- Centre for Malaria Elimination, Institute of Tropical Medicine, Mount Kenya University, Thika, Kenya
Human malaria, caused by five Plasmodium species (P. falciparum, P. vivax, P. malariae, P. ovale, and P. knowlesi), remains a significant global health burden. While most interventions target P. falciparum, the species associated with high mortality rates and severe clinical symptoms, non-falciparum species exhibit different transmission dynamics, remain hugely neglected, and pose a significant challenge to malaria elimination efforts. Recent studies have reported the presence of antigens associated with cross-protective immunity, which can potentially disrupt the transmission of various Plasmodium species. With the sequencing of the Plasmodium genome and the development of immunoinformatic tools, in this study, we sought to exploit the evolutionary history of Plasmodium species to identify conserved cross-species B-cell linear epitopes in merozoite proteins. We retrieved Plasmodium proteomes associated with human malaria and applied a subtractive proteomics approach focusing on merozoite stage proteins. Bepipred 2.0 and Epidope were used to predict B-cell linear epitopes using P. falciparum as the reference species. The predictions were further compared against human and non-falciparum databases and their antigenicity, toxicity, and allergenicity assessed. Subsequently, epitope conservation was carried out using locally sequenced P. falciparum isolates from a malaria-endemic region in western Kenya (n=27) and Kenyan isolates from MalariaGEN version 6 (n=131). Finally, physiochemical characteristics and tertiary structure of the B-cell linear epitopes were determined. The analysis revealed eight epitopes that showed high similarity (70-100%) between falciparum and non-falciparum species. These epitopes were highly conserved when assessed across local isolates and those from the MalariaGEN database and showed desirable physiochemical properties. Our results show the presence of conserved cross-species B-cell linear epitopes that could aid in targeting multiple Plasmodium species. Nevertheless, validating their efficacy in-vitro and in-vivo experimentally is essential.
Introduction
Malaria remains a significant health burden in Sub-Saharan Africa, especially for children under five years and pregnant women. Among the five Plasmodium species (P. falciparum, P. vivax, P. ovale, P. malariae, and the zoonotic P. knowlesi) that cause human malaria, P. falciparum accounts for the highest number of malaria cases globally while P. vivax shows a broader geographical distribution (1, 2). While significant progress has been made in malaria control efforts over the past two decades, the focus is primarily on P. falciparum due to its clinical severity. Despite evidence of different transmission patterns of P. falciparum, less attention is paid to non-falciparum species. For instance, despite a decline in P. falciparum malaria cases in Tanzania, persistent transmission of P. ovale and P. malariae between 2010 and 2016 continued to account for the increased number of malaria cases (3). Similarly, P. knowlesi emerged as the primary cause of malaria in Sabah Malaysia after a significant decline in cases due to P. vivax and P. falciparum infections (4, 5). The differences in transmission in non-falciparum species may explained by the presence of hypnozoite stages (6), differing seasonality and asymptomatic infections (7), and differences in gametocytogenesis (8). To successfully eliminate malaria, it is crucial to implement strategies targeting all Plasmodium species linked with human malaria to improve the health outcomes of vulnerable populations globally.
The evolutionary relationship between Plasmodium species is a valuable resource for developing interventions targeting multiple species. This approach has already been exploited to identify potential vaccine targets for P. vivax from P. falciparum homologs (9). Since Plasmodium species emerged and evolved from a common ancestor, the structure and function of some proteins remained similar, increasing the possibility of homologous proteins recognizing similar B-cell linear epitopes during infections. Existing evidence already points out cross-reactivity between homologous proteins in Plasmodium. For example, recombinant P. vivax apical asparagine (Asn)-rich protein (PvAARP) was strongly recognized by P. knowlesi patient sera, cross-reacted with the apical side of the P. knowlesi merozoites and inhibited erythrocyte invasion by P. knowlesi with the mechanism linked to similar B-cell epitopes in both species (10). Additionally, functionally, non-reciprocal cross-species immunity between P. vivax Duffy Binding Protein (PvDBP), a protein mediating reticulocyte invasion, and P. falciparum VAR2CSA, a protein involved in parasite sequestration in the placenta has been observed (11, 12). VAR2CSA and PvDBP are not functionally related but share a Duffy binding-like domain, with cross-reactive epitopes in P. vivax blocking the adhesion of VAR2CSA to its placental receptor, chondroitin Sulfate A (11, 12). Antibodies arising from PvDBP cross react with the DBL3X region of VAR2CSA through a 34 amino acid conserved region and elicit adhesion binding antibodies against CSA, suggesting their role as a potential vaccine candidate (13). Thus, by leveraging the evolutionary relationship and shared epitopes among Plasmodium species, interventions that provide broad protection against different malaria-causing parasites can be developed, thereby contributing to global efforts aimed at malaria elimination.
The sequencing of the Plasmodium genomes in the post-genomic era has greatly accelerated the discovery of potential vaccine candidates. When coupled with subtractive proteomics, which seeks to subtract pathogen targets needed for the organism’s survival but not in the host, and immuno-informatics, which predicts the presence of epitopes, this reduces the time required to identify antigen targets for experimental validation. Herein, we utilized a subtractive proteomics and immunoinformatic approach to identify homologous proteins present in merozoites in P. falciparum. Cross-species B-cell linear epitopes sharing high similarity with P. falciparum were identified, and their conservation was assessed using locally sequenced isolates from a malaria endemic region and those deposited in MalariaGEN version 6. We identified eight conserved cross-species B-cell linear epitopes that need further assessment as potential vaccine targets that can be developed for falciparum and non-falciparum malaria as part of the consented efforts to control and eliminate the scourge.
Materials and methods
Removal of redundant proteins
The workflow applied in identification of conserved B-cell linear epitopes is summarized in Figure 1. Initially, the latest complete proteome sequences for P. falciparum 3D7, P. vivax P01, P. malariae UG01, P. ovale GH01, P. knowlesi Strain H were downloaded from PlasmoDB version 63, and the human proteome from NCBI. CD-HIT v4.8.1 was used to remove redundant protein sequences using a threshold of 90% (14). After that, the P. falciparum proteome was used as the reference for downstream analysis.
