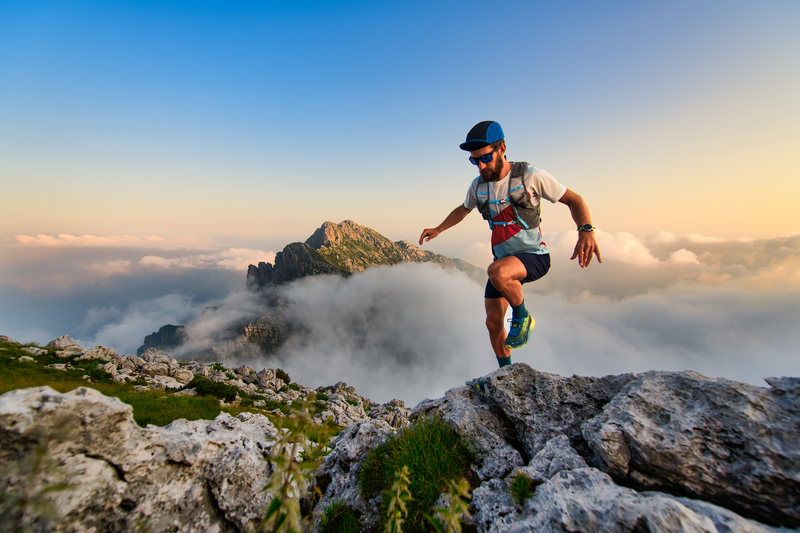
94% of researchers rate our articles as excellent or good
Learn more about the work of our research integrity team to safeguard the quality of each article we publish.
Find out more
ORIGINAL RESEARCH article
Front. Immunol. , 20 March 2024
Sec. Autoimmune and Autoinflammatory Disorders : Autoimmune Disorders
Volume 15 - 2024 | https://doi.org/10.3389/fimmu.2024.1334772
This article is part of the Research Topic Immune system disorders: from molecular mechanisms to clinical implications View all 28 articles
Background: Autoimmune thyroid disease (AITD) ranks among the most prevalent thyroid diseases, with inflammatory cytokines playing a decisive role in its pathophysiological process. However, the causal relationship between the inflammatory cytokines and AITD remains elusive.
Methods: A two-sample Mendelian randomization (MR) analysis was performed to elucidate the causal connection between AITD and 41 inflammatory cytokines. Genetic variations associated with inflammatory cytokines were sourced from the FinnGen biobank, whereas a comprehensive meta-analysis of genome-wide association studies (GWASs) yielded data on Graves’ disease (GD) and Hashimoto thyroiditis. Regarding the MR analysis, the inverse variance-weighted, MR-Egger, and weighted median methods were utilized. Additionally, sensitivity analysis was conducted using MR-Egger regression, MR-pleiotropy residual sum, and outliers.
Results: Seven causal associations were identified between inflammatory cytokines and AITD. High levels of tumor necrosis factor–β and low levels of stem cell growth factor–β were indicative of a higher risk of GD. In contrast, high levels of interleukin-12p70 (IL-12p70), IL-13, and interferon-γ and low levels of monocyte chemotactic protein–1 (MCP-1) and TNF-α suggested a higher risk of HD. Moreover, 14 causal associations were detected between AITD and inflammatory cytokines. GD increases the levels of macrophage inflammatory protein–1β, MCP-1, monokine induced by interferon-γ (MIG), interferon γ–induced protein 10 (IP-10), stromal cell–derived factor–1α, platelet-derived growth factor BB, β–nerve growth factor, IL-2ra, IL-4, and IL-17 in blood, whereas HD increases the levels of MIG, IL-2ra, IP-10, and IL-16 levels.
Conclusion: Our bidirectional MR analysis revealed a causal relationship between inflammatory cytokines and AITD. These findings offer valuable insights into the pathophysiological mechanisms underlying AITD.
Autoimmune thyroid disease (AITD) encompasses a group of organ-specific autoimmune diseases characterized by the production of antithyroid antibodies and infiltration of lymphocytes in the thyroid gland (1). Epidemiological investigations established that its prevalence is approximately 5% in the general population and has steadily increased in recent years (2, 3). Clinically, Graves’ Disease (GD) and Hashimoto thyroiditis (HT) are the most common forms of AITD, hallmarked by hyperthyroidism and hypothyroidism, respectively (4). Among them, the former represents a specific autoimmune disease featured by increased thyroid hormone secretion driven by an immune system imbalance that promotes the production of thyroid-stimulating antibodies (5). Additionally, as an AITD, the pathogenesis of HT is similar to that of GD. Specifically, the pathogenesis of AITD involves genetic defects and genetic susceptibility, psychological factors, infections, and stress reactions. Meanwhile, environmental pollution and a high-iodine diet can contribute to or exacerbate autoimmune reactions leading to HT (6). At present, there is an urgent need to investigate the pathogenesis of AITD to develop insights into clinical interventions.
Inflammatory cytokines are a group of proteins or peptides generated by immune cells and exhibit a diverse range of biological activities. Consequently, they play a pivotal role in autoimmune responses (7, 8). Previous studies have documented a close correlation between inflammatory cytokines and the development and progression of AITD (9, 10). On the one hand, cytokine imbalance directly or indirectly induces thyroid epithelial cell growth, abnormal differentiation, and immune dysfunction and can also change the state of immunoreactive cells and participate in the occurrence and development of AITD (11). On the other hand, lymphocytes infiltrating the thyroid in patients with AITD can also contribute to cytokine production. For example, in the helper T cell Th1/Th2 subgroups, Th1 cells, predominantly expressing interleukin-2 (IL-2) and interferon-γ (IFN-γ), stimulate delayed hypersensitivity and mediate cellular immune responses. In contrast, Th2 cells, characterized by expression of IL-4, IL-5, IL-10, and IL-13, modulate antibody production and humoral immune responses (12). At the same time, a growing body of evidence has highlighted the pivotal role of Th17 cells in the development of AITD. Of note, Th17 cells predominantly synthesize IL-17A, IL-17F, IL-21, and IL-22, along with their associated cellular and secretory components (13). Although an increasing number of studies have documented an association between inflammatory cytokines and AITD, their causal relationship remains to be investigated.
Given that genetic predisposition plays a key role in the development of AITD, it should be explained from the perspective of genetics (14). Mendelian randomization (MR) is a superior analytical approach for drawing etiological conclusions by employing genetic variation as an instrumental variable (IV) for exposure. This approach has a lower susceptibility to confounding factors owing to the random allocation of germline genetic variation during meiosis, allowing it to accurately represent exposure without being influenced by reverse causation (15). Importantly, MR studies can be performed to explore numerous dependable genetic variants owing to the public release of extensive gene-wide association data. Hence, this investigation aimed to examine the causal relationship between inflammatory cytokines and AITD.
As depicted in Figure 1, MR relies on three fundamental assumptions: (1) IVs exhibit a robust association with the exposure factor; (2) IVs are not correlated with any confounding factors; and (3) IVs solely impact the results through the exposure and not via any other mechanisms (16). In the current study, pooled data from the published genome-wide association studies (GWASs) of 41 inflammatory cytokines and AITD were utilized. Firstly, genetic variants associated with each inflammatory factor were selected to identify the causal relationship of each inflammatory cytokine with GD and HT. Secondly, AITD-associated genetic variants were employed to infer causality between GD and HT and inflammatory cytokines, respectively.
