- 1Center of Clinical Neuroscience, Department of Neurology, Faculty of Medicine and University Hospital Carl Gustav Carus, Technische Universität Dresden, Dresden, Germany
- 2Research Group Digital Health, Faculty of Business and Economics, Technische Universität Dresden, Dresden, Germany
Recent advances in the field of artificial intelligence (AI) could yield new insights into the potential causes of multiple sclerosis (MS) and factors influencing its course as the use of AI opens new possibilities regarding the interpretation and use of big data from not only a cross-sectional, but also a longitudinal perspective. For each patient with MS, there is a vast amount of multimodal data being accumulated over time. But for the application of AI and related technologies, these data need to be available in a machine-readable format and need to be collected in a standardized and structured manner. Through the use of mobile electronic devices and the internet it has also become possible to provide healthcare services from remote and collect information on a patient’s state of health outside of regular check-ups on site. Against this background, we argue that the concept of pathways in healthcare now could be applied to structure the collection of information across multiple devices and stakeholders in the virtual sphere, enabling us to exploit the full potential of AI technology by e.g., building digital twins. By going digital and using pathways, we can virtually link patients and their caregivers. Stakeholders then could rely on digital pathways for evidence-based guidance in the sequence of procedures and selection of therapy options based on advanced analytics supported by AI as well as for communication and education purposes. As far as we aware of, however, pathway modelling with respect to MS management and treatment has not been thoroughly investigated yet and still needs to be discussed. In this paper, we thus present our ideas for a modular-integrative framework for the development of digital patient pathways for MS treatment.
1 Introduction
Many roads lead to Rome, or so the saying goes. The same is true for multiple sclerosis (MS). There is no such thing as one unique disease course in MS. MS is one of the most common disabling neurological diseases in young adults (1, 2). Focal and diffuse neuroinflammation combined with neurodegeneration in the central nervous system translates into a chronic disease which evolves very differently over time, with people experiencing an array of diverse symptoms or functional impairment in form of relapses and gradual disability progression (3). A cure for MS has not been found yet, but, in particular in the last decade, a growing number of MS-specific disease modifying therapies have become available which can be applied to modify the pathophysiological processes and to thus slow and mitigate its progression (4).
For all of these reasons, there is no straight-forward way to treat MS. In effect, MS course is characterized by a high intra- and inter-individual variability (5–7). For achieving best-possible outcomes in a patient, health care professionals (HCPs) need to carefully consider the specific disease course of the patient in front of them. Hence, MS treatment needs to be tailored to the individual patient’s needs and requirements to take full effect. MS treatment and management are thus highly complicated and complex. People with MS (pwMS) and their doctors, nurses and caregivers alike would thus presumably greatly benefit from a tool helping them to navigate MS treatment and to coordinate their efforts to keep the disease in check.
We are convinced that digital patient pathways – if properly designed and applied – are the best tool available for this purpose. Recent advances in the field of artificial intelligence (AI) open new possibilities regarding the interpretation and use of big data from not only a cross-sectional, but also a longitudinal perspective. This is also why digital pathways are essential for putting modern MS management into practice, i.e., by building and introducing digital MS twins to MS care (8, 9). However, as far as we are aware, the potential use of digital pathways in MS management and approaches to the implementation of pathways systems in MS care have not been further investigated yet. In this paper, we thus set out to close this research gap and present a modular-integrative framework for the further development of digital patient pathways for MS treatment.
The concept of pathways in medicine goes a long way back. Therefore, we will first go on to elaborate on the history, characteristics and functions of pathways in healthcare in general in, before explaining our vision of digital patient pathways for MS in more detail.
In conclusion, we will explain how digital patient pathways in combination with AI may also lead to the discovery of new MS phenotypes and why digital patients, metaphorically speaking, may be considered the arteries of any kind of digital twin for MS. In the fourth section then, we will touch upon major challenges that are likely to arise when it comes to building digital patient pathways in the real world. Finally, we will provide a short summary of all sections and an outlook on future MS research.
2 Past and present: pathways and their application in healthcare
Ever since money became an issue in healthcare, process optimization and quality management have attracted growing attention among healthcare researchers, practitioners and policy makers (10). In search of solutions, they started to bring management techniques such as the Critical Path Method (CPM), Six Sigma, or the Just-In-Time concept by Toyota into healthcare (11). This eventually led to the introduction of clinical pathways in the 1980s. Since then, the concept of pathways has spread around the world and has steadily evolved in different directions and under various names [see Figure 1 based on (12–24)] which include, e.g., the following to name just a few: critical pathway, clinical pathway, integrated care pathway, care pathway and care map (16).
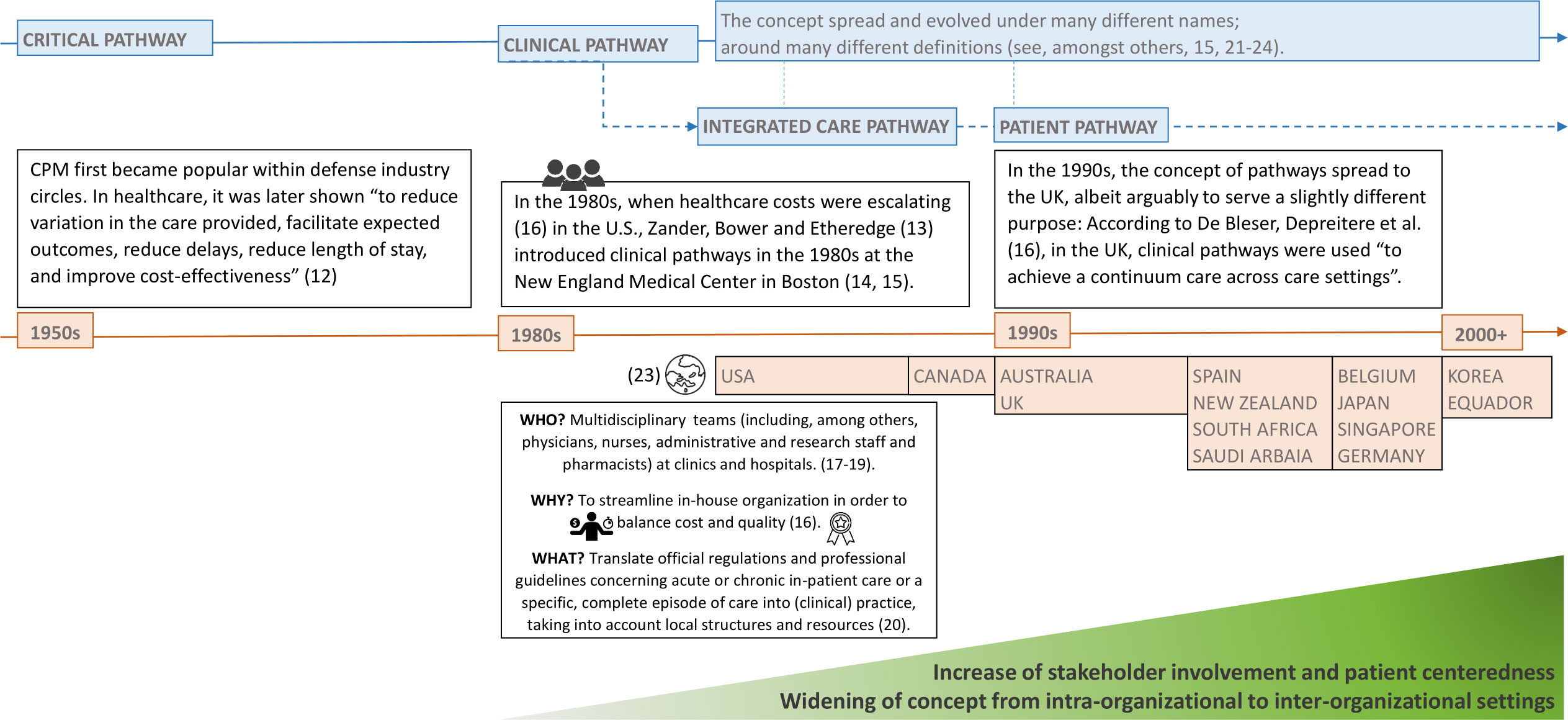
Figure 1 The conceptual evolvement of pathways in healthcare over time. Source: Self-prepared, icons created by Freepik – Flaticon, https://www.flaticon.com/free-icons/.
Kinsman, Rotter et al. (24) set out to resolve the conceptual confusion and developed an operational definition for what could actually be deemed a clinical pathway, independent of the exact terminology used. They came up with five criteria and tested their usefulness against 263 publications (22, 23). In their review they also included studies which did not explicitly use the term clinical pathway, but used terms such as “care model”, “care map” or even plainly “protocol” instead. More recently, Richter and Schlieter conducted a scoping review (25) in relation to the term patient pathway as well as a later survey (26) to validate their concept of patient pathways.
Both pathway concepts are presented in Table 1, highlighting overlapping contents and differences, which mirror the conceptual evolvement over time. As shown there, patient pathways constitute an extended version of clinical pathways: They include more stakeholders at all stages of their life cycle (development, implementation and use) and shift the focus to the patient. This was not common in the past, but has been increasingly advocated by researchers (18–20).
Karen Zander, one of the early pioneers promoting the implementation of clinical pathways in the 1980s, however, already back then prompted the evolution of pathways in this direction. According to De Bleser, Depreitere et al. (16), in her article of 1988 (27), she also suggested to widen the concept of pathways to incorporate all aspects of patient care, i.e., including prospective plans for all disciplines involved in patient care. In this regard, Zander then started to speak of integrated care pathways [see, e.g., (21)].
With regard to chronic diseases such as MS, and the variety of different HCPs being involved in MS care due to the disease’s overall complexity, this conceptual development deserves special attention. Up until now, there are only few articles available on pathways concerning MS care, even though pathways in healthcare in general received a lot of attention in research and in practice in the past. Examples in the area of MS include integrated care pathways (paper-based) for MS rehabilitation (28), a clinical pathway (paper-based, albeit it was suggested that this pathway could also be used in an electronic format) for the care of pwMS at a hospital in Spain (29), and a logic model (implementation yet to be explored) for the design of clinical pathways for the identification and management of cognitive problems in pwMS (30). Considering that patient pathways are a better fit for MS due to their more holistic perspective and with digitalization on the rise, the concept of pathways may now well become more popular within the domain of MS. One key feature which might prove beneficial there is variance analysis.
Zander described its importance as follows (21, 31): “Real progress in healthcare practice will not be made until variances from measurable intermediate goals and patient responses and outcomes are combined with other data and transformed into knowledge.” This is to say that a pathway also serves as a benchmarking tool. It allows the care team to detect any deviations for a given individual patient from procedures and outcomes that have been defined as standards or milestones along the pathway for a specific homogenous patient population. Retrospective pathway data then can also be used for the further investigation of the potential reasons for observed variances. Among others, Du, Huang and Zhou (32) conducted a review of 496 papers on pathway variance research, covering a 25-year time period from 1994 to 2018, and provide a comprehensive overview of different approaches that can be used for variance analysis.
As Zander (21) points out, assuming a bell curve, a 32% variance is always to be expected as well-designed pathways then ought to reflect patterns in care provided to approximately 68% of discreet patient populations in relation to a given disease and its treatment across time. Variance analysis is a key function of pathways as it is crucial for quality management and thereby for the standardization and harmonization of clinical practice and healthcare services across all disciplines and providers involved (20).
A list of all pathway characteristics touched upon in this section and examples derived from a comprehensive literature study can also be found in De Bleser, Depreitere et al. (16). From our perspective, however, some of the characteristics mentioned by them can rather be classified as functions than as characteristics (e.g., management of patient care and variance analysis).
2.1 Next level: pathways going digital
In the past, pathways used to consist of multiple paper-forms, including preprinted order sets, a documentation system for outcomes, a variance reporting system, and frequently, patient education materials. For the organization of entries two designs were common: the matrix design similar to a Gantt chart and the algorithm design similar to a decision tree (33). The matrix design essentially corresponds to the chain models described by Vanhaecht, Panella et al. (15) who further stated that pathways could also come in the shape of hub models or web models.
Nowadays, data previously recorded on paper are either digitized post hoc (ideally automatically using optical character recognition applications) or are recorded via digital applications on electronic devices a priori. This also affects pathways in healthcare as thereby digitalization sets in and changes how data can be generated, collected and used. It brings about fundamental changes in how information on a patient’s health state can be gained, saved, retrieved as well as merged and analyzed in conjunction with relevant context information, e.g., on a patient’s lifestyle or that of other patients with the same disease and similar characteristics.
