- 1Department of Surgery, Medical Faculty Mannheim, University Medical Center Mannheim, Heidelberg University, Mannheim, Germany
- 2Department of Dermatology, Venereology and Allergology, University Medical Center Mannheim, Heidelberg University, Mannheim, Germany
- 3Skin Cancer Unit, German Cancer Research Center (DKFZ), Heidelberg, Germany
- 4German Cancer Research Center (DKFZ)-Hector Cancer Institute, University Medical Centre Mannheim, Mannheim, Germany
- 5Department of Biometry and Statistics, Medical Faculty Mannheim, University Medical Center Mannheim, Heidelberg University, Mannheim, Germany
- 6Department of Library and Information Sciences, Medical Faculty Mannheim, Heidelberg University, Mannheim, Germany
Background: Immunotherapeutic approaches, including immune checkpoint inhibitor (ICI) therapy, are increasingly recognized for their potential. Despite notable successes, patient responses to these treatments vary significantly. The absence of reliable predictive and prognostic biomarkers hampers the ability to foresee outcomes. This meta-analysis aims to evaluate the predictive significance of circulating myeloid-derived suppressor cells (MDSC) in patients with solid tumors undergoing ICI therapy, focusing on progression-free survival (PFS) and overall survival (OS).
Methods: A comprehensive literature search was performed across PubMed and EMBASE from January 2007 to November 2023, utilizing keywords related to MDSC and ICI. We extracted hazard ratios (HRs) and 95% confidence intervals (CIs) directly from the publications or calculated them based on the reported data. A hazard ratio greater than 1 indicated a beneficial effect of low MDSC levels. We assessed heterogeneity and effect size through subgroup analyses.
Results: Our search yielded 4,023 articles, of which 17 studies involving 1,035 patients were included. The analysis revealed that patients with lower levels of circulating MDSC experienced significantly improved OS (HR=2.13 [95% CI 1.51–2.99]) and PFS (HR=1.87 [95% CI 1.29–2.72]) in response to ICI therapy. Notably, heterogeneity across these outcomes was primarily attributed to differences in polymorphonuclear MDSC (PMN-MDSC) subpopulations and varying cutoff methodologies used in the studies. The monocytic MDSC (M-MDSC) subpopulation emerged as a consistent and significant prognostic marker across various subgroup analyses, including ethnicity, tumor type, ICI target, sample size, and cutoff methodology.
Conclusions: Our findings suggest that standardized assessment of MDSC, particularly M-MDSC, should be integral to ICI therapy strategies. These cells hold the promise of identifying patients at risk of poor response to ICI therapy, enabling tailored treatment approaches. Further research focusing on the standardization of markers and validation of cutoff methods is crucial for integrating MDSC into clinical practice.
Systematic Review Registration: https://www.crd.york.ac.uk/prospero/display_record.php?ID=CRD42023420095, identifier CRD42023420095.
1 Introduction
Despite modern therapies, cancer is still one of the most common causes of death in industrialized countries. For example, in 2019, solid tumors such as tracheobronchial lung cancer, prostate and colon cancer were among the leading causes of death worldwide from cancer in men while it was breast, colon cancer and tracheobronchial lungs in women (1). The approval of immune checkpoint inhibitor (ICI) treatments, by the Food and Drug Administration in 2011provides alternative therapies to the standard chemotherapy regimens, particularly for the treatment of solid tumor malignancies It has been shown that T cells become anergic in cancer patients due to the interaction of programmed death -1 (PD-1) or cytotoxic-T-lymphocyte-associated protein-4 (CTLA-4) upregulated on activated T cells with their ligands PD-L1 and CD80 or CD86 respectively. Blocking this interaction could result in regaining anti-tumor T cell functions (2, 3).
With the introduction of Ipilimumab in the treatment of malignant melanoma, the median overall survival was increased from 6.4 months to 10 months compared to the control group (4). The survival curve in a cohort of patients with non-resectable malignant melanoma treated with ipilimumab reached a plateau between 20–26% after three years, indicating a long-term response (5). Another breakthrough was found in the treatment of non-small-cell lung carcinoma. Here, recent studies have shown that immunotherapy combined with chemotherapy already shows a survival advantage in the first line therapy compared to the single chemotherapy (median overall survival after 12 months: 69.2% in the combination group and 49.4% in the chemotherapy group) (6).
Despite these advancements, a subset of patients either fails to respond initially or loses responsiveness over time to such therapies. The search for explanations has increasingly focused on immunosuppressive mechanisms, including the role of myeloid-derived suppressor cells (MDSC). Studies have indicated an inverse relationship between the prognosis of solid tumor patients and the presence of immunosuppressive cell types such as MDSC and regulatory T-cells (Treg) within the tumor microenvironment (TME) and peripheral blood (7–9).
MDSCs represent a heterogeneous population of myeloid cells known for their immunosuppressive activities. They originate from immature myeloid cells that fail to differentiate under chronic inflammatory conditions, such as cancer (10, 11). In addition, normal mature myeloid cells could be converted into MDSC in cancer patients (12, 13). MDSCs are categorized into two subpopulations based on their phenotypic characteristics: monocytic MDSCs (M-MDSCs) and polymorphonuclear MDSCs (PMN-MDSCs). M-MDSCs are identified by the expression of surface markers CD11b+CD14+HLA-DRlow/-CD15-, with CD33 also serving as an alternative marker to CD11b. This subgroup is morphologically comparable to monocytes. On the other hand, PMN-MDSCs, which express CD11b+CD14-CD15+(CD66 as an alternative to CD15) markers, are morphologically akin to neutrophils (10, 11).
The discovery of Lectin-type oxidized LDL receptor 1 (LOX-1) as a specific ligand has refined the identification and separation of these cell types, facilitating a more accurate characterization and understanding of their roles within the tumor microenvironment (TME) and systemic circulation (14). A standardized gating strategy to identify M-MDSCs, based on common morphological criteria such as CD14+ and HLA-DR expression, has been established recently, noting that functional examination of the immunosuppressive properties of MDSCs is the safest way to identify them (10). This advancement in methodology has been crucial for consistent and reproducible analysis of MDSC populations across various studies.
The suppressive mechanisms of MDSC include inhibiting T cells and other components of immune systems to facilitate tumor growth and survival (11). One of the major mechanisms of MDSC-mediated immunosuppression is linked to the upregulation of programmed cell death ligand 1 (PD-L1) interacting with its receptor PD-1 expressed on tumor-infiltrating T cells (10, 15),
They are also capable to inhibit anti-tumor T cell functions via production of nitric oxide (NO) and reactive oxygen species (ROS) as well as by upregulation of arginase 1 and Indolamin-2,3-Dioxygenase (11, 16–19).
They also interact synergistically with regulatory T cells (Tregs), promoting their expansion within the TME via the CD40 receptor (20). This interaction highlights the complex network of immunosuppressive pathways that contribute to tumor growth and survival.
Given these extensive immunosuppressive capabilities, MDSC subpopulations represent potential biomarkers for predicting patient outcomes, including responses to immunotherapies (11, 21–23). Furthermore, the recruitment of MDSCs from the bone marrow to the TME, driven by various cytokines (24) suggests that an early increase in circulating MDSC levels could serve as a negative prognostic indicator.
Despite existing research demonstrating a correlation between high levels of MDSCs in peripheral blood and adverse outcomes in solid tumors (9), the specific impact of MDSCs on the efficacy of immune checkpoint inhibitor (ICI) therapy remains underexplored. To date, no systematic review or meta-analysis has separately assessed the predictive value of circulating MDSCs on the response to ICI therapy in patients with solid tumors. This gap in the literature underscores the need for a comprehensive analysis that can elucidate the influence of circulating MDSC populations on overall survival and therapeutic response, thereby informing clinical decision-making and potentially guiding the development of more effective treatment strategies. The goal of this meta-analysis is to address this need by examining the relationship between MDSC levels in peripheral blood and patient outcomes in the context of ICI therapy.
2 Methods
This study was conceived as a meta-analysis to investigate whether elevated levels MDSCs and their subpopulations in peripheral blood serve as predictive markers for the response to immune checkpoint inhibitor (ICI) therapy or survival outcomes in patients with solid tumors. The PICO scheme for our research question was defined as:
● Population. Patients with solid tumors treated with ICI
● Intervention: measurement of MDSC in patients’ peripheral blood by flow cytometry
● Comparison: high concentrations of MDSC compared to low concentrations of MDSC
● Outcome: Progression-free survival and overall survival
The study protocol was prospectively registered with PROSPERO (registration number CRD42023420095), adhering to the Preferred Reporting Items for Systematic Reviews and Meta-Analysis Protocols 2020 (PRISMA-P) guidelines, which underpin both the protocol and the manuscript structure (see Supplementary Table 2 for details).
