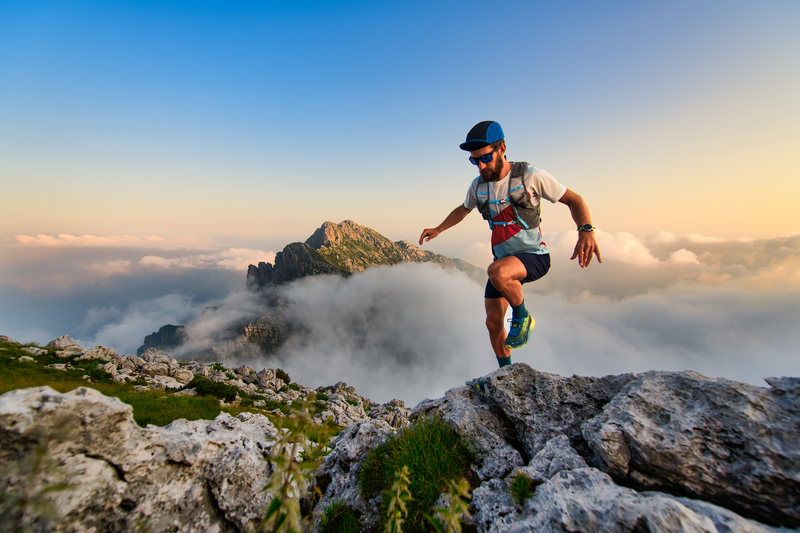
95% of researchers rate our articles as excellent or good
Learn more about the work of our research integrity team to safeguard the quality of each article we publish.
Find out more
ORIGINAL RESEARCH article
Front. Immunol. , 12 February 2024
Sec. Mucosal Immunity
Volume 15 - 2024 | https://doi.org/10.3389/fimmu.2024.1330991
This article is part of the Research Topic Small non-coding RNAs in Gram negative bacteria View all 5 articles
Bronchiolitis, a viral lower respiratory infection, is the leading cause of infant hospitalization, which is associated with an increased risk for developing asthma later in life. Bronchiolitis can be caused by several respiratory viruses, such as respiratory syncytial virus (RSV), rhinovirus (RV), and others. It can also be caused by a solo infection (e.g., RSV- or RV-only bronchiolitis) or co-infection with two or more viruses. Studies have shown viral etiology-related differences between RSV- and RV-only bronchiolitis in the immune response, human microRNA (miRNA) profiles, and dominance of certain airway microbiome constituents. Here, we identified bacterial small RNAs (sRNAs), the prokaryotic equivalent to eukaryotic miRNAs, that differ between infants of the 35th Multicenter Airway Research Collaboration (MARC-35) cohort with RSV- versus RV-only bronchiolitis. We first derived reference sRNA datasets from cultures of four bacteria known to be associated with bronchiolitis (i.e., Haemophilus influenzae, Moraxella catarrhalis, Moraxella nonliquefaciens, and Streptococcus pneumoniae). Using these reference sRNA datasets, we found several sRNAs associated with RSV- and RV-only bronchiolitis in our human nasal RNA-Seq MARC-35 data. We also determined potential human transcript targets of the bacterial sRNAs and compared expression of the sRNAs between RSV- and RV-only cases. sRNAs are known to downregulate their mRNA target, we found that, compared to those associated with RV-only bronchiolitis, sRNAs associated with RSV-only bronchiolitis may relatively activate the IL-6 and IL-8 pathways and relatively inhibit the IL-17A pathway. These data support that bacteria may be contributing to inflammation differences seen in RSV- and RV-only bronchiolitis, and for the first time indicate that the potential mechanism in doing so may be through bacterial sRNAs.
Bronchiolitis is a viral lower respiratory infection that manifests as typical cold symptoms (sneezing, coughing, wheezing, fever) (1). Globally, lower respiratory infections are the leading cause of death among young children (<5 years old), accounting for approximately 900,000 deaths annually (2). Bronchiolitis is the leading cause of hospitalization among U.S. infants (2), resulting in about 130,000 hospitalizations every year (3). The effects of bronchiolitis can linger well after recovery; approximately 30% of infants who are hospitalized for bronchiolitis subsequently develop childhood asthma years after hospitalization (4, 5). The 35th Multicenter Airway Research Collaboration (MARC-35) has enrolled over 1,000 infants hospitalized for severe bronchiolitis order to identify risk factors during hospitalization and individuals at a higher risk for developing asthma at 5-years-old and 6-years-old.
Bronchiolitis can be caused by a variety of viruses but respiratory syncytial virus (RSV) and rhinovirus (RV) are two of the most common etiologies (6–8). Although bronchiolitis is considered one disease, there are differences in human metabolites (9), microRNA (miRNA) (10), DNA methylation (11), and immune responses (12–14) depending on virus—specifically, if RSV is the only detected infecting virus (RSV-only bronchiolitis) or if the only detected virus is RV (RV-only bronchiolitis).
Despite bronchiolitis being a viral disease, certain respiratory bacteria such as Haemophilus influenzae, Moraxella catarrhalis, Moraxella nonliquefaciens, and Streptococcus pneumoniae are also involved (8, 9, 15–18). Specifically, bronchiolitis caused by RSV tends to be associated with S. pneumoniae dominance among other microbiome constituents, while bronchiolitis caused by RV is frequently associated with H. influenzae dominance (9, 10). However, the mechanisms by which these bacteria affect bronchiolitis are unknown. While both human metabolites and human miRNAs differed by etiology, bacterial metabolites were not found to be different (9, 10). Additionally, the bacterial analog of miRNAs, small RNAs (sRNAs), were not addressed in these studies. In the present study, we sought to determine if there were any bacterial sRNAs associated with the viral etiology of bronchiolitis.
Bacteria produce regulatory RNAs called small RNAs (sRNAs) which can negatively regulate target transcripts (19, 20). These functional sRNAs can be shuttled between cells through extracellular vesicles (EVs), similar to the transport of eukaryotic miRNAs. These bacterial sRNAs in EVs have been shown not only to be taken up by mammalian cells, but also to be functionally intact after uptake, impact target cell gene expression, and even be incorporated into mammalian miRNA machinery (21–23). While sRNAs from opportunistic airway and periodontal bacteria have been implicated in altering the host immune system (22, 23), it has not been previously described for these species in bronchiolitis.
Herein, we found both previously identified and novel bacterial sRNAs in nasal swab samples from infants with severe bronchiolitis (i.e., bronchiolitis requiring hospitalization) and determined their associations with virus etiology. We hypothesized that S. pneumoniae sRNAs would be associated with RSV-only cases and H. influenzae sRNAs would be associated with RV-only cases.