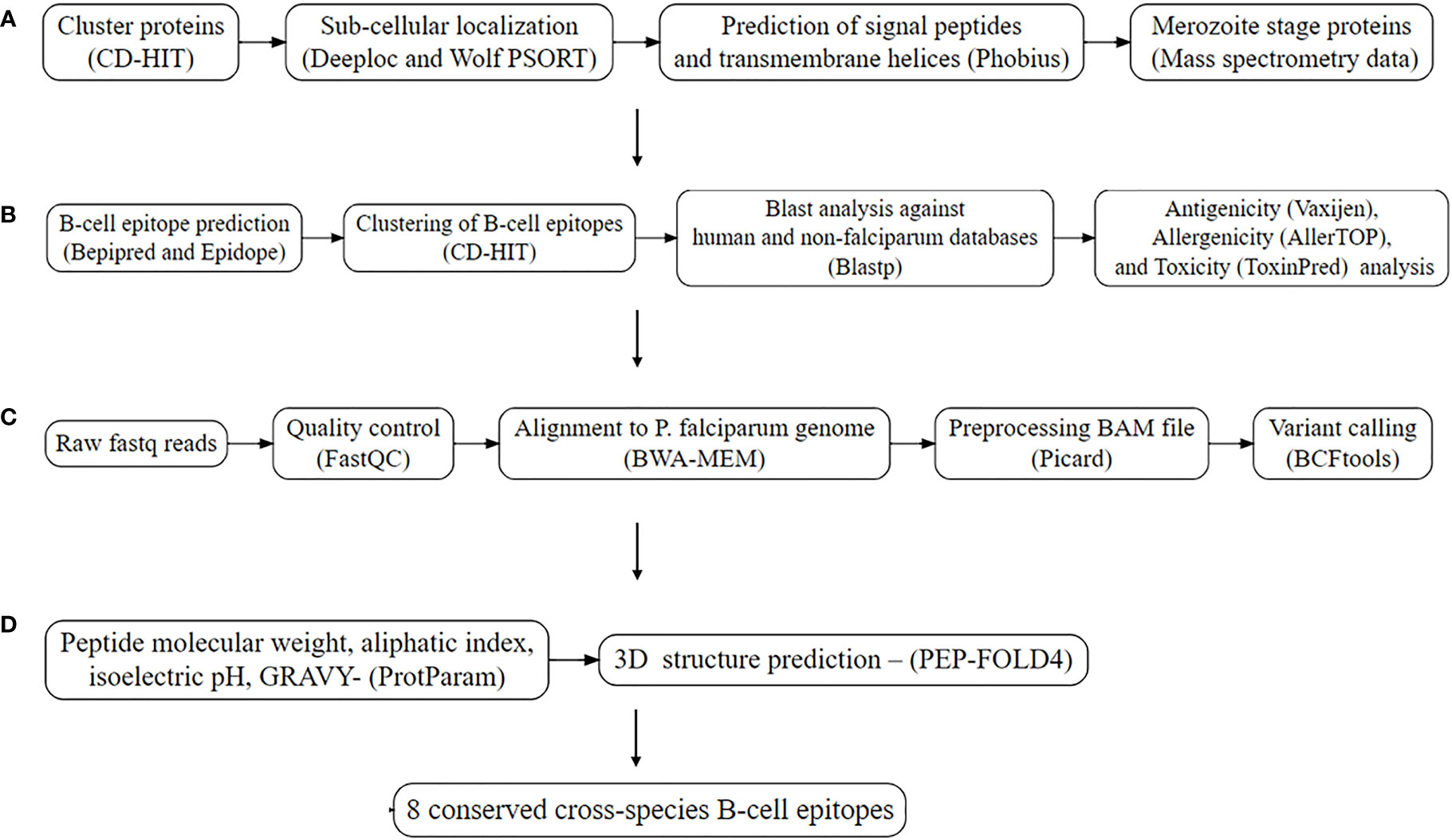
Figure 1 Workflow utilized for the prediction of conserved B-cell linear epitopes. (A). Subtractive proteomics. (B) Epitope prediction and analysis. (C) Conservation analysis from me Conservation analysis from merozoite protein from local Kenyan isolates (n=27) and MalariaGEN (n=131). (D) Structural and physicochemical properties.
Subcellular localization and removal of intracellular proteins
WoLF PSORT (https://wolfpsort.hgc.jp/) and Deeploc(https://services.healthtech.dtu.dk/services/DeepLoc-2.0/) were employed to predict the subcellular location of the selected proteins. In WoLF PSORT, amino acid sequences are converted to numerical localization features based on their sorting signals, functional motifs, and amino acid composition. Then, the nearest neighbor classifier is used to predict the location of proteins in 10 different sites (15). Deeploc predicts utilizing a deep learning approach by using datasets from eukaryotic and human proteins (16). Intracellular proteins, such as those in the cytoplasm and nucleus, were excluded from further analysis. However, proteins present in P. falciparum-specific membrane-bound organelles such as rhoptries or micronemes cannot be accurately classified by WoLF PSORT and Deeploc since these organelles are absent from their predictions. To cater to proteins in the rhoptries and micronemes, membrane-bound organelles including those predicted to be present in mitochondria, golgi apparatus, lysosome, vacuoles, peroxisome and plastid were included for analysis. Afterward, proteins found in intracellular organelles such as the mitochondria, ribosomes, and apicoplast were removed using specific keywords in their protein names. Transcription, translation, RNA, and DNA binding proteins were eliminated using the same approach. Lastly, enzymes were also excluded from the analysis.
Prediction of surface proteins
The presence of a signal peptide and transmembrane helix was assessed to narrow down the proteins of interest. Signal peptides (SPs) are short peptide sequences majorly present on the N-terminus of protein sequences destined towards secretory cells or on the cell membrane. At the same time, transmembrane helices are membrane-bound proteins that play various roles, including anchoring transmembrane proteins to the cell membrane (17, 18). Phobius (https://phobius.sbc.su.se/) was used to predict the presence of a signal peptide and transmembrane helix. Phobius utilizes hidden Markov models with sub-models capable of differentiating between signal peptides and transmembrane helices (19). Proteins with either one signal peptide or transmembrane helix were selected for further analysis.
Merozoite protein selection
Malaria infections begin when sporozoites are injected into the bloodstream through a bite of an infected female Anopheles mosquito. The sporozoites migrate to the liver where they infect hepatocytes, and within 7-10 days, the parasite develops to become merozoites which are released into the bloodstream to infect red blood cells, initiating the intra-erythrocytic stage of the disease. The replication cycle in the blood stage takes approximately 24 hours in P. knowlesi, 48 hours in P. falciparum, P. ovale, and P. vivax, and 72 hours in P. malariae (20). Merozoite surface or apical organelle proteins represent valuable targets for vaccine development due to their exposure to the host immune system. Additionally, these proteins play a crucial role in invading red blood cells. Antibodies to merozoites antigens mediate immunity by directly inhibiting egress of merozoites from mature schizonts, or invasion of red blood cells (21). Moreover, antibodies facilitate Fc-mediated effector functions such as opsonic phagocytosis, complement activation and antibody-dependent cellular cytotoxicity (21, 22). Therefore, we mined mass spectrometry data from PlasmoDB to identify proteins expressed during the late stage schizont and merozoite stages. Both the minimum number of unique peptide sequences from the mass spectrometry data per sample and minimum number of spectra per gene applied per sample was 1. The list of the mined proteins is provided as Supplementary Table 1.
B-cell linear epitope prediction
B-cell linear epitopes are specific regions within the primary structure of proteins that interact with antibodies. The presence of B-cell linear epitopes was predicted using Bepipred 2.0 (https://services.healthtech.dtu.dk/services/BepiPred-2.0/) and Epidope (https://github.com/flomock/EpiDope) both using their default settings. Bepipred 2.0 utilizes a random forest algorithm which has been trained on epitopes annotated from 649 antigen-antibody structures derived from the Protein Data Bank (PDB), while Epidope utilizes deep neural networks using data derived from Immune Epitope Database (IEDB) (23, 24). The resulting predictions were then converted into FASTA format, where each entry in the header consisted of the indexed Plasmodium protein from the database, along with the corresponding start and end positions of the predicted epitopes separated by a colon and underscore for Bepipred and Epidope, respectively. The sequence of the predicted epitopes was included in the second line of each entry to complete the FASTA file. The predicted B-cell linear epitopes were clustered together using CD-HIT v4.8.1 to identify common epitopes using a similarity of threshold 0.9. The start and end positions of the predicted B-cell linear epitopes were individually visualized using Protter (https://wlab.ethz.ch/protter/start/) to ensure they were not present in the signal peptide or transmembrane domain region (25).