Digital information from pwMS can be stored in virtual databases. Virtual databases may allow the automatic integration of complex multimodal information with AI. This might include not only brain imaging and regular neurological examination, but also data from wearables, ambient sensors or omics (e.g., metabolomics) (34). Some information, such as the date of recording, can even be added automatically by the computer (e.g., the time and date when a patient’s information is being changed or updated).
Moreover, in consequence of digitalization, some steps along the pathway that could only be carried out at a certain time (e.g., within consultation hours) and place (e.g., hospital) in the past may now be completed virtually and even from remote (e.g., collecting patient reported outcomes) while other steps still require face-to-face contact (e.g., taking of blood samples) and can only be digitalized in part (e.g., electronic submission of blood work results). This, in turn, suggests that healthcare processes now overall may comprise even more activities due to digitalization: Telehealth interventions such as virtual meetings with a physical therapist and virtual consultations with a neurologist may now complement regular inpatient and outpatient appointments. Hence, the range of health care services may be extended.
On the other hand, any such service (e.g., regular check-up) usually relies on the execution of more than one process which again consist of multiple steps (scheduling of appointment, attendance of appointment, any tasks that need to be carried out to complete the check-up itself, follow-up tasks such as handing out of prescriptions and letters of referral etc.). Therefore, at the same time, digitalization may also lead to a reduction in the number of steps needed for the completion of any processes that might belong to a service (e.g., online transfer of prescriptions rather than printing out prescriptions, giving them to the patient who then may take them to the pharmacy where they are scanned before the patient is given the prescribed medications). Hence, the number of steps needed for a particular service may be reduced.
Researchers describe this integration of telehealth interventions into standard care as “hybrid telehealth model” (35–37) or, in general, speak of “hybrid models” (38, 39) or “hybridization” (40). Phrasing it this way highlights the fact that physical face-to-face interaction neither is nor will be entirely be replaced by digital means. Conducting a literature review of telehealth interventions studies in the field of MS, Xiang and Bernard (41) stated that telehealth interventions had been found to reduce missed work days as well as travel costs associated with follow-up appointments and that patient and healthcare providers overall were satisfied with their utilization while disparities in the access to virtual tools amongst vulnerable populations and completion of neurological exams from remote were still posing a challenge. Telehealth interventions studied and used so far include, e.g., MSCopilot (42) and MS Sherpa (43). A comprehensive list and discussion of potential telehealth interventions applied in pwMS can be found in (1).
Despite all progress made in this regard, care will partially still have to be provided on site and in person outside of the virtual space and through humans, not machines. But in light of ongoing digitalization efforts, the functions of the traditional paper-based pathway concept in healthcare can be upgraded.
Organizations have only just started to adjust their pathways accordingly in recent years, and researchers have increasingly presented new approaches for pathway development considering the changes brought about by digitalization and new technological advances such as AI. Again, different terms are being used in this context: E.g., “digital health pathway” (44) or “digital care pathway” (45–48), “partially digital pathway” (49), “human-centered integrated care pathways” or simply “integrated care pathways” (50), or “digitally enabled care pathway” (51–53).
Table 2 provides a comprehensive, albeit not exhaustive overview of corresponding changes in selected pathway functions (traditional paper-based vs. digital pathway) along with potential benefits and drawbacks.
In conclusion, digital pathways can be used to virtually track when, where, and what types of data containing relevant patient information are being created by whom (using which instruments and applications) in the care process as part of the patient’s journey through the health system. In addition, digital pathways can also provide virtual guidance to patients and HCPs alike about what ought to be done next. Providing a defined sequence of activities and events as guiding reference is also a key function of their traditional paper-based counterparts, but by means of software design and programming, navigating pathways in the digital sphere becomes comparably more fluid: E.g., automated searching for a certain piece of information in a virtual database with a computer is much quicker than going through individual paper records by hand, and events can be automatically ordered by time and matched and compared to those of a given standard pathway (pathway template).
2.2 How to: the digitalization of pathways
Up until now, however, the number of examples for digital pathways that can be found in the literature is still fairly low. Examples we found refer to conditions such as arrhythmia (45), acute kidney injury (51–53), HIV (60), genetic testing for breast cancer (49) and Parkinson’s Disease (53). But, as far as we know, a digital pathway for MS, or an approach for how such a pathway could be built has not been put forward yet.
In general, connected health “where patient-centered care results from following defined healthcare pathways undertaken by healthcare professionals, patients and/or carers who are supported by the use of health information technology (software and/or hardware), regulated when used as a Medical Device, and facilitating appropriate health data sharing” (59), is still far from being what many of us experience in our daily lives. A very recent report of the Organization for Economic Development confirms that: “[ … ] innovative ways of delivering healthcare and supportive ICT [information and communication technology] are typically deployed as pilots or research projects, with project specific funding” and “[ … ] many digital tools are not scaled to reach a larger population even if they are successful or show promise” (61).
Recent advances in AI technology, however, might change that as these advances have also steered policy makers around the globe to direct their attention to the potential and risks of this technology for all kinds of industries including healthcare, see, e.g., the recent negotiation of the AI Act within the European Union and a recently study prepared to assist Members and staff of the European Parliament in their parliamentary work (62).
In general, AI as such refers to an algorithm that mimics the cognitive skills and behavior of humans. Machine learning (ML) is a subset thereof and comprises data-driven algorithms that enable machines to learn from data while deep learning, on the other hand, is considered a more advanced subset of ML as the data-driven models used for it resemble multi-layer neural networks (63, 64).
AI technology cannot only be applied in telehealth interventions, but also in the modelling of digital pathways (see Figure 2 along with Table 3). E.g., Schlieter, Benedict et al. (65) propose a conceptual framework for the design and setup of personalized dynamic pathways in the digital sphere. Their reference architecture for the integration of such pathways into given health information systems enables the ongoing dynamic adaptation of pathways to the specific personal needs of patients and caregivers for decision support, education and recommendations.
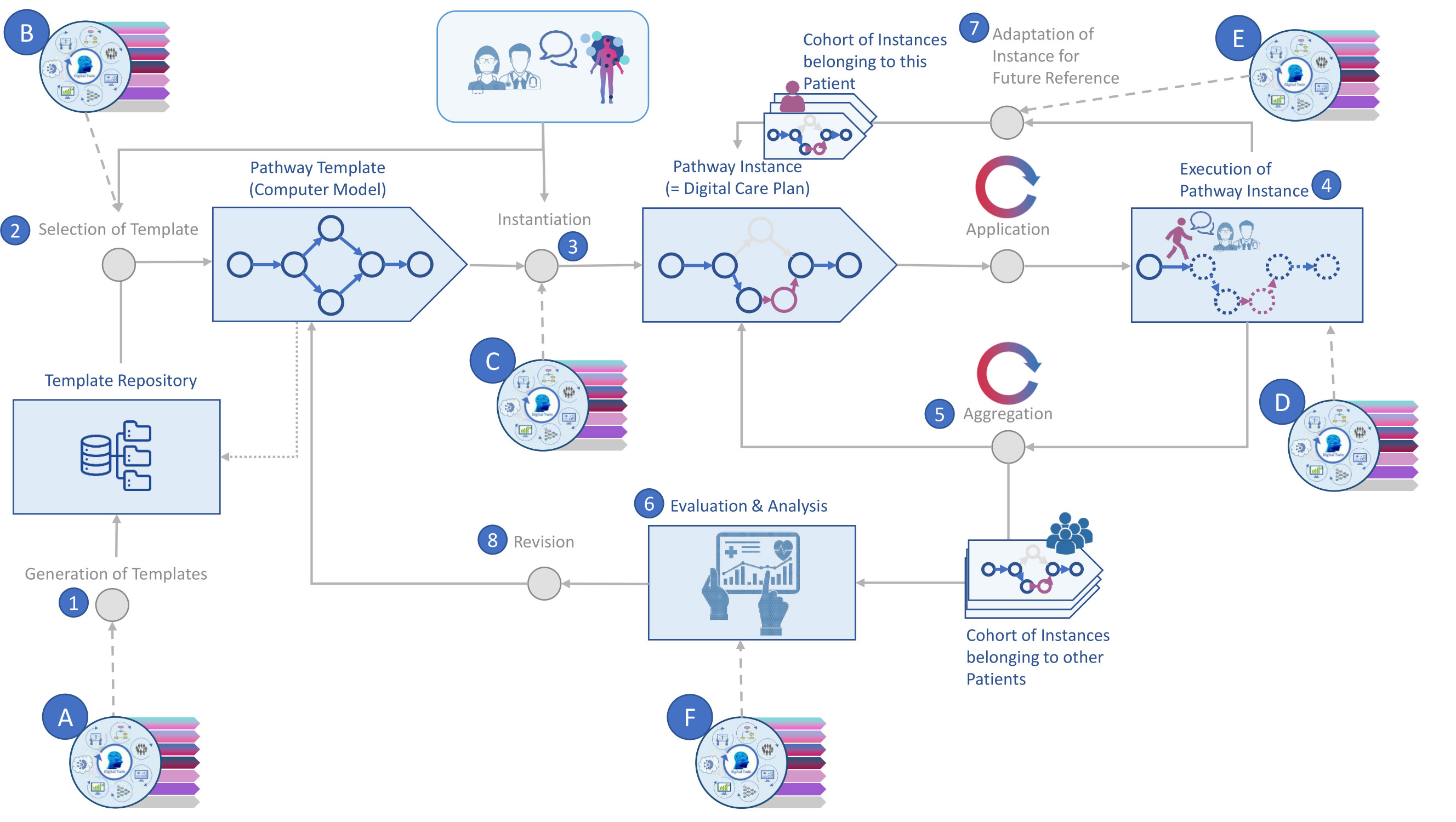
Figure 2 Pathway modelling supported by AI. Source: Self-prepared, using icons created by Freepik – Flaticon, https://www.flaticon.com/free-icons/ and Vecteezy, https://de.vecteezy.com/gratis-vektor. For a detailed description of this figure, please refer to Table 3.
Pathway modelling coupled with AI technology can be broken down into eight core activities (65, 66). Thereof 1) only takes place occasionally (e.g., substantial changes in professional guidelines) while 2) and 3) only refer to newly diagnosed patients or patients visiting the facility where digital patient pathways are being used and modelled this way for the first time. Table 3 provides a short description of the relevant activities.
The application of this modelling approach yields pathways that support the intra- and interorganizational management, standardization and harmonization of all patient data that are being generated along the pathway across multiple data sources by multiple stakeholders. A specific digital patient pathway then provides insights in how different procedures are carried out in a specific healthcare setting and enables the virtual integration of multimodal data. In consequence, it also allows for the structured assessment of every step that any one patient takes on their (path)way through the healthcare system, i.e., the evaluation of any health care services that have been or are planned to be carried out. This, in turn, is essential for quality management.
3 Wanted: digital pathways for MS care
The digitalization of MS care, including the development of digital pathways, may lead us to better grasp and handle the disease’s complexity and could help us to overcome related challenges in MS care. The interplay between neuroinflammation and neurodegeneration which varies for each individual patient leads to heterogeneity in symptoms, course and outcomes in pwMS (67). This is what makes MS diagnosis and treatment particularly challenging.
Even though the introduction (in 2001) and recurrent revision of the McDonald criteria (in 2005, 2010 and 2017, respectively) have significantly shortened the time to diagnosis and improved sensitivity overall (68–71), those criteria need to be applied with caution as they were not designed for the differential diagnosis of patients, but rather to identify MS or the likelihood of manifesting MS in patients presenting with a clinical isolated syndrome (CIS) (72, 73). Despite all progress made in this regard, an extended time to diagnosis from the onset of symptoms is still common (74–76), and MS misdiagnosis also still poses a problem in practice (6, 77–79).
According to the authors of the Brain Health Report, diagnostic delay is primarily caused by inadequate access to specialist healthcare (i.e., a low per capita number of neurologists and lack of diagnostic tools such as MRI scanners) and insufficient awareness of the disease among family members and primary care physicians. Atypical symptoms (76) or older age at the onset of disease (75) may additionally contribute to diagnostic delay. Ultimately, delays may occur at two points in time along the pathway: One the one hand time may be lost until a patient finally decides to seek professional help. On the other hand, it then may take even more time until a specialist finally diagnoses MS in a patient (80).