Figure 1 Assumptions and study design of the MR study of the associations between 41 inflammatory factors and autoimmune thyroid disease. BMI, body mass index; IVs, instrumental variables; SNPs, single-nucleotide polymorphisms; MR-PRESSO, Mendelian randomization pleiotropy residual sum and outlier.
As detailed in Table 1, the genetic associations of 41 inflammatory cytokines were analyzed using data from 8,293 Finnish participants in the Cardiovascular Risk in Finnish Youth Study and the FINRISK Study (17). The first step involved genetic adjustment for 10 principal genetic components, comprising age, gender, and body mass index, as well as for population stratification and cryptic kin using genomic control.
The GWAS dataset associated with GD and HD was derived from the UK Biobank Project (18). In order to expand the genetic association map beyond European populations, 220 in-depth GWASs were conducted using data from the Biobank in Japan, integrating text mining of past medical histories and electronic medical records. A meta-analysis involving the UK Biobank and FinnGen yielded approximately 5,000 new loci, thereby enhancing the resolution of the human trait genome map. Finally, a statistical decomposition of the overall phenomenon’s summary statistical matrix, coupled with the identification of potential genetic components, assisted in identifying relevant variants and biological mechanisms associated with current disease classifications in the population.
In the present study, single-nucleotide polymorphisms (SNPs) that are significantly associated with the relative abundance of 41 inflammatory cytokines were selected as IVs. Prior investigations have established that incorporating multiple IVs enhances the interpretation of exposure variation, thereby enhancing the accuracy and reliability of the results. Consequently, the 41 inflammatory cytokines were analyzed on the basis of the outcomes of a significant association analysis with P < 1 × 10−5. The linkage disequilibrium criteria were set at r2 < 0.001, whereas the genetic distance was established as 10,000 kb. To ensure the independence of the included SNPs, highly correlated SNPs were excluded. Subsequently, SNPs associated with the relative abundance of the 41 inflammatory cytokines were integrated into the GWAS data of AITD, and corresponding statistical parameters were extracted. The data were harmonized by comparing the statistical parameters at the same sites in the GWAS results of inflammatory cytokines relative abundance and AITD, which facilitated the alignment of the effect values of exposure and outcome with the same effect allele.
In the present study, the inverse variance-weighted (IVW), MR-Egger, and weighted median (WME) methods were employed to comprehensively assess causal effects. The IVW approach assumes the validity of all genetic variants. The causal effect value of each individual IV is calculated by IVs using the ratio method. Afterward, each estimate is meticulously summarized for weighted linear regression, yielding the total effect value (19). Regarding regression, the MR-Egger method and the IVW method primarily diverge in their consideration of the intercept term (20). The WME approach utilizes the intermediate impacts of every accessible genetic variation. Estimates were acquired by weighing the inverse variance of each SNP’s association with the outcome (21).
The IVW method is superior to the other two MR methods. Therefore, the IVW method was selected as the preferred method for estimating causal effects. In order to mitigate the risk of false positives, the Benjamini–Hochberg (BH) method was used to control the false discovery rate (FDR). For a clearer explanation of the findings, the impact of the 41 inflammatory cytokines on GD and HT was present as odds ratios (ORs) for each 1 standard deviation genetic predicted change in cytokine levels. In contrast, the impact of GD and HT on the levels of inflammatory cytokines was expressed as β coefficient and 95% confidence intervals (95% CI). Considering that the connection between effect estimates and testing causation might be influenced by weak instrumental biases, the F-statistic was employed to assess the strength of the IVs using the following formula: F = R2 (n − K −1)/k (1 − R2) (22), where R2 represents the variance (per circulating cytokine) explained by the independent variable (IV), and n represents the sample size. R2 was estimated on the basis of the minor allele frequency (MAF) and b-value, calculated using the equation: R2 = 2 × MAF × (1 − MAF) × b2 (23).
Furthermore, to ensure the stability and reliability of the findings, quality control consisting of sensitivity analysis, heterogeneity test, and gene pleiotropy test were carried out at every level. To assess the impact of each SNP on the outcome heterogeneity test, sensitivity analysis was employed using the leave-one-out method, and the combined effect size of the remaining SNPs was determined through sequential deletion of individual SNPs. In the case of significant heterogeneity between IVs (Q_pval < 0.05), the MR effect size was estimated using random-effect IVW. Otherwise, fixed-effect IVW was adopted (24). In order to examine the strength of the findings, additional sensitivity analyses were performed, including MR-Egger regression, MR-pleiotropy residual sum, and outliers (MR-PRESSO) tests. Specifically, WME accurately estimates the causal impact even with less than half of the data originating from the unreliable IV (21). The P-value of the intercept term in MR-Egger regression can serve as an indication of directional pleiotropy (25). Concerning the MR-PRESSO test, the multi-effect effect was corrected by excluding the outlier. In this study, MR analysis and quality control were conducted using R version 4.0.3 and TwoSampleMR version 0.5.6.
The flow chart of 41 inflammatory cytokines and AITD is shown in Figure 1. The calculated F-values ranged from 11.156 to 788.955, all meeting the threshold of greater than 10, indicating that weak instrumental bias was unlikely (Supplementary Tables 1–4). Finally, we identified 21 causal associations between inflammatory cytokines and AITD (Tables 2, 3).
Table 2 Effects of the relationship between meaningful inflammatory cytokines and autoimmune thyroid disease in MR analysis.
Table 3 Effects of the relationship between autoimmune thyroid disease and meaningful inflammatory cytokines in reverse MR analysis.
In the MR analysis, the level of TNF-β was positively correlated with the risk of GD (OR, 1.115; 95% CI, 1.024–1.215; P = 0.013). However, the level of stem cell growth factor–β (SCGF-β) was negatively correlated with the risk of GD (OR, 0.913; 95% CI, 0.839–0.994; P = 0.035). Interestingly, no correlation was noted between the levels of inflammatory cytokines and GD following BH correction (Figure 2; Table 2).
Figure 2 Associations between genetically predicted inflammatory cytokines and Graves’ disease. SNPs, single-nucleotide polymorphisms; OR, odds ratio; 95% CI, 95% confidence interval; red means positive correlation; green means negative correlation.
Furthermore, the WME method did not detect a significant correlation between the level of tumor necrosis factor–β (TNF-β) (OR, 1.096; 95% CI, 0.981–1.225; P = 0.104) and SCG-β (OR, 0.989; 95% CI, 0.872–1.121; P = 0.857) on the risk of GD, but the direction of the effect was consistent with that of IVW (Table 2; Supplementary Figure 1). As anticipated, the MR-Egger regression intercept showed no evidence of pleiotropy between TNF-β, SCGF-β, and GD (intercept P = 0.511 for TNF-β and intercept P = 0.915 for SCGF-β). No outliers were detected by MR-PRESSO regression. Heterogeneity and sensitivity analysis results corroborated the accuracy of the results (Table 4). Lastly, the leave-one-out method further validated the robustness of the data (Supplementary Figure 5).