Homology analysis
The predicted B-cell linear epitopes from P. falciparum were blasted against an indexed non-falciparum database comprising of the CD-HIT clustered proteins from P. ovale, P. malariae, P. knowlesi, and P. vivax and human proteome using an e-value of 1e-05. P. falciparum B-cell linear epitopes that showed similarities with human proteins can induce autoimmune reactions or downregulate the human response and were removed from further analysis. The remaining cross-species epitopes of length between 10-30 amino acids with a percentage identity >=70 and query coverage >=95% were selected for further analysis.
Antigenicity, toxicity, and allergenicity
The antigenicity, toxicity, and allergenicity of P. falciparum B-cell linear epitope sequences were evaluated. Vaxijen 2.0 (https://www.ddg-pharmfac.net/vaxijen/VaxiJen/VaxiJen.html), a tool that allows for antigenicity prediction based on the physicochemical properties of proteins without relying on sequence alignment, was employed to determine the antigenicity of the selected peptides. Peptides were considered antigenic if the obtained score was greater than 0.5 for parasites (26). Toxinpred2 (https://webs.iiitd.edu.in/raghava/toxinpred2/) was used to assess peptide toxicity, while AllergenPro (http://nabic.rda.go.kr/allergen/) was employed to evaluate allergenicity. ToxinPred2 classifies toxins based on their BLAST similarity with existing known toxic peptides, similar motifs, and prediction models (27). AllergenPro compares peptide sequences with known allergen sequences and motifs from NCBI PubMed, UniProtKB/Swiss-Prot, and IUIS allergen databases (28). B-cell linear epitopes that were antigenic, non-toxic, and non-allergens were selected for the next step.
Conservation analysis
Parasite DNA extracted from whole blood samples (n=27) (Supplementary Table 2) of short-term cultures (1 month) in vitro until the schizont-merozoite stages from a surveillance study in four selected islands in Lake Victoria between July 2014 and July 2016, together with MalariaGen data version 6 (Supplementary Table 3) from Kenyan isolates (n=131) was used to assess the conservation of the predicted B-cell linear epitopes. The previously published study was approved by the Mount Kenya University Ethics Review Committee (038/2014) and Kenyatta National Hospital - University of Nairobi (KNH-UoN) ethical review committee (P609/10/2014), and the sequencing data was used here as secondary analysis (29). For bioinformatics analysis, the quality of paired-end reads was assessed using FastQC (30). The Plasmodium falciparum genome was retrieved from PlasmoDB and was indexed using the Burrows-Wheeler Algorithm (BWA) v0.7.17 using default settings (31). The paired-end reads were mapped to the index genome to generate a SAM file. Samtools v1.19 view was used to convert the generated SAM file to BAM format. After sorting and indexing the BAM file, Picard v2.18.7 was used to preprocess the files before variant calling using default settings (http://broadinstitute.github.io/picard). Bcftools v1.19 utilities were used to call, and normalize variants using the default options while variants were filtered based on a haploid model, including those with a base quality score > 20 and minimum read depth >=5. Bcftools consensus was then used to replace specific positions in the reference where variants occurred for each sample (32). The generated FASTA files were translated with their 3D7 reference PlasmoDB and aligned in MUSCLE v5.1 using its default options (33). The aligned protein file was downloaded, and the regions corresponding to the predicted epitope of interest were retrieved using an in-house bash script (https://github.com/sebymusundi/epitope_analysis). The resulting conservation logos were plotted using WebLogo (https://weblogo.berkeley.edu/logo.cgi).
Structural and physiochemical properties
The PEP-Fold4 server(http://bioserv.rpbs.univ-paris-diderot.fr/services/PEP-FOLD4) was used to predict the tertiary structure of peptides with lengths of 5-50 amino acids (34). ProtoParam from Expasy assessed the physiochemical properties of conserved B-cell linear epitopes. The parameters evaluated by ProtoParam included molecular weight, instability index, aliphatic index, isoelectric pH (pI), and grand average of hydropathicity index (GRAVY) (35). The instability index estimates the protein stability in a test tube. An instability index greater than 40 is regarded as unstable, while a value below 40 indicates the peptide is stable. The aliphatic index refers to the relative volume occupied by aliphatic side chains and is regarded as a key factor in increasing the thermostability of globular proteins. High aliphatic index proteins tend to be more thermally stable. GRAVY indicates the sum of hydropathy values of all amino acids divided by all residues present. Negative GRAVY scores indicate the peptide is polar, while positive GRAVY scores indicate the protein is non-polar (26).
Results
Removal of redundant proteins
The whole proteomes of the five Plasmodium species were passed through a subtractive proteomics pipeline. CD-HIT refined each proteome, removing redundant proteins at the 90% threshold. The P. ovale proteome had the highest number of proteins after removing paralogs, while P. malariae had the greatest number of paralogs (Supplementary Table 4).
Subcellular classification
The cellular location of the remaining 5289 proteins from P. falciparum was carried out using Deeploc and WoLF PSORT. Subcellular location of 3 proteins in WoLF PSORT were not predicted since they were too small (<30 amino acid) or too large (>10000 amino acids while in Deeploc the location of 19 proteins with more than 6000 amino acid sequences were not predicted. The total number of proteins present in the intracellular and extracellular regions for Deeploc was 2025 and 3218 while those for WoLF PSORT were 3188, and 2098, respectively (Figure 2). A consensus approach was used to select proteins in the extracellular region from Deeploc and WoLF PSORT, resulting in 3488 proteins. Membrane-bound proteins were excluded using key search names, including those in the apicoplast, mitochondria, golgi body and endoplasmic reticulum. Similarly, enzymes, ribosomal proteins, eukaryotic transcription, and translation factors were excluded. In total, 1046 proteins were removed from the remaining proteins, leaving behind 2442 proteins.
