, and offer critical clues for the development of novel immunotherapy strategies. Only individual checkpoints on monocytes in tumors and diseases have been studied in previous studies, with a lack of comprehensive systematic investigation of checkpoints on monocytes reported (17–19). As the utilization of checkpoint inhibitor immunotherapies continues to expand within the realm of cancer research and clinical practice, there emerges a pressing requirement for assays capable of accurately characterizing the phenotypes of immune cells in patients (20). We offer a comprehensive panel to assess the expression of monocyte checkpoints, facilitating a thorough understanding of the immunomodulatory effects of monocytes in diseases and the identification of effective immunotherapy targets.
Cytokine/chemokine receptors, a class of membranous receptors, play pivotal roles in intercellular signaling, such as monocytes and MSCs, orchestrating fundamental biological processes containing immune response, inflammation, and cellular proliferation (21). A comprehensive understanding of monocyte characteristics requires an exploration of cytokine receptors. Insights gained from studying these receptors will aid researchers in understanding the intricate regulatory mechanisms of the immune system, offering valuable guidance for disease treatment and the development of immunotherapies.
Flow cytometry stands out as a highly powerful tool for unraveling the diversity and functional alterations of monocytes. This elucidation is crucial for comprehending their mechanisms and devising therapeutic strategies for diseases. Hence, we employ a multi-color spectral flow cytometry approach to investigate the panel of 50 markers in peripheral blood monocytes. By examining multiple costimulatory signals, activation markers, cytokine receptors, differentiation markers, and immune checkpoints, we aim to explore the surface marker expression profile of monocytes and dissect the differences among the three monocyte subgroups. This initiative facilitates the early detection of diseases, provides the target for immunotherapy, and enhances comprehension of the functions performed by monocytes.
2 Materials and methods
2.1 Blood donors and ethics statement
For screening experiments, peripheral venous blood samples (2 mL) were collected in BD K2-EDTA anticoagulant (BD Biosciences) by venipuncture from 20 healthy volunteers including 11 males (55%) and 9 females (45%). The average age for males is 48.7 (35-61) years old, and the females are 43.5 (16-63) years old (P=0.61).
This study received approval from the Ethics Committee of the Guangdong Provincial Hospital (Ethical Approval Number: KY-Z-2021-300-02). In strict accordance with the Declaration of Helsinki, we rigorously implemented informed consent procedures. Written consent, informed and comprehensive, was obtained from every participant enrolled in this study.
2.2 Flow cytometry sample preparation
Peripheral blood samples were collected with K2-EDTA as an anticoagulant, and mature red blood cells were lysed within 15 mins using 1 ml of 1x Lysing Buffer (BD Biosciences, San Jose, CA, USA) without any fixative. The resulting cell suspension was maintained in phosphate-buffered saline (PBS) containing 2% fetal bovine serum (GIBCO). The cell suspension was allocated into 4 test tubes, ensuring that the number of nucleated cells in each tube did not exceed 1×106. Cells were stained with the following monoclonal antibodies (5 μL/sample) within 50μL BV buffer (BD), distributed evenly across the 4 tubes:
(1) BB515-conjugated CD45RA, PE-CY7-conjugated CD197(CCR7), PE-CF594-conjugated CD25, BV605-conjugated CD28, APC-R700-conjugated CD127 (IL-7Ra), APC-conjugated CD161, APC-R700-conjugated CD27, BV786-conjugated CD38; PE-conjugated CD57; BV421-conjugated CD69
(2) BC515-conjugated NKP44(CD336), PE-Dazzle594-conjugated NKG2A(CD159A), PE-Fire 810-conjugated CD86, BV605-conjugated CD80, R718-conjugated KIR3DL1(CD158E1), APC-conjugated CD163, APC-H7-conjugated HLA-DR, BV785-conjugated NKG2D(CD314); PE-conjugated KIR2DL1(CD158A), BV421-conjugated NKp46(CD335);
(3) BB515-conjugated CX3CR1, PE-Dazzle594-conjugated CD39, PE-Fire 810-conjugated CD194(CCR4), BV605-conjugated CD47, AF700-conjugated CD64, APC-conjugated CD34, APC-CY7-conjugated CD36, BV785-conjugated CD274(PD-L1); PE-conjugated CD73, BV421-conjugated CD123(IL-3R);
(4) BB515-conjugated CD278(ICOS), PE-Dazzle594-conjugated Tim-3(CD366), PE-Fire 810-conjugated TIGIT, BV605-conjugated CD152(CTLA-4), APC-R700-conjugated CD223(LAG-3), APC-conjugated CD272(BTLA), APC-CY7-conjugated CD117(c-kit), BV785-conjugated CXCR5(CD185); PE-conjugated CD294(CRTH2), BV421-conjugated CD279 (PD-1);
All cells were stained with the following shared monoclonal antibodies: PerCP-conjugated CD45; BV510-conjugated CD3; PerCP-eFluor 710-conjugated TCR-γδ; BV480-conjugated CD19; AF647-conjugated CD56; BV711-conjugated CD16; BV570-conjugated CD11b; efluor 450-conjugated CD11c; Spark Blue 550-conjugated CD14; FITC-conjugated CD15; Detailed antibody information can be found in Table 1. After staining, samples were incubated at room temperature for 30 minutes in the dark, then treated in 2 ml PBS containing 2% fetal bovine serum (GIBCO). After two consecutive washing steps, centrifugation was performed at 300 × g for 5 min before samples were collected on a Cytek Aurora/NL (Cytek Biosciences) using Cytek SpectroFlo software.
2.3 Flow cytometry data analysis
Flow cytometry data was analyzed using Flowjo (BD Biosciences, San Jose, CA, USA) (Version 10.6.2, https://www.flowjo.com/). The expression pattern of each marker of 4 panels was assessed in the monocytes. FlowSOM, utilizing Self-Organizing Maps (SOMs), based on marker expression phenotype, was employed to assign all individual cells into clusters and metaclusters (group of clusters) (22), FlowSOM was used with default settings unless otherwise noted.
3 Results
3.1 Full spectrum flow cytometry measures the subsets of human peripheral blood cells
Utilizing full spectrum flow cytometry, we selected the major immune cell lineage markers listed in Table 1 to establish a multicolor panel for the comprehensive immuno-phenotyping of human peripheral blood samples. Duplexes were excluded from the total cell population using FSC-H versus FSC-A plots. Mononuclear cells were gated using side scatter (SSC) versus CD45 dot plots after the exclusion of debris. Initial gating procedures involved: (i) identifying pan-leukocytes through CD45 expression, (ii) excluding deceased cells and debris based on FSC/SSC attributes, (iii) eliminating doublets and aggregates using distinct FSC signals, and, if necessary, (iv) excluding counting bead contamination. Subsequently, PBMCs were isolated from granulocytes utilizing position-based gating strategies, which allowed for the precise separation of these distinct cell populations (Figure 1A). By strategically positioning gates, PBMCs were effectively distinguished, facilitating subsequent analyses focused on this particular cell subset by not gate strategies. T cells were isolated from granulocytes utilizing a bivariate CD3/CD14 plot. CD3+ T cells are subsequently divided into αβ/γδ T cells by CD3/TCR γδ plot. The NKT cells were divided from the αβ T cells by CD56/CD3 plot. Within the CD3+ T cell-excluded PBMC compartment, CD19+ B cells are defined. The bulk of CD3/CD19 double-negative cells contain CD11b-/CD14- natural killer (NK) cells expressing CD56 (and for the most part CD16) as well as populations of CD11b/CD14 single- or double-positive cells. This latter fraction comprises monocyte populations that express CD14 and/or CD16 as well as a population of CD14/CD16 double-negative cells. The CD14-/CD16- fraction contains both CD11c+ myeloid dendritic cells (mDCs) and CD11c-/CD11b+ plasmacytoid dendritic cells (pDCs). CD15+ granulocytes are subsequently divided into CD16+ neutrophils and CD16- eosinophils (Figure 1B).
