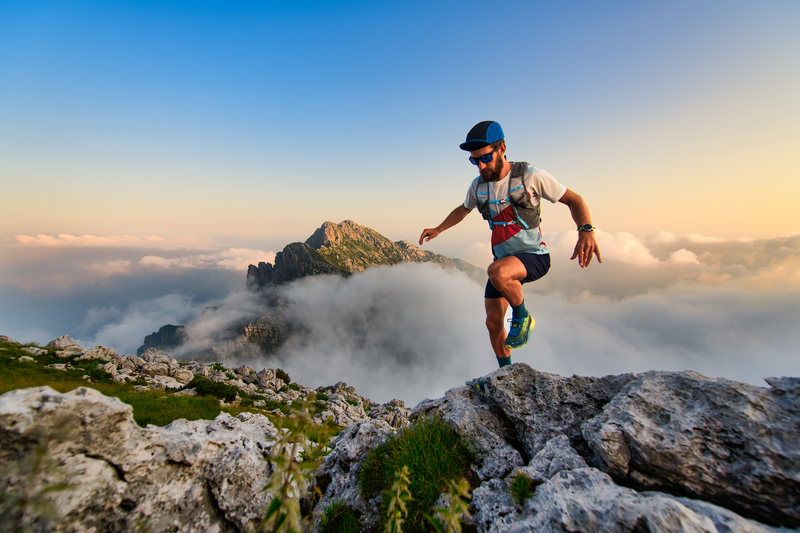
95% of researchers rate our articles as excellent or good
Learn more about the work of our research integrity team to safeguard the quality of each article we publish.
Find out more
ORIGINAL RESEARCH article
Front. Immunol. , 24 May 2024
Sec. Multiple Sclerosis and Neuroimmunology
Volume 15 - 2024 | https://doi.org/10.3389/fimmu.2024.1374350
A commentary has been posted on this article:
Commentary: Evaluation of the causal effects of immune cells on ischemic stroke: a Mendelian randomization study
Background: Ischemic stroke (IS) is a cerebrovascular disease caused by various factors, and its etiology remains inadequately understood. The role of immune system dysfunction in IS has been increasingly recognized. Our objective was to evaluate whether circulating immune cells causally impact IS risk.
Methods: We conducted two-sample Mendelian randomization analyses to evaluate the causal effects of 731 immune cell traits on IS, utilizing publicly available genome-wide association studies (GWAS) summary statistics for 731 immune cell traits as exposure data, and two GWAS statistics for IS as outcome data. A set of sensitivity analyses, including Cochran’s Q test, I2 statistics, MR-Egger intercept test, MR-PRESSO global test, and leave-one-out sensitivity analyses, were performed to assess the robustness of the results. Additionally, meta-analyses were conducted to combine the results from the two different IS datasets. Finally, we extracted instrumental variables of immune cell traits with causal effects on IS in both IS datasets for SNP annotation.
Results: A total of 41 and 35 immune cell traits were identified to have significant causal effects on IS based on two different IS datasets, respectively. Among them, the immune cell trait CD62L- plasmacytoid Dendritic Cell AC and CD4+ CD8dim T cell%leukocyte respectively served as risk factor and protective element in both IS datasets. The robustness of the causal effects was confirmed through the sensitivity analyses. The results of the meta-analyses further support the causal effects of CD62L- plasmacytoid Dendritic Cell AC (pooled OR=1.030, 95%CI: 1.011–1.049, P=0.002) and CD4+ CD8dim T cell%leukocyte (pooled OR=0.959, 95%CI: 0.935–0.984, P=0.001). Based on these two immune cell traits, 33 genes that may be related to the causal effects were mapped.
Conclusions: Our study demonstrated the potential causal effects of circulating immune cells on IS, providing valuable insights for future studies aimed at preventing IS.
Stroke ranks as the second most common cause of death and the third leading cause of death and disability combined worldwide, causing a huge burden to the economy and society (1). Ischemic stroke (IS) is the predominant type of stroke, resulting from a blockage in the blood supply to the brain and clinically manifested by transient or permanent brain dysfunction. In 2020, the global incidence of stroke was 11.71 million people, with IS accounting for approximately 65% of all cases (2). Current treatments for IS rely on rapidly clearing the blockage through thrombolysis or mechanical approaches, and the treatment effectiveness closely tied to the intervention time window (3). The pathogenesis of IS is complex, often resulting from the combined effect of multiple factors. Hypertension, hyperlipidemia, atrial fibrillation, cigarette smoking, excessive alcohol consumption, and diabetes mellitus are well-established risk factors for IS (4–6). However, these traditional risk factors can only partially explain the risk of IS. Therefore, accurately identifying novel IS-related risk factors has become crucial for its prevention and treatment.
The role of inflammation and immune system dysfunction in IS has been increasingly recognized (7). Immune cells, crucial components of the immune system, circulate in the bloodstream or reside within tissues. A transcriptomic study revealed the involvement of immune cells in IS, demonstrating significant differences in peripheral blood immune cells of IS patients compared to the normal control group (8). Studies have indicated that both acute and chronic inflammation in IS is primarily linked to immune cells such as B cells, T cells, monocytes, neutrophils (7, 9). A complex interdependent relationship exists among different types of immune cells in IS. They not only collaborate to clear necrotic brain tissue but may also trigger an inflammatory response, leading to damage of healthy neurons (7, 9). However, a significant portion of the existing evidence is derived from observational studies, which might be constrained by confounding factors and reverse causality. This means that while specific changes in immune cells may be observed in associated with IS, it cannot be determined whether the changes in immune cells are a direct cause of IS or a result of it.
Mendelian randomization (MR) is a widely used analytical method aimed at investigating potential causal impacts of exposures on outcomes using data obtained from genome-wide association studies (GWAS) (10). MR effectively reduces the influence of confounding factors and avoids the issue of reverse causation commonly encountered in observational studies, as allelic variants are randomly allocated and fixed at conception (11).
To the best of our knowledge, the causal association between a broad range of immune cell traits and IS has not been established using MR. To address this gap, based on the available GWAS data on peripheral blood immune cells and IS, two-sample MR analyses were performed to explore the causal links of 731 types of immune cell trait on IS risk.
We conducted two-sample MR analyses to evaluate the causal effects of 731 immune cell traits on the risk of IS. Each immune cell trait served as an exposure variable, and IS served as the outcome variable. Eligible single nucleotide polymorphisms (SNPs) that represented immune cell traits were employed as instrumental variables (IVs). In the MR analysis, adherence to three fundamental assumptions is essential: (1) relevance assumption: IVs are strongly associated with immune cells; (2) independence assumption: IVs are independent of potential confounders; and (3) exclusion restriction assumption: IVs affect IS only via immune cells (12). Sensitivity analyses were performed to ensure the robustness of the results. The design of MR analysis is illustrated in Figure 1. All studies included in our analysis received approval from the relevant institutional review boards.
We obtained the GWAS data for immune cell traits and IS from the IEU Open GWAS project website (https://gwas.mrcieu.ac.uk/datasets). For the exposure data, we used the GWAS summary statistics for a total of 731 immune cell traits derived from a cohort of 3,757 normal Europeans (13). These immune cell traits were classified into four groups: absolute cell counts (AC) (n=118), relative cell counts (RC) (n=192), median fluorescence intensities (MFI) (n=389), and morphological parameters (MP) (n=32). Specifically, AC, RC, and MFI contain TBNK (T cell, B cell, natural killer cell), Treg, maturation stages of T cell, dendritic cell (DC), B cell, monocyte, and myeloid cell panels, while MP contains DC and TBNK panels. To ensure comparability in ancestry, outcome data for IS were extracted from two GWAS statistics of Europeans with the largest sample sizes, consisting of 11,929 cases/472,192 controls (GWAS ID: ebi-a-GCST90018864; designated as the discovery dataset) (14) and 34,217 cases/406,111 controls (GWAS ID: ebi-a-GCST005843; designated as the validation dataset) (15), respectively. Detailed information of datasets is presented in Table 1.
To ensure the authenticity and reliability of IVs, a set of quality control measures was implemented. Firstly, in accordance with the recent studies (16, 17), the significance level of IVs for each immune cell trait was set at 1×10−5. Secondly, to obtain independent IVs, the linkage disequilibrium r2 threshold was set to 0.001 within a 10,000kb distance based on the reference panel of 1000 Genomes Project (18). Thirdly, SNPs significantly correlated with confounders such as arterial hypertension and diabetes mellitus as previously reported (19) were excluded using PhenoScanner (http://www.phenoscanner.medschl.cam.ac.uk/) to preliminarily mitigate the effect of horizontal pleiotropy (Supplementary Table 1). Fourthly, to avoid bias from weak instruments, only IVs with F-statistics greater than 10 were considered as strong instruments. Finally, the GWAS data for each immune cell trait dataset and each IS dataset were harmonized with the selected IVs.
To evaluate the potential causal effects of 731 immune cell traits on IS, inverse variance weighting (IVW) as the primary method and MR-Egger as the supplementary method were conducted. The obtained results were visualized using scatter plots. Subsequently, a range of sensitivity analyses were performed to assess the robustness of the results. Cochran’s Q test and I2 statistics were used to detect the heterogeneity among IVs. MR-Egger intercept test was utilized to evaluate the presence of horizontal pleiotropy (20). The MR-PRESSO global test, known for higher statistical power, was also employed to further examine possible horizontal pleiotropy (21). Additionally, leave-one-out sensitivity analyses were performed to determine whether an individual SNP could influence the bias of causal estimate. Finally, to facilitate the integration of results from the two different IS datasets, meta-analyses were conducted to consolidate the findings. The analyses were carried out using the packages TwoSampleMR (version 0.5.6), MR-PRESSO (version 1.0), and meta (version 6.5) in R (version 4.1.0). Detailed procedure code is provided in Supplementary Materials.
An rs-codes of SNP converter g:SNPense was utilized for SNP annotation (22). g:SNPense is an online tool for mapping human SNP identifiers to their corresponding genes and providing their predicted variant effects, with the Ensembl Variation data. Mapping is only available for SNPs which overlap with at least one Ensembl gene.
Following stringent quality control measures, we identified 2 to 729 independent IVs for different immune cell traits. The F-statistics for these IVs ranged from 19.548 to 2435.818, indicating a lack of potential bias from weak instruments. Comprehensive details about the IVs, including rs-codes, effect allele, other allele, beta value, standard error, P-value, and other information, are systematically summarized in Supplementary Table 2.
Regarding the discovery dataset, the results of the IVW analyses revealed 41 immune cell traits exhibiting significant causal associations with IS risk, including 11 in the B cell panel, 10 in the TBNK panel, 7 in the Treg panel, 5 in the maturation stages of T cell panel, 3 in the monocyte panel, 3 in the myeloid cell panel, and 2 in the DC panel (Figure 2). A total of 20 immune cell traits, such as CD25 on CD28+ CD4+ T cell (OR=1.071, 95%CI: 1.005–1.140, P=0.033), BAFF-R on IgD- CD38dim B cell (OR=1.057, 95%CI: 1.005–1.112, P=0.031), and IgD+ CD24+ B cell AC (OR=1.046, 95%CI: 1.010–1.082, P=0.012), were found to significantly increase the risk of IS. Conversely, 21 immune cell traits, such as CD28 on resting CD4 regulatory T cell (OR=0.926, 95%CI: 0.887–0.965, P<0.001), CD62L- HLA DR++ monocyte AC (OR=0.952, 95%CI: 0.917–0.989, P=0.011), and CD19 on IgD- CD27- B cell (OR=0.953, 95%CI: 0.921–0.986, P=0.006), significantly decreased the risk of IS (Figure 2).
Figure 2 Forest plot for the causal effects of immune cell traits on IS risk derived from inverse variance weighted based on discovery dataset. OR, odds ratio; CI, confidence interval.
Regarding the validation dataset, the results of the IVW analyses demonstrated 35 immune cell traits exhibiting significant causal associations with IS risk, of which 10 were in the B cell panel, 5 in the TBNK panel, 7 in the Treg panel, 6 in the maturation stages of T cell panel, 3 in the myeloid cell panel, and 4 in the DC panel (Figure 3). Among these immune cell traits, 22 of them, such as CD27 on IgD- CD38+ B cell (OR=1.071, 95%CI: 1.029–1.115, P<0.001), IgD+ CD38dim B cell%B cell (OR=1.059, 95%CI: 1.004–1.116, P=0.035), and CD20 on IgD- CD27- B cell (OR=1.044, 95%CI: 1.005–1.084, P=0.025), were positively associated with the risk of IS. On the contrary, 13 immune cell traits, such as Plasma Blast-Plasma Cell AC (OR=0.960, 95%CI: 0.935–0.986, P=0.003), CD4+ CD8dim T cell%leukocyte (OR=0.961, 95%CI: 0.928–0.995, P=0.024), and CD11b on Granulocytic Myeloid-Derived Suppressor Cells (OR=0.963, 95%CI: 0.941–0.987, P=0.002), were negatively associated with the risk of IS (Figure 3). Details of MR analyses, including the results estimated by MR-Egger, are summarized in Supplementary Table 3.
Figure 3 Forest plot for the causal effects of immune cell traits on IS risk derived from inverse variance weighted based on validation dataset. OR, odds ratio; CI, confidence interval.
Based on the results of MR analyses of the discovery and validation datasets, we found that CD62L- plasmacytoid Dendritic Cell AC and CD4+ CD8dim T cell%leukocyte were linked with the susceptibility to IS in both datasets, serving as a risk factor and a protective element, respectively (Table 2). Scatter plots illustrating the causal effects of CD62L- plasmacytoid Dendritic Cell AC and CD4+ CD8dim T cell%leukocyte in different IS datasets are presented in Supplementary Figure 1.
The results of sensitivity analyses confirmed the robustness of the causal associations (Table 3). When I² statistics>50% or the P-value for Cochran’s Q test<0.05, heterogeneity among IVs needs to be considered. No evidence of heterogeneity was detected in our results. Visualized funnel plots are presented in Supplementary Figure 2. Neither the Egger intercept test nor the MR-PRESSO global test identified significant horizontal pleiotropy, except for the Egger intercept test for CD62L- plasmacytoid Dendritic Cell AC in the discovery dataset (P=0.0022). It is worth noting that, compared to the Egger intercept test, the MR-PRESSO global test demonstrates higher statistical power (21). Therefore, it is justifiable to prioritize the results of the MR-PRESSO global test. However, considering the potential presence of horizontal pleiotropy, we conducted MR-Egger causal estimation to complement the MR analysis, which can identify and adjust for horizontal pleiotropy (20). The MR-Egger estimation also revealed a consistent causal effect for CD62L- plasmacytoid Dendritic Cell AC on IS (OR=1.085, 95%CI: 1.045–1.126, P<0.001) (Supplementary Table 3), aligning with the IVW result in the discovery dataset, demonstrating the reliability of the finding. The results of the leave-one-out sensitivity analyses demonstrated that no single SNP could significantly influence the causal estimates (Supplementary Figure 3).
Subsequently, we conducted meta-analyses to combine the MR estimates from the two different datasets. For CD62L- plasmacytoid Dendritic Cell AC, the meta-analysis results indicated that an increase in CD62L- plasmacytoid Dendritic Cell AC led to a higher risk of IS (pooled OR=1.030, 95%CI: 1.011–1.049, P=0.002) without any heterogeneity observed (I2 = 0.0%, τ2 = 0.0%, P=0.82) (Figure 4A). Regarding CD4+ CD8dim T cell%leukocyte, the meta-analysis results showed that an increase in this trait decreased the risk of IS (pooled OR=0.959, 95%CI: 0.935–0.984, P=0.001) without any heterogeneity observed (I2 = 0.0%, τ2 = 0.0%, P=0.90) (Figure 4B).
Figure 4 Forest plots for meta-analyses of MR estimates in two IS datasets. (A) CD62L- plasmacytoid Dendritic Cell AC. (B) CD4+ CD8dim T cell%leukocyte.
We annotated the SNPs as IVs of the immune cell traits of CD62L- plasmacytoid Dendritic Cell AC and CD4+ CD8dim T cell%leukocyte. A total of 33 Ensembl genes were mapped using g:SNPense (Table 4). These identified genes may be relevant to the causal effect of CD62L- plasmacytoid Dendritic Cell AC and CD4+ CD8dim T cell%leukocyte on IS risk.
Understanding the impact of immune cells on IS will provide valuable insights into the role of inflammation and immune system dysfunction in the onset and progression of IS. Recent research has brought attention to the noteworthy influence of immunity on IS risk, as evidenced by elevated levels of inflammatory markers in the bloodstream, such as interleukin-6 (23), monocyte chemotactic protein-1 (24), and C-reactive protein (25), as well as a rise in total white blood cell count (26–28) and neutrophil count (27, 28). However, due to the inherent limitations of observational studies (29), these investigations can only establish the involvement of inflammation and immune cells in the development of IS but cannot offer reliable proof of causality. Given the methodological advantages of MR analysis in causal inference (11), this study’s evaluation of the causal effects of immune cells on the risk of IS may be more dependable than previous observational studies. In our study, utilizing large-scale publicly available genetic data, we conducted two-sample MR analyses to explore genetic evidence supporting causal associations between immune cell traits and IS. The results of this study demonstrated that 41 and 35 immune cell traits had significant causal effects on IS based on the discovery and validation dataset, respectively. Furthermore, the immune cell traits CD62L- plasmacytoid Dendritic Cell AC and CD4+ CD8dim T cell%leukocyte were significant in both the discovery and validation datasets. In addition, the meta-analyses, combining the MR estimates, further confirm that CD62L- plasmacytoid Dendritic Cell AC and CD4+ CD8dim T cell%leukocyte have causal effects on IS.