Table 4 Heterogeneity and sensitivity analysis of meaningful inflammatory cytokines and risk of autoimmune thyroid disease.
At the same time, high levels of IL-12p70 (OR, 1.088; 95% CI, 1.019–1.162, P = 0.012), IL-13 (OR, 1.055; 95% CI, 1.002–1.111, P = 0.041), and IFN-γ (OR, 1.150; 95% CI, 1.018–1.299; P = 0.025) were positively associated with the risk of HD. Whereas, high levels of monocyte chemotactic protein–1 (MCP-1) (OR, 0.930; 95% CI, 0.866–0.999; P = 0.047) and TNF-α (OR, 0.892; 95% CI, 0.812–0.981; P = 0.018) were negatively associated with the risk of HD, and no correlation between inflammatory cytokines and HD was found after BH correction (Figure 3; Table 2).
Figure 3 Associations between genetically predicted inflammatory cytokines and Hashimoto thyroiditis. SNPs, single-nucleotide polymorphisms; OR, odds ratio; 95% CI, 95% confidence interval; red means positive correlation; green means negative correlation.
WME method did not detect IL-12p70 (OR, 1.073; 95% CI, 0.990–1.162; P = 0.086), IL-13 (OR, 1.059; 95% CI, 0.9866–1.136, P = 0.115), IFN-γ (OR, 1.106; 95% CI, 0.935–1.308; P = 0.239), MCP-1 (OR, 0.917; 95% CI, 0.823–1.021; P = 0.113), TNF-α (OR, 0.887; 95% CI, 0.7855–1.003; P = 0.056) had a significant effect on the risk of HD, but the direction of effect was consistent with that of IVW (Table 2; Supplementary Figure 2). The MR-Egger regression intercept showed no evidence of pleiotropy between these inflammatory cytokines and AITD (intercept P = 0.656 for IL-12p70, intercept P = 0.759 for IL-13, intercept P = 0.533 for IFN-γ, intercept P = 0.330 for MCP-1, and intercept P = 0.444 for TNF-α). No outliers were detected by MR-PRESSO regression. Heterogeneity analysis results confirmed the accuracy of the results (Table 4). At the same time, leave-one-out method further verified the robustness of the data (Supplementary Figure 6).
In reverse MR analysis, IVW method revealed that GD is associated with higher levels of macrophage inflammatory protein–1β (MIP-1β) [Beat (β), 0.049; 95% CI, 0.010–0.088; P = 0.014], MCP-1 (β, 0.055; 95% CI, 0.019–0.092; P = 0.003), monokine induced by interferon-γ (MIG) (β, 0.080; 95% CI, 0.014–0.146; P = 0.018), interferon γ–induced protein 10 (IP-10) (β, 0.076; 95% CI, 0.008–0.145; P = 0.028), stromal cell–derived factor–1α (SDF-1α) (β, 0.042; 95% CI, 0.004–0.080; P = 0.030), platelet-derived growth factor BB (PDGFbb) (β, 0.052; 95% CI, 0.015–0.089; P = 0.006), β–nerve growth factor (βNGF) (β, 0.066; 95% CI, 0.006–0.126; P = 0.032), IL-2ra (β, 0.062; 95% CI, 0.007–0.117; P = 0.026), IL-4 (β, 0.054; 95% CI, 0.017–0.092; P = 0.004), and IL-17 (β, 0.044; 95% CI, 0.006–0.082; P = 0.025). On the other hand, no correlation was found between GD and inflammatory cytokines after BH correction (Figure 4; Table 3).
Figure 4 Associations between genetically predicted Graves’ disease and inflammatory cytokines. SNPs, single-nucleotide polymorphisms; 95% CI, 95% confidence interval; red means positive correlation.
WME exposed that the levels of MIP-1β (β, 0.055; 95% CI, 0.001–0.111; P = 0.054), MIG (β, 0.048; 95% CI, 0.032–0.128; P = 0.242), IP-10 (β, 0.049; 95% CI, 0.038–0.136; P = 0.269), PDGFbb (β, 0.047; 95% CI, 0.006–0.099; P = 0.081), and IL-2ra (β, 0.045; 95% CI, −0.031–0.122; P = 0.247) were significantly associated with the risk of GD. Similarly, there was a positive correlation between the risk and GD and the levels of MCP-1 (β, 0.063; 95% CI, 0.011–0.114; P = 0.017), SDF-1α (β, 0.060; 95% CI, 0.006–0.113; P = 0.029), βNGF (β, 0.140; 95% CI, 0.058–0.222; P = 0.001), IL-4 (β, 0.068; 95% CI, 0.017–0.119, P = 0.009), and IL-17 (β, 0.056; 95% CI = 0.001–0.110, P = 0.045) (Table 3; Supplementary Figure 3). At the same time, MR-Egger regression analysis displayed no evidence of directional pleiotropy (intercept P = 0.570 for MIP-1β, intercept P = 0.986 for MCP-1, intercept P = 0.054 for MIG, intercept P = 0.270 for IP-10, intercept P = 0.684 for SDF-1α, intercept P = 0.986 for MCP-1, intercept P = 0.054 for MIG, intercept P = 0.270 for IP-10, intercept P = 0.533 for PDGFbb, intercept P = 0.330 for βNGF, intercept P = 0.533 for IL-2ra, intercept P = 0.330 for IL-4, and intercept P = 0.444 for IL-17). No outliers were detected by MR-PRESSO regression. Heterogeneity analysis results confirmed the accuracy of the results (Table 5), whereas the leave-one-out method verified the robustness of the data (Supplementary Figure 7).
Table 5 Heterogeneity and sensitivity analysis of autoimmune thyroid disease with meaningful inflammatory cytokines.
In addition, we found that HD induced MIG (β, 0.234; 95% CI, 0.109–0.359; P = 2.35E-4), IL-2ra (β, 0.136; 95% CI, 0.035–0.236; P = 0.008), IP-10 (β, 0.183; 95% CI, 0.052–0.315; P = 0.006), and IL-16 (β, 0.124; 95% CI, 0.034–0.213; P = 0.007) increased levels. However, after BH correction, there was a significant positive correlation between HD risk and MIG expression level (PFDR = 0.010) (Figure 5; Table 3).
Figure 5 Associations between genetically predicted Hashimoto thyroiditis and inflammatory cytokines. SNPs, single-nucleotide polymorphisms; 95% CI, 95% confidence interval; red means positive correlation.
WME method showed that the risk of HD was associated with serum MIG (β, 0.152; 95% CI, 0.010–0.295; P = 0.037), IP-10 (β, 0.150; 95% CI, 0.010–0.290; P = 0.036), and IL-16 (β, 0.134; 95% CI, 0.009–0.259, P = 0.036) expression level was positively correlated with HD. No correlation was detected between HD risk and IL-2ra (β, 0.100; 95% CI, −0.033–0.234; P = 0.141) expression levels (Table 3; Supplementary Figure 4). In addition, the MR-Egger regression intercept showed no evidence of pleiotropy between HD and these circulating cytokines (intercept P = 0.353 for MIG, intercept P = 0.576 for IL-2ra, intercept P = 0.647 for IP-10, and intercept P = 0.640 for IL-16). No outliers were detected by MR-PRESSO regression. Heterogeneity analysis results confirmed the accuracy of the results (Table 5). At the same time, the remaining result further verified the robustness of the data (Supplementary Figure 8).
This study utilized MR to investigate the causal connection between AITD and inflammatory cytokines. To the best of our knowledge, this is the first study to uncover a causal association between the levels of circulating cytokines and AITD. Specifically, high levels of circulating TNF-β and low levels of SCGF-β were associated with a higher risk of GD. TGF-β belongs to the growth and transformational growth family (26) and plays a key role in the pathogenesis of GD (27, 28). In patients with GD, the TGF-β–stimulated humoral response of Th2 cells to infiltrate the thyroid gland leads to the synthesis of thyroid-stimulating hormone receptor (TSH) autoantibodies (TRAb), thereby promoting the development of GD (29–31). SCGF-β plays a vital role in promoting hematopoiesis and hematopoietic recovery. In tissues and organs beyond the bone marrow, SCGF-β can rapidly activate dormant stem cells and stimulate their growth, simultaneously regulating the internal microenvironment to create favorable growth conditions for stem cells (32, 33). Therefore, we postulate that the decrease in the level of SCGF-β may be associated with the inhibition of thyroid stem cell activity in patients with GD. Concurrently, patients with high circulating levels of IL-12p70, IL-13, and IFN-γ and low levels of MCP-1 and TNF-α are at a higher risk of developing HD. IL-12p70, also referred to as natural killer (NK) cell–stimulating factor, serves as a ligand for IL-12 (34). Its primary immunomodulatory function lies in inducing the differentiation of early helper T cells into Th1 cells, which, in turn, generate pro-inflammatory proteins that trigger macrophage activation, ultimately leading to the initiation of cytotoxic effects (35). Earlier studies have established that IL-12 can exacerbate cytotoxic effects in mice with autoimmune thyroiditis by eliciting a Th1 immune response (36). IL-13, a member of the interleukin family, is largely secreted by activated Th2 cells and plays a critical role in allergic inflammation (37). It promotes M2 polarization. Furthermore, IL-13 actively contributes to the survival, activation, and recruitment of eosinophils (38). Presently, studies investigating the association between IL-13 and AITD are scarce. Therefore, it is essential to undertake comprehensive studies in the future to elucidate this relationship. IFN-γ is the sole member of type II interferons and is produced not only by T cells, macrophages, and NK cells but also by thyroid cells (39). Its immunomodulatory effects include increased phagocytosis of macrophages, NK cell activation, enhancing the expression level of its antigens, and the toxicity of sensitized lymphocytes on target cells (9). Animal studies have documented that IFN-γ–induced apoptosis of thyroid cells is implicated in the pathogenesis of HT (40). Clinical studies further unveiled a significant rise in the number of IFN-γ+ cells in patients with HT (41).
MCP-1 belongs to the CC chemokine family and plays a fundamental role in the inflammatory process (42). Currently, numerous clinical studies have observed an elevated expression level of MCP-1 in both thyroid tissue and serum of patients with AITD (43, 44). Importantly, our study found that low levels of MCP-1 were associated with a higher risk of HD, whereas reverse MR analysis revealed up-regulated MCP-1 expression in patients with GD. TNF-α plays an instrumental role in modulating the inflammatory response, apoptosis, and immune cell activity. Additionally, it triggers the transcription and expression of a diverse array of cytokines, thereby facilitating their synthesis and release (45). Given its central role in the inflammatory response and immune regulation, researchers have developed numerous drugs targeting TNF-α for conditions such as rheumatoid arthritis (46) and Crohn’s disease (47). Notably, our study detected a negative association between TNF-α levels and HD. Further investigations with more extensive databases are warranted to validate this correlation.
Reverse MR analysis showed that GD can increase the blood levels of MIP-1β, MCP-1, MIG, IP-10, SDF-1α, PDGFbb, βNGF, IL-2ra, IL-4, and IL-17, whereas HD can increase the blood levels of MIG, IL-2ra, IP-10, and IL-16. After BH correction, a strong positive correlation was identified between the risk of HD and the expression level of MIG. MIP-1β is a CC chemokine that selectively binds to the CCR5 receptor. It chemotaxes NK cells, monocytes, and various other immune cells and can be generated by mast cells, endothelial cells, macrophages, and CD8+ T cells (48). Kemp et al. (46) observed an increased expression of MIP-1β in the thyroid tissue of patients with AITD, thereby confirming the significant role of MIP-1 in pro-inflammatory processes. MIG belongs to the glutamic acid-leucine-arginine (ELR)-negative CXC chemokine subfamily and can be triggered by IFN-γ (49). CXCL9 plays an instrumental role in modulating the immune system and promoting inflammation. Of note, the recruitment, transport, and maintenance of particular subgroups of activated lymphocytes are essential for initiating and sustaining AITD (50). The secretion of chemokines that bind to CXCR3 by thyroid cells is stimulated by IFN-γ, subsequently attracting Th1 lymphocytes that express CXCR3 and secreting IFN-γ (51). Disrupting MIG could alleviate inflammatory reactions. Therefore, we postulate that targeting MIG may constitute a therapeutic target for the treatment of AITD. IP-10 is a member of the CXC chemokine family (52) and shares receptors such as CXCR3 with MIG. Inflammatory cells, attracted by IP-10, include chemotactic T lymphocytes that infiltrate and proliferate, thereby mediating antibody-specific autoimmune responses and resulting in gland destruction (53). Romagnani et al. (54) documented that the expression of IP-10, MIG, and CXCR3 was downregulated or absent in healthy thyroid tissue, whereas chemokines and their receptors were abundant in the thyroid glands of the majority of patients with GD. IP-10 and MIG were localized in infiltrating lymphocytes, macrophages, and resident epithelial follicular cells, whereas CXCR3 was predominantly localized in infiltrating inflammatory cells. SDF-1α belongs to the α subfamily of chemokines. Acting as a ligand, it binds to the CXCR4 receptor. The SDF-1α/CXCR4 signaling pathway plays an essential role in various physiological processes, including cell transport, angiogenesis, embryogenesis, tumor invasion, and metastasis (55, 56). Consequently, existing studies predominantly focused on investigating the value of SDF-1α as a therapeutic target in thyroid malignancies. However, research addressing its role in AITD is limited (57, 58). PDGFbb participates in regulating cell growth and division. Following injury, platelets secrete PDGFbb, which facilitates the infiltration of neutrophils and macrophages. Simultaneously, PDGFbb promotes the secretion of a new extracellular matrix by fibroblasts and conduces to Insulin-like growth factor (IGF)-1–mediated reepithelialization (59, 60). Previous investigations have evinced that PDGFbb possesses the capability to induce orbital fibroblast proliferation, as well as the production of hyaluronic acid and cytokines in the orbital tissue of patients with Graves’ ophthalmopathy (61). βNGF primarily stimulates the growth, development, differentiation, and maturation of both central and peripheral neurons, concurrently upholding the physiological functioning of the nervous system (62). Simultaneously, it is involved in immunomodulation, directly influencing the innate and adaptive immune responses of B and T cells. Importantly, it induces the release of neuropeptides and neurotransmitters, thereby modulating immune system activation within inflammatory tissues (63). It is worthwhile mentioning that elevated levels of autoantibodies against βNGF were noted in the blood and tissues of individuals with AITD. Moreover, it has the potential to activate mast cells, triggering the release of inflammatory mediators, exacerbating the inflammatory state, and contributing to tissue damage in AITD (64, 65). The expression of IL-2ra is paramount for the proliferation and growth of T cells (66). Recent research has identified the occurrence of polymorphisms in this gene among patients with AITD (14), whereas Nakanishi et al. (67) observed elevated serum IL-2ra levels in patients with AITD. IL-4 is crucial for the development and function of helper Th2 cells (68). According to a previous study, the proportion of IL-4+ cells was increased within the thyroid tissues of HT patients (66), consistent with the results of our study. IL-17 serves as a primary effector of Th17 cells, playing a vital role in promoting their activation (69). Xue et al. (70) pointed out that the expression level of CD4+, IL-17+, and Th17 cells in the blood of untreated patients with AITD was higher than that of healthy individuals. Additionally, El-Zawawy et al. (71) reported a significant elevation in the expression level of serum IL-17A in patients with AITD compared to the healthy control group. IL-16 is a pro-inflammatory cytokine that exerts chemotactic effects on CD4 T lymphocytes, monocytes, and eosinophils, playing a role in both the inflammatory response and tumor development. Ongoing research has provided evidence of the expression of the IL-16 protein in the thyroid glands of patients diagnosed with GD and HT (72). Furthermore, existing evidence suggests that the expression levels of IL-16 in the serum could be a candidate marker for assessing disease activity and the severity of AITD (73).
To sum up, cytokines are involved in the pathogenesis of AITD (74). Their secretion profile can be either pro-inflammatory or anti-inflammatory and either pro-apoptotic or anti-apoptotic (75). These cytokines can participate in governing the immune system and the differentiation, growth, and secretory function of thyroid cells through their endocrine, autocrine, and paracrine modes. By modulating TSH levels, thyroid cells induce the abnormal expression of major histocompatibility complex–II antigens, which are transformed into antigen-presenting cells and resulting in autoimmune pathological damage and the development of AITD (76). Th1 lymphocytes generate pro-inflammatory proteins, including IFN-γ and IL-2, driving macrophage activation and inducing cytotoxic effects. On the other hand, Th2 lymphocytes generate anti-inflammatory proteins that suppress the production of Th1 cytokines. Additionally, they primarily stimulate B cells to generate antibodies and trigger the activation of anti-apoptotic molecules (77). Th17 lymphocytes release pro-inflammatory cytokines such as IL17 and exert a significant impact on persistent inflammation (78, 79).
While this study established a causal relationship and identified candidate targets for subsequent functional studies, it also has limitations that should not be overlooked. (1) This study used European population GWAS data for MR analysis, necessitating further studies in other populations. (2) Although MR is a highly efficient method for causality analysis, future animal tests are warranted to corroborate our findings. (3) The relationship between inflammatory cytokines and AITD is multifaceted, and elucidating the etiology and pathogenesis of circulating cytokines requires exploration from multiple aspects. (4) Ascribed to the complex etiology of AITD, circulating cytokines cannot fully explain the pathogenesis, necessitating more comprehensive data for further investigation.
In summary, the present study utilized the MR approach to investigate the causal association between inflammatory cytokines and AITD. Our results collectively unveiled that high levels of TNF-β and low levels of SCGF-β were associated with a high risk of developing GD. At the same time, high levels of IL-12p70, IL-13, and IFN-γ and low levels of MCP-1 and TNF-α suggest a higher risk of developing HD. Moreover, GD can increase the blood levels of MIP-1β, MCP-1, MIG, IP-10, SDF-1α, PDGFbb, βNGF, IL-2ra, IL-4, and IL-17, whereas HD can lead to elevated levels of MIG, IL-2ra, IP-10, and IL-16 levels. These cytokines hold significant implications for noninvasive early diagnosis of AITD. Finally, these cytokines may represent novel targets for the prevention, treatment, and long-term management of AITD.
The original contributions presented in the study are included in the article/Supplementary Material. Further inquiries can be directed to the corresponding authors.
Since the data used are publicly available in the database, no additional ethical approval was needed in this case.
ZY: Investigation, Methodology, Project administration, Resources, Funding acquisition, Writing – review & editing. FG: Software, Supervision, Validation, Visualization, Writing – original draft. YT: Conceptualization, Methodology, Resources, Validation, Writing – original draft. YZ: Project administration, Resources, Software, Supervision, Writing – review & editing. YG: Conceptualization, Data curation, Project administration, Writing – original draft. GY: Data curation, Investigation, Project administration, Visualization, Writing – review & editing. SW: Conceptualization, Data curation, Formal analysis, Funding acquisition, Writing – original draft, Writing – review & editing.
The author(s) declare financial support was received for the research, authorship, and/or publication of this article. This work was supported by the Projects of Binzhou Technology Development Program (BY2020KJ27) and Shandong Province medical health science and technology project (202304011426) to FG. Shandong Province medical health science and technology project (202307010965) and Science and Technology Innovation Plan of Medical Staff in Shandong Province(SDYWZGKCJHLH2023096) to ZY. The funding bodies played no role in the study design, data collection, analysis,interpretation, or manuscript writing.
The authors declare that the research was conducted in the absence of any commercial or financial relationships that could be construed as a potential conflict of interest.
All claims expressed in this article are solely those of the authors and do not necessarily represent those of their affiliated organizations, or those of the publisher, the editors and the reviewers. Any product that may be evaluated in this article, or claim that may be made by its manufacturer, is not guaranteed or endorsed by the publisher.
The Supplementary Material for this article can be found online at: https://www.frontiersin.org/articles/10.3389/fimmu.2024.1334772/full#supplementary-material
Supplementary Table 1 | The F number and R2 detect the intensity of the IVs between 41 Inflammatory cytokines and GD in MR analyses. GD, Graves’ Disease; IVs, instrumental variables.
Supplementary Table 2 | The F number and R2 detect the intensity of the IVs between GD and 41 Inflammatory cytokines in MR analyses. GD, Graves’ Disease; IVs, instrumental variables.
Supplementary Table 3 | The F number and R2 detect the intensity of the IVs between 41 Inflammatory cytokines and HT in MR analyses. HT, Hashimoto thyroiditis; IVs, instrumental variables.
Supplementary Table 4 | The F number and R2 detect the intensity of the IVs between HT and 41 Inflammatory cytokines in MR analyses. HT, Hashimoto thyroiditis; IVs, instrumental variables.