We examined nasal swab RNA-Seq data collected from 589 infants with severe bronchiolitis who were part of the MARC-35 cohort-a multicenter prospective cohort study-comprised of 1,016 infants. These infants (aged <12 months) were enrolled during three consecutive bronchiolitis seasons from 2011-2014, as described previously (10, 24). At index hospitalization, trained investigators collected nasal swab samples using a standardized protocol (25) within the first day of hospitalization, as described previously (10). Nasal swab samples were stored at -80°C until RNA was isolated.
Total RNA, including small RNA, was isolated from the nasal swab samples using Trizol LS reagent (ThermoFisher Scientific, Waltham, USA) in combination with the Direct-zol RNA Miniprep Kit (Zymo Research, Irvine, USA). RNA quantity was measured with the Qubit 2.0 fluorometer (ThermoFisher Scientific) and RNA quality was assessed with the Agilent Bioanalyzer 2100 (Agilent, Palo Alto, USA) using the RNA 6000 Nano kit.
The type strains of each of the following bacteria were purchased from American Type Culture Collect (Manassas, USA): H. influenzae (ATCC 33391) (NCBI RefSeq Assembly Accession GCF_001457655.1), M. catarrhalis (ATCC 25238) (GCF_001679005.1), M. nonliquefaciens (ATCC 19975) (GCF_900476075.1), and S. pneumoniae (ATCC 33400) (GCF_001457635.1). Each species was rehydrated with 7mL of its corresponding broth media (below), incubated overnight in a 37°C, shaking incubator at 225 rotations per minutes (rpm), and stored at -20°C with 15% glycerol.
ATCC 814 GC agar for H. influenzae was made with the following ingredients: Dehydrated Culture Media: Gonococcal (GC) Medium Base (Thermo Fisher Scientific, DF0289-17-3), BBL freeze-dried hemoglobin bovine culture media (VWR International, Radnor, USA; 90000-662), and IsoVitaleX Enrichment (Thermo Fisher Scientific, B11876). The medium base and hemoglobin were autoclaved separately (121°C, liquid cycle). When the solutions were cool, the latter solution was mixed into former, along with rehydrated IsoVitaleX, and poured into in 60x15mm petri dishes (VWR International, 25384). Brain heart infusion (BHI) agar (VWR, 90003-040) was made for M. catarrhalis and S. pneumoniae, set in the same petri dishes. 5% rabbit blood agars (Thermo Fisher Scientific, R01210) were purchased for M. nonliquefaciens.
BHI broth (VWR International, 90000-066) was made for each of the bacteria with the following additions: that for M. nonliquefaciens was supplemented with 0.7μL beta-nicotinamide adenine diphosphate (Sigma Aldrich, N8285) to every 10mL of broth; that for H. influenzae was supplemented with the same amount of beta- nicotinamide adenine diphosphate in addition to 10mL IsoVitaleX to 1L of broth.
Bacterial stock solutions (100-200μL) were inoculated on their respective agar plates and grown for 24-48h (37°C, ambient air). Colony forming units from the agars were collected and used to inoculate respective broth media (50-75mL), which were grown for another 18-24h (37°C, ambient air, shaking at 225rpm).
Broth media were centrifuged at 4,300g for 35 minutes at 4°C to pellet the bacterial cells but leave the bacterial EVs in suspension. The supernatant was separated from the cell pellet by decanting. The cell pellet was resuspended in 250μL PBS.
The supernatant was concentrated to 1-2mL via centrifuging at 4,300g for 35 minutes to 1 hour in 3kDa Amicon Ultra-15 Centrifugal Filter Units (EMD Millipore, Burlington, USA; UFC900324). We added ExoQuick (System Biosciences LLC, Palo Alto, CA; EXOQ20A-1) in a 1:4 (v/v) ratio, incubated at 4°C for 30 minutes, and centrifuged at 1,500g for 30 minutes to precipitate EVs, and resuspended them in 500μL PBS. We used qEV Original resin columns (Izon Science, Christchurch, New Zealand; ICO-35) and the Automatic Fraction Collector (Izon Science, AFC-V1) to purify the EVs, and combined the first three output fractions (total volume = 1.5mL). We again added ExoQuick in a 1:4 (v/v) ratio to these combined fractions incubated at 4°C for 30 minutes, centrifuged at 1,500g for 30 minutes to pellet EVs, and resuspended them in 250μL PBS.
RNA was extracted using a Direct-zol Miniprep kit (Zymo Research, R2053) following the manufacturer’s instructions. Each of the three RNA isolations was performed in triplicate. For EVs and cells of H. influenzae, M. catarrhalis, and M. nonliquefaciens, each of the RNA triplicates was combined across three experiments, ending with three biological RNA replicates for each species. The same procedure was followed for the cells of S. pneumoniae, but for S. pneumoniae EVs, the biological replicates across three experiments were combined and then re-aliquoted into three technical replicates as the yields were non-uniform from the biological replicates.
All RNA samples were prepared for small RNA-Seq using the PerkinElmer NEXTFLEX® small RNA-Seq v3 kit with Unique Dual Indexes (PerkinElmer, Waltham, USA) and sequenced on an Illumina NovaSeq6000 sequencer using an S2 50bp PE Flowcell (Illumina, San Diego, USA).
The workflow for the bioinformatic pipeline used is shown in Figure 1. First, bacterial small RNA-Seq data were trimmed using Trimmomatic (version 0.33) (26) and uploaded to Partek Flow (Partek Inc., Chesterfield, USA) (27). Genome and annotation files for each bacterial species were retrieved from NCBI (using the NCBI RefSeq Assembly Accession numbers above) and made into Bowtie2 (version 2.5.5) (28) indices in Partek Flow. RNA sequences from the bacterial cells and EVs were aligned to genomes according to species. This output, along with other relevant input files, was run in sRNA-Detect (29), followed by Promotech (30), sRNACharP (31), and sRNARanking (31, 32) to identify novel and known sRNAs for the reference sRNA datasets (see Supplementary Methods for more details).
Figure 1 Bioinformatic pipeline to identify bacterial sRNAs from cell pellet RNA and EV pellet RNA. Each species was run independently of each other. Cell and EV pellet RNA were isolated and sequenced. First, genome files (i.e., FASTA sequence and annotation files) were retrieved from NCBI and the RNA sequences were uploaded to Partek to align the sequences to the respective genomes. The subsequent output alignment files and the same genome files were run through sRNA-Detect to generate a broad list of small RNA transcripts. Simultaneously, the genome files were run through Promotech to generate lists of promoters required for sRNACharP. Finally, sRNACharP and sRNARanking were used to create the reference sRNAs for each bacteria from genome files, promoter lists, and output of sRNA-Detect. EV, extracellular vesicle; sRNA, small RNA.