DCs play a crucial role in initiating and coordinating the immune response as professional antigen-presenting cells, which can be classified into inflammatory DCs, Langerhans cells, conventional DCs, and plasmacytoid DCs (30). The role of DCs has not been sufficiently investigated in the context of IS. Regarding clinical study, compared with healthy individuals, the number of circulating plasmacytoid DC precursors was transiently reduced in IS patients, while a dense infiltration of plasmacytoid DCs was observed in the infarcted brain, indicating the potential recruitment of plasmacytoid DC precursors from blood into the infarcted brain (31). Using a murine model of experimental IS, Barbara et al. observed an induction of DC migration and maturation under ischemic conditions, and inhibiting DC function can reduce the infarct area and improve neurological function scores (32). The adhesion molecule CD62L- DCs are considered immature with relatively low migratory capability (13). According to our research, elevated levels of CD62L- plasmacytoid Dendritic Cell AC can increase the risk of IS. Self-DNA released from dying cells can activate neutrophils to release the DNA-binding antimicrobial peptide LL37 (known as Cramp in mice), which in can subsequently convert self-DNA into a trigger for plasmacytoid DC activation through Toll-like receptor 9, leading to the production of large amounts of interferon-α (33, 34). The mechanism of plasmacytoid DC activation by self-DNA is closely related to the occurrence and progression of atherosclerosis and diabetes (35, 36). Both atherosclerosis and diabetes are intricately connected to the onset and development of IS (4, 5), which may partly explain why CD62L- plasmacytoid Dendritic Cell AC could serve as a risk factor for IS.
In the realm of immune components, T cells are particularly significant due to their potency in both innate and adaptive immune responses. They are crucially involved in post-stroke inflammation, primarily through the release of inflammatory cytokines and their intricate interplay with other cells, thereby amplifying the cascade of inflammation (37, 38). It has been reported that IS induced a dramatic and immediate loss of circulating T cells within 12 hours after onset (39). However, in the infarcted brain samples of IS patients, T cell numbers have been shown to increase for at least 3 months (40). A recent study demonstrated that the decrease in the percentage of circulating CD4+ naïve T cells is a risk factor for IS in patients on hemodialysis (41). In our study, CD4+ CD8dim T cell%leukocyte in TBNK panel was shown to be significantly associated with decreased risk of IS. Interleukin-10 plays a significant role in regulating pro-inflammatory cytokines and exerting immunomodulatory and neuroprotective effects in the context of IS (42). In a murine model of experimental IS, Dan et al. demonstrated that the adoptive transfer of interleukin-10-producing CD4+ T cells resulted in a reduction in ischemic infarct size (43). Further exploration is needed to elucidate the mechanisms underlying the involvement of CD4+ CD8dim T cell%leukocyte in the occurrence and progression of IS.
We identified 33 genes that may be associated with the causal effect of CD62L- plasmacytoid Dendritic Cell AC and CD4+ CD8dim T cell%leukocyte on IS risk by SNP annotation. Among these genes, NEDD4L (44) and ABCC1 (45) have been reported to be involved in IS through non-immune mechanisms. NEDD4L deletion can exacerbate ischemic brain damage by diminishing α-Synuclein polyubiquitination (44). ABCC1 is downregulated in response to IS, which could be reversed by the deletion of apolipoprotein E (45).
There are several limitations of the present MR study. First, due to the lack of detailed individual information, we could not delve deeper into the causal effects of immune cell traits on subgroups of the population. Second, since the dataset solely represents a European population, caution must be exercised when extrapolating the findings to other ethnic groups, necessitating additional scrutiny. Third, all causal effects uncovered through our MR study were derived from IVs at a relatively loose threshold, which may potentially affect the precision of the results. However, considering all F-statistics were greater than 10, it appears unlikely that weak IVs could have influenced our findings.
In summary, our results could offer novel perspectives on the causal connections between immune cell traits and IS, and highlight the intricate interactions between the immune system and IS. The findings indicate that CD62L- plasmacytoid Dendritic Cell AC and CD4+ CD8dim T cell%leukocyte hold potential as biomarkers for IS risk, which could facilitate earlier diagnosis and more effective treatment options. Furthermore, we call for experimental research to explore the underlying mechanisms linking identified immune cell traits to the risk of IS.
The original contributions presented in the study are included in the article/Supplementary Material. Further inquiries can be directed to the corresponding author.
KW: Formal analysis, Visualization, Writing – original draft. BZ: Conceptualization, Supervision, Writing – review & editing. ML: Formal analysis, Visualization, Writing – review & editing. HD: Formal analysis, Visualization, Writing – review & editing. ZJ: Formal analysis, Visualization, Writing – review & editing. SG: Formal analysis, Visualization, Writing – review & editing. JC: Formal analysis, Visualization, Writing – review & editing. SF: Conceptualization, Funding acquisition, Supervision, Writing – review & editing.