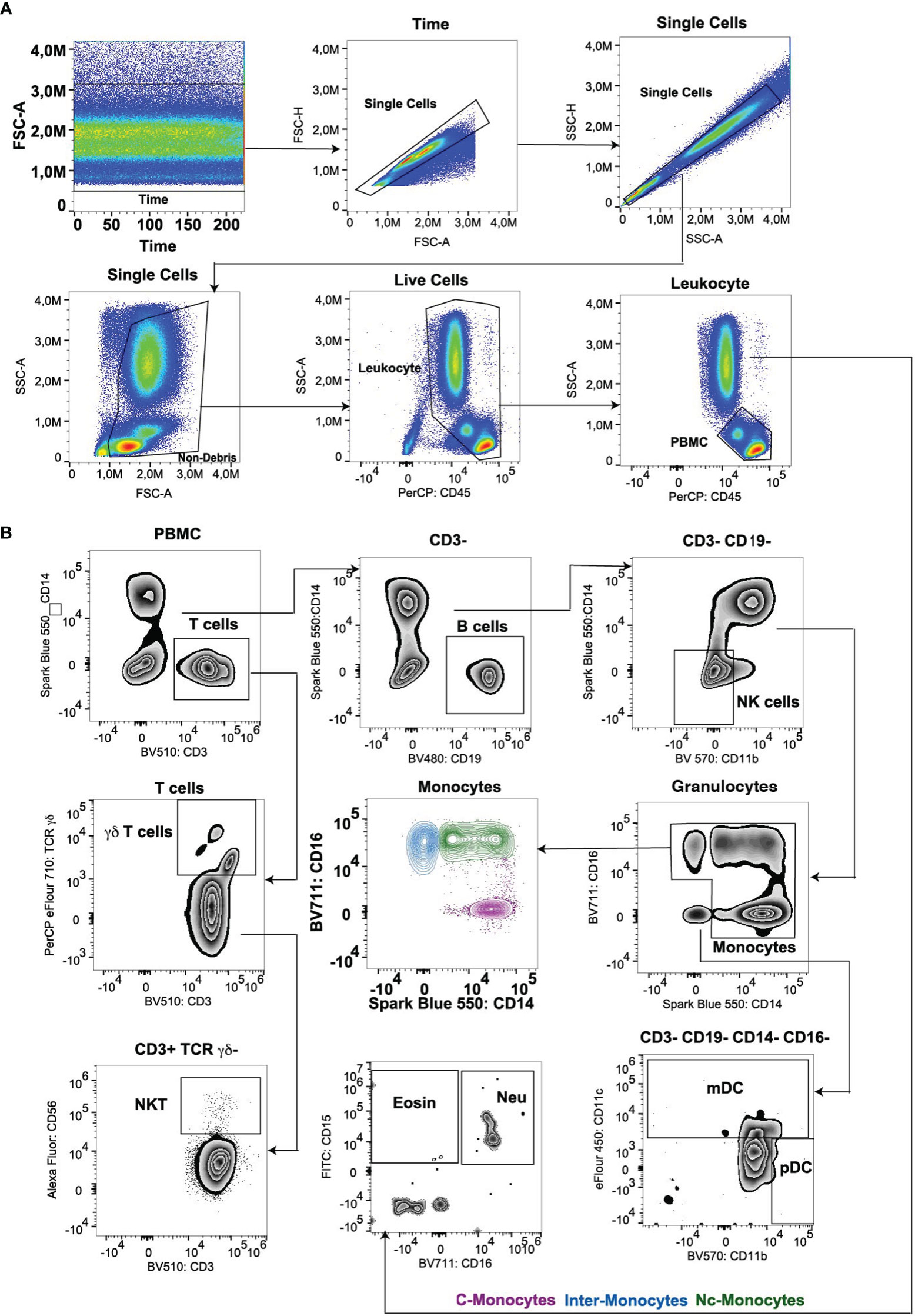
Figure 1 (A) Gating strategy for lineage panel of peripheral blood leukocyte subsets. (B) Gating main PBMC cell subsets (T, B, NK, monocytes, and DCs) and Myeloids (eosinophil and neutrophil). C-Mono, classical monocytes; Inter-Mono, intermediate monocytes; Nc-Mono, non-classical monocytes; Eosin, Eosinophil; Neu, neutrophil.
3.2 Comprehensive analysis of multiple myeloid markers in human monocyte subsets
In our investigation of monocyte subsets utilizing an extensive panel of surface markers, including CD14, CD16, CD64, CD123, CD34, CD36, CD123, CD47, CX3CR1, CCR4, CXCXR5, CD39, and CD73, we observed a diversity of phenotypic profiles within the monocyte population. While the classical (CD14++CD16-), intermediate (CD14++CD16+), and non-classical (CD14-CD16++) subsets were readily discernible, our multi-marker approach unveiled transitional populations exhibiting intermediate phenotypes (Figure 2C).
Simultaneously, a comparison was made on DCs (Figure 2A). Expression levels of CD64, CD123, and CD34 significantly contributed to delineating monocyte subsets and their functional attributes. Elevated expression of CD64 was associated with classical monocytes and pDCs, and correlated with enhanced phagocytic activity and pro-inflammatory cytokine secretion (Figures 2B, D). Conversely, higher levels of CD34 expression were observed in intermediate and non-classical monocytes, indicative of anti-inflammatory and tissue-resident properties (Figures 2B, D). Elevated CD123 expression on monocytes has been linked to certain pathological conditions, such as hematological malignancies (23) and autoimmune disorders (24), highlighting its potential as a biomarker for disease monitoring and therapeutic targeting, while normal monocytes exhibited a lack of CD123 expression (Figures 2B, D). The expression of CD36, CD47, and CX3CR1 delineated distinct functional states within monocyte subsets. CD36 expression is associated with enhancing lipid uptake and metabolic functions (25) predominantly expressed in classical monocytes. CD36hi monocytes may contribute to the peripheral development of Foxp3+ T-bet+ T cells with regulatory functions (26). While CD47 was expressed in normal DCs (mDCs and pDCs subsets) associated with immune evasion and anti-phagocytic properties (Figures 2B, D), in autoimmune diseases and inflammatory-related diseases, CD47 is highly expressed in monocytes (27, 28). CX3CR1 expression correlated with tissue homing and surveillance functions, suggesting a role in intermediate monocyte subset trafficking and localization within peripheral tissues (Figures 2B, D).