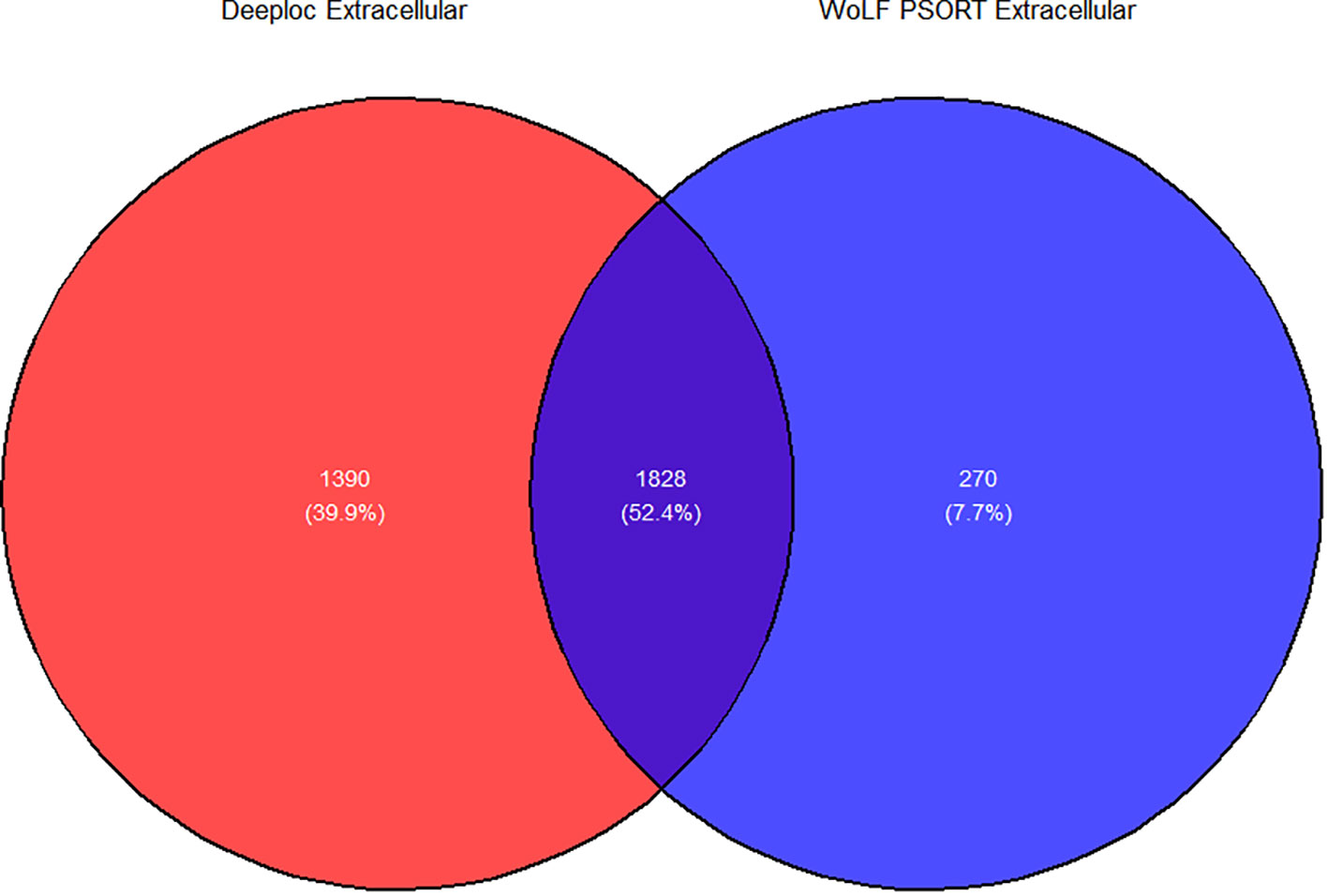
Figure 2 Venn diagram illustrating the predicted proteins in the extracellular region for Deeploc and WoLF PSORT. Extracellular proteins comprise those predicted as being present in the extracellular region and membrane-bound organelles for both prediction tools.
Surfaceome analysis and protein selection
Signal peptides and transmembrane helices are key features of proteins located on the extracellular surface. From the remaining 2442 proteins, Phobius predicted 412 proteins to either contain a signal peptide and one transmembrane helix or a signal peptide without a transmembrane helix. Since Phobius differentiates signal peptide and transmembrane helices, it predicted 711 proteins to have one transmembrane helix. A consensus approach was also applied, resulting in 953 proteins either containing a signal peptide or having one transmembrane helix. Down-selection of the 953 proteins against a list of published proteins in the merozoite stage reduced the total number of proteins to 366.
B-cell epitope analysis
Linear B-cell epitope prediction was done using the IEDB tool Bepipred 2.0 and Epidope on the 366 proteins. Bepipred 2.0 and Epidope generated 3827 and 1745 B-cell linear epitopes with a minimum length of 9 amino acids. Cluster analysis for the predicted epitopes using a threshold of 0.9 from CD-HIT generated 3637 clusters (Supplementary Table 5). Blast analysis for the predicted B-cell linear epitopes against non-falciparum species and human proteome revealed a total of 919 and 32 epitopes, respectively. The homologous human epitopes were excluded from further analysis due to their ability to induce auto-antibodies. Subsequent filtering of homologous non-falciparum regions, which showed a similarity >=95%, minimum coverage of 70%, and alignment length between 9-30 amino acids, resulted in 159 cross-species epitopes. Antigenicity analysis using Vaxijen 2.0 revealed the presence of 69 antigenic epitopes. Of these, 31 epitopes were predicted to be allergens from AllerTop, while 14 epitopes were predicted to be toxins from Toxinpred2.0 (Supplementary Table 6). Six of the remaining 24 epitopes were located either within the signal peptide region or within the intracellular region of transmembrane helix using Protter, resulting in a final 18 epitopes (Supplementary Table 7). B-cell linear epitopes in present in more than 3 Plasmodium species were further selected for conservation analysis. Additional information regarding P. falciparum antigen selected, start and stop position of the epitope, antigenicity, phenotype information from PhenoPlasm, and their presence in non-falciparum species was extracted (Table 1).