1. Mammen JSR, Cappola AR. Autoimmune thyroid disease in women. Jama. (2021) 325:2392–3. doi: 10.1001/jama.2020.22196
2. Conrad N, Misra S, Verbakel JY, Verbeke G, Molenberghs G, Taylor PN, et al. Incidence, prevalence, and co-occurrence of autoimmune disorders over time and by age, sex, and socioeconomic status: a population-based cohort study of 22 million individuals in the UK. Lancet. (2023) 401:1878–90. doi: 10.1016/s0140-6736(23)00457-9
3. Antonelli A, Ferrari SM, Corrado A, Di Domenicantonio A, Fallahi P. Autoimmune thyroid disorders. Autoimmun Rev. (2015) 14:174–80. doi: 10.1016/j.autrev.2014.10.016
4. Milo T, Korem Kohanim Y, Toledano Y, Alon U. Autoimmune thyroid diseases as a cost of physiological autoimmune surveillance. Trends Immunol. (2023) 44:365–71. doi: 10.1016/j.it.2023.03.007
5. Radziszewski M, Kuś A, Bednarczuk T. Genotype-phenotype correlations in Graves' disease. Best Pract Res Clin Endocrinol Metab. (2023) 37:101745. doi: 10.1016/j.beem.2023.101745
6. Vargas-Uricoechea H. Molecular mechanisms in autoimmune thyroid disease. Cells. (2023) 12. doi: 10.3390/cells12060918
7. Liu C, Chu D, Kalantar-Zadeh K, George J, Young HA, Liu G. Cytokines: from clinical significance to quantification. Adv Sci (Weinh). (2021) 8:e2004433. doi: 10.1002/advs.202004433
8. Jarczak D, Nierhaus A. Cytokine storm-definition, causes, and implications. Int J Mol Sci. (2022) 23. doi: 10.3390/ijms231911740
9. Ferrari SM, Paparo SR, Ragusa F, Elia G, Mazzi V, Patrizio A, et al. Chemokines in thyroid autoimmunity. Best Pract Res Clin Endocrinol Metab. (2023) 37:101773. doi: 10.1016/j.beem.2023.101773
10. Xiaoheng C, Yizhou M, Bei H, Huilong L, Xin W, Rui H, et al. General and specific genetic polymorphism of cytokines-related gene in AITD. Mediators Inflamm. (2017) 2017:3916395. doi: 10.1155/2017/3916395
11. Vasu C, Holterman MJ, Prabhakar BS. Modulation of dendritic cell function and cytokine production to prevent thyroid autoimmunity. Autoimmunity. (2003) 36:389–96. doi: 10.1080/08916930310001603073
12. Li Q, Wang B, Mu K, Zhang JA. The pathogenesis of thyroid autoimmune diseases: New T lymphocytes - Cytokines circuits beyond the Th1-Th2 paradigm. J Cell Physiol. (2019) 234:2204–16. doi: 10.1002/jcp.27180
13. Wang Y, Fang S, Zhou H. Pathogenic role of Th17 cells in autoimmune thyroid disease and their underlying mechanisms. Best Pract Res Clin Endocrinol Metab. (2023) 37:101743. doi: 10.1016/j.beem.2023.101743
14. Hwangbo Y, Park YJ. Genome-wide association studies of autoimmune thyroid diseases, thyroid function, and thyroid cancer. Endocrinol Metab (Seoul). (2018) 33:175–84. doi: 10.3803/EnM.2018.33.2.175
15. Burgess S, Daniel RM, Butterworth AS, Thompson SG. Network Mendelian randomization: using genetic variants as instrumental variables to investigate mediation in causal pathways. Int J Epidemiol. (2015) 44:484–95. doi: 10.1093/ije/dyu176
16. Lawlor DA, Harbord RM, Sterne JA, Timpson N, Davey Smith G. Mendelian randomization: using genes as instruments for making causal inferences in epidemiology. Stat Med. (2008) 27:1133–63. doi: 10.1002/sim.3034
17. Ahola-Olli AV, Würtz P, Havulinna AS, Aalto K, Pitkänen N, Lehtimäki T, et al. Genome-wide association study identifies 27 loci influencing concentrations of circulating cytokines and growth factors. Am J Hum Genet. (2017) 100:40–50. doi: 10.1016/j.ajhg.2016.11.007
18. Sakaue S, Kanai M, Tanigawa Y, Karjalainen J, Kurki M, Koshiba S, et al. A cross-population atlas of genetic associations for 220 human phenotypes. Nat Genet. (2021) 53:1415–24. doi: 10.1038/s41588-021-00931-x
19. Boehm FJ, Zhou X. Statistical methods for Mendelian randomization in genome-wide association studies: A review. Comput Struct Biotechnol J. (2022) 20:2338–51. doi: 10.1016/j.csbj.2022.05.015
20. Burgess S, Thompson SG. Interpreting findings from Mendelian randomization using the MR-Egger method. Eur J Epidemiol. (2017) 32:377–89. doi: 10.1007/s10654-017-0255-x
21. Bowden J, Davey Smith G, Haycock PC, Burgess S. Consistent estimation in mendelian randomization with some invalid instruments using a weighted median estimator. Genet Epidemiol. (2016) 40:304–14. doi: 10.1002/gepi.21965
22. Palmer TM, Lawlor DA, Harbord RM, Sheehan NA, Tobias JH, Timpson NJ, et al. Using multiple genetic variants as instrumental variables for modifiable risk factors. Stat Methods Med Res. (2012) 21:223–42. doi: 10.1177/0962280210394459
23. Meng C, Deng P, Miao R, Tang H, Li Y, Wang J, et al. Gut microbiome and risk of ischaemic stroke: a comprehensive Mendelian randomization study. Eur J Prev Cardiol. (2023) 30:613–20. doi: 10.1093/eurjpc/zwad052
24. Greco MF, Minelli C, Sheehan NA, Thompson JR. Detecting pleiotropy in Mendelian randomisation studies with summary data and a continuous outcome. Stat Med. (2015) 34:2926–40. doi: 10.1002/sim.6522
25. Bowden J, Davey Smith G, Burgess S. Mendelian randomization with invalid instruments: effect estimation and bias detection through Egger regression. Int J Epidemiol. (2015) 44:512–25. doi: 10.1093/ije/dyv080
26. Hinck AP, Mueller TD, Springer TA. Structural biology and evolution of the TGF-β Family. Cold Spring Harb Perspect Biol. (2016) 8. doi: 10.1101/cshperspect.a022103
27. Bidey SP, Hill DJ, Eggo MC. Growth factors and goitrogenesis. J Endocrinol. (1999) 160:321–32. doi: 10.1677/joe.0.1600321
28. Stassi G, De Maria R. Autoimmune thyroid disease: new models of cell death in autoimmunity. Nat Rev Immunol. (2002) 2:195–204. doi: 10.1038/nri750
29. Vander Ark A, Cao J, Li X. TGF-β receptors: In and beyond TGF-β signaling. Cell Signal. (2018) 52:112–20. doi: 10.1016/j.cellsig.2018.09.002
30. Derynck R, Zhang YE. Smad-dependent and Smad-independent pathways in TGF-beta family signalling. Nature. (2003) 425:577–84. doi: 10.1038/nature02006
31. Widder J, Dorfinger K, Wilfing A, Trieb K, Pirich K, Loebenstein R, et al. The immunoregulatory influence of transforming growth factor beta in thyroid autoimmunity: TGF beta inhibits autoreactivity in Graves' disease. J Autoimmun. (1991) 4:689–701. doi: 10.1016/0896-8411(91)90186-g