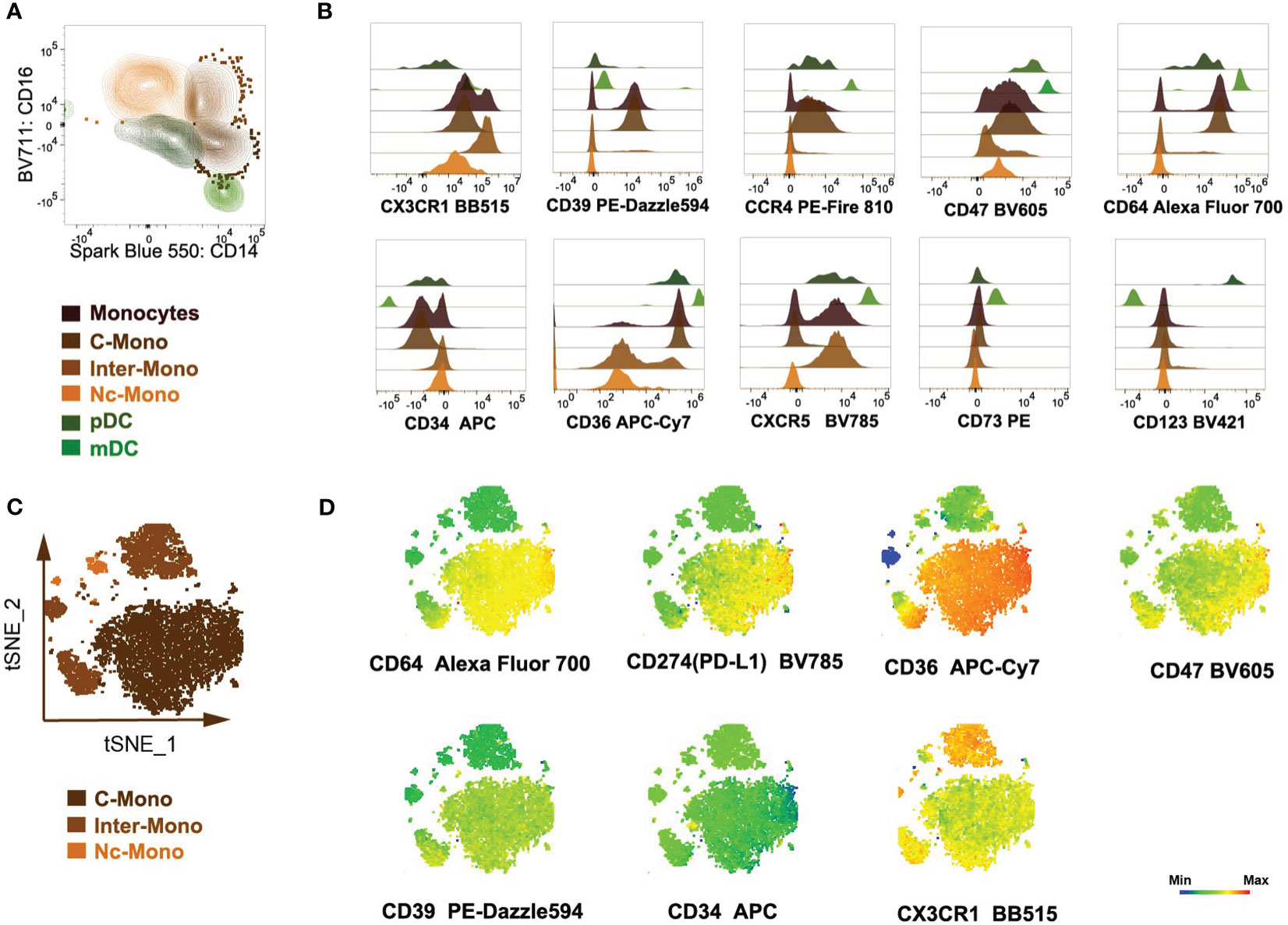
Figure 2 Analysis of peripheral blood monocytes cells of myeloid markers and chemokine receptors. (A) Monocytes and DCs were isolated utilizing a bivariate CD14/CD16 plot. (B) Histogram overlays depicted the expression of myeloid markers and chemokine receptor markers within monocytes and DCs population. (C, D) Semi-automated analysis of flow cytometry data by tSNE. Scale bars of the tSNE plot represented color-coded scaled fluorescence intensity or cell count levels.
Analysis of chemokine receptor expression revealed differential patterns among monocyte subsets. While CCR4 expression was enriched in classical monocytes, CXCR5 expression was predominantly observed in intermediate monocytes (Figures 2B, D), implicating differential chemotactic responses and migratory behaviors within the monocyte pool. CD73 is expressed on approximately 50-70% of lymphoid cells, whereas it is not expressed on normal monocytes. CD39 is expressed on >90% of monocytes (13), mostly on classical monocytes (Figure 2D).
3.3 Co-stimulatory and activation markers are differently expressed in monocyte subpopulations
Through the utilization of t-distributed stochastic neighbor embedding (tSNE) analysis, distinct subsets including classical monocytes (C-Mono), intermediate monocytes (Inter-Mono), non-classical monocytes (Nc-Mono), pDCs, and mDCs were delineated (Figure 3A). We compared the co-stimulatory and activation markers of different monocyte subpopulations. HLA-DR, CD163, CD80, and CD86 revealed intricate patterns of expression indicative of functional diversity within the monocyte subpopulations (Figure 3B). Monocytes exhibited heterogeneous expression patterns of the co-stimulatory molecules CD86, critical for antigen presentation and T cell activation. Classical and intermediate monocytes displayed high expression levels of CD86, suggesting an activated phenotype involved in antigen presentation, with the up-regulated expression on intermediate monocytes correlating with disease severity and impacting immunomodulatory processes (11, 29). CD80 expression did not exhibit subset-specific variations suggesting that its expression may be regulated by common mechanisms across all monocyte subsets (Figure 3B). Additionally, the expression of HLA-DR varied among monocyte subsets, with classical monocytes showing high levels of expression, indicative of their involvement in antigen presentation and immune surveillance (Figure 3B). The presence of CD163 on classical monocytes suggests their anti-inflammatory properties and potential involvement in phagocytic clearance and inflammation resolution (12) (Figure 3B). Furthermore, to assess the specificity of our monocyte sorting process, we utilized negative controls targeting markers specifically associated with NK cells (NKp44, NKp46, NKG2A, KIR2DL1, NKG2D, and KIR3DL1) (Figure 3C). These negative controls confirmed the absence of NK cell contamination in the sorted cell population, ensuring the purity and specificity of the isolated monocyte subset.
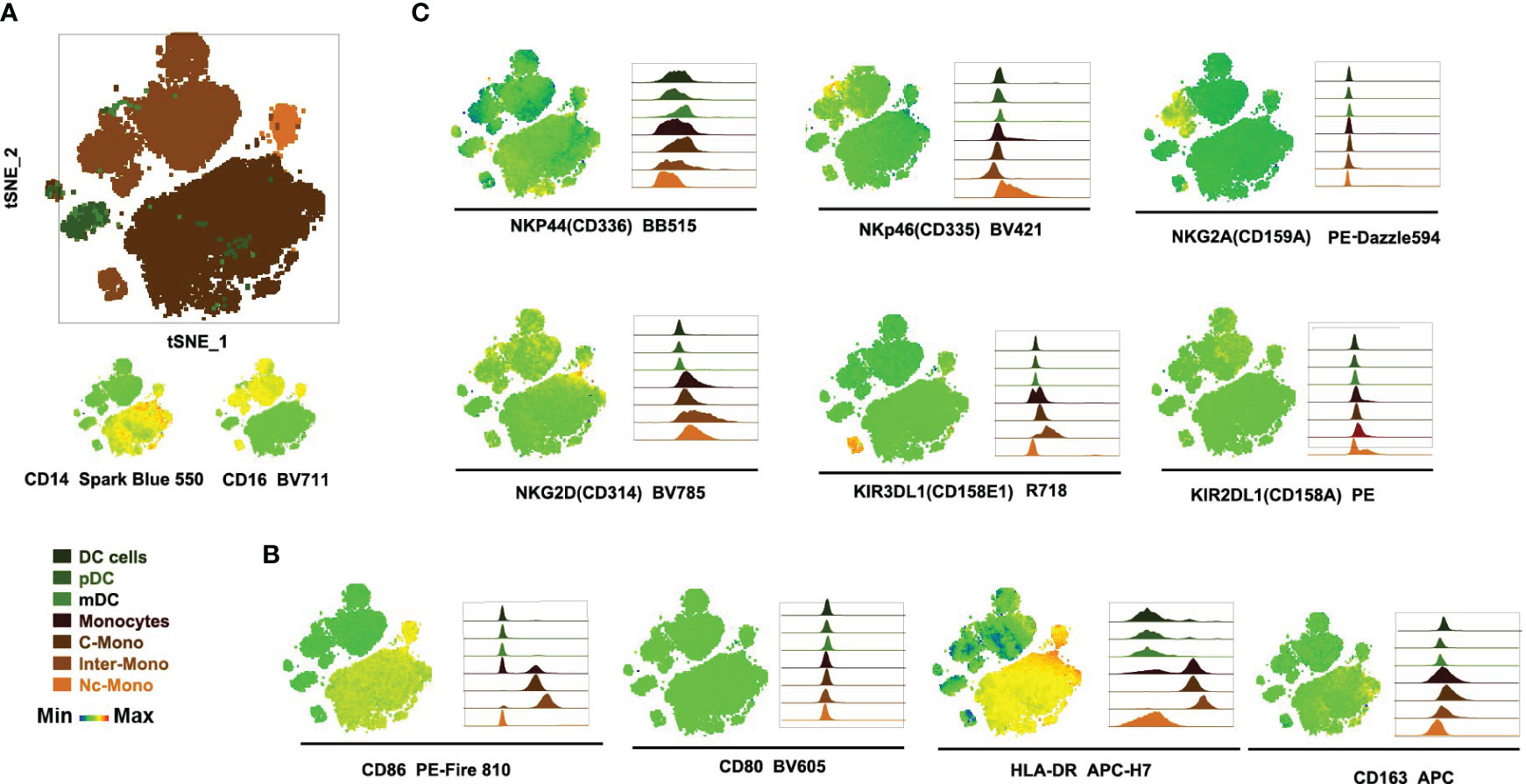
Figure 3 Expression of activating receptors and co-stimulation markers by monocyte subsets. (A) Three monocyte subsets clustered by tSNE. (B, C) Visualization of co-stimulation and activation markers in monocyte subsets, with the left side showing tSNE plots and the right side showing histogram plots.
3.4 Checkpoint markers expression pattern analysis between monocyte subsets
Next, the expression profiles of immune checkpoint molecules and chemokine receptors on monocytes and DCs were investigated, including CTLA4, CD272, CRTH2/CD294, LAG-3, ICOS/CD278, TIM-3, TIGIT, PD-1, CD117, CXCR5, elucidated the nuanced heterogeneity and regulatory potential within the monocyte and DCs population. CD294 exhibits a subdued expression within the Inter-mono subset while manifesting discernible expression levels within mDCs and pDCs subsets. CD117, CXCR5, and PD-1 are mildly expressed within the mDCs subset but remain absent in the pDCs subset as well as across all monocyte subsets (Figure 4A), with PD-1 highly expressed in monocytes in patients with hepatocellular carcinoma, presenting a preference toward M2 polarization and had a deficiency in supporting CD8 T cells (18). CTLA-4 demonstrates ubiquitous expression across all DC subsets, contrasting with its absence within all monocyte subsets (Figure 4A). Regarding CD278/ICOS, there is a distinctive distribution observed within the C-Mono subset, where a minority of cells exhibit diminished levels of CD278/ICOS expression (Figure 4C). TIGIT displays a scarce expression within a limited population of Nc-Mono cells (Figure 4A), while decreased expression of TIGIT on CD14 + monocytes correlates with clinical features and laboratory parameters of patients with primary Sjögren’s syndrome (19). Furthermore, we observed that co-inhibitory molecules and exhaustion (CD272, LAG-3/CD223, and TIM-3) were not differently expressed on three monocyte subsets under normal physiological conditions (Figures 4A, C), although increased Tim-3+ monocytes/macrophages are associated with disease severity in patients with IgA nephropathy (30). Activated platelets promote increased monocyte expression of CXCR5 through prostaglandin E2-related mechanisms and enhance the anti-inflammatory effects of CXCL13 (21), whereas CXCR5 is upregulated in Ly6Clow inflammatory monocyte cells from all mice, presenting anti-inflammatory/atherogenic features (31). Although the expression of CD294 was low, it was specifically expressed by the non-classical monocyte subset at the RNA level (32). CD117, a cytokine receptor expressed on the surface of hematopoietic stem cells with a likely role in cell survival, proliferation, and differentiation (33), was lacking in three monocyte subsets. These findings underscore the diversity and regulatory complexity of monocytes in immune homeostasis and pathology, emphasizing the need for further investigation into the functional implications of immune checkpoint expression in monocyte-mediated immune responses.
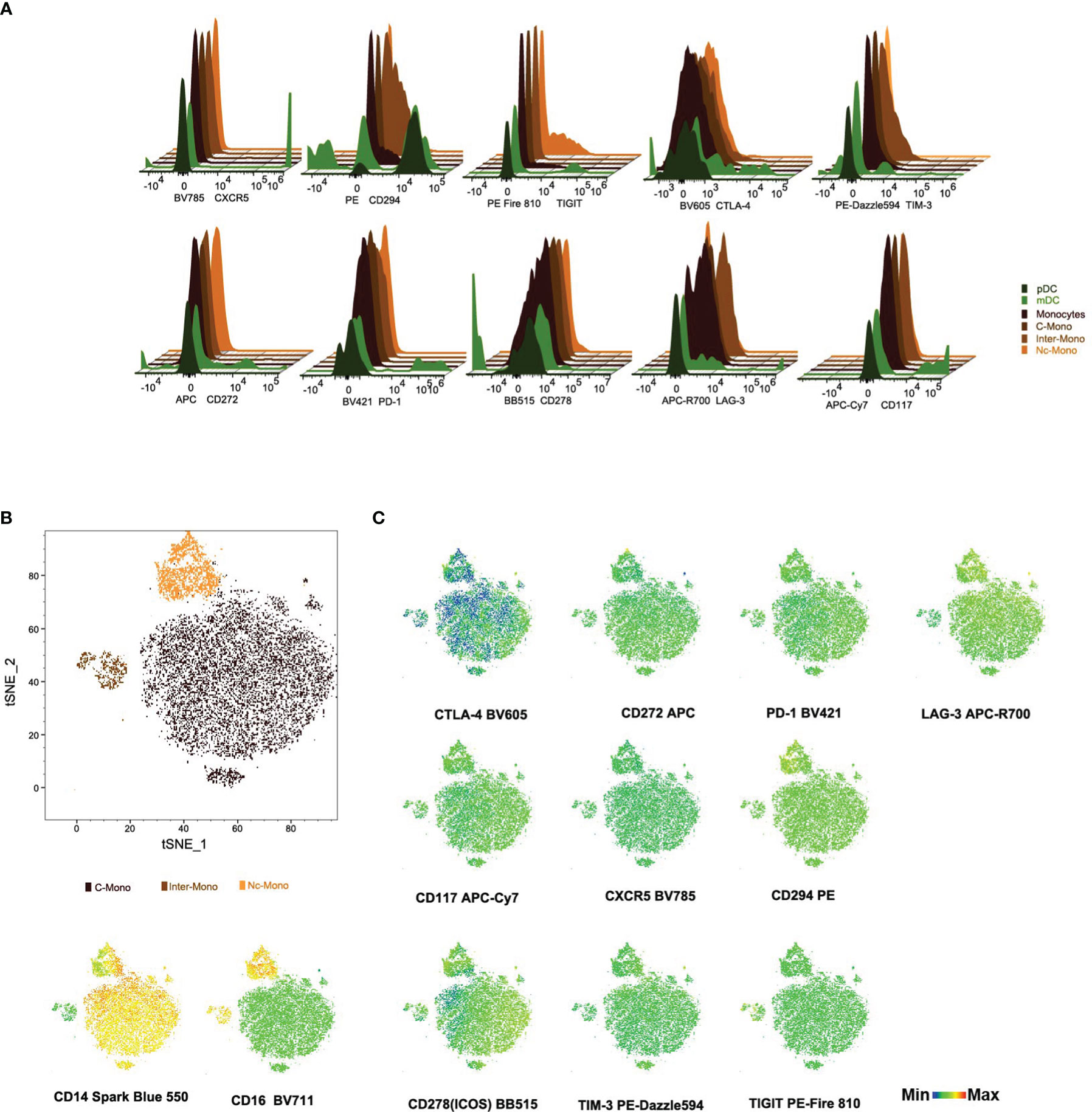
Figure 4 Immune checkpoint molecule profiles on subsets of monocytes and dendritic Cells (A) Histogram plots illustrate checkpoint markers distribution among monocytes and DCs subsets. (B, C) tSNE visualization of checkpoint molecule expression and distribution in monocyte subsets from PBMCs. This figure maps 12 checkpoint molecules and cell counts in PBMC-derived monocytes, with scale bars indicating fluorescence and distribution metrics.