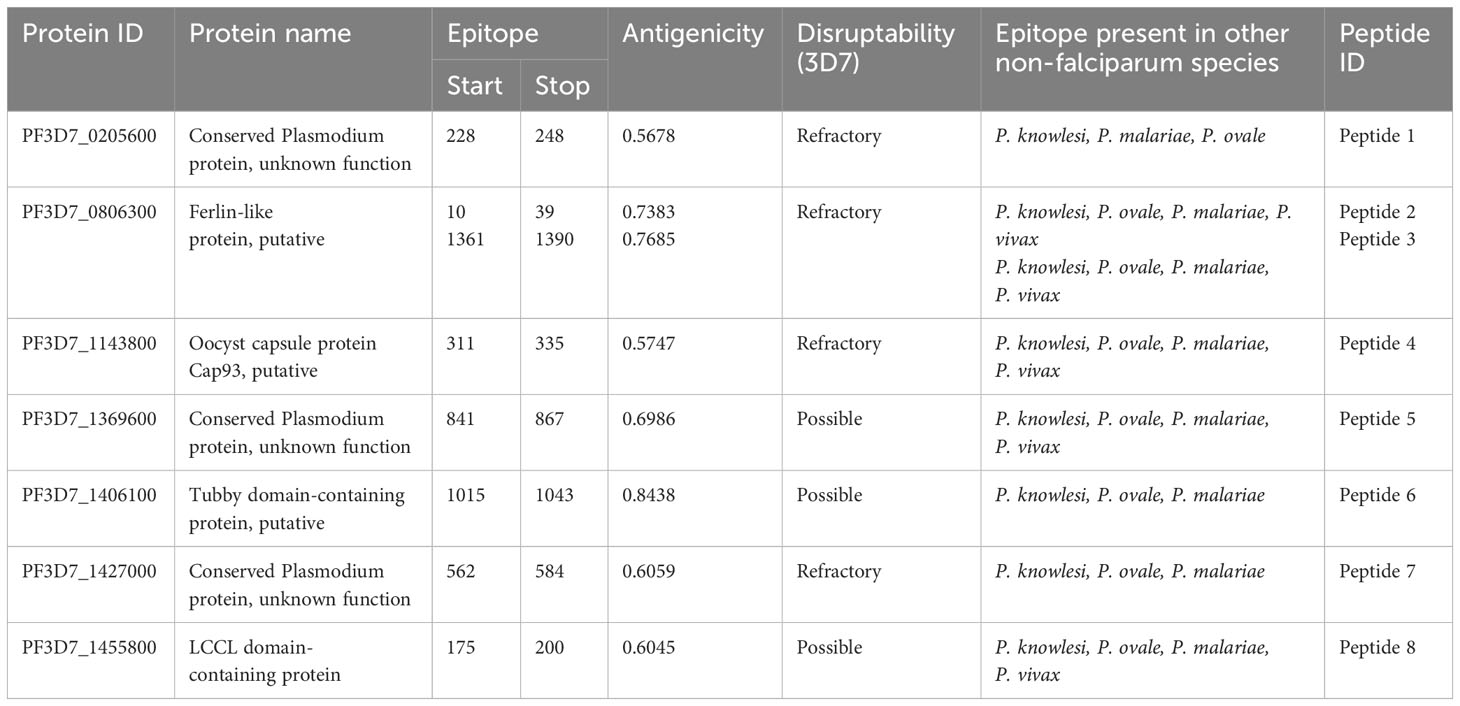
Table 1 Plasmodium falciparum protein containing the selected B-cell linear epitopes, antigenicity scores, essentiality of the gene and presence in other non-falciparum species Complete list is shown is Supplementary Table 3.
Cross-species blast analysis of the predicted B-cell linear epitopes revealed the presence of 8 epitopes in more than four Plasmodium species based on the applied blast parameters (Supplementary Table 7). Some predicted B-cell linear epitopes showed high similarity between P. falciparum and one or two other Plasmodium species (Supplementary Table 8). The antigenicity score of the predicted peptides ranged from 0.5040 to 1.2264 (Table 1). Proteins, PF3D7_0205600, PF3D7_0806300, PF3D7_1143800, and PF3D7_1427000 containing the B-cell linear epitopes showed an essential role in the parasite) and were designated as refractory in Phenoplasm while the proteins PF3D7_1369600, PF3D7_1406100 and PF3D7_1455800 containing did not hinder parasite growth (Table 1). Peptides 2 and 4 showed the least changes in their amino acid sequences when plasmodium species were compared against the other non-falciparum species (Figure 3). The conservancy assessment of the eight peptides based on locally sequenced isolates in P. falciparum (n=27) and Kenyan isolates from MalariaGEN (n=131) showed high conservation with no change in the amino acid sequence being reported in the predicted region (Figure 3).
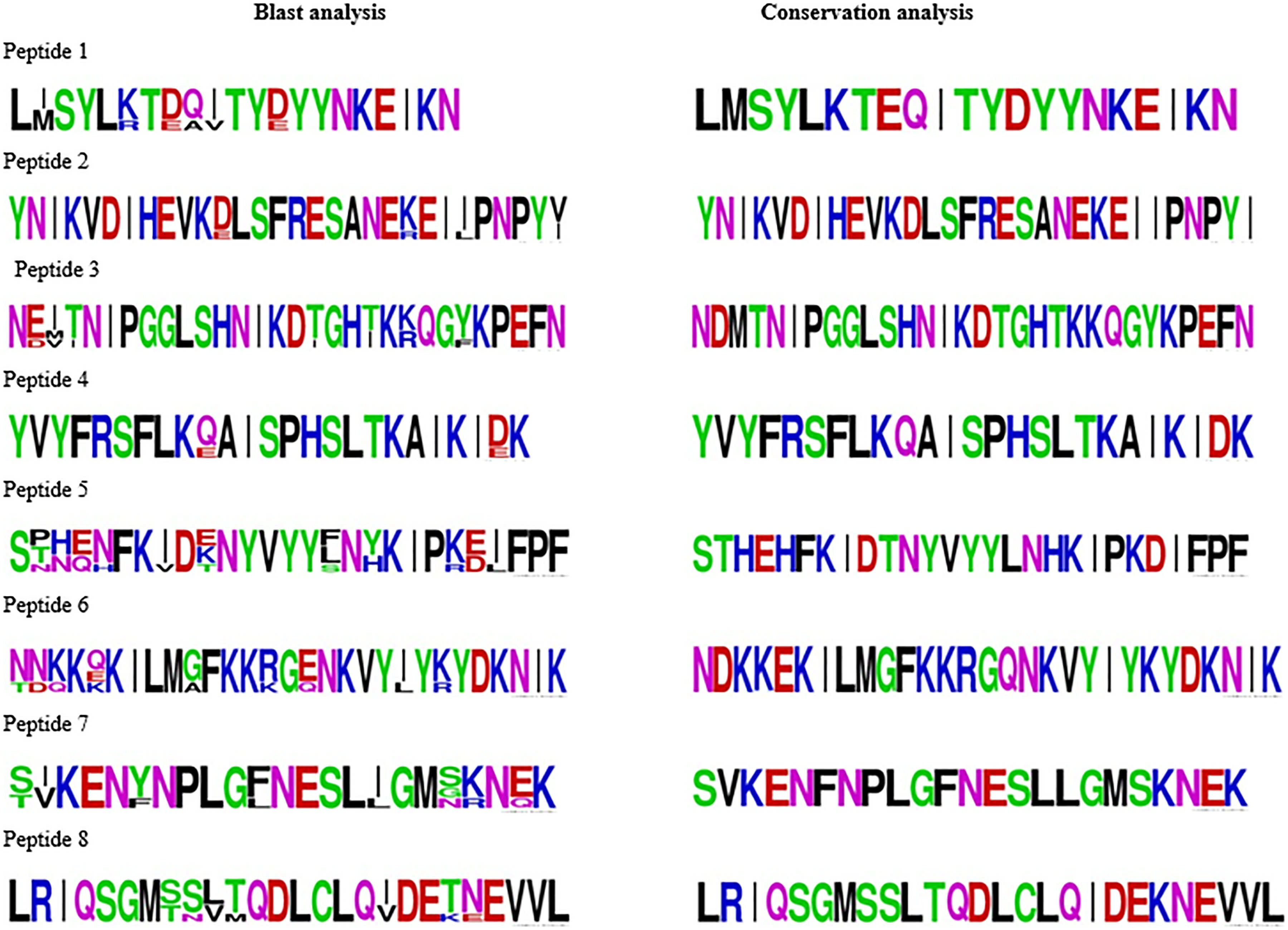
Figure 3 Blast and conservation analysis of the 8 predicted B-cell linear epitopes. Blast analysis incorporates predicted B-cell linear epitopes in falciparum against non-falciparum species with percentage similarity >70%, alignment length <=30 and coverage >=95. B-cell linear epitopes conservation analysis was against local sequenced isolates from Homabay (n=27) and MalariaGEN (n=131) from Kenya. The height of the amino acid sequence at each position represents its relative frequency in both the blast and conservation analysis.
