- 1Department of General Surgery, Renmin Hospital of Wuhan University, Wuhan, China
- 2Hubei Key Laboratory of Digestive System Disease, Wuhan, China
Background: Immune checkpoint inhibitors (ICIs) represent a paradigm shift in the development of cancer therapy. However, the improved efficacy of ICIs remains to be further investigated. We conducted a systematic review and meta-analysis to evaluate the pan-immunoinflammatory value (PIV) and PILE score used to predict response to ICI therapy.
Methods: We searched selected databases for studies on pan-immune inflammation values and their association with outcomes of treatment with immune checkpoint inhibitors. We used hazard ratios (HRS) and 95% confidence intervals (CI) to summarize survival outcomes. All data analyses were performed using STATA 15.0.
Results: 7 studies comprising 982 patients were included in the meta-analysis. The pooled results showed that higher PIV was significantly associated with shorter overall survival OS (HR = 1.895, 95%CI: 1.548-2.318) and progression-free survival (PFS) (HR = 1.582, 95%CI: 1.324-1.890). Subgroup analyses also confirmed the reliability of the results.
Conclusions: High PIV and PILE metrics are associated with lower survival in cancer patients receiving ICIs.
1 Introduction
Cancer, being the primary cause of mortality, constitutes a substantial menace to global public health as its burden continues to escalate (1). The recent emergence of monoclonal antibodies targeting immune checkpoints has fundamentally transformed the field of cancer therapy (2, 3). The success of treatment based on immune checkpoint blockade (ICB) largely relies on blocking PD-1/PD-L1 and CTLA-4 (4, 5). Immunotherapy can activate or enhance the body’s immune system, prompting it to actively target malignant tumor cells, and has demonstrated promising efficacy across multiple tumor types (6). However, the limited overall response as well as the lack of reliable biomarkers to predict patient efficacy are hampered by large (7). This underscores the pressing necessity to not only identify novel targets for immunotherapy but also easily obtainable peripheral blood indicators. These will broaden the scope of ICB-based strategies, ultimately maximizing efficacy and benefits for cancer patients.
Consequently, pan-immune inflammation value (PIV), as a novel biomarker of immune-inflammatory response, is progressively emerging as a crucial indicator for predicting the efficacy of immunotherapy and guiding individualized treatment decisions (8). Unlike traditional single-measurement biomarkers, the pan-immune inflammation value differs significantly (9). It considers the comprehensive state of the body’s immune system, along with the levels of various inflammatory signaling molecules. This integrated analysis has afforded us deeper insights into the diversity and complexity of patients’ immune statuses (10). In addition, composite prognostic scores, which incorporate many factors, have demonstrated a promising capacity for predicting prognosis in patients undergoing immunotherapy (11). The PILE score is determined by three parameters: the PIV value, the Eastern Cooperative Oncology Group Performance Status (ECOG PS), and the LDH value. Among the 120 advanced cancer patients who received anti-PD-1 or anti-PD-L1 inhibitors for various types of cancer, it was shown that a higher PILE score is linked to reduced progression-free survival (PFS) and overall survival (OS). This suggests that the PILE score has potential as a prognostic scoring system for immunotherapy (12).
This meta-analysis aimed to explore the relationship between cancer, immunotherapy, and pan-immune inflammation values. Through the integration of existing data, we sought to assess the predictive capabilities of pan-immune inflammation values in immunotherapy and their potential role in informing individualized treatment decisions. We anticipate that a comprehensive analysis of these associations will furnish robust scientific backing for refining immunotherapy strategies, enhancing treatment predictions’ precision, and ultimately advancing clinical benefits for patients.
2 Methods
2.1 Literature search
This study was conducted in compliance with the PRISMA statement guidelines (13). We conducted a systematic literature search across several electronic databases, including PubMed, EMBASE, and the Cochrane Library. The search strategy amalgamates Medical Subject Headings (MeSH) terms and pertinent keywords, such as “Immune Checkpoint Inhibitors [MeSH]”, “PD-1 Inhibitors”, “PD-L1 Inhibitors”, “CTLA-4 Inhibitors”, “Pembrolizumab”, “Nivolumab”, “Atezolizumab”, “Ipilimumab”, “pan-immune-inflammation-value”, and “ PILE “. The search is confined to studies published within a predefined time frame. Detailed search strategies are illustrated in Supplementary Material 1.
2.2 Study selection criteria
Rigorous inclusion and exclusion criteria have been systematically implemented to meticulously curate studies that adhere to high-quality standards. Eligible studies included (1) Cancer patients receiving immunotherapy; (2) Reporting of pan-immuno-inflammatory values; (3) Furthermore, these articles must report at least one of the following outcomes: overall survival (OS) or progression-free survival (PFS). We consider a diverse range of research sources, including published articles, conference abstracts, and clinical trial reports.
2.3 Data extraction
We meticulously extract pertinent data from the chosen studies. Key parameters include study characteristics (e.g., author, publication year), patient demographics, tumor types, treatment regimens, pan-immune inflammation value measurement methