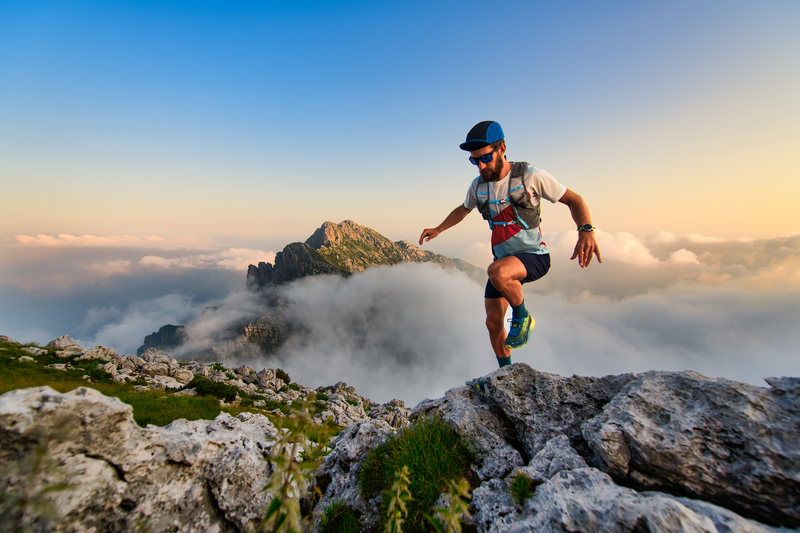
95% of researchers rate our articles as excellent or good
Learn more about the work of our research integrity team to safeguard the quality of each article we publish.
Find out more
ORIGINAL RESEARCH article
Front. Immunol. , 27 September 2024
Sec. Microbial Immunology
Volume 15 - 2024 | https://doi.org/10.3389/fimmu.2024.1395580
Background: Several observational studies have indicated an association between interstitial cystitis and the composition of the gut microbiota; however, the causality and underlying mechanisms remain unclear. Understanding the link between gut microbiota and interstitial cystitis could inform strategies for prevention and treatment.
Methods: A two-sample Mendelian randomization analysis was conducted using published genome-wide association study summary statistics. We employed inverse variance weighted, weighted mode, MR-Egger, weighted median, simple mode, and cML-MA methods to investigate the causal relationship between gut microbiota and interstitial cystitis. Sensitivity analysis was performed to validate the results. Relevant gut microbiota was examined through reverse MR. Single nucleotide polymorphisms were annotated using FUMA to identify genes associated with these genetic variants, thereby revealing potential host gene-microbiota associations in interstitial cystitis patients.
Results: Eight bacterial taxa were identified in our analysis as associated with interstitial cystitis. Among these, Butyricimonas, Coprococcus, Lactobacillales, Lentisphaerae, and Bilophila wadsworthia were positively correlated with interstitial cystitis risk, while taxa such as Desulfovibrio piger, Oscillibacter unclassified and Ruminococcus lactaris exhibited protective effects against interstitial cystitis. The robustness of these associations was confirmed through sensitivity analyses. Reverse MR analysis did not reveal evidence of reverse causality. Single nucleotide polymorphisms were annotated using FUMA and subjected to biological analysis. Seven hub genes (SPTBN1, PSME4, CHAC2, ERLEC1, ASB3, STAT5A, and STAT3) were identified as differentially expressed between interstitial cystitis patients and healthy individuals, representing potential therapeutic targets.
Conclusion: Our two-sample Mendelian randomization study established a causal relationship between gut microbiota and interstitial cystitis. Furthermore, our identification of a host gene-microbiota association offers a new avenue for investigating the potential pathogenesis of interstitial cystitis and suggests avenues for the development of personalized treatment strategies.
Interstitial cystitis (IC), also termed bladder pain syndrome (BPS), presents as a chronic non-bacterial inflammation of the bladder. It is characterized by varying degrees of frequency, urgency, dysuria, and/or suprapubic pain among affected individuals, sometimes extending to the urethra, perineum, vagina, and during sexual intercourse (1). The condition predominantly affects women, with a male-to-female ratio of approximately 1:10 (2) and an incidence rate as high as 6.53% (3) among adult women. Despite extensive research, the etiology of IC remains elusive (4), complicating the search for specific treatment modalities. Prolonged medical interventions for IC render patients vulnerable to mental health disorders such as depression and anxiety, contributing to a substantial suicide risk rate of 38.10% (5).
The human gut harbors a diverse microbiota comprising bacteria, fungi, archaea, viruses, and protozoa. This gut microbiota exists in symbiosis with the intestinal mucosa, playing crucial roles in immunomodulation, metabolism, and gastrointestinal protection in individuals with good health (6). Chronic dysbiosis can disrupt multiple bodily homeostatic mechanisms, potentially precipitating local and systemic inflammatory reactions (7). Researchers are increasingly intrigued by the interplay between gut microbiota and chronic inflammation-related disorders, as it unveils novel therapeutic avenues for conditions like inflammatory bowel disease (8). The gut microbiota has emerged as a critical factor in the pathogenesis of various inflammatory diseases, including asthma, inflammatory bowel disease, non-alcoholic fatty liver disease, rheumatoid arthritis, and type II diabetes mellitus (9). Recent studies have emphasized the “gut-bladder axis” in the interaction between the gut microbiota and bladder (10, 11). Similarly, research has explored the role of gut microbiota in the development and progression of IC and its implications for diagnostic and therapeutic strategies (12, 13). Comparisons with healthy controls revealed significant reductions in the levels of Eggerthella sinensis, Collinsella aerofaciens, Faecalibacterium prausnitzii, Odoribacter splanchnicus, and Lactonifactor longoviformis. An animal experiment demonstrated that fecal transplantation from wild-type mice into IC model mice alleviated IC symptoms, while fecal transplantation from IC model mice exacerbated pain and anxiety behaviors, suggesting the potential involvement of gut microbiota in IC development. However, comprehensive causal analysis of the interactions between gut microbiota and IC is still lacking in current literature.
Mendelian randomization (MR) is an innovative epidemiological method that utilizes genome-wide association study (GWAS) data and employs single nucleotide polymorphisms (SNPs) as instrumental variables (IVs) to establish causality and discern the causal relationship between an exposure and an outcome. The MR approach operates on the principle that distinct genotypes determine varied phenotypes, and adheres to Mendel’s Law of Inheritance, which stipulates that “parental alleles are randomly allocated to offspring during gamete formation.” Furthermore, alleles are randomly assigned to individuals at conception, predating both exposure and outcome, thereby obviating concerns of reverse causation. Theoretically, genetic variation remains unaffected by common confounders, such as postnatal environment and economic status. Notably, the MiBioGen consortium and the Dutch Microbiome Project (DMP) have recently published numerous loci linked to microbiome abundance, presenting an unparalleled opportunity to investigate causal relationships between gut microbiota and IC. Accordingly, we conducted a bidirectional two-sample MR analysis to elucidate the causal link between gut microbiota and IC while further probing the host gene-microbiota associations.
Figure 1 presents an overview of the study. Since our study was based on a public database, no additional ethical approval or informed consent was required.
Summary statistics of the human gut microbiome from phylum to genus level utilized in this research were acquired from the MiBioGen consortium. This consortium conducted a large-scale, multiethnic GWAS that integrated 16S ribosomal RNA gene sequencing and genotyping data from 18,340 participants across 24 cohorts spanning the United States, Canada, Israel, South Korea, Germany, Denmark, the Netherlands, Belgium, Sweden, Finland, and the United Kingdom. The aim was to investigate the relationship between autosomal human genetic variation and the gut microbiome. The dataset consisted of 211 taxa across 9 phyla, 16 classes, 20 orders, 35 families, and 131 genera. Notably, there were 12 unidentified genera and 3 unidentified families (14), with the genus being the lowest taxonomic level considered in this study. Furthermore, our investigation incorporated 105 additional species identified in a GWAS involving 7,738 individuals of European descent from the DMP. The composition of the gut microbiome was determined through shotgun metagenomic sequencing of stool samples as part of this initiative (15). Consequently, our study analyzed 9 phyla, 16 classes, 20 orders, 32 families, 119 genera, and 105 species-level taxa. Summary statistics for IC were extracted from the GWAS catalog website (16). The study utilized the phenotype “chronic interstitial cystitis” with the GWAS summary data encompassing 456,348 European adult female participants, comprising 240 cases and 456,108 controls. Additional details regarding the GWAS conducted in this Mendelian randomization study are available in Supplementary Table S1.
The usage and interpretation of our MR study adhere to the STROBE-MR (Strengthening the Reporting of Observational Studies in Epidemiology-Mendelian Randomization) checklist (17, 18) (Supplementary Table S2).