Structural and physicochemical properties
The physicochemical properties of the final eight antigenic, non-toxic, and non-allergens epitopes were assessed. The properties, including the molecular weight, aliphatic index, pI, GRAVY, instability index, and total number of positively and negatively charged amino acids, are summarized in Table 2. The molecular weight of the eight selected peptides ranged from 2582.91 to 4813.77. The results also showed that all peptides were hydrophilic except peptide 8, while only peptide 1 was predicted to be unstable. The pI of the epitopes ranged from 4.32 to 10.22, while the most aliphatic and thermostable was peptide 8. The 3D prediction results from PEP-FOLD-4 revealed that peptides 1, 3, 5, and 7 had helices and coils, while peptides 2, 4, 6, and 8 comprised helices, strands, and coils. (Supplementary Figure 1).
Discussion
Malaria, the most common tropical disease, remains a major threat to Sub-Saharan Africa. The different transmission patterns associated with Plasmodium species threaten malaria elimination efforts. Recent studies have documented that cross-protective immunity may play a key role in developing vaccines that may halt malaria transmission (10, 36). Combining subtractive proteomics and immune-informatic approaches offers a faster identification of potential targets before experimental validation. The increase in high-throughput genomic sequencing of various malaria isolates across the globe also allows us to examine if the predicted targets are conserved, which is essential in vaccine development.
Prioritization of potential targets from the proteome level relies on subtractive proteomics to narrow down to a few essential proteins. The decision tree implemented followed commonly established procedures, including proteome clustering, subcellular localization, surfaceome predictions, and similarity analysis using BLAST (37). The use of CD-HIT ensured that only non-redundant proteins were assessed. Two tools, WoLF PSORT and Deeploc, were utilized to predict the subcellular location of the potential targets. Unlike bacterial organisms whose tools for determining subcellular locations are well established, a significant gap still exists in determining the subcellular location of protozoan parasites since they contain special organelles such as dense granules, rhoptries and micronemes. Thus, utilizing a consensus approach from two tools that predict the location using different approaches increases the chance of picking up more targets. A similar analysis carried out by Componovo et al. also revealed that following strict classification by only choosing extracellular and plasma membrane proteins also left out numerous proteins misclassified in the other organelles, which showed high immunoreactivity against the patient’s sera (38). Therefore, adding an extra step involving eliminating specific protein names found in intracellular organelles not involved in the invasion process improves the subcellular classification of proteins.
Blood-stage antigens such as those on merozoites are essential for red blood cells invasion, hence excellent vaccine candidates. Ideal vaccine candidates, especially in malaria, should possess low levels of polymorphism and be highly antigenic (39). Our results identified eight B-cell linear epitopes, which were predicted to be antigenic and showed high levels of conservation, especially across P. falciparum isolates. In addition, the isolates showed high similarity with other non-falciparum species linear epitope regions. The conserved nature of the epitopes and their high similarity with non-falciparum species indicates the likelihood of cross-species reactivity and immunogenicity, although this needs to be experimentally validated. P. falciparum antigen VAR2CSA and P. vivax DBP (11), Pfs48/45 and Pvs48/45 (40), and P. vivax AMA1, and P. falciparum AMA1 (41) have shown cross-species reactivity. Cross-protective immunity towards human malaria has also been shown experimentally between P. vivax AARP and P. knowlesi AARP and P. vivax RhopH2 and P. knowlesi RhopH2 (10, 42). Furthermore, the presence of conserved B-cell epitopes between P. vivax RhopH2 and P. knowlesi RhopH2 has already been established (42). Experiments in mice models utilizing Pvs48/45 and Pfs48/45 antigens also showed the ability of the two antigens to cross-boost each other (43). In natural populations, mixed infections of various species occur, increasing the possibility of inducing cross-protection against multiple species. In the current context, we postulate an immune response targeting multiple different parasite species, driven by their shared B-cell epitopes. Additionally, we anticipate a reinforced secondary immune response upon repeated exposure to either parasites species.
A stable structure among other physiochemical properties are key for a novel vaccine candidate. Here, six epitopes were identified and predicted to have a stable structure, while one epitope had an unstable structure. Cyclization of the peptide sequence is one of the approaches that could be applied to make the peptides more stable before further downstream analysis (44). Furthermore, epitopes were shown to have an isoelectric pH (pI) ranging from 4.32 to 10.22. The index pI is an important factor in selecting peptide vaccine since, at their pI, they have no electric charge, and considering that B-cell receptors prefer charged and polar antigen residues, their immunogenicity tend to decrease (45). Therefore, it ideal for the pI to not fall within the range of human body tissues (pH 7.2 to 7.6) as in the case of all epitopes. All these parameters suggest these epitopes are appropriate candidates for in-vitro and in-vivo validation experiments.
In conclusion, in this study, we utilized subtractive proteomics and immunoinformatics techniques to identify conserved cross-species B-cell linear epitopes in the merozoite stage. These epitopes are antigenic, non-toxic, non-similar to humans, non-allergens, and have suitable physiochemical properties. Evaluation of the identified conserved B-cell epitopes could contribute to development of vaccines that induce cross-protective immunity. Nevertheless, there is a need to confirm the functionality of the individual conserved epitopes in-vitro and in-vivo experiments.
Data availability statement
The data presented in the study are deposited in the European Nucleotide Archive repository, accession number PRJEB46180.
Ethics statement
The studies involving humans were approved by Mount Kenya University Ethics Review Committee (038/2014) and Kenyatta National Hospital - University of Nairobi (KNH-UoN) ethical review committee (P609/10/2014). The studies were conducted in accordance with the local legislation and institutional requirements. Written informed consent for participation in this study was provided by the participants’ legal guardians/next of kin.
Author contributions
SM: Formal analysis, Investigation, Methodology, Visualization, Writing – original draft. JG: Conceptualization, Resources, Supervision, Writing – review & editing. BK: Conceptualization, Resources, Supervision, Writing – review & editing.
Funding
The author(s) declare financial support was received for the research, authorship, and/or publication of this article. BK is an EDCTP fellow under the EDCTP2 program supported by the European Union grant number TMA2020CDF-3203-EndPAMAL. JG is supported by the African Academy of Sciences and Royal Society FLAIR grant number FLR\R1\201314.
Acknowledgments
We wish to acknowledge the Mount Kenya University research team for their insights into this work.
Conflict of interest
The authors declare that the research was conducted in the absence of any commercial or financial relationships that could be construed as potential conflict of interest.
Publisher’s note
All claims expressed in this article are solely those of the authors and do not necessarily represent those of their affiliated organizations, or those of the publisher, the editors and the reviewers. Any product that may be evaluated in this article, or claim that may be made by its manufacturer, is not guaranteed or endorsed by the publisher.
Supplementary material
The Supplementary Material for this article can be found online at: https://www.frontiersin.org/articles/10.3389/fimmu.2024.1352618/full#supplementary-material
Supplementary Table 1 | List of merozoite proteins retrieved from mass spectrometry data from PlasmoDB.