The author(s) declare financial support was received for the research, authorship, and/or publication of this article. This study was supported by the National Natural Science Foundation of China (No. 82371304) and the Science and Technology Department of Jilin Province (YDZJ202201ZYTS684).
The authors declare that the research was conducted in the absence of any commercial or financial relationships that could be construed as a potential conflict of interest.
All claims expressed in this article are solely those of the authors and do not necessarily represent those of their affiliated organizations, or those of the publisher, the editors and the reviewers. Any product that may be evaluated in this article, or claim that may be made by its manufacturer, is not guaranteed or endorsed by the publisher.
The Supplementary Material for this article can be found online at: https://www.frontiersin.org/articles/10.3389/fimmu.2024.1374350/full#supplementary-material
1. GBD 2019 Stroke Collaborators. Global, regional, and national burden of stroke and its risk factors, 1990-2019: a systematic analysis for the Global Burden of Disease Study 2019. Lancet Neurol. (2021) 20:795–820. doi: 10.1016/S1474-4422(21)00252-0
2. Tsao CW, Aday AW, Almarzooq ZI, Alonso A, Beaton AZ, Bittencourt MS, et al. Heart disease and stroke statistics-2022 update: a report from the American Heart Association. Circulation. (2022) 145:e153–639. doi: 10.1161/CIR.0000000000001052
3. Barthels D, Das H. Current advances in ischemic stroke research and therapies. Biochim Biophys Acta Mol Basis Dis. (2020) 1866:165260. doi: 10.1016/j.bbadis.2018.09.012
6. Larsson SC, Burgess S, Michaëlsson K. Smoking and stroke: a mendelian randomization study. Ann Neurol. (2019) 86:468–71. doi: 10.1002/ana.25534
7. Endres M, Moro MA, Nolte CH, Dames C, Buckwalter MS, Meisel A. Immune pathways in etiology, acute phase, and chronic sequelae of ischemic stroke. Circ Res. (2022) 130:1167–86. doi: 10.1161/CIRCRESAHA.121.319994
8. Liu R, Song P, Gu X, Liang W, Sun W, Hua Q, et al. Comprehensive landscape of immune infiltration and aberrant pathway activation in ischemic stroke. Front Immunol. (2021) 12:766724. doi: 10.3389/fimmu.2021.766724
9. Qiu Y-M, Zhang C-L, Chen A-Q, Wang H-L, Zhou Y-F, Li Y-N, et al. Immune cells in the BBB disruption after acute ischemic stroke: targets for immune therapy? Front Immunol. (2021) 12:678744. doi: 10.3389/fimmu.2021.678744
10. Smith GD, Hemani G. Mendelian randomization: genetic anchors for causal inference in epidemiological studies. Hum Mol Genet. (2014) 23:R89–98. doi: 10.1093/hmg/ddu328
11. Sekula P, Del Greco MF, Pattaro C, Köttgen A. Mendelian randomization as an approach to assess causality using observational data. J Am Soc Nephrol. (2016) 27:3253–65. doi: 10.1681/ASN.2016010098
12. Bowden J, Holmes MV. Meta-analysis and Mendelian randomization: a review. Res Synth Methods. (2019) 10:486–96. doi: 10.1002/jrsm.1346
13. Orrù V, Steri M, Sidore C, Marongiu M, Serra V, Olla S, et al. Complex genetic signatures in immune cells underlie autoimmunity and inform therapy. Nat Genet. (2020) 52:1036–45. doi: 10.1038/s41588-020-0684-4
14. Sakaue S, Kanai M, Tanigawa Y, Karjalainen J, Kurki M, Koshiba S, et al. A cross-population atlas of genetic associations for 220 human phenotypes. Nat Genet. (2021) 53:1415–24. doi: 10.1038/s41588-021-00931-x
15. Malik R, Chauhan G, Traylor M, Sargurupremraj M, Okada Y, Mishra A, et al. Multiancestry genome-wide association study of 520,000 subjects identifies 32 loci associated with stroke and stroke subtypes. Nat Genet. (2018) 50:524–37. doi: 10.1038/s41588-018-0058-3
16. Wang C, Zhu D, Zhang D, Zuo X, Yao L, Liu T, et al. Causal role of immune cells in schizophrenia: Mendelian randomization (MR) study. BMC Psychiatry. (2023) 23:590. doi: 10.1186/s12888-023-05081-4