2.1 Search strategy
The literature search was conducted in the PubMed and EMBASE databases from January 2007 to November 2023, utilizing the PubMed and EMBASE databases. A detailed search strategy was developed in collaboration with a medical librarian, incorporating terms and synonyms related to immune checkpoint inhibitors and “myeloid-derived suppressor cells” [MeSH], utilizing both OR and AND Boolean operators for term combination. Additionally, Google Scholar was employed to identify grey literature, and the reference lists of relevant articles were reviewed to uncover further studies.
2.2 Inclusion criteria
Inclusion criteria mandated that studies: (1) were prospective or retrospective cohort studies, clinical trials, or randomized controlled trials; (2) included patients diagnosed with solid neoplasms; (3) involved treatment with an immune checkpoint inhibitor; (4) measured MDSC levels in peripheral blood at a minimum of two time points, one of which must be prior to therapy initiation; (5) used cutoff values for MDSC levels to stratify patients; and (6) performed a correlation analysis with survival or other outcome parameters, including either (7) hazard ratios with 95% confidence intervals or provided sufficient data for their calculation.
2.3 Exclusion criteria
Excluded were studies that: (1) were reviews, case reports, animal studies, or in vitro studies; (2) did not measure MDSC levels using flow cytometry or measured them peritumorally or directly within tumor tissues; (3) targeted MDSCs directly as a therapeutic intervention; (4) provided insufficient data for hazard ratio calculations.
The eligible studies were screened in full text by two authors (MM, VO) with discrepancies resolved via a third author (SS).
2.4 Data extraction
The data were collected by both authors independently in a data matrix that included the first author, year of publication, country of origin, number of patients and age (median and/or range) of the study population, tumor type and stage, type of therapy (immune checkpoint inhibitor with target) MDSC subpopulation, MDSC markers, cutoff values, and method of cutoff value determination, observed endpoints. We extracted hazard ratios with 95% confidence intervals for the endpoints overall survival (OS), progression free survival (PFS), disease free survival (DFS). If these were not specified, we calculated the hazard ratios according to method of Tierney (25) by estimating the necessary data from the Kaplan Meier curves. Alternatively, we extracted the HR from other sources if the data were already calculated there.
2.5 Risk of bias assessment
Using the QUIPS tool, one author (MM) assessed the risk of bias of the included studies. A second author (VO) independently reviewed the assessment. Disagreements were resolved by a third author (SS). The tool contains six categories of bias due to study participation, study attrition, prognostic factor measurement, outcome measurement, adjustment for other prognostic factors and bias due to statistical analysis and reporting. In each category, the authors could choose between low, moderate and high risk of bias (26).
2.6 Statistical analysis
For the statistical analysis, we used RevMan 5.4 (Review Manager Version 5.4. The Cochrane Collaboration, 2020) and Comprehensive Meta-Analysis software Version 4 (Biostat, Englewood, NJ 2022). We weighted them according to the generic inverse variance method. A hazard ratio >1 defined a preference for a low MDSC level at baseline. To detect heterogeneity, we used a χ² test and the I² value, which were considered significant if the χ² test assumed a value of P<0.1 or I²>50% (27). Subgroup and sensitivity analyses further explored heterogeneity, while publication bias was assessed visually with a funnel plot and quantitatively via the Egger test. The Duval and Tweedie’s trim-and-fill method was applied in cases of detected asymmetry, with a significance threshold set at P<0.05 (28). If the heterogeneity was significant, we used the random-effects model; otherwise, we used the fixed-effects model.
3 Results
3.1 Study characteristics
In our comprehensive search across three databases (PubMed, EMBASE, Google Scholar), we initially identified 4,023 articles. Upon removal of 1,197 duplicates, 2,731 articles remained for consideration. The initial screening of titles and abstracts facilitated the exclusion of 2,650 articles deemed not relevant to our research objectives. Further detailed examination of the full texts led to the exclusion of additional articles for various reasons: 12 articles were excluded due to lack of stratification of MDSC levels into high or low categories; 5 articles were omitted because they failed to collect baseline data; 7 articles were excluded for not incorporating immune checkpoint inhibitor (ICI) therapy; 5 articles were disregarded due to insufficient data for calculating Hazard ratios; 3 articles were eliminated because they did not measure MDSC in peripheral blood; and 32 articles were excluded for other reasons or because they did not align with the study’s focus. Ultimately, 17 studies (29–45) were selected for inclusion in our meta-analysis, as illustrated in the PRISMA flow diagram (Figure 1).
These 17 studies collectively encompassed 1,035 patients, with the majority being melanoma cases (10 studies involving 720 patients). The next most significant group was patients with non-small cell lung cancer (NSCLC), represented by 207 patients across 3 studies. Other cancer types included in the analysis were prostate cancer (2 studies with 45 patients) and urothelial carcinoma (1 study with 30 patients), along with a study that pooled data from patients with various solid tumors (33 patients across 11 entities). Regarding ICI specificity, 8 studies targeted PD-1/PD-L1 exclusively, involving 428 patients; 5 studies focused solely on CTLA-4, including 359 patients; and 4 studies, comprising 248 patients, pooled effect measurements for patients treated with either PD-1/PD-L1 or CTLA-4 antibodies.
A more detailed breakdown of these studies is provided in Table 1. Out of the included studies, 15 reported data on OS, and 9 included PFS data. The analysis of MDSC subtypes revealed that 2 studies examined total MDSC levels, 2 focused exclusively on PMN-MDSC, and 7 investigated monocytic MDSC (M-MDSC). Additionally, 6 studies presented data relevant to both PMN-MDSC and M-MDSC subgroups, offering a comprehensive overview of the impact of these immune cells on patient outcomes in the context of ICI therapy.
3.2 Progression-free survival
A high baseline MDSC value indicated a poorer response to ICI, according to PFS. The HR was 1.87 [95%CI 1.29–2.72] with an I2 of 79%. Here, however, only the M-MDSC achieved a significant result (HR= 2.03 [95% CI 1.42–2.90]) with a moderate heterogeneity I2 = 34% %. The other three populations were not significant (PMN MDSC HR=1.68 [95% CI 0.94–3.01] I2 = 78%); total MDSC HR=2.21 (95%CI [0.68–7.16] I2 = 48%). Due to the overall high heterogeneity, the HRs were also calculated here using a random effects model (Figure 2).