Supplementary Table 2 | Metadata for the sequenced Kenyan isolates from Homabay county (n=27)
Supplementary Table 3 | Metadata for the MalariaGen version 6 Kenyan isolates (n=131)
Supplementary Table 4 | Number of redundant and non-redundant proteins across different Plasmodium species associated with human malaria.
Supplementary Table 5 | Clusters of predicted B-cell linear epitopes after combining Bepipred 2.0 and Epidope.
Supplementary Table 6 | Antigenicity, allergenicity and toxicity predictions for cross-species epitopes.
Supplementary Table 7 | P. falciparum protein containing the selected b-cell linear epitopes position, antigenicity scores, essentiality of the gene and presence in other non-falciparum species.
Supplementary Table 8 | Blast output for the selected B-cell linear epitopes present in P. falciparum and non-falciparum species.
References
1. Price RN, Commons RJ, Battle KE, Thriemer K, Mendis K. Plasmodium vivax in the Era of the Shrinking P. falciparum Map. Trends Parasitol (2020) 36:560–70. doi: 10.1016/j.pt.2020.03.009
2. World Health Organization. World malaria report 2022 (2022). Available at: https://iris.who.int/bitstream/handle/10665/365169/9789240064898-eng.pdf?sequence=1 (Accessed July 12, 2023).
3. Yman V, Wandell G, Mutemi DD, Miglar A, Asghar M, Hammar U, et al. Persistent transmission of Plasmodium malariae and Plasmodium ovale species in an area of declining Plasmodium falciparum transmission in eastern Tanzania. PloS Negl Trop Dis (2019) 13. doi: 10.1371/JOURNAL.PNTD.0007414
4. Cooper DJ, Rajahram GS, William T, Jelip J, Mohammad R, Benedict J, et al. Plasmodium knowlesi Malaria in Sabah, Malaysia, 2015-2017: Ongoing increase in incidence despite nearelimination of the human-only plasmodium species. Clin Infect Dis (2020) 70:361–7. doi: 10.1093/cid/ciz237
5. William T, Rahman HA, Jelip J, Ibrahim MY, Menon J, Grigg MJ, et al. Increasing Incidence of Plasmodium knowlesi Malaria following Control of P. falciparum and P. vivax Malaria in Sabah, Malaysia. PloS Negl Trop Dis (2013) 7. doi: 10.1371/JOURNAL.PNTD.0002026
6. Collins WE, Jeffery GM. Plasmodium ovale: Parasite and disease. Clin Microbiol Rev (2005) 18:570–81. doi: 10.1128/CMR.18.3.570-581.2005
7. Tarimo BB, Nyasembe VO, Ngasala B, Basham C, Rutagi IJ, Muller M, et al. Seasonality and transmissibility of Plasmodium ovale in Bagamoyo District, Tanzania. Parasites Vectors (2022) 15. doi: 10.1186/s13071-022-05181-2
8. Holzschuh A, Gruenberg M, Hofmann NE, Wampfler R, Kiniboro B, Robinson LJ, et al. Co-infection of the four major Plasmodium species: Effects on densities and gametocyte carriage. PloS Negl Trop Dis (2022) 16. doi: 10.1371/JOURNAL.PNTD.0010760
9. Duffy PE, Patrick Gorres J. Malaria vaccines since 2000: progress, priorities, products. NPJ Vaccines (2020) 5. doi: 10.1038/s41541-020-0196-3
10. Muh F, Ahmed MA, Han JH, Nyunt MH, Lee SK, Lau YL, et al. Cross-species analysis of apical asparagine-rich protein of Plasmodium vivax and Plasmodium knowlesi. Sci Rep 2018 81 (2018) 8:1–11. doi: 10.1038/s41598-018-23728-1
11. Gnidehou S, Mitran CJ, Arango E, Banman S, Mena A, Medawar E, et al. Cross-species immune recognition between plasmodium vivax duffy binding protein antibodies and the plasmodium falciparum surface antigen VAR2CSA. J Infect Dis (2019) 219:110–20. doi: 10.1093/infdis/jiy467
12. Mitran CJ, Mena A, Gnidehou S, Banman S, Arango E, Lima BAS, et al. Antibodies to cryptic epitopes in distant homologues underpin a mechanism of heterologous immunity between plasmodium vivax PvDBP and plasmodium falciparum VAR2CSA. MBio (2019) 10:e02343–19. doi: 10.1128/mBio.02343-19
13. Iyamu U, Vinals DF, Tornyigah B, Arango E, Bhat R, Adra TR, et al. A conserved epitope in VAR2CSA is targeted by a cross-reactive antibody originating from Plasmodium vivax Duffy binding protein. Front Cell Infect Microbiol (2023) 13:1202276. doi: 10.3389/fcimb.2023.1202276
14. Fu L, Niu B, Zhu Z, Wu S, Li W. CD-HIT: Accelerated for clustering the next-generation sequencing data. Bioinformatics (2012) 28:3150–2. doi: 10.1093/bioinformatics/bts565
15. Horton P, Park KJ, Obayashi T, Fujita N, Harada H, Adams-Collier CJ, et al. WoLF PSORT: Protein localization predictor. Nucleic Acids Res (2007) 35:W585–7. doi: 10.1093/nar/gkm259
16. Thumuluri V, Almagro Armenteros JJ, Johansen AR, Nielsen H, Winther O. DeepLoc 2.0: multi-label subcellular localization prediction using protein language models. Nucleic Acids Res (2022) 50:W228–34. doi: 10.1093/nar/gkac278
17. Krogh A, Larsson B, Von Heijne G, Sonnhammer ELL. Predicting transmembrane protein topology with a hidden Markov model: Application to complete genomes. J Mol Biol (2001) 305:567–80. doi: 10.1006/jmbi.2000.4315
18. Owji H, Nezafat N, Negahdaripour M, Hajiebrahimi A, Ghasemi Y. A comprehensive review of signal peptides: Structure, roles, and applications. Eur J Cell Biol (2018) 97:422–41. doi: 10.1016/j.ejcb.2018.06.003
19. Käll L, Krogh A, Sonnhammer ELL. A combined transmembrane topology and signal peptide prediction method. J Mol Biol (2004) 338:1027–36. doi: 10.1016/j.jmb.2004.03.016
20. Watson RB. Malaria parasites and ther aemosporidia. Am J Trop Med Hyg (1967) 16:561–3. doi: 10.4269/ajtmh.1967.16.561
21. Beeson JG, Drew DR, Boyle MJ, Feng G, Fowkes FJI, Richards JS. Merozoite surface proteins in red blood cell invasion, immunity and vaccines against malaria. FEMS Microbiol Rev (2016) 40:343–72. doi: 10.1093/femsre/fuw001
22. Gonzales SJ, Reyes RA, Braddom AE, Batugedara G, Bol S, Bunnik EM. Naturally cquired umoral mmunity gainst lasmodium falciparum alaria. Front Immunol (2020) 11:594653. doi: 10.3389/fimmu.2020.594653
23. Jespersen MC, Peters B, Nielsen M, Marcatili P. BepiPred-2.0: Improving sequence-based B-cell epitope prediction using conformational epitopes. Nucleic Acids Res (2017) 45:W24–9. doi: 10.1093/nar/gkx346