The MR analysis relies on three key assumptions: 1) the IVs are strongly associated with the exposure, 2) the IVs are not associated with confounding factors between exposure and outcome, and 3) the IVs influence outcomes solely through the exposure. In summary, the gut microbiota was considered the exposure, with IC serving as the outcome. Bacterial taxa were examined at six levels (phylum, class, order, family, genus, and species), and a unique taxon was defined as a characteristic. To ensure the reliability and accuracy of the conclusions regarding the causal relationship between gut microbiota and IC, the following quality control measures were implemented to select IVs. The selection criteria were as follows (19, 20): 1) Criteria were relaxed appropriately due to the limited number of IVs at P < 5 × 10-8. SNPs associated with each taxon were chosen as potential IVs at the genome-wide significance threshold (P < 1 × 10-5); 2) Using data from 1,000 samples from the European Genome Initiative as a reference panel, the linkage disequilibrium (LD) between these SNPs was calculated, and SNPs meeting an r2 < 0.001 (clumping window size = 10,000kb) were selected to reduce the likelihood of biased results; 3) SNPs with minor allele frequencies (MAF) ≤ 0.01 were excluded; and (4) An essential step in MR is to ensure that the effect of SNPs on exposure corresponds to the same allele as the effect on the outcome. To mitigate distortions in strand orientation or allele coding, palindromic SNPs were excluded. Additionally, for each SNP, the strength of the IVs was evaluated using the F-statistic, calculated by the formula F=(R2× (N-2))/(1- R2), and R2=(2 × β2 × EAF × (1-EAF))/(2 ×β2 × EAF × (1-EAF) + 2 × SE2 × N × EAF × (1-EAF)) with R2 representing the proportion of variance in exposure explained by the genetic variables, N indicating the number of participants, EAF denoting the effect allele frequency, and β representing the estimated effect of SNP to assess its ability to predict the outcome uniquely (21–23). A significant weak instrumental bias was deemed absent if the corresponding F-statistic exceeded 10 (24).
To evaluate the causal relationship between gut microbiota and IC, we conducted reverse MR analysis using the same selection criteria for IVs and MR analysis methodology as described above. This analysis was performed on the SNPs associated with IC to investigate whether IC exerts a causal influence on gut microbiota. The data sources used for reverse Mendelian randomization were identical to those utilized for forward Mendelian randomization.
MR analysis was conducted to explore the causal relationship between microbiota features and IC. The Wald ratio test estimated the association between identified IVs and IC for features with only one IV (25). For features with multiple IVs, five widely used MR methods were employed: inverse variance weighted (IVW) (26), weighted mode (27), MR-Egger regression (28), weighted median (29), and simple mode (27). Additionally, this study utilized a model based on the Constrained Maximum Likelihood and Model Averaging MR method (cML-MA) (30), which does not depend on internal assumptions and is used to account for correlated and uncorrelated multivariate effects. IVW was used as the primary MR Analysis method to assess the relationship between gut microbiota and IC, supplemented by the other five methods. Estimates were presented as odds ratios (ORs) with 95% confidence intervals (CIs).
Sensitivity analyses were conducted to assess the robustness of the results, including heterogeneity tests, pleiotropy tests, and leave-one-out sensitivity tests. The pleiotropy test comprised MR-Egger intercept and MR-pleiotropy residual sum and outlier (MR-PRESSO) tests. A significant intercept term (P ≤ 0.05) in the MR-Egger analysis indicated potential pleiotropy. Horizontal pleiotropy was identified using MR-PRESSO, and any outliers detected prompted their removal, followed by reanalysis of the remaining IVs (31). Cochran’s Q test determined the heterogeneity of single nucleotide polymorphisms (SNPs), with a statistically significant Cochran’s Q test (P ≤ 0.05) indicating significant heterogeneity in the analysis results (32, 33). Leave-one-out sensitivity tests were conducted to ascertain whether a single SNP was driving the causal signal. This method compared differences in exposure and outcome of IVs. If the IVs explained more of the difference in exposure than in outcome, the identified causal link could be considered directionally plausible (34).
Additionally, we applied the Bonferroni method at each taxonomic level (phylum, order, family, genus, and species) to determine distinct significance thresholds for multiple testing based on the number of bacteria at each level. The significance threshold was defined as P ≤ 0.05/n, where n represents the effective number of independent bacterial taxa at the corresponding level. For example, at the phylum level, the threshold was 5.6 × 10-3 (0.05/9), at the class level, it was 3.1 × 10-3 (0.05/16), at the order level, it was 2.5 × 10-3 (0.05/20), at the family level, it was 1.6 × 10-3 (0.05/32), at the genus level, it was 4.2 × 10-4 (0.05/119), and at the species level, it was 4.8 × 10-4 (0.05/105). Bacterial taxa with P ≤ 0.05, although not statistically significant after Bonferroni correction, were considered potentially associated, and corrected P-values that remain significant are considered highly likely to be associated with IC.
The analysis was conducted using the “TwoSampleMR (version 0.5.7) (34)”, “MRcML (30)”, and “MRPRESSO (version 1.0) (31)” packages within the R program (version 4.3.1).
To delve deeper into the mechanism of gut microbiota’s influence on IC, we identified IVs that passed the screening criteria in the MR analysis for each taxon as lead SNPs in FUMA GWAS (35), a comprehensive platform for annotating, prioritizing, visualizing, and interpreting GWAS results. Using the SNP2GENE tool in FUMA, we localized these SNPs to genes (36). Independent significant SNPs aid in identifying multiple genetic signals associated with traits or diseases, ensuring signal independence and preventing redundancy due to linkage disequilibrium. Lead SNPs are further refined to select the most representative variants, which are then used to define genomic risk loci and for subsequent functional studies or further analysis as key genetic markers. Independent significant SNPs were defined with a P < 1×0-5 and pairwise LD (r2) < 0.6, whereas lead SNPs were identified with a stricter threshold of r2 < 0.1. Closely located independent significant SNPs (< 250kb based on the most right and left SNPs from each LD block) formed a genomic risk locus. We employed three strategies—position mapping (with default 10kb window), expression quantitative trait loci (eQTL) mapping (tissue type for eQTLGen cis-eQTLs and eQTLGen trans-eQTLs), and 3D chromatin interaction mapping (builtin chromatin interaction data for bladder)—to annotate SNPs to genes and generate a set of genes. To explore gene interactions at the protein level, we constructed a protein-protein interaction (PPI) network of mapped genes using STRING (37), with 0.4 serving as the recommended minimum interaction index and all other variables set to default values. We conducted Gene Ontology (GO) enrichment analysis of these genes using KOBAS (38) to understand the molecular mechanisms associated with the potential relationship between gut microbiota influence and IC. The significance threshold was set at P ≤ 0.05. Finally, we utilized the Cytoscape plugin “CytoHubba” to identify hub genes within the PPI network. We applied the Maximal Clique Centrality (MCC) method to pinpoint the top five hub genes, as gene nodes with numerous interactions are pivotal for maintaining the stability of the entire network (39).
We obtained data GSE11783 from the Gene Expression Omnibus (GEO) database (40). This dataset presents reliable gene expression profiles of IC bladder tissues exclusively from human samples, comprising 10 IC patient bladder samples and 6 healthy control bladder samples. The dataset was generated using the GPL570[HG-U133_Plus_2] Affymetrix Human Genome U133 Plus 2.0 Array platform. After standardizing, normalizing, annotating, and cleaning the clinical information within the GSE11783 dataset, we identified differentially expressed genes (DEGs) between the IC patient group and the healthy control group using GEO’s GEO2R tool. The criterion for identifying DEGs was set at P ≤ 0.05.
We utilized 2875 SNPs as IVs for the gut microbiota according to the IV selection criteria. Effect alleles, alternative alleles, β coefficients, standard errors (SE), and P-values of these SNPs were extracted for MR analyses. The F-statistics of the IVs were all >10, indicating no evidence of weak instrumental bias. Supplementary Table S3 provides detailed information on the selected IVs.
Figure 2 and Supplementary Table S4 illustrate that eight bacterial taxa are associated with IC, namely genus.Butyricimonas.id.945, genus.Coprococcus1.id.11301, order.Lactobacillales.id.1800, phylum.Lentisphaerae.id.2238, speices.Bilophila_wadsworthia, speices.Desulfovibrio_piger, speices.Oscillibacter_unclassified, and speices.Ruminococcus_lactaris. The cML-MA, MR-Egger regression, weighted mode, simple mode, and weighted median methods provided comparable causal estimates to IVW in terms of magnitude and direction (Supplementary Figure S1).