For characterization, sRNA sequences were batch-searched in NCBI (33) and Rfam (34) databases to determine if any sRNAs had previously been identified and to obtain relevant annotations of the sequences. All rho-independent transcription terminators (RITT) sequences in each genome were identified by uploading the entire genome to ARNold (http://rssf.i2bc.paris-saclay.fr/toolbox/arnold/index.php), a web-based service that uses ERPIN (35), RNAmotif (36), and RNAfold (37). BLAST was used to find any overlap between sRNA reference sequences and the RITT sequences identified by ARNold. The reference sRNA datasets and their descriptions can be found in Supplementary Data 1–4.
To determine potential human transcript targets, the energy of interaction was calculated for all bacterial sRNA sequences and all accessible human 3’ UTR sequences with IntaRNA (38) (see Supplementary Methods for more details). Every human 3’UTR sequence available in the Ensembl database (39) that was not “N” or less than 8bp long was examined, following IntaRNA requirements (38) (3,507 unique genes; 19,461 individual 3’UTR sequences; Supplementary Data 5). Both the sRNA and 3’UTR sequences were reverse complemented to ensure the interaction between the transcript sequences, would be calculated, and not the gene sequences. The strongest unique interactions between the sRNAs and 3’UTRs were decided by the most negative hybridization energy and the top 250 “potential human transcript targets” of the paired sRNA were considered. We selected 250 targets based on rarefaction curves that showed saturation at that number of targets for the parent processes the targets were involved with via MetaScape (40).
Qiagen’s Ingenuity Pathway Analysis (IPA, version 94302991; https://digitalinsights.qiagen.com/IPA) was used to identify changes in pathway signaling that could be caused by bronchiolitis-associated bacterial sRNAs. This is assuming they act as miRNA and target human transcripts via base-paring at their 3’UTR leading to downregulation of its direct target (the gene of the 3’UTR).
Assuming that sRNAs act as miRNA and target human transcripts via base-paring at their 3’UTR leading to downregulation of its direct target (the gene of the 3’UTR). Qiagen’s Ingenuity Pathway Analysis (IPA, version 94302991; https://digitalinsights.qiagen.com/IPA) was used to identify sRNA targets and subsequent pathway signaling alterations that could be caused by bronchiolitis-associated bacterial sRNAs.
Instead of using miRNA sequences and applying a target filter to identify experimentally validated and predicted miRNA-mRNA interactions, as is typical, we identified the potential targets of the sRNAs through IntaRNA. The sRNAs were not directly put into IPA as they are unavailable in the IPA database. Fold change (FC) and false discovery rate (FDR) of the differentially expressed sRNAs (FC>|1.1| and FDR<0.05) were applied to the respective 250 targets, which were then input into IPA. We conducted a core analysis to identify signaling pathways significantly (p-value < 0.05 by Fisher’s exact test; z-score > |2|) affected by the changes of the differentially expressed sRNAs (Supplementary Table 1). For statistically significant pathways, red and green indicated the targets of sRNAs, specifically the those of the sRNAs upregulated in RSV-only bronchiolitis and sRNAs upregulated in RV-only bronchiolitis, respectively. Similarly, blue indicated a predicted pathway activation in RSV-bronchiolitis due to the sRNAs (and predicted inhibition in RV-only cases) while orange indicated the opposite.
We analyzed cytokine levels from previously described MARC-35 data (41). Briefly, cytokine levels in nasal swab samples from index hospitalization were analyzed using multiplex the Meso Scale Discovery (MSD) electrochemiluminescent V-Plex multiplex immunoassay (Meso Scale Diagnostics, Rockville, MD), on the MESO QuickPlex SQ 120 system (Meso Scale Diagnostics). In the present study, we analyzed IL-1, IL-2, IL-6, and IL-8 measurements by viral etiology (RSV-only versus RV-only).
The cohort used comprised of infants of the MARC-35 cohort with nasal swab sequencing data (n=589). For this analysis, only infants with an RSV infection (RSV-only) or an RV infection (RV-only) as the only detected infecting virus were examined. Differences in patient characteristics were calculated using a Student’s T-test. To search for bacterial sRNAs in the MARC-35 nasal swab sample RNA-Seq data, the sequences in the sRNA reference datasets were converted into Bowtie2 indices (one per species) in Partek Flow. Differential expression analysis was performed via the native implementation of DESeq2 (42) in Partek Flow (normalized by Median ratio) using a Wald test with a Benjamin and Hochberg correction. Cytokine data comparisons used a Mann-Whitney U test with correction, which were computed in Rstudio (v4.1.2), and samples with cytokine levels less than lower limit of detection were removed. All other data were visualized in Rstudio (v4.1.2) using the tidyverse (v1.3.2) package.
We created reference datasets of sRNAs for each species by running small RNA sequencing reads from cultured bacteria and used a multi-program pipeline to bioinformatically identify sRNAs within the bacterial RNA-Seq data (Figure 1). Our reference datasets contained 449 putative H. influenzae sRNAs, 466 putative M. catarrhalis sRNAs, 49 putative M. nonliquefaciens sRNAs, and 183 putative S. pneumoniae sRNAs (Figure 2; see detailed descriptions in Supplementary Data 1–4).
Figure 2 Stacked bar graph depicting the number of reference sRNAs for each species that were detected in the 589 MARC-35 nasal swab samples from infants with severe bronchiolitis. Blue indicates the number of sRNAs in the reference datasets for each bacteria that were detected in human samples, and gray indicates the number of those that were not detected in human samples. Hi, Haemophilus influenzae; Mc, Moraxella catarrhalis; Mn, Moraxella nonliquefaciens; Sp, Streptococcus pneumoniae.
We annotated our putative sRNAs by first assessing if they contained rho-independent transcription terminatory (RITT) sequences. Approximately 31-51% of the sRNAs contained RITT sequences depending on the species (Table 1). We also searched the sRNA sequences in the BLAST and Rfam databases to obtain annotations. As low as 6% (M. nonliquefaciens) and up to 40% (S. pneumoniae) of the sRNAs aligned to some known genomic feature. Our reference datasets contained 11 H. influenzae sRNAs and 15 S. pneumoniae sRNAs that had been previously documented as sRNA sequences in the databases, but no M. catarrhalis or M. nonliquefaciens sRNAs previously documented as such (Table 1). The rest of the sRNAs in the datasets contained sequences that have not been documented as sRNAs and are thus potentially novel.
We examined the expression of our reference sRNAs in an analytical cohort of 464 (46%) infants of the 1,016 infant MARC-35 cohort who were hospitalized for bronchiolitis. The analytical cohort was composed of 420 infants with RSV-only and 44 infants with RV-only bronchiolitis. There were no characteristics that differed significantly between the analytic and non-analytic cohorts, except for viral etiology. In the analytical cohort, 41% were female, 47% were non-Hispanic white, 21% were non-Hispanic black, and 29% were Hispanic (Table 2). The median age was 3.0 months (IQR = 1.6 - 5.6 months). Additionally, there were no characteristics that differed significantly between the RSV-only group and the RV-only group except that those of the RV-only group were a few months older and weighed more (Table 2).
We found 30 sRNAs differentially expressed (FC>|1.1|; FDR<0.05) between RV- and RSV-only bronchiolitis samples, as shown in Figure 3, spanning across all four species we looked at. Almost all (26) sRNAs were upregulated in RV-only cases, and thus downregulated in RSV-only cases comparatively (Figure 3). Only four sRNAs were upregulated in RSV-only cases, and comparatively downregulated in RV-only cases (Figure 3).
Figure 3 Volcano plots depicting differentially expressed (Fold Change > |1.1|, FDR < 0.05) sRNAs for each bacteria associated with the RV-only group (red dots; n=44) and RSV-only group (blue dots; n=420). Table below shows the number of differentially expressed sRNAs for each group per species. Hi, Haemophilus influenzae; Mc, Moraxella catarrhalis; Mn, Moraxella nonliquefaciens; Sp, Streptococcus pneumoniae.
All pathways predicted to be significantly different between RV-only cases and RSV-only cases due to the predicted inhibition of transcript targets by the etiology-associated bacterial sRNAs can be found in Supplementary Table 1. In this comparative analysis, red and green indicated the targets of sRNAs, which are subsequently downregulated by the respective sRNA, specifically the sRNAs upregulated and associated with RSV-only bronchiolitis and sRNAs upregulated and associated with RV-only bronchiolitis, respectively. Similarly, blue indicated a predicted pathway activation in RSV-bronchiolitis due to the sRNAs (and predicted inhibition in RV-only cases) while orange indicates the opposite.
Several components of the interleukin (IL)-8 signaling pathway were targeted by RV-only bronchiolitis-associated bacterial sRNAs (green) (z-score = -5.048; p-value = 0.001) (Figure 4). MAP4K4, IKK, and NFKBIB (green), all proteins involved in the pathway signaling of NFkB, were predicted targets of the sRNAs that were upregulated in RV-bronchiolitis, leading to a predicted inhibition of the pathway and subsequent inhibition of inflammation in RV-bronchiolitis compared to RSV-bronchiolitis (Figure 4).
Figure 4 Pathway analysis of sRNA targets affecting the IL-8 pathway in IPA. Compared to RSV-only bronchiolitis, those in green are targets of sRNAs associated with RV-only bronchiolitis, which are assumed to be downregulated in RV-only bronchiolitis, while those in red are targets of sRNAs associated with RSV-only bronchiolitis, which are assumed to be upregulated in RV-only bronchiolitis. Those in blue are predicted to be inhibited, while those in orange are predicted to activated in RV-only bronchiolitis. RSV, respiratory syncytial virus; RV, rhinovirus.
IL-6 signaling was also predicted to be downregulated in RV-only bronchiolitis and upregulated in RSV-only bronchiolitis comparatively (z-score = -4.041; p-value = 0.002) (Figure 5). Similarly, proteins involved in NFkB pathway signaling such as TNF, the Tnf receptor, IKK, and others (green) were all predicted targets of sRNAs associated with RV-only bronchiolitis, leading to their predicted downregulation (Figure 5). However, Ikb was a predicted target of sRNAs associated with both RSV- and RV-only bronchiolitis (green to red gradient) (Figure 5). Despite the predicted downregulation of the pathway, IL-6 was a target of sRNAs associated with RSV-only cases, and therefore there was a higher predicted level of IL-6 and subsequent activation of STAT3 in RV-only bronchiolitis comparatively (Figure 5).
Figure 5 Pathway analysis of sRNA targets affecting the IL-6 pathway in IPA. Compared to RSV-only bronchiolitis, those in green are targets of sRNAs associated with RV-only bronchiolitis, which are assumed to be downregulated in RV-only bronchiolitis, while those in red are targets of sRNAs associated with RSV-only bronchiolitis, which are assumed to be upregulated in RV-only bronchiolitis. Those in blue are predicted to be inhibited, while those in orange are predicted to activated in RV-only bronchiolitis. RSV, respiratory syncytial virus; RV, rhinovirus.
We analyzed the levels of IL-6 and IL-8 in a subset of our cohort (RSV-only = 356; RV-only = 33). There were no significant differences in either cytokine by viral etiology (Supplementary Table 2).
Signaling molecules targeted by bacterial sRNAs involved in IL-17A signaling in airway cells were predicted to be overall downregulated in RV-only cases and upregulated in RSV-only cases (z-score = -2; p-value = 0.003) (Figure 6). Despite this, the pathway was predicted to result in higher activation of chemoattraction, inflammation, neutrophil recruitment, and mucous hypersecretion in RV-only cases compared to RSV-only cases (Figure 6). sRNAs associated with RV-only cases were predicted to target signaling molecules such as MAP3K7, IKK, and Ikb (green), while sRNAs associated with RSV-only cases were predicted to target IL-6 and IL-17RA (Figure 6).
Figure 6 Pathway analysis of sRNA targets affecting IL17A signaling in airway cells. Compared to RSV-only bronchiolitis, those in green are targets of sRNAs associated with RV-only bronchiolitis, which are assumed to be downregulated in RV-only bronchiolitis, while those in red are targets of sRNAs associated with RSV-only bronchiolitis, which are assumed to be upregulated in RV-only bronchiolitis. Those in blue are predicted to be inhibited, while those in orange are predicted to activated in RV-only bronchiolitis. RSV, respiratory syncytial virus; RV, rhinovirus.
Pathways of several cytokines, such as IL-1, IL-2, and IL-23 were predicted to be significantly upregulated in RSV- compared to RV-only bronchiolitis (z-score = -2.84, p-value = 0.003; z-score = -3.357, p-value = 0.004; and z-score = -2.53, p-value = 0.034, respectively) (Supplementary Table 1). We also analyzed the levels of IL-1 and IL-2 in a subset of our cohort (RSV-only = 356; RV-only = 33). There were no significant differences in either cytokine by viral etiology (Supplementary Table 2).
In this study, we identified numerous novel bacterial sRNAs that were differentially expressed between infants with RSV-only bronchiolitis and infants with RV-only bronchiolitis. These differentially expressed bacterial sRNAs were predicted to interact with several human 3’UTRs in bronchiolitis and potentially lead to the activation of the IL-8 and IL-6 pathways and inhibition of the IL-17A in RSV-only cases, and thus the inhibition of the IL-8 and IL-6 pathways and activation of the IL-17A pathway in RV-bronchiolitis.
IL-8 and IL-6 are two proinflammatory cytokines that have been proposed as potential biomarkers for bronchiolitis severity in several studies (43, 44). While the association with either viral etiology remains unclear, they remain important factors in the pathology of bronchiolitis (43, 45).
Our results indicate that bacterial sRNAs associated with bronchiolitis result in the upregulation of IL-8 and IL-6 pathways in RSV-only cases compared to RV-only cases. The complexity of the decreased predicted measurement of these cytokines, particularly IL-6, with the predicted activation of their signaling pathway seen in RSV-only bronchiolitis is reflected in the literature. One study by Diaz et al. compared cytokine levels of healthy infants to those with RV-only and RSV-only bronchiolitis and showed that those with RSV-only bronchiolitis had significantly higher IL-8 levels than both the healthy individuals and those with RV-only bronchiolitis (45). However, some studies have suggested that IL-8 and IL-6 production are not different between viral groups, consistent with our cytokine measurement data, but between severity (43). Nonetheless, although the difference was not quite significant, infants with RSV-bronchiolitis trended higher in IL-8 and IL-6 production than those with RV-bronchiolitis (43). This, and Diaz’s findings, are consistent with our predictions that IL-8 and IL-6 signaling may be upregulated in RSV-bronchiolitis and comparatively downregulated by bacteria in RV-bronchiolitis.
Morbidity of severe bronchiolitis has repeatedly been associated with a higher risk for developing asthma (4, 5, 46). While IL-17A does not appear to be specifically correlated with either RSV- or RV-bronchiolitis, IL-17A may contribute to airway hyperresponsiveness seen in asthma (47). Here, activation of the IL-17A pathway was associated with RV-only bronchiolitis compared to RSV-only bronchiolitis. Previous studies attempted to create endotypes based on transcriptomic, microbiome, and virus data collected from infants with bronchiolitis (15). Of five endotypes, one was associated with the dominance of H. influenzae, RSV/RV co-infection, and upregulation of Th17 pathways (15). Additionally, this endotype was associated with a significantly higher risk of developing asthma (15). While there were significantly different sRNAs of each of the four species between RV-only and RSV-only bronchiolitis, 11 of 30 were of H. influenzae origin. It is interesting that bacterial sRNAs are predicted to result in the activation of the IL-17A pathway, specifically in RV-only bronchiolitis, indicating that these sRNAs could be involved in changes to the immune system during and after bronchiolitis that may contribute to asthma.
Additionally, while IL-17A levels in bronchioaveolar lavage fluid (BALF) are not different between mice infected with RV and RSV, mice infected with RV were shown to produce higher BALF levels of neutrophils detected than those with RSV in early infection (48). IL-17A production has been associated with recruiting neutrophils in response to bacterial infection (49, 50). As IL-17A signaling, but not the cytokine itself, and subsequent neutrophil recruitment were predicted to be activated more in RV- than RSV-cases, our data corroborate this and indicate a potential role of RV-bronchiolitis-associated bacterial sRNAs to induce comparatively higher neutrophil activation and recruitment than in RSV-bronchiolitis. On the other hand, signaling of IL-6 and IL-8, common neutrophil attractants, were higher in RSV- than RV-cases. IL-6 was predicted to be a direct target of the bacterial sRNAs in higher abundance in RSV, indicating a direct decrease of the cytokine in RSV cases. Also, although the pathways predicted to be altered by bacterial sRNAs in higher abundance in RSV were related to IL-6 and IL-8 signaling, higher IL-8 signaling was predicted to result in higher general inflammation in RV cases, but it was not predicted to contribute to neutrophil recruitment and activation. Overall, our results are summarized in Figure 7.
Figure 7 Predicted effects on human gene expression of bacterial sRNAs associated with RV- and RSV-bronchiolitis via target repression. (A) Bacteria produce sRNAs, the abundance and presence may be induced or changed depending on the infecting virus. (B) Bacterial sRNAs are taken up by human cells, potentially through extracellular vesicles. (C) The bacterial sRNAs that are upregulated in RV-bronchiolitis target the IL-8 pathway via NFKB signaling and lead to a decrease in IL-8 signaling, which subsequently leads to a decrease in IL-8 associated inflammation relative to RSV-bronchiolitis. (D) The bacterial sRNAs that are upregulated in RSV-bronchiolitis target the IL-17A pathway via IL-17RA and lead to a decrease in IL-17A signaling which subsequently leads to a decrease in neutrophil attraction via IL-17A relative to RV-bronchiolitis.
Several other proinflammatory cytokine pathways were predicted to be affected potential bacterial sRNA modulation, such as IL-1, IL-2, and IL-23, some of which have previously been implicated in bronchiolitis (10, 12, 48, 51, 52) (Supplementary Table 1). All these cytokines were predicted to be inhibited in RV-only bronchiolitis compared to RSV-only bronchiolitis due to the bacterial sRNAs (Supplementary Table 1). Similar to IL-6 and IL-8, we analyzed the levels of IL-1-beta and IL-2 in nasal swab samples of MARC-35 infants taken at hospitalization, but there were no significant differences in either cytokine by viral etiology (RSV-only versus RV-only). Production of IL-23 in response to Gram-negative bacterial infection can induce production of IL-17A (53, 54), but this is inconsistent with our predictions that IL-17A is a direct target of bacterial sRNAs positively associated with RSV.
Additionally, a notable pathway that has been implicated as different between RSV- and RV-bronchiolitis was NFkB activation by viruses (Supplementary Table 1) which was predicted to be upregulated in RSV-only bronchiolitis compared to RV-only bronchiolitis. NFkB is a transcription factor important for immune response and as a mediator of inflammation. RV-bronchiolitis has been associated with a marked increase in NFkB signaling compared to RSV, particularly via human miRNAs. Although the direction of the findings is inconsistent, in both the current study and in earlier work (10), NFkB was predicted to be a target of the regulator RNAs.
There are several potential limitations to this work. First, we use two primary assumptions: 1) that sRNAs can be used like miRNAs and result in the downregulation of its transcript target; and 2) that the transcript targets of our reference sRNAs can be identified by its strongest interactions with human 3’UTR sequences determined by hybridization energy. There is strong evidence to support that sRNAs have been associated with human miRNA machinery (21, 23). Moreover, Furuse et al. found that overexpression of a putative bacterial sRNA in a human cell line was enough to result in downregulation of an artificial target mRNA via a reporter construct (21). Thus, we consider these assumptions to be supported by the literature. Another possible limitation of this work is that no healthy infant controls were used, and thus we cannot make comparisons between viral infection and healthy state. Further, this work focuses on the differences between RSV-only infection versus RV-only infection—the two major viruses of infant bronchiolitis (6–8). There are several viruses that can cause bronchiolitis, and multiple viruses can co-infect an infant to induce bronchiolitis. Thus, the results may not be generalizable to all infants with bronchiolitis. Furthermore, our sample size of infants with RV-only bronchiolitis is much smaller than that of infants with RSV-only bronchiolitis. Another limitation of this study is that our results are computational and require in vitro experiments for validation of the interactions and effects of the bacterial sRNAs. Despite our study limitations, we provided novel insights into the mechanisms by which bacteria may be contributing to the clinical differences seen in RSV- and RV-only bronchiolitis.