To counter these challenges, researchers and MS professionals advocate the establishment and implementation of quality standards and recommend that specialists with expertise in relevant functional domains should be involved in MS care (81, 82). Digital patient pathways can help put these recommendations into practice as they can support the management and coordination of MS care across the whole care chain. For this purpose, e.g., teams from specialized clinics (also called MS care units [82)] may be put in charge of the overall administration of digital patient pathways. Members from these teams can virtually share their knowledge and experience, e.g., first of all, by defining a set of standard pathways, and, second, by providing their colleagues and patients following a pathway with virtual counselling services (e.g., via virtual notes, video sessions). In doing so, the team may oversee MS management and treatment from afar and on site.
There is a large body of MS research available as well as several evidenced-based medical national and international guidelines [e.g., (83–85)] which would jointly constitute another valuable source of content for pathway modelling (see also Section 2.2). Resulting digital patient pathways could provide their users with the specialist knowledge gained from all of these sources and could thus also be used to educate and train general neurologists and MS experts in the correct application of diagnostic criteria and MRI interpretation to avoid misdiagnosis as suggested by researchers (78).
A set of predefined standard pathways in the pathway repository, enriched with scientific findings from accredited sources forms a pool of machine-readable knowledge. Everyone who has access to a digital patient pathway thereby also gains access to this knowledge, and to the specialized team administering it. What makes using digital patient pathways worthwhile though is their flexibility. Looking at the next steps suggested, a neurologist may still decide to change the pathway and, e.g., initiate a different type of treatment than was recommended by the pathway. As depicted in Figure 2, with the help of AI this change of plan can be compared against what happened in other cases, such that the prediction of the further pathway will be adopted accordingly.
Even without the added benefits of AI in terms of predictions and without the AI-supported automatic adjustment of suggestions, predefined digital standard pathways by themselves provide a virtual structure, which may guide HCPs and patients in their doings, offering significant advantages over paper-based pathways (see Table 3). Their virtual structure may help HCPs and patients (1) better grasp the disease and treatment strategies in their complexity by keeping them focused in the present while enabling them to take a step back and take a look into the past and future when needed, simply following the pathway in either direction. As digital patient pathways, if used properly, also govern data collection, all one needs to know is virtually available in one place: expert knowledge as described above, aggregated health data from MS patient populations and health data of the individual patient of interest. Connecting these different pools of knowledge and running further analyses over the data available has not been possible in times of paper. But through this virtual connection and thereby through the integration of competences, digital patient pathways may eventually (2) speed up diagnoses, (3) support the timely initiation of interventions and (4) patient involvement in the decision making process, (5) facilitate monitoring, (6) counter the shortage of MS neurologists, (7) help minimize risks and may (8) even contribute to the post-marketing refinement of drug profiles.
One major benefit of digital patient pathways is that they can promote the standardization and harmonization of MS care by providing a virtual structure for everything that needs to be done. By applying the modular approach from Section 3.1., digital patient pathways may still be manually adopted in an agile manner. This requires a virtual dashboard application, but not necessarily the use of AI.
Integration of competences means that digital patient pathways also constitute a virtual link between patients and their caregivers – everyone having access to the virtual dashboard application where the pathway is displayed can tap on its embodied knowledge, but also contact service providers that have been linked to its modules, i.e., the individual steps belonging to that pathway. Hence, overall access to specialized care can be improved through the inclusion of online services from specialized clinics (e.g., second opinions on MRI scans, test results etc.; online consultations) in the pathway. Digital patient pathways thereby would provide a platform and structure for MS-related communication and education which might also help increase general awareness of the disease.
Even though diagnostic delay is a common problem, pwMS are on average still comparably young at diagnosis, i.e., 20 to 40 years old (67, 86), and their median life expectancy is estimated to be only about 7 years lower than that of the general population (87, 88). Hence, most patients who have recently been diagnosed with MS will usually go on to live with the disease and its symptoms for many decades. This leads to a huge amount of multimodal data [see, e.g., (89)] being accumulated over time for each patient, including, among others, MRI scans, laboratory test results, results from cognitive tests, values recorded via gait and jump analysis.
All of these data characterize the individual state of disease in a given patient. Collecting relevant information from different sources (e.g., other HCPs), sorting and ordering all this information for one single patient can already pose a challenge and contribute to delays in diagnosis and treatment in practice. Moreover, the number of features (variables) recorded for one individual patient may also well exceed the total number of patients in a given MS dataset, or, in more general terms, the number of features available for one data record may well exceed the total number of records included in a dataset.
Such high-dimensional datasets are often sparse, noisy, cross-sectional and lack statistical power. This is even more likely to be true for high-dimensional datasets comprising multiple modalities. Traditional data analysis methods are not fit to cope with these kinds of datasets. ML and AI-based approaches supporting big data analytics on the other hand can help tackle these problems (90). But for the application of such, data need to be available in a machine-readable format and need to be collected in a standardized and structured manner – one of the key functions digital pathways have to offer.
Despite posing analytical challenges, the multimodality of MS data can also be considered an advantage. Regarding the interpretation of image data in general, it has been shown that results improve when practitioners have access to clinical and laboratory data which provide them with critical context information (91, 92). The availability of additional information is not only deemed important by radiologists (93), but also affects image-data interpretation in other disciplines such as pathology, ophthalmology, and dermatology (94). Huang, Pareek et al. (95) conducted a systematic literature review and found that the same was true for the application of AI/ML in the medical field as multimodality fusion models exhibited an increased accuracy of 1.2% to 27.7% compared to single modality models when used for the same task.
The number of data records in the dataset(s), i.e., data volume, and modalities included, determines what ML technique and what algorithm can be applied. With regard to data volume the following typically holds true: The higher the sample-to-feature ratio (the number of data samples divided by the number of features), the easier it will be to obtain any meaningful results through the use of ML, in particular supervised learning. Hence, combining multiple data sources and techniques as well as tracking and analyzing of ML metadata which are being generated in the process to validate the usefulness of generated models is highly recommended (90).
All of the challenges presented in this section call for new approaches to improve MS diagnosis and treatment. And digital pathways could literally lead the way to the application of big data analytics in MS care which eventually could help us to overcome these challenges. In the following sections, we thus present our ideas for a modular-integrative framework for the building of digital pathways in MS.
3.1 Piece by piece: a modular service portfolio for digital pathways in MS care
The idea of modularity was originally confined to the manufacturing of goods, but the idea has long spread into services. Following the concept of modularity, a product or service can be split up into individual standardized components, i.e., modules, which then may be mixed and matched together to obtain a functioning final version of the product or service in question. Each resulting module needs to constitute a self-contained unit such that it can be shuffled around or skipped as desired when building the final version (96). Modularity thus facilitates process management. On top, it allows for the customization and personalization of products or services but does not require one to start from scratch (97–99).
Meanwhile, the concept of modularity has also been applied to healthcare services. Recently, Peters and Richter (100) conducted a literature review and survey for the modularization of healthcare services. Taking into account given process dependencies, Peters and Richter (100) finally presented a list of thirteen modularization parameters, grouped into four categories. For each parameter, they provided an exemplary question through which respective process dependencies could be identified, e.g., for patient needs dependency: Do the processes contribute to satisfying the same patient need?
Mirroring the approach presented by Peters and Richter (100), we have identified different health care services which are relevant for MS patients. Following the example of Peters and Richter (100), those services can be grouped together forming a modular service portfolio (Figure 3). We propose that those categories themselves can be seen as parallel paths, implying that a patient’s pathway can be separated into different paths as services belonging to the same category could also be tracked along one and the same path. Basically, this constitutes a filtered view on the patient’s pathway, setting the focus on the chronological sequence of services for a specific category and the longitudinal development of corresponding data input values (e.g., choice of medication, dosage etc.) and values of outcome measures (e.g., EDSS scores, MRI data).
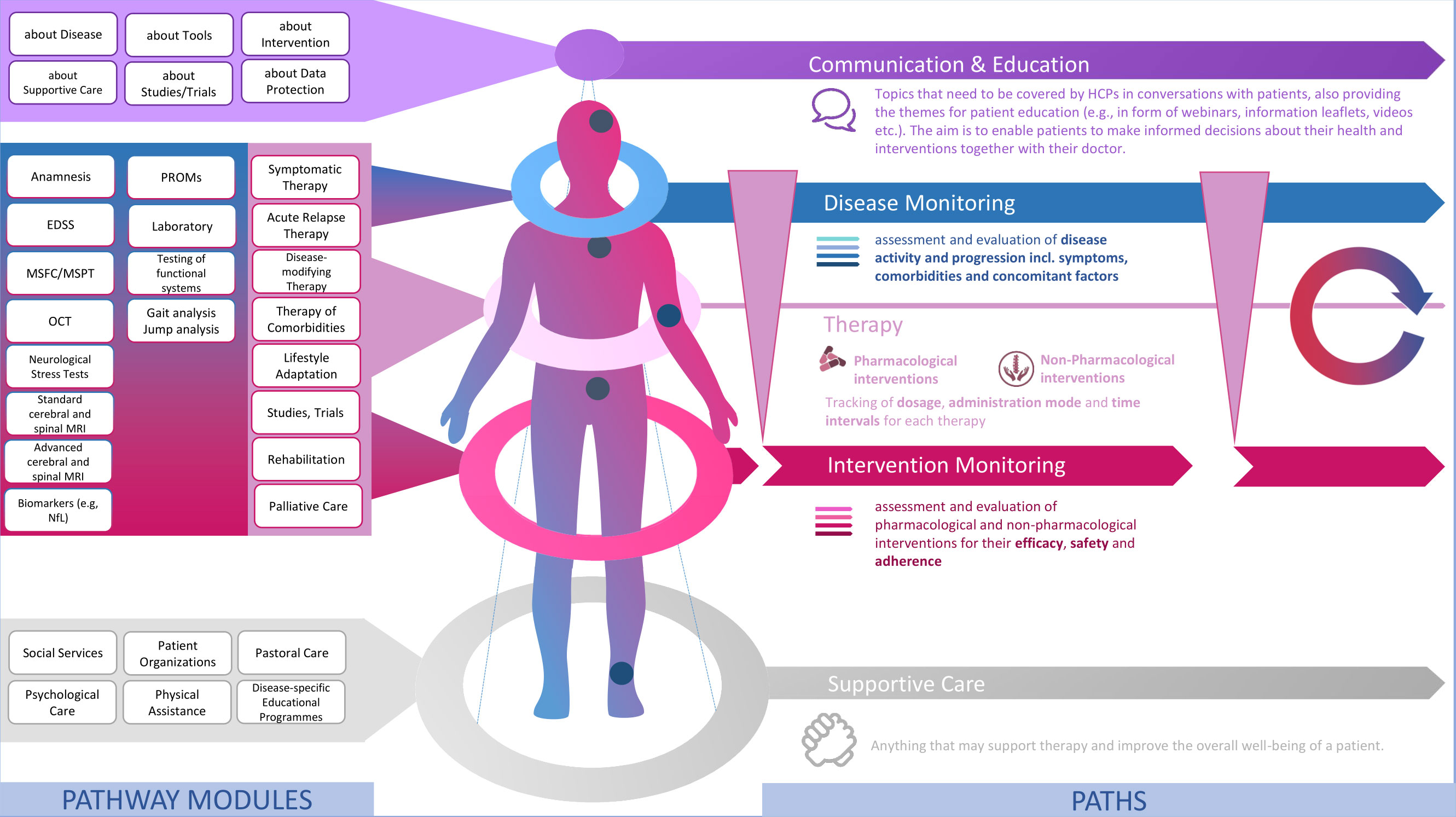
Figure 3 A modular service portfolio for the design of digital patient pathways in MS care. Source: Self-prepared, using icons created by Vecteezy, https://de.vecteezy.com/gratis-vektor.
3.2 Paving the way: the building blocks of digital pathways for MS
Modules of the same category serve one common, dominant purpose as outlined in Figure 3. Equally, modules of the same category are characterized by similar process dependencies, e.g., in terms of location and stakeholders. Modules in the top category Communication & Education include any services which aim to inform and educate patients on MS and all aspects relating to their life with MS and anything they may encounter in the course of MS treatment. Therefore, modules of this category address patients’ needs for information and education.