Figure 2. Forest plot of the associations between genetically determined 8 gut microbial genera with the risks of IC. OR, odds ratio; CI, confidence interval; SNP, single nucleotide polymorphism.
The study revealed a positive association between IC risk and five gut microbiota: genus.Butyricimonas.id.945 (OR = 2.273, 95% CI: 1.052-4.915, P = 0.037), genus.Coprococcus1.id.11301 (OR = 3.055, 95% CI: 1.150-8.117, P = 0.025), order.Lactobacillales.id.1800 (OR = 3.196, 95% CI: 1.365-7.485, P = 0.007), phylum.Lentisphaerae.id.2238 (OR = 1.970, 95% CI: 1.045-3.714, P = 0.036), and speices.Bilophila_wadsworthia (OR = 3.184, 95% CI: 1.260-8.043, P = 0.014). These findings suggest a potential increase in IC risk associated with these bacteria. Conversely, three gut microbiota were linked to a reduced risk of IC: speices.Desulfovibrio_piger (OR = 0.364, 95% CI: 0.193-0.690, P = 0.002), speices.Oscillibacter_unclassified (OR = 0.247, 95% CI: 0.070-0.868, P = 0.029), and speices.Ruminococcus_lactaris (OR = 0.273, 95% CI: 0.089-0.835, P = 0.023). These findings suggest a potential protective role against IC. However, after applying multiple comparison correction, these associations are no longer significant. We recognize the importance of the Bonferroni correction in controlling for multiple comparisons. Nonetheless, Bonferroni correction is highly conservative and can lead to over-correction, potentially obscuring biologically meaningful signals when dealing with a large number of comparisons. This is particularly relevant in studies of complex traits, where certain small yet biologically significant effects may not remain significant after rigorous correction. Although the associations are no longer significant post-correction, the P-values still suggest potential biological mechanisms (41, 42) and may indicate a link between specific gut microbiota and IC. We propose that these preliminary findings serve as important clues for future research and can provide valuable insights for developing new hypotheses and experimental designs. Therefore, we highlight the significance of these results and advocate for further investigation into their potential biological relevance.
The MR-Egger intercept analysis yielded non-significant results (P > 0.05), suggesting no genetic pleiotropy bias in the results. MR-PRESSO analysis also detected no outliers (P > 0.05), and Cochran’s Q test found no significant heterogeneity (P > 0.05). Collectively, these sensitivity analyses, including Cochran’s Q test, MR-Egger intercept, MR-PRESSO global test, and leave-one-out test, underscored the robustness of the MR results across both samples (Supplementary Tables S5-S7). Leave-one-out analysis revealed that the majority of correlated signals were not driven by a single genetic marker (Supplementary Figure S2). Additionally, funnel plots demonstrating a symmetrical pattern were provided to affirm the reliability of the results (Supplementary Figure S3).
To investigate potential reverse causal effects, we examined IC as the exposure and gut microbiota as the outcome. Employing the same IV selection criteria as the forward MR analysis, detailed IV information is provided in Supplementary Table S8. Subsequently, we conducted a reverse MR analysis using identical methodology to explore the causal relationship between the eight bacterial taxa and IC.
Results depicted in Supplementary Table S9 showed that none of the gut microbiota exhibited a significant inverse causal relationship with IC, including genus.Butyricimonas.id.945 (P = 0.381), genus.Coprococcus1.id.11301 (P = 0.183), order.Lactobacillales.id.1800 (P = 0.593), phylum.Lentisphaerae.id.2238 (P = 0.646), speices. Bilophila_wadsworthia (P = 0.736), speices.Desulfovibrio_piger (P = 0.894), speices. Oscillibacter_unclassified (P = 0.168), and speices.Ruminococcus_lactaris (P = 0.486). Cochran’s Q test indicated no significant heterogeneity in IVs. Additionally, results from MR-Egger intercept analysis and MR-PRESSO analysis did not reveal significant horizontal pleiotropy (Supplementary Tables S10-S12).
Initially, we employed the SNP2GENE tool in FUMA to target SNPs associated with bacterial taxa influencing increased and decreased IC risk, resulting in the identification of 116 and 38 genes, respectively (Supplementary Table S13). To elucidate gene interactions at the protein level, we utilized STRING to construct PPI networks (Figures 3A, B), illustrating the first 5 nodes as hub genes. Notably, hub genes for bacterial taxa associated with increased IC risk included STAT3, GHDC, HCRT, STAT5A, and CAVIN1, while those linked with decreased IC risk comprised ASB3, ERLEC1, CHAC2, PSME4, and SPTBN1. Furthermore, we performed GO enrichment analysis on the genes mapped through the aforementioned methods, categorizing them into molecular function, cellular composition, and biological process. Our findings revealed that bacterial taxa elevating IC risk (Figure 3C) predominantly localized within the cytosol, cytoplasm, plasma membrane, and nucleus. Moreover, they were primarily associated with protein binding at the molecular level, while being involved in signal transduction and cell adhesion processes at the biological level. Conversely, bacterial taxa mitigating IC risk (Figure 3D) exhibited molecular functions primarily related to protein binding, cadherin binding, and channel regulator activity. Their biological processes primarily pertained to the regulation of transmembrane transporter activity.
Figure 3. Biological annotation of genes. (A) PPI network of genes associated with gut microbiota linked to decreased IC risk. (B) PPI network of genes associated with gut microbiota linked to increased IC risk. Edge colors represent different evidence underlying predicted protein–protein interactions in the STRING database. Line thickness indicates the strength of data support and disconnected nodes are hidden in the network. (C) Enrichment analysis of GO terms for genes associated with gut microbiota linked to decreased IC risk. (D) Enrichment analysis of GO terms for genes associated with gut microbiota linked to increased IC risk. BP, biological process; CC, cellular component; MF, molecular.
Using the GSE11783 dataset, the normalized results are depicted in Figure S4. We conducted differential gene expression analysis between healthy individuals and IC patients using GEO2R. Our findings revealed differential expression in 7 out of 10 central genes compared to healthy individuals. Notably, SPTBN1 and PSME4 exhibited significant down-regulation in IC patients, while CHAC2, ERLEC1, ASB3, STAT5A, and STAT3 showed significant up-regulation in IC patients (Figure 4). Therefore, these differentially expressed genes (DEGs) may play pivotal roles in IC development influenced by gut microbiota.
Figure 4. Gene expression in healthy people and IC patients. Expression of ASB3(A), CHAC2 (B), ERLEC1 (C), STAT3 (D), SPTBN1 (E), PSME4 (F), and STAT5A (G) in healthy people and IC patients.
The investigation initiated with MR analysis of two samples to explore the potential causal link between gut microbiota and IC, employing summary statistics from GWAS. Subsequently, we delved into the molecular mechanisms through which gut microbiota influences IC development and elucidated potential host gene-microbiota associations in IC patients. To our knowledge, this represents the inaugural MR study probing the causal association between gut microbiota and IC. Our study not only holds promise for effective IC prevention and treatment strategies but also furnishes novel insights into IC pathogenesis from a gut microbiota perspective.
In our present MR investigation, we identified a causal link between eight bacterial taxa and the risk of IC. Notably, Butyricimonas, Coprococcus, Lactobacillales, Lentisphaerae, and Bilophila wadsworthia exhibited positive associations with IC risk. Conversely, taxa like Desulfovibrio piger, Oscillibacter, and Ruminococcus lactaris demonstrated protective effects against IC.