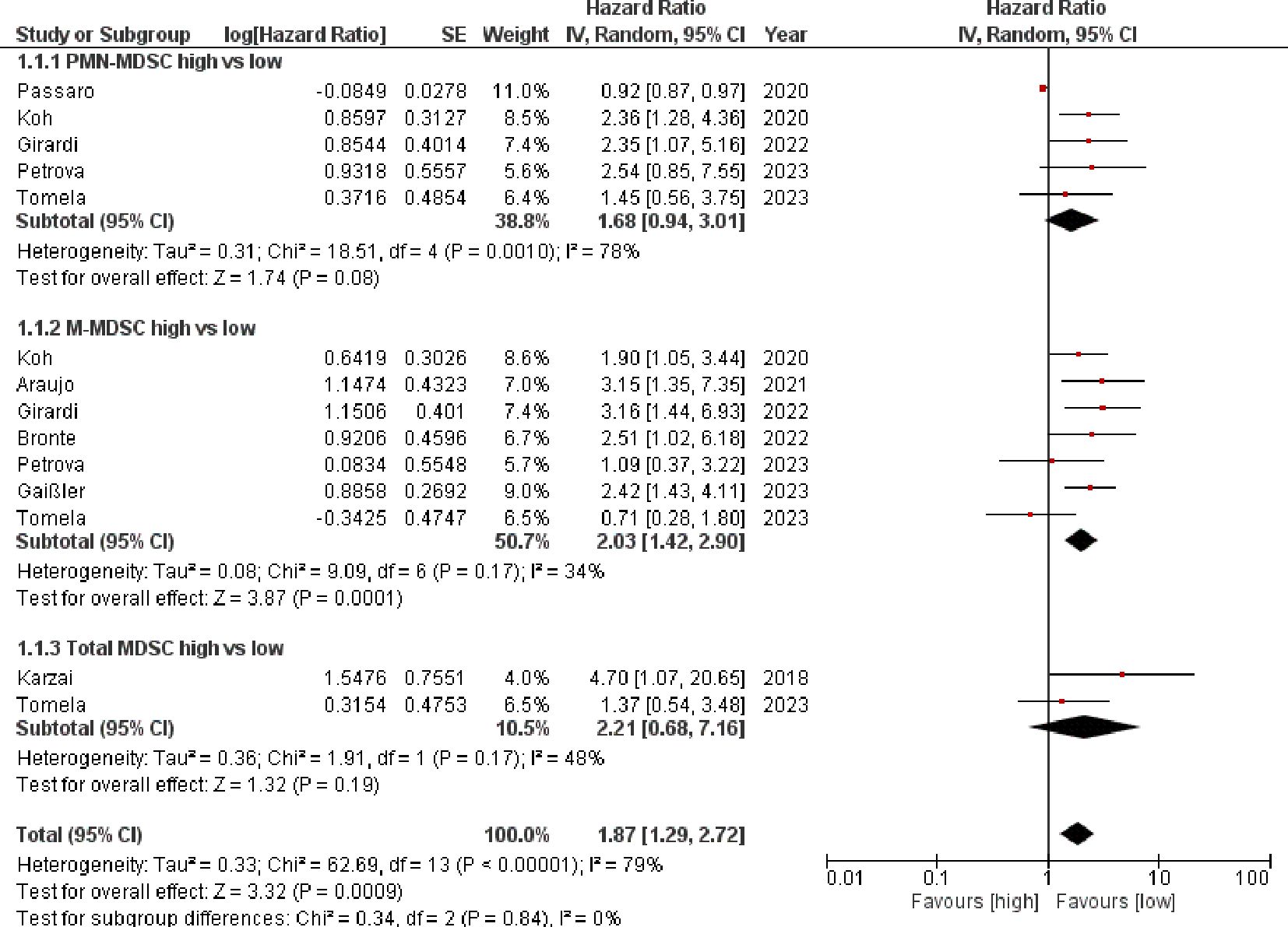
Figure 2 Forest plot illustrating the impact of myeloid-derived suppressor cell levels on progression-free survival in patients receiving immune checkpoint inhibitor therapy.
3.3 Overall survival
OS was lower with a high MDSC baseline value. This resulted in an HR=2.13 [95% CI 1.51–2.99] for OS after pooling the studies. The heterogeneity amounted to I2 = 82%. However, only the M-MDSC reached a significant level. We calculated an HR=2.45 [95% CI 1.89–3.18] I2 = 23%) for the M-MDSC. For the PMN-MDSC population, the HR was 1.47 [95%CI 0.90–2.42]. This resulted in I2 = 68%. Overall, the heterogeneity was relatively high, which is why the HR was calculated using the random effects model (Figure 3).
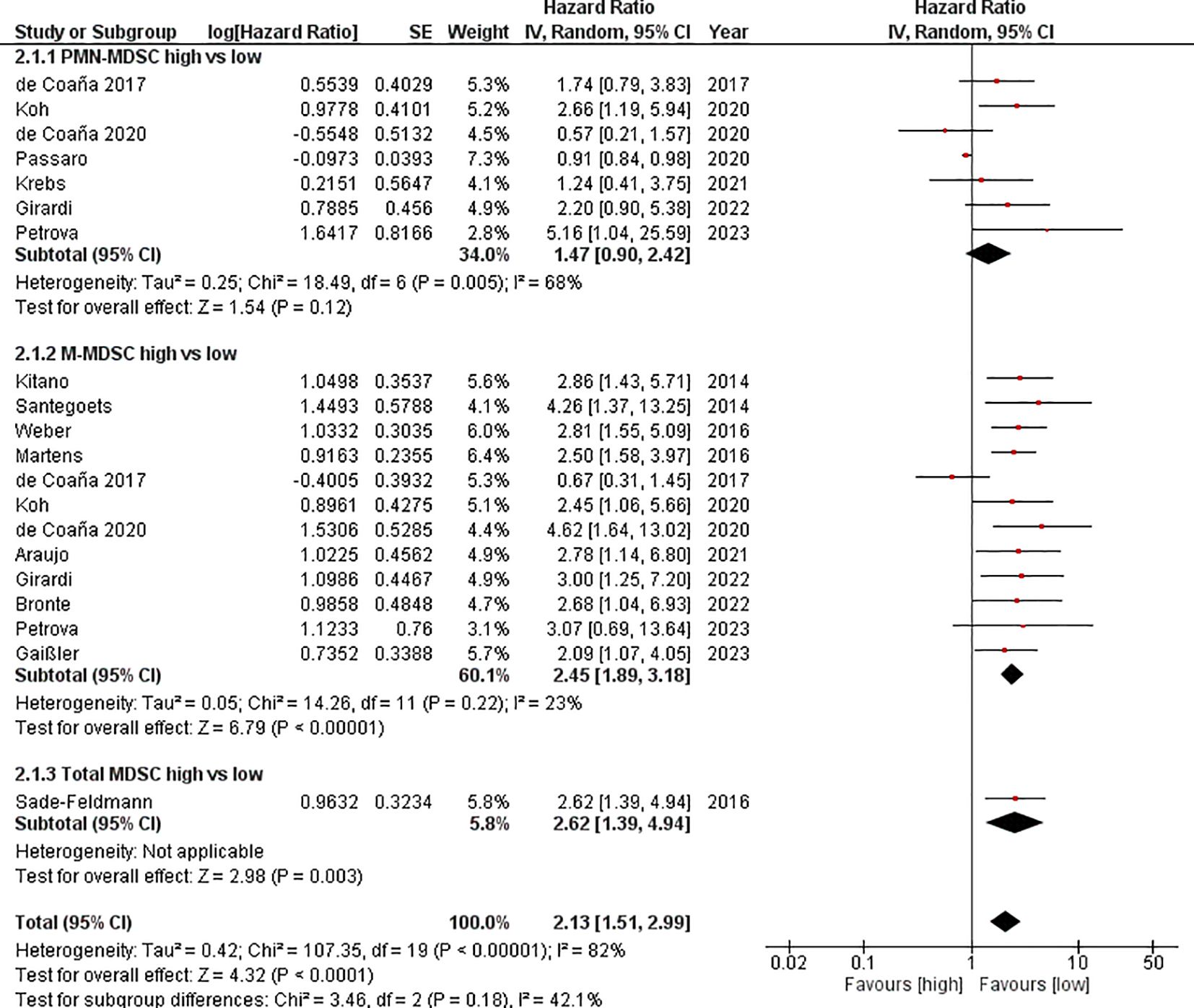
Figure 3 Forest plot illustrating the impact of myeloid-derived suppressor cell levels on overall survival in patients receiving immune checkpoint inhibitor therapy.
3.4 Subgroup analysis
To delve deeper into the heterogeneity observed within our meta-analysis, we conducted detailed subgroup analyses focusing on variables such as geographic region, patient cohort size, type of cancer, stage of cancer, method and value used for cutoff determination, and the specific immune checkpoint target (PD-1/PD-L1 versus CTLA-4). Stratification for the number of patients and cutoff values was based on the median values within each respective group. The comprehensive findings of these subgroup analyses are presented in Supplementary Table 1. A reduction in heterogeneity was observed when studies utilized consistent methods for determining cutoff values, highlighting this as a significant factor in our analysis. The type of cancer entity did not impact heterogeneity. Predominantly, PMN-MDSC emerged as a primary source of heterogeneity, both in our primary and subgroup analyses.
In instances where a high degree of pooled heterogeneity was noted, it was often accompanied by significant heterogeneity within the PMN-MDSC subgroup, as detailed in the Supplementary Material. A visual inspection of the Forest plot readily identified the study by Passaro et al. as a potential primary contributor to this observed heterogeneity. Further analysis confirmed that the inclusion of Passaro et al. markedly influenced the heterogeneity levels: for the PMN-MDSC subgroup analyzing PFS, heterogeneity dramatically decreased from I²=78% to 0% upon excluding this study, resulting in an adjusted HR of 2.18 (95% confidence interval [CI]: 1.46–3.26) and a revised pooled HR of 2.10 (95% CI: 1.68–2.62) with an I² of 0%. A similar pattern emerged within the overall survival subgroup; the exclusion of Passaro et al. halved the heterogeneity from I²=68% to 39%, with an HR of 1.73 (95% CI: 1.04–2.88), leading to a pooled HR of 2.26 (95% CI: 1.81–2.83) and an I² of 27%.