Then there is a number of modules which are made up of the diagnostic tools that may be used for the monitoring of the disease itself and that of any interventions. Hence, there are two distinct monitoring paths along which related modules can be tracked: Disease Monitoring and Intervention Monitoring. From our perspective, this distinction allows for a more systematic and agile monitoring, paving the way to precision medicine in MS care. Modules of this category equal the structured set of components from our monitoring matrix (101) and are derived from evidence-based guidelines for MS treatment which mostly takes place at a specialized MS clinic, hospital or at a neurologist’s office. I.e., those modules mainly share healthcare system-related and general dependencies.
Intervention Monitoring, in turn, is tightly linked to therapy. The category of the same name comprises different types of Therapy. Along this path, dosages, administration mode (self-administered vs. administration by medical staff; oral, infusion, injection etc.) and time intervals can be tracked. Again, modules of this category are highly healthcare system dependent as the prescription of any kind medical treatment is strongly governed by national regulations and public authorities such as the U.S. Food and Drug Administration (FDA) and the European Medicines Agency (EMA) overseeing the approval of medicines for marketing.
At the bottom then follow services which do not necessarily require medical expertise but may still have a positive effect on the overall well-being of patients, i.e., may make a positive difference in a patient’s life with the disease and support MS therapy. As the impact and potential benefits of such Supportive Care should not be neglected in MS care we argue that these should also be integrated in digital patient pathways. Modules of this category again strongly cater to patient needs and heavily depend on patient engagement and personal encounters with service providers.
When it comes to process modeling, services represent the procedural operations of health care providers and are also referred to as activities. With regard to the MS use case at hand, the service modules included in the modular service portfolio in Figure 3 represent a set of activities or reusable (sub-)processes. As outlined in Peters and Richter (100) with reference to (102), in general, each module thereby contains at least one activity which is carried out to provide patients with the service it stands for.
If a patient visits a healthcare facility, a number of activities are usually performed by different actors (e.g., administrative and medical staff). The visit itself is the (start) event which triggers these activities. The sequence of activities may be determined by interdependencies with other activities (103), e.g., IT and general infrastructure of the facility, availability of staff and rooms at the facility. Among others, AI technology can help find the optimal sequence of activities taking into account given constraints like these (see Section 2.2).
There is a wide range of activities which may take place in a healthcare setting. With regard to activities specific to MS care see, e.g., the modules which refer to typical clinical and paraclinical procedures used for MS monitoring such as the application of the Expanded Disability Status Scale (EDSS) or magnetic resonance imaging (MRI). In the literature, a broad distinction is drawn between clinical processes (e.g., disease and intervention monitoring) and organizational processes (e.g., administrative processes such as billing). Even though our exemplary MS service portfolio does not explicitly refer to administrative processes (activities) such as the registration of patients at the counter and the writing of prescriptions, those naturally occur and may be carried out as part of the modules listed in Figure 3. Besides that, one could also add another category including modules referring to the general administration of MS care. Modules of this category could include activities (e.g. billing, bookkeeping etc.) which need to be carried out for each of the modules listed in the other categories and could thus be aggregated to form a module of their own (e.g., accounting).
3.3 Filling in the blanks: adding another layer of information
Well-designed health information systems can be configured to automatically add and store meta-information in database tables (event logs) for later analyses. The so-called process execution data may contain some basic information such as what particular activity was performed when and by whom (e.g., which staff member), concerning which patient. Hence, event logs show what happened when the process was executed in reality. Insights gained from the analysis of event logs, i.e., from process mining, can be used for the creation of new and the optimization of existing processes (104, 105) and therefore provide valuable insights for quality management and the refinement of pathways by means of process engineering (106).
This is why we propose to also specify corresponding attributes according to local conditions for any modules that are to be included in the final modular service portfolio. From our perspective this would increase the value of the portfolio as it would likely support the subsequent translation of modular pathways into a modeling language such as BPMN for the development of a virtual dashboard for instance.
Modeling languages have commonly been used for the graphical depiction of pathways, defining the logical sequence of events and activities of a process, e.g., for software programming. The use and interpretation of such languages, albeit to a variable extent, requires expert knowledge whereas modular building blocks can easily be pieced together to quickly draw up a specific patient pathway. Peters and Richter (100) therefore conclude that resulting modular representations of patient pathways might also be easier to grasp for patients. Along this line, we propose that our modular-integrative framework could also enhance doctor-patient communication and thus support shared-decision making in MS care.
In Figure 4, with reference to De Roock and Martin (104) and Munoz-Gama, Martin et al. (105), we further list possible attributes which could enhance the utility of pathways. Moreover, we find that the modularization parameters suggested by Peters and Richter (100) could also be used to identify suitable attributes which should be recorded by information systems supporting healthcare as corresponding attributes largely overlap with the contents of the modularization parameters they describe in their paper (see Section 3.1).
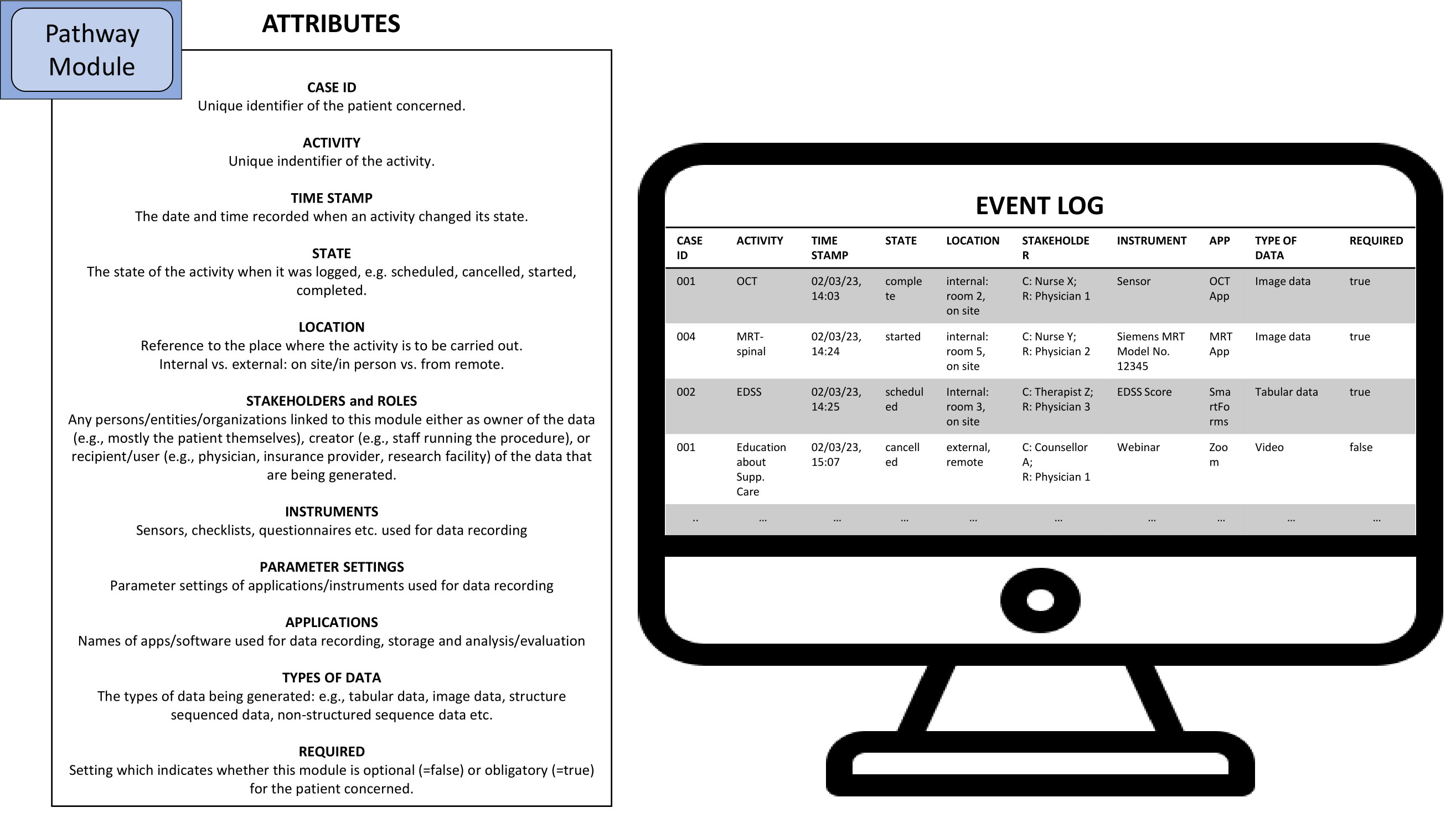
Figure 4 Possible attributes of MS pathway modules which can be used for the generation of event logs (C=Creator, R=Recipient). Source: Self-prepared, using icons created by Freepik – Flaticon, https://www.flaticon.com/free-icons/.
Hence, if a new health information system is to be chosen or is even specifically being developed, considering which attributes should be stored in event logs in the first place can greatly enhance the variance analysis function of digital pathways.
3.4 Coming together: digital pathways à la carte for people with MS
With regard to the definition of digital patient pathways, categorizing pwMS into different patient groups (subtyping), or the identification of respective patient groups (clustering) in the first place is of particular interest. This is essential to obtain more homogenous patient populations which can be used for defining a set of standard pathways and a common use case for AI/ML.
Once homogenous patient populations have been identified, corresponding pathway prototypes, i.e., standard pathways, can be derived from their data via data and process mining. A prototype pathway equals the chronological sequence of pathway modules belonging to a specified subclass of patients. Designated modules may then be added, deleted or switched to fit the individual patient as needed. Instead of building digital patient pathways from scratch (menu-based), the prototype approach allows practitioners to make intelligent use of any knowledge buried in the data which has been collected from patients in the past (96, 97).
Accordingly, on the premises of a virtual data repository and shared access for all members of the MS care unit, external providers of MS care services and their patients, i.e., all the stakeholders of the pathway, the data stored along the pathway can be processed and analyzed using AI/ML techniques (see also Section 2.2).
Table 4 provides an overview of ML techniques and potential use cases in healthcare in general and MS in particular.
The journey of the patient can be tracked and modelled along multiple paths as shown in Figure 5 which allow for a detailed analysis of the individual data that has been collected during the execution of pathway modules. Moreover, this way new individual patient data can be compared to population data fitting the identified subtype of the individual patient. AI/ML-supported variance analysis can then be used to forecast potential future pathways. Based on these, potentially fitting treatment options for the patient may also be identified. In essence, the combination of different ML techniques for these purposes enables the personalization of MS care as it widens the number of dimensions that can be processed to support clinical decision making.
