- 1Host-Pathogen-Interactions, Paul-Ehrlich-Institut, Langen, Germany
- 2TRON - Translational Oncology at the University Medical Center of the Johannes Gutenberg University gGmbH, Mainz, Germany
Cancer immunotherapy has witnessed rapid advancement in recent years, with a particular focus on neoantigens as promising targets for personalized treatments. The convergence of immunogenomics, bioinformatics, and artificial intelligence (AI) has propelled the development of innovative neoantigen discovery tools and pipelines. These tools have revolutionized our ability to identify tumor-specific antigens, providing the foundation for precision cancer immunotherapy. AI-driven algorithms can process extensive amounts of data, identify patterns, and make predictions that were once challenging to achieve. However, the integration of AI comes with its own set of challenges, leaving space for further research. With particular focus on the computational approaches, in this article we have explored the current landscape of neoantigen prediction, the fundamental concepts behind, the challenges and their potential solutions providing a comprehensive overview of this rapidly evolving field.
1 Introduction
Recently, there has been an increasing number of reports on promising treatment paradigms based on reactivation of the immune system against cancer cells. Cancer immunotherapies aim to counteract the tactics employed by tumors that deactivate the immune system. Nevertheless, solely reactivating the immune system is not enough for the thorough elimination of tumors. It is essential that the reactivated immune system can distinguish malignant cells from their healthy counterparts.
The immune recognition of tumor tissues primarily relies on tumor antigens. Short antigenic peptides derived from tumor antigens are presented on the surface of the tumor cell by major histocompatibility complex (MHC) molecules serving as targets for the antitumor immune response. In humans, the MHC-I and MHC-II proteins are encoded by Human Leukocyte Antigen (HLA) genes, which are polymorphic in the human population. Given that the tumor antigens are the major target for antitumor T cells, they play a pivotal role in effective tumor elimination. Tumor antigens are typically categorized as tumor-associated antigens (TAA) and tumor-specific antigens (TSA). TAAs include antigens derived from genes overexpressed in cancer cells due to their malignant transformation, and comprise a class of normal self-proteins that are minimally expressed by healthy tissues. TAAs are generally weakly immunogenic due to central immune tolerance mechanisms. In contrast, TSAs are expressed exclusively on tumor cells. Most TSAs are neoantigens resulting from somatic mutations, such as insertion or deletions (INDELs), single nucleotide variants (SNVs), frameshifts and gene fusions (1). Since these neoantigens are products of tumor-specific irregularities, they are less susceptible to central immune tolerance, making them suitable candidates for therapeutic targeting.
Neoantigen cancer vaccines have emerged as a novel clinical approach to treat cancer (2). The purpose of a personalized anticancer vaccine is to direct T cells towards tumor eradication by leveraging neoantigens while preserving healthy tissue. There are two broad categories of immunotherapy treatments. Vaccinating against cancer induces long-lasting de novo antitumor immunity and is termed active immunotherapy (3, 4). Adoptive cell therapy (ACT) approaches, such as adoptive transfer of tumor-infiltrating lymphocytes (TILs), transgenic T cells, or chimeric antigen receptor T cells are based on the in vitro generation of tumor-specific T cells with subsequent infusion to the patient (passive immunotherapy). Currently, there is a variety of clinical trials, testing neoantigen-based anticancer vaccines either independently or in conjunction with other immunotherapies, checkpoint inhibitors or novel drugs under investigation. Numerous articles comprehensively review the field of mutation-derived neoantigen cancer vaccines. For detailed insights into preclinical and clinical studies, we recommend the review of Aurisicchio et al. (5). The review paper of Shemesh et al. (6) presents the clinical trial landscape of personalized therapeutic cancer vaccines, highlighting their opportunities and emerging challenges. Further insights into the challenges associated with targeting cancer neoantigens are outlined in the work of Chen et al. (7). Designing neoantigen cancer vaccines, trials, and trial outcomes are described in Biswas et al.’s work (8).
Detection of neoantigens is crucial for developing personalized cancer immunotherapies. Currently artificial intelligence (AI) is widely used to assess the factors that shape tumor immunogenicity. The use of AI for neoantigen prediction enhances the accuracy, efficiency, and personalized nature of cancer immunotherapy development by effectively analyzing and interpreting complex genomic data. However, the identification of putative neoantigens from genomic data still remains a challenge. To address this, specialized software tools have been developed for specific sub-tasks such as HLA typing and in silico prediction of peptide binding affinity to MHC molecules. Complex pipelines that encompass multiple analytical tasks have also been created. Current strategies for the identification of neoantigens are extensively reviewed in multiple articles (9–11).
For the successful implementation of AI vast amount of data is required. Genomic data comes in various forms, such as DNA sequences, RNA expression profiles. AI models can be trained to handle diverse data types, allowing for a more comprehensive, fast analysis of the factors influencing neoantigen formation. Significant amounts of high-throughput biomedical data, including omics and immunological data, have been accumulated in public databases, and can be transformed into novel insights. These data can be used for training a model with AI - based computational algorithm to properly interpret the data and learn from it in order to make accurate decisions based on the input information provided (Figure 1). Additionally, AI models can help to identify novel neoantigens by recognizing patterns and associations in the molecular and cellular profiling data that may be challenging with the traditional methods.
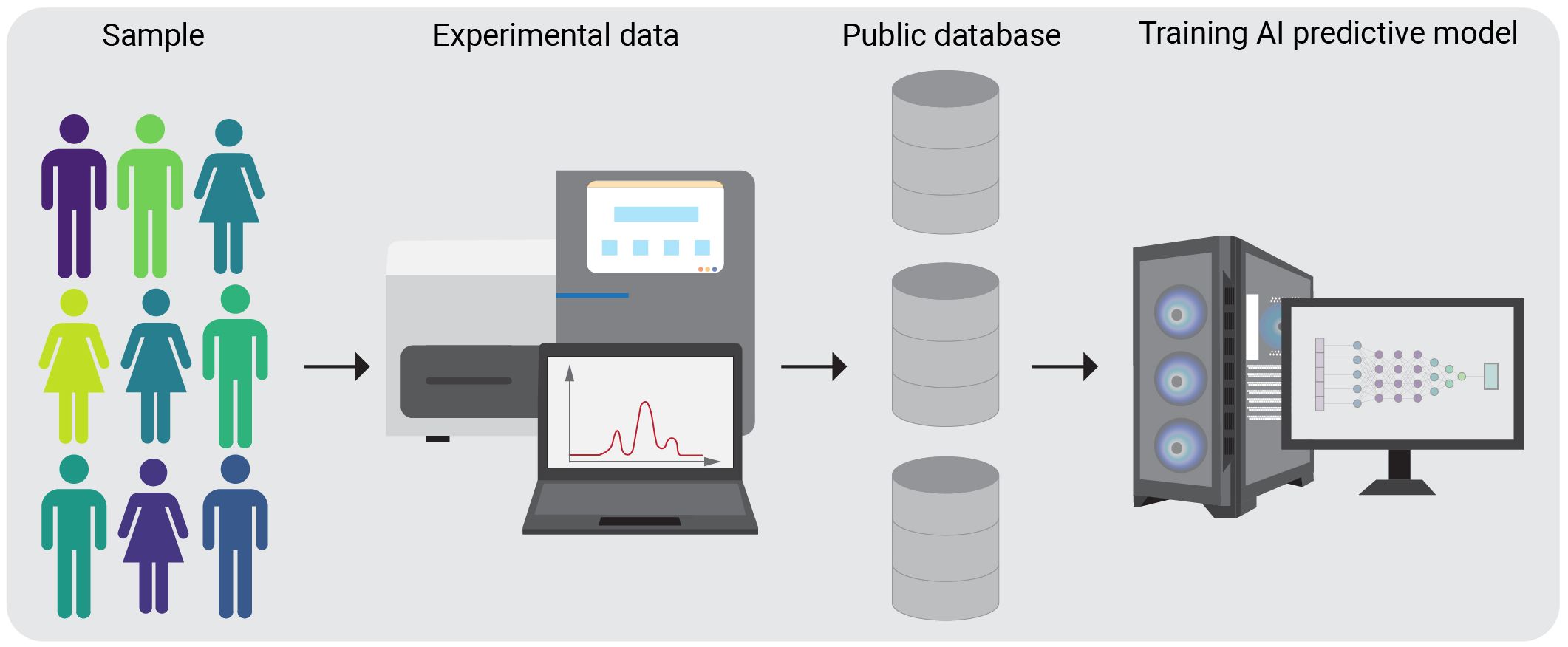
Figure 1 Schematic overview of AI algorithm training on public databases. A group of subjects, specific for the condition of interest is chosen for the experimental procedures. After completing the experimental pipelines, the generated data is stored in a public database. AI algorithms can then be trained on these datasets.
Most state-of-the-art computational approaches for ranking and selecting candidate neoantigens predominantly rely on prediction methods, rooted in conventional machine learning (ML) algorithms, including artificial neural networks (ANNs), and modern AI architectures, trained on large experimental datasets.
Artificial Neural Networks are computational models inspired by biological neural networks. They learn the relationship between the inputs and outputs using samples from the training dataset (e.g., peptide sequences) and make predictions for the new samples. ANN’s are optimized by adjusting their parameters (weights and biases) based on the difference between the predicted values and actual values, utilizing the error-correction-learning rule known as back propagation.
Deep Learning (DL), a subset of machine learning and artificial intelligence stemming from ANNs, has gained increasing attention over the past years. The most commonly applied architectures include deep neural networks (DNNs) and convolutional neural networks (CNNs). DNNs consist of an input layer, multiple hidden layers, and an output layer with nodes in adjacent layers fully interconnected. CNNs primarily feature convolutional and pooling layers, often followed by fully connected layers. For an in-depth understanding of deep learning principles and concepts, we recommend the book of Goodfellow et al. (12). For definitions of AI and DL-related terms, please refer to our AI glossary (Table 1).
Notable applications of deep learning in biomedicine, including medical imaging and drug discovery, are comprehensively covered in Wainberg et al. (13), while Wen et al. (14) delve into DL methods in proteomics.
Deep learning requires all input and output variables to be numeric. One important aspect of DL is data preprocessing or input encoding, which transforms raw data, such as peptide or protein sequences, into a suitable format for learning. Designing novel representation methods for protein sequence data is an active research direction. For example, the DeepLigand (15) approach treats each peptide sequence as a sentence, and each amino acid as a word, using the deep language model ELMo (16) to embed peptides into vector representations for tasks like peptide-MHC binding affinity prediction.
In addition to DNN and CNN, other DL architectures, such as gated recurrent unit (GRU) and long short-term memory (LSTM) neural networks, have proven effective for the peptide sequence-based prediction tasks. These methods can model dependences between amino acid residues within peptides of varying lengths without artificial lengthening or shortening, and they tend to be substantially faster than standard neural networks.
Recent advances in Natural Language Processing (NLP) have demonstrated the effectiveness of complex models, such as Transformers, including BERT (Bidirectional Encoder Representations from Transformers) (17), and GPT (Generative Pretrained Transformer) (Radford et al., 2018)1, in learning rich contextual word representations. They can be trained to understand semantics from text without labels (self-supervised learning) (18). Similar techniques have also been applied to learn features from a large corpus of protein sequence data from public datasets (19, 20).
Another important characteristic of DL is transfer learning, which involves initializing training with representations learned from a previous task. Instead of training a new network from scratch, pretrained models can be downloaded and further trained for a new task by adding additional layers or fine-tuned using the new data. Examples include BERTMHC (21), MHCRoBERTa (22) which use transformers and transfer learning for peptide-MHC binding prediction. The authors found that leveraging self-supervised pretraining on large protein sequence corpora can lead to improved performance, particularly when training data is limited.
Achieving optimal prediction accuracy requires the tuning of model settings, or hyperparameters, e.g. determining how fast the weights of NN should be adjusted during training. Hyperparameter search techniques use validation examples that are held out from training. We provide the reader with a helpful background for understanding approaches assessing the performance of AI systems and establishing the trust in it.
Numerous publications have explored the application of AI in cancer research, precision medicine (23), cancer immunotherapy (24), and neoantigen identification (25). To gauge the potential of AI-driven software solutions, several benchmarking studies have been conducted. Evaluating and comparing tools is an essential part for their future application in the medical field and everyday clinical practice, as no single approach is universally applicable and having a dependable predictor or genotyper is vital. Despite the continually improving performance, critical questions regarding the application of AI technology in cancer immunotherapy remain. In this review, we summarize the core neoantigen calling pipeline, the recent research progress, and discuss the potential of artificial intelligence-enabled neoantigen identification, along with its current limitations and challenges.
2 Computational hunting for neoantigens
The core computational pipeline established for the process of identification and selection of genomically encoded antigens that are of immunological significance includes the following steps (25):
Whole exome or genome sequencing (WES or WGS) data of tumor and matched normal DNA samples
Somatic mutation calling
Conversion of detected coding DNA somatic mutations to corresponding mutated peptide sequences
HLA-allele typing
Peptide prioritization, neoantigen calling
o Prediction of peptide-MHC binding affinity
o Prediction of T cell receptor (TCR) recognition, TCR binding affinity and T cell response
o Immunogenicity prediction
o Expression analysis of putative neoantigens, using e.g. RNA-seq data
The effective pattern recognition by AI allows for the development of personalized cancer treatments by considering the unique genomic profile of each patient’s tumor. As standard practice, neoantigens are predicted from the mutated peptides by assessing their ability to trigger an immune response. The development of AI-based prediction tools allows immunologists to streamline the search for neoantigen candidates that require experimental validation (Figure 2).
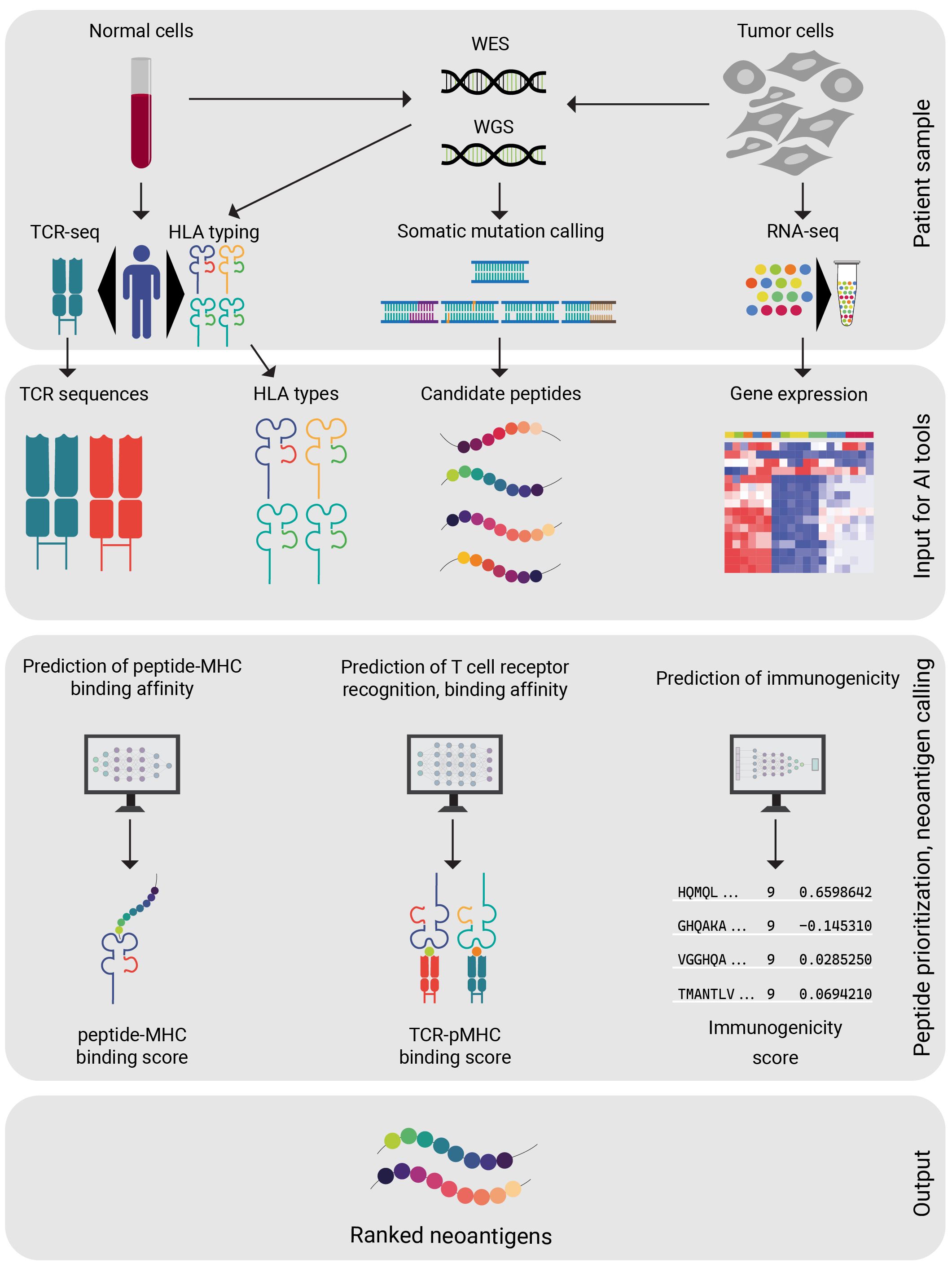
Figure 2 Steps of neoantigen selection from patient data. A set of diagnostic procedures are completed on patient derived samples. Ideally all of the above-mentioned patient data (WES, WGS, HLA typing, RNA-seq) are available before proceeding. After a candidate peptide selection is generated from the patient data, the AI model of preference is applied. The AI model will compute a ranked peptide list from the candidate peptides. Careful design of personalized vaccine is available, based on the peptide rankings.
In the following, we provide an overview of the most common computational methods used in the neoantigen identification pipeline and outline the challenges associated with the process.
2.1 Somatic mutation calling
The process of somatic mutation calling is well-established and includes several critical steps, such as quality control of sequencing reads, alignment to the reference genome, base quality recalibration and INDEL realignment, comparison of healthy and tumor alignments. For quality control of sequencing reads in a WES (or WGS) dataset, FastQC (26) is commonly used, and BWA (27) is a widely employed aligner. Base quality recalibration and INDEL realignment around clusters of putative somatic mutations are both integral tools of Genome Analysis Toolkit (GATK) (28). There are numerous somatic mutation callers available, including MuTect (29), Abra (30), Strelka (31), and VarScan (32). For best practices in variant calling in clinical sequencing, readers are referred to the work of Koboldt (33). A comprehensive overview of the variant calling tools and their pros and cons is provided in the paper of Cai et al. (25).
Various databases can be used for variant annotation, such as CancerHotspots (34), and the Catalogue Of Somatic Mutations In Cancer COSMIC (35). The Variant Interpretation for Cancer Consortium (VICC) has standardized the curation, representation, and interpretation of clinically-relevant evidence associated with genomic variation in cancers. VICC guidelines (36) can be used to classify variants in known cancer genes (37).
2.2 False-positive mutation calls
There is a possibility that an identified mutation may yield a false-positive result potentially leading to the treatment of a patient with a drug targeting a nonexistent somatic mutation. To mitigate clinical efficacy risk, mutation calls from DNA sequencing should be cross-verified with the results of replicate sequencing runs. Moreover, utilizing extra sequencing data, like RNA-seq from the same tumor sample, to identify somatic mutations and check for overlaps reduces false positives. Yet, it may raise the risk of false negatives due to transient gene expression and variable read coverage (38). Combining multiple somatic mutation callers has been observed to significantly reduce the false positive rate (39, 40).
2.3 Identified mutation is a SNP
There is a possibility that an identified mutation exists in both tumor and healthy (germline) cells, representing a single nucleotide polymorphism (SNP) rather than a somatic mutation. Deep sequencing of germline DNA samples is essential to identify potential SNPs with high sensitivity.
2.4 False-negative mutation calls
There is a possibility that variant calling may fail to detect a somatic mutation that could produce a highly immunogenic neoantigen. While this omission does not harm the patient directly, it can result in a missed candidate neoantigen for the vaccine. To minimize this risk, deep sequencing of DNA samples (typically ~200x) is recommended to ensure high coverage across the entire protein-coding region. Unlike germline testing, which typically requires a minimum of 30x coverage with balanced reads, the identification of somatic variants in tumor specimens demands significantly higher read depths. This necessity arises from the presence of tissue heterogeneity, encompassing malignant cells, supporting stromal cells, inflammatory cells, and contaminant tissue. Additionally, intra-tumoral heterogeneity, represented by various tumor subclones, and considerations of tumor viability further underscore the need for elevated coverage. In instances of low tumor cellularity in tissue specimens, achieving an average coverage of at least 1000x may be essential to confidently detect heterogeneous variants. Additionally, the option to include multiple targets (e.g., up to 20 candidate neoantigens) in an individual drug product should limit the impact of missed mutations.
2.5 Sources of cancer neoantigens beyond single-nucleotide variants
Emerging evidence suggests the existence of alternative sources of cancer neoantigens, such as alternative splicing variants (41), post-translational modifications (42), and transposable elements (1), and gene fusions (43). These alternative sources may serve as attractive novel targets for immunotherapy (44). Nevertheless, addressing the tumor-specificity still remains a challenge.
3 HLA-allele typing
HLA typing of the individual patient samples, specifically the accurate identification of the individual set of HLA alleles (HLA allotypes), is essential. Peptide-MHC affinity strongly depends on HLA alleles, resulting in distinct immune responses among individuals (45). Genotyping the class I genes HLA-A, -B and -C, as well as the class II genes HLA-DRB1, -DQB1, and -DPB1 presents a non-trivial task.
Sequence-based typing (SBT) based on Sanger sequencing can be used for HLA typing. However, due to certain limitations, such as the need for additional sequencing to identify cis/trans polymorphism, the concordance rate of Sanger sequencing-based HLA genotyping is approximately 84% among different laboratories (46). Commercial software, such as uTYPE (Life Technologies. Brown Deer, WI), Assign-SBT (Conexio, San Francisco, CA) (47), and SBTEngine (GenDx, Utrecht, Netherlands) (48), along with some open-source tools, e.g. SOAPTyping (49) are capable of producing predictions from Sanger sequencing data. However, they are increasingly being replaced by NGS-based methods. High-throughput WES and RNA-seq sequencing data also serve as a foundation for HLA typing. Most HLA genotyping tools take NGS sequencing data as the input and output HLA types. The algorithms employed by the tools primarily differ in how they map sequencing reads to a panel of reference HLA allele sequences and the strategy they use to subsequently score candidate alleles (50).
OptiType (51) is a HLA genotyping algorithm based on integer linear programming, capable of producing accurate 4-digit HLA genotyping predictions (for example, A01:01) from NGS data. To maximize the number of explained reads by simultaneously considering all major and minor HLA-I loci when predicting 4-digit HLA genotypes, this process involves aligning sequences from whole exome/genome/transcriptome sequencing data with a known MHC class I allele reference. Many tools for HLA typing are freely available for academic use, such as seq2HLA, ATHLATES, HLAminer, SOAP-HLA-2.2. A comprehensive list is provided in Table 2. Figure 3 depicts a generalised workflow for NGS-based HLA genotyping.
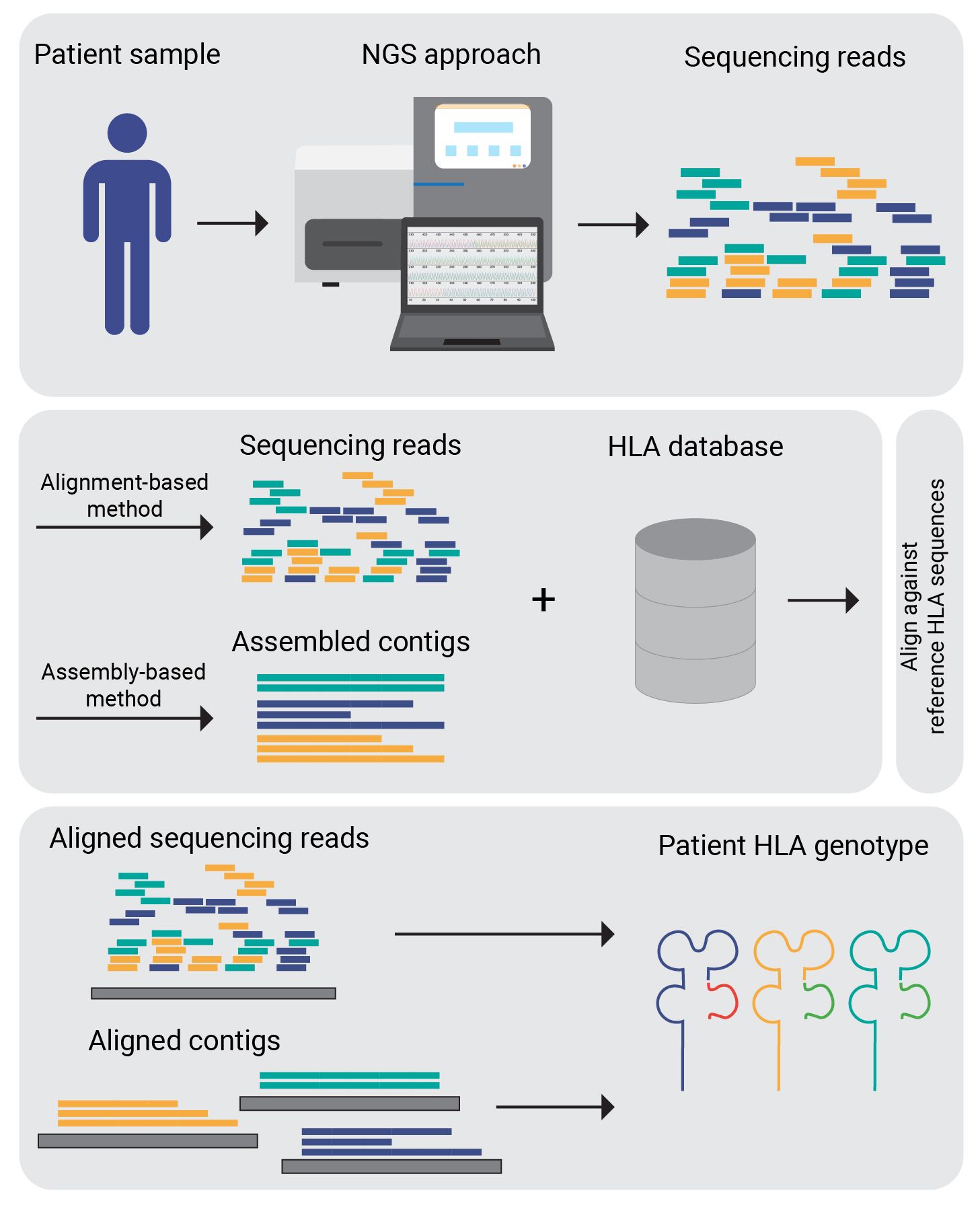
Figure 3 NGS-based HLA genotyping. Sequence data generated by sequencing technologies is mapped against the reference allele repository (IPD-IMGT). Corresponding to the HLA genotyping algorithm used either the raw reads or assembled contigs are aligned.
3.1 Benchmarking of HLA genotyping tools
There are multiple studies benchmarking HLA genotyping tools. Matey-Hernandez et al. (67) found that HLA typing tools based on WES and RNA-seq data exhibit prediction power almost equivalent to gold standards like PCR. Li X. et al. (45) focused on TCGA (68) cohorts, revealing superior performance of HLA class I over class II, with POLYSOLVER (60), OptiType (51) and xHLA (63) demonstrating high accuracy in HLA class I calling, and an ensemble HLA calling from the top-3 tools outperformed individual ones. Claeys et al.’s (69) study assessed 13 MHC class I and/or class II HLA callers, highlighting OptiType and arcasHLA (66) for MHC-I calling accuracy and HLA-HD (62) for MHC-II calling accuracy. The study concludes that the optimal HLA genotyping strategy from NGS data depends on factors like data type, dataset size, and computational resources, recommending OptiType and HLA-HD if resources permit (69).
4 Peptide-MHC binding prediction
T cells recognize peptides presented on MHC molecules of tumor cell. These molecules come in two main classes: peptide-MHC class I complexes, found on nucleated cells and recognized by CD8 + T cells, and peptide-MHC class II complexes, displayed on antigen-presenting cells like dendritic cells, activating CD4 + T cells. The diverse peptide repertoire is influenced by allele-specific amino acid preferences of MHC molecules. Due to individual variations in MHC alleles, the presented repertoire varies across people, with certain alleles being more common. The peptide-MHC interaction determines neoepitope presentation, impacting the level and type of T cell responses generated. While experimental MHC binding assays involve synthesizing and testing peptides, this is laborious and expensive on a large scale. Consequently, various computational algorithms and tools have been developed to predict peptide-MHC binding or assess binding affinity between mutated peptides and the patient’s MHC alleles (70).
It is important to note that other biologic processes can impact antigen presentation and immunogenicity of a particular neoantigen beyond MHC binding. Other factors, such as delivery of antigen to antigen presenting cells, antigen cleavage and processing by immunoproteasomes, peptide-MHC complex stability, are also important determinants of immunogenicity (7).
Early prediction tools relying on techniques as position-specific scoring matrices (PSSM) or sequence-scoring functions, such as SYFPEITHI (71), RANKPEP (72), PickPocket-1.1 (73), MixMHCpred (74), encountered difficulties in recognizing correlated effects. These effects manifest when an amino acid’s binding is influenced by the other amino acids in the peptide. The limitations of earlier tools in recognizing such correlated effects emphasize the suitability of neural networks as methods adept at considering these complex interactions (75).
Over the last decade, MS-based MHC peptidomics has become the dominant source of information about MHC binding specificities, with the ability to analyze ligands at greater depths than in vitro binding assays. Compilation of MHC ligandome data – the entirety of HLA presented peptides has been advanced by mass spectrometry (MS) based immunopeptidomics, in which the whole immunopeptidome of the cell is harvested and then eluted ligands (EL) are identified using MS. First application of direct neoepitope candidate identification using MS in native human tumors was presented in the paper of Bassani-Sternberg et al. (76). The authors assembled the ligandomes from human melanomas to a depth of 95,500 ligands. Eleven ligands were derived from candidate neoantigens, and four were proven to be immunogenic in T cell validation assays. MS profiling of HLA-associated peptidomes in mono-allelic cells enabled more accurate MHC-I epitope prediction in the study of Abelin et al. (77). MS immunopeptidomics is also able to identify protein hotspots, or regions within a protein prone to proteasomal cleavage and ligand production (78). Freudenmann et al. (79) constructed their own dataset and identified thousands of peptides bound to 16 different HLA class-I alleles to assess critical factors needed to epitope presentation.
However, in EL MS workflows typically pan- or locus-specific antibodies are used for immunoprecipitation (IP) during the purification of peptide–MHC complexes. This results in inherently poly-specific or Multi Allelic (MA) data, which comprises peptides that align with multiple cognate MHC binding motifs (80). For example, any of the six different MHC-I proteins present in a cell might be responsible for a peptide observation. These data need to be deconvoluted, i.e. transformed to Single Allelic (SA) or single peptide-MHC annotations, to be employed for the training of MHC-specific binding predictors. The method NNAlign_MA (81) resolved this limitation by incorporating into the prediction algorithm training procedure a strategy called pseudolabeling, which clustered EL sequences with ambiguous cognate MHCs into single MHC specificities.
Various AI-based tools have been developed to predict peptide-MHC binding using a range of neural network architectures and strategies in an attempt to improve predictive performance and generalizability of their models. They work on multiple data types including peptide sequences and mass spectrometry profiles.
One major issue impeding the generalizability of ML models is the lack of binding affinity data for rare MHC alleles. This can be addressed using various approaches such as using the sequence homology of rare MHC alleles with common MHC alleles to infer potential ligand preferences as NetMHCpan (82, 83) does. Also, NNAlign_MA was deployed in NetMHCpan to deconvolute ligandomes from MS datasets (80).
Another way is to use transfer learning by pre-training models on more common MHC classes and fine-tuning the models on the data for rare MHC classes. This approach is used by tools such as MHCnuggets (84), ImmunoBERT (85) and MHCRoBERTa (22). ImmunoBERT used transfer learning from the Tasks Assessing Protein Embeddings (TAPE) (86). The TAPE model was trained on a dataset of over 31 million protein sequences from the Pfam database. The authors of MHCRoBERTa used self-supervised training with label-agnostic protein sequences from UniProtKB (87) and Swiss-prot databases, and then fine-tuned the training with data from the Immune Epitope Database and Analysis Resource (IEDB) (88).
Many tools use approaches adopted from other domains. From the image processing domain comes the convolutional neural network which can learn multiple intrinsic features of the peptide sequence that can be used to predict binding affinity. Examples of these tools include ConvMHC (89), HLA-CNN (90) and DeepMHC (91). MHCSeqNet (92) uses techniques from the natural language processing domain by treating epitope peptide sequences as sentences composed from amino acids as individual words.
Some tools use ensemble learning, a technique that combines the output of several models using a weighted or uniform consensus. The concept behind the consensus methods is that prediction performance can be further improved by integrating the outputs from several individual tools using a weighted scheme. This includes tools such as MHCflurry (93) and NetMHCcons (94). MHCflurry is supporting only a fixed set of alleles (95).
Others tools provide or require additional data. Tools such as HABIT (96) provides an interpretation of the impact of amino acid variants alongside the binding affinity prediction. EDGE (97) and MARIA (98) require transcript abundances and flanking sequence in addition to the peptide sequence and MHC allele.
A class of tool use mass spectrometry and immunopeptidomics data as input data instead of peptide sequence data. This class of tool includes HLAthena (99) which shows 1.5-fold enhanced accuracy compared to sequence based tools and SHERPA (100).
An overview of tools used for MHC binding prediction is shown in Table 3.
Other tools focus on visualizing and comparing different MHC molecule binding specificities to aid the understanding of main binding properties An example of such as tool is MHC Motif Atlas (128, 129) which contains 1,013,733 ligands interacting with 135 MHC-I and 88 MHC-II molecules, including information about binding motifs, peptide length distributions, motifs of phosphorylated ligands, multiple specificities and enables users to download curated datasets of MHC ligands, MHC sequences and MHC X-ray crystallography structures.
4.1 Identification of MHC class II neoantigens is challenging
Predicting MHC class II binding poses an extra challenge compared to class I due to limited training data and the complex nature of HLA-II ligands. In humans, HLA class II is encoded by three different loci (HLA-DR, -DQ, and -DP) with numerous allelic variants and polymorphisms clustered around the peptide-binding groove, resulting in a wide range of distinct peptide binding specificities. This complexity of HLA-II ligands results in binders with longer and more heterogeneous peptide sequences and varying peptide length distributions, making their prediction more challenging (106, 130). A comprehensive trans-allelic model for prediction of peptide-MHC-II interactions for all three human MHC-II loci was proposed by Degoot et al. (131). The authors investigated contributions of certain binding pockets to the binding energy and found that binding pocket P5 of HLA-DP contributes strongly to the binding energy. Most HLA class II prediction algorithms have primarily targeted HLA-DR molecules, given the extensive data available for them (127). On the other hand, HLA-DQ molecules are more complex to study experimentally.
NetMHCIIpan-3.2 (132) and NetMHCIIpan-4.0 (80) predict antigen presentation for any HLA class II molecule. For HLA-DQ and DP heterodimers, both α- and β-chain sequences are needed. Nilsson et al. (127) used a DQ-specific antibody during purification to obtain immunopeptidome data for 14 different HLA-DQ molecules from 16 homozygous B Lymphoblastoid Cell Lines (BLCLs) using liquid chromatography coupled with mass spectrometry (LC-MS/MS) to train NetMHCIIpan-4.2. Benchmarked against MixMHC2pred-2.0 (122), on independent DQ data consisting of EL data from 15 donor samples enriched with random negative peptides, NetMHCIIpan-4.2 excelled in motif deconvolution and identifying DQ ligands. BERTMHC is an transformer-based peptide-MHC class II interaction prediction method (21). The pretrained BERT from TAPE repository was used to model the input amino acid sequences. Additionally, multiple instance learning was employed to account for the limitation that mass spectrometry data often cannot precisely identify the exact MHC molecule to which a peptide was bound.
Four methods (MHCnuggets (133), AI-MHC (102), PUFFIN (105), and USMPep (111)) can make predictions for both MHC classes. A majority of the responses to neoantigens in preclinical and clinical setting are MHC class II restricted (134). Therefore, improvement of algorithms on MHC class II binding interactions is crucial, since it will significantly enhance the selection of MHC-class II restricted neoantigens.
4.2 Challenges of mass spectrometry limiting MHC ligandome datasets
MS data has inherent biases such as overrepresentation of “flyable” peptides and neglect of cysteine-containing peptides, limiting the detectable set of ligands (80). Some MHC molecules, such as HLA-C and HLA-DQ, have limited ligand datasets (80). The performance of AI-based approaches used for predictions relies on quality and diversity of the training data. Therefore,high-quality data sets covering a broad range of HLA alleles, are crucial. Future work exploiting antibodies with improved specificities or using engineered cell lines with tagged HLA molecules might help to resolve this.
4.3 Benchmarking of peptide-MHC binding prediction tools
Benchmarking peptide-MHC binding predictors is not straightforward due to differences in the MHC alleles, peptide sizes, and non-standardized outputs of the methods. In 2014, the Immune Epitope Database automated benchmark was established to address the need for an unbiased evaluation of the MHC-I binding predictors (135). They assembled a blind test which ensures that the data will be new to all of the participating tools (135, 136). Based on the criteria established by the benchmark a peptide is deemed a binder if it was experimentally reported to qualitatively bind to an MHC, or its half-life (T1/2) bound to the MHC is reported to be longer than 120 min, or its IC50 is reported to be lower than 500 nM (135). Peptides that do not meet any of those criteria are considered non-binders (137).
Trevizani et al. (137) investigated predictor rankings using a benchmark. They found that due to the benchmark’s data update rate, a new method had to wait at least four years to be compared with existing ones. The top-performing tools consist of NetMHCcons-1.1, NetMHCpan-4.0, ANN 3.4 (138) (updated to ANN 4.0 (101) in 2016), NetMHCpan-3.0 (82) and NetMHCpan-2.8 (139), with statistically indistinguishable scores. The authors also determined that using percentile-ranked results from original metrics provided reliable rankings across different data sets.
Another comprehensive performance assessment of 15 in silico tools for MHC class I peptide binding prediction, including 6 scoring function-based, 7 ML-based and 2 consensus methods, was described in Mei et al. (140). Extensive benchmarking tests showed that MixMHCpred (141) performs best across most HLA-I allotypes, while NetMHCpan and NetMHCcons achieve the overall best performance among ML-based and consensus-based tools.
5 T cell receptor recognition
T cell receptors (TCRs) play a pivotal role in surveillance and response to disease by recognizing peptide-MHC (pMHC) complexes. However, not all neoantigen candidates elicit an immune response from T cells even though they are expressed and presented on the cell surface (11). Understanding the rules governing how T cells recognize cognate antigen-MHC complexes remains a challenge in systems immunology.
The TCR is a heterodimeric protein comprising an α- and β-chain. Peptide specificity is primarily defined by the complementarity-determining region 3 (CDR3) loops. The diversity of the CDR3s results from genomic recombination of the variable (V), diversity (D), and joining (J) genes (142). The majority of previous studies have focused on the β-chain alone due to its higher diversity, resulting from the V-, D-, J genes together (142). In contrast, the α-chain results from V- and J recombination which leads to lower diversity and less interest. However recent research has highlighted the importance of both α- and β-chain CDR3s in TCR specificity (143, 144).
T cell receptor sequencing (TCR-Seq) is an NGS approach allowing scientists to study clonal expansion by selectively amplifying and sequencing antigen-specific CDR3 regions of the T cell receptor. However, TCR-Seq data analytics is challenging as tumor-specific T cell responses constitute a small proportion of the overall pool of in vivo T cell responses with irrelevant specificities (145). New analytical tools have been developed to parse and draw meaningful sequence concepts or motifs from the TCR-Seq data (146). The TCRdb database contains more than 277 million TCR sequences from over 8265 TCR-Seq samples across hundreds of tissues, clinical conditions and cell types (147).
Assessing the interactions between neoepitopes and TCRs is essential for designing immunotherapies. For instance, identifying compatible TCRs in the patient’s circulation can help inform the selection of neoantigen vaccine candidates. Various experimental approaches, such as tetramer analysis (148), TetTCR-seq (149) and T-scan (150), have been developed to detect pairing of TCR–pMHC complexes. However, in vitro experiments associated with the testing of a large number of putative candidates demand experimental time and costs.
TCRdist (143) represents an unsupervised distance-based method exploiting the similarity between TCRs to produce clusters of TCR sequences that likely recognize the same antigen, and predicting binding for a given epitope sequence. The methods like TCRex (151) and DeepTCR (152) trained antigen-specific TCR models and would have problems to generalize to unseen peptides. In response, the scientific community has turned to ML and AI-based approaches to develop computational solutions for TCRs and peptide–MHC and TCR–peptide interaction prediction.
NetTCR (153) facilitates sequence-based prediction of TCR binding to pMHC complexes using CNNs. CNN is an appropriate model to handle unaligned peptide and TCR sequences differing in length. The model was trained on the IEDB data, containing TCR β-chain CDR3 sequences and corresponding peptide targets presented by most common MHC-I HLA-A*02:01 allele. Negative data examples were supplied for the learning by generating wrong combinations of TCRs and peptides, and additional negatives constructed from the TCRs of healthy donors. For NetTCR-2.0 (142) is a “shallow” CNN model, similar to NetTCR (153), it was exploited, but trained on paired TCR α and β chain sequence data. Nonbinding peptide-CDR3β pairs derived from 10X Genomics (154) Chromium Single Cell Immune Profiling of four donors were used as negative data set. The model has the potential to infer not only which TCRs are specific for a given peptide, but also which peptide is specific for a given TCR. This work also underlined the need for technologies for high-throughput paired sequencing of TCRs with known pMHC targets. The current optimal way to pair TCR α- and β- chain is through single-cell TCR sequencing (scTCR-Seq) (155). The authors of NetTCR-2.1 (156) provide lessons and guidance on how to develop models for TCR specificity predictions, how to best define negative data, and why it is recommended to apply similarity-based modeling, and include a performance evaluation as a function of “distance” to the training data when validating predictive power of ML-based approaches.
Most of the peptides in the published databases originate from viruses but not from tumor-associated antigens and there are only a few CDR3α sequences in databases available. Therefore, AI-driven approaches with improved generalization ability are needed, which do not show significant performance drop when evaluated on peptide sequences not used during model training. This challenge can be addressed by approaches based on transfer learning and NLP, capable to benefit from unsupervised pre-training.
As an example for the application of a newly emerging DL approach, Lu et al. (157) used transfer learning to develop pMTnet, a model predicting the TCR binding specificity of class I pMHCs. Utilizing the “Atchley factor” (158) they encoded TCR CDR3β sequences with five numeric values per amino acid, providing comprehensive biochemical characterization. These “Atchley matrices” were input into a stacked auto-encoder, an effective unsupervised learning algorithm. During training, the auto-encoder reconstructed input data, generating a 30-neuron numeric vector that encapsulates the inherent structure of the original CDR3s. The embedding of pMHCs closely followed the NetMHCpan algorithm. Fixed numeric encodings of TCRs and pMHCs were integrated into a DL network with a single neuron as the final layer for pairing prediction. To train this model, Lu et al. (157) employed a differential learning schema, using known interactions as positive data and introducing true and mismatched pairs for negative data, resulting in ten times more negative data by randomly mismatching TCRs and pMHCs. This approach allowed them to capitalize on a substantial volume of related TCR and pMHC data without explicit pairing information, showcasing the effectiveness of transfer learning.
For their NLP-based approach BERTrand (159) the authors constructed a hypothetical human TCR-peptide repertoire pre-training set comprising peptides from MHC-I MS peptide presentation experiments and TCRs from healthy donors, and this hypothetical TCR-peptide repertoire was used to perform masked language modeling (MLM), pre-training of the BERT model. Then the pre-trained BERT model was fine-tuned to predict TCR-peptide binding using the dataset of known TCR binders with their cognate epitopes and negative decoy examples generated by random pairing of reference TCRs with peptides. ERGO (pEptide tcR matchinG predictiOn) (160) and ERGO-II (161) utilize unsupervised TCR pre-training and use a pre-trained LSTM neural network architecture.
Further published tools for TCR-pMHC binding prediction are shown in our Table 4.
5.1 Limitations of current data sets for TCR–peptide binding prediction
Current datasets for TCR-peptide binding prediction present challenges for the development of accurate and generalizable models. As discussed in the perspective article of Hudson et al. (171), the current data sets cover only a limited fraction of the universe of possible TCR–antigen binding pairs. These datasets also inadequately represent the universe of self and pathogenic epitopes and of the varied MHC contexts in which they may be presented. Furthermore, a significant proportion of known antigens reported as binding a TCR are of viral origin, limiting their relevance to human health.
Current sources of publicly available data for AI-based methods to predict the interaction between TCR and pMHC complexes include manually curated catalogs of pathology-associated TCR sequences such as McPAS-TCR (172), Immune Epitope Database IEDB (88), VDJdb (173), and TBAdb (174) databases. Additionally, positive data samples generated by Klinger et al. (175), known as the MIRA set, are publicly available in the NetTCR-2.0 repository (176). For successful training and development, balanced training data is required. However, the publicly available datasets of TCR-pMHC sequences almost exclusively contain examples of positive binding pairs. Only the published 10X Genomics dataset contains both positive and negative data points. The choice of negative data is a critical factor when developing a binary classification model. NetTCR and pMTnet chose 10X Genomics Immune Profiling data, which contains validated non-binding complexes. Swapped negatives are randomly generated negative data, generated by other prediction tools (TCRGP (164), ERGO-I, ERGO-II, TITAN (166)), by mispairing positive validated TCR–peptide pairs. However, this approach risks to introduce false non-bindings into the ground truth.
In the future, as high-throughput technologies such as T-scan and 10X Immune Profiling are becoming more prevalent, it is expected that more training data for TCR-pMHC pairing will be available, providing a more accurate representation of the entire space of potential epitopes for training. Frank et al. (177) provide an overview of TCR sequencing platforms and the T cell repertoire analysis methods.
5.2 TCR binding predictors fail to generalize to unseen peptides
While many TCR-pMHC binding prediction methods perform well with test sets containing peptides from the training set, the ability to generalize to unseen peptides is crucial for neoantigen-based cancer vaccine development. Grazioli et al. (178) investigated the impact of various training/test splitting techniques on models’ test performance. They introduced Tchard, a sample collection with positive samples from the databases IEDB, VDJdb, McPAS-TCR, and the MIRA, along with negative samples from randomization and 10X Genomics assays. After ensuring that testing samples were not present in the training dataset, they found that modern DL methods may struggle with generalization to unseen peptides. Deng et al. (179) addressed this by comparing the performance of different TCR-pMHC prediction tools on various datasets. Regardless of model complexity, all tools, including TITAN, NetTCR-2.0, ERGO, DLpTCR and ImRex, faced challenges predicting unseen peptide examples. These challenges emphasize the necessity for ongoing research to enhance the generalization of TCR-pMHC binding predictors across a wider range of peptides.
6 Criteria for epitope selection
Only a small fraction of predicted neoepitopes can be experimentally validated in vitro as true neoepitopes (180). Several general criteria are currently employed in the field to narrow down and prioritize the candidate epitopes. These criteria guide the selection of epitopes to induce specific “on target” immunogenic response while overcoming self-tolerance.
6.1 MHC binding affinity
Mutant peptides must be presented by MHC-I or MHC-II in order to be recognized by T cells. Most neoantigen prioritization pipelines typically use the output values of the MHC-I or MHC-II binding prediction methods as the primary ranking parameter. The generally used MHC binding affinity threshold type is IC50 (half maximum inhibition concentration) measured in nM. The lower value shows stronger binding affinity. Usual thresholds are IC50 ≤ 50nM (strong) and IC50 ≤ 500nM (low). Another threshold type is percentile rank (%-rank) which allows to better compare scores between MHC molecules. Usually %-rank ≤ 0.5 is strong affinity and %-rank ≤ 2 shows lower affinity. NetMHCpan-4.1 differentiates %-rank prediction based on either LC-MS eluted ligands (EL) or binding affinity (BA). The third type is Score, as in SYFPEITHI (71). They typically do not recommend any threshold. Here, the higher binding score shows increased chances of binding.
It is important to note that these commonly used threshold values for identifying potential binders can be excessively strict in many cases (76) that can result in missing potential binders. To improve the sensitivity and accuracy of 13 already existing prediction tools Bonsack et al. (181) calculated new thresholds, recommended for each of them. They also developed MHCcombine (182) to facilitate the application of their prediction-improving recommendations and also to simultaneously compare the outputs of the selected predictors.
6.2 TCR binding affinity
As mentioned before, the T cell recognition and activation is a vital part of the immune response. In order to trigger immune response T cells need to recognize the peptides presented by the MHC molecules. Addressing the T cell activation outcome still remains challenging however generally can be determined based on the biochemical parameters of the pMHC-TCR interaction (11). The mostly used parameter is TCR-pMHC binding affinity. Gálvez et al. (183) aimed to uncover the shaping forces behind the TCR binding affinity with 12 phenotypic models and as a result they provide valuable insight and observations in the field of TCR binding affinity. As described in the review by Schaap-Johansen et al. (11) a number of structure-based methods have been developed lately which can greatly improve the overall TCR binding predictions by reducing the false positive predictions.
6.3 Agretopicity
The differential agretopicity index (DAI) has been proposed as a neoantigen quality metric (184). DAI is a property of the epitope and defined as the numerical difference between the NetMHC (138) scores of the WT peptides and their mutated counterparts (184). In an study of 6,324 patients across 27 cancer types, Rech et al. (185) found that high DAI neoantigens correlated with patient survival. The work of Ghorani et al. (186) also supported the hypothesis that DAI is a determinant of cancer peptide immunogenicity, by investigating the association between mean DAI, survival, and measures of immune activity.
6.4 Binding stability
Assuming that a more stable epitope presentation on the MHC increases the likelihood of T cell recognition, peptide stability, measured as the half-life of the binding interaction in units of hours, has been postulated to correlate with immunogenicity. Tools such as NetMHCstabpan (187) are often used in epitope selection pipelines to assess binding stability. Borden et al. (188) used a model-based approach to find the neoantigen properties that have predictive value of immunogenicity. The binding stability of the pMHC class I complex, along with the dissociation constant and the expression (mRNA and variant allele frequency) were the characteristics that were of predictive value. These findings were in consistence with previous studies (189). The authors integrated binding stability together with other factors such as neoantigen expression level and dissociation constant into an immunogenicity score called NeoScore (188).
6.5 Differential expression between tumor and healthy tissue
In contrast to pathogens seen as foreign invaders, most epitopes presented on the cancer cell surface are self-peptides unrecognized by tumor immunosurveillance. Neoepitopes, typically absent in benign tissues, may escape tolerance and become immunogenic. Databases such as TissGDB (190), GTEx (191), TCGA (68), THPA (192, 193) can be consulted to compare gene expression between healthy and tumor tissues, identifying cancer-specific signatures (194).
6.6 Dissimilarity to the self-proteome
As observed in the literature, sequence dissimilarity to non-mutated proteome was predictive of peptide immunogenicity (195, 196). Devlin et al. (197) demonstrated that structural dissimilarity between the wildtype and mutated peptide in non-anchor positions can influence T cell recognition and immunogenicity.
6.7 Expression of a peptide source gene in thymocytes
Medullary thymic epithelial cells (mTEC) contribute to the development of T cell tolerance by facilitating the recognition of “self” and expressing tissue-restricted antigens (TRA) (198). This allows developing T cells to assess the self-reactivity of their antigen receptors before leaving the thymus (198). The expression of a peptide source gene in mTEC is considered as a negative characteristic for epitope selection, as it may decrease the chances of immunogenicity due to the central tolerance.
6.8 Hydrophobicity
As described in the methods of TESLA consortium, the number of hydrophobic residues in the neoantigen can be divided by the total number of residues to create a “hydrophobicity fraction” (189). Additionally, the grand average of hydropathicity index (GRAVY) is used to estimate the hydrophobicity of a given amino acid string and is calculated as the average of the hydrophobicity of the individual residues forming the peptide (199). Immunogenic pMHC were significantly less hydrophobic than non-immunogenic pMHC (199).
6.9 Clonality
Clonality refers to the fraction of the tumor containing the neoantigen of interest and of particular importance for prioritization. The presence of a variant expressed by a small, sub-clonal population of the tumor makes it less attractive candidate for tumor therapy (200). In the review of Lang et al. (201) the impact of clonality on neoantigen recognition is discussed. Depending on whether the neoantigen is truncal clonal, truncal clonal but lost in a metastasis (by deletion or gene silencing), clonal in a certain metastasis (or specific for a certain subclone within a single metastasis), neoepitope-specific T cells would target either all tumor cells, all tumor cells of selected lesions, or merely a single tumor subclone (201). The tools PyClone (202) and its improved version PyClone-VI (203) provide a numerical estimation of cancer cell fraction using observed alternate allele frequencies, copy number, and loss of heterozygosity (LOH) information.
Other characteristics associated with immune response, such as the variant allele frequency of mutations, the number of predicted neoepitopes per mutation, peptide proteasomal cleavage probability, potential for TAP transport in the endoplasmic reticulum, tumor heterogeneity and HLA loss of heterozygosity (LOH), are used to further rank candidate neoantigens (200).
7 Integrated software for neoantigen detection and prioritization
Several integrated software and comprehensive pipelines have been developed for tumor-specific neoantigen detection. The purpose of these tools is to make the prediction and prioritization of neoantigen candidates accessible. Here, we describe some of the notable tools and frameworks and their approaches.
For seamless vaccine design there have been several end-to-end pipelines developed. One of the frequently used end-to-end pipelines is FRED2 (FRamework for Epitope Detection), a Python-based immunoinformatic framework (204). Among the included tools there are several HLA genotyping tools (e.g.: OptiType), as well as peptide-MHC binding predictors (e.g.: NetMHCpan, NetMHCIIpan), and also the proteasomal cleavage predictor NetChop (205) is integrated. FRED2 ensures straightforward workflow and provides analysis tools to epitope detection and vaccine design (204). Another end-to-end pipeline is pVACtools, which produces an end-to-end solution for neoantigen characterization (206). To aid the vaccine design, pVACtools supports the identification of altered peptides and prioritizes them by incorporating various data sources, such as clonality of the mutation, mutant allele expression and peptide binding affinities. Among the tools integrated inside pVACtools there are binding predictors (e.g.: MHCflurry), databases (e.g.: IEDB), and a proteasomal cleavage predictor (NetChop). To extract neoepitopes from tumor sequencing data such as VCF files and expression files generated from RNA-seq, MuPeXI (Mutant peptide extractor and informer) provides a prioritization suggestion based on a combined score named priority score (207). It generates an output file with the list of mutated peptides and all the information needed (expression level, similarities to self-peptides, mutant allele frequency) to select the peptides for vaccine design (207). For HLA binding prediction NetMHCpan is integrated. It is a web-based tool, and also available as a command-line tool. TIminer is also a computational framework that provides complex immunogenomic analysis including HLA typing (Optitype), neoantigen prediction (NetMHCpan), characterization of immune infiltrates and quantification of tumor immunogenicity (208).
Another solution for peptide design includes prioritization algorithms. One such predictor is PRIME (predictor of immunogenic epitopes) (209). It captures molecular properties of both antigen presentation and TCR recognition. PRIME reveals experimentally validated biophysical determinants of TCR recognition and also establishes correlations with T cell potency. MixMHCpred is integrated for predictions of antigen presentation and TCR recognition. Beside the above-mentioned features, it improves the overall prioritization of neoepitopes. Another notable prioritization algorithm is DeepImmuno (210), a CNN based tool that predicts the epitope immunogenicity for CD8+ cells of 9-10-mer peptides. The prediction can run from the command line or from their web interface. The easy-to-use web interface has MHCflurry integrated to not only predict the immunogenicity of the specific HLA-peptide pairs, but the binding affinity score as well. DeepImmuno includes an independent generative adversarial network model, which can generate immunogenic peptide with the possibility of training your own model.
Most of the tools can predict neoepitopes from SNVs, some also incorporate INDELs (pVACseq (211), MuPeXI (207), TSNAD (212), CloudNeo (213), Epidisco (214), pTuneos (215), antigen.garnish (195), NeoPredPipe (216), NeoEpiScope (217), OpenVax (218)). A few focus solely on INDELs (ScanNeo (219)) or gene fusions (NeoFuse (220), INTEGRATE-neo (221)), while others allow users to input the variants as peptides (EDGE (97), DeepHLApan (103)).
A summary of various integrated pipelines and software tools for neoantigen discovery is provided in Table 5.
8 Tumor neoantigen data collection
The training of novel and improved algorithms requires continuous accumulation of verified tumor neoantigen data. Several studies have curated cancer antigen data, and constructed publicly available cancer antigen resources. These databases support the community in understanding the landscape of antigen presentation and provide necessary information for the development of neoantigen prediction tools. In addition to the well-curated data sets, several so-called in silico neoantigen databases that omit the experimental validation step have been built by taking advantage of existing neoantigen prediction software.
There are several well-curated datasets. One of the widely used, well-known resource is the Immune Epitope Database and Analysis Resource (IEDB) (88). It is a freely available comprehensive repository for diverse immunological data. This database contains experimental data from various host organisms about peptidic and non-peptidic epitopes, MHC ligand (Class I and II), T cell and B cell assays with a chance to gain insight into the possible disease context such as allergy, autoimmune or infectious diseases (234, 235). The database exists since 2003 and due to its enormous data content with over 1,600,000 epitopes and availability, this database is integrated in many other databases we have mentioned. However, IEDB’s data sets of verified T cell epitopes primarily consists of epitopes from bacteria or viruses and were not obtained by standardized experimental methodologies in the context of cancer. Furthermore, CEDAR (236) is the cancer epitope focused companion site of IEDB. This freely available database is similarily built to its companion and houses over 1,290,000 epitopes. Here, B cell, T cell and MHC ligand assay results are available in various hosts focusing on cancer types and stages.
Further curated databases include NeoPeptide (237), dbPepNeo (238), dbPepNeo 2.0 (239), TANTIGEN (240) and NEPdb (241). NeoPeptide focuses on cataloguing neoantigens from somatic mutations across different cancer types from clinical trials and in vitro experiments. At the time of its creation in 2019 it already contained 36,000 antigens and over 180,000 epitopes which has been expanded since (10). It provides details on various neoantigen characteristic such as mutation site, sequence and MHC restriction. The dbPepNeo databases include curated information about neoantigen data validated by mass spectrometry or immunoassays in human tumors. While version 1 focuses on validated MHC-I antigens in various tumor types, in version 2 the included neoepitope candidates increased to over 840,000 while also adding MHC-II data. Both versions help the user by categorizing all neoantigen’s confidence based on the strength of the experimental validation. TANTIGEN focuses on cancer antigens whose HLA binding is experimentally validated from tumor tissues. Over 1,000 tumor peptides from close to 300 proteins are catalogued based on which the T cell epitopes and HLA ligands are easy-to-list. However, it does not include peptides shown to be ineffective and lacks any association with clinical data. NEPdb was constructed via curating published literature with a semi-automatic pipeline by parsing and filtering abstracts with NLP toolkit. It includes curated data of 173 MHC-I and MHC-II neoepitopes and over 17,000 non-immunogenic peptides from 23 tumor types. The validation focuses both on in vitro and in vivo T cell assays.