32. Schiro A, Wilkinson FL, Weston R, Smyth JV, Serracino-Inglott F, Alexander MY. Elevated levels of endothelial-derived microparticles, and serum CXCL9 and SCGF-β are associated with unstable asymptomatic carotid plaques. Sci Rep. (2015) 5:16658. doi: 10.1038/srep16658
33. Chen Z, Hu Z, Hu Y, Sheng Y, Li Y, Song J. Novel potential biomarker of adult cardiac surgery-associated acute kidney injury. Front Physiol. (2020) 11:587204. doi: 10.3389/fphys.2020.587204
34. Vignali DA, Kuchroo VK. IL-12 family cytokines: immunological playmakers. Nat Immunol. (2012) 13:722–8. doi: 10.1038/ni.2366
35. Inoue N, Watanabe M, Nakaguchi A, Ueda D, Kawaguti H, Hidaka Y, et al. Functional polymorphisms affecting Th1 differentiation are associated with the severity of autoimmune thyroid diseases. Endocr J. (2017) 64:695–703. doi: 10.1507/endocrj.EJ16-0551
36. Zhang W, Flynn JC, Kong YC. IL-12 prevents tolerance induction with mouse thyroglobulin by priming pathogenic T cells in experimental autoimmune thyroiditis: role of IFN-gamma and the costimulatory molecules CD40l and CD28. Cell Immunol. (2001) 208:52–61. doi: 10.1006/cimm.2001.1767
37. Matera MG, Ora J, Calzetta L, Rogliani P, Cazzola M. Investigational anti IL-13 asthma treatments: a 2023 update. Expert Opin Investig Drugs. (2023) 32:373–86. doi: 10.1080/13543784.2023.2215425
38. Shankar A, McAlees JW, Lewkowich IP. Modulation of IL-4/IL-13 cytokine signaling in the context of allergic disease. J Allergy Clin Immunol. (2022) 150:266–76. doi: 10.1016/j.jaci.2022.06.012
39. Thuner J, Coutant F. IFN-γ: An overlooked cytokine in dermatomyositis with anti-MDA5 antibodies. Autoimmun Rev. (2023) 22:103420. doi: 10.1016/j.autrev.2023.103420
40. Salzano M, Russo E, Postiglione L, Guerra A, Marotta V, Esposito S, et al. Interferon-γ inhibits integrin-mediated adhesion to fibronectin and survival signaling in thyroid cells. J Endocrinol. (2012) 215:439–44. doi: 10.1530/joe-12-0335
41. Bossowski A, Harasymczuk J, Moniuszko A, Bossowska A, Hilczer M, Ratomski K. Cytometric evaluation of intracellular IFN-γ and IL-4 levels in thyroid follicular cells from patients with autoimmune thyroid diseases. Thyroid Res. (2011) 4:13. doi: 10.1186/1756-6614-4-13
42. Emi Aikawa N, de Carvalho JF, Artur Almeida Silva C, Bonfá E. Immunogenicity of Anti-TNF-alpha agents in autoimmune diseases. Clin Rev Allergy Immunol. (2010) 38:82–9. doi: 10.1007/s12016-009-8140-3
43. Jin J, Chang Y, Wei W. Clinical application and evaluation of anti-TNF-alpha agents for the treatment of rheumatoid arthritis. Acta Pharmacol Sin. (2010) 31:1133–40. doi: 10.1038/aps.2010.134
44. van Hogezand RA, Verspaget HW. The future role of anti-tumour necrosis factor-alpha products in the treatment of Crohn's disease. Drugs. (1998) 56:299–305. doi: 10.2165/00003495-199856030-00001
45. Singh S, Anshita D, Ravichandiran V. MCP-1: Function, regulation, and involvement in disease. Int Immunopharmacol. (2021) 101:107598. doi: 10.1016/j.intimp.2021.107598
46. Kemp EH, Metcalfe RA, Smith KA, Woodroofe MN, Watson PF, Weetman AP. Detection and localization of chemokine gene expression in autoimmune thyroid disease. Clin Endocrinol (Oxf). (2003) 59:207–13. doi: 10.1046/j.1365-2265.2003.01824.x
47. Ozisik H, Cekin A, Suner A, Durmaz B, Ozel B, Gunel NS, et al. Evaluation of IL-10, MCP-1, IFN gamma, and protectin D1 levels in patients with Hashimoto’s thyroiditis. Ir J Med Sci. (2023) 192:177–84. doi: 10.1007/s11845-022-03231-3
48. Maurer M, von Stebut E. Macrophage inflammatory protein-1. Int J Biochem Cell Biol. (2004) 36:1882–6. doi: 10.1016/j.biocel.2003.10.019
49. Irving SG, Zipfel PF, Balke J, McBride OW, Morton CC, Burd PR, et al. Two inflammatory mediator cytokine genes are closely linked and variably amplified on chromosome 17q. Nucleic Acids Res. (1990) 18:3261–70. doi: 10.1093/nar/18.11.3261
50. Ferrari SM, Fallahi P, Elia G, Ragusa F, Camastra S, Paparo SR, et al. Novel therapies for thyroid autoimmune diseases: An update. Best Pract Res Clin Endocrinol Metab. (2020) 34:101366. doi: 10.1016/j.beem.2019.101366
51. Rotondi M, Chiovato L. The chemokine system as a therapeutic target in autoimmune thyroid diseases: a focus on the interferon-γ inducible chemokines and their receptor. Curr Pharm Des. (2011) 17:3202–16. doi: 10.2174/138161211798157559
52. Loetscher M, Gerber B, Loetscher P, Jones SA, Piali L, Clark-Lewis I, et al. Chemokine receptor specific for IP10 and mig: structure, function, and expression in activated T-lymphocytes. J Exp Med. (1996) 184:963–9. doi: 10.1084/jem.184.3.963
53. García-López MA, Sancho D, Sánchez-Madrid F, Marazuela M. Thyrocytes from autoimmune thyroid disorders produce the chemokines IP-10 and Mig and attract CXCR3+ lymphocytes. J Clin Endocrinol Metab. (2001) 86:5008–16. doi: 10.1210/jcem.86.10.7953
54. Romagnani P, Rotondi M, Lazzeri E, Lasagni L, Francalanci M, Buonamano A, et al. Expression of IP-10/CXCL10 and MIG/CXCL9 in the thyroid and increased levels of IP-10/CXCL10 in the serum of patients with recent-onset Graves’ disease. Am J Pathol. (2002) 161:195–206. doi: 10.1016/s0002-9440(10)64171-5
55. Ghadge SK, Mühlstedt S, Ozcelik C, Bader M. SDF-1α as a therapeutic stem cell homing factor in myocardial infarction. Pharmacol Ther. (2011) 129:97–108. doi: 10.1016/j.pharmthera.2010.09.011
56. Meng Z, Feng G, Hu X, Yang L, Yang X, Jin Q. SDF factor-1α Promotes the migration, proliferation, and osteogenic differentiation of mouse bone marrow mesenchymal stem cells through the wnt/β-catenin pathway. Stem Cells Dev. (2021) 30:106–17. doi: 10.1089/scd.2020.0165
57. Werner TA, Forster CM, Dizdar L, Verde PE, Raba K, Schott M, et al. CXCR4/CXCR7/CXCL12-axis in follicular thyroid carcinoma. J Cancer. (2018) 9:929–40. doi: 10.7150/jca.23042
58. Castellone MD, Guarino V, De Falco V, Carlomagno F, Basolo F, Faviana P, et al. Functional expression of the CXCR4 chemokine receptor is induced by RET/PTC oncogenes and is a common event in human papillary thyroid carcinomas. Oncogene. (2004) 23:5958–67. doi: 10.1038/sj.onc.1207790