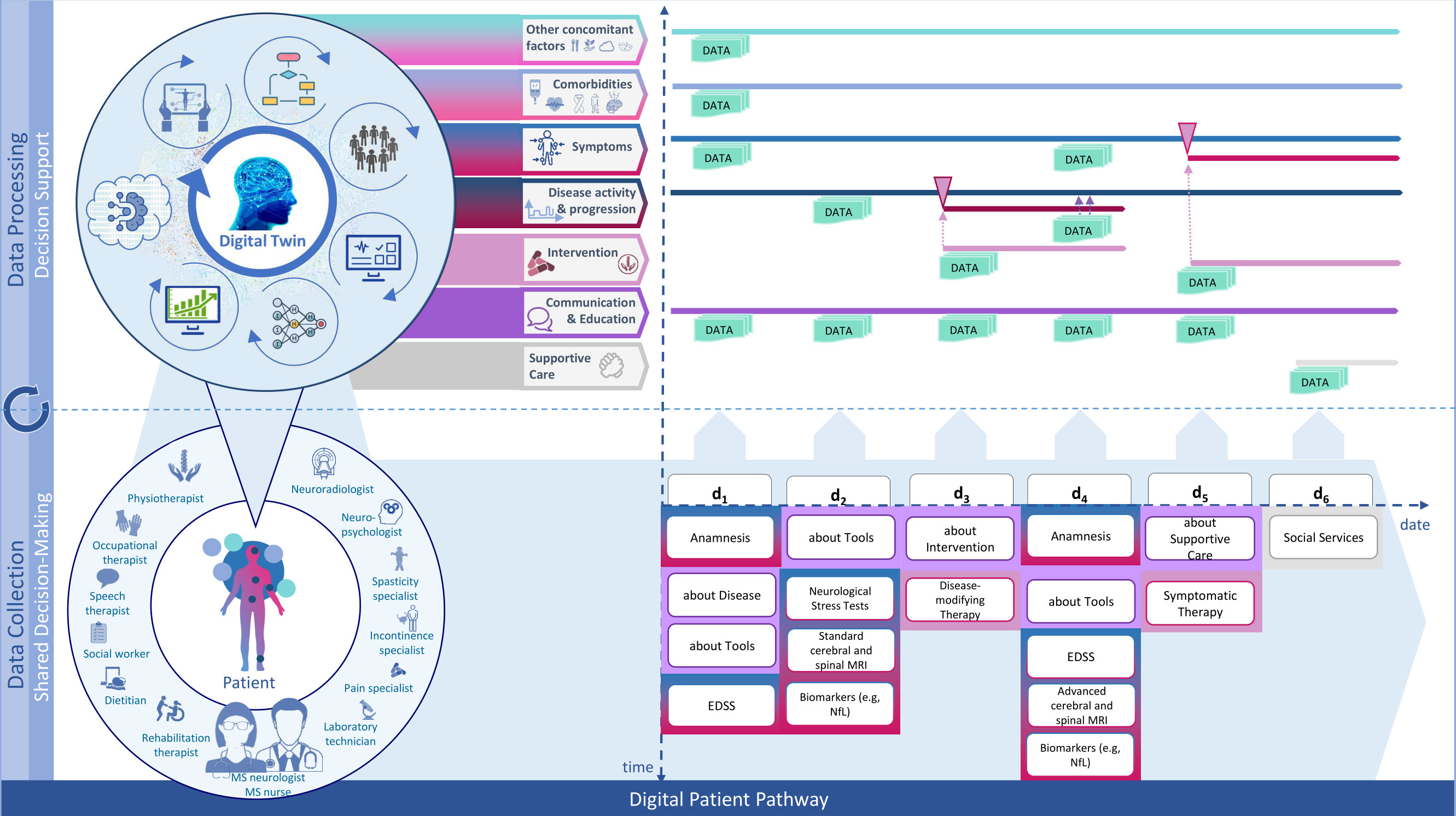
Figure 5 Modular-integrative framework for the design of digital patient pathways. Source: Self-prepared, using icons created by Vecteezy, https://de.vecteezy.com/gratis-vektor.
This is how personal digital twins may be generated which mirror different dimensions of accumulated patient data and outcomes. All digital twins of one patient together present the most complete picture of the health state of that particular patient. They add up to being the actual Digital Twin of the patient.
In healthcare, clinical decision support systems (CDSS) have already been investigated for a variety of use cases [for a scoping review in the medical field see, e.g., Noll, Schaaf and Storf (113)], e.g., to determine the future direction of disease course and options for therapy (8, 114–116). One prominent example of such a CDSS in MS care is MSProDiscuss (117). In contrast, to the Digital Twin however, existing CDSS do not incorporate data from all dimensions which is why the envisioned Digital Twin would be superior to those.
3.5 Walking along the pathway: MS treatment could change for the better
MS symptoms may last for days or weeks but may also disappear again (remission). However, if left untreated most pwMS will develop disease symptoms that will gradually worsen over time (relapsing). At one point in time, there will then be no more discernible relapses and remissions implying that pwMS will have transitioned from relapsing-remitting MS (RRMS) to secondary progressive MS (SPMS) (86).
About 10-15% of pwMS experience gradual worsening from the start, which is thus referred to as primary progressive MS (PPMS). In rare cases, some people with PPMS will also suffer a relapse, which is known as progressive-relapsing MS (PRMS). The majority of patients, however, exhibit RRMS, with percentages of respective total populations studied varying from 76% (76) to 85% (67).
Up until now, the diagnosis of MS implies that pwMS are assigned either one of these phenotypes. With regard to MS, the definition of phenotype traditionally follows the description of the clinical courses of the disease (118, 119), which are also part of the McDonald criteria in their last revised version of 2017. In addition to that, imaging and other paraclinical markers have been incorporated into the criteria over time (6).
In general, the term phenotype, however, refers to the observable characteristics of a disease whereas the word endotype is used to describe the molecular, pathological or pathophysiological mechanisms underlying a disease (120–122). In the literature, especially with regard to asthma and allergies, it has been highlighted that phenotypes neither necessarily relate to nor provide insights on the underlying pathogenetic mechanisms of a disease. In addition to that, it has been found that phenotypes frequently overlap and may change over time. Therefore, research in the field of asthma and allergies has increasingly been steered towards the identification of disease endotypes, i.e., identifying compilations of disease mechanisms explaining the disease expression in groups of patients (123). In this ongoing discussion, biomarkers constitute a link between both concepts as they may provide information about the pathophysiology of an underlying disease, the course of an illness, and/or the response to treatment (124, 125).
With regard to allergies the argument has been made that the focus on phenotypes where one single phenotype comprises multiple molecular endotypes constitutes an oversimplification diminishing the efficacy of treatment (126). Resolving phenotype populations into endotypes where people with the same endotype exhibit the same disease mechanism has also been advocated in pharmacological research (127, 128) where a paradigm shift towards the targeting of the mechanism(s) and cause of a disease instead of targeting symptoms and affected organs can be observed. This goes hand in hand with a shift towards precision medicine according to which therapies should be tailored to fit the individual patient. Patients within the same endotype are assumed to be more consistent in their response to a selected treatment because of a common pathophysiology (127).
In MS research, it has also been proposed to change the design of clinical trials along these lines: Manouchehri, Zhang et al. (129) have argued that more research into immunopathological factors that drive disease activity is needed. If current clinical and MRI outcome measures were to be replaced by objectively measurable genomic and proteomic biomarkers, trials would likely become more efficient as it would allow us to narrow down the group of patients to be included in a trial in a more targeted manner. This would lead to more homogenous study groups and minimize the number of non-responders therein. Manouchehri, Zhang et al. (129) even go one step further and root their argumentation on the concept of endophenotypes which represent a subclass of endotypes: Patients within an endophenotype “share a measurable indicator or pattern of disease that lies along the causal pathway between gene expression and the phenotype” (127).
One promising treatment-sensitive biomarker for disease activity in pwMS currently being studied is the assessment of high-frequency serum neurofilament light chain levels (130). ML and AI techniques are also increasingly employed in the quest for new MS biomarkers [see, e.g., (131)]. One more reason, to finally leave the traditional pathway and to go digital in MS care.
4 Implementing digital patient pathways in the real world: challenges to expect
However, getting there will not be easy. The successful implementation of digital patient pathways will greatly depend on patients’ willingness to share their data. Digital patient pathways by design strongly differ from the current implementation of electronic health records as patient would directly benefit from sharing their data along the pathway. In return for their data, they get access to educational contents and instant feedback on their state of health (as far as appropriate) along their pathway. Besides that they are directly connected to a team from a specialized clinic who they can turn to for personal help or by whom they may also be contacted directly if need be. Last but not least, accompanied by their doctor, patients may zoom in and out of their pathway on the virtual dashboard, look at personalized analyses of their data and future prognoses and may thus be able to take a more active stance in managing their disease. From our perspective, all of these things – which we have described in more detail in previous sections – pose a strong incentive for patients to share their data. Even more so in what they stand to gain in terms of drug design and research (see Section 3.5).
Despite all these incentives, patients’ willingness to use digital patient pathways may still be hampered by data privacy and security concerns as health data count among the most vulnerable type of data. These kind of concerns, however, do not only apply to the implementation of digital patient pathways, but to the utilization of data and modern technologies in the realm of healthcare in general. E.g., Paul, Maglaras et al. (132) provide a detailed overview of concerns raised in this regard and also describe a variety of safeguard measures that are currently being discussed in the literature and may be applied to reduce the risk of data theft and misuse.
Related to these concerns is the demand for explainability of AI models, which in turn also needs to be considered when AI technology is to be used for pathway modelling, or for the integration of a chatbot or other AI supported functions into digital patient pathways. Stakeholders interested in implementing digital patient pathways ought to keep that demand in mind, which is also already widely being discussed by researchers [see, e.g., (133–135)].
Another major, but also well-studied problem [see. e.g., (136–138)] which might complicate the implementation of digital patient pathways is the lack of interoperability between different information systems and digital applications in the domain of healthcare.
What these challenges will look like in detail and what other hurdles one might have to take will largely depend on conditions prevailing at the time and location of implementation. Suitable strategies for overcoming these challenges therefore will have to be developed as part of the implementation process. But, as indicated, at least the most prominent concerns are also topics of great interest for other researchers and practitioners in the realm of healthcare and there is already a great amount of ideas available that might prove helpful in the process.
5 Discussion and conclusion
Ongoing digital transformation processes around the world and across industries have prompted the use of computer-based systems. The development of CDSSs that exploit the full potential of current AI technology and ultimately Digital Twins, however, requires agile, interoperable data such that data points, i.e., the multidimensional records from different datasets, as well as individual features thereof can be processed and exchanged separately and in conjunction for the purpose of analyses (139).
In Germany, current implementations of EHRs, information systems and applications used in in- and outpatient settings still lack interoperability, albeit its importance has been widely voiced and acknowledged by different stakeholders (140–143). Developers of CDSSs still likewise fail to adhere to interoperability standards which inhibits their wider adoption in healthcare settings (144).
Once these and other issues (see Section 4) have been resolved and digital pathways for MS have been implemented, however, physicians could employ AI technologies to analyze all available health data of a specific MS patient to get a better picture of the individual disease state of that patient (micro level), leveraging recent research findings and group data from MS registries. Researchers, on the other hand, could use AI technologies to analyze combined data sets from multiple MS patients (macro level) to detect any unknown patterns therein, which eventually could lead to the discovery of new endophenotypes and/or endotypes. E.g., Soenksen, Ma et al. (89) have already developed a methodology for the integration of multimodal datasets into AI/ML systems which could serve as a blueprint for future MS research looking to leverage the AI and ML techniques.
It can be concluded that recent advancements in AI technologies could (1) support the personalization of MS care; (2) help us gain new insights in the origin, potential causes and disease triggers of MS; and (3) result in the discovery of new options for therapy. Further research into respective AI applications for the use case MS considering the whole range of multimodal MS data, however, is still needed. Last but not least the implementation of digital pathways has the potential to give us good push heading into this direction. Hence, we consider the modular-integrative framework presented in this paper as a tool for paving the way towards building digital twins for pwMS. Next, we plan to review its usefulness in practice.
Author contributions
JW: Conceptualization, Project administration, Visualization, Writing – original draft, Writing – review & editing. IV: Conceptualization, Funding acquisition, Writing – review & editing. HI: Funding acquisition, Writing – review & editing. HS: Visualization, Writing – review & editing. TZ: Conceptualization, Funding acquisition, Supervision, Writing – review & editing.
Funding
The author(s) declare financial support was received for the research, authorship, and/or publication of this article. We are grateful that our research project DigiPhenoMS is being funded by the Free State of Saxony, Germany (Funding Guideline: eHealthSax). Diese Maßnahme wird mitfinanziert mit Steuermitteln auf Grundlage des vom Sächsischen Landtag beschlossenen Haushaltes.
Conflict of interest
Hernan Inojosa received speaker honoraria from Roche and financial support for research activities from Biogen and Alexion. Tjalf Ziemssen reports: scientific advisory board and/or consulting for Biogen, Roche, Novartis, Celgene, and Merck; compensation for serving on speakers bureaus for Roche, Novartis, Merck, Sanofi, Celgene, and Biogen; research support from Biogen, Novartis, Merck, and Sanofi.
The remaining authors declare that the research was conducted in the absence of any commercial or financial relationships that could be construed as a potential conflict of interest.
Publisher’s note
All claims expressed in this article are solely those of the authors and do not necessarily represent those of their affiliated organizations, or those of the publisher, the editors and the reviewers. Any product that may be evaluated in this article, or claim that may be made by its manufacturer, is not guaranteed or endorsed by the publisher.
References
1. Scholz M, Haase R, Schriefer D, Voigt I, Ziemssen T. Electronic health interventions in the case of multiple sclerosis: from theory to practice. Brain Sci. (2021) 11:180. doi: 10.3390/brainsci11020180.