Desulfovibrio piger, one of the most prevalent pairs of sulfate-reducing bacteria in feces (43), releases hydrogen sulfide (H2S), a third gas signaling molecule widely involved in various biochemical reactions in the animal body. It plays a physiological role in the gastrointestinal tract, regulating cellular and tissue functions (44, 45). It can downregulate the expression of cyclooxygenase-2 mRNA and prostaglandin synthesis, as well as reduce the expression of the pro-inflammatory cytokine tumor necrosis factor-alpha mRNA, thereby exerting either pro-inflammatory or anti-inflammatory effects. Its anti-inflammatory effects may be one of the mechanisms through which it benefits individuals with IC (46, 47). Additionally, H₂S may also function as a signaling molecule in the host, potentially providing beneficial effects such as cardioprotection (48). Recent research has revealed Desulfovibrio piger’s positive impact on the growth and function of Faecalibacterium prausnitzii (49). Faecalibacterium prausnitzii, a crucial bacterium in the human gut flora, is a major producer of butyric acid, known for its anti-inflammatory effects, enzyme activity maintenance, and protection of the digestive system against intestinal pathogens (50). However, observational studies indicate significantly reduced levels of Faecalibacterium prausnitzii in the feces of IC patients (13). Ruminococcus, one of the earliest gastric bacteria identified, significantly influences metabolism, digestion, and the metabolism of resistant starch. Patients with inflammatory bowel disease often exhibit varied Ruminococcus numbers and activities in their intestines, affecting gut health and inflammation levels (51). Anomalies in Ruminococcus may contribute to immune-related diseases like allergy, eczema, and asthma (52). Although the precise mechanism remains unclear, studies suggest that Ruminococcus may impact the pathogenesis of immune-mediated diseases by influencing intestinal immune system regulation and inflammatory responses. Bilophila, an opportunistic pathogen group, represented by Bilophila wadsworthia, was initially isolated from patients with gangrenous and perforated appendicitis (53). It synergizes with a high-fat diet, leading to increased inflammatory responses, intestinal barrier dysfunction, and bile acid metabolism abnormalities, consequently raising the risk of glucose metabolism abnormalities and hepatic steatosis (54). Bilophila wadsworthia eventually metabolizes 2,3-dihydroxypropane-1-sulfonate, producing H2S through a recently discovered metabolic pathway (55). Butyricimonas, a beneficial bacterium, produces short chain fatty acids (SCFAs), the primary metabolites of anaerobic bacterial fermentation in the gut (56). SCFAs serve as crucial fuel for intestinal epithelial cells and exhibit potential anti-inflammatory effects, along with immune cell modulation (57). For example, SCFAs influence epithelial barrier function and mucosal and systemic immunity through G protein-coupled receptor signaling or histone deacetylase activity. SCFAs can also directly or indirectly affect immune responses in extramural sites such as the liver, lungs, reproductive tract, and brain, and are associated with various diseases. Coprococcus, a significant member of the Firmicutes phylum and Lachnospiraceae family, resembles Faecalibacterium prausnitzii in terms of its beneficial role in intestinal health and immune system balance. Actively fermenting carbohydrates, Coprococcus is a key butyric acid producer and serves as a microbial biomarker for assessing gastrointestinal health (58). Butyric acid exerts anti-inflammatory effects by directly influencing the differentiation of intestinal epithelial cells, phagocytes, B cells, plasma cells, as well as regulatory and effector T cells (57). Interestingly, we observed deleterious effects of the Butyricimonas and Coprococcus on IC, offering a novel perspective for future investigations.
Studies have demonstrated that gut microbiota can regulate host physiology and potentially induce disease by influencing gene expression (59–61). Thus, we utilized FUMA to map SNPs to genes and explore genes that might exhibit a causal relationship with IC. To gain insight into the biological functions of these genes in disease, we conducted GO enrichment analysis. Our findings suggest that gut microbiota with beneficial roles may impact channel regulator activity, protein binding, cadherin binding, and other pathways. Conversely, gut microbiota with detrimental roles may influence protein repair, paranodal junction, cell-cell junction, protein binding, and other pathways. Subsequently, we conducted a comparative analysis of hub gene expression between IC patients and healthy controls using bulk RNA analysis. The results revealed significant down-regulation of SPTBN1 and PSME4 in IC patients, while CHAC2, ERLEC1, ASB3, STAT5A, and STAT3 were significantly up-regulated. Therefore, SPTBN1, PSME4, CHAC2, ERLEC1, ASB3, STAT5A, and STAT3 could potentially represent novel genes implicated in the underlying pathogenesis of IC and serve as therapeutic targets.
Spectrin Beta, Non-Erythrocytic 1 (SPTBN1) is a protein-coding gene known for its role in maintaining neuronal morphological stability, cellular structure, and cell signaling. Additionally, it contributes to synapse formation and normal neuronal function (62). Several studies have indicated a correlation between IC and neurological disorders such as fibromyalgia, depression, and memory impairment (63, 64). Moreover, neurogenic pain is considered a potential etiological factor in IC, wherein pain may arise from dysfunction in peripheral, central, and/or endogenous inhibitory pathways (65). Proteasome Activator Subunit 4 (PSME4) encodes a protein that regulates proteasome activity, thereby influencing the rate and selectivity of protein degradation (66). However, its association with inflammation and IC remains unclear. One of the primary intracellular functions of the protein encoded by the ChaC Glutathione Specific Gamma-Glutamylcyclotransferase 2 (CHAC2) gene, is its involvement in glutathione metabolism. Glutathione, a crucial antioxidant, safeguards cells from oxidative stress (OS)-induced damage within the cell. Research indicates that the expression level of the CHAC2 protein is influenced by intracellular OS levels, thereby regulating intracellular glutathione levels and subsequently impacting the cell’s redox state and its ability to counter OS (67, 68). OS is recognized as a significant contributing factor in inflammatory diseases. Notably, one of the key features underlying the pathobiology of IC is the excessive production of reactive oxygen species and cytokines, leading to pronounced OS (69). Mitigating OS has emerged as a potential therapeutic approach for IC (70, 71). The Endoplasmic Reticulum Lectin 1(ERLEC1) gene encodes a protein pivotal in the endoplasmic reticulum, particularly in protein quality control and folding. It aids in identifying proteins folding abnormally or with specific sugar groups, thereby participating in their subsequent processing and modification, including their targeting to appropriate pathways for degradation or repair (72). However, its association with inflammation and IC remains unclear. Ankyrin Repeat and SOCS Box Containing 3 (ASB3) is a protein-coding gene containing the SOCS box, facilitating the binding and degradation of various proteins. Members of the SOCS protein family are known to impede cytokine signaling by directly interacting with JAK kinase or activated cytokine receptors (73). Although ASB3 genes have been extensively studied in oncological disorders, such as promoting colorectal cancer cell growth and metastasis when mutated or down-regulated (74), their connection with inflammation and IC remains unelucidated. Signal Transducer and Activator of Transcription 5A (STAT5A) and Signal Transducer and Activator of Transcription 3 (STAT3) are pivotal transcription factors involved in various biological processes. Both are integral components of the Janus kinase/signal transducer and activator of transcription (JAK/STAT) signaling pathway, which plays a critical role in regulating cell growth, differentiation, survival, and immune response (75). Moreover, the JAK/STAT pathway modulates the production of numerous inflammatory mediators, thereby regulating inflammation development (76). There is now a degree of certainty that inflammation plays a key role in the pathophysiology of IC (77). A prior investigation observed significant enrichment of the JAK/STAT signaling pathway in a cyclophosphamide (CYP)-induced IC mouse model (78), where pSTAT3 expression increased post-CYP treatment in a rat cystitis model (79). Inhibition of the JAK/STAT signaling pathway demonstrated effectiveness in reducing CYP-induced inflammation in rat ulcerative cystitis (80). Hence, we hypothesize that gut microbiota may contribute to the onset and progression of IC inflammation by modulating the JAK/STAT signaling pathway, thereby influencing IC inflammation development. In conclusion, further studies are warranted to elucidate the mechanisms underlying the action of these genes in IC and their therapeutic potential.
IC, an autoimmune disease, is characterized by the infiltration of immune cells such as lymphocytes, macrophages, and mast cells into the bladder tissue of affected individuals. The activation and proliferation of these immune cells can lead to inflammation and damage to the bladder mucosa, resulting in symptoms such as pain, frequent urination, and urgency (81). Previous studies have shown that the development of IC involves the infiltration of various immune cells, including T cells, B cells, plasma cells, macrophages, neutrophils, and mast cells (81, 82). Growing evidence suggests that the gut microbiota plays a crucial role in shaping the immune system. First, the gut microbiota influences the systemic immune response by regulating intestinal barrier function. A healthy microbiota maintains the integrity of the intestinal barrier, preventing the invasion of harmful substances and pathogens, thereby reducing systemic inflammation. Conversely, an imbalanced gut microbiota can lead to barrier disruption, loss of immune tolerance, and chronic inflammation, which can affect autoimmune responses (83). Second, the gut microbiota directly impacts immune cell function through the production of various metabolites, such as SCFAs. For example, SCFAs produced from the metabolism of dietary fibers stimulate oxidative phosphorylation and glycolytic activity in CD8+ T cells, enhancing their effector functions. SCFAs also activate glycolysis in B cells via mTOR signaling (84). These metabolites can influence the immune cells in the bladder through the bloodstream. Moreover, a key role of the gut microbiota in the immune system is the promotion of immune tolerance. The immune system must recognize and attack pathogenic microbes while tolerating the normal microbiota. The gut microbiota assists in distinguishing harmful microbes from benign ones by producing signaling molecules and regulating immune cell functions. This helps prevent autoimmune diseases, although dysbiosis can disrupt immune tolerance and trigger autoimmune reactions (85). Looking ahead, integrating GWAS summary statistics on interstitial cystitis with single-cell RNA sequencing data (86), or employing new analytical methods, such as ‘scPagwas’ (87), could provide deeper insights into the immune cell heterogeneity in the bladder tissue of IC patients and elucidate the specific roles of these cells in IC pathogenesis. In summary, the interaction between the gut microbiota and the immune system is pivotal in the pathogenesis of IC. Understanding how the gut microbiota affects immune cells and their roles in IC could offer new insights for the diagnosis and treatment of this condition.