This significant reduction in heterogeneity, particularly in the PFS PMN-MDSC population, from I²=78% to a null value (I²=0%) following the removal of Passaro et al., underscores the substantial impact this study had on the heterogeneity levels. Similarly, in the OS analysis, the heterogeneity within the PMN-MDSC subgroup was notably reduced by half (from I²=68% to 39%), with the pooled HR adjusting to 2.26 (95% CI: 1.81–2.83) and an I² of 27%. These findings highlight the critical influence of specific studies on the heterogeneity of meta-analytic outcomes and underscore the importance of scrutinizing individual study contributions to the OS.
In addition, we explored the role of MDSCs as prognostic markers across various subgroups:
As shown in Table 2, MDSCs consistently demonstrated robust prognostic value for OS across all subgroups, except for non-small cell lung cancer (NSCLC), where the HR was 1.85 [95% CI 0.88–3.90]. For PFS, MDSCs showed strong predictive value, particularly in patients with advanced (stage IV) cancer, with an HR of 2.66 [95% CI 1.84–3.86]. Notably, MDSCs maintained low heterogeneity and high predictive value when the median cutoff method was employed.
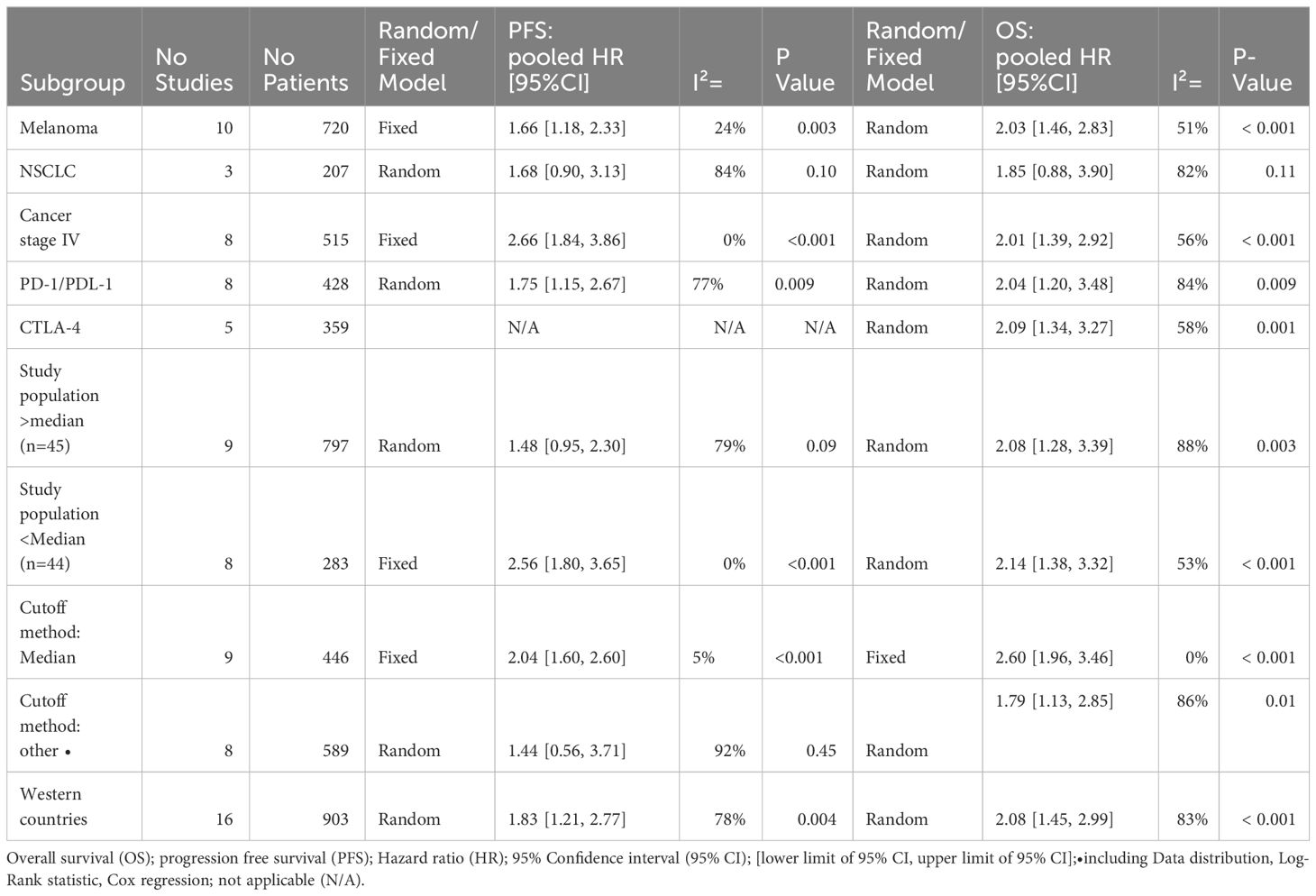
Table 2 Subgroup analysis of myeloid-derived suppressor cell and progression free survival or overall survival.
In our detailed analysis (see Supplementary Table 1 for details), M-MDSC were identified as robust predictive markers for both OS and PFS in nearly all examined subgroups. In NSCLC specifically, M-MDSCs were predictive and prognostic for both outcome measures (OS: HR=2.55 [95% CI 1.36–4.78], PFS: HR=2.07 [95% CI 1.26–3.39]). Additionally, M-MDSCs showed strong predictive value for PFS across different cutoff determination methods (Cutoff method median: HR=1.96 [95% CI 1.40–2.72]; pooled different cutoff methods: HR=2.06 [95% CI 1.22–3.49]). Conversely, PMN-MDSCs were significant prognostic markers only in subgroups utilizing the median as the cutoff method, underscoring their potential as predictive markers in specific contexts (OS: HR=1.74 [95% CI 1.11–2.75]; PFS: HR= 2.29 [95% CI 1.39–3.77]).
3.5 Risk of bias
Our assessment of the risk of bias across various categories yielded heterogeneous outcomes, as detailed in Figures 4A, B. A notable observation was that the employment of non-standardized markers significantly elevated the risk of bias within the prognostic marker category. Specifically, the study attrition and confounder categories were identified as areas with a particularly high risk of bias. This heightened risk was primarily attributed to inadequate descriptions of potential confounding variables or insufficient information regarding participants not included in the analysis. In the category of statistical analysis and reporting, a high risk of bias was frequently encountered; 8 out of 17 studies did not directly report hazard ratios, necessitating their estimation through the method proposed by Tierney et al. (25) or extracted from alternative sources. Specifically, hazard ratios were estimated for 6 studies using the Tierney et al. methodology, while for one study, hazard ratios were obtained from other published sources. Additionally, one study provided hazard ratios upon our direct request, highlighting the challenges and variability in data reporting practices across studies included in our meta-analysis.
3.6 Sensitivity analysis
To validate the reliability of our findings, we conducted a sensitivity analysis by excluding studies identified with a high risk of bias in any category. This stringent approach aimed to mitigate potential biases impacting our conclusions. Despite these exclusions, the pooled hazard ratios for both PFS and OS remained statistically significant, with PFS showing a hazard ratio of 2.59 [95% CI 1.73–3.87] and OS demonstrating a hazard ratio of 1.93 [95% CI 1.30–2.87]. However, heterogeneity remained in OS across all groups. This analysis was limited by the fact that PMN-MDSC or total MDSC could not be considered for PFS due to the lack of studies with low risk of bias here. The sensitivity analysis continues to show significant results, especially for the M-MDSC in OS: 2.23 [95% CI 1.49–3.35]. However, we observed persistent heterogeneity in OS across all evaluated groups, indicating variability that could not be fully accounted for by excluding studies with high bias risk.
A limitation of our sensitivity analysis emerged when considering the specific subtypes of MDSCs, particularly PMN-MDSC and total MDSC, for PFS outcomes. The absence of studies with a low risk of bias for these subgroups precluded their evaluation, underscoring a gap in the available literature. Despite these constraints, the sensitivity analysis underscored the significance of M-MDSC in predicting OS, with a hazard ratio of 2.23 [95% CI 1.49–3.35], reinforcing the potential prognostic value of this MDSC subtype in the context of immune checkpoint inhibitor therapy.
3.7 Publication bias
The analysis of OS and PFS data revealed asymmetry in the funnel plots, indicative of potential publication bias or other small-study effects (Figures 5A, B). This observation was further substantiated by the results of the Egger test, which demonstrated statistical significance with P<0.001 for PFS and P< 0.001 for OS, suggesting the presence of bias in the reported. In alignment with our predefined protocol, we employed the trim-and-fill method as a corrective measure to address this asymmetry, aiming to estimate the effect of potentially unpublished studies on our meta-analysis outcomes. The application of this method led to adjusted hazard ratios (HR) of 1.72 (95%CI 1.23, 2.41) for the PFS and 1.89 (95%CI 1.39, 2.58) for the OS. These revised estimates further underscore the robustness and statistical significance of our findings, reinforcing the predictive value of MDSC levels on the outcomes of patients undergoing immune checkpoint inhibitor therapy, even after accounting for potential publication bias.
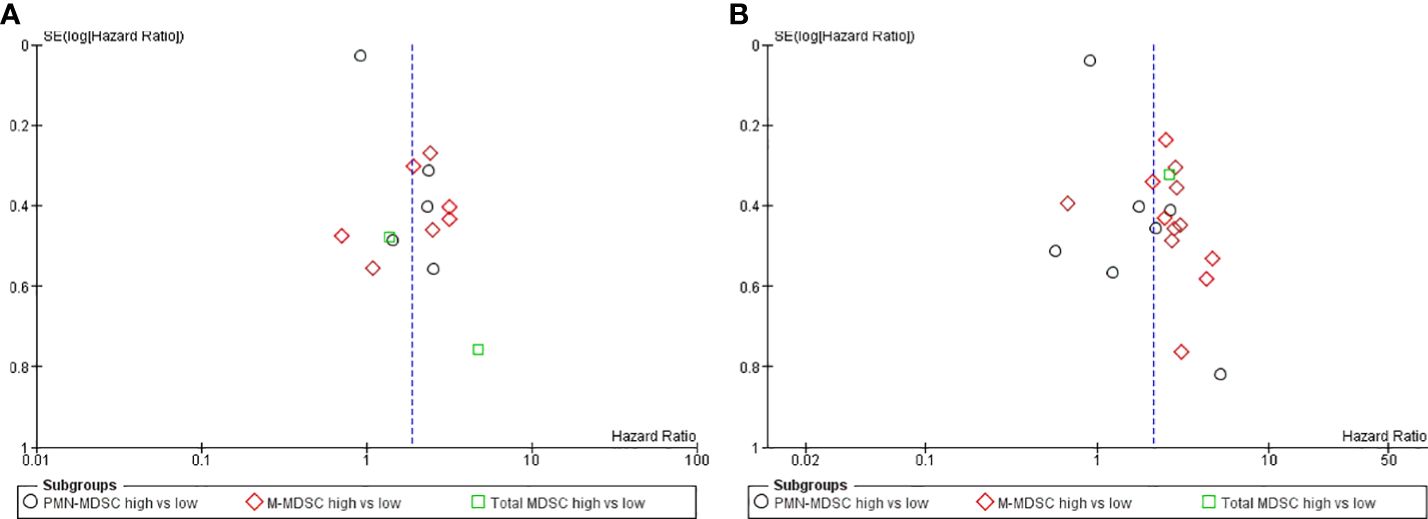
Figure 5 (A) Funnel plot progression free survival: Assessing the publication bias, the hazard ratio was plotted on the X-axis and the standard error corresponding to the logarithm of hazard ratio on the Y-axis. (B) Funnel plot overall survival: Assessing the publication bias, the hazard ratio was plotted on the X-axis and the standard error corresponding to the logarithm of hazard ratio on the Y-axis.
4 Discussion
In our systematic review, we included 17 studies from which we were able to extract data for a meta-analysis. We included data from patients with melanoma (29–31, 34, 36, 37, 41–43, 45), NSCLC (33, 38, 39) prostate cancer (40, 44) and urothelial carcinoma (32). One study pooled data from solid tumors encompassing patients with 11 tumor entities (35).
Our findings indicate that MDSCs can predict both survival and response to ICIs, according to our endpoints of OS and PFS. Particularly, M-MDSCs are shown to be both a predictive and a prognostic marker. Analysis of the sub-cell populations revealed that M-MDSCs are significantly inversely correlated with OS and PFS. Although PMN-MDSCs did not reach statistical significance in our analysis, a trend was observed for both OS and PFS, suggesting the entire MDSC population as significant.
This meta-analysis aligns with previous meta-analyses that investigated MDSCs as predictive and prognostic markers in patients predominantly undergoing chemotherapy (9, 46).
The study of Koh et al. highlighted that M-MDSCs have even better predictive power of response and survival in NSCLC patients than PD-L1 expression on tumor cells (39), suggesting that MDSCs could enhance the predictive accuracy for OS and response rate across various tumor entities. Thus, the currently established markers such as PDL (in NSCLC) or LDH (in melanoma) could be supported by MDSC to predict a better correlation for OS and response rate in other tumor entities, as it has already been shown that patients (NSCLC) with a low PDL status also benefit from ICI (47).
The significance of our findings is underscored by Krebs et al. (34), who identified a subgroup of clinical non-responders to ICI, with an immune profile akin to responders, thus exhibiting prolonged OS. Similarly, Tomela et al. (29), found an inverse correlation between PMN-MDSC levels and PFS in the responder group.
Further research with a standardized gating strategy is crucial for establishing validated cut-off limits for MDSCs, thus facilitating their application in clinical practice as a dynamic prognostic marker. This could help identify patients who might benefit from ICI therapy or those at high risk of non-response, potentially making MDSCs a therapeutic target. The feasibility of this approach has been demonstrated by Tobin et al. (48). and the consideration of a cut-off value defined by healthy subjects as practiced by Kitano et al. (45) is also suggested.
Our analysis encountered significant heterogeneity, particularly notable within the PMN-MDSC subgroup. This variability arose partly from the diverse methods used across studies to define cut-off values, typically applying these thresholds as percentages of living peripheral blood mononuclear cells (PBMCs). Such methodological disparities were a key factor contributing to the observed heterogeneity.
Moreover, the landscape of immunotherapy, particularly with the introduction of ipilimumab in 2011, has undergone significant evolution. This breakthrough marked the beginning of an era characterized by the development and approval of various immune checkpoint inhibitors (ICIs), each with distinct indications. The evolving use of ICIs, including their combination therapies and earlier application in treatment protocols, has led to diverse study designs and populations. These changes reflect the dynamic nature of cancer treatment protocols and have inevitably influenced the heterogeneity observed in our meta-analysis.
This heterogeneity underscores the complexity of drawing generalized conclusions from the available data and highlights the need for standardized approaches in future research. This heterogeneity was addressed using a random effects model, as noted in the protocol, aiming to minimize its impact.
Lower MDSC correlated with a better prognosis, but it has not yet been possible to find a uniform cut-off value to use and implement the cell population as a clinically marker.
Subgroup analyses revealed significant effects for PFS and OS for both M-MDSC and PMN-MDSC populations when studies utilized consistent methods for determining cutoffs, with low heterogeneity. Notably, heterogeneity was attributed not only to tumor entities but also to inherent characteristics within each entity, though limited to NSCLC and melanoma due to the number of studies. Variations in the methodologies used to categorize PMN-MDSC, particularly in terms of gating strategies and the markers employed, have contributed to the observed heterogeneity in our analysis. An illustrative example is the approach taken by Passaro et al. (38), which stood out by utilizing absolute cell counts instead of percentage values for defining PMN-MDSC levels. This deviation underscores the broader issue of inconsistency in measurement techniques across studies, which adds to the challenge of synthesizing data and drawing uniform conclusions.
Despite the significant heterogeneity introduced by such methodological differences, our rigorous assessment process confirmed that the study by Passaro et al. satisfied all predefined inclusion criteria. Therefore, in adherence to our commitment to a comprehensive and inclusive review, we retained the study by Passaro et al. within our meta-analysis. This decision reflects our endeavor to capture a wide spectrum of data and insights, even when faced with high heterogeneity, to ensure the robustness and breadth of our analysis.