3.5 Hierarchical clustering reveals hierarchy of markers discriminating monocyte subsets
tSNE and FlowSOM algorithms were applied to clustering to categorize monocytes based on 11 lineage markers, resulting in 7 clusters (Figures 5A, B). Subsequently, using CD14, CD16, CD11c, and CD11b, we delineated three monocyte subsets (Figure 5C). Our analysis unveiled distinct expression patterns of investigated markers (CD28, CD27, CD45RA, CD127, CD57, CCR7, CD69, CD161, CD38, and CD25) across classical, non-classical, and intermediate monocyte subsets (Figures 5C, D). Classical monocytes showed high CD28 and CD27 expression, suggesting antigen presentation and T-cell co-stimulation. Non-classical monocytes displayed elevated CD45RA expression and reduced CD127 expression, indicative of a mature and tissue-resident phenotype. CD57 expression was predominantly in intermediate monocytes, suggesting a terminal differentiation. CCR7 expression was weak in classical monocytes, implying similar migratory ability across subpopulations (Figures 5C, D). CD69 expression was moderate in classical and non-classical monocytes but low in intermediates, potentially impacting T-cell differentiation. The absence of CD161 was observed across all monocyte subsets. CD38 was expressed on classical and intermediate monocytes mostly in healthy volunteers, while CD25 was expressed on intermediate monocytes. These findings shed light on monocyte subset diversity and activation in various physiological and pathological contexts.
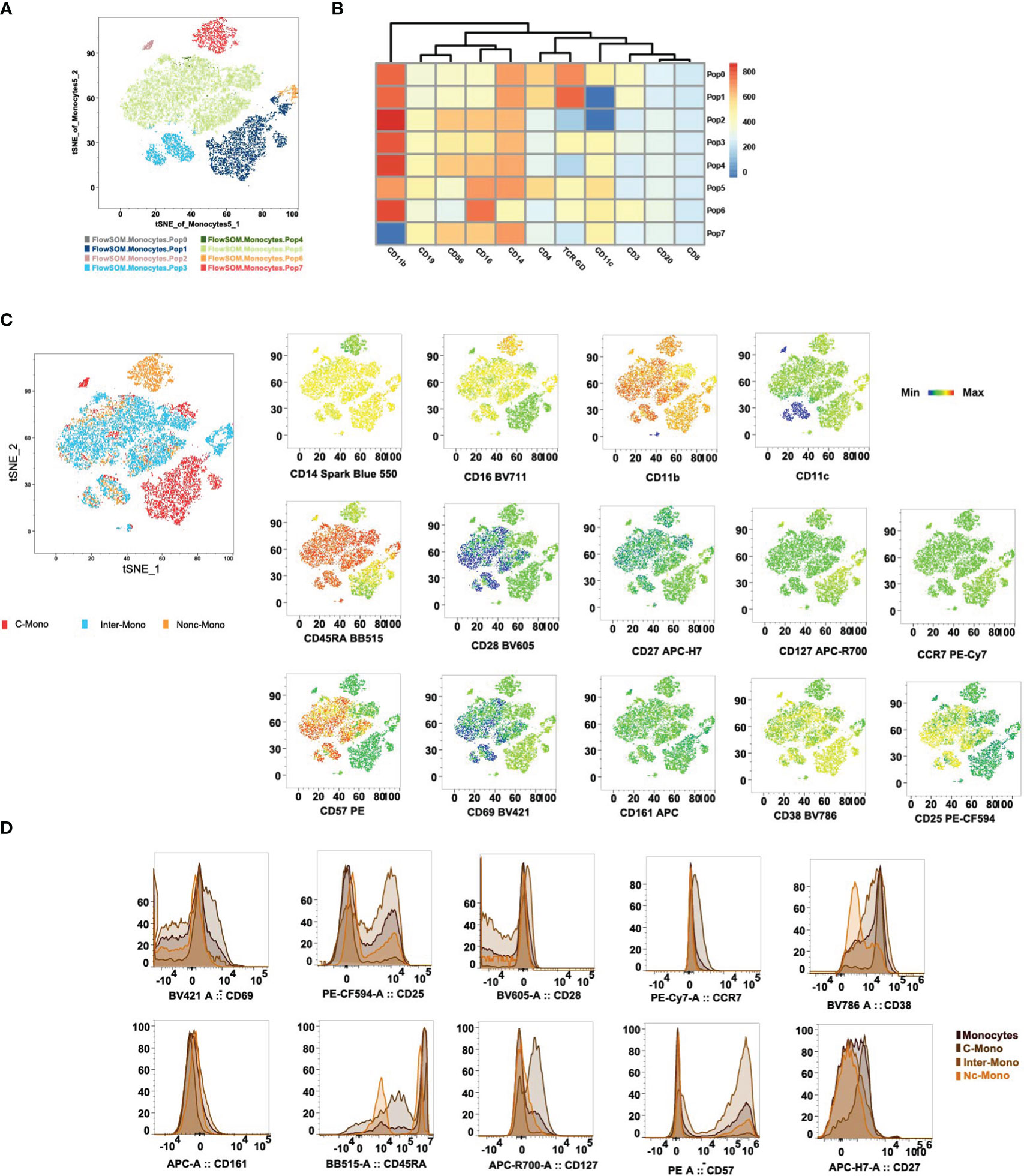
Figure 5 Differential expression of co-stimulatory, activation, and terminal differentiation/exhaustion markers cross monocyte subpopulations. (A) Visualization by tSNE reveals distinct monocyte populations, with the PhenoGraph algorithm identifying 8 unique cell clusters. Each cluster is visualized in a different color. The ClusterExplorer algorithm quantifies cell counts within these PhenoGraph clusters and facilitates the generation of a heatmap to examine protein expression patterns across each identified cluster[36]. (B) tSNE plots delineate the distribution of phenotypic markers among C-Mono, Inter-Mono, and Nc-Mono. (C) Histograms detail the distribution of phenotypic markers within monocyte subsets, highlighting differential expression profiles that distinguish monocyte subsets, shown are monocyte, C-Mono, Inter-Mono, and Nc-Mono. C-Mono, classical monocytes; Inter-Mono, intermediate monocytes; Nc-Mono.
4 Discussion
The critical role of monocytes within the TME presents a nuanced landscape for understanding cancer progression and the efficacy of immunotherapies. Our study’s exploration of monocyte subsets through spectral flow cytometry has revealed significant insights into the complex dynamics at play within the TME. The differential expression profiles of classical and non-classical monocytes, particularly in the context of CMML (8) and MDS (34), underscore the potential and limitation of these cells as both diagnostic biomarkers and therapeutic targets.
In this study, we utilized advanced dimensionality reduction techniques, including FlowSom and t-SNE, to quantify forty key immunological cell surface markers in parallel, enabling precise categorization and role delineation of monocyte subsets across different pathological contexts. The identification of specific surface markers related to co-stimulation, differentiation, and immune checkpoint modulation in monocytes enriches our understanding of their immunoregulatory functions. Notably, the engagement of monocytes in antigen presentation and the modulation of T cell activity highlight their pivotal role in orchestrating anti-tumor immunity. The variation in the expression of markers such as CD25, CD28, and CD127, which indicate potential interactions between monocytes and T cells, could significantly influence T cell activation and lineage specification, ultimately affecting the immune response to tumors (35–38). Recent evidence suggests that reverse signaling involving ICOSL is also important in directing the differentiation of monocyte-derived cells (39). Furthermore, the expression of HLA-DR, CD80, and CD86 underscores monocytes’ capability in antigen processing and presentation, crucial for the potentiation of adaptive immunity (11, 40–42). HLA-DR serves as an important immunological marker in both diagnostic and prognostic settings. For instance, decreased HLA-DR expression levels on monocytes are associated with immunosuppression and can be observed in conditions such as sepsis or after hematopoietic stem cell transplantation (43). Additionally, CD38 and CD161 presence suggests roles in modulating inflammatory responses and NK cell functionalities, respectively (44, 45). The detection of CD127 (IL-7Ra) further points to monocytes’ sensitivity to IL-7 signals, essential for their survival and operational integrity (46). Moreover, CX3CR1 and CXCR5 expressions imply their engagement in site-specific migration and homing, critical for targeted recruitment to inflamed and lymphoid tissues (47, 48). Expression patterns of CD39 and CD73 indicate monocytes’ involvement in adenosine-dependent immune regulation, influencing inflammation resolution and tissue repair mechanisms (13). Moreover, the presence of immune checkpoints on monocytes within the TME, such as PD-1 and LAG-3, points to a complex mechanism of immune evasion employed by tumors. The expression of these checkpoints suggests that monocytes could be manipulated by tumor cells to create an immunosuppressive environment conducive to cancer progression (20). This insight opens new avenues for targeting the monocyte-mediated immunosuppression in cancer therapy, potentially through the use of checkpoint inhibitors designed to reactivate the anti-tumor immune response (17). Additionally, our findings emphasize the importance of the TME in shaping the phenotypic and functional properties of monocytes. The interaction between monocytes and other cells within the TME, including MSCs (9, 10) and tumor cells, further complicates the immune landscape. This interaction, mediated through a network of cytokines, chemokines, and cell surface molecules, could provide critical clues for developing strategies to manipulate the TME in favor of more effective cancer therapies.