In summary, we identified many novel sRNAs from several bacterial species that are associated with RV-only and RSV-only bronchiolitis during infancy. In RV bronchiolitis, there is a predicted relative inhibition of IL-8 and IL-6 signaling compared to RSV bronchiolitis, leading to lower predicted inflammation in RV-bronchiolitis (Figure 7). Additionally, there is a predicted relative activation of IL-17A in RV bronchiolitis compared to RSV bronchiolitis due to differential expression of bacterial sRNAs, leading to higher predicted neutrophil recruitment in RV-bronchiolitis, and comparatively less so in RSV-bronchiolitis (Figure 7). These data indicate that bacteria may be contributing to different mechanisms of inflammation in RSV-only and RV-only bronchiolitis by their sRNAs and may explain in part the heterogeneity seen in infants with bronchiolitis. However, more research is needed to confirm these relationships and identify if these bacterial sRNA-driven alterations also contribute to the heterogeneity seen in asthma risk.
The datasets presented in this study can be found in online repositories. The names of the repository/repositories and accession number(s) can be found below: https://www.immport.org/shared/study/SDY2306, SDY2306, https://www.immport.org/shared/study/SDY2157, SDY2157.
The studies involving humans were approved by Institutional Review Board of Children’s National Hospital. The studies were conducted in accordance with the local legislation and institutional requirements. The human samples used in this study were acquired from primarily isolated as part of your previous study for which ethical approval was obtained. Written informed consent for participation was not required from the participants or the participants’ legal guardians/next of kin in accordance with the national legislation and institutional requirements.
KK: Writing – original draft, Writing – review & editing. MP-L: Writing – review & editing. IR-T: Writing – review & editing. ZZ: Writing – review & editing. KH: Writing – review & editing. CC: Writing – review & editing. BH: Writing – review & editing. JE: Writing – review & editing. LRC: Writing – review & editing. RB: Writing – review & editing. RF: Writing – review & editing. AH: Writing – review & editing.
The author(s) declare financial support was received for the research, authorship, and/or publication of this article. This study was supported by grants from the National Institutes of Health (Bethesda, MD): U01 AI-087881 (CC), R01 AI-127507 (CC and RF), R01 AI-134940 (KH), and R01 AI-137091 (KH). The content of this manuscript is solely the responsibility of the authors and does not necessarily represent the official views of the National Institutes of Health.
The authors would like to acknowledge Dr. Claudio Anselmi and the Office of the Chief Research Information Officer for assistance and computing time on the high-performance computer at Children’s National Research and Innovation Campus (HPC@CNRIC). We would like to thank the participants and researchers from the Multicenter Airway Research Collaboration (MARC) who significantly contributed or collected data.
The authors declare that the research was conducted in the absence of any commercial or financial relationships that could be construed as a potential conflict of interest.
The author(s) declared that they were an editorial board member of Frontiers, at the time of submission. This had no impact on the peer review process and the final decision.
All claims expressed in this article are solely those of the authors and do not necessarily represent those of their affiliated organizations, or those of the publisher, the editors and the reviewers. Any product that may be evaluated in this article, or claim that may be made by its manufacturer, is not guaranteed or endorsed by the publisher.
The Supplementary Material for this article can be found online at: https://www.frontiersin.org/articles/10.3389/fimmu.2024.1330991/full#supplementary-material
1. Florin TA, Plint AC, Zorc JJ. Viral bronchiolitis. Lancet (2017) 389:211–24. doi: 10.1016/S0140-6736(16)30951-5
2. Kyu HH, Pinho C, Wagner JA, Brown JC, Bertozzi-Villa A, Charlson FJ, et al. Global and national burden of diseases and injuries among children and adolescents between 1990 and 2013. JAMA Pediatr (2016) 170:267. doi: 10.1001/jamapediatrics.2015.4276
3. Hasegawa K, Tsugawa Y, Brown DFM, Mansbach JM, Camargo CA Jr. Trends in bronchiolitis hospitalizations in the United States, 2000–2009. Pediatrics (2013) 132:28–36. doi: 10.1542/peds.2012-3877
4. Hasegawa K, Mansbach JM, Camargo CA. Infectious pathogens and bronchiolitis outcomes. Expert Rev Anti Infect Ther (2014) 12:817–28. doi: 10.1586/14787210.2014.906901
5. Ruotsalainen M, Hyvärinen MK, Piippo-Savolainen E, Korppi M. Adolescent asthma after rhinovirus and respiratory syncytial virus bronchiolitis. Pediatr Pulmonol. (2013) 48:633–9. doi: 10.1002/ppul.22692
6. Marguet C, Lubrano M, Gueudin M, Le Roux P, Deschildre A, Forget C, et al. In very young infants severity of acute bronchiolitis depends on carried viruses. PloS One (2009) 4:e4596. doi: 10.1371/journal.pone.0004596
7. Midulla F, Scagnolari C, Bonci E, Pierangeli A, Antonelli G, De Angelis D, et al. Respiratory syncytial virus, human bocavirus and rhinovirus bronchiolitis in infants. Arch Dis Child. (2010) 95:35–41. doi: 10.1136/adc.2008.153361
8. Mansbach JM, Hasegawa K, Henke DM, Ajami NJ, Petrosino JF, Shaw CA, et al. Respiratory syncytial virus and rhinovirus severe bronchiolitis are associated with distinct nasopharyngeal microbiota. J Allergy Clin Immunol (2016) 137:1909–1913.e4. doi: 10.1016/j.jaci.2016.01.036
9. Stewart CJ, Hasegawa K, Wong MC, Ajami NJ, Petrosino JF, Piedra PA, et al. Respiratory syncytial virus and rhinovirus bronchiolitis are associated with distinct metabolic pathways. J Infect Dis (2018) 217:1160–9. doi: 10.1093/infdis/jix680