Also, there are databases on verified binding and presentation. This category includes caAtlas (242), SPENCER (243), IEAtlas (244), HLA Ligand Atlas (245) and CARMEN (246). caAtlas is a database that contains information about mass spectrometry results of 9 cancer types and non-tumor samples. The data focuses both on MHC-I and MHC-II molecules and comprises around 140,000 modified peptides. SPENCER focuses on small peptides in cancer patients that are encoded by non-coding RNAs. The database contains mass spectrometry data of 15 cancer types from over 1,700 patients resulting in the identification of near 30,000 small peptides encoded by non-coding RNA in tumors. IEAtlas collects the immunopeptidome data of mass spectrometry datasets to find epitopes that bind MHC-I/II from non-coding regions. Currently over 245,000 such epitopes are identified from 15 tumor types and 30 non-tumor tissues. the database HLA Ligand Atlas provides a collection of natural HLA ligands presented on benign tissues. Natural HLA ligand information could be important for further tool development.
Besides the experimentally verified databases there are also a number of in silico predicted neoantigen databases with an enormous variety of potential neoantigens. TSNAdb v1 (247) collected information about millions of potential neoantigens from somatic mutation data. The predictions of version 1.0 are based on the HLA data of 16 tumor types collected from TCGA (68) and TCIA (248) and are generated by NetMHCpan. TSNAdb v2.0 (249) upgrades its toolkit to use DeepHLApan, MHCflurry and NetMHCpan and predicted neoantigens not only from SNVs but from INDELs and fusions. The altered criteria in v2.0 decreased the false-positive predictions resulting in almost 400,000 SNV-derived, around 140,000 INDEL derived and over 11,000 fusion-derived predicted neoantigens. TSNAdb includes HLA binding info for both mutant and wild-type peptides thus, facilitating the assessment of the DAI (247). TRON Cell Line Portal (TCLP) (250) catalogues MHC types and predicted neoepitopes amongst other publicly available data of 1,082 cancer cell lines. The data focuses on both MHC-I/II neoantigens in a cell-line-specific manner.
The set of verified neo-epitopes is still limited, and we envisage that larger neo-epitope datasets will lead to additional refinements in immunogenicity predictions. For a summarized overview of the above-mentioned neoantigen databases, see Table 6, for a summary on immunology related databases and datasets see, Table 7.
9 Benchmark for neoantigen prediction
In 2016, the Tumor Neoantigen Selection Alliance (TESLA) was established as a collaborative effort to identify the most effective predictive algorithms for targeting neoantigens through large scale validation. Supported by the Parker Institute for Cancer Immunotherapy and the Cancer Research Institute (CRI) (189, 258), TESLA involved 35 public and private research teams worldwide. Each team employed its own unique neoantigen prediction algorithms to identify and prioritize neoantigens. The initial focus was on advanced melanoma, colorectal cancer and non-small cell lung cancer (NSCLC). Genomic data from the same six patient samples (3 melanoma, 3 NSCLC) was provided by the Alliance. The immunogenicity of candidate neoantigens was validated through MHC-restricted T cells in subject-matched peripheral blood mononuclear cells (PBMC). This study highlighted the significant differences in the prediction methodologies among the groups. No single methodology identified every neoantigen, nor a large majority of neoantigens, indicating the need for a standardized approach.
Besides testing the already existing predicting algorithms, the other goal of the TESLA was to identify key parameters shaping tumor epitope immunogenicity. The Alliance determined that approximately 50% of immunogenic epitopes are characterized by strong MHC binding affinity, prolonged half-life, high expression, and either low agretopicity or high foreignness. A model based on these five peptide features associated with presentation and recognition was developed and tested against independent cohorts of cancer samples. TESLA data is available (259) to qualified investigators and provides opportunities to benchmark the performance of neoantigen workflows.
Using the TESLA dataset, Buckley et al. (260) evaluated performance of seven publicly available methods - IEDB model (261), NetTepi (262), iPred (263), Repitope (264), PRIME (209), DeepImmuno (210) and Gao (265) - predicting whether an MHC-presented peptide might invoke a T cell response (i.e. whether a peptide is immunogenic). Filtering the TESLA dataset, originally comprising cancer peptides from 13 class I alleles, to retain alleles for which all models are applicable, and excluding peptides observed in any model’s training data, resulted in 27 immunogenic and 372 non-immunogenic peptides (lengths 9 or 10 aminoacids) that were experimentally tested against seven HLAs. They observed high numbers of false positives for all model. In this benchmark, PRIME identified 26 neoantigen from the total 27, successfully reaching the highest number of identified TESLA neoantigens.
10 Challenges and potential solutions to gain widespread adoption of AI applications for neoantigens discovery
Learning from a large set of data and identifying patterns of interest is the greatest strength of AI. The integration of AI applications in cancer immunotherapy and personalized medicine holds great promise, however, also comes with various technical and implementation challenges. Figure 4 summarizes the introduced bottlenecks of AI-based neoantigens discovery along with their potential solutions.
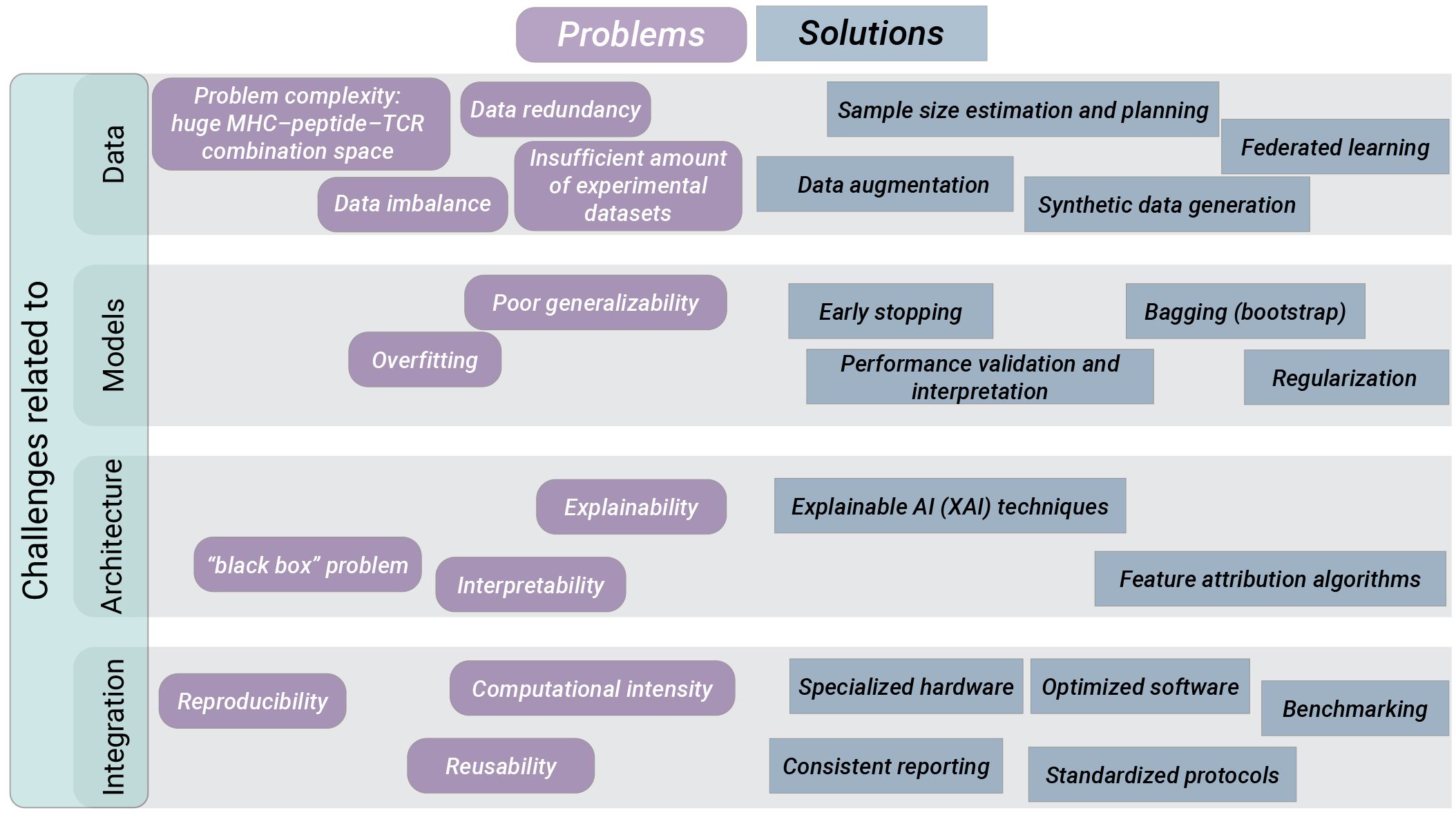
Figure 4 Challenges and potential solutions to promote widespread clinical use of AI applications for neoantigens discovery. We distinguish challenges that must be addressed for successful AI integration into clinical praxis as related to data, models, AI architecture and technical integration. For each group of challenges we list various algorithmic, experimental and organizational approaches carrying the potential to overcome the respective challenges.
10.1 Challenges related to data
10.1.1 Insufficient amount of available well-curated data
Data scarceness, data accuracy, and problem complexity contribute to challenges with models training. Available experimental datasets are limited in volume, diversity and standardization. Additionally, there is a lack of experimental data of binding affinity and antigen presentation for many HLA alleles. Furthermore, for many datasets consistent biological definitions are not considered or differ between studies, e.g. distinguishing between pre-existing and de novo T cell responses upon neoantigen vaccination.
Problem complexity is imposed by the huge MHC–peptide–TCR combination space, the length variations of TCRs, and inter- and intra-patient variability of TCRs or MHCs. Running AI training procedures on a limited or disparate data may result in overfitting and biased outcomes, compromising the reliability of future predictions.
10.1.2 The lack of experimentally verified negative data and the issue of data imbalance
EL/MS experimental approach reports only the presence of a peptide at the cell’s surface, but cannot identify the absence of a peptide from the individuals’ immunopeptidome. The prediction of peptide-MHC binding is a quintessential classification problem. For binary classification, there should be a sufficient number of observations in both positive and negative classes. Otherwise, the imbalance will lead to a bias of the classifier trained on these data and therefore, the creation of artificial negative examples (decoys) is required. However, insufficient consideration of the source of the negative examples can lead to further biases (266). Recently a homology-based method Neglog was proposed (267) to infer more negative data from very limited experimentally verified Negatome (i.e., pairs of proteins that do not interact). Neglog outperformed pure random sampling, and independent test on negative data is indispensable for bias control, which is usually neglected by existing studies (267). Negative data sampling also needs to be properly addressed for computational prediction of peptide-MHC and TCR–peptide binding.
10.1.3 The influence of dataset homology
Another problem is data similarity. Datasets contain many epitopes that are either identical or very similar to each other, which results in data redundancy. If not properly managed, redundancy can lead to overfitting. By performing homology reduction procedures, some of the tools take redundancy into account. The influence of dataset homology on protein secondary structure prediction was investigated by Chen et al. (268), and a rigorous evaluation strategy was proposed.
10.1.4 The lack of sample size determination
How much training data is required for AI application? The minimum dataset size required for effective training of AI models remains unclear in the biomedical sector. The rule “the more data, the better” is not realistic in the biomedical sector which faces technological limitations in acquiring data. Theoretical investigations concerning sample size planning for classification models (269) and sample size estimation for effective modelling of classification problems (270) are available and should be contemplated.
10.1.5 Algorithmic and model-driven solutions to data challenges
There are approaches in the biomedical and general domain aiming to balance the dataset used for AI training. Data reweighting helps to compensate under-represented subgroups by duplicating the minority class data. Data perturbation increases the diversity of the dataset by adding “noise” to existing samples. Data augmentation is a process of generating synthetic data exploiting algorithms such as generative adversarial networks (GANs). GANs consist of two main components trained simultaneously using adversarial training: a generator model generating samples similar to real data, and the discriminator model attempting to distinguish between real and generated samples. We already mentioned DeepImmuno (210) using GANs to generate immunogenic peptides. Federated learning is another approach to work with limited data sources or skewed distribution in the dataset. In federated learning, a central machine aggregates learning from other devices referred to as clients, collaboratively training a model while ensuring that their data remains decentralized. The idea to generate a global model via exchanging parameters (e.g. the weights and biases of a deep neural network) between the local nodes without explicitly exchanging data samples was motivated by the issues such as data privacy and data access rights.
10.2 Challenges related to models
10.2.1 The problem of overfitting and lack of generalizability
Memorizing the training examples without learning any generalizable patterns by the model is a problem called overfitting. If a predictor overfits to the training data, its actual prediction accuracy on a new data will be worse than the one reported (271). Increasing the complexity of AI model (e.g. increasing the number of layers of ANN and thus the number of parameters) can result in overfitting and consequently in poor generalizability of the model. To address this issue, various methods can be employed. Early stopping technique prevents overfitting by stopping the training process at the moment the test error starts to increase. Resampling methods such as Bagging or Bootstrap, in particular the optimism-adjusted bootstrap (OAD) (272), aim to increase the generalization capability of the model by training multiple base learners on randomly sampled portions of data and then aggregating the learners. Regularization improves the model’s generalization capability by setting the weights of features in the model closer to zero, reducing the influence of insignificant features. Dropout is a kind of regularization technique employed in deep learning, working by randomly dropping neurons out of the network during the training with the aim to prevent any neuron from becoming too influential. Cross-validation divides the dataset into multiple equal parts and evaluates the model’s performance by using each segment as a test set in turn. Performance validation and interpretation, identification and correction of biases, are essential for more reliable, accurate, and generalizable AI models.
10.2.2 Performance metrics demonstrating the quality of a model are not standardized
To assess the prediction performance of AI algorithms, numerous performance metrices are alternatively used. These include accuracy (Acc), sensitivity (Sn), specificity (Sp), F1 score, the Matthews Correlation Coefficient (MCC), the area under the receiver operating characteristic (ROC) curve (AUC), and Positive Predictive Value (PPV). The findings of in silico studies are presented in a heterogeneous manner and are difficult to compare. The suitability of performance metrics may also depend on the data situation at hand. For example, when diagnosing classification model performance on highly imbalanced datasets, ROC-AUC can underrepresent the minority class and be therefore misleading, while precision–recall area under the curve (PR-AUC), which summarizes model precision and recall, represents the balance of classes within the testing dataset more accurately (273).
10.3 The challenge of interpretability: AI models operate as a “black box”
“Has artificial intelligence become alchemy?” (274) Another important obstacle experienced by AI applications is the lack of understanding the methodology and the human inability in explaining the precise steps leading to predictions. How the models make the predictions and what the models learn from the input data remains largely unknown. The AI is in its golden era and the advances and possibilities are almost endless. However, to trust model predictions completely, it is vital to understand the processes that transforms inputs into outputs. There have been several attempts to improve the interpretability of ML models. Vig et al. (275) used the transformers attention mechanism to show that some of the transformer’s nodes were able to learn biological properties of proteins (e.g. secondary structure, binding sites etc.).
In the context of peptide presentation by MHC class I proteins it will be important to identify the most influential parts of the input amino acid sequences contributing to the output. To tackle this challenge, the authors of ImmunoBERT (85) presented application of two interpretability techniques developed in the field of computer vision, SHapley Additive exPlanations (SHAP) (276) and Local Interpretable Model-agnostic Explanations (LIME) (277), for interpreting BERT architecture predictions. Using the tool Captum (278), one can apply a wide range of feature attribution algorithms to attribute the predictions of a DL-based image classifier to their corresponding image features. Adoption of such algorithms to the analysis of sequence information would provide new insights in the field.
10.4 Difficulty in integration of AI applications
10.4.1 Benchmarking the different AI or ML tools
AI or ML tools are excessively difficult to benchmark in the clinical setting despite the fact that they can be trained with existing databases on patient data. One clinical study with a prediction tool cannot be directly compared to another clinical study that uses another tool, since the patients and the neoantigens are different.
10.4.2 Reproducibility and reusability of AI models
To improve transparency and reproducibility, guidelines have been established for developing and reporting ML predictive models in biomedical research (279). These guidelines promote consistent reporting of model specifications, including potential limitations of the model such as assumed input and output data format, pitfalls in interpreting the model, potential bias of the data used in modeling, generalizability of the data. In addition, sharing of well documented code for the model together with transparent descriptions of the optimized hyperparameters and hardware specifications is another aspect that would ensure that AI algorithms are transparent and reproducible. Collaborative initiatives for generation of joint guidelines and consensus recommendations, as well as translation them into standardized protocols will play a crucial role in driving the widespread adoption of AI-based solutions.
10.4.3 AI is computationally intensive
Successful application of AI requires proper computational infrastructure, including specialized hardware such as graphics processing units (GPUs), as well as optimized software for reduced computational needs (e.g. Q SLAM Technology), and solutions for integrated management of data and resources.
10.5 The ethical and legal implications of using AI
Algorithms do not accept responsibility or legal liability for their decisions and errors. Careful development, testing, and evaluation is required before integrating AI systems for patient care (280, 281). These challenges must be addressed to fully harness the potential of AI in cancer immunotherapy and personalized medicine.
11 Discussion
AI has already proven to be useful in everyday life from refining the text of manuscripts to troubleshooting codes (282). However, the risks are higher when applying AI to human health. The implementation of AI in general clinical practice can be a sensitive topic. Medical professionals spend decades learning, practicing, improving and the gained experience along the way is extremely valuable. Comparing AI that has unknown or unexplainable processes to the medical professional when it comes to diagnosis and decision making related to possible therapy or necessary surgery, is a rather delicate topic for discussion (283).
Nonetheless, it is undeniable that AI technology is currently needed in the medical field. One such field where AI´s involvement is certainly required is cancer immunotherapies. In the past decades, immunotherapy has become increasingly important as a new form of cancer therapy. For the development of cancer vaccines, quick and efficient processing of large data is required. One challenge is to identify tumor-specific antigens, the majority of which are unique for individual patients. Combining tumor sequencing data with the use of predictive algorithms based on machine learning and artificial intelligence allows clinical investigators to accelerate identification of therapeutically relevant neoantigens.
We reviewed multiple tools and a broad selection of prediction servers for neoantigen detection based on advanced AI methodologies. These tools are still far from widespread use in clinical practice as it can be difficult for users to choose the best server. There is a lack of reference data that should serve as an open benchmark to compare the approaches and validate the concordance of predictions among different tools. We encourage the standardization of techniques and harmonized protocols for sequencing, mutation detection, immunogenicity testing, and neoantigen candidate prioritization.
Our work highlights the barriers of applicability and clinical adoption of AI approaches. The insufficiency of experimental data for training and associated with it the lack of generalizability of AI-based models represents the major challenge. Novel approaches capable to overcome the critical role of data limitations are required for further development of in silico methods. Transfer learning has become increasingly relevant in this regard. AI models that can efficiently use all of the limited available data and transfer knowledge from other sources are extremely valuable.
Carefulness must be applied to the issue of performance guarantees both for training the model and for assessing how it will perform when deployed. Standard statistical and ML methods should be employed, such as bootstrap or a Bayesian method to assess prediction confidence intervals, to quantify the uncertainty of AI model in the output, and analyzing the sensitivity of the model’s output to certain parameters. Often the target and loss function used for training may not match the target and loss function important for the users. Bridging this training-application gap can be addressed by grounding methods, i.e. supplementing the model’s training with context-specific information, improving its ability to function effectively in disparate real-life situations.