Recent studies, including those by Thomas et al. (5) and Hoffmann et al. (49), have advanced our understanding of monocyte heterogeneity by delineating subsets based on surface marker expression. Classical monocytes are distinguished by high expression of CD14, CD36, and CCR2, while intermediate monocytes show elevated HLA-DR along with CD14, CD16, CD11c, and CD36. Non-classical monocytes are primarily identified by CD16 and CD11c, featuring lower HLA-DR levels. Novel markers such as BLTR1, CD35, and CD38 for classical; CD39, CD275, and CD305 for intermediate; and CD29 and CD132 for non-classical monocytes further refine these categorizations. Another study used multiparameter flow cytometry in the evaluation of myelodysplasia, SSCint/CD45hiCD33hi, and/or CD14hi used to gate the monocytes (50).
Our findings corroborate and expand upon this framework. Specifically, classical monocytes exhibit a broad marker profile including CD14, CD64, and notably CD38, which, alongside CD86, is significantly elevated compared to intermediate and non-classical monocytes. This differential expression diminishes as monocytes mature into the non-classical subset, marked by a distinctive downregulation of CD38 and CD86, highlighting their evolving functional capacities. CD69 emerges as a pivotal marker, with moderate expression in classical and non-classical subsets contrasting its notable absence in intermediate monocytes. This variation underscores CD69’s role in immune modulation, from influencing regulatory T cell differentiation via the Jak3/Stat5 pathway (51) to its association with Alzheimer’s disease pathology (52). Additionally, the absence of CCR7 in monocytes disrupts migration and facilitates immunosuppression, leading to conditions such as chronic cutaneous leishmaniasis (53). Further, our analysis reveals a nuanced view of CD161 and CD25 (IL-2R α subunit) expressions. While CD161 is generally absent in healthy monocytes, its presence increases upon immune stimulation, indicating a responsive adaptation to immune challenges (54). CD25, indicative of T cell activation marker (55), shows elevated expressions in intermediate monocytes under health and disease states, linking it to thrombosis and JAK2V617F mutation in myeloproliferative neoplasms (35). The monocyte can be considered a maestro within the TME in both solid tumors and hematologic malignancies, moreover, the applications of checkpoint inhibitors, such as PD-1, CTLA-4, and BTLA, have emerged as a new upsurge of tumor immunotherapy. The new findings can not only detect the evolution of monocytes but also monitor changes in the expression of checkpoints (56–58). Together, these findings illustrate the complex expression patterns of co-stimulatory, activation, and exhaustion markers. Our study highlights how the TME intricately influences monocyte functions, emphasizing the complex interplay between monocytes, MSCs, and tumor cells. Through detailed analysis of co-stimulatory signals and activation markers, we underline monocytes’ crucial roles in antigen presentation and their potential in shaping therapeutic strategies for cancer, autoimmune, and inflammatory diseases. This research enriches our understanding of monocyte biology, paving the way for novel immunotherapeutic interventions.
The variance observed between our findings and previous research stems from the utilization of freshly collected peripheral blood samples, contrasting with the inherent limitations associated with frozen peripheral blood mononuclear cell preparations (5). Our study benefits from the advantage of utilizing fresh, non-cryopreserved samples, allowing for a more accurate representation of cellular phenotypes. By exploring certain surface markers not previously investigated, we offer novel insights into immune function and cellular interactions, enriching our understanding of monocyte biology. Moreover, this study may represent one of the first comprehensive and systematic assessments of immune checkpoint expression across monocyte subsets. Despite minimal differences observed among the three monocyte subsets, our findings enhance our comprehension of monocyte biology and present potential avenues for therapeutic intervention across various disease states, including autoimmune disorders, infectious diseases, and cancer.
However, this research acknowledges several limitations. While we explored diverse immune-relevant cell surface markers on monocytes using spectral flow cytometry, our focus remained primarily on expression patterns and their associations with immune function, without delving deeply into functional implications or disease-specific roles. Therefore, further investigations are warranted to fully characterize monocyte subsets and their interactions in immune regulation and disease pathology. Additionally, comprehensive assessments of immune checkpoint molecules across monocyte subsets in various diseases are imperative to understand their functional implications fully. As the field of monocyte biology continues to evolve rapidly, there is potential for the discovery of additional markers and mechanisms underlying immune regulation, which could further enhance our understanding of immune responses and disease processes.
Data availability statement
The original contributions presented in the study are included in the article/supplementary material. Further inquiries can be directed to the corresponding authors.
Ethics statement
The studies involving humans were approved by the Ethics Committee of the Guangdong Provincial Hospital (Ethical Approval Number: KY-Z-2021-300-02). The studies were conducted in accordance with the local legislation and institutional requirements. The participants provided their written informed consent to participate in this study.
Author contributions
CL: Formal analysis, Visualization, Writing – original draft, Writing – review & editing. MX: Formal analysis, Investigation, Writing – original draft. SG: Data curation, Writing – original draft. YW: Software, Writing – original draft. LZ: Investigation, Writing – original draft. PL: Supervision, Writing – original draft. YG: Conceptualization, Methodology, Writing – review & editing, Writing – original draft. XC: Conceptualization, Project administration, Resources, Supervision, Writing – review & editing, Writing – original draft.
Funding
The author(s) declare financial support was received for the research, authorship, and/or publication of this article. This research was supported by the National Natural Science Foundation of China (No. 82100228, 82270161, 82202978), the Guangzhou Basic and Applied Basic Research Foundation (No.2024A04J10011, 2023A04J2359), and Southern Medical University college students innovation and entrepreneurship training program (S202312121108).
Conflict of interest
The authors declare that the research was conducted in the absence of any commercial or financial relationships that could be construed as a potential conflict of interest.
Publisher’s note
All claims expressed in this article are solely those of the authors and do not necessarily represent those of their affiliated organizations, or those of the publisher, the editors and the reviewers. Any product that may be evaluated in this article, or claim that may be made by its manufacturer, is not guaranteed or endorsed by the publisher.