In recent years, there has been a growing consensus regarding the identification of similar subpopulations of MDSCs using specific markers (10). This emerging agreement highlights the need for standardized markers to distinguish MDSC subpopulations clearly, especially to avoid confusion with neutrophils. A uniform marker, such as Lox-1, could serve this purpose effectively by providing a clear distinction. Additionally, the adoption of a myeloid score, which incorporates multiple validated markers as proposed by Huber et al., could offer a more nuanced understanding of MDSCs’ role within the immune system (49). Furthermore, considering the complex interplay within the immune system, an alternative approach involves using an index that not only assesses the immunosuppressive impact of myeloid cells but also includes cytotoxic cells. This comprehensive index, as utilized by Araujo et al. (35), offers a more holistic view of the immune landscape. Such an index has the potential not only to enhance our understanding of the immune system’s dynamics but also to serve as a valuable prognostic tool throughout the course of a disease. This multi-faceted approach acknowledges the intricate nature of immune responses and the importance of a comprehensive evaluation for both research and clinical applications (35). Here, the PMN-MDSC group is not significant, so they are not as immunosuppressive as initially expected and possibly the M-MDSC population takes on this characteristic. The two subgroups of MDSCs differ not only in terms of their phenotype, but also in terms of their mechanism of action: while PMN-MDSC are mainly antigen-specific, M-MDSC can be both antigen-specific and antigen-nonspecific (11). These differences may be the reason why PMN-MDSCs did not reach significance in this analysis: some studies have shown a stronger immunosuppressive capacity of M-MDSCs compared to PMN-MDSCs on T cells (16, 50, 51). This could explain the difference in significance in general, as T-cells are the main effectors in ICI. Bronte et al. (46) also note that M-MDSCs have been shown to have a continuous immunosuppressive effect on neoantigen-specific T cells (46, 52). Neoantigen load was negatively correlated with outcome in NSCLC patients (46, 53), suggesting that neoantigen inhibition is more relevant specifically in this tumor subgroup, which can only be addressed by M-MDSCs. This could be the reason for the altered behavior of PMN-MDSCs especially in this patient population. In addition, it should also be noted that the number of studies on PMN-MDSCs in this meta-analysis was relatively small and that the elimination of the study by Passaro et al. (38) as part of a sensitivity analysis increased the prognostic and predictive relevance of PMN-MDSCs.
Studies like Gaißler et al. highlight the prognostic significance of M-MDSC dynamics, showing that patients with initially high MDSC levels but subsequent reductions can achieve similar OS to those with consistently low levels (31). This is corroborated by findings from de Coaña (2017) et al.; after three weeks of therapy patients with lower M-MDSC had a better OS (HR= 2.89 (1.59–6.99) P= 0.002), nevertheless the baseline was not significant (36). The study conducted by Tarhini et al. (54) presents findings that align with a key observation: a significant reduction in the levels of total MDSCs is associated with improved PFS. This indicates that patients who experience a larger decrease in MDSC levels tend to have a longer period without disease progression, underscoring the potential role of MDSCs as dynamic biomarkers for treatment outcomes in cancer therapy.
The limitations of our study include several critical aspects that affect the interpretation and reliability of our results. Firstly, the variability of markers in our meta-analysis posed a significant challenge. Since 2016, there has been a convergence towards the use of standardized markers and gating strategies for MDSCs and their subpopulations, as recommended by Bronte et al. (10). Future research should adhere to these standardized markers to reduce variability. Secondly, our meta-analysis showed a high degree of heterogeneity, possible due to the use of different markers, study designs, and populations. We used a random effects model to address this issue. Furthermore, the assessment of risk of bias added complexity. The observed heterogeneous results necessitated a sensitivity analysis to assess the robustness of our results, particularly with regard to discrepancies in hazard ratio reporting. Seven of the 17 studies did not report hazard ratios with 95% confidence intervals. For one study we were able to obtain this by contacting the author, whereas for the remaining six studies we had to estimate it from Kaplan-Meier curves or other sources. This led to some uncertainty, which we considered in our risk of bias analysis. In addition, our analysis raised concerns about publication bias. Visual inspection of the funnel plot for OS and PFS indicated possible publication bias, which was confirmed by the Egger test. We estimated the impact of unpublished studies using Duval and Tweedie’s trim-and-fill method.
In conclusion, the role of MDSCs, especially M-MDSCs, has been increasingly recognized and validated in the context of cancer immunotherapy. These cells have emerged as significant prognostic markers for predicting the response to immune checkpoint inhibitors. Their utility extends beyond mere prognostication; MDSCs offer a window into identifying patients who may not initially respond to therapy based on their baseline myeloid cell profiles. Such insights are invaluable for tailoring treatment approaches, potentially guiding the escalation of therapy to overcome resistance mechanisms.
Furthermore, MDSCs present a dynamic aspect of the tumor microenvironment that could be monitored over the course of treatment. By observing changes in MDSC levels, clinicians can gain insights into treatment efficacy in real-time, allowing for adjustments to therapy that could enhance outcomes. The potential of MDSCs extends to their viability as therapeutic targets themselves, suggesting that manipulating their levels or function could directly improve the efficacy of ICIs.
Despite the promising horizon that MDSCs represent in the realm of cancer therapy, several challenges remain. A critical barrier to the clinical integration of MDSCs as a biomarker is the lack of standardization in identifying and quantifying these cells. The field would greatly benefit from consensus on the markers used to define MDSC subpopulations and uniform cutoff methods to categorize their levels accurately. Addressing these challenges through future research is essential to harnessing the full potential of MDSCs in improving patient outcomes. By establishing standardized methodologies and integrating MDSC assessments into clinical practice, we can move closer to a future where cancer therapy is more personalized, predictive, and potent.
Data availability statement
The original contributions presented in the study are included in the article/Supplementary Material. Further inquiries can be directed to the corresponding author.
Author contributions
MM: Writing – original draft. VO: Writing – original draft. VU: Writing – review & editing. SH: Writing – review & editing. VB: Writing – review & editing. CR: Writing – review & editing. JH: Writing – original draft. SS: Writing – original draft.
Funding
The author(s) declare that no financial support was received for the research, authorship, and/or publication of this article.
Conflict of interest
The authors declare that the research was conducted in the absence of any commercial or financial relationships that could be construed as a potential conflict of interest.
Publisher’s note
All claims expressed in this article are solely those of the authors and do not necessarily represent those of their affiliated organizations, or those of the publisher, the editors and the reviewers. Any product that may be evaluated in this article, or claim that may be made by its manufacturer, is not guaranteed or endorsed by the publisher.
Supplementary material
The Supplementary Material for this article can be found online at: https://www.frontiersin.org/articles/10.3389/fimmu.2024.1403771/full#supplementary-material
Abbreviations
ICI, immune checkpoint inhibitor therapy; PD-1, programmed death -1; PD-L1, programmed death - Ligand 1; CTLA-4, cytotoxic-T-lymphocyte-associated protein-4, Treg, regulatory T-cells; TME, tumor microenvironment; PRISMA-P, Preferred Reporting Items for Systematic Reviews and Meta-Analysis Protocols 2020; QUIPS, Quality in Prognostic Studies; OS, overall survival; PFS, progression free survival; HR, Hazard ratio; 95% CI, 95% Confidence interval ; [lower limit of 95% CI, upper limit of 95% CI]; USA, United states of America; NSCLC, non-small cell lung cancer; NR, not reported; PBMC, Peripheral blood mononuclear cells; MDSC, myeloid-derived suppressor cells; M-MDSC, monocytic MDSC; PMN-MDSC, polymorphonuclear.