This study has several strengths. By employing MR analysis, we established a causal relationship between gut microbiota and IC, thereby controlling for confounding factors and reverse causation. Additionally, we utilized the cML-MA method to address biases due to correlated and uncorrelated pleiotropy and applied a two-sample MR design with non-overlapping exposure and outcome summary data to mitigate bias. While most current research focuses on analyses at the genus level, our study is the first to identify bacteria closely related to IC at the species level, specifically Desulfovibrio piger, Oscillibacter unclassified, and Ruminococcus lactaris. Identifying these species provides new insights into the gut microbiota’s role in IC. Future research should explore the potential of regulating these key bacterial taxa through fecal microbiota transplantation or probiotic treatments to alleviate IC symptoms. Increasing the abundance of Desulfovibrio piger, Oscillibacter unclassified, and Ruminococcus lactaris could offer effective intervention targets. For instance, enhancing the abundance of Desulfovibrio piger has been shown to positively influence the growth and function of the gut probiotic Faecalibacterium prausnitzii, suggesting new possibilities for personalized IC treatment. These new findings not only enhance our understanding of the etiology of IC but also provide clear directions for developing new clinical treatment strategies, particularly through microbiota modulation for symptom management. Such strategies may involve targeted supplementation or suppression of specific bacterial species, offering new therapeutic hope for IC patients. Additionally, we analyzed the associations between host genes and the microbiota, further exploring potential therapeutic targets and mechanisms underlying IC pathogenesis.
However, our study has several limitations. Firstly, the majority of patients in the GWAS summary data used were of European descent, with limited representation from other ethnicities. This imbalance may introduce estimation bias and limit the generalizability of our findings. Consequently, the results may not be fully applicable to individuals of non-European origin. Future MR studies exploring the causal relationship between gut microbiota and IC should include diverse European and non-European populations to enhance generalizability. Secondly, subgroup analyses, such as with Hunner-type and non-Hunner-type, were not possible since summary data, rather than raw data, were used in the analyses, whereas symptoms are usually more severe in the former (88). Moreover, IC predominantly affects the female population, and gender-specific differences exist in gut microbiota composition (89). Unfortunately, our study did not conduct separate analyses for both genders. Future investigations should incorporate gender-specific MR analysis to provide comprehensive insights into the relationship between gut microbiota and IC across different populations. Then, although relaxing the P-value threshold increased the number of IVs, it may have introduced additional false positives. Employing a stringent LD threshold (r² < 0.001) to ensure SNP independence might have excluded some effective SNPs. Excluding SNPs with a MAF≤ 0.01 improved the stability of effect estimates but may have omitted biologically relevant low-frequency variants. While removing palindromic SNPs reduced data errors, it might have overlooked critical genetic signals. Future research should focus on optimizing SNP selection criteria, exploring different P-value and LD thresholds, using updated reference panels, expanding sample sizes, and employing advanced techniques to manage palindromic SNPs, thereby enhancing the reliability and comprehensiveness of research findings. Finally, our study has a relatively small sample size, with only 240 cases of IC, which is indeed a limitation and may affect statistical power. Although we conducted rigorous analyses and used the largest available dataset, we recognize that the small sample size might impact the robustness of our conclusions. To address this, we employed F-statistic calculations to assess statistical power, with instrumental variables yielding results greater than 10, indicating statistical significance. Additionally, we performed sensitivity analyses, all of which support the reliability of our findings. Nevertheless, we understand that using larger datasets in future studies is essential for improving statistical power and validating our results. Despite the limited sample size, we believe our study provides a valuable foundation for exploring the potential associations between gut microbiota and interstitial cystitis, guiding future research in this field. We once again thank the reviewer for their valuable comments, and we look forward to addressing the sample size issue in future research to further enhance our contribution to this field.
Our study suggests a potential causal relationship between gut microbiota and IC based on MR analysis, biological annotation, and bulk RNA data analysis. However, these conclusions are primarily derived from predictive analysis tools and lack experimental validation. Future research should integrate in vivo and in vitro experiments to confirm the actual impact of relevant gut microbiota species and their metabolites on IC. Additionally, experimental validation is required to verify whether the key genes and bacteria identified in the analysis are genuinely involved in the pathogenesis of IC. Such validation will contribute to a more comprehensive understanding of the role of gut microbiota in IC and further substantiate the proposed mechanisms, providing a more reliable scientific foundation for the prevention and treatment of IC.
In this study, we employed a MR design to investigate the association between gut microbiota and IC, marking the first exploration of its kind. Through this approach, we successfully pinpointed gut microbiota linked to IC, comprising both beneficial and harmful microbial communities, thus serving as potential biomarkers. Furthermore, leveraging FUMA, we annotated SNPs to discern the genes harboring these genetic variants, indicating a potential role of gut microbiota in IC pathogenesis through gene regulation. Additionally, by comparing the expression profiles of hub genes in healthy individuals and IC patients using bulk RNA sequencing data, we potentially identified diagnostic and prognostic biomarkers associated with IC. Overall, this study offers a novel avenue for delving into the underlying pathogenesis of IC, providing insights for the development of personalized therapeutic strategies and identifying preliminary targets for future drug development.
The original contributions presented in the study are included in the article/Supplementary Material. Further inquiries can be directed to the corresponding authors.
CF: Writing – original draft, Visualization, Software, Methodology, Formal analysis, Data curation, Conceptualization. YZ: Writing – original draft, Software, Formal analysis, Data curation, Conceptualization. XZ: Writing – original draft, Formal analysis, Data curation, Conceptualization. JL: Writing – original draft, Investigation, Data curation. SJ: Writing – original draft, Investigation, Data curation. YHZ: Writing – original draft, Investigation, Data curation. FL: Writing – review & editing, Validation, Supervision, Resources, Project administration. NF: Writing – review & editing, Validation, Supervision, Resources, Project administration, Funding acquisition.
The author(s) declare financial support was received for the research, authorship, and/or publication of this article. This work was supported by the National Natural Science Foundation of China (Grant No. 82370777).
Thanks to the public databases for providing us with data, and thanks to the developers of R software and R packages for their contributions and convenience.
The authors declare that the research was conducted in the absence of any commercial or financial relationships that could be construed as a potential conflict of interest.
All claims expressed in this article are solely those of the authors and do not necessarily represent those of their affiliated organizations, or those of the publisher, the editors and the reviewers. Any product that may be evaluated in this article, or claim that may be made by its manufacturer, is not guaranteed or endorsed by the publisher.
The Supplementary Material for this article can be found online at: https://www.frontiersin.org/articles/10.3389/fimmu.2024.1395580/full#supplementary-material
IC, Interstitial cystitis; BP S, Bladder pain syndrome; GWAS, Genome-wide association study; DMP, Dutch microbiome project; SNPs, Single nucleotide polymorphisms; MAF, Minor allele frequencies; cML-MA, A constrained maximum likelihood and model averaging based MR method; IVs, Instrument variables; IVW, Inverse-variance weighted; LD, Linkage disequilibrium; eQTL, Expression quantitative trait locus; GO, Gene Ontology; GEO, Gene Expression Omnibus; MR, Mendelian randomization; OR, Odds ratio; CI, Confidence interval; MR-PRESSO, MR pleiotropy residual sum and outlier; MCC, Maximal clique centrality; DEGs, Differentially expressed genes; SE, Standard errors; H2S, Hydrogen sulphide; SCFAs, Short-chain fatty acids; OS, Oxidative stress; SPTBN1 , Spectrin beta, non-erythrocytic 1; PSME4, Proteasome activator subunit 4; CHAC2 , Chac glutathione specific gamma-glutamylcyclotransferase 2; ERLEC1 , Endoplasmic reticulum lectin 1; ASB3, Ankyrin repeat and SOCS box containing 3; JAK/STAT, Janus kinase/signal transducer and activator of transcription; STAT5A , Signal transducer and activator of transcription 5A; STAT3 , Signal transducer and activator of transcription 3.