References
1. Chen X, Li Y, Xia H, Chen YH. Monocytes in tumorigenesis and tumor immunotherapy. Cells. (2023) 12:1673. doi: 10.3390/cells12131673
2. Olingy CE, Dinh HQ, Hedrick CC. Monocyte heterogeneity and functions in cancer. J Leukoc Biol. (2019) 106:309–22. doi: 10.1002/JLB.4RI0818-311R
3. Jakubzick CV, Randolph GJ, Henson PM. Monocyte differentiation and antigen-presenting functions. Nat Rev Immunol. (2017) 17:349–62. doi: 10.1038/nri.2017.28
4. Ziegler-Heitbrock L, Ancuta P, Crowe S, Dalod M, Grau V, Hart DN, et al. Nomenclature of monocytes and dendritic cells in blood. Blood. (2010) 116:e74–80. doi: 10.1182/blood-2010-02-258558
5. Thomas GD, Hamers AAJ, Nakao C, Marcovecchio P, Taylor AM, McSkimming C, et al. Human blood monocyte subsets: A new gating strategy defined using cell surface markers identified by mass cytometry. Arterioscler Thromb Vasc Biol. (2017) 37:1548–58. doi: 10.1161/ATVBAHA.117.309145
6. Ugel S, Cane S, De Sanctis F, Bronte V. Monocytes in the tumor microenvironment. Annu Rev Pathol. (2021) 16:93–122. doi: 10.1146/annurev-pathmechdis-012418-013058
7. Selimoglu-Buet D, Wagner-Ballon O, Saada V, Bardet V, Itzykson R, Bencheikh L, et al. Characteristic repartition of monocyte subsets as a diagnostic signature of chronic myelomonocytic leukemia. Blood. (2015) 125:3618–26. doi: 10.1182/blood-2015-01-620781
8. Hudson CA, Burack WR, Leary PC, Bennett JM. Clinical utility of classical and nonclassical monocyte percentage in the diagnosis of chronic myelomonocytic leukemia. Am J Clin Pathol. (2018) 150:293–302. doi: 10.1093/ajcp/aqy054
9. Najar M, Raicevic G, Fayyad-Kazan H, De Bruyn C, Bron D, Toungouz M, et al. Immune-related antigens, surface molecules and regulatory factors in human-derived mesenchymal stromal cells: the expression and impact of inflammatory priming. Stem Cell Rev Rep. (2012) 8:1188–98. doi: 10.1007/s12015-012-9408-1
10. Stevens HY, Bowles AC, Yeago C, Roy K. Molecular crosstalk between macrophages and mesenchymal stromal cells. Front Cell Dev Biol. (2020) 8:600160. doi: 10.3389/fcell.2020.600160
11. Pinto BF, Medeiros NI, Teixeira-Carvalho A, Eloi-Santos SM, Fontes-Cal TCM, Rocha DA, et al. CD86 expression by monocytes influences an immunomodulatory profile in asymptomatic patients with chronic chagas disease. Front Immunol. (2018) 9:454. doi: 10.3389/fimmu.2018.00454
12. Kowalska W. Expression of CD163 and HLA-DR molecules on the monocytes in chronic lymphocytic leukemia patients. Folia Histochem Cytobiol. (2020) 58:17–24. doi: 10.5603/FHC.a2020.0002
13. Allard B, Longhi MS, Robson SC, Stagg J. The ectonucleotidases CD39 and CD73: Novel checkpoint inhibitor targets. Immunol Rev. (2017) 276:121–44. doi: 10.1111/imr.12528
14. Chen L, Flies DB. Molecular mechanisms of T cell co-stimulation and co-inhibition. Nat Rev Immunol. (2013) 13:227–42. doi: 10.1038/nri3405
15. Guerder S, Flavell RA. Costimulation in tolerance and autoimmunity. Int Rev Immunol. (1995) 13:135–46. doi: 10.3109/08830189509061743
16. Binnewies M, Roberts EW, Kersten K, Chan V, Fearon DF, Merad M, et al. Understanding the tumor immune microenvironment (TIME) for effective therapy. Nat Med. (2018) 24:541–50. doi: 10.1038/s41591-018-0014-x
17. Fendl B, Berghoff AS, Preusser M, Maier B. Macrophage and monocyte subsets as new therapeutic targets in cancer immunotherapy. ESMO Open. (2023) 8:100776. doi: 10.1016/j.esmoop.2022.100776
18. Yun J, Yu G, Hu P, Chao Y, Li X, Chen X, et al. PD-1 expression is elevated in monocytes from hepatocellular carcinoma patients and contributes to CD8 T cell suppression. Immunol Res. (2020) 68:436–44. doi: 10.1007/s12026-020-09155-3
19. Zhao P, Peng C, Chang X, Cheng W, Yang Y, Shen Y, et al. Decreased expression of TIGIT on CD14 + monocytes correlates with clinical features and laboratory parameters of patients with primary Sjogren's syndrome. Clin Rheumatol. (2024) 43:297–306. doi: 10.1007/s10067-023-06759-6
20. Versluis JM, Long GV, Blank CU. Learning from clinical trials of neoadjuvant checkpoint blockade. Nat Med. (2020) 26:475–84. doi: 10.1038/s41591-020-0829-0
21. Halvorsen B, Smedbakken LM, Michelsen AE, Skjelland M, Bjerkeli V, Sagen EL, et al. Activated platelets promote increased monocyte expression of CXCR5 through prostaglandin E2-related mechanisms and enhance the anti-inflammatory effects of CXCL13. Atherosclerosis. (2014) 234:352–9. doi: 10.1016/j.atherosclerosis.2014.03.021
22. Van Gassen S, Callebaut B, Van Helden MJ, Lambrecht BN, Demeester P, Dhaene T, et al. FlowSOM: Using self-organizing maps for visualization and interpretation of cytometry data. Cytometry A. (2015) 87:636–45. doi: 10.1002/cyto.a.22625
23. Liang KL, Laurenti E, Taghon T. Circulating IRF8-expressing CD123(+)CD127(+) lymphoid progenitors: key players in human hematopoiesis. Trends Immunol. (2023) 44:678–92. doi: 10.1016/j.it.2023.07.004
24. Wong J, Roy SF, McNiff JM, Xu ML. IRF8 in conjunction with CD123 and CD20 to distinguish lupus erythematosus panniculitis from subcutaneous panniculitis-like T-cell lymphoma. Am J Surg Pathol. (2023) 47:1425–31. doi: 10.1097/PAS.0000000000002133
25. Hao JW, Wang J, Guo H, Zhao YY, Sun HH, Li YF, et al. CD36 facilitates fatty acid uptake by dynamic palmitoylation-regulated endocytosis. Nat Commun. (2020) 11:4765. doi: 10.1038/s41467-020-18565-8
26. Lee JG, Jaeger KE, Seki Y, Wei Lim Y, Cunha C, Vuchkovska A, et al. Human CD36(hi) monocytes induce Foxp3(+) CD25(+) T cells with regulatory functions from CD4 and CD8 subsets. Immunology. (2021) 163:293–309. doi: 10.1111/imm.13316
27. Su X, Jin W, Liu L, Zhu Z, Li C. Regulation of CD47 expression on CD14(+) monocytes by interferon-alpha in PBC patients. Front Immunol. (2023) 14:1256995. doi: 10.3389/fimmu.2023.1256995
28. Park JK, Lee YJ, Park JS, Lee EB, Song YW. CD47 potentiates inflammatory response in systemic lupus erythematosus. Cells. (2021) 10:1151. doi: 10.3390/cells10051151
29. Nguyen CTH, Kambe N, Yamazaki F, Ueda-Hayakawa I, Kishimoto I, Okamoto H. Up-regulated expression of CD86 on circulating intermediate monocytes correlated with disease severity in psoriasis. J Dermatol Sci. (2018) 90:135–43. doi: 10.1016/j.jdermsci.2018.01.005
30. Hou J, Zhang L, Wu H, Gao P, Xu Z. Increased Tim-3(+) monocytes/macrophages are associated with disease severity in patients with IgA nephropathy. Int Immunopharmacol. (2021) 97:107666. doi: 10.1016/j.intimp.2021.107666
31. Yang P, Wu Q, Sun L, Fang P, Liu L, Ji Y, et al. Adaptive immune response signaling is suppressed in Ly6C(high) monocyte but upregulated in monocyte subsets of ApoE(-/-) mice - functional implication in atherosclerosis. Front Immunol. (2021) 12:809208. doi: 10.3389/fimmu.2021.809208
32. Wong KL, Tai JJ, Wong WC, Han H, Sem X, Yeap WH, et al. Gene expression profiling reveals the defining features of the classical, intermediate, and nonclassical human monocyte subsets. Blood. (2011) 118:e16–31. doi: 10.1182/blood-2010-12-326355
33. Ohshima S, Mori S, Shigenari A, Miyamoto A, Takasu M, Imaeda N, et al. Differentiation ability of multipotent hematopoietic stem/progenitor cells detected by a porcine specific anti-CD117 monoclonal antibody. Biosci Trends. (2014) 8:308–15. doi: 10.5582/bst.2014.01084