References
1. Global Burden of Disease Cancer C, Kocarnik JM, Compton K, Dean FE, Fu W, Gaw BL, et al. Cancer incidence, mortality, years of life lost, years lived with disability, and disability-adjusted life years for 29 cancer groups from 2010 to 2019: A systematic analysis for the global burden of disease study 2019. JAMA Oncol. (2022) 8:420–44. doi: 10.1001/jamaoncol.2021.6987
2. Leach DR, Krummel MF, Allison JP. Enhancement of antitumor immunity by CTLA-4 blockade. Science. (1996) 271:1734–6. doi: 10.1126/science.271.5256.1734
3. Topalian SL, Drake CG, Pardoll DM. Targeting the PD-1/B7-H1(PD-L1) pathway to activate anti-tumor immunity. Curr Opin Immunol. (2012) 24:207–12. doi: 10.1016/j.coi.2011.12.009
4. Hodi FS, O’Day SJ, McDermott DF, Weber RW, Sosman JA, Haanen JB, et al. Improved survival with ipilimumab in patients with metastatic melanoma. N Engl J Med. (2010) 363:711–23. doi: 10.1056/NEJMoa1003466
5. SChadendorf D, Hodi FS, Robert C, Weber JS, Margolin K, Hamid O, et al. Pooled analysis of long-term survival data from phase II and phase III trials of ipilimumab in unresectable or metastatic melanoma. J Clin Oncol. (2015) 33:1889–94. doi: 10.1200/JCO.2014.56.2736
6. Gandhi L, Rodriguez-Abreu D, Gadgeel S, Esteban E, Felip E, De Angelis F, et al. Pembrolizumab plus chemotherapy in metastatic non-small-cell lung cancer. N Engl J Med. (2018) 378:2078–92. doi: 10.1056/NEJMoa1801005
7. Chevolet I, Speeckaert R, Schreuer M, Neyns B, Krysko O, Bachert C, et al. Clinical significance of plasmacytoid dendritic cells and myeloid-derived suppressor cells in melanoma. J Transl Med. (2015) 13:9. doi: 10.1186/s12967-014-0376-x
8. Shang B, Liu Y, Jiang SJ, Liu Y. Prognostic value of tumor-infiltrating FoxP3+ regulatory T cells in cancers: a systematic review and meta-analysis. Sci Rep. (2015) 5:15179. doi: 10.1038/srep15179
9. Wang PF, Song SY, Wang TJ, Ji WJ, Li SW, Liu N, et al. Prognostic role of pretreatment circulating MDSCs in patients with solid Malignancies: A meta-analysis of 40 studies. Oncoimmunology. (2018) 7:e1494113. doi: 10.1080/2162402X.2018.1494113
10. Bronte V, Brandau S, Chen SH, Colombo MP, Frey AB, Greten TF, et al. Recommendations for myeloid-derived suppressor cell nomenclature and characterization standards. Nat Commun. (2016) 7:12150. doi: 10.1038/ncomms12150
11. Gabrilovich DI. Myeloid-derived suppressor cells. Cancer Immunol Res. (2017) 5:3–8. doi: 10.1158/2326-6066.CIR-16-0297
12. Valenti R, Huber V, Iero M, Filipazzi P, Parmiani G, Rivoltini L. Tumor-released microvesicles as vehicles of immunosuppression. Cancer Res. (2007) 67:2912–5. doi: 10.1158/0008-5472.CAN-07-0520
13. Fleming V, Hu X, Weller C, Weber R, Groth C, Riester Z, et al. Melanoma extracellular vesicles generate immunosuppressive myeloid cells by upregulating PD-L1 via TLR4 signaling. Cancer Res. (2019) 79:4715–28. doi: 10.1158/0008-5472.CAN-19-0053
14. Condamine T, Dominguez GA, Youn JI, Kossenkov AV, Mony S, Alicea-Torres K, et al. Lectin-type oxidized LDL receptor-1 distinguishes population of human polymorphonuclear myeloid-derived suppressor cells in cancer patients. Sci Immunol. (2016) 1:aaf8943. doi: 10.1126/sciimmunol.aaf8943
15. Noman MZ, Desantis G, Janji B, Hasmim M, Karray S, Dessen P, et al. PD-L1 is a novel direct target of HIF-1alpha, and its blockade under hypoxia enhanced MDSC-mediated T cell activation. J Exp Med. (2014) 211:781–90. doi: 10.1084/jem.20131916
16. Movahedi K, Guilliams M, Van den Bossche J, Van den Bergh R, Gysemans C, Beschin A, et al. Identification of discrete tumor-induced myeloid-derived suppressor cell subpopulations with distinct T cell-suppressive activity. Blood. (2008) 111:4233–44. doi: 10.1182/blood-2007-07-099226
17. Gabrilovich DI, Ostrand-Rosenberg S, Bronte V. Coordinated regulation of myeloid cells by tumours. Nat Rev Immunol. (2012) 12:253–68. doi: 10.1038/nri3175
18. Nagaraj S, Gupta K, Pisarev V, Kinarsky L, Sherman S, Kang L, et al. Altered recognition of antigen is a mechanism of CD8+ T cell tolerance in cancer. Nat Med. (2007) 13:828–35. doi: 10.1038/nm1609
19. Youn JI, Nagaraj S, Collazo M, Gabrilovich DI. Subsets of myeloid-derived suppressor cells in tumor-bearing mice. J Immunol. (2008) 181:5791–802. doi: 10.4049/jimmunol.181.8.5791
20. Pan PY, Ma G, Weber KJ, Ozao-Choy J, Wang G, Yin B, et al. Immune stimulatory receptor CD40 is required for T-cell suppression and T regulatory cell activation mediated by myeloid-derived suppressor cells in cancer. Cancer Res. (2010) 70:99–108. doi: 10.1158/0008-5472.CAN-09-1882
21. Lasser SA, Ozbay Kurt FG, Arkhypov I, Utikal J, Umansky V. Myeloid-derived suppressor cells in cancer and cancer therapy. Nat Rev Clin Oncol. (2024) 21:147–64. doi: 10.1038/s41571-023-00846-y
22. De Sanctis F, Solito S, Ugel S, Molon B, Bronte V, Marigo I. MDSCs in cancer: Conceiving new prognostic and therapeutic targets. Biochim Biophys Acta. (2016) 1865:35–48. doi: 10.1016/j.bbcan.2015.08.001
23. Ozbay Kurt FG, Lasser S, Arkhypov I, Utikal J, Umansky V. Enhancing immunotherapy response in melanoma: myeloid-derived suppressor cells as a therapeutic target. J Clin Invest. (2023) 133. doi: 10.1172/JCI170762
24. Kumar V, Patel S, Tcyganov E, Gabrilovich DI. The nature of myeloid-derived suppressor cells in the tumor microenvironment. Trends Immunol. (2016) 37:208–20. doi: 10.1016/j.it.2016.01.004
25. Tierney JF, Stewart LA, Ghersi D, Burdett S, Sydes MR. Practical methods for incorporating summary time-to-event data into meta-analysis. Trials. (2007) 8:16. doi: 10.1186/1745-6215-8-16
26. Hayden JA, van der Windt DA, Cartwright JL, Cote P, Bombardier C. Assessing bias in studies of prognostic factors. Ann Intern Med. (2013) 158:280–6. doi: 10.7326/0003-4819-158-4-201302190-00009
27. Deeks JJ, Higgins JP, Altman DG. Chapter 10.10 heterogeneity. In: Higgins JP, Thomas J, Chandler J, Cumpston M, Li T, Page MJ, Welch VA, editors. Cochrane Handbook for Systematic Reviews of Interventions Version 6.2 (updated February 2021). Cochrane, 2021. London (GB): The Cochrane Collaboration (2021). Available at: http://training.cochrane.org/handbook.