A mechanistic explanation of the relationship between the peptide sequence, HLA allele and binding affinity remains an open topic of investigation. AI-based tools provide a potential solution in two ways: 1) Deep learning approaches can learn features automatically from unstructured data, bypassing the need to discover a mechanistic explanation. 2) Explainable AI techniques, such as attention mechanism, may be able to provide clues about aspects of the relationship that require further investigation. The two possibilities are not mutually exclusive and if early efforts focus on producing accurate and generalizable black-box models, then later efforts should attempt to use explainable AI techniques to understand the reasoning the model uses to make its predictions. As we navigate the path forward in personalized cancer immunotherapy, several questions remain. How can we expand the collection of well-curated neoantigen data, particularly for rare cancer types? What additional factors beyond peptide properties, such as protein structure and post-translational modifications, should be considered for neoantigen prediction? How can we enhance the interpretability of AI models, making them more transparent and accountable? These questions, among others, represent exciting avenues for future research and innovation.
By depositing the results of experiments and clinical trials in public databases, investigators will assist in making neoantigen prediction models more generalizable. Companies should agree to mutually exchange information beneficial to all parties in a benchmarking group and share the results within the group. As clinical studies will continually evolve to become more inclusive, harmonized and easily accessible, the aforementioned challenges of clinical integration of AI will also be bridged.
This review focuses specifically on AI and neoantigens, however, the use of AI approaches to predict cancer immunotherapy efficacy (284) and patient’s response to immunotherapy (285) is also worth mentioning. AI can utilize complex images such as histopathological slides and follow-up CT scans, extract information from multi-omics data (genomics, transcriptomics, epigenomics, proteomics, radiomics), integrating it with clinical data (medical history, laboratory tests, demographic information) to distinguish immunotherapy responders from non-responders. One of the major challenges in immunotherapy is to determine which patients are likely to benefit from the therapy. Tumor mutational burden (TMB) was proposed as biomarker and approved by the FDA to select patients eligible to receive pembrolizumab. The review of Addala et al. (285) discusses cancer-intrinsic and cancer-extrinsic features that can be analysed. Besides TMB, genomic intratumor heterogeneity (ITH) can also be used as cancer-intrinsic feature for outcome prediction, as it was linked to treatment resistance, recurrence and reduced patient survival. Advances in single-cell analysis technologies enable further insights into genomic ITH, neoantigen formation and presentation at single-cell level. Cancer-extrinsic features encompass the cellular composition of the tumor microenvironment (TME). AI deconvolution tools, e.g. CIBERSORTx (286), provide estimates of the immune cell proportions in the TME. The complex model capable to integrate multiple factors including tumor purity, TME composition, tumor evolution, genomic ITH and immunogenic neoantigen load would be of great importance. The parameters that govern the immunogenicity still remain largely unknown. The review of Xie et al. (287) outlines further barriers that must be overcome to enable effective anti-cancer immunotherapies. Tumors can escape from immunological surveillance through a number of mechanisms, including the loss of neoantigens induced e.g. by transcriptional repression or epigenetic silencing, disruption of neoantigen peptides presentation, and immunosuppressive TME. To compensate for the loss of targetable neoantigens, personalized neoantigen-specific immunotherapy should target multiple neoantigens (288). In the work of Xie et al. (287) additional compensatory strategies to address the issue of immune evasion of tumor cells are discussed.
The recent publication of Donisi et al. (289) also considers the mechanisms behind the resistance to immune therapeutic agents, in particular, the tumor immune microenvironment (TIME), a part of the TME, or microbiome influencing immune cells in the TME etc., and reviews multi-omics and AI approaches, e.g. those for dissecting the TME or inferring novel microbiome-linked biomarkers (289).
In conclusion, the field of neoantigen prediction is at the forefront of personalized cancer immunotherapy. The collaborative efforts of researchers, computational biologists, and immunologists have brought us closer to harnessing the full potential of neoantigens for precision medicine. With continued advancements in software, databases, and AI, we are on the cusp of a new era in cancer treatment, one that holds the promise of tailored immunotherapies that target the unique molecular signatures of each patient’s tumor. As both academic and industrial endeavors keep on to tackle the challenges outlined in this article, the future of personalized cancer immunotherapy appears brighter than ever.
Author contributions
AB: Conceptualization, Investigation, Writing – original draft, Writing – review & editing. ZN: Conceptualization, Investigation, Visualization, Writing – original draft, Writing – review & editing. FL: Writing – review & editing. MB: Writing – review & editing. MM: Writing – review & editing. MD: Funding acquisition, Writing – review & editing. LC: Writing – review & editing. RK: Conceptualization, Funding acquisition, Supervision, Writing – original draft, Writing – review & editing.
Funding
The author(s) declare financial support was received for the research, authorship, and/or publication of this article. Funded by the Deutsche Forschungsgemeinschaft (DFG, German Research Foundation) – Project-ID 318346496 – SFB 1292/2 TP04 to RK; Project ID 318346496, SFB1292/2 TP17 to MD and by Federal Government German Ministry of Health (BMG) BMG-RENUBIA ZMI5-2521FSB412 to RK.
Acknowledgments
We would like to thank Silvia Vogl and Dóra Spekhardt for their helpful discussions and insights in connection with this manuscript.
Conflict of interest
The authors declare that the research was conducted in the absence of any commercial or financial relationships that could be construed as a potential conflict of interest.
Publisher’s note
All claims expressed in this article are solely those of the authors and do not necessarily represent those of their affiliated organizations, or those of the publisher, the editors and the reviewers. Any product that may be evaluated in this article, or claim that may be made by its manufacturer, is not guaranteed or endorsed by the publisher.
Footnotes
- ^ Radford A, Narasimhan K, Salimans T, Sutskever I. Improving Language Understanding. (2018) 4. OpenAI.com.
References
1. Haen SP, Löffler MW, Rammensee H-G, Brossart P. Towards new horizons: characterization, classification and implications of the tumour antigenic repertoire. Nat Rev Clin Oncol. (2020) 17:595–610. doi: 10.1038/s41571-020-0387-x
2. Sahin U, Türeci Ö. Personalized vaccines for cancer immunotherapy. Science. (2018) 359:1355–60. doi: 10.1126/science.aar7112
3. Hu Z, Leet DE, Allesøe RL, Oliveira G, Li S, Luoma AM, et al. Personal neoantigen vaccines induce persistent memory T cell responses and epitope spreading in patients with melanoma. Nat Med. (2021) 27:515–25. doi: 10.1038/s41591-020-01206-4
4. Rojas LA, Sethna Z, Soares KC, Olcese C, Pang N, Patterson E, et al. Personalized RNA neoantigen vaccines stimulate T cells in pancreatic cancer. Nature. (2023) 618:144–50. doi: 10.1038/s41586-023-06063-y
5. Aurisicchio L, Pallocca M, Ciliberto G, Palombo F. The perfect personalized cancer therapy: cancer vaccines against neoantigens. J Exp Clin Cancer Res. (2018) 37:86. doi: 10.1186/s13046-018-0751-1
6. Shemesh CS, Hsu JC, Hosseini I, Shen B-Q, Rotte A, Twomey P, et al. Personalized cancer vaccines: clinical landscape, challenges, and opportunities. Mol Ther. (2021) 29:555–70. doi: 10.1016/j.ymthe.2020.09.038
7. Chen I, Chen MY, Goedegebuure SP, Gillanders WE. Challenges targeting cancer neoantigens in 2021: a systematic literature review. Expert Rev Vaccines. (2021) 20:827–37. doi: 10.1080/14760584.2021.1935248
8. Biswas N, Chakrabarti S, Padul V, Jones LD, Ashili S. Designing neoantigen cancer vaccines, trials, and outcomes. Front Immunol. (2023) 14:1105420. doi: 10.3389/fimmu.2023.1105420
9. Richters MM, Xia H, Campbell KM, Gillanders WE, Griffith OL, Griffith M. Best practices for bioinformatic characterization of neoantigens for clinical utility. Genome Med. (2019) 11:56. doi: 10.1186/s13073-019-0666-2
10. Gopanenko AV, Kosobokova EN, Kosorukov VS. Main strategies for the identification of neoantigens. Cancers. (2020) 12:2879. doi: 10.3390/cancers12102879
11. Schaap-Johansen A-L, Vujović M, Borch A, Hadrup SR, Marcatili P. T cell epitope prediction and its application to immunotherapy. Front Immunol. (2021) 12:712488. doi: 10.3389/fimmu.2021.712488
12. Goodfellow I, Bengio Y, Courville A. Deep learning. Cambridge, Massachusetts, London, England: The MIT Press (2016).
13. Wainberg M, Merico D, Delong A, Frey BJ. Deep learning in biomedicine. Nat Biotechnol. (2018) 36:829–38. doi: 10.1038/nbt.4233
14. Wen B, Zeng W-F, Liao Y, Shi Z, Savage SR, Jiang W, et al. Deep learning in proteomics. Proteomics. (2020) 20:e1900335. doi: 10.1002/pmic.201900335
15. Zeng H, Gifford DK. DeepLigand: accurate prediction of MHC class I ligands using peptide embedding. Bioinformatics. (2019) 35:i278–83. doi: 10.1093/bioinformatics/btz330
16. Peters M, Neumann M, Iyyer M, Gardner M, Clark C, Lee K, et al. (2018). Deep contextualized word representations, in: Proceedings of the 2018 Conference of the North American Chapter of the Association for Computational Linguistics: Demonstrations. (New Orleans, Louisiana: Association for Computational Linguistics), pp. 2227–37.
17. Devlin J, Chang M-W, Lee K, Toutanova K. BERT: Pre-training of Deep Bidirectional Transformers for Language Understanding (2018). Available online at: https://arxiv.org/pdf/1810.04805.pdf.
18. Patwardhan N, Marrone S, Sansone C. Transformers in the real world: A survey on NLP applications. Inf (Switzerland). (2023) 14:242. doi: 10.3390/info14040242
19. Heinzinger M, Elnaggar A, Wang Yu, Dallago C, Nechaev D, Matthes F, et al. Modeling aspects of the language of life through transfer-learning protein sequences. BMC Bioinf. (2019) 20:723. doi: 10.1186/s12859-019-3220-8
20. Nambiar A, Heflin M, Liu S, Maslov S, Hopkins M, Ritz A. (2020). Transforming the language of life, in: Proceedings of the 11th ACM International Conference on Bioinformatics, Computational Biology and Health Informatics, New York, NY, USA: ACM. (New York, United States: Association for Computing Machinery), pp. 1–8.
21. Cheng J, Bendjama K, Rittner K, Malone B. BERTMHC: improved MHC-peptide class II interaction prediction with transformer and multiple instance learning. Bioinformatics. (2021) 37:4172–9. doi: 10.1093/bioinformatics/btab422
22. Wang F, Wang H, Wang L, Lu H, Qiu S, Zang T, et al. MHCRoBERTa: pan-specific peptide–MHC class I binding prediction through transfer learning with label-agnostic protein sequences. Briefings Bioinf. (2022) 23:bbab595. doi: 10.1093/bib/bbab595
23. Bhinder B, Gilvary C, Madhukar NS, Elemento O. Artificial intelligence in cancer research and precision medicine. Cancer Discovery. (2021) 11:900–15. doi: 10.1158/2159-8290.CD-21-0090
24. Xu Z, Wang X, Zeng S, Ren X, Yan Y, Gong Z. Applying artificial intelligence for cancer immunotherapy. Acta Pharm Sin B. (2021) 11:3393–405. doi: 10.1016/j.apsb.2021.02.007
25. Cai Y, Chen R, Gao S, Li W, Liu Y, Su G, et al. Artificial intelligence applied in neoantigen identification facilitates personalized cancer immunotherapy. Front Oncol. (2022) 12:1054231. doi: 10.3389/fonc.2022.1054231
26. Wingett SW, Andrews S. FastQ Screen: A tool for multi-genome mapping and quality control. F1000Research. (2018) 7:1338. doi: 10.12688/f1000research
27. Li H, Durbin R. Fast and accurate long-read alignment with Burrows-Wheeler transform. Bioinformatics. (2010) 26:589–95. doi: 10.1093/bioinformatics/btp698
28. DePristo MA, Banks E, Poplin R, Garimella KV, Maguire JR, Hartl C, et al. A framework for variation discovery and genotyping using next-generation DNA sequencing data. Nat Genet. (2011) 43:491–8. doi: 10.1038/ng.806
29. Cibulskis K, Lawrence MS, Carter SL, Sivachenko A, Jaffe D, Sougnez C, et al. Sensitive detection of somatic point mutations in impure and heterogeneous cancer samples. Nat Biotechnol. (2013) 31:213–9. doi: 10.1038/nbt.2514
30. Mose LE, Wilkerson MD, Hayes DN, Perou CM, Parker JS. ABRA: improved coding indel detection via assembly-based realignment. Bioinformatics. (2014) 30:2813–5. doi: 10.1093/bioinformatics/btu376
31. Saunders CT, Wong WSW, Swamy S, Becq J, Murray LJ, Cheetham RK. Strelka: accurate somatic small-variant calling from sequenced tumor-normal sample pairs. Bioinformatics. (2012) 28:1811–7. doi: 10.1093/bioinformatics/bts271
32. Koboldt DC, Chen K, Wylie T, Larson DE, McLellan MD, Mardis ER, et al. VarScan: variant detection in massively parallel sequencing of individual and pooled samples. Bioinformatics. (2009) 25:2283–5. doi: 10.1093/bioinformatics/btp373
33. Koboldt DC. Best practices for variant calling in clinical sequencing. Genome Med. (2020) 12:91. doi: 10.1186/s13073-020-00791-w
34. Cancer Hotspots (2023). Available online at: https://www.cancerhotspots.org/#/home.
35. Cosmic. CMC (2023). Available online at: https://cancer.sanger.ac.uk/cmc/home.
36. VICC. Standardizing cancer variant knowledge to enable precision oncology (2023). Available online at: https://cancervariants.org/.
37. Wagner AH, Walsh B, Mayfield G, Tamborero D, Sonkin D, Krysiak K, et al. A harmonized meta-knowledgebase of clinical interpretations of somatic genomic variants in cancer. Nat Genet. (2020) 52:448–57. doi: 10.1038/s41588-020-0603-8
38. Sivachenko A. Comparison and validation of somatic mutation callers. In: The Cancer Genome Atlas First Annual Scientific Symposium. National Human Genome Research Institute, Washington, D.C (2011). Available at: https://www.genome.gov/27546242/the-cancer-genome-atlas-first-annual-scientific-symposium.
39. Warden CD, Adamson AW, Neuhausen SL, Wu X. Detailed comparison of two popular variant calling packages for exome and targeted exon studies. PeerJ. (2014) 2:e600. doi: 10.7717/peerj.600
40. Alioto TS, Buchhalter I, Derdak S, Hutter B, Eldridge MD, Hovig E, et al. A comprehensive assessment of somatic mutation detection in cancer using whole-genome sequencing. Nat Commun. (2015) 6:10001. doi: 10.1038/ncomms10001
41. Frankiw L, Baltimore D, Li G. Alternative mRNA splicing in cancer immunotherapy. Nat Rev Immunol. (2019) 19:675–87. doi: 10.1038/s41577-019-0195-7
42. Srivastava AK, Guadagnin G, Cappello P, Novelli F. Post-translational modifications in tumor-associated antigens as a platform for novel immuno-oncology therapies. Cancers. (2022) 15:138. doi: 10.3390/cancers15010138
43. Wang Y, Shi T, Song X, Liu B, Wei J. Gene fusion neoantigens: Emerging targets for cancer immunotherapy. Cancer Lett. (2021) 506:45–54. doi: 10.1016/j.canlet.2021.02.023
44. Capietto A-H, Hoshyar R, Delamarre L. Sources of cancer neoantigens beyond single-nucleotide variants. Int J Mol Sci. (2022) 23:10131. doi: 10.3390/ijms231710131
45. Li X, Zhou C, Chen K, Huang B, Liu Q, Ye H. Benchmarking HLA genotyping and clarifying HLA impact on survival in tumor immunotherapy. Mol Oncol. (2021) 15:1764–82. doi: 10.1002/1878-0261.12895
46. Bauer DC, Zadoorian A, Wilson LOW, Thorne NP. Evaluation of computational programs to predict HLA genotypes from genomic sequencing data. Briefings Bioinf. (2018) 19:179–87. doi: 10.1093/bib/bbw097
47. Wirtz C, Sayer D. Data analysis of HLA sequencing using Assign-SBT v3.6+ from Conexio. Methods Mol Biol. (2012) 882:87–121. doi: 10.1007/978-1-61779-842-9_6
48. Rozemuller EH, Geerligs J, Penning MT, Mulder W. P077 : IMPROVED SBTENGINE BATCH ANALYSIS MODULE. Hum Immunol. (2014) 75:103. doi: 10.1016/j.humimm.2014.08.139
49. Zhang Y, Chen Y, Xu H, Fang J, Zhao Z, Hu W, et al. SOAPTyping: an open-source and cross-platform tool for sequence-based typing for HLA class I and II alleles. BMC Bioinf. (2020) 21:295. doi: 10.1186/s12859-020-03624-0
50. Klasberg S, Surendranath V, Lange V, Schöfl G. Bioinformatics strategies, challenges, and opportunities for next generation sequencing-based HLA genotyping. Transfus Med Hemother. (2019) 46:312–25. doi: 10.1159/000502487
51. Szolek A, Schubert B, Mohr C, Sturm M, Feldhahn M, Kohlbacher O. OptiType: precision HLA typing from next-generation sequencing data. Bioinformatics. (2014) 30:3310–6. doi: 10.1093/bioinformatics/btu548
52. Boegel S, Löwer M, Schäfer M, Bukur T, de GJ, Boisguérin V, et al. HLA typing from RNA-Seq sequence reads. Genome Med. (2012) 4:102. doi: 10.1186/gm403
53. Warren RL, Choe G, Freeman DJ, Castellarin M, Munro S, Moore R, et al. Derivation of HLA types from shotgun sequence datasets. Genome Med. (2012) 4:95. doi: 10.1186/gm396
54. Liu C, Yang X, Duffy B, Mohanakumar T, Mitra RD, Zody MC, et al. ATHLATES: accurate typing of human leukocyte antigen through exome sequencing. Nucleic Acids Res. (2013) 41:e142. doi: 10.1093/nar/gkt481
55. Cao H, Wu J, Wang Y, Jiang H, Zhang T, Liu X, et al. An integrated tool to study MHC region: accurate SNV detection and HLA genes typing in human MHC region using targeted high-throughput sequencing. PloS One. (2013) 8:e69388. doi: 10.1371/journal.pone.0069388
56. Kim HJ, Pourmand N. HLA typing from RNA-seq data using hierarchical read weighting corrected. PloS One. (2013) 8:e67885. doi: 10.1371/journal.pone.0067885
57. Bai Y, Ni M, Cooper B, Wei Y, Fury W. Inference of high resolution HLA types using genome-wide RNA or DNA sequencing reads. BMC Genomics. (2014) 15:325. doi: 10.1186/1471-2164-15-325
58. Farrell JJ, Jun G, Farrer LA, DeStefano A, Sebastiani P. (2014). HLA-genotyper prediction of HLA genotypes from next generation sequencing data, in: 64th Annual Meeting of The American Society of Human Genetics, San Diego, CA.