2. Wallin MT, Culpepper WJ, Nichols E, Bhutta ZA, Gebrehiwot TT, Hay SI, et al. Global, regional, and national burden of multiple sclerosis 1990–2016: a systematic analysis for the Global Burden of Disease Study 2016. Lancet Neurol. (2019) 18:269–85. doi: 10.1016/S1474-4422(18)30443-5.
3. Disanto G, Berlanga AJ, Handel AE, Para AE, Burrell AM, Fries A, et al. Heterogeneity in multiple sclerosis: scratching the surface of a complex disease. Autoimmune Dis. (2010) 2011:932351. doi: 10.4061/2011/932351.
4. Rommer PS, Milo R, Han MH, Satyanarayan S, Sellner J, Hauer L, et al. Immunological aspects of approved MS therapeutics. Front Immunol. (2019) 10:1564. doi: 10.3389/fimmu.2019.01564.
5. Wojtowicz MA, Ishigami Y, Mazerolle EL, Fisk JD. Stability of intraindividual variability as a marker of neurologic dysfunction in relapsing remitting multiple sclerosis. J Clin Exp Neuropsychol. (2014) 36:455–63. doi: 10.1080/13803395.2014.903898.
6. Thompson AJ, Banwell BL, Barkhof F, Carroll WM, Coetzee T, Comi G, et al. Diagnosis of multiple sclerosis: 2017 revisions of the McDonald criteria. Lancet Neurol. (2018) 17:162–73. doi: 10.1016/S1474-4422(17)30470-2.
7. Reynders T, D'Haeseleer M, De Keyser J, Nagels G, D'Hooghe MB. Definition, prevalence and predictive factors of benign multiple sclerosis. eNeurologicalSci. (2017) 7:37–43. doi: 10.1016/j.ensci.2017.05.002.
8. Voigt I, Inojosa H, Dillenseger A, Haase R, Akgun K, Ziemssen T. Digital twins for multiple sclerosis. Front Immunol. (2021) 12. doi: 10.3389/fimmu.2021.669811.
9. Elkefi S, Asan O. Digital twins for managing health care systems: rapid literature review. J Med Internet Res. (2022) 24:e37641. doi: 10.2196/37641.
10. Rais A, Viana A. Operations research in healthcare: a survey. Int Trans Operational Res. (2010) 18:1–31. doi: 10.1111/itor.2010.18.issue-1.
11. Schrijvers G, van Hoorn A, Huiskes N. The care pathway: concepts and theories: an introduction. Int J Integr Care. (2012) 12:e192. doi: 10.5334/ijic.812.
12. Coffey RJ, Richards JS, Remmert CS, LeRoy SS, Schoville RR, Baldwin PJ. An introduction to critical paths. Qual Manag Health Care. (2005) 14:46–55. doi: 10.1097/00019514-200501000-00006.
13. Zander KS, Bower KA, Etheredge MLS. Nursing Case Management: Blueprints for Transformation. Boston: New England Medical Center Hospitals (1985).
14. Panella M, Van Zelm R, Sermeus W, Vanhaecht K. Care pathways for the organization of patients' care. Bull: Econ Organisation Inf Healthc. (2012) 28:111–22. doi: 10.2478/v10221-011-0026-z.
15. Vanhaecht K, Panella M, van Zelm R, Sermeus W. An overview on the history and concept of care pathways as complex interventions. Int J Care Pathways. (2010) 14:117–23. doi: 10.1258/jicp.2010.010019.
16. De Bleser L, Depreitere R, Waele KD, Vanhaecht K, Vlayen J, Sermeus W. Defining pathways. J Nurs Manage. (2006) 14:553–63. doi: 10.1111/j.1365-2934.2006.00702.x.
17. Dobesh PP, Bosso J, Wortman S, Dager WE, Karpiuk EL, Ma Q, et al. Critical pathways: the role of pharmacy today and tomorrow. Pharmacotherapy. (2006) 26:1358–68. doi: 10.1592/phco.26.9.1358.
18. Ersek JL, Nadler E, Freeman-Daily J, Mazharuddin S, Kim ES. Clinical pathways and the patient perspective in the pursuit of value-based oncology care. Am Soc Clin Oncol Educ Book. (2017) 37):597–606. doi: 10.1200/EDBK_174794.
19. Wind A, van der Linden C, Hartman E, Siesling S, van Harten W. Patient involvement in clinical pathway development, implementation and evaluation – A scoping review of international literature. Patient Educ Couns. (2022) 105:1441–8. doi: 10.1016/j.pec.2021.10.007.
21. Zander K. Integrated care pathways: eleven international trends. J integrated Care Pathways. (2002) 6:101–7. doi: 10.1177/147322970200600302.
22. Aspland E, Gartner D, Harper P. Clinical pathway modelling: a literature review. Health Syst (Basingstoke). (2019) 10:1–23. doi: 10.1080/20476965.2019.1652547.
23. Berger E, Brownwood I, Busse R, Cacace M, Cheng M, Daval A, et al. Improving healthcare quality in Europe. United Kingdom: World Health Organization and OECD (2019). doi: 10.1787/b11a6e8f-en
24. Kinsman L, Rotter T, James E, Snow P, Willis J. What is a clinical pathway? Development of a definition to inform the debate. BMC Med. (2010) 8:31. doi: 10.1186/1741-7015-8-31.
25. Richter P, Schlieter H. Understanding Patient Pathways in the Context of Integrated Health Care Services-Implications from a Scoping Review. In 14th International Conference on Wirtschaftsinformatik, February 24-27, 2019, Siegen, Germany. (2019).
26. Richter P, Hickmann E, Schlieter H. (2021). Validating the concept of patient pathways: A European survey on their characteristics, definition and state of practice, in: Twenty-fifth Pacific Asia Conference on Information Systems. Dubai: UAE. Available at: https://aisel.aisnet.org/pacis2021/32/.
27. Zander K. Nursing case management: strategic management of cost and quality outcomes. J Nurs Adm. (1988) 18:23–30. doi: 10.1016/S0029-6465(22)01407-4.
28. Rossiter DA, Edmondson A, Al-Shahi R, Thompson AJ. Integrated care pathways in multiple sclerosis rehabilitation: completing the audit cycle. Multiple Sclerosis J. (1998) 4:85–9. doi: 10.1191/135245898678919519.
29. Oreja-Guevara C, Miralles A, García-Caballero J, Noval S, Gabaldón L, Esteban-Vasallo MD, et al. Clinical pathways for the care of multiple sclerosis patients. Neurología (English Edition). (2010) 25:156–62. doi: 10.1016/S2173-5808(10)70031-6.
30. Smith L, Elwick H, Mhizha-Murira JR, Topcu G, Bale C, Evangelou N, et al. Developing a clinical pathway to identify and manage cognitive problems in Multiple Sclerosis: Qualitative findings from patients, family members, charity volunteers, clinicians and healthcare commissioners. Multiple Sclerosis Related Disord. (2021) 49:102563. doi: 10.1016/j.msard.2020.102563.
31. Zander K. Use of variance from clinical paths: coming of age. Clin Perform Qual Health Care. (1997) 5:20–30.
32. Du G, Huang L, Zhou M. Variance analysis and handling of clinical pathway: an overview of the state of knowledge. IEEE Access. (2020) 8:158208–23. doi: 10.1109/Access.6287639.
33. Petitta A. Assessing the value of pharmacists' health-systemwide services: clinical pathways and treatment guidelines. Pharmacotherapy. (2000) 20:327S–32S. doi: 10.1592/phco.20.16.327S.35006.
34. Acosta JN, Falcone GJ, Rajpurkar P, Topol EJ. Multimodal biomedical AI. Nat Med. (2022) 28:1773–84. doi: 10.1038/s41591-022-01981-2.
35. Raj Westwood A. Is hybrid telehealth model the next step for private healthcare in India? Health Serv Insights. (2021) 14:11786329211043301. doi: 10.1177/11786329211043301.
36. List R, Compton M, Soper M, Bruschwein H, Gettle L, Bailey M, et al. Preserving multidisciplinary care model and patient safety during reopening of ambulatory cystic fibrosis clinic for nonurgent care: A hybrid telehealth model. Telemedicine e-Health. (2020) 27:193–9. doi: 10.1089/tmj.2020.0247.
37. Scaglione T, Kuzbyt B. Tinnitus management: the utilization of a hybrid telehealth and in-person delivery model. Semin Hear. (2021) 42:115–22. doi: 10.1055/s-0041-1731692.
38. Chan SR, Torous J, Hinton L, Yellowlees P. Mobile tele-mental health: increasing applications and a move to hybrid models of care. Healthcare. (2014) 2:220–33. doi: 10.3390/healthcare2020220.
39. Amundson C, Marupuru S, Kliethermes MA, Axon DR, Warholak TL, Turgeon J, et al. Comprehensive medication management services provided via telehealth or hybrid models: A scoping review. JACCP: J Am Coll Of Clin Pharm. (2023) 6:53–72. doi: 10.1002/jac5.1742.
40. Bailey RB. Highlighting hybridization: a case report of virtual reality-augmented interventions to improve chronic post-stroke recovery. Medicine. (2022) 101:e29357. doi: 10.1097/MD.0000000000029357.
41. Xiang XM, Bernard J. Telehealth in multiple sclerosis clinical care and research. Curr Neurol Neurosci Rep. (2021) 21:14. doi: 10.1007/s11910-021-01103-4.
42. Maillart E, Labauge P, Cohen M, Maarouf A, Vukusic S, Donzé C, et al. MSCopilot, a new multiple sclerosis self-assessment digital solution: results of a comparative study versus standard tests. Eur J Neurol. (2020) 27:429–36. doi: 10.1111/ene.14091.
43. van Oirschot P, Heerings M, Wendrich K, den Teuling B, Martens MB, Jongen PJ. Symbol digit modalities test variant in a smartphone app for persons with multiple sclerosis: validation study. JMIR Mhealth Uhealth. (2020) 8:e18160. doi: 10.2196/18160.
44. Klucken J, Krüger R, Schmidt P, Bloem BR. Management of Parkinson’s disease 20 years from now: towards digital health pathways. J Parkinson's Dis. (2018) 8:S85–94. doi: 10.3233/JPD-181519.
45. Liljamo P, Sailynoja H, Tuomikoski K, Henner A, Koivunen K. Patient-centered development of a digital care pathway for arrhythmia patients. Stud Health Technol Inform. (2020) 275:127–31. doi: 10.3233/SHTI200708
46. Ponder M, Ansah-Yeboah AA, Charalambous LT, Adil SM, Venkatraman V, Abd-El-Barr M, et al. A smartphone app with a digital care pathway for patients undergoing spine surgery: development and feasibility study. JMIR Perioper Med. (2020) 3:e21138. doi: 10.2196/21138.
47. Liljamo P, Wahlberg J, Mikkonen H, Reponen J. A digital care pathway to access healthcare without time and place restrictions. Stud Health Technol Inform. (2021) 284:163–5. doi: 10.3233/SHTI210690
48. Heijsters F, Santema J, Mullender M, Bouman M-B, Bruijne Md, van Nassau F. Stakeholders barriers and facilitators for the implementation of a personalised digital care pathway: a qualitative study. BMJ Open. (2022) 12:e065778. doi: 10.1136/bmjopen-2022-065778.
49. Torr B, Jones C, Choi S, Allen S, Kavanaugh G, Hamill M, et al. A digital pathway for genetic testing in UK NHS patients with cancer: BRCA-DIRECT randomised study internal pilot. J Med Genet. (2022) 59:1179–88. doi: 10.1136/jmg-2022-108655.
50. Turk E, Wontor V, Vera-Munoz C, Comnes L, Rodrigues N, Ferrari G, et al. Human-centered integrated care pathways for co-creating a digital, user-centric health information solution. J Integr Care. (2022) 30:296–309. doi: 10.1108/JICA-01-2022-0007.
51. Connell A, Raine R, Martin P, Barbosa EC, Morris S, Nightingale C, et al. Implementation of a digitally enabled care pathway (Part 1): impact on clinical outcomes and associated health care costs. J Med Internet Res. (2019) 21:e13147. doi: 10.2196/13147.
52. Connell A, Montgomery H, Morris S, Nightingale C, Stanley S, Emerson M, et al. Service evaluation of the implementation of a digitally-enabled care pathway for the recognition and management of acute kidney injury. F1000Res. (2017) 6:1033. doi: 10.12688/f1000research.