17. Gu J, Yan G-M, Kong X-L, Zhang Y-Y, Huang L-H, Lu H-M. Assessing the causal relationship between immune traits and systemic lupus erythematosus by bi-directional Mendelian randomization analysis. Mol Genet Genomics. (2023) 298:1493–503. doi: 10.1007/s00438-023-02071-9
18. 1000 Genomes Project Consortium, Auton A, Brooks LD, Durbin RM, Garrison EP, Kang HM, et al. A global reference for human genetic variation. Nature. (2015) 526:68–74. doi: 10.1038/nature15393
19. Grau AJ, Weimar C, Buggle F, Heinrich A, Goertler M, Neumaier S, et al. Risk factors, outcome, and treatment in subtypes of ischemic stroke: the German stroke data bank. Stroke. (2001) 32:2559–66. doi: 10.1161/hs1101.098524
20. Burgess S, Thompson SG. Interpreting findings from Mendelian randomization using the MR-Egger method. Eur J Epidemiol. (2017) 32:377–89. doi: 10.1007/s10654-017-0255-x
21. Verbanck M, Chen C-Y, Neale B, Do R. Detection of widespread horizontal pleiotropy in causal relationships inferred from Mendelian randomization between complex traits and diseases. Nat Genet. (2018) 50:693–8. doi: 10.1038/s41588-018-0099-7
22. Kolberg L, Raudvere U, Kuzmin I, Adler P, Vilo J, Peterson H. g:Profiler-interoperable web service for functional enrichment analysis and gene identifier mapping (2023 update). Nucleic Acids Res. (2023) 51:W207–W12. doi: 10.1093/nar/gkad347
23. Jenny NS, Callas PW, Judd SE, McClure LA, Kissela B, Zakai NA, et al. Inflammatory cytokines and ischemic stroke risk: the REGARDS cohort. Neurology. (2019) 92:e2375–e84. doi: 10.1212/WNL.0000000000007416
24. Georgakis MK, Malik R, Björkbacka H, Pana TA, Demissie S, Ayers C, et al. Circulating monocyte chemoattractant protein-1 and risk of stroke: meta-analysis of population-based studies involving 17 180 individuals. Circ Res. (2019) 125:773–82. doi: 10.1161/CIRCRESAHA.119.315380
25. Emerging Risk Factors Collaboration, Kaptoge S, Di Angelantonio E, Lowe G, Pepys MB, Thompson SG, et al. C-reactive protein concentration and risk of coronary heart disease, stroke, and mortality: an individual participant meta-analysis. Lancet. (2010) 375:132–40. doi: 10.1016/S0140-6736(09)61717-7
26. Kwon S, Kim TJ, Choi E-K, Ahn H-J, Lee E, Lee S-R, et al. Predictors of ischemic stroke for low-risk patients with atrial fibrillation: a matched case-control study. Heart Rhythm. (2021) 18:702–8. doi: 10.1016/j.hrthm.2021.01.016
27. Huh JY, Ross GW, Chen R, Abbott RD, Bell C, Willcox B, et al. Total and differential white blood cell counts in late life predict 8-year incident stroke: the Honolulu Heart Program. J Am Geriatr Soc. (2015) 63:439–46. doi: 10.1111/jgs.13298
28. Wu T-H, Chien K-L, Lin H-J, Hsu H-C, Su T-C, Chen M-F, et al. Total white blood cell count or neutrophil count predict ischemic stroke events among adult Taiwanese: report from a community-based cohort study. BMC Neurol. (2013) 13:7. doi: 10.1186/1471-2377-13-7
29. Ebrahim S, Smith GD. Mendelian randomization: can genetic epidemiology help redress the failures of observational epidemiology? Hum Genet. (2008) 123:15–33. doi: 10.1007/s00439-007-0448-6
30. Balan S, Saxena M, Bhardwaj N. Dendritic cell subsets and locations. Int Rev Cell Mol Biol. (2019) 348:1–68. doi: 10.1016/bs.ircmb.2019.07.004
31. Yilmaz A, Fuchs T, Dietel B, Altendorf R, Cicha I, Stumpf C, et al. Transient decrease in circulating dendritic cell precursors after acute stroke: potential recruitment into the brain. Clin Sci. (2009) 118:147–57. doi: 10.1042/CS20090154
32. Dietel B, Cicha I, Kallmünzer B, Tauchi M, Yilmaz A, Daniel WG, et al. Suppression of dendritic cell functions contributes to the anti-inflammatory action of granulocyte-colony stimulating factor in experimental stroke. Exp Neurol. (2012) 237:379–87. doi: 10.1016/j.expneurol.2012.06.019
33. Lande R, Gregorio J, Facchinetti V, Chatterjee B, Wang Y-H, Homey B, et al. Plasmacytoid dendritic cells sense self-DNA coupled with antimicrobial peptide. Nature. (2007) 449:564–9. doi: 10.1038/nature06116