24. Collatz M, Mock F, Barth E, Holzer M, Sachse K, Marz M. Epidope: A deep neural network for linear b-cell epitope prediction. Bioinformatics (2021) 37:448–55. doi: 10.1093/bioinformatics/btaa773
25. Omasits U, Ahrens CH, Müller S, Wollscheid B. Protter: Interactive protein feature visualization and integration with experimental proteomic data. Bioinformatics (2014) 30:884–6. doi: 10.1093/bioinformatics/btt607
26. Doytchinova IA, Flower DR. VaxiJen: A server for prediction of protective antigens, tumour antigens and subunit vaccines. BMC Bioinf (2007) 8. doi: 10.1186/1471-2105-8-4
27. Sharma N, Naorem LD, Jain S, Raghava GPS. ToxinPred2: An improved method for predicting toxicity of proteins. Brief Bioinform (2022) 23:1–12. doi: 10.1093/bib/bbac174
28. Kim C, Kwon S, Lee G, Lee H, Choi J, Kim Y, et al. and tools for allergenicity prediction. Bioinformation (2009) 3:344–5. doi: 10.6026/97320630003344
29. Gitaka JN, Takeda M, Kimura M, Idris ZM, Chan CW, Kongere J, et al. Selections, frameshift mutations, and copy number variation detected on the surf 4.1 gene in the western Kenyan Plasmodium falciparum population. Malar J (2017) 16:98. doi: 10.1186/s12936-017-1743-x
30. Leggett RM, Ramirez-Gonzalez RH, Clavijo BJ, Waite D, Davey RP. Sequencing quality assessment tools to enable data-driven informatics for high throughput genomics. Front Genet (2013) 4:288. doi: 10.3389/fgene.2013.00288
31. Li H, Durbin R. Fast and accurate long-read alignment with Burrows-Wheeler transform. Bioinformatics (2010) 26:589–95. doi: 10.1093/bioinformatics/btp698
32. Danecek P, Bonfield JK, Liddle J, Marshall J, Ohan V, Pollard MO, et al. Twelve years of SAMtools and BCFtools. Gigascience (2021) 10:1–4. doi: 10.1093/gigascience/giab008
33. Edgar RC. MUSCLE: Multiple sequence alignment with high accuracy and high throughput. Nucleic Acids Res (2004) 32:1792–7. doi: 10.1093/nar/gkh340
34. Rey J, Murail S, De Vries S, Derreumaux P, Tuffery P. PEP-FOLD4: A pH-dependent force field for peptide structure prediction in aqueous solution. Nucleic Acids Res (2023) 51:W432–7. doi: 10.1093/nar/gkad376
35. Wilkins MR, Gasteiger E, Bairoch A, Sanchez JC, Williams KL, Appel RD, et al. Protein identification and analysis tools in the ExPASy server. Methods Mol Biol (1999) 112:531–52. doi: 10.1385/1-59259-584-7:531
36. Muh F, Kim N, Nyunt MH, Firdaus ER, Han JH, Hoque MR, et al. Cross-species reactivity of antibodies against plasmodium vivax blood-stage antigens to plasmodium knowlesi. PloS Negl Trop Dis (2020) 14:1–21. doi: 10.1371/JOURNAL.PNTD.0008323
37. Aguttu C, Okech BA, Mukisa A, Lubega GW. Screening and characterization of hypothetical proteins of Plasmodium falciparum as novel vaccine candidates in the fight against malaria using reverse vaccinology. J Genet Eng Biotechnol (2021) 19. doi: 10.1186/s43141-021-00199-y
38. Camponovo F, Campo JJ, Le TQ, Oberai A, Hung C, Pablo JV, et al. Proteome-wide analysis of a malaria vaccine study reveals personalized humoral immune profiles in Tanzanian adults. Elife (2020) 9:1–21. doi: 10.7554/eLife.53080
39. Ouattara A, Barry AE, Dutta S, Remarque EJ, Beeson JG, Plowe CV. Designing malaria vaccines to circumvent antigen variability. Vaccine (2015) 33:7506–12. doi: 10.1016/j.vaccine.2015.09.110
40. Bansal GP, Vengesai A, Cao Y, Mduluza T, Kumar N. Antibodies elicited during natural infection in a predominantly Plasmodium falciparum transmission area cross-react with sexual stage-specific antigen in P. vivax. Acta Trop (2017) 170:105–11. doi: 10.1016/j.actatropica.2017.02.032
41. Igonet S, Vulliez-Le Normand B, Faure G, Riottot MM, Kocken CHM, Thomas AW, et al. Cross-reactivity tudies of an nti-lasmodium vivax pical membrane antigen 1 conoclonal antibody finding and structural characterisation. J Mol Biol (2007) 366:1523–37. doi: 10.1016/j.jmb.2006.12.028
42. Ahmed MA, Deshmukh GY, Zaidi RH, Saif A, Alshahrani MA, Wazid SW, et al. Identification, Mapping, and Genetic Diversity of Novel Conserved Cross-Species Epitopes of RhopH2 in Plasmodium knowlesi With Plasmodium vivax. Front Cell Infect Microbiol (2022) 11:810398/BIBTEX. doi: 10.3389/FCIMB.2021.810398/BIBTEX
43. Cao Y, Bansal GP, Merino K, Kumar N. Immunological Cross-Reactivity between Malaria Vaccine Target Antigen P48/45 in Plasmodium vivax and P. falciparum and Cross-Boosting of Immune Responses. PloS One (2016) 11. doi: 10.1371/JOURNAL.PONE.0158212
44. Hamley IW. Peptides for vaccinedevelopment. ACS Appl Bio Mater (2022) 5:905–44. doi: 10.1021/acsabm.1c01238
Keywords: conserved cross-species, B-cell epitope, P. falciparum, immunoinformatics, subtractive proteomics
Citation: Musundi SD, Gitaka J and Kanoi BN (2024) Identification of conserved cross-species B-cell linear epitopes in human malaria: a subtractive proteomics and immuno-informatics approach targeting merozoite stage proteins. Front. Immunol. 15:1352618. doi: 10.3389/fimmu.2024.1352618
Received: 08 December 2023; Accepted: 25 January 2024;
Published: 09 February 2024.
Edited by:
Elke Bergmann-Leitner, Walter Reed Army Institute of Research, United StatesReviewed by:
Shahab Zaki Pour, University of São Paulo, BrazilMichal Fried, National Institute of Allergy and Infectious Diseases (NIH), United States
Copyright © 2024 Musundi, Gitaka and Kanoi. This is an open-access article distributed under the terms of the Creative Commons Attribution License (CC BY). The use, distribution or reproduction in other forums is permitted, provided the original author(s) and the copyright owner(s) are credited and that the original publication in this journal is cited, in accordance with accepted academic practice. No use, distribution or reproduction is permitted which does not comply with these terms.
*Correspondence: Bernard N. Kanoi, Ymthbm9pQG1rdS5hYy5rZQ==