59. Huang Y, Yang J, Ying D, Zhang Y, Shotelersuk V, Hirankarn N, et al. HLAreporter: a tool for HLA typing from next generation sequencing data. Genome Med. (2015) 7:25. doi: 10.1186/s13073-015-0145-3
60. Shukla SA, Rooney MS, Rajasagi M, Tiao G, Dixon PM, Lawrence MS, et al. Comprehensive analysis of cancer-associated somatic mutations in class I HLA genes. Nat Biotechnol. (2015) 33:1152–8. doi: 10.1038/nbt.3344
61. Nariai N, Kojima K, Saito S, Mimori T, Sato Y, Kawai Y, et al. HLA-VBSeq: accurate HLA typing at full resolution from whole-genome sequencing data. BMC Genomics. (2015) 16 Suppl 2:S7. doi: 10.1186/1471-2164-16-S2-S7
62. Kawaguchi S, Higasa K, Shimizu M, Yamada R, Matsuda F. HLA-HD: An accurate HLA typing algorithm for next-generation sequencing data. Hum Mutat. (2017) 38:788–97. doi: 10.1002/humu.23230
63. Xie C, Yeo ZX, Wong M, Piper J, Long T, Kirkness EF, et al. Fast and accurate HLA typing from short-read next-generation sequence data with xHLA. Proc Natl Acad Sci United States America. (2017) 114:8059–64. doi: 10.1073/pnas.1707945114
64. Lee H, Kingsford C. Kourami: graph-guided assembly for novel human leukocyte antigen allele discovery. Genome Biol. (2018) 19:16. doi: 10.1186/s13059-018-1388-2
65. Dilthey AT, Mentzer AJ, Carapito R, Cutland C, Cereb N, Madhi SA, et al. HLA*LA-HLA typing from linearly projected graph alignments. Bioinformatics. (2019) 35:4394–6. doi: 10.1093/bioinformatics/btz235
66. Orenbuch R, Filip I, Comito D, Shaman J, Pe'er I, Rabadan R. arcasHLA: high-resolution HLA typing from RNAseq. Bioinformatics. (2020) 36:33–40. doi: 10.1093/bioinformatics/btz474
67. Matey-Hernandez ML, Brunak S, Izarzugaza JMG. Benchmarking the HLA typing performance of Polysolver and Optitype in 50 Danish parental trios. BMC Bioinf. (2018) 19:239. doi: 10.1186/s12859-018-2239-6
68. ccg - National Cancer Institute. The Cancer Genome Atlas Program (TCGA) (2023). Available online at: https://www.cancer.gov/ccg/research/genome-sequencing/tcga.
69. Claeys A, Merseburger P, Staut J, Marchal K, van den Eynden J. Benchmark of tools for in silico prediction of MHC class I and class II genotypes from NGS data. BMC Genomics. (2023) 24:247. doi: 10.1186/s12864-023-09351-z
70. Nielsen M, Andreatta M, Peters B, Buus S. Immunoinformatics: predicting peptide-MHC binding. Annu Rev Biomed Data Sci. (2020) 3:191–215. doi: 10.1146/annurev-biodatasci-021920-100259
71. Rammensee H, Bachmann J, Emmerich NP, Bachor OA, Stevanović S. SYFPEITHI: database for MHC ligands and peptide motifs. Immunogenetics. (1999) 50:213–9. doi: 10.1007/s002510050595
72. Reche PA, Glutting J-P, Reinherz EL. Prediction of MHC class I binding peptides using profile motifs. Hum Immunol. (2002) 63:701–9. doi: 10.1016/S0198-8859(02)00432-9
73. Zhang H, Lund O, Nielsen M. The PickPocket method for predicting binding specificities for receptors based on receptor pocket similarities: application to MHC-peptide binding. Bioinformatics. (2009) 25:1293–9. doi: 10.1093/bioinformatics/btp137
74. Bassani-Sternberg M, Chong C, Guillaume P, Solleder M, Pak H, Gannon PO, et al. Deciphering HLA-I motifs across HLA peptidomes improves neo-antigen predictions and identifies allostery regulating HLA specificity. PloS Comput Biol. (2017) 13:e1005725. doi: 10.1371/journal.pcbi.1005725
75. Nielsen M, Lundegaard C, Worning P, Lauemøller SL, Lamberth K, Buus S, et al. Reliable prediction of T-cell epitopes using neural networks with novel sequence representations. Protein Sci. (2003) 12:1007–17. doi: 10.1110/ps.0239403
76. Bassani-Sternberg M, Bräunlein E, Klar R, Engleitner T, Sinitcyn P, Audehm S, et al. Direct identification of clinically relevant neoepitopes presented on native human melanoma tissue by mass spectrometry. Nat Commun. (2016) 7:13404. doi: 10.1038/ncomms13404
77. Abelin JG, Keskin DB, Sarkizova S, Hartigan CR, Zhang W, Sidney J, et al. Mass spectrometry profiling of HLA-associated peptidomes in mono-allelic cells enables more accurate epitope prediction. Immunity. (2017) 46:315–26. doi: 10.1016/j.immuni.2017.02.007
78. Müller M, Gfeller D, Coukos G, Bassani-Sternberg M. 'Hotspots' of antigen presentation revealed by human leukocyte antigen ligandomics for neoantigen prioritization. Front Immunol. (2017) 8:1367. doi: 10.3389/fimmu.2017.01367
79. Freudenmann LK, Marcu A, Stevanović S. Mapping the tumour human leukocyte antigen (HLA) ligandome by mass spectrometry. Immunology. (2018) 154:331–45. doi: 10.1111/imm.12936
80. Reynisson B, Alvarez B, Paul S, Peters B, Nielsen M. NetMHCpan-4.1 and NetMHCIIpan-4.0: improved predictions of MHC antigen presentation by concurrent motif deconvolution and integration of MS MHC eluted ligand data. Nucleic Acids Res. (2020) 48:W449–54. doi: 10.1093/nar/gkaa379
81. Alvarez B, Reynisson B, Barra C, Buus Søren, Ternette N, Connelley T, et al. NNAlign_MA; MHC peptidome deconvolution for accurate MHC binding motif characterization and improved T-cell epitope predictions. Mol Cell Proteomics MCP. (2019) 18:2459–77. doi: 10.1074/mcp.TIR119.001658
82. Nielsen M, Andreatta M. NetMHCpan-3.0; improved prediction of binding to MHC class I molecules integrating information from multiple receptor and peptide length datasets. Genome Med. (2016) 8:33. doi: 10.1186/s13073-016-0288-x
83. Jurtz V, Paul S, Andreatta M, Marcatili P, Peters B, Nielsen M. NetMHCpan-4.0: improved peptide-MHC class I interaction predictions integrating eluted ligand and peptide binding affinity data. J Immunol. (2017) 199:3360–8. doi: 10.4049/jimmunol.1700893
84. Shao XM, Bhattacharya R, Huang J, Sivakumar IK, Tokheim C, Zheng L, et al. High-throughput prediction of MHC Class i and II neoantigens with MH cnuggets. Cancer Immunol Res. (2020) 8:396–408. doi: 10.1158/2326-6066.CIR-19-0464
85. Gasser H-C, Bedran G, Ren B, Goodlett D, Alfaro J, Rajan A. Interpreting BERT architecture predictions for peptide presentation by MHC class I proteins. arXiv preprint arXiv (2021).
86. Rao R, Bhattacharya N, Thomas N, Duan Y, Chen P, Canny J, et al. Evaluating protein transfer learning with TAPE. In: Advances in Neural Information Processing Systems. New York, United States: Curran Associates, Inc (2019). Available at: https://proceedings.neurips.cc/paper_files/paper/2019/file/37f65c068b7723cd7809ee2d31d7861c-Paper.pdf.
87. UniProt: the universal protein knowledgebase in 2023. Nucleic Acids Res. (2023) 51:D523–31. doi: 10.1093/nar/gkac1052
88. Vita R, Mahajan S, Overton JA, Dhanda SK, Martini S, Cantrell JR, et al. The immune epitope database (IEDB): 2018 update. Nucleic Acids Res. (2019) 47:D339–43. doi: 10.1093/nar/gky1006
89. Han Y, Kim D. Deep convolutional neural networks for pan-specific peptide-MHC class I binding prediction. BMC Bioinf. (2017) 18:585. doi: 10.1186/s12859-017-1997-x
90. Vang YS, Xie X. HLA class I binding prediction via convolutional neural networks. Bioinformatics. (2017) 33:2658–65. doi: 10.1093/bioinformatics/btx264
91. Hu J, Liu Z. DeepMHC: deep convolutional neural networks for high-performance peptide-MHC binding affinity prediction. bioRxiv (2017). doi: 10.1101/239236
92. Phloyphisut P, Pornputtapong N, Sriswasdi S, Chuangsuwanich E. MHCSeqNet: a deep neural network model for universal MHC binding prediction. BMC Bioinf. (2019) 20:270. doi: 10.1186/s12859-019-2892-4
93. O'Donnell TJ, Rubinsteyn A, Bonsack M, Riemer AB, Laserson U, Hammerbacher J. MHCflurry: open-source class I MHC binding affinity prediction. Cell Syst. (2018) 7:129–32.e4. doi: 10.1016/j.cels.2018.05.014
94. Karosiene E, Lundegaard C, Lund O, Nielsen M. NetMHCcons: a consensus method for the major histocompatibility complex class I predictions. Immunogenetics. (2012) 64:177–86. doi: 10.1007/s00251-011-0579-8
95. O'Donnell TJ, Rubinsteyn A, Laserson U. MHCflurry 2.0: improved pan-allele prediction of MHC class I-presented peptides by incorporating antigen processing. Cell Syst. (2020) 11:42–48.e7. doi: 10.1016/j.cels.2020.06.010
96. Martins J, Magalhães C, Vieira V, Rocha M, Osório NS. HABIT – a webserver for interactive T cell neoepitope discovery. bioRxiv (2019). doi: 10.1101/535716
97. Bulik-Sullivan B, Busby J, Palmer CD, Davis MJ, Murphy T, Clark A, et al. Deep learning using tumor HLA peptide mass spectrometry datasets improves neoantigen identification. Nat Biotechnol. (2018) 37 (1), 55-63. doi: 10.1038/nbt.4313
98. Chen B, Khodadoust MS, Olsson N, Wagar LE, Fast E, Liu CL, et al. Predicting HLA class II antigen presentation through integrated deep learning. Nat Biotechnol. (2019) 37:1332–43. doi: 10.1038/s41587-019-0280-2
99. Sarkizova S, Klaeger S, Le PM, Li LW, Oliveira G, Keshishian H, et al. A large peptidome dataset improves HLA class I epitope prediction across most of the human population. Nat Biotechnol. (2020) 38:199–209. doi: 10.1038/s41587-019-0322-9
100. Pyke RM, Mellacheruvu D, Dea S, Abbott C, Zhang SV, Phillips NA, et al. Precision neoantigen discovery using large-scale immunopeptidomes and composite modeling of MHC peptide presentation. Mol Cell Proteomics MCP. (2023) 22:100506. doi: 10.1016/j.mcpro.2023.100506
101. Andreatta M, Nielsen M. Gapped sequence alignment using artificial neural networks: application to the MHC class I system. Bioinformatics. (2016) 32:511–7. doi: 10.1093/bioinformatics/btv639
102. Sidhom J-W, Pardoll D, Baras A. AI-MHC: an allele-integrated deep learning framework for improving Class I & Class II HLA-binding predictions. BioRxiv (2018). doi: 10.1101/318881
103. Wu J, Wang W, Zhang J, Zhou B, Zhao W, Su Z, et al. DeepHLApan: A deep learning approach for neoantigen prediction considering both HLA-peptide binding and immunogenicity. Front Immunol. (2019) 10:2559. doi: 10.3389/fimmu.2019.02559
104. Zhao T, Cheng L, Zang T, Hu Y. Peptide-major histocompatibility complex class I binding prediction based on deep learning with novel feature. Front Genet. (2019) 10:1191. doi: 10.3389/fgene.2019.01191
105. Zeng H, Gifford DK. Quantification of uncertainty in peptide-MHC binding prediction improves high-affinity peptide selection for therapeutic design. Cell Syst. (2019) 9:159–166.e3. doi: 10.1016/j.cels.2019.05.004
106. Abelin JG, Harjanto D, Malloy M, Suri P, Colson T, Goulding SP, et al. Defining HLA-II ligand processing and binding rules with mass spectrometry enhances cancer epitope prediction. Immunity. (2019) 51:766–779.e17. doi: 10.1016/j.immuni.2020.12.005
107. Xie X, Han Y, Zhang K. (2019). MHCherryPan. a novel model to predict the binding affinity of pan-specific class I HLA-peptide, in: 2019 IEEE International Conference on Bioinformatics and Biomedicine: November 18-21, 2019, San Diego, CA, USA : proceedings. Piscataway, NJ, USA: IEEE. pp. 548–54.
108. Liu Z, Cui Y, Xiong Z, Nasiri A, Zhang A, Hu J. DeepSeqPan, a novel deep convolutional neural network model for pan-specific class I HLA-peptide binding affinity prediction. Sci Rep. (2019) 9:794. doi: 10.1038/s41598-018-37214-1
109. Liu Z, Jin J, Cui Y, Xiong Z, Nasiri A, Zhao Y, et al. DeepSeqPanII: an interpretable recurrent neural network model with attention mechanism for peptide-HLA class II binding prediction. IEEE/ACM Trans Comput Biol Bioinf. (2022) 19:2188–96. doi: 10.1109/TCBB.2021.3074927
110. Hu Y, Wang Z, Hu H, Wan F, Chen L, Xiong Y, et al. ACME: pan-specific peptide-MHC class I binding prediction through attention-based deep neural networks. Bioinformatics. (2019) 35:4946–54. doi: 10.1093/bioinformatics/btz427
111. Vielhaben J, Wenzel M, Samek W, Strodthoff N. USMPep: universal sequence models for major histocompatibility complex binding affinity prediction. BMC Bioinf. (2020) 21:279. doi: 10.1186/s12859-020-03631-1
112. Pei B, Hsu Y-H. IConMHC: a deep learning convolutional neural network model to predict peptide and MHC-I binding affinity. Immunogenetics. (2020) 72:295–304. doi: 10.1007/s00251-020-01163-9
113. Venkatesh G, Grover A, Srinivasaraghavan G, Rao S. MHCAttnNet: predicting MHC-peptide bindings for MHC alleles classes I and II using an attention-based deep neural model. Bioinformatics. (2020) 36:i399–406. doi: 10.1093/bioinformatics/btaa479
114. Jin J, Liu Z, Nasiri A, Cui Y, Louis S-Y, Zhang A, et al. Deep learning pan-specific model for interpretable MHC-I peptide binding prediction with improved attention mechanism. Proteins. (2021) 89:866–83. doi: 10.1002/prot.26065
115. Yang X, Zhao L, Wei F, Li J. DeepNetBim: deep learning model for predicting HLA-epitope interactions based on network analysis by harnessing binding and immunogenicity information. BMC Bioinf. (2021) 22:231. doi: 10.1186/s12859-021-04155-y
116. Ye Y, Wang J, Xu Y, Wang Y, Pan Y, Song Q, et al. MATHLA: a robust framework for HLA-peptide binding prediction integrating bidirectional LSTM and multiple head attention mechanism. BMC Bioinf. (2021) 22:7. doi: 10.1186/s12859-020-03946-z
117. Xu S, Wang X, Fei C. A highly effective system for predicting MHC-II epitopes with immunogenicity. Front Oncol. (2022) 12:888556. doi: 10.3389/fonc.2022.888556
118. Thrift WJ, Lounsbury NW, Broadwell Q, Heidersbach A, Freund E, Abdolazimi Y, et al. HLApollo: A superior transformer model for pan-allelic peptide-MHC-I presentation prediction, with diverse negative coverage, deconvolution and protein language features. bioRxiv (2022) 2022.12.08.519673. doi: 10.1101/2022.12.08.519673
119. Zhang Y, Zhu G, Li K, Li F, Huang L, Duan M, et al. HLAB: learning the BiLSTM features from the ProtBert-encoded proteins for the class I HLA-peptide binding prediction. Briefings Bioinf. (2022) 23:bbac173. doi: 10.1093/bib/bbac173
120. Kim JY, Bang H, Noh S-J, Choi JK. DeepNeo: a webserver for predicting immunogenic neoantigens. Nucleic Acids Res. (2023) 51:W134–40. doi: 10.1093/nar/gkad275
121. Deng J, Zhou X, Zhang P, Cheng W, Liu M, Tian J. IEPAPI: a method for immune epitope prediction by incorporating antigen presentation and immunogenicity. Briefings Bioinf. (2023) 24:bbad171. doi: 10.1093/bib/bbad171
122. Racle J, Guillaume P, Schmidt J, Michaux J, Larabi A, Lau K, et al. Machine learning predictions of MHC-II specificities reveal alternative binding mode of class II epitopes. Immunity. (2023) 56:1359–75.e13. doi: 10.1016/j.immuni.2023.03.009
123. Kalemati M, Darvishi S, Koohi S. CapsNet-MHC predicts peptide-MHC class I binding based on capsule neural networks. Commun Biol. (2023) 6:492. doi: 10.1038/s42003-023-04867-2
124. Qu W, You R, Mamitsuka H, Zhu S. DeepMHCI: an anchor position-aware deep interaction model for accurate MHC-I peptide binding affinity prediction. Bioinformatics. (2023) 39:btad551. doi: 10.1093/bioinformatics/btad551
125. Gfeller D, Schmidt J, Croce G, Guillaume P, Bobisse S, Genolet R, et al. Improved predictions of antigen presentation and TCR recognition with MixMHCpred2.2 and PRIME2.0 reveal potent SARS-CoV-2 CD8+ T-cell epitopes. Cell Syst. (2023) 14:72–83.e5. doi: 10.1016/j.cels.2022.12.002
126. Wang G, Wu T, Ning W, Diao K, Sun X, Wang J, et al. TLimmuno2: predicting MHC class II antigen immunogenicity through transfer learning. Briefings Bioinf. (2023) 24:bbad116. doi: 10.1093/bib/bbad116
127. Nilsson JB, Kaabinejadian S, Yari H, Peters B, Barra C, Gragert L, et al. Machine learning reveals limited contribution of trans-only encoded variants to the HLA-DQ immunopeptidome. Commun Biol. (2023) 6:442. doi: 10.1038/s42003-023-04749-7
128. Tadros DM, Eggenschwiler S, Racle J, Gfeller D. The MHC Motif Atlas: a database of MHC binding specificities and ligands. Nucleic Acids Res. (2023) 51:D428–37. doi: 10.1093/nar/gkac965
129. MHC Motif Atlas (2023). Available online at: http://mhcmotifatlas.org/home.
130. Racle J, Michaux J, Rockinger GA, Arnaud M, Bobisse S, Chong C, et al. Robust prediction of HLA class II epitopes by deep motif deconvolution of immunopeptidomes. Nat Biotechnol. (2019) 37:1283–6. doi: 10.1038/s41587-019-0289-6
131. Degoot AM, Chirove F, Ndifon W. Trans-allelic model for prediction of peptide:MHC-II interactions. Front Immunol. (2018) 9:1410. doi: 10.3389/fimmu.2018.01410
132. Jensen KK, Andreatta M, Marcatili P, Buus S, Greenbaum JA, Yan Z, et al. Improved methods for predicting peptide binding affinity to MHC class II molecules. Immunology. (2018) 154:394–406. doi: 10.1111/imm.12889
133. Bhattacharya R, Tokheim C, Sivakumar A, Guthrie VB, Anagnostou V, Velculescu VE, et al. Prediction of peptide binding to MHC Class I proteins in the age of deep learning. bioRxiv. (2017), 154757. doi: 10.1101/154757
134. Kreiter S, Vormehr M, van de Roemer N, Diken M, Löwer M, Diekmann J, et al. Mutant MHC class II epitopes drive therapeutic immune responses to cancer. Nature. (2015) 520:692–6. doi: 10.1038/nature14426
135. Trolle T, Metushi IG, Greenbaum JA, Kim Y, Sidney J, Lund O, et al. Automated benchmarking of peptide-MHC class I binding predictions. Bioinformatics. (2015) 31:2174–81. doi: 10.1093/bioinformatics/btv123