34. Means TK, Latz E, Hayashi F, Murali MR, Golenbock DT, Luster AD. Human lupus autoantibody-DNA complexes activate DCs through cooperation of CD32 and TLR9. J Clin Invest. (2005) 115:407–17. doi: 10.1172/JCI23025
35. Döring Y, Manthey HD, Drechsler M, Lievens D, Megens RTA, Soehnlein O, et al. Auto-antigenic protein-DNA complexes stimulate plasmacytoid dendritic cells to promote atherosclerosis. Circulation. (2012) 125:1673–83. doi: 10.1161/CIRCULATIONAHA.111.046755
36. Diana J, Simoni Y, Furio L, Beaudoin L, Agerberth B, Barrat F, et al. Crosstalk between neutrophils, B-1a cells and plasmacytoid dendritic cells initiates autoimmune diabetes. Nat Med. (2013) 19:65–73. doi: 10.1038/nm.3042
37. Gill D, Veltkamp R. Dynamics of T cell responses after stroke. Curr Opin Pharmacol. (2016) 26:26–32. doi: 10.1016/j.coph.2015.09.009
38. Zhang D, Ren J, Luo Y, He Q, Zhao R, Chang J, et al. T cell response in ischemic stroke: from mechanisms to translational insights. Front Immunol. (2021) 12:707972. doi: 10.3389/fimmu.2021.707972
39. Vogelgesang A, Grunwald U, Langner S, Jack R, Bröker BM, Kessler C, et al. Analysis of lymphocyte subsets in patients with stroke and their influence on infection after stroke. Stroke. (2008) 39:237–41. doi: 10.1161/STROKEAHA.107.493635
40. Heindl S, Ricci A, Carofiglio O, Zhou Q, Arzberger T, Lenart N, et al. Chronic T cell proliferation in brains after stroke could interfere with the efficacy of immunotherapies. J Exp Med. (2021) 218:e20202411. doi: 10.1084/jem.20202411
41. Chen R, Hu J, Xiang F, Tan X, Shen B, Liu Z, et al. Decreased percentage of peripheral naïve T cells is independently associated with ischemic stroke in patients on hemodialysis. Int Urol Nephrol. (2017) 49:2051–60. doi: 10.1007/s11255-017-1691-y
42. Liesz A, Suri-Payer E, Veltkamp C, Doerr H, Sommer C, Rivest S, et al. Regulatory T cells are key cerebroprotective immunomodulators in acute experimental stroke. Nat Med. (2009) 15:192–9. doi: 10.1038/nm.1927
43. Frenkel D, Huang Z, Maron R, Koldzic DN, Hancock WW, Moskowitz MA, et al. Nasal vaccination with myelin oligodendrocyte glycoprotein reduces stroke size by inducing IL-10-producing CD4+ T cells. J Immunol. (2003) 171:6549–55. doi: 10.4049/jimmunol.171.12.6549
44. Kim T, Chokkalla AK, Vemuganti R. Deletion of ubiquitin ligase Nedd4l exacerbates ischemic brain damage. J Cereb Blood Flow Metab. (2021) 41:1058–66. doi: 10.1177/0271678X20943804
Keywords: immune cells, ischemic stroke, Mendelian randomization, causal effect, single nucleotide polymorphism
Citation: Wang K, Zhang B, Li M, Duan H, Jiang Z, Gao S, Chen J and Fang S (2024) Evaluation of the causal effects of immune cells on ischemic stroke: a Mendelian randomization study. Front. Immunol. 15:1374350. doi: 10.3389/fimmu.2024.1374350
Received: 22 January 2024; Accepted: 10 May 2024;
Published: 24 May 2024.
Edited by:
Xiao-Yi Xiong, Chengdu University of Traditional Chinese Medicine, ChinaReviewed by:
Rui Xu, Xinqiao Hospital, ChinaCopyright © 2024 Wang, Zhang, Li, Duan, Jiang, Gao, Chen and Fang. This is an open-access article distributed under the terms of the Creative Commons Attribution License (CC BY). The use, distribution or reproduction in other forums is permitted, provided the original author(s) and the copyright owner(s) are credited and that the original publication in this journal is cited, in accordance with accepted academic practice. No use, distribution or reproduction is permitted which does not comply with these terms.
*Correspondence: Shaokuan Fang, ZmFuZ3NrQGpsdS5lZHUuY24=
Disclaimer: All claims expressed in this article are solely those of the authors and do not necessarily represent those of their affiliated organizations, or those of the publisher, the editors and the reviewers. Any product that may be evaluated in this article or claim that may be made by its manufacturer is not guaranteed or endorsed by the publisher.
Research integrity at Frontiers
Learn more about the work of our research integrity team to safeguard the quality of each article we publish.