53. Connell A, Black G, Montgomery H, Martin P, Nightingale C, King D, et al. Implementation of a digitally enabled care pathway (Part 2): qualitative analysis of experiences of health care professionals. J Med Internet Res. (2019) 21:e13143. doi: 10.2196/13143.
54. Alahmar A, Benlamri R. (2020). Optimizing Hospital Resources using Big Data Analytics with Standardized e-Clinical Pathways, in: 2020 IEEE Intl Conf on Dependable, Autonomic and Secure Computing, Intl Conf on Pervasive Intelligence and Computing, Intl Conf on Cloud and Big Data Computing, Intl Conf on Cyber Science and Technology Congress (DASC/PiCom/CBDCom/CyberSciTech), , 2020 17-22 Aug. doi: 10.1109/DASC/PiCom/CBDCom/Cy49142.2020.
55. Han S, Ma L. Data-driven integrated care pathways: Standardization of delivering patient-centered care. Front Med. (2022) 9. doi: 10.3389/fmed.2022.883874.
56. Hipp R, Abel E, Weber RJ. A primer on clinical pathways. Hosp Pharm. (2016) 51:416–21. doi: 10.1310/hpj5105-416.
57. Awad A, Trenfield SJ, Pollard TD, Ong JJ, Elbadawi M, McCoubrey LE, et al. Connected healthcare: Improving patient care using digital health technologies. Adv Drug Deliv Rev. (2021) 178:113958. doi: 10.1016/j.addr.2021.113958.
58. Stoumpos AI, Kitsios F, Talias MA. Digital transformation in healthcare: technology acceptance and its applications. Int J Environ Res Public Health. (2023) 20. doi: 10.3390/ijerph20043407.
59. MacMahon ST, Richardson I. Pathways, technology and the patient—connected health through the lifecycle. Front Digital Health. (2023) 5. doi: 10.3389/fdgth.2023.1057518.
60. Garate FJ, Chausa P, Whetham J, Jones CI, Garcia F, Caceres C, et al. EmERGE mHealth platform: implementation and technical evaluation of a digital supported pathway of care for medically stable HIV. Int J Environ Res Public Health. (2021) 18. doi: 10.3390/ijerph18063156.
61. Organisation for Economic Co-operation and Development. Integrating Care to Prevent and Manage Chronic Diseases. Paris: OECD Publishing (2023). doi: 10.1787/9acc1b1d-en
62. Lekadir K, Quagli G, Garmendia AT, Gallin C. Artificial intelligence in healthcare : Applications, risks, and ethical and societal impacts. Brussels: European Parliament (2022).
63. Khan P, Kader MF, Islam SMR, Rahman AB, Kamal MS, Toha MU, et al. Machine learning and deep learning approaches for brain disease diagnosis: principles and recent advances. IEEE Access. (2021) 9:37622–55. doi: 10.1109/Access.6287639.
64. Sarker IH. Deep learning: A comprehensive overview on techniques, taxonomy, applications and research directions. SN Comput Sci. (2021) 2. doi: 10.1007/s42979-021-00815-1.
65. Schlieter H, Benedict M, Gand K, Burwitz M. (2017). Towards adaptive pathways: reference architecture for personalized dynamic pathways, in: 2017 IEEE 19th Conference on Business Informatics (CBI), , 24-27 July 2017. doi: 10.1109/CBI.2017.55.
66. Scheplitz T, Burwitz M, Weimann T. PathwAI systems in healthcare - a framework for coupling AI and pathway-based health information systems. (2022), 1–10.
67. Klineova S, Lublin FD. Clinical course of multiple sclerosis. Cold Spring Harb Perspect Med. (2018) 8. doi: 10.1101/cshperspect.a028928.
68. Schwenkenbecher P, Wurster U, Konen FF, Gingele S, Sühs K-W, Wattjes MP, et al. Impact of the mcDonald criteria 2017 on early diagnosis of relapsing-remitting multiple sclerosis. Front Neurol. (2019) 10. doi: 10.3389/fneur.2019.00188.
69. McNicholas N, Lockhart A, Yap SM, O’Connell K, Tubridy N, Hutchinson M, et al. New versus old: Implications of evolving diagnostic criteria for relapsingâ“remitting multiple sclerosis. Multiple Sclerosis J. (2019) 25(6):867–70. doi: 10.1177/1352458518770088.
70. Jakimovski D, Kavak KS, Zakalik K, Coetzee T, Gottesman M, Coyle PK, et al. Improvement in time to multiple sclerosis diagnosis: 25-year retrospective analysis from New York State MS Consortium (NYSMSC). Multiple Sclerosis J. (2022) 0:1352–4585. doi: 10.1177/13524585221140271.
71. Blaschke SJ, Ellenberger D, Flachenecker P, Hellwig K, Paul F, Pöhlau D, et al. Time to diagnosis in multiple sclerosis: Epidemiological data from the German Multiple Sclerosis Registry. Multiple Sclerosis J. (2022) 28:865–71. doi: 10.1177/13524585211039753.
72. Hartung H-P, Graf J, Aktas O, Mares J, Barnett MH. Diagnosis of multiple sclerosis: revisions of the McDonald criteria 2017 – continuity and change. Curr Opin Neurol. (2019) 32:327–37. doi: 10.1097/WCO.0000000000000699.
73. Ford H. Clinical presentation and diagnosis of multiple sclerosis. Clin Med. (2020) 20:380–3. doi: 10.7861/clinmed.2020-0292.
74. Patti F, Chisari CG, Arena S, Toscano S, Finocchiaro C, Fermo SL, et al. Factors driving delayed time to multiple sclerosis diagnosis: Results from a population-based study. Multiple Sclerosis Related Disord. (2022) 57:103361. doi: 10.1016/j.msard.2021.103361.
75. Aires A, Barros A, MaChado C, Fitas D, Cação G, Pedrosa R, et al. Diagnostic delay of multiple sclerosis in a Portuguese population. Acta Médica Portuguesa. (2019) 32:289–94. doi: 10.20344/amp.11187.
76. Kaufmann M, Kuhle J, Puhan MA, Kamm CP, Chan A, Salmen A, et al. Factors associated with time from first-symptoms to diagnosis and treatment initiation of Multiple Sclerosis in Switzerland. Mult Scler J Exp Transl Clin. (2018) 4:2055217318814562. doi: 10.1177/2055217318814562.
77. Solomon AJ, Bourdette DN, Cross AH, Applebee A, Skidd PM, Howard DB, et al. The contemporary spectrum of multiple sclerosis misdiagnosis. A Multicenter Study. (2016) 87:1393–9. doi: 10.1212/WNL.0000000000003152.
78. Brownlee WJ, Solomon AJ. Misdiagnosis of multiple sclerosis: Time for action. Multiple Sclerosis J. (2021) 27:805–6. doi: 10.1177/13524585211005367.
79. Kaisey M, Solomon AJ, Luu M, Giesser BS, Sicotte NL. Incidence of multiple sclerosis misdiagnosis in referrals to two academic centers. Multiple Sclerosis Related Disord. (2019) 30:51–6. doi: 10.1016/j.msard.2019.01.048.
80. Giovannoni G, Butzkueven H, Dhib-Jalbut S, Hobart J, Kobelt G, Pepper G, et al. Brain health: time matters in multiple sclerosis. Multiple Sclerosis Related Disord. (2016) 9:S5–S48. doi: 10.1016/j.msard.2016.07.003.
81. Hobart J, Butzkueven H, Haartsen J, Ziemssen T, Lane T, Giovannoni G. Timely intervention, monitoring and education MATTERS in MS (TIME MATTERS in MS): Development of a globally applicable quality improvement tool. Multiple Sclerosis J Exp Trans Clin. (2022) 8:20552173221124023. doi: 10.1177/20552173221124023.
82. Soelberg Sorensen P, Giovannoni G, Montalban X, Thalheim C, Zaratin P, Comi G. The multiple sclerosis care unit. Multiple Sclerosis J. (2019) 25:627–36. doi: 10.1177/1352458518807082.
83. Giovannoni GB, Peer, Butzkueven H, Campbell T, Drulovic J, Haartsen J, et al. MS Brain Health: Oxford Health Policy Forum (2021). Available online at: https://www.msbrainhealth.org/.
84. Hemmer BB, Antonios, Berthele A, Domurath B, Faßhauer E, Flachenecker P, et al. Diagnose und Therapie der Multiplen Sklerose, Neuromyelitis-optica-Spektrum-Erkrankungen und MOG-IgG-assoziierten Erkrankungen. Deutsche Gesellschaft für Neurologie: S2k-Leitlinie (2023).
85. Aktas O, Kleiter I, Kümpfel T, Trebst C. Qualitätshandbuch MS / NMOSD : Empfehlungen zur Therapie der Multiplen Sklerose / Neuromyelitis-optica-Spektrum-Erkrankungen für Ärzte. 6 ed. Münster: Krankheitsbezogenes Kompetenznetz Multiple Sklerose e. V (2021).
86. Tullman MJ. Overview of the epidemiology, diagnosis, and disease progression associated with multiple sclerosis. Am J Manag Care. (2013) 19:S15–20.
87. Lunde HMB, Assmus J, Myhr KM, Bø L, Grytten N. Survival and cause of death in multiple sclerosis: a 60-year longitudinal population study. J Neurol Neurosurg Psychiatry. (2017) 88:621–5. doi: 10.1136/jnnp-2016-315238.
88. Marrie RA, Elliott L, Marriott J, Cossoy M, Blanchard J, Leung S, et al. Effect of comorbidity on mortality in multiple sclerosis. Neurology. (2015) 85:240–7. doi: 10.1212/WNL.0000000000001718.
89. Soenksen LR, Ma Y, Zeng C, Boussioux L, Villalobos Carballo K, Na L, et al. Integrated multimodal artificial intelligence framework for healthcare applications. NPJ Digital Med. (2022) 5:149. doi: 10.1038/s41746-022-00689-4.
90. Myszczynska MA, Ojamies PN, Lacoste AMB, Neil D, Saffari A, Mead R, et al. Applications of machine learning to diagnosis and treatment of neurodegenerative diseases. Nat Rev Neurol. (2020) 16:440–56. doi: 10.1038/s41582-020-0377-8.
91. Leslie A, Jones AJ, Goddard PR. The influence of clinical information on the reporting of CT by radiologists. Br J Radiol. (2000) 73:1052–5. doi: 10.1259/bjr.73.874.11271897.
92. Maizlin NN, Somers S. The role of clinical history collected by diagnostic imaging staff in interpreting of imaging examinations. J Med Imaging Radiat Sci. (2019) 50:31–5. doi: 10.1016/j.jmir.2018.07.009.
93. Boonn WW, Langlotz CP. Radiologist use of and perceived need for patient data access. J Digital Imaging. (2009) 22:357–62. doi: 10.1007/s10278-008-9115-2.
94. Raab SS, Oweity T, Hughes JH, Salomao DR, Kelley CM, Flynn CM, et al. Effect of clinical history on diagnostic accuracy in the cytologic interpretation of bronchial brush specimens. Am J Clin Pathol. (2000) 114:78–83. doi: 10.1309/4099-QALD-NVGF-TM4G.
95. Huang S-C, Pareek A, Seyyedi S, Banerjee I, Lungren MP. Fusion of medical imaging and electronic health records using deep learning: a systematic review and implementation guidelines. NPJ Digital Med. (2020) 3:136. doi: 10.1038/s41746-020-00341-z
96. Meijboom B, Vaessen P, van der Heijden K, Van Sambeeck M, Gemmel P eds. (2018). Customization and personalization in clinical pathways using a modular perspective, in: 25th Annual Euroma Conference, Budapest, Hungary.
97. Van Dam A, Metz M, Meijboom B. Improving customisation in clinical pathways by using a modular perspective. Int J Environ Res Public Health. (2021) 18:11129. doi: 10.3390/ijerph182111129.
98. de Blok C, Meijboom B, Luijkx K, Schols J, Schroeder R. Interfaces in service modularity: A typology developed in modular health care provision. J Operations Manage. (2014) 32:175–89. doi: 10.1016/j.jom.2014.03.001.
99. Silander K, Torkki P, Peltokorpi A, Lepäntalo A, Tarkkanen M, Bono P, et al. Modularising outpatient care delivery: A mixed methods case study at a Finnish University Hospital. Health Serv Manage Res. (2018) 31:195–204. doi: 10.1177/0951484817752629.