1. van de Merwe JP, Nordling J, Bouchelouche P, Bouchelouche K, Cervigni M, Daha LK, et al. Diagnostic criteria, classification, and nomenclature for painful bladder syndrome/interstitial cystitis: an ESSIC proposal. Eur Urol. (2008) 53:60–7. doi: 10.1016/j.eururo.2007.09.019
2. Davis NF, Brady CM, Creagh T. Interstitial cystitis/painful bladder syndrome: epidemiology, pathophysiology and evidence-based treatment options. Eur J Obstetrics Gynecology Reprod Biol. (2014) 175:30–7. doi: 10.1016/j.ejogrb.2013.12.041
3. Berry SH, Elliott MN, Suttorp M, Bogart LM, Stoto MA, Eggers P, et al. Prevalence of symptoms of bladder pain syndrome/interstitial cystitis among adult females in the United States. J Urol. (2011) 186:540–4. doi: 10.1016/j.juro.2011.03.132
4. Masterson JM, Castañeda PR, Kim J. Pathophysiology and clinical biomarkers in interstitial cystitis. Urologic Clinics North America. (2023) 50:39–52. doi: 10.1016/j.ucl.2022.09.006
5. Tripp DA, Dueck J, Holden RR, Moreau J, Doiron RC, Nickel JC. Biopsychosocial predictors of suicide risk in patients with interstitial cystitis/bladder pain syndrome. CUAJ. (2021) 15:E630–6. doi: 10.5489/cuaj.7337
6. Jandhyala SM. Role of the normal gut microbiota. WJG. (2015) 21:8787. doi: 10.3748/wjg.v21.i29.8787
7. Stachowska E, Wi M, Dzie A, Bohatyrewicz A. Could the use of butyric acid have a positive effect on microbiota and treatment of type 2 diabetes? Eur Rev Med Pharmacol Sci. (2021) 25(13):4570–8. doi: 10.26355/eurrev_202107_26250
8. Cammarota G, Ianiro G, Cianci R, Bibbò S, Gasbarrini A, Currò D. The involvement of gut microbiota in inflammatory bowel disease pathogenesis: Potential for therapy. Pharmacol Ther. (2015) 149:191–212. doi: 10.1016/j.pharmthera.2014.12.006
9. Zhao M, Chu J, Feng S, Guo C, Xue B, He K, et al. Immunological mechanisms of inflammatory diseases caused by gut microbiota dysbiosis: A review. Biomedicine Pharmacotherapy. (2023) 164:114985. doi: 10.1016/j.biopha.2023.114985
10. Salazar AM, Neugent ML, De Nisco NJ, Mysorekar IU. Gut-bladder axis enters the stage: Implication for recurrent urinary tract infections. Cell Host Microbe. (2022) 30:1066–9. doi: 10.1016/j.chom.2022.07.008
11. Worby CJ, Schreiber HL, Straub TJ. Longitudinal multi-omics analyses link gut microbiome dysbiosis with recurrent urinary tract infections in women. Nat Microbiol. (2022) 7(5):630–9. doi: 10.1038/s41564-022-01107-x
12. Rahman-Enyart A, Yang W, Yaggie RE, White BA, Welge M, Auvil L, et al. Acyloxyacyl hydrolase is a host determinant of gut microbiome-mediated pelvic pain. Am J Physiology-Regulatory Integr Comp Physiol. (2021) 321:R396–412. doi: 10.1152/ajpregu.00106.2021
13. Braundmeier-Fleming A, Russell NT, Yang W, Nas MY, Yaggie RE, Berry M, et al. Stool-based biomarkers of interstitial cystitis/bladder pain syndrome. Sci Rep. (2016) 6:26083. doi: 10.1038/srep26083
14. Kurilshikov A, Medina-Gomez C, Bacigalupe R, Radjabzadeh D, Wang J, Demirkan A, et al. Large-scale association analyses identify host factors influencing human gut microbiome composition. Nat Genet. (2021) 53:156–65. doi: 10.1038/s41588-020-00763-1
15. Lopera-Maya EA, Kurilshikov A, van der Graaf A, Hu S, Andreu-Sánchez S, Chen L, et al. Effect of host genetics on the gut microbiome in 7,738 participants of the Dutch Microbiome Project. Nat Genet. (2022) 54:143–51. doi: 10.1038/s41588-021-00992-y
16. Jiang L, Zheng Z, Fang H, Yang J. A generalized linear mixed model association tool for biobank-scale data. Nat Genet. (2021) 53:1616–21. doi: 10.1038/s41588-021-00954-4
17. Skrivankova VW, Richmond RC, Woolf BAR, Davies NM, Swanson SA, VanderWeele TJ, et al. Strengthening the reporting of observational studies in epidemiology using mendelian randomisation (STROBE-MR): explanation and elaboration. BMJ. (2021) 375:n2233. doi: 10.1136/bmj.n2233
18. Skrivankova VW, Richmond RC, Woolf BAR, Yarmolinsky J, Davies NM, Swanson SA, et al. Strengthening the reporting of observational studies in epidemiology using mendelian randomization: the STROBE-MR statement. JAMA. (2021) 326:1614. doi: 10.1001/jama.2021.18236
19. Sanna S, Van Zuydam NR, Mahajan A, Kurilshikov A, Vich Vila A, Võsa U, et al. Causal relationships among the gut microbiome, short-chain fatty acids and metabolic diseases. Nat Genet. (2019) 51:600–5. doi: 10.1038/s41588-019-0350-x
20. Long Y, Tang L, Zhou Y, Zhao S, Zhu H. Causal relationship between gut microbiota and cancers: a two-sample Mendelian randomisation study. BMC Med. (2023) 21:66. doi: 10.1186/s12916-023-02761-6
21. Palmer TM, Lawlor DA, Harbord RM, Sheehan NA, Tobias JH, Timpson NJ, et al. Using multiple genetic variants as instrumental variables for modifiable risk factors. Stat Methods Med Res. (2012) 21:223–42. doi: 10.1177/0962280210394459
22. Levin MG, Judy R, Gill D, Vujkovic M, Verma SS, Bradford Y, et al. Genetics of height and risk of atrial fibrillation: A Mendelian randomization study. PloS Med. (2020) 17:e1003288. doi: 10.1371/journal.pmed.1003288
23. Gill D, Efstathiadou A, Cawood K, Tzoulaki I, Dehghan A. Education protects against coronary heart disease and stroke independently of cognitive function: evidence from Mendelian randomization. Int J Epidemiol. (2019) 48:1468–77. doi: 10.1093/ije/dyz200
24. Burgess S, Davies NM, Thompson SG. Bias due to participant overlap in two-sample Mendelian randomization. Genet Epidemiol. (2016) 40:597–608. doi: 10.1002/gepi.21998
25. Burgess S, Small DS, Thompson SG. A review of instrumental variable estimators for Mendelian randomization. Stat Methods Med Res. (2017) 26:2333–55. doi: 10.1177/0962280215597579
26. Burgess S, Butterworth A, Thompson SG. Mendelian randomization analysis with multiple genetic variants using summarized data. Genet Epidemiol. (2013) 37:658–65. doi: 10.1002/gepi.21758
27. Hartwig FP, Davey Smith G, Bowden J. Robust inference in summary data Mendelian randomization via the zero modal pleiotropy assumption. Int J Epidemiol. (2017) 46:1985–98. doi: 10.1093/ije/dyx102
28. Bowden J, Davey Smith G, Burgess S. Mendelian randomization with invalid instruments: effect estimation and bias detection through Egger regression. Int J Epidemiol. (2015) 44:512–25. doi: 10.1093/ije/dyv080
29. Bowden J, Davey Smith G, Haycock PC, Burgess S. Consistent estimation in mendelian randomization with some invalid instruments using a weighted median estimator. Genet Epidemiol. (2016) 40:304–14. doi: 10.1002/gepi.21965
30. Xue H, Shen X, Pan W. Constrained maximum likelihood-based Mendelian randomization robust to both correlated and uncorrelated pleiotropic effects. Am J Hum Genet. (2021) 108:1251–69. doi: 10.1016/j.ajhg.2021.05.014
31. Verbanck M, Chen C-Y, Neale B, Do R. Detection of widespread horizontal pleiotropy in causal relationships inferred from Mendelian randomization between complex traits and diseases. Nat Genet. (2018) 50:693–8. doi: 10.1038/s41588-018-0099-7
32. Greco M FD, Minelli C, Sheehan NA, Thompson JR. Detecting pleiotropy in Mendelian randomisation studies with summary data and a continuous outcome. Stat Med. (2015) 34:2926–40. doi: 10.1002/sim.6522