136. Automated Server Benchmarks (2023). Available online at: http://tools.iedb.org/auto_bench/mhci/weekly/.
137. Trevizani R, Yan Z, Greenbaum JA, Sette A, Nielsen M, Peters B. A comprehensive analysis of the IEDB MHC class-I automated benchmark. Briefings Bioinf. (2022) 23:bbac259. doi: 10.1093/bib/bbac259
138. Lundegaard C, Lund O, Nielsen M. Accurate approximation method for prediction of class I MHC affinities for peptides of length 8, 10 and 11 using prediction tools trained on 9mers. Bioinformatics. (2008) 24:1397–8. doi: 10.1093/bioinformatics/btn128
139. Hoof I, Peters B, Sidney J, Pedersen LE, Sette A, Lund O, et al. NetMHCpan, a method for MHC class I binding prediction beyond humans. Immunogenetics. (2009) 61:1–13. doi: 10.1007/s00251-008-0341-z
140. Mei S, Li F, Leier A, Marquez-Lago TT, Giam K, Croft NP, et al. A comprehensive review and performance evaluation of bioinformatics tools for HLA class I peptide-binding prediction. Briefings Bioinf. (2020) 21:1119–35. doi: 10.1093/bib/bbz051
141. Gfeller D, Guillaume P, Michaux J, Pak H-S, Daniel RT, Racle J, et al. The length distribution and multiple specificity of naturally presented HLA-I ligands. J Immunol. (2018) 201:3705–16. doi: 10.4049/jimmunol.1800914
142. Montemurro A, Schuster V, Povlsen HR, Bentzen AK, Jurtz V, Chronister WD, et al. NetTCR-2.0 enables accurate prediction of TCR-peptide binding by using paired TCRα and β sequence data. Commun Biol. (2021) 4:1060. doi: 10.1038/s42003-021-02610-3
143. Dash P, Fiore-Gartland AJ, Hertz T, Wang GC, Sharma S, Souquette A, et al. Quantifiable predictive features define epitope-specific T cell receptor repertoires. Nature. (2017) 547:89–93. doi: 10.1038/nature22383
144. Lanzarotti E, Marcatili P, Nielsen M. T-cell receptor cognate target prediction based on paired α and β Chain sequence and structural CDR loop similarities. Front Immunol. (2019) 10:2080. doi: 10.3389/fimmu.2019.02080
145. Scheper W, Kelderman S, Fanchi LF, Linnemann C, Bendle G, de RMAJ, et al. Low and variable tumor reactivity of the intratumoral TCR repertoire in human cancers. Nat Med. (2019) 25:89–94. doi: 10.1038/s41591-018-0266-5
146. Sidhom J-W, Bessell CA, Havel JJ, Kosmides A, Chan TA, Schneck JP. ImmunoMap: A bioinformatics tool for T-cell repertoire analysis. Cancer Immunol Res. (2018) 6:151–62. doi: 10.1158/2326-6066.CIR-17-0114
147. Chen S-Y, Yue T, Lei Q, Guo A-Y. TCRdb: a comprehensive database for T-cell receptor sequences with powerful search function. Nucleic Acids Res. (2021) 49:D468–74. doi: 10.1093/nar/gkaa796
148. Altman JD, Moss PA, Goulder PJ, Barouch DH, McHeyzer-Williams MG, Bell JI, et al. Phenotypic analysis of antigen-specific T lymphocytes. Science. (1996) 274:94–6. doi: 10.1126/science.274.5284.94
149. Zhang S-Q, Ma K-Y, Schonnesen AA, Zhang M, He C, Sun E, et al. High-throughput determination of the antigen specificities of T cell receptors in single cells. Nat Biotechnol. (2018). doi: 10.1101/457069
150. Kula T, Dezfulian MH, Wang CI, Abdelfattah NS, Hartman ZC, Wucherpfennig KW, et al. T-scan: A genome-wide method for the systematic discovery of T cell epitopes. Cell. (2019) 178:1016–28.e13. doi: 10.1016/j.cell.2019.07.009
151. Gielis S, Moris P, Bittremieux W, de Neuter N, Ogunjimi B, Laukens K, et al. Detection of enriched T cell epitope specificity in full T cell receptor sequence repertoires. Front Immunol. (2019) 10:2820. doi: 10.3389/fimmu.2019.02820
152. Sidhom J-W, Larman HB, Pardoll DM, Baras AS. DeepTCR is a deep learning framework for revealing sequence concepts within T-cell repertoires. Nat Commun. (2021) 12:1605. doi: 10.1038/s41467-021-21879-w
153. Jurtz VI, Jessen LE, Bentzen AK, Jespersen MC, Mahajan S, Vita R, et al. NetTCR: sequence-based prediction of TCR binding to peptide-MHC complexes using convolutional neural networks. bioRxiv (2018) 433706. doi: 10.1101/433706
154. Home Page - 10x Genomics (2023). Available online at: https://www.10xgenomics.com/.
155. Sanromán ÁF, Joshi K, Au L, Chain B, Turajlic S. TCR sequencing: applications in immuno-oncology research. Immunooncol Technol. (2023) 17:100373. doi: 10.1016/j.iotech.2023.100373
156. Montemurro A, Jessen LE, Nielsen M. NetTCR-2.1: Lessons and guidance on how to develop models for TCR specificity predictions. Front Immunol. (2022) 13:1055151. doi: 10.3389/fimmu.2022.1055151
157. Lu T, Zhang Z, Zhu J, Wang Y, Jiang P, Xiao X, et al. Deep learning-based prediction of the T cell receptor-antigen binding specificity. Nat Mach Intell. (2021) 3:864–75. doi: 10.1038/s42256-021-00383-2
158. Atchley WR, Zhao J, Fernandes AD, Drüke T. Solving the protein sequence metric problem. Proc Natl Acad Sci. (2005) 102:6395–400. doi: 10.1073/pnas.0408677102
159. Myronov A, Mazzocco G, Król P, Plewczynski D. BERTrand-peptide:TCR binding prediction using Bidirectional Encoder Representations from Transformers augmented with random TCR pairing. Bioinformatics. (2023) 39:btad468. doi: 10.1093/bioinformatics/btad468
160. Springer I, Besser H, Tickotsky-Moskovitz N, Dvorkin S, Louzoun Y. Prediction of specific TCR-peptide binding from large dictionaries of TCR-peptide pairs. Front Immunol. (2020) 11:1803. doi: 10.3389/fimmu.2020.01803
161. Springer I, Tickotsky N, Louzoun Y. Contribution of T cell receptor alpha and beta CDR3, MHC typing, V and J genes to peptide binding prediction. Front Immunol. (2021) 12:664514. doi: 10.3389/fimmu.2021.664514
162. Xu Z, Luo M, Lin W, Xue G, Wang P, Jin X, et al. DLpTCR: an ensemble deep learning framework for predicting immunogenic peptide recognized by T cell receptor. Briefings Bioinf. (2021) 22:bbab335. doi: 10.1093/bib/bbab335
163. Zhang W, Hawkins PG, He J, Gupta NT, Liu J, Choonoo G, et al. A framework for highly multiplexed dextramer mapping and prediction of T cell receptor sequences to antigen specificity. Sci Adv. (2021) 7:eabf5835. doi: 10.1126/sciadv.abf5835
164. Jokinen E, Huuhtanen J, Mustjoki S, Heinonen M, Lähdesmäki H. Predicting recognition between T cell receptors and epitopes with TCRGP. PloS Comput Biol. (2021) 17:e1008814. doi: 10.1371/journal.pcbi.1008814
165. Moris P, de PJ, Postovskaya A, Gielis S, de Neuter N, Bittremieux W, et al. Current challenges for unseen-epitope TCR interaction prediction and a new perspective derived from image classification. Briefings Bioinf. (2021) 22:bbaa318. doi: 10.1093/bib/bbaa318
166. Weber A, Born J, Rodriguez Martínez M. TITAN: T-cell receptor specificity prediction with bimodal attention networks. Bioinformatics. (2021) 37:i237–44. doi: 10.1093/bioinformatics/btab294
167. Xu Y, Qian X, Tong Y, Li F, Wang K, Zhang X, et al. AttnTAP: A dual-input framework incorporating the attention mechanism for accurately predicting TCR-peptide binding. Front Genet. (2022) 13:942491. doi: 10.3389/fgene.2022.942491
168. Cai M, Bang S, Zhang P, Lee H. ATM-TCR: TCR-epitope binding affinity prediction using a multi-head self-attention model. Front Immunol. (2022) 13:893247. doi: 10.3389/fimmu.2022.893247
169. Pham M-DN, Nguyen T-N, Le Tran S, Nguyen Q-TB, Nguyen T-PH, Pham TMQ, et al. epiTCR: a highly sensitive predictor for TCR-peptide binding. Bioinformatics. (2023) 39:btad284. doi: 10.1093/bioinformatics/btad284
170. Zhang Y, Jian X, Xu L, Zhao J, Lu M, Lin Y, et al. iTCep: a deep learning framework for identification of T cell epitopes by harnessing fusion features. Front Genet. (2023) 14:1141535. doi: 10.3389/fgene.2023.1141535
171. Hudson D, Fernandes RA, Basham M, Ogg G, Koohy H. Can we predict T cell specificity with digital biology and machine learning? Nat Rev Immunol. (2023) 23:511–21. doi: 10.1038/s41577-023-00835-3
172. Tickotsky N, Sagiv T, Prilusky J, Shifrut E, Friedman N. McPAS-TCR: a manually curated catalogue of pathology-associated T cell receptor sequences. Bioinformatics. (2017) 33:2924–9. doi: 10.1093/bioinformatics/btx286
173. Bagaev DV, Vroomans RMA, Samir J, Stervbo U, Rius C, Dolton G, et al. VDJdb in 2019: database extension, new analysis infrastructure and a T-cell receptor motif compendium. Nucleic Acids Res. (2020) 48:D1057–62. doi: 10.1093/nar/gkz874
174. Zhang W, Wang L, Liu K, Wei X, Yang K, Du W, et al. PIRD: pan immune repertoire database. Bioinformatics. (2020) 36:897–903. doi: 10.1093/bioinformatics/btz614
175. Klinger M, Pepin F, Wilkins J, Asbury T, Wittkop T, Zheng J, et al. Multiplex identification of antigen-specific T cell receptors using a combination of immune assays and immune receptor sequencing. PloS One. (2015) 10:e0141561. doi: 10.1371/journal.pone.0141561
176. GitHub. NetTCR-2.0/data at main · mnielLab/NetTCR-2.0 (2023). Available online at: https://github.com/mnielLab/NetTCR-2.0/tree/main/data.
177. Frank ML, Lu K, Erdogan C, Han Y, Hu J, Wang T, et al. T-cell receptor repertoire sequencing in the era of cancer immunotherapy. Clin Cancer Res. (2023) 29:994–1008. doi: 10.1158/1078-0432.CCR-22-2469
178. Grazioli F, Mösch A, Machart P, Li K, Alqassem I, O'Donnell TJ, et al. On TCR binding predictors failing to generalize to unseen peptides. Front Immunol. (2022) 13:1014256. doi: 10.3389/fimmu.2022.1014256
179. Deng L, Ly C, Abdollahi S, Zhao Y, Prinz I, Bonn S. Performance comparison of TCR-pMHC prediction tools reveals a strong data dependency. Front Immunol. (2023) 14:1128326. doi: 10.3389/fimmu.2023.1128326
180. Garcia-Garijo A, Fajardo CA, Gros A. Determinants for neoantigen identification. Front Immunol. (2019) 10:1392. doi: 10.3389/fimmu.2019.01392
181. Bonsack M, Hoppe S, Winter J, Tichy D, Zeller C, Küpper MD, et al. Performance evaluation of MHC class-I binding prediction tools based on an experimentally validated MHC-peptide binding data set. Cancer Immunol Res. (2019) 7:719–36. doi: 10.1158/2326-6066.CIR-18-0584
182. MHCcombine Web-Application 2.0 (2021). Available online at: https://mhccombine.dkfz.de/mhccombine/index.html.
183. Gálvez J, Gálvez JJ, García-Peñarrubia P. Is TCR/pMHC affinity a good estimate of the T-cell response? An answer based on predictions from 12 phenotypic models. Front Immunol. (2019) 10:349. doi: 10.3389/fimmu.2019.00349
184. Duan F, Duitama J, Al Seesi S, Ayres CM, Corcelli SA, Pawashe AP, et al. Genomic and bioinformatic profiling of mutational neoepitopes reveals new rules to predict anticancer immunogenicity. J Exp Med. (2014) 211:2231–48. doi: 10.1084/jem.20141308
185. Rech AJ, Balli D, Mantero A, Ishwaran H, Nathanson KL, Stanger BZ, et al. Tumor immunity and survival as a function of alternative neopeptides in human cancer. Cancer Immunol Res. (2018) 6:276–87. doi: 10.1158/2326-6066.CIR-17-0559
186. Ghorani E, Rosenthal R, McGranahan N, Reading JL, Lynch M, Peggs KS, et al. Differential binding affinity of mutated peptides for MHC class I is a predictor of survival in advanced lung cancer and melanoma. Ann Oncol. (2018) 29:271–9. doi: 10.1093/annonc/mdx687
187. Rasmussen M, Fenoy E, Harndahl M, Kristensen AB, Nielsen IK, Nielsen M, et al. Pan-specific prediction of peptide-MHC class I complex stability, a correlate of T cell immunogenicity. J Immunol. (2016) 197:1517–24. doi: 10.4049/jimmunol.1600582
188. Borden ES, Ghafoor S, Buetow KH, LaFleur BJ, Wilson MA, Hastings KT. NeoScore integrates characteristics of the neoantigen:MHC class I interaction and expression to accurately prioritize immunogenic neoantigens. J Immunol. (2022) 208:1813–27. doi: 10.4049/jimmunol.2100700
189. Wells DK, van Buuren MM, Dang KK, Hubbard-Lucey VM, Sheehan KCF, Campbell KM, et al. Key parameters of tumor epitope immunogenicity revealed through a consortium approach improve neoantigen prediction. Cell. (2020) 183:818–34.e13. doi: 10.1016/j.cell.2020.09.015
190. Kim P, Park A, Han G, Sun H, Jia P, Zhao Z. TissGDB: tissue-specific gene database in cancer. Nucleic Acids Res. (2018) 46:D1031–8. doi: 10.1093/nar/gkx850
191. Lonsdale J, Thomas J, Salvatore M, Phillips R, Lo E, Shad S, et al. The genotype-tissue expression (GTEx) project. Nat Genet. (2013) 45:580–5. doi: 10.1038/ng.2653
192. Uhlen M, Zhang C, Lee S, Sjöstedt E, Fagerberg L, Bidkhori G, et al. A pathology atlas of the human cancer transcriptome. Science. (2017) 357:eaan2507. doi: 10.1126/science.aan2507