34. Talati C, Zhang L, Shaheen G, Kuykendall A, Ball M, Zhang Q, et al. Monocyte subset analysis accurately distinguishes CMML from MDS and is associated with a favorable MDS prognosis. Blood. (2017) 129:1881–3. doi: 10.1182/blood-2016-12-753210
35. Goette NP, Lev PR, Heller PG, Kornblihtt LI, Korin L, Molinas FC, et al. Monocyte IL-2Ralpha expression is associated with thrombosis and the JAK2V617F mutation in myeloproliferative neoplasms. Cytokine. (2010) 51:67–72. doi: 10.1016/j.cyto.2010.04.011
36. Charron L, Doctrinal A, Ni Choileain S, Astier AL. Monocyte:T-cell interaction regulates human T-cell activation through a CD28/CD46 crosstalk. Immunol Cell Biol. (2015) 93:796–803. doi: 10.1038/icb.2015.42
37. Estrada-Capetillo L, Aragoneses-Fenoll L, Dominguez-Soto A, Fuentelsaz-Romero S, Nieto C, Simon-Fuentes M, et al. CD28 is expressed by macrophages with anti-inflammatory potential and limits their T-cell activating capacity. Eur J Immunol. (2021) 51:824–34. doi: 10.1002/eji.202048806
38. Zhang B, Zhang Y, Xiong L, Li Y, Zhang Y, Zhao J, et al. CD127 imprints functional heterogeneity to diversify monocyte responses in inflammatory diseases. J Exp Med. (2022) 219:e20211191. doi: 10.1084/jem.20211191
39. Provera A, Ramavath NN, Gadipudi LL, Gigliotti CL, Boggio E, Vecchio C, et al. Role of the co-stimulatory molecule inducible T-cell co-stimulator ligand (ICOSL) in the progression of experimental metabolic dysfunction-associated steatohepatitis. Front Immunol. (2023) 14:1290391. doi: 10.3389/fimmu.2023.1290391
40. Tampe D, Hakroush S, Biggemann L, Winkler MS, Tampe B. Kinetics of human leukocyte antigen receptor HLA-DR(+) monocytes and T lymphocytes during remission induction therapy in ANCA-associated vasculitis. J Nephrol. (2022) 35:1283–7. doi: 10.1007/s40620-022-01330-z
41. Joshi I, Carney WP, Rock EP. Utility of monocyte HLA-DR and rationale for therapeutic GM-CSF in sepsis immunoparalysis. Front Immunol. (2023) 14:1130214. doi: 10.3389/fimmu.2023.1130214
42. Holmannova D, Kolackova M, Kunes P, Krejsek J, Mandak J, Andrys C. Impact of cardiac surgery on the expression of CD40, CD80, CD86 and HLA-DR on B cells and monocytes. Perfusion. (2016) 31:391–400. doi: 10.1177/0267659115612905
43. Döring M, Haufe S, Erbacher A, Müller I, Handgretinger R, Hofbeck M, et al. Surface HLA-DR expression in monocyte subpopulations during adverse events after hematopoietic stem cell transplantation. Blood. (2011) 118:2161–1. doi: 10.1182/blood.V118.21.2161.2161
44. Zilber MT, Gregory S, Mallone R, Deaglio S, Malavasi F, Charron D, et al. CD38 expressed on human monocytes: a coaccessory molecule in the superantigen-induced proliferation. Proc Natl Acad Sci U.S.A. (2000) 97:2840–5. doi: 10.1073/pnas.050583197
45. Steiniger B, Stehling O, Scriba A, Grau V. Monocytes in the rat: phenotype and function during acute allograft rejection. Immunol Rev. (2001) 184:38–44. doi: 10.1034/j.1600-065x.2001.1840104.x
46. Hehenkamp P, Hoffmann M, Kummer S, Reinauer C, Doing C, Fortsch K, et al. Interleukin-7-dependent nonclassical monocytes and CD40 expression are affected in children with type 1 diabetes. Eur J Immunol. (2021) 51:3214–27. doi: 10.1002/eji.202149229
47. Oggero S, Cecconello C, Silva R, Zeboudj L, Sideris-Lampretsas G, Perretti M, et al. Dorsal root ganglia CX3CR1 expressing monocytes/macrophages contribute to arthritis pain. Brain Behav Immun. (2022) 106:289–306. doi: 10.1016/j.bbi.2022.09.008
48. Gonzalez-Serna D, Shi C, Kerick M, Hankinson J, Ding J, McGovern A, et al. Identification of mechanisms by which genetic susceptibility loci influence systemic sclerosis risk using functional genomics in primary T cells and monocytes. Arthritis Rheumatol. (2023) 75:1007–20. doi: 10.1002/art.42396
49. Hoffmann J, Fiser K, Liebetrau C, Staubach N, Kost D, Voss S, et al. High-content immunophenotyping and hierarchical clustering reveal sources of heterogeneity and new surface markers of human blood monocyte subsets. Thromb Haemost. (2020) 120:141–55. doi: 10.1055/s-0039-1700871
50. Porwit A, Bene MC, Duetz C, Matarraz S, Oelschlaegel U, Westers TM, et al. Multiparameter flow cytometry in the evaluation of myelodysplasia: Analytical issues: Recommendations from the European LeukemiaNet/International Myelodysplastic Syndrome Flow Cytometry Working Group. Cytometry B Clin Cytom. (2023) 104:27–50. doi: 10.1002/cyto.b.22108
51. Martin P, Gomez M, Lamana A, Cruz-Adalia A, Ramirez-Huesca M, Ursa MA, et al. CD69 association with Jak3/Stat5 proteins regulates Th17 cell differentiation. Mol Cell Biol. (2010) 30:4877–89. doi: 10.1128/MCB.00456-10
52. Kusdra L, Rempel H, Yaffe K, Pulliam L. Elevation of CD69+ monocyte/macrophages in patients with Alzheimer's disease. Immunobiology. (2000) 202:26–33. doi: 10.1016/S0171-2985(00)80049-2
53. Kling JC, Mack M, Korner H. The absence of CCR7 results in dysregulated monocyte migration and immunosuppression facilitating chronic cutaneous leishmaniasis. PloS One. (2013) 8:e79098. doi: 10.1371/journal.pone.0079098
54. Chalan P, Bijzet J, Huitema MG, Kroesen BJ, Brouwer E, Boots AM. Expression of lectin-like transcript 1, the ligand for CD161, in rheumatoid arthritis. PloS One. (2015) 10:e0132436. doi: 10.1371/journal.pone.0132436
55. Dutton RW, Bradley LM, Swain SL. T cell memory. Annu Rev Immunol. (1998) 16:201–23. doi: 10.1146/annurev.immunol.16.1.201
56. Amer HT, Stein U, El Tayebi HM. The monocyte, a maestro in the tumor microenvironment (TME) of breast cancer. Cancers (Basel). (2022) 14:5460. doi: 10.3390/cancers14215460
57. Zannoni J, Mauz N, Seyve L, Meunier M, Pernet-Gallay K, Brault J, et al. Tumor microenvironment and clonal monocytes from chronic myelomonocytic leukemia induce a procoagulant climate. Blood Adv. (2019) 3:1868–80. doi: 10.1182/bloodadvances.2018026955
Keywords: spectral flow cytometry, monocytes, tumor microenvironment (TME), immunophenotyping, immune checkpoints, t-distributed stochastic neighbor embedding (tSNE) analysis, mesenchymal stromal cells (MSCs)
Citation: Li C, Xiao M, Geng S, Wang Y, Zeng L, Lai P, Gong Y and Chen X (2024) Comprehensive analysis of human monocyte subsets using full-spectrum flow cytometry and hierarchical marker clustering. Front. Immunol. 15:1405249. doi: 10.3389/fimmu.2024.1405249
Received: 22 March 2024; Accepted: 16 April 2024;
Published: 29 April 2024.
Edited by:
Haiyang Wu, Tianjin Medical University, ChinaReviewed by:
Xiaojun Li, Gannan Medical University, ChinaFu Xiaoying, Shenzhen Children’s Hospital, China
Ziyi Chen, University of North Carolina at Chapel Hill, United States
Copyright © 2024 Li, Xiao, Geng, Wang, Zeng, Lai, Gong and Chen. This is an open-access article distributed under the terms of the Creative Commons Attribution License (CC BY). The use, distribution or reproduction in other forums is permitted, provided the original author(s) and the copyright owner(s) are credited and that the original publication in this journal is cited, in accordance with accepted academic practice. No use, distribution or reproduction is permitted which does not comply with these terms.
*Correspondence: Xiaomei Chen, chenxiaomei@gdph.org.cn; Ying Gong, gongy3@mail2.sysu.edu.cn; Peilong Lai, lai_peilong@163.com
†These authors have contributed equally to this work