33. Bowden J, Del Greco MF, Minelli C, Zhao Q, Lawlor DA, Sheehan NA, et al. Improving the accuracy of two-sample summary-data Mendelian randomization: moving beyond the NOME assumption. Int J Epidemiol. (2019) 48:728–42. doi: 10.1093/ije/dyy258
34. Hemani G, Tilling K, Davey Smith G. Orienting the causal relationship between imprecisely measured traits using GWAS summary data. PloS Genet. (2017) 13:e1007081. doi: 10.1371/journal.pgen.1007081
35. Watanabe K, Taskesen E, Van Bochoven A, Posthuma D. Functional mapping and annotation of genetic associations with FUMA. Nat Commun. (2017) 8:1826. doi: 10.1038/s41467-017-01261-5
36. Xiao L, Liu S, Wu Y, Huang Y, Tao S, Liu Y, et al. The interactions between host genome and gut microbiome increase the risk of psychiatric disorders: Mendelian randomization and biological annotation. Brain Behavior Immun. (2023) 113:389–400. doi: 10.1016/j.bbi.2023.08.003
37. Szklarczyk D, Gable AL, Nastou KC, Lyon D, Kirsch R, Pyysalo S, et al. The STRING database in 2021: customizable protein–protein networks, and functional characterization of user-uploaded gene/measurement sets. Nucleic Acids Res. (2021) 49:D605–12. doi: 10.1093/nar/gkaa1074
38. Bu D, Luo H, Huo P, Wang Z, Zhang S, He Z, et al. KOBAS-i: intelligent prioritization and exploratory visualization of biological functions for gene enrichment analysis. Nucleic Acids Res. (2021) 49:W317–25. doi: 10.1093/nar/gkab447
39. Chin C-H, Chen S-H, Wu H-H, Ho C-W, Ko M-T, Lin C-Y. cytoHubba: identifying hub objects and sub-networks from complex interactome. BMC Syst Biol. (2014) 8:S11. doi: 10.1186/1752-0509-8-S4-S11
40. Gamper M, Viereck V, Geissbühler V, Eberhard J, Binder J, Moll C, et al. Gene expression profile of bladder tissue of patients with ulcerative interstitial cystitis. BMC Genomics. (2009) 10:199. doi: 10.1186/1471-2164-10-199
41. Yarmolinsky J, Bouras E, Constantinescu A, Burrows K, Bull CJ, Vincent EE, et al. Genetically proxied glucose-lowering drug target perturbation and risk of cancer: a Mendelian randomisation analysis. Diabetologia. (2023) 66:1481–500. doi: 10.1007/s00125-023-05925-4
42. Su Q, Jin C, Bo Z, Yang Y, Wang J, Wang J, et al. Association between gut microbiota and gastrointestinal cancer: a two-sample bi-directional Mendelian randomization study. Front Microbiol. (2023) 14:1181328. doi: 10.3389/fmicb.2023.1181328
43. Rey FE, Gonzalez MD, Cheng J, Wu M, Ahern PP, Gordon JI. Metabolic niche of a prominent sulfate-reducing human gut bacterium. Proc Natl Acad Sci USA. (2013) 110:13582–7. doi: 10.1073/pnas.1312524110
44. Fiorucci S, Antonelli E, Distrutti E, Rizzo G, Mencarelli A, Orlandi S, et al. Inhibition of hydrogen sulfide generation contributes to gastric injury caused by anti-inflammatory nonsteroidal drugs. Gastroenterology. (2005) 129:1210–24. doi: 10.1053/j.gastro.2005.07.060
45. Schicho R, Krueger D, Zeller F, Von Weyhern CWH, Frieling T, Kimura H, et al. Hydrogen sulfide is a novel prosecretory neuromodulator in the Guinea-pig and human colon. Gastroenterology. (2006) 131:1542–52. doi: 10.1053/j.gastro.2006.08.035
46. Wallace JL, Vong L, McKnight W, Dicay M, Martin GR. Endogenous and exogenous hydrogen sulfide promotes resolution of colitis in rats. Gastroenterology. (2009) 137:569–578.e1. doi: 10.1053/j.gastro.2009.04.012
47. Pitcher MCL. The contribution of sulphate reducing bacteria and 5-aminosalicylic acid to faecal sulphide in patients with ulcerative colitis. Gut. (2000) 46:64–72. doi: 10.1136/gut.46.1.64
48. Tomasova L, Konopelski P, Ufnal M. Gut bacteria and hydrogen sulfide: the new old players in circulatory system homeostasis. Molecules. (2016) 21:1558. doi: 10.3390/molecules21111558
49. Khan MT, Dwibedi C, Sundh D, Pradhan M, Kraft JD, Caesar R, et al. Synergy and oxygen adaptation for development of next-generation probiotics. Nature. (2023) 620:381–5. doi: 10.1038/s41586-023-06378-w
50. Lopez-Siles M, Duncan SH, Garcia-Gil LJ, Martinez-Medina M. Faecalibacterium prausnitzii : from microbiology to diagnostics and prognostics. ISME J. (2017) 11:841–52. doi: 10.1038/ismej.2016.176
51. Hall AB, Yassour M, Sauk J, Garner A, Jiang X, Arthur T, et al. A novel Ruminococcus gnavus clade enriched in inflammatory bowel disease patients. Genome Med. (2017) 9:103. doi: 10.1186/s13073-017-0490-5
52. Henke MT, Brown EM, Cassilly CD, Vlamakis H, Xavier RJ, Clardy J. Capsular polysaccharide correlates with immune response to the human gut microbe Ruminococcus gnavus. Proc Natl Acad Sci USA. (2021) 118:e2007595118. doi: 10.1073/pnas.2007595118
53. Baron EJ, Curren M, Henderson G, Lee K, Lechowitz K, Strong CA, et al. Bilophila wadsworthia isolates from clinical specimens. J Clin Microbiol. (1992) 30(7):1882–4. doi: 10.1128/jcm.30.7.1882-1884.1992
54. Natividad JM, Lamas B, Pham HP, Michel M-L, Rainteau D, Bridonneau C, et al. Bilophila wadsworthia aggravates high fat diet induced metabolic dysfunctions in mice. Nat Commun. (2018) 9(1):2802. doi: 10.1038/s41467-018-05249-7
55. Hanson BT, Dimitri Kits K, Löffler J, Burrichter AG, Fiedler A, Denger K, et al. Sulfoquinovose is a select nutrient of prominent bacteria and a source of hydrogen sulfide in the human gut. ISME J. (2021) 15:2779–91. doi: 10.1038/s41396-021-00968-0
56. Vinolo MAR, Rodrigues HG, Nachbar RT, Curi R. "Modulation of inflammatory and immune responses by short-chain fatty acids". In: Diet, immunity and inflammation. Woodhead Publishing (2013). p. 435–58. doi: 10.1533/9780857095749.3.435
57. Mann ER, Lam YK, Uhlig HH. Short-chain fatty acids: linking diet, the microbiome and immunity. Nat Rev Immunol. (2024) 24:577–95. doi: 10.1038/s41577-024-01014-8
58. Louis P, Flint HJ. Diversity, metabolism and microbial ecology of butyrate-producing bacteria from the human large intestine. FEMS Microbiol Lett. (2009) 294:1–8. doi: 10.1111/j.1574-6968.2009.01514.x
59. Camp JG, Frank CL, Lickwar CR, Guturu H, Rube T, Wenger AM, et al. Microbiota modulate transcription in the intestinal epithelium without remodeling the accessible chromatin landscape. Genome Res. (2014) 24:1504–16. doi: 10.1101/gr.165845.113
60. Pan W-H, Sommer F, Falk-Paulsen M, Ulas T, Best P, Fazio A, et al. Exposure to the gut microbiota drives distinct methylome and transcriptome changes in intestinal epithelial cells during postnatal development. Genome Med. (2018) 10:27. doi: 10.1186/s13073-018-0534-5
61. Davison JM, Lickwar CR, Song L, Breton G, Crawford GE, Rawls JF. Microbiota regulate intestinal epithelial gene expression by suppressing the transcription factor Hepatocyte nuclear factor 4 alpha. Genome Res. (2017) 27:1195–206. doi: 10.1101/gr.220111.116
62. Cousin MA, Creighton BA, Breau KA, Spillmann RC, Torti E, Dontu S, et al. Pathogenic SPTBN1 variants cause an autosomal dominant neurodevelopmental syndrome. Nat Genet. (2021) 53:1006–21. doi: 10.1038/s41588-021-00886-z
63. Hanno PM, Erickson D, Moldwin R, Faraday MM. Diagnosis and treatment of interstitial cystitis/bladder pain syndrome: AUA guideline amendment. J Urol. (2015) 193:1545–53. doi: 10.1016/j.juro.2015.01.086
64. Homma Y, Akiyama Y, Tomoe H, Furuta A, Ueda T, Maeda D, et al. Clinical guidelines for interstitial cystitis/bladder pain syndrome. Int J Urol. (2020) 27:578–89. doi: 10.1111/iju.14234
65. Belknap S, Blalock E, Erickson D. The challenges of interstitial cystitis: current status and future prospects. Drugs. (2015) 75:2057–63. doi: 10.1007/s40265-015-0504-9
66. Javitt A, Shmueli MD, Kramer MP, Kolodziejczyk AA, Cohen IJ, Radomir L, et al. The proteasome regulator PSME4 modulates proteasome activity and antigen diversity to abrogate antitumor immunity in NSCLC. Nat Cancer. (2023) 4:629–47. doi: 10.1038/s43018-023-00557-4
67. Bachhawat AK, Kaur A. Glutathione degradation. Antioxidants Redox Signaling. (2017) 27:1200–16. doi: 10.1089/ars.2017.7136
68. Wang C-K, Yang S-C, Hsu S-C, Chang F-P, Lin Y-T, Chen S-F, et al. CHAC2 is essential for self-renewal and glutathione maintenance in human embryonic stem cells. Free Radical Biol Med. (2017) 113:439–51. doi: 10.1016/j.freeradbiomed.2017.10.345
69. Mohammad A, Laboulaye MA, Shenhar C, Dobberfuhl AD. Mechanisms of oxidative stress in interstitial cystitis/bladder pain syndrome. Nat Rev Urol. (2024) 21:433–49. doi: 10.1038/s41585-023-00850-y
70. D’Amico R, Salinaro AT, Cordaro M, Fusco R, Impellizzeri D, Interdonato L, et al. Hidrox® and chronic cystitis: biochemical evaluation of inflammation, oxidative stress, and pain. Antioxidants (Basel). (2021) 10(7):1046. doi: 10.3390/antiox10071046
71. Li J, Luo H, Dong X, Liu Q, Wu C, Zhang T, et al. Therapeutic effect of urine-derived stem cells for protamine/lipopolysaccharide-induced interstitial cystitis in a rat model. Stem Cell Res Ther. (2017) 8:107. doi: 10.1186/s13287-017-0547-9
72. Cruciat C-M, Hassler C, Niehrs C. The MRH protein erlectin is a member of the endoplasmic reticulum synexpression group and functions in N-glycan recognition. J Biol Chem. (2006) 281:12986–93. doi: 10.1074/jbc.M511872200
73. Kile BT, Viney EM, Willson TA, Brodnicki TC, Cancilla MR, Herlihy AS, et al. Cloning and characterization of the genes encoding the ankyrin repeat and SOCS box-containing proteins Asb-1, Asb-2, Asb-3 and Asb-4. Gene. (2000) 258:31–41. doi: 10.1016/S0378-1119(00)00402-9
74. Du W-Y, Lu Z-H, Ye W, Fu X, Zhou Y, Kuang C-M, et al. The loss-of-function mutations and down-regulated expression of ASB3 gene promote the growth and metastasis of colorectal cancer cells. Chin J Cancer. (2017) 36:11. doi: 10.1186/s40880-017-0180-0
75. Ott N, Faletti L, Heeg M, Andreani V, Grimbacher B. JAKs and STATs from a clinical perspective: loss-of-function mutations, gain-of-function mutations, and their multidimensional consequences. J Clin Immunol. (2023) 43:1326–59. doi: 10.1007/s10875-023-01483-x
76. Banerjee S, Biehl A, Gadina M, Hasni S, Schwartz DM. JAK–STAT signaling as a target for inflammatory and autoimmune diseases: current and future prospects. Drugs. (2017) 77:521–46. doi: 10.1007/s40265-017-0701-9
77. Offiah I, Didangelos A, Dawes J, Cartwright R, Khullar V, Bradbury EJ, et al. The expression of inflammatory mediators in bladder pain syndrome. Eur Urol. (2016) 70:283–90. doi: 10.1016/j.eururo.2016.02.058
78. Peskar D, Kuret T, Lakota K, Erman A. Molecular profiling of inflammatory processes in a mouse model of IC/BPS: from the complete transcriptome to major sex-related histological features of the urinary bladder. IJMS. (2023) 24:5758. doi: 10.3390/ijms24065758
79. Cheppudira BP, Girard BM, Malley SE, Dattilio A, Schutz KC, May V, et al. Involvement of JAK-STAT signaling/function after cyclophosphamide-induced bladder inflammation in female rats. Am J Physiology-Renal Physiol. (2009) 297:F1038–44. doi: 10.1152/ajprenal.00110.2009
80. Song Y, Cao J, Jin Z, Hu W, Wu R, Tian L, et al. Inhibition of microRNA-132 attenuates inflammatory response and detrusor fibrosis in rats with interstitial cystitis via the JAK-STAT signaling pathway. J Cell Biochem. (2019) 120:9147–58. doi: 10.1002/jcb.28190
81. Peng L, Jin X, Li B, Zeng X, Liao B, Jin T, et al. Integrating single-cell RNA sequencing with spatial transcriptomics reveals immune landscape for interstitial cystitis. Sig Transduct Target Ther. (2022) 7:161. doi: 10.1038/s41392-022-00962-8
82. MacDermott JP, Miller CH, Levy N, Stone AR. Cellular immunity in interstitial cystitis. J Urol. (1991) 145:274–8. doi: 10.1016/s0022-5347(17)38313-1
83. da Fonseca DM, Hand TW, Han S-J, Gerner MY, Zaretsky AG, Byrd AL, et al. Microbiota-dependent sequelae of acute infection compromise tissue-specific immunity. Cell. (2015) 163:354–66. doi: 10.1016/j.cell.2015.08.030
84. Michaudel C, Sokol H. The gut microbiota at the service of immunometabolism. Cell Metab. (2020) 32:514–23. doi: 10.1016/j.cmet.2020.09.004
85. Wu H-J, Wu E. The role of gut microbiota in immune homeostasis and autoimmunity. Gut Microbes. (2012) 3:4–14. doi: 10.4161/gmic.19320
86. Ma Y, Qiu F, Deng C, Li J, Huang Y, Wu Z, et al. Integrating single-cell sequencing data with GWAS summary statistics reveals CD16+monocytes and memory CD8+T cells involved in severe COVID-19. Genome Med. (2022) 14:16. doi: 10.1186/s13073-022-01021-1
87. Ma Y, Deng C, Zhou Y, Zhang Y, Qiu F, Jiang D, et al. Polygenic regression uncovers trait-relevant cellular contexts through pathway activation transformation of single-cell RNA sequencing data. Cell Genomics. (2023) 3:100383. doi: 10.1016/j.xgen.2023.100383
88. Lai HH, Pickersgill NA, Vetter JM. Hunner lesion phenotype in interstitial cystitis/bladder pain syndrome: A systematic review and meta-analysis. J Urol. (2020) 204:518–23. doi: 10.1097/JU.0000000000001031
Keywords: Mendelian randomization study, interstitial cystitis, gut microbiota, gut-bladder axis, gene, causal relationship
Citation: Fu C, Zhao Y, Zhou X, Lv J, Jin S, Zhou Y, Liu F and Feng N (2024) Gut microbiota and interstitial cystitis: exploring the gut-bladder axis through mendelian randomization, biological annotation and bulk RNA sequencing. Front. Immunol. 15:1395580. doi: 10.3389/fimmu.2024.1395580
Received: 04 March 2024; Accepted: 10 September 2024;
Published: 27 September 2024.
Edited by:
Youcef Shahali, Centre Hospitalier Universitaire de Besançon, FranceReviewed by:
Maryam Dadar, Razi Vaccine and Serum Research Institute, IranCopyright © 2024 Fu, Zhao, Zhou, Lv, Jin, Zhou, Liu and Feng. This is an open-access article distributed under the terms of the Creative Commons Attribution License (CC BY). The use, distribution or reproduction in other forums is permitted, provided the original author(s) and the copyright owner(s) are credited and that the original publication in this journal is cited, in accordance with accepted academic practice. No use, distribution or reproduction is permitted which does not comply with these terms.
*Correspondence: Ninghan Feng, bi5mZW5nQG5qbXUuZWR1LmNu; Fengping Liu, bGl1bGFvc2hpeWNAMTYzLmNvbQ==
Disclaimer: All claims expressed in this article are solely those of the authors and do not necessarily represent those of their affiliated organizations, or those of the publisher, the editors and the reviewers. Any product that may be evaluated in this article or claim that may be made by its manufacturer is not guaranteed or endorsed by the publisher.
Research integrity at Frontiers
Learn more about the work of our research integrity team to safeguard the quality of each article we publish.