193. The Human Protein Atlas (2023). Available online at: https://www.proteinatlas.org/.
194. Chen H-M, MacDonald JA. Network analysis of TCGA and GTEx gene expression datasets for identification of trait-associated biomarkers in human cancer. STAR Protoc. (2022) 3:101168. doi: 10.1016/j.xpro.2022.101168
195. Richman LP, Vonderheide RH, Rech AJ. Neoantigen dissimilarity to the self-proteome predicts immunogenicity and response to immune checkpoint blockade. Cell Syst. (2019) 9:375–82.e4. doi: 10.1016/j.cels.2019.08.009
196. Bjerregaard A-M, Nielsen M, Jurtz V, Barra CM, Hadrup SR, Szallasi Z, et al. An analysis of natural T cell responses to predicted tumor neoepitopes. Front Immunol. (2017) 8:1566. doi: 10.3389/fimmu.2017.01566
197. Devlin JR, Alonso JA, Ayres CM, Keller GLJ, Bobisse S, Vander Kooi CW, et al. Structural dissimilarity from self drives neoepitope escape from immune tolerance. Nat Chem Biol. (2020) 16:1269–76. doi: 10.1038/s41589-020-0610-1
198. Lebel M-È, Coutelier M, Galipeau M, Kleinman CL, Moon JJ, Melichar HJ. Differential expression of tissue-restricted antigens among mTEC is associated with distinct autoreactive T cell fates. Nat Commun. (2020) 11:3734. doi: 10.1038/s41467-020-17544-3
199. Kyte J, Doolittle RF. A simple method for displaying the hydropathic character of a protein. J Mol Biol. (1982) 157:105–32. doi: 10.1016/0022-2836(82)90515-0
200. Borden ES, Buetow KH, Wilson MA, Hastings KT. Cancer neoantigens: challenges and future directions for prediction, prioritization, and validation. Front Oncol. (2022) 12:836821. doi: 10.3389/fonc.2022.836821
201. Lang F, Schrörs B, Löwer M, Türeci Ö, Sahin U. Identification of neoantigens for individualized therapeutic cancer vaccines. Nat Rev Drug Discovery. (2022) 21:261–82. doi: 10.1038/s41573-021-00387-y
202. Roth A, Khattra J, Yap D, Wan A, Laks E, Biele J, et al. PyClone: statistical inference of clonal population structure in cancer. Nat Methods. (2014) 11:396–8. doi: 10.1038/nmeth.2883
203. Gillis S, Roth A. PyClone-VI: scalable inference of clonal population structures using whole genome data. BMC Bioinf. (2020) 21:571. doi: 10.1186/s12859-020-03919-2
204. Schubert B, Walzer M, Brachvogel H-P, Szolek A, Mohr C, Kohlbacher O. FRED 2: an immunoinformatics framework for Python. Bioinformatics. (2016) 32:2044–6. doi: 10.1093/bioinformatics/btw113
205. Nielsen M, Lundegaard C, Lund O, Keşmir C. The role of the proteasome in generating cytotoxic T-cell epitopes: insights obtained from improved predictions of proteasomal cleavage. Immunogenetics. (2005) 57:33–41. doi: 10.1007/s00251-005-0781-7
206. Hundal J, Kiwala S, McMichael J, Miller CA, Xia H, Wollam AT, et al. pVACtools: A computational toolkit to identify and visualize cancer neoantigens. Cancer Immunol Res. (2020) 8:409–20. doi: 10.1158/2326-6066.CIR-19-0401
207. Bjerregaard A-M, Nielsen M, Hadrup SR, Szallasi Z, Eklund AC. MuPeXI: prediction of neo-epitopes from tumor sequencing data. Cancer Immunol Immunother. (2017) 66:1123–30. doi: 10.1007/s00262-017-2001-3
208. Tappeiner E, Finotello F, Charoentong P, Mayer C, Rieder D, Trajanoski Z. TIminer: NGS data mining pipeline for cancer immunology and immunotherapy. Bioinformatics. (2017) 33:3140–1. doi: 10.1093/bioinformatics/btx377
209. Schmidt J, Smith AR, Magnin M, Racle J, Devlin JR, Bobisse S, et al. Prediction of neo-epitope immunogenicity reveals TCR recognition determinants and provides insight into immunoediting. Cell Rep Med. (2021) 2:100194. doi: 10.1016/j.xcrm.2021.100194
210. Li G, Iyer B, Prasath VBS, Ni Y, Salomonis N. DeepImmuno: deep learning-empowered prediction and generation of immunogenic peptides for T-cell immunity. Briefings Bioinf. (2021) 22:bbab160. doi: 10.1093/bib/bbab160
211. Hundal J, Carreno BM, Petti AA, Linette GP, Griffith OL, Mardis ER, et al. pVAC-Seq: A genome-guided in silico approach to identifying tumor neoantigens. Genome Med. (2016) 8:11. doi: 10.1186/s13073-016-0264-5
212. Zhou Z, Lyu X, Wu J, Yang X, Wu S, Zhou J, et al. TSNAD: an integrated software for cancer somatic mutation and tumour-specific neoantigen detection. R Soc Open Sci. (2017) 4:170050. doi: 10.1098/rsos.170050
213. Bais P, Namburi S, Gatti DM, Zhang X, Chuang JH. CloudNeo: a cloud pipeline for identifying patient-specific tumor neoantigens. Bioinformatics. (2017) 33:3110–2. doi: 10.1093/bioinformatics/btx375
214. Mondet S, Aksoy BA, Rozenberg L, Hodes I, Hammerbacher J. Bioinformatics Workflow Management With The Wobidisco Ecosystem. (2017), 213884. doi: 10.1101/213884
215. Zhou C, Wei Z, Zhang Z, Zhang B, Zhu C, Chen K, et al. pTuneos: prioritizing tumor neoantigens from next-generation sequencing data. Genome Med. (2019) 11:67. doi: 10.1186/s13073-019-0679-x
216. Schenck RO, Lakatos E, Gatenbee C, Graham TA, Anderson ARA. NeoPredPipe: high-throughput neoantigen prediction and recognition potential pipeline. BMC Bioinf. (2019) 20:264. doi: 10.1186/s12859-019-2876-4
217. Wood MA, Nguyen A, Struck AJ, Ellrott K, Nellore A, Thompson RF. neoepiscope improves neoepitope prediction with multivariant phasing. Bioinf (Oxford England). (2020) 36:713–20. doi: 10.1093/bioinformatics/btz653
218. Kodysh J, Rubinsteyn A. OpenVax: an open-source computational pipeline for cancer neoantigen prediction. Methods Mol Biol. (2020) 2120:147–60. doi: 10.1007/978-1-0716-0327-7_10
219. Wang T-Y, Wang L, Alam SK, Hoeppner LH, Yang R. ScanNeo: identifying indel-derived neoantigens using RNA-Seq data. Bioinf (Oxford England). (2019) 35:4159–61. doi: 10.1093/bioinformatics/btz193
220. Fotakis G, Rieder D, Haider M, Trajanoski Z, Finotello F. NeoFuse: predicting fusion neoantigens from RNA sequencing data. Bioinf (Oxford England). (2020) 36:2260–1. doi: 10.1093/bioinformatics/btz879
221. Zhang J, Mardis ER, Maher CA. INTEGRATE-neo: a pipeline for personalized gene fusion neoantigen discovery. Bioinformatics. (2017) 33:555–7. doi: 10.1093/bioinformatics/btw674
222. Kim S, Kim HS, Kim E, Lee MG, Shin E-C, Paik S. Neopepsee: accurate genome-level prediction of neoantigens by harnessing sequence and amino acid immunogenicity information. Ann Oncol. (2018) 29:1030–6. doi: 10.1093/annonc/mdy022
223. Wang G, Wan H, Jian X, Li Y, Ouyang J, Tan X, et al. INeo-epp: A novel T-cell HLA class-I immunogenicity or neoantigenic epitope prediction method based on sequence-related amino acid features. BioMed Res Int. (2020) 2020:5798356. doi: 10.1155/2020/5798356
224. Coelho ACMF, Fonseca AL, Martins DL, Lins PBR, Da Cunha LM, de Souza SJ. neoANT-HILL: an integrated tool for identification of potential neoantigens. BMC Med Genomics. (2020) 13:30. doi: 10.1186/s12920-020-0694-1
225. Shi Y, Guo Z, Su X, Meng L, Zhang M, Sun J, et al. DeepAntigen: a novel method for neoantigen prioritization via 3D genome and deep sparse learning. Bioinformatics. (2020) 36:4894–901. doi: 10.1093/bioinformatics/btaa596
226. Tang Y, Wang Y, Wang J, Li M, Peng L, Wei G, et al. TruNeo: an integrated pipeline improves personalized true tumor neoantigen identification. BMC Bioinf. (2020) 21:532. doi: 10.1186/s12859-020-03869-9
227. Lang F, Riesgo-Ferreiro P, Löwer M, Sahin U, Schrörs B. NeoFox: annotating neoantigen candidates with neoantigen features. Bioinf (Oxford England). (2021) 37:4246–7. doi: 10.1093/bioinformatics/btab344
228. Zhou Z, Wu J, Ren J, Chen W, Zhao W, Gu X, et al. TSNAD v2.0: A one-stop software solution for tumor-specific neoantigen detection. Comput Struct Biotechnol J. (2021) 19:4510–6. doi: 10.1016/j.csbj.2021.08.016
229. Liu C, Zhang Y, Jian X, Tan X, Lu M, Ouyang J, et al. ProGeo-neo v2.0: A one-stop software for neoantigen prediction and filtering based on the proteogenomics strategy. Genes. (2022) 13:783. doi: 10.3390/genes13050783
230. Diao K, Chen J, Wu T, Wang X, Wang G, Sun X, et al. Seq2Neo: A comprehensive pipeline for cancer neoantigen immunogenicity prediction. Int J Mol Sci. (2022) 23:11624. doi: 10.1101/2022.09.14.507872
231. Tan X, Xu L, Jian X, Ouyang J, Hu B, Yang X, et al. PGNneo: A proteogenomics-based neoantigen prediction pipeline in noncoding regions. Cells. (2023) 12:782. doi: 10.3390/cells12050782
232. Vensko SP, Olsen K, Bortone D, Smith CC, Chai S, Beckabir W, et al. LENS: landscape of effective neoantigens software. Bioinformatics. (2023) 39:btad322. doi: 10.1093/bioinformatics/btad322
233. Al Seesi S, Al-Okaily A, Shcheglova TV, Sherafat E, Alqahtani FH, Hagymasi AT, et al. GeNeo: A bioinformatics toolbox for genomics-guided neoepitope prediction. J Comput Biol. (2023) 30:538–51. doi: 10.1089/cmb.2022.0491
234. Fleri W, Vaughan K, Salimi N, Vita R, Peters B, Sette A. The immune epitope database: how data are entered and retrieved. J Immunol Res. (2017) 2017:5974574. doi: 10.1155/2017/5974574
235. Martini S, Nielsen M, Peters B, Sette A. The Immune Epitope Database and Analysis Resource Program 2003-2018: reflections and outlook. Immunogenetics. (2020) 72:57–76. doi: 10.1007/s00251-019-01137-6
236. Koşaloğlu-Yalçın Z, Blazeska N, Vita R, Carter H, Nielsen M, Schoenberger S, et al. The cancer epitope database and analysis resource (CEDAR). Nucleic Acids Res. (2023) 51:D845–52. doi: 10.1093/nar/gkac902
237. Zhou W-J, Qu Z, Song C-Y, Sun Y, Lai A-L, Luo M-Y, et al. NeoPeptide: an immunoinformatic database of T-cell-defined neoantigens. Database. (2019) 2019:baz128. doi: 10.1093/database/baz128
238. Tan X, Li D, Huang P, Jian X, Wan H, Wang G, et al. dbPepNeo: a manually curated database for human tumor neoantigen peptides. Database. (2020) 2020:baaa004. doi: 10.1093/database/baaa004
239. Lu M, Xu L, Jian X, Tan X, Zhao J, Liu Z, et al. dbPepNeo2.0: A database for human tumor neoantigen peptides from mass spectrometry and TCR recognition. Front Immunol. (2022) 13:855976. doi: 10.3389/fimmu.2022.855976
240. Zhang G, Chitkushev L, Olsen LR, Keskin DB, Brusic V. TANTIGEN 2.0: a knowledge base of tumor T cell antigens and epitopes. BMC Bioinf. (2021) 22:40. doi: 10.1186/s12859-021-03962-7
241. Xia J, Bai P, Fan W, Li Q, Li Y, Wang D, et al. NEPdb: A database of T-cell experimentally-validated neoantigens and pan-cancer predicted neoepitopes for cancer immunotherapy. Front Immunol. (2021) 12:644637. doi: 10.3389/fimmu.2021.644637
242. Yi X, Liao Y, Wen B, Li K, Dou Y, Savage SR, et al. caAtlas: An immunopeptidome atlas of human cancer. iScience. (2021) 24:103107. doi: 10.1016/j.isci.2021.103107
243. Luo X, Huang Y, Li H, Luo Y, Zuo Z, Ren J, et al. SPENCER: a comprehensive database for small peptides encoded by noncoding RNAs in cancer patients. Nucleic Acids Res. (2022) 50:D1373–81. doi: 10.1093/nar/gkab822
244. Cai Y, Lv D, Li D, Yin J, Ma Y, Luo Y, et al. IEAtlas: an atlas of HLA-presented immune epitopes derived from non-coding regions. Nucleic Acids Res. (2023) 51:D409–17. doi: 10.1093/nar/gkac776
245. Marcu A, Bichmann L, Kuchenbecker L, Kowalewski DJ, Freudenmann LK, Backert L, et al. HLA Ligand Atlas: a benign reference of HLA-presented peptides to improve T-cell-based cancer immunotherapy. J ImmunoTherapy Cancer. (2021) 9:e002071. doi: 10.1136/jitc-2020-002071
246. Kallor AA, Waleron M, Bedran G, Eugénio P, Pesquita C, Faria D, et al. Abstract 6577: CARMEN: A pan-HLA and pan-cancer proteogenomic database on antigen presentation to support cancer immunotherapy. Cancer Res. (2023) 83:6577. doi: 10.1158/1538-7445.AM2023-6577
247. Wu J, Zhao W, Zhou B, Su Z, Gu X, Zhou Z, et al. TSNAdb: A database for tumor-specific neoantigens from immunogenomics data analysis. Genomics Proteomics Bioinf. (2018) 16:276–82. doi: 10.1016/j.gpb.2018.06.003
248. https://tcia.at/home (2023). Available online at: https://tcia.at/home.
249. Wu J, Chen W, Zhou Y, Chi Y, Hua X, Wu J, et al. TSNAdb v2.0: the updated version of tumor-specific neoantigen database. Genomics Proteomics Bioinf. (2023) 21:259–66. doi: 10.1016/j.gpb.2022.09.012
250. Scholtalbers J, Boegel S, Bukur T, Byl M, Goerges S, Sorn P, et al. TCLP: an online cancer cell line catalogue integrating HLA type, predicted neo-epitopes, virus and gene expression. Genome Med. (2015) 7:118. doi: 10.1186/s13073-015-0240-5
251. Yu J, Wang L, Kong X, Cao Y, Zhang M, Sun Z, et al. CAD v1.0: cancer antigens database platform for cancer antigen algorithm development and information exploration. Front Bioengineering Biotechnol. (2022) 10:819583. doi: 10.3389/fbioe.2022.819583
252. Wu T, Chen J, Diao K, Wang G, Wang J, Yao H, et al. Neodb: a comprehensive neoantigen database and discovery platform for cancer immunotherapy. Database. (2023) 2023:baad041. doi: 10.1093/database/baad041
253. Lefranc M-P, Giudicelli V, Duroux P, Jabado-Michaloud J, Folch G, Aouinti S, et al. IMGT®, the international ImMunoGeneTics information system® 25 years on. Nucleic Acids Res. (2015) 43:D413–22. doi: 10.1093/nar/gku1056
254. Charoentong P, Finotello F, Angelova M, Mayer C, Efremova M, Rieder D, et al. Pan-cancer immunogenomic analyses reveal genotype-immunophenotype relationships and predictors of response to checkpoint blockade. Cell Rep. (2017) 18:248–62. doi: 10.1016/j.celrep.2016.12.019
255. Shao W, Pedrioli PGA, Wolski W, Scurtescu C, Schmid E, Vizcaíno JA, et al. The systeMHC atlas project. Nucleic Acids Res. (2018) 46:D1237–47. doi: 10.1093/nar/gkx664
256. Shugay M, Bagaev DV, Zvyagin IV, Vroomans RM, Crawford JC, Dolton G, et al. VDJdb: a curated database of T-cell receptor sequences with known antigen specificity. Nucleic Acids Res. (2018) 46:D419–27. doi: 10.1093/nar/gkx760
257. Dou Y, Shan S, Zhang J. UcTCRdb: An unconventional T cell receptor sequence database with online analysis functions. Front Immunol. (2023) 14:1158295. doi: 10.3389/fimmu.2023.1158295
258. Team PD. Tumor Neoantigen Selection Alliance (TESLA). Parker Institute for Cancer Immunotherapy (2017). Available at: https://www.parkerici.org/research-project/tumor-neoantigen-selection-alliance-tesla/.
259. Bionetworks S. Synapse. Sage Bionetworks (2023). Available online at: https://www.synapse.org/#!Synapse:syn21048999/wiki/603788.
260. Buckley PR, Lee CH, Ma R, Woodhouse I, Woo J, Tsvetkov VO, et al. Evaluating performance of existing computational models in predicting CD8+ T cell pathogenic epitopes and cancer neoantigens. Briefings Bioinf. (2022) 23:bbac141. doi: 10.1093/bib/bbac141
261. Calis JJA, Maybeno M, Greenbaum JA, Weiskopf D, de Silva AD, Sette A, et al. Properties of MHC class I presented peptides that enhance immunogenicity. PloS Comput Biol. (2013) 9:e1003266. doi: 10.1371/journal.pcbi.1003266
262. Trolle T, Nielsen M. NetTepi: an integrated method for the prediction of T cell epitopes. Immunogenetics. (2014) 66:449–56. doi: 10.1007/s00251-014-0779-0
263. Pogorelyy MV, Fedorova AD, McLaren JE, Ladell K, Bagaev DV, Eliseev AV, et al. Exploring the pre-immune landscape of antigen-specific T cells. Genome Med. (2018) 10:68. doi: 10.1186/s13073-018-0577-7
264. Ogishi M, Yotsuyanagi H. Quantitative prediction of the landscape of T cell epitope immunogenicity in sequence space. Front Immunol. (2019) 10:827. doi: 10.3389/fimmu.2019.00827
265. Gao A, Chen Z, Segal FP, Carrington M, Streeck H, Chakraborty AK, et al. Predicting the Immunogenicity of T cell epitopes: From HIV to SARS-CoV-2. bioRxiv. (2020), 2020.05.14.095885. doi: 10.1101/2020.05.14.095885
266. Sidorczuk K, Gagat P, Pietluch F, Kała J, Rafacz D, Bąkała L, et al. Benchmarks in antimicrobial peptide prediction are biased due to the selection of negative data. Briefings Bioinf. (2022) 23:bbac343. doi: 10.1093/bib/bbac343
267. Mei S, Zhang K. Neglog: homology-based negative data sampling method for genome-scale reconstruction of human protein-protein interaction networks. Int J Mol Sci. (2019) 20:5075. doi: 10.3390/ijms20205075
268. Chen T-R, Lo C-H, Juan S-H, Lo W-C. The influence of dataset homology and a rigorous evaluation strategy on protein secondary structure prediction. PloS One. (2021) 16:e0254555. doi: 10.1371/journal.pone.0254555
269. Beleites C, Neugebauer U, Bocklitz T, Krafft C, Popp J. Sample size planning for classification models. Analytica Chimica Acta. (2013) 760:25–33. doi: 10.1016/j.aca.2012.11.007
270. Dhurandher SK, Pattanaik KK, Verma A, Verma P, Woungang I eds. Advanced network technologies and intelligent computing Vol. 1798. Cham: Springer (2023).
271. Hawkins DM. The problem of overfitting. J Chem Inf Comput Sci. (2004) 44:1–12. doi: 10.1021/ci0342472
273. Saito T, Rehmsmeier M. The precision-recall plot is more informative than the ROC plot when evaluating binary classifiers on imbalanced datasets. PloS One. (2015) 10:e0118432. doi: 10.1371/journal.pone.0118432
274. Hutson M. Has artificial intelligence become alchemy? Science. (2018) 360:478. doi: 10.1126/science.360.6388.478
275. Vig J, Madani A, Varshney LR, Xiong C, Socher R, Rajani NF. BERTology Meets Biology: Interpreting Attention in Protein Language Models. (2020), arXiv:2006.15222. doi: 10.1101/2020.06.26.174417
276. Lundberg SM. (2017). Lee S-I. A unified approach to interpreting model predictions, in: Proceedings of the 31st International Conference on Neural Information Processing Systems, Red Hook, NY, USA: Curran Associates Inc. pp. 4768–77, (NIPS’17).
277. Ribeiro MT, Singh S, Guestrin C. (2016). Why should I trust you?, in: Proceedings of the 22nd ACM SIGKDD International Conference on Knowledge Discovery and Data Mining, New York, NY, USA: ACM. pp. 1135–44.
278. Captum · Model Interpretability for PyTorch (2023). Available online at: https://captum.ai/.
279. Luo W, Phung D, Tran T, Gupta S, Rana S, Karmakar C, et al. Guidelines for developing and reporting machine learning predictive models in biomedical research: A multidisciplinary view. J Med Internet Res. (2016) 18:e323. doi: 10.2196/jmir.5870
280. Carter SM, Rogers W, Win KT, Frazer H, Richards B, Houssami N. The ethical, legal and social implications of using artificial intelligence systems in breast cancer care. Breast. (2020) 49:25–32. doi: 10.1016/j.breast.2019.10.001
281. Nundy S, Montgomery T, Wachter RM. Promoting trust between patients and physicians in the era of artificial intelligence. JAMA - J Am Med Assoc. (2019) 322:497–8. doi: 10.1001/jama.2018.20563
282. Nordling L. How ChatGPT is transforming the postdoc experience. Nature. (2023) 622:655–7. doi: 10.1038/d41586-023-03235-8
283. Esteva A, Kuprel B, Novoa RA, Ko J, Swetter SM, Blau HM, et al. Dermatologist-level classification of skin cancer with deep neural networks. Nature. (2017) 542:115–8. doi: 10.1038/nature21056
284. Xie J, Luo X, Deng X, Tang Y, Tian W, Cheng H, et al. Advances in artificial intelligence to predict cancer immunotherapy efficacy. Front Immunol. (2022) 13:1076883. doi: 10.3389/fimmu.2022.1076883
285. Addala V, Newell F, Pearson JV, Redwood A, Robinson BW, Creaney J, et al. Computational immunogenomic approaches to predict response to cancer immunotherapies. Nat Rev Clin Oncol. (2024) 21:28–46. doi: 10.1038/s41571-023-00830-6
286. Newman AM, Steen CB, Liu CL, Gentles AJ, Chaudhuri AA, Scherer F, et al. Determining cell type abundance and expression from bulk tissues with digital cytometry. Nat Biotechnol. (2019) 37:773–82. doi: 10.1038/s41587-019-0114-2
287. Xie N, Shen G, Gao W, Huang Z, Huang C, Fu L. Neoantigens: promising targets for cancer therapy. Signal Transduction Targeted Ther. (2023) 8:9. doi: 10.1038/s41392-022-01270-x
288. Jhunjhunwala S, Hammer C, Delamarre L. Antigen presentation in cancer: insights into tumour immunogenicity and immune evasion. Nat Rev Cancer. (2021) 21:298–312. doi: 10.1038/s41568-021-00339-z
Keywords: neoantigen prediction, artificial intelligence, immunopeptidomics, cancer immunotherapy, precision medicine
Citation: Bulashevska A, Nacsa Z, Lang F, Braun M, Machyna M, Diken M, Childs L and König R (2024) Artificial intelligence and neoantigens: paving the path for precision cancer immunotherapy. Front. Immunol. 15:1394003. doi: 10.3389/fimmu.2024.1394003
Received: 29 February 2024; Accepted: 13 May 2024;
Published: 29 May 2024.
Edited by:
Zlatko Trajanoski, Medical University of Innsbruck, AustriaReviewed by:
Jindong Xie, Sun Yat-sen University Cancer Center (SYSUCC), ChinaSergio Navarro-Velázquez, Hospital Clinic of Barcelona, Spain
Copyright © 2024 Bulashevska, Nacsa, Lang, Braun, Machyna, Diken, Childs and König. This is an open-access article distributed under the terms of the Creative Commons Attribution License (CC BY). The use, distribution or reproduction in other forums is permitted, provided the original author(s) and the copyright owner(s) are credited and that the original publication in this journal is cited, in accordance with accepted academic practice. No use, distribution or reproduction is permitted which does not comply with these terms.
*Correspondence: Renate König, cmVuYXRlLmtvZW5pZ0BwZWkuZGU=