100. Peters C, Richter P. (2023). Individualizing patient pathways through modularization: design and evaluation of healthcare-specific modularization parameters, in: Conference: Hawaii International Conference on System Sciences, Hawaii.
101. Voigt I, Inojosa H, Wenk J, Akgün K, Ziemssen T. Building a monitoring matrix for the management of multiple sclerosis. Autoimmun Rev. (2023) 22:103358. doi: 10.1016/j.autrev.2023.103358.
102. Peters C. Modularization of Services: A Modularization Method for the Field of Telemedicine. Kassel: Kassel University Press (2016). doi: 10.19211/KUP9783737600279
103. Ingvar M, Blom MC, Winsnes C, Robinson G, Vanfleteren L, Huff S. On the annotation of health care pathways to allow the application of care-plans that generate data for multiple purposes. Front Digital Health. (2021) 3. doi: 10.3389/fdgth.2021.688218.
104. De Roock E, Martin N. Process mining in healthcare – An updated perspective on the state of the art. J Biomed Inf. (2022) 127:103995. doi: 10.1016/j.jbi.2022.103995.
105. Munoz-Gama J, Martin N, Fernandez-Llatas C, Johnson OA, Sepúlveda M, Helm E, et al. Process mining for healthcare: Characteristics and challenges. J Biomed Inf. (2022) 127:103994. doi: 10.1016/j.jbi.2022.103994
106. Guzzo A, Rullo A, Vocaturo E. Process mining applications in the healthcare domain: A comprehensive review. WIREs Data Min Knowledge Discovery. (2022) 12:e1442. doi: 10.1002/widm.1442.
107. Pruenza C, Díaz J, Solano MT, Arroyo R, Izquierdo G. Model for prediction of progression in multiple sclerosis. Int J Interactive Multimedia Artif Intell. (2019) 5:48–53. doi: 10.9781/ijimai.2019.06.005.
108. Eshaghi A, Young AL, Wijeratne PA, Prados F, Arnold DL, Narayanan S, et al. Identifying multiple sclerosis subtypes using unsupervised machine learning and MRI data. Nat Commun. (2021) 12:2078. doi: 10.1038/s41467-021-22265-2.
109. Pontillo G, Penna S, Cocozza S, Quarantelli M, Gravina M, Lanzillo R, et al. Stratification of multiple sclerosis patients using unsupervised machine learning: a single-visit MRI-driven approach. Eur Radiol. (2022) 32:5382–91. doi: 10.1007/s00330-022-08610-z.
110. Popova M, Isayev O, Tropsha A. Deep reinforcement learning for de novo drug design. Sci Adv. (2018) 4:eaap7885. doi: 10.1126/sciadv.aap7885.
111. Liu M, Shen X, Pan W. Deep reinforcement learning for personalized treatment recommendation. Stat Med. (2022) 41:4034–56. doi: 10.1002/sim.9491.
112. Zhang Z. Reinforcement learning in clinical medicine: a method to optimize dynamic treatment regime over time. Ann Trans Med. (2019) 7:345–. doi: 10.21037/atm.
113. Noll R, Schaaf J, Storf H. The Use of Computer-Assisted Case-Based Reasoning to Support Clinical Decision-Making – A Scoping Review. Cham: Springer International Publishing (2022) p. 395–409. doi: 10.1007/978-3-031-14923-8_26
114. Sadoughi F, Arani LA. 5 - Intelligent computer systems for multiple sclerosis diagnosis. In: Pillai AS, Menon B, editors. Augmenting Neurological Disorder Prediction and Rehabilitation Using Artificial Intelligence. Academic Press (2022). p. 75–105. doi: 10.1016/B978-0-323-90037-9.00018-7
115. Alshamrani R, Althbiti A, Alshamrani Y, Alkomah F, Ma X. Model-driven decision making in multiple sclerosis research: existing works and latest trends. Patterns. (2020) 1:100121. doi: 10.1016/j.patter.2020.100121.
116. Afzal M, Riazul Islam SM, Hussain M, Lee S. Precision medicine informatics: principles, prospects, and challenges. IEEE Access. (2020) 8:13593–612. doi: 10.1109/Access.6287639.
117. Ziemssen T, Vandercappellen J, Jordan Mondragon V, Giovannoni G. MSProDiscuss™ Clinical decision support tool for identifying multiple sclerosis progression. J Clin Med. (2022) 11:4401. doi: 10.3390/jcm11154401.
118. Lublin FD. New multiple sclerosis phenotypic classification. Eur Neurol. (2014) 72:1–5. doi: 10.1159/000367614.
119. Lublin FD, Reingold SC, Cohen JA, Cutter GR, Sørensen PS, Thompson AJ, et al. Defining the clinical course of multiple sclerosis: the 2013 revisions. Neurology. (2014) 83:278–86. doi: 10.1212/WNL.0000000000000560.
120. Anderson GP. Endotyping asthma: new insights into key pathogenic mechanisms in a complex, heterogeneous disease. Lancet. (2008) 372:1107–19. doi: 10.1016/S0140-6736(08)61452-X.
121. Thyssen JP. Subtyping, phenotyping or endotyping rosacea: how can we improve disease understanding and patient care? Br J Dermatol. (2018) 179:551–2. doi: 10.1111/bjd.16942.
122. Lötvall J, Akdis CA, Bacharier LB, Bjermer L, Casale TB, Custovic A, et al. Asthma endotypes: a new approach to classification of disease entities within the asthma syndrome. J Allergy Clin Immunol. (2011) 127:355–60. doi: 10.1016/j.jaci.2010.11.037
123. Agache I, Akdis CA. Precision medicine and phenotypes, endotypes, genotypes, regiotypes, and theratypes of allergic diseases. J Clin Invest. (2019) 129:1493–503. doi: 10.1172/JCI124611.
124. Agache I, Akdis CA. Endotypes of allergic diseases and asthma: An important step in building blocks for the future of precision medicine. Allergol Int. (2016) 65:243–52. doi: 10.1016/j.alit.2016.04.011.
125. Maggi E, Parronchi P, Azzarone BG, Moretta L. A pathogenic integrated view explaining the different endotypes of asthma and allergic disorders. Allergy. (2022) 77:3171–479. doi: 10.1111/all.15445.
126. Mersha TB, Afanador Y, Johansson E, Proper SP, Bernstein JA, Rothenberg ME, et al. Resolving clinical phenotypes into endotypes in allergy: molecular and omics approaches. Clin Rev Allergy Immunol. (2020) 60:200–19. doi: 10.1007/s12016-020-08787-5.
127. Azad TD, Shah PP, Kim HB, Stevens RD. Endotypes and the path to precision in moderate and severe traumatic brain injury. Neurocritical Care. (2022) 37:259–66. doi: 10.1007/s12028-022-01475-6.
128. Nogales C, Mamdouh ZM, List M, Kiel C, Casas AI, Schmidt HHHW. Network pharmacology: curing causal mechanisms instead of treating symptoms. Trends Pharmacol Sci. (2022) 43:136–50. doi: 10.1016/j.tips.2021.11.004.
129. Manouchehri N, Zhang Y, Salter A, Hussain R, Hartung H, Hemmer B, et al. Clinical trials in multiple sclerosis: potential future trial designs. Ther Adv neurological Disord. (2019) 12:1756286419847095. doi: 10.1177/1756286419847095.
130. Akgün K, Kretschmann N, Haase R, Proschmann U, Kitzler HH, Reichmann H, et al. Profiling individual clinical responses by high-frequency serum neurofilament assessment in MS. Neurol Neuroimmunology Neuroinflamm. (2019) 6:e555. doi: 10.1212/NXI.0000000000000555.
131. Kennedy KE, Rosbo N, Uccelli A, Cellerino M, Ivaldi F, Contini P, et al. Multiscale networks in multiple sclerosis. bioRxiv. (2023). doi: 10.1101/2023.02.26.530153.
132. Paul M, Maglaras L, Ferrag MA, Almomani I. Digitization of healthcare sector: A study on privacy and security concerns. ICT Express. (2023) 9:571–88. doi: 10.1016/j.icte.2023.02.007.
133. Ali S, Akhlaq F, Imran AS, Kastrati Z, Daudpota SM, Moosa M. The enlightening role of explainable artificial intelligence in medical & healthcare domains: A systematic literature review. Comput Biol Med. (2023) 166:107555. doi: 10.1016/j.compbiomed.2023.107555
134. Ueda D, Kakinuma T, Fujita S, Kamagata K, Fushimi Y, Ito R, et al. Fairness of artificial intelligence in healthcare: review and recommendations. Japanese J Radiol. (2024) 42:3–15. doi: 10.1007/s11604-023-01474-3.
135. Farah L, Murris JM, Borget I, Guilloux A, Martelli NM, Katsahian SIM. Assessment of performance, interpretability, and explainability in artificial intelligence–based health technologies: what healthcare stakeholders need to know. Mayo Clinic Proceedings: Digital Health. (2023) 1:120–38. doi: 10.1016/j.mcpdig.2023.02.004.
136. Balch JA, Ruppert MM, Loftus TJ, Guan Z, Ren Y, Upchurch GR, et al. Machine learning–enabled clinical information systems using fast healthcare interoperability resources data standards: scoping review. JMIR Med Inform. (2023) 11:e48297. doi: 10.2196/48297.
137. Villarreal ERD, García-Alonso J, Moguel E, Alegría JAH. Blockchain for healthcare management systems: A survey on interoperability and security. IEEE Access. (2023) 11:5629–52. doi: 10.1109/ACCESS.2023.3236505.
138. Torab-Miandoab A, Samad-Soltani T, Jodati A, Rezaei-Hachesu P. Interoperability of heterogeneous health information systems: a systematic literature review. BMC Med Inf Decision Making. (2023) 23:18. doi: 10.1186/s12911-023-02115-5.
139. Sutton RT, Pincock D, Baumgart DC, Sadowski DC, Fedorak RN, Kroeker KI. An overview of clinical decision support systems: benefits, risks, and strategies for success. NPJ Digital Med. (2020) 3:17. doi: 10.1038/s41746-020-0221-y.
140. Heitmann KU, Grode A, Schenk A, Ihls A, Becker K. Interoperabilität 2025 Teil A: Voraussetzungen für ein interoperables Gesundheitswesen schaffen2020 23.02.2023. Available online at: https://www.bitkom.org/sites/default/files/2020-08/interoperabilitat-2025-teil-a-v15.pdf.
141. gematik GmbH. Medizinische Informationsobjekte: Interoperabilität dank einheitlicher Datenformate Berlin. Berlin: gematik GmbH (2023). Available at: https://www.ina.gematik.de/themenbereiche/medizinische-informationsobjekte.
142. gematik GmbH. Digital Health und Interoperabilität in Deutschland: Auf Basis gemeinsamer Standards Berlin. Berlin: gematik GmbH (2023). Available at: https://www.ina.gematik.de/themenbereiche/digital-health-und-interoperabilitaet-in-deutschland.
143. Gesundheits-IT-Interoperabilität-Governance-Verordnung (IOP-Governance-Verordnung – GIGV). Sect. Teil I. Cologne: Bundesanzeiger Verlag GmbH (2021).
Keywords: multiple sclerosis, patient pathway, clinical pathway, digital pathway, artificial intelligence, digital health, connected health, digital twin
Citation: Wenk J, Voigt I, Inojosa H, Schlieter H and Ziemssen T (2024) Building digital patient pathways for the management and treatment of multiple sclerosis. Front. Immunol. 15:1356436. doi: 10.3389/fimmu.2024.1356436
Received: 15 December 2023; Accepted: 30 January 2024;
Published: 15 February 2024.
Edited by:
Gloria Dalla Costa, San Raffaele Scientific Institute (IRCCS), ItalyReviewed by:
Iván Murrieta-Álvarez, Baylor College of Medicine, United StatesYolanda Aladro, European University of Madrid, Spain
Copyright © 2024 Wenk, Voigt, Inojosa, Schlieter and Ziemssen. This is an open-access article distributed under the terms of the Creative Commons Attribution License (CC BY). The use, distribution or reproduction in other forums is permitted, provided the original author(s) and the copyright owner(s) are credited and that the original publication in this journal is cited, in accordance with accepted academic practice. No use, distribution or reproduction is permitted which does not comply with these terms.
*Correspondence: Tjalf Ziemssen, dGphbGYuemllbXNzZW5AdW5pa2xpbmlrdW0tZHJlc2Rlbi5kZQ==