10. Hasegawa K, Pérez-Losada M, Hoptay CE, Epstein S, Mansbach JM, Teach SJ, et al. RSV vs. rhinovirus bronchiolitis: difference in nasal airway microRNA profiles and NFκB signaling. Pediatr Res (2018) 83:606–14. doi: 10.1038/pr.2017.309
11. Zhu Z, Li Y, Freishtat RJ, Celedón JC, Espinola JA, Harmon B, et al. Epigenome-wide association analysis of infant bronchiolitis severity: a multicenter prospective cohort study. Nat Commun (2023) 14:5495. doi: 10.1038/s41467-023-41300-y
12. Yuan X, Li Y, Shen Y, Yang J, Jin Y. Clinical and Th1/Th2 immune response features of hospitalized children with human rhinovirus infection. J Med Virol (2020) 92:26–33. doi: 10.1002/jmv.25587
13. Hurme P, Komulainen M, Tulkki M, Leino A, Rückert B, Turunen R, et al. Cytokine expression in rhinovirus- vs. respiratory syncytial virus-induced first wheezing episode and its relation to clinical course. Front Immunol (2022) 13. doi: 10.3389/fimmu.2022.1044621
14. Vandini S, Calamelli E, Faldella G, Lanari M. Immune and inflammatory response in bronchiolitis due to respiratory Syncytial Virus and Rhinovirus infections in infants. Paediatr Respir Rev (2017) 24:60–4. doi: 10.1016/j.prrv.2016.11.006
15. Raita Y, Pérez-Losada M, Freishtat RJ, Hahn A, Castro-Nallar E, Ramos-Tapia I, et al. Nasopharyngeal metatranscriptome profiles of infants with bronchiolitis and risk of childhood asthma: a multicentre prospective study. Eur Respir J (2022) 60:2102293. doi: 10.1183/13993003.02293-2021
16. Hasegawa K, Mansbach JM, Ajami NJ, Espinola JA, Henke DM, Petrosino JF, et al. Association of nasopharyngeal microbiota profiles with bronchiolitis severity in infants hospitalised for bronchiolitis. Eur Respir J (2016) 48:1329–39. doi: 10.1183/13993003.00152-2016
17. Hasegawa K, Linnemann RW, Mansbach JM, Ajami NJ, Espinola JA, Petrosino JF, et al. The fecal microbiota profile and bronchiolitis in infants. Pediatrics (2016) 138(1):e20160218. doi: 10.1542/peds.2016-0218
18. Fujiogi M, Raita Y, Pérez-Losada M, Freishtat RJ, Celedón JC, Mansbach JM, et al. Integrated relationship of nasopharyngeal airway host response and microbiome associates with bronchiolitis severity. Nat Commun (2022) 13:4970. doi: 10.1038/s41467-022-32323-y
19. Bouvier M, Sharma CM, Mika F, Nierhaus KH, Vogel J. Small RNA binding to 5′ mRNA coding region inhibits translational initiation. Mol Cell (2008) 32:827–37. doi: 10.1016/j.molcel.2008.10.027
20. Boisset S, Geissmann T, Huntzinger E, Fechter P, Bendridi N, Possedko M, et al. Staphylococcus aureus RNAIII coordinately represses the synthesis of virulence factors and the transcription regulator Rot by an antisense mechanism. Genes Dev (2007) 21:1353–66. doi: 10.1101/gad.423507
21. Furuse Y, Finethy R, Saka HA, Xet-Mull AM, Sisk DM, Jurcic Smith KL, et al. Search for microRNAs expressed by intracellular bacterial pathogens in infected mammalian cells. PloS One (2014) 9:e106434. doi: 10.1371/journal.pone.0106434
22. Koeppen K, Hampton TH, Jarek M, Scharfe M, Gerber SA, Mielcarz DW, et al. A Novel Mechanism of Host-Pathogen Interaction through sRNA in Bacterial Outer Membrane Vesicles. PloS Pathog (2016) 12:e1005672. doi: 10.1371/journal.ppat.1005672
23. Han E-C, Choi S-Y, Lee Y, Park J-W, Hong S-H, Lee HJ. Extracellular RNAs in periodontopathogenic outer membrane vesicles promote TNF-α production in human macrophages and cross the blood–brain barrier in mice. FASEB J (2019) 33:13412–22. doi: 10.1096/fj.201901575R
24. Raita Y, Pérez-Losada M, Freishtat RJ, Harmon B, Mansbach JM, Piedra PA, et al. Integrated omics endotyping of infants with respiratory syncytial virus bronchiolitis and risk of childhood asthma. Nat Commun (2021) 12:3601. doi: 10.1038/s41467-021-23859-6
25. Lambert SB, Ware RS, Al C, Maguire FA, Whiley DM, Bialasiewicz S, et al. Observational Research in Childhood Infectious Diseases (ORChID): a dynamic birth cohort study. BMJ Open (2012) 2:e002134. doi: 10.1136/bmjopen-2012-002134
26. Bolger AM, Lohse M, Usadel B. Trimmomatic: a flexible trimmer for Illumina sequence data. Bioinformatics (2014) 30:2114–20. doi: 10.1093/bioinformatics/btu170
27. Partek Inc. Partek® Flow®. (2020). Available at: https://www.partek.com/partek-flow/.
28. Langmead B, Salzberg SL. Fast gapped-read alignment with Bowtie 2. Nat Methods (2012) 9:357–9. doi: 10.1038/nmeth.1923
29. Peña-Castillo L, Grüell M, Mulligan ME, Lang AS. Detection of bacterial small transcripts from RNA-SEQ data: a comparative assessment. Biocomputing 2016 (2016) 21:456–67. doi: 10.1142/9789814749411_0042
30. Chevez-Guardado R, Peña-Castillo L. Promotech: a general tool for bacterial promoter recognition. Genome Biol (2021) 22:318. doi: 10.1186/s13059-021-02514-9
31. Eppenhof EJJ, Peña-Castillo L. Prioritizing bona fide bacterial small RNAs with machine learning classifiers. PeerJ (2019) 7:e6304. doi: 10.7717/peerj.6304
32. Sorkhian M, Nagari M, Elsisy M, Peña-CasFllo L. Improving bacterial sRNA identification by combining genomic context and sequence-derived features. Lec Notes in Comp Sci Comput Intl Methods for Bioinform Biostat (2022) 13483:67–78. doi: 10.1007/978-3-031-20837-9_6
33. Johnson M, Zaretskaya I, Raytselis Y, Merezhuk Y, McGinnis S, Madden TL. NCBI BLAST: a better web interface. Nucleic Acids Res (2008) 36:W5–9. doi: 10.1093/nar/gkn201
34. Kalvari I, Nawrocki EP, Ontiveros-Palacios N, Argasinska J, Lamkiewicz K, Marz M, et al. Rfam 14: expanded coverage of metagenomic, viral and microRNA families. Nucleic Acids Res (2021) 49:D192–200. doi: 10.1093/nar/gkaa1047
35. Gautheret D, Lambert A. Direct RNA motif definition and identification from multiple sequence alignments using secondary structure profiles 1 1Edited by J. Doudna. J Mol Biol (2001) 313:1003–11. doi: 10.1006/jmbi.2001.5102
36. Macke TJ. RNAMotif, an RNA secondary structure definition and search algorithm. Nucleic Acids Res (2001) 29:4724–35. doi: 10.1093/nar/29.22.4724
37. Hofacker IL, Fontana W, Stadler PF, Bonhoeffer LS, Tacker M, Schuster P. Fast folding and comparison of RNA secondary structures. Monatshefte Chemie Chem Monthly. (1994) 125:167–88. doi: 10.1007/BF00818163
38. Mann M, Wright PR, Backofen R. IntaRNA 2.0: enhanced and customizable prediction of RNA–RNA interactions. Nucleic Acids Res (2017) 45:W435–9. doi: 10.1093/nar/gkx279
39. Cunningham F, Allen JE, Allen J, Alvarez-Jarreta J, Amode MR, Armean IM, et al. Ensembl 2022. Nucleic Acids Res (2022) 50:D988–95. doi: 10.1093/nar/gkab1049
40. Zhou Y, Zhou B, Pache L, Chang M, Hadj Khodabakhshi A, Tanaseichuk O, et al. Metascape provides a biologist-oriented resource for the analysis of systems-level datasets. Nat Commun (2019) 10:1523. doi: 10.1038/s41467-019-09234-6
41. Zhu Z, Freishtat RJ, Harmon B, Hahn A, Teach SJ, Pérez-Losada K, et al. MARC-35 Investigators. Nasal airway microRNA profiling of infants with severe bronchiolitis and risk of childhood asthma: a multicentre prospective study. Eur Respir J. (2023) 62(2):2300502. doi: 10.1183/13993003.00502-2023
42. Love MI, Huber W, Anders S. Moderated estimation of fold change and dispersion for RNA-seq data with DESeq2. Genome Biol (2014) 15:550. doi: 10.1186/s13059-014-0550-8
43. Lee CY, Sung CH, Wu MC, Chang YC, Chang JC, Fang YP, et al. Clinical characteristics and differential cytokine expression in hospitalized Taiwanese children with respiratory syncytial virus and rhinovirus bronchiolitis. J Microbiology Immunol Infection. (2023) 56:282–91. doi: 10.1016/j.jmii.2022.08.013
44. Vázquez Y, González L, Noguera L, González PA, Riedel CA, Bertrand P, et al. Cytokines in the respiratory airway as biomarkers of severity and prognosis for respiratory syncytial virus infection: an update. Front Immunol (2019) 10. doi: 10.3389/fimmu.2019.01154
45. Díaz PV, Valdivia G, Gaggero AA, Bono MR, Zepeda G, Rivas M, et al. Pro-inflammatory cytokines in nasopharyngeal aspirate from hospitalized children with respiratory syncytial virus infection with or without rhinovirus bronchiolitis, and use of the cytokines as predictors of illness severity. Medicine (2015) 94:e1512. doi: 10.1097/MD.0000000000001512
46. Bergroth E, Aakula M, Elenius V, Remes S, Piippo-Savolainen E, Korppi M, et al. Rhinovirus type in severe bronchiolitis and the development of asthma. J Allergy Clin Immunol Pract (2020) 8:588–595.e4. doi: 10.1016/j.jaip.2019.08.043
47. Habib N, Pasha MA, Tang DD. Current understanding of asthma pathogenesis and biomarkers. Cells (2022) 11:2764. doi: 10.3390/cells11172764
48. Liu X, Nguyen TH, Sokulsky L, Li X, Garcia Nero K, Hsu AC-Y, et al. IL-17A is a common and critical driver of impaired lung function and immunopathology induced by influenza virus, rhinovirus and respiratory syncytial virus. Respirology (2021) 26:1049–59. doi: 10.1111/resp.14141
49. Deshmukh HS, Liu Y, Menkiti OR, Mei J, Dai N, O'Leary CE, et al. The microbiota regulates neutrophil homeostasis and host resistance to Escherichia coli K1 sepsis in neonatal mice. Nat Med (2014) 20:524–30. doi: 10.1038/nm.3542
50. Flannigan KL, Ngo VL, Geem D, Harusato A, Hirota SA, Parkos CA, et al. IL-17A-mediated neutrophil recruitment limits expansion of segmented filamentous bacteria. Mucosal Immunol (2017) 10:673–84. doi: 10.1038/mi.2016.80
51. Norlander AE, Peebles RS. Innate type 2 responses to respiratory syncytial virus infection. Viruses (2020) 12:521. doi: 10.3390/v12050521
52. Cortegano I, Rodríguez M, Hernángómez S, Arrabal A, Garcia-Vao C, Rodríguez J, et al. Age-dependent nasal immune responses in non-hospitalized bronchiolitis children. Front Immunol (2022) 13. doi: 10.3389/fimmu.2022.1011607
53. Happel KI, Dubin PJ, Zheng M, Ghilardi N, Lockhart C, Quinton LJ, et al. Divergent roles of IL-23 and IL-12 in host defense against Klebsiella pneumoniae. J Exp Med (2005) 202:761–9. doi: 10.1084/jem.20050193
Keywords: bronchiolitis, respiratory syncytial virus, rhinovirus, Haemophilus influenzae, Streptococcus pneumoniae, RNA, extra cellular vesicles
Citation: Krohmaly KI, Perez-Losada M, Ramos-Tapia I, Zhu Z, Hasegawa K, Camargo Jr. CA, Harmon B, Espinola JA, Reck Cechinel L, Batabyal R, Freishtat RJ and Hahn A (2024) Bacterial small RNAs may mediate immune response differences seen in respiratory syncytial virus versus rhinovirus bronchiolitis. Front. Immunol. 15:1330991. doi: 10.3389/fimmu.2024.1330991
Received: 03 November 2023; Accepted: 24 January 2024;
Published: 12 February 2024.
Edited by:
Susetta Finotto, Universitätsklinikum Erlangen, GermanyReviewed by:
Glenn Paul Dorsam, North Dakota State University, United StatesCopyright © 2024 Krohmaly, Perez-Losada, Ramos-Tapia, Zhu, Hasegawa, Camargo Jr., Harmon, Espinola, Reck Cechinel, Batabyal, Freishtat and Hahn. This is an open-access article distributed under the terms of the Creative Commons Attribution License (CC BY). The use, distribution or reproduction in other forums is permitted, provided the original author(s) and the copyright owner(s) are credited and that the original publication in this journal is cited, in accordance with accepted academic practice. No use, distribution or reproduction is permitted which does not comply with these terms.
*Correspondence: Kylie I. Krohmaly, a2tyb2htYWx5QGNoaWxkcmVuc25hdGlvbmFsLm9yZw==
Disclaimer: All claims expressed in this article are solely those of the authors and do not necessarily represent those of their affiliated organizations, or those of the publisher, the editors and the reviewers. Any product that may be evaluated in this article or claim that may be made by its manufacturer is not guaranteed or endorsed by the publisher.
Research integrity at Frontiers
Learn more about the work of our research integrity team to safeguard the quality of each article we publish.