59. Hoch RV, Soriano P. Roles of PDGF in animal development. Development. (2003) 130:4769–84. doi: 10.1242/dev.00721
60. Andrae J, Gallini R, Betsholtz C. Role of platelet-derived growth factors in physiology and medicine. Genes Dev. (2008) 22:1276–312. doi: 10.1101/gad.1653708
61. Virakul S, van Steensel L, Dalm VA, Paridaens D, van Hagen PM, Dik WA. Platelet-derived growth factor: a key factor in the pathogenesis of graves' ophthalmopathy and potential target for treatment. Eur Thyroid J. (2014) 3:217–26. doi: 10.1159/000367968
62. Berry A, Bindocci E, Alleva E. NGF, brain and behavioral plasticity. Neural Plast. (2012) 2012:784040. doi: 10.1155/2012/784040
63. Terracina S, Ferraguti G, Tarani L, Fanfarillo F, Tirassa P, Ralli M, et al. Nerve growth factor and autoimmune diseases. Curr Issues Mol Biol. (2023) 45:8950–73. doi: 10.3390/cimb45110562
64. Dicou E, Nerrière V. Evidence that natural autoantibodies against the nerve growth factor (NGF) may be potential carriers of NGF. J Neuroimmunol. (1997) 75:200–3. doi: 10.1016/s0165-5728(97)00008-8
65. Dicou E, Masson C, Jabbour W, Nerriere V. Increased frequency of NGF in sera of rheumatoid arthritis and systemic lupus erythematosus patients. Neuroreport. (1993) 5:321–4. doi: 10.1097/00001756-199312000-00036
66. Zwirska-Korczala K, Berdowska A, Jochem J, Sitkiewicz A, Birkner E, Polaniak R, et al. Influence of thyroxine on serum soluble interleukin-2 receptor alpha levels in thyroid disorders. J Clin Pharm Ther. (2004) 29:151–6. doi: 10.1111/j.1365-2710.2004.00547.x
67. Chistiakov DA, Chistiakova EI, Voronova NV, Turakulov RI, Savost’anov KV. A variant of the Il2ra / Cd25 gene predisposing to graves’ disease is associated with increased levels of soluble interleukin-2 receptor. Scand J Immunol. (2011) 74:496–501. doi: 10.1111/j.1365-3083.2011.02608.x
68. Keegan AD, Zamorano J. Regulation of gene expression, growth, and cell survival by IL-4: contribution of multiple signaling pathways. Cell Res. (1998) 8:1–13. doi: 10.1038/cr.1998.1
69. Huangfu L, Li R, Huang Y, Wang S. The IL-17 family in diseases: from bench to bedside. Signal Transduct Target Ther. (2023) 8:402. doi: 10.1038/s41392-023-01620-3
70. Xue H, Yu X, Ma L, Song S, Li Y, Zhang L, et al. The possible role of CD4+CD25(high)Foxp3+/CD4+IL-17A+ cell imbalance in the autoimmunity of patients with Hashimoto thyroiditis. Endocrine. (2015) 50:665–73. doi: 10.1007/s12020-015-0569-y
71. El-Zawawy HT, Farag HF, Tolba MM, Abdalsamea HA. Improving Hashimoto's thyroiditis by eradicating Blastocystis hominis: Relation to IL-17. Ther Adv Endocrinol Metab. (2020) 11:2042018820907013. doi: 10.1177/2042018820907013
72. Kemp EH, Ajjan RA, Metcalfe RA, Watson PF, Weetman AP. IL-14 and IL-16 are expressed in the thyroid of patients with either Graves’ disease or Hashimoto’s thyroiditis. Clin Endocrinol (Oxf). (2015) 83:726–32. doi: 10.1111/cen.12810
73. Gu X, Zheng L, Chen X, Ruan L, Zhang H, Ge S, et al. Elevated serum IL-16 and RANTES levels in patients with autoimmune thyroid diseases and modulation by methimazole therapy. Horm Metab Res. (2012) 44:482–7. doi: 10.1055/s-0032-1308973
74. Ferrari SM, Fallahi P, Elia G, Ragusa F, Ruffilli I, Paparo SR, et al. Thyroid autoimmune disorders and cancer. Semin Cancer Biol. (2020) 64:135–46. doi: 10.1016/j.semcancer.2019.05.019
75. Ramani T, Auletta CS, Weinstock D, Mounho-Zamora B, Ryan PC, Salcedo TW, et al. Cytokines: the good, the bad, and the deadly. Int J Toxicol. (2015) 34:355–65. doi: 10.1177/1091581815584918
76. Kong YC, Lomo LC, Motte RW, Giraldo AA, Baisch J, Strauss G, et al. HLA-DRB1 polymorphism determines susceptibility to autoimmune thyroiditis in transgenic mice: definitive association with HLA-DRB1*0301 (DR3) gene. J Exp Med. (1996) 184:1167–72. doi: 10.1084/jem.184.3.1167
77. Berger A. Th1 and Th2 responses: what are they? Bmj. (2000) 321:424. doi: 10.1136/bmj.321.7258.424
78. Prud'homme GJ, Piccirillo CA. The inhibitory effects of transforming growth factor-beta-1 (TGF-beta1) in autoimmune diseases. J Autoimmun. (2000) 14:23–42. doi: 10.1006/jaut.1999.0339
Keywords: inflammatory cytokines, autoimmune thyroid disease, causality, GWAS, Mendelian randomization
Citation: Yao Z, Guo F, Tan Y, Zhang Y, Geng Y, Yang G and Wang S (2024) Causal relationship between inflammatory cytokines and autoimmune thyroid disease: a bidirectional two-sample Mendelian randomization analysis. Front. Immunol. 15:1334772. doi: 10.3389/fimmu.2024.1334772
Received: 07 November 2023; Accepted: 27 February 2024;
Published: 20 March 2024.
Edited by:
Marisa Mariel Fernandez, Universidad de Buenos Aires, ArgentinaReviewed by:
Evie Melanitou, Institut Pasteur, FranceCopyright © 2024 Yao, Guo, Tan, Zhang, Geng, Yang and Wang. This is an open-access article distributed under the terms of the Creative Commons Attribution License (CC BY). The use, distribution or reproduction in other forums is permitted, provided the original author(s) and the copyright owner(s) are credited and that the original publication in this journal is cited, in accordance with accepted academic practice. No use, distribution or reproduction is permitted which does not comply with these terms.
*Correspondence: Song Wang, d3MxNzg2NTIwMDYxMUAxNjMuY29t; Guang Yang, bHlmeWcxMjFAMTYzLmNvbQ==; Zhiwei Yao, eXp3MTY4QHRtdS5lZHUuY24=
†These authors have contributed equally to this work
Disclaimer: All claims expressed in this article are solely those of the authors and do not necessarily represent those of their affiliated organizations, or those of the publisher, the editors and the reviewers. Any product that may be evaluated in this article or claim that may be made by its manufacturer is not guaranteed or endorsed by the publisher.
Research integrity at Frontiers
Learn more about the work of our research integrity team to safeguard the quality of each article we publish.