28. McGuinness LA, Higgins JPT. Risk-of-bias VISualization (robvis): An R package and Shiny web app for visualizing risk-of-bias assessments. Res Synth Methods. (2021) 12:55–61. doi: 10.1002/jrsm.1411
29. Tomela K, Pietrzak B, Galus Ł, Mackiewicz J, Schmidt M, Mackiewicz AA, et al. Myeloid-derived suppressor cells (MDSC) in melanoma patients treated with anti-PD-1 immunotherapy. Cells. (2023) 12:789. doi: 10.3390/cells12050789
30. Petrova V, Groth C, Bitsch R, Arkhypov I, Simon SCS, Hetjens S, et al. Immunosuppressive capacity of circulating MDSC predicts response to immune checkpoint inhibitors in melanoma patients. Front Immunol. (2023) 14:1065767. doi: 10.3389/fimmu.2023.1065767
31. Gaißler A, Bochem J, Spreuer J, Ottmann S, Martens A, Amaral T, et al. Early decrease of blood myeloid-derived suppressor cells during checkpoint inhibition is a favorable biomarker in metastatic melanoma. J Immunother Cancer. (2023) 11:e006802. doi: 10.1136/jitc-2023-006802
32. Girardi DM, Niglio SA, Mortazavi A, Nadal R, Lara P, Pal SK, et al. Cabozantinib plus nivolumab phase I expansion study in patients with metastatic urothelial carcinoma refractory to immune checkpoint inhibitor therapy. Clin Cancer Res. (2022) 28:1353–62. doi: 10.1158/1078-0432.CCR-21-3726
33. Bronte G, Petracci E, De Matteis S, Canale M, Zampiva I, Priano I, et al. High levels of circulating monocytic myeloid-derived suppressive-like cells are associated with the primary resistance to immune checkpoint inhibitors in advanced non-small cell lung cancer: an exploratory analysis. Front Immunol. (2022) 13:866561. doi: 10.3389/fimmu.2022.866561
34. Krebs FK, Trzeciak ER, Zimmer S, Özistanbullu D, Mitzel-Rink H, Meissner M, et al. Immune signature as predictive marker for response to checkpoint inhibitor immunotherapy and overall survival in melanoma. Cancer Med. (2021) 10:1562–75. doi: 10.1002/cam4.3710
35. Araujo B, Hansen M, Spanggaard I, Rohrberg K, Reker Hadrup S, Lassen U, et al. Immune cell profiling of peripheral blood as signature for response during checkpoint inhibition across cancer types. Front Oncol. (2021) 11:558248. doi: 10.3389/fonc.2021.558248
36. de Coaña YP, Wolodarski M, Poschke I, Yoshimoto Y, Yang Y, Nyström M, et al. Ipilimumab treatment decreases monocytic MDSCs and increases CD8 effector memory T cells in long-term survivors with advanced melanoma. Oncotarget. (2017) 8:21539–53. doi: 10.18632/oncotarget.v8i13
37. Pico de Coaña Y, Wolodarski M, van der Haar Àvila I, Nakajima T, Rentouli S, Lundqvist A, et al. PD-1 checkpoint blockade in advanced melanoma patients: NK cells, monocytic subsets and host PD-L1 expression as predictive biomarker candidates. Oncoimmunology. (2020) 9:1786888. doi: 10.1080/2162402X.2020.1786888
38. Passaro A, Mancuso P, Gandini S, Spitaleri G, Labanca V, Guerini-Rocco E, et al. Gr-MDSC-linked asset as a potential immune biomarker in pretreated NSCLC receiving nivolumab as second-line therapy. Clin Transl Oncol. (2020) 22:603–11. doi: 10.1007/s12094-019-02166-z
39. Koh J, Kim Y, Lee KY, Hur JY, Kim MS, Kim B, et al. MDSC subtypes and CD39 expression on CD8(+) T cells predict the efficacy of anti-PD-1 immunotherapy in patients with advanced NSCLC. Eur J Immunol. (2020) 50:1810–9. doi: 10.1002/eji.202048534
40. Karzai F, VanderWeele D, Madan RA, Owens H, Cordes LM, Hankin A, et al. Activity of durvalumab plus olaparib in metastatic castration-resistant prostate cancer in men with and without DNA damage repair mutations. J Immunother Cancer. (2018) 6:141. doi: 10.1186/s40425-018-0463-2
41. Weber J, Gibney G, KudChadkar R, Yu B, Cheng P, Martinez AJ, et al. Phase I/II study of metastatic melanoma patients treated with nivolumab who had progressed after ipilimumab. Cancer Immunol Res. (2016) 4:345–53. doi: 10.1158/2326-6066.CIR-15-0193
42. Sade-Feldman M, Kanterman J, Klieger Y, Ish-Shalom E, Olga M, Saragovi A, et al. Clinical significance of circulating CD33+CD11b+HLA-DR- myeloid cells in patients with stage IV melanoma treated with ipilimumab. Clin Cancer Res. (2016) 22:5661–72. doi: 10.1158/1078-0432.CCR-15-3104
43. Martens A, Wistuba-Hamprecht K, Geukes Foppen M, Yuan J, Postow MA, Wong P, et al. Baseline peripheral blood biomarkers associated with clinical outcome of advanced melanoma patients treated with ipilimumab. Clin Cancer Res. (2016) 22:2908–18. doi: 10.1158/1078-0432.CCR-15-2412
44. Santegoets SJ, Stam AG, Lougheed SM, Gall H, Jooss K, Sacks N, et al. Myeloid derived suppressor and dendritic cell subsets are related to clinical outcome in prostate cancer patients treated with prostate GVAX and ipilimumab. J Immunother Cancer. (2014) 2:31. doi: 10.1186/s40425-014-0031-3
45. Kitano S, Postow MA, Ziegler CG, Kuk D, Panageas KS, Cortez C, et al. Computational algorithm-driven evaluation of monocytic myeloid-derived suppressor cell frequency for prediction of clinical outcomes. Cancer Immunol Res. (2014) 2:812–21. doi: 10.1158/2326-6066.CIR-14-0013
46. Bronte G, Calabro L, Olivieri F, Procopio AD, Crino L. The prognostic effects of circulating myeloid-derived suppressor cells in non-small cell lung cancer: systematic review and meta-analysis. Clin Exp Med. (2023) 23:1551–61. doi: 10.1007/s10238-022-00946-6
47. Doroshow DB, Sanmamed MF, Hastings K, Politi K, Rimm DL, Chen L, et al. Immunotherapy in non-small cell lung cancer: facts and hopes. Clin Cancer Res. (2019) 25:4592–602. doi: 10.1158/1078-0432.CCR-18-1538
48. Tobin RP, Cogswell DT, Cates VM, Davis DM, Borgers JSW, Van Gulick RJ, et al. Targeting MDSC differentiation using ATRA: A phase I/II clinical trial combining pembrolizumab and all-trans retinoic acid for metastatic melanoma. Clin Cancer Res. (2023) 29:1209–19. doi: 10.1158/1078-0432.CCR-22-2495
49. Huber V, Di Guardo L, Lalli L, Giardiello D, Cova A, Squarcina P, et al. Back to simplicity: a four-marker blood cell score to quantify prognostically relevant myeloid cells in melanoma patients. J Immunother Cancer. (2021) 9:e001167. doi: 10.1136/jitc-2020-001167
50. Wang SH, Lu QY, Guo YH, Song YY, Liu PJ, Wang YC. The blockage of Notch signalling promoted the generation of polymorphonuclear myeloid-derived suppressor cells with lower immunosuppression. Eur J Cancer. (2016) 68:90–105. doi: 10.1016/j.ejca.2016.08.019
51. Dolcetti L, Peranzoni E, Ugel S, Marigo I, Fernandez Gomez A, Mesa C, et al. Hierarchy of immunosuppressive strength among myeloid-derived suppressor cell subsets is determined by GM-CSF. Eur J Immunol. (2010) 40:22–35. doi: 10.1002/eji.200939903
52. Haverkamp JM, Smith AM, Weinlich R, Dillon CP, Qualls JE, Neale G, et al. Myeloid-derived suppressor activity is mediated by monocytic lineages maintained by continuous inhibition of extrinsic and intrinsic death pathways. Immunity. (2014) 41:947–59. doi: 10.1016/j.immuni.2014.10.020
53. Gong L, He R, Xu Y, Luo T, Jin K, Yuan W, et al. Neoantigen load as a prognostic and predictive marker for stage II/III non-small cell lung cancer in Chinese patients. Thorac Cancer. (2021) 12:2170–81. doi: 10.1111/1759-7714.14046
Keywords: immune checkpoint inhibitors, immunotherapy, myeloid-derived suppressor cells, MDSC, neoplasms, solid malignancies, prognosis, biomarkers
Citation: Möller M, Orth V, Umansky V, Hetjens S, Braun V, Reißfelder C, Hardt J and Seyfried S (2024) Myeloid-derived suppressor cells in peripheral blood as predictive biomarkers in patients with solid tumors undergoing immune checkpoint therapy: systematic review and meta-analysis. Front. Immunol. 15:1403771. doi: 10.3389/fimmu.2024.1403771
Received: 19 March 2024; Accepted: 09 May 2024;
Published: 24 May 2024.
Edited by:
Raquel Tarazona, University of Extremadura, SpainReviewed by:
Luis De La Cruz-Merino, Virgen Macarena University Hospital, SpainNikoleta Bizymi, University of Crete, Greece
Katherine Bline, Nationwide Children’s Hospital, United States
Copyright © 2024 Möller, Orth, Umansky, Hetjens, Braun, Reißfelder, Hardt and Seyfried. This is an open-access article distributed under the terms of the Creative Commons Attribution License (CC BY). The use, distribution or reproduction in other forums is permitted, provided the original author(s) and the copyright owner(s) are credited and that the original publication in this journal is cited, in accordance with accepted academic practice. No use, distribution or reproduction is permitted which does not comply with these terms.
*Correspondence: Steffen Seyfried, c3RlZmZlbi5zZXlmcmllZEB1bW0uZGU=
†These authors share first authorship
‡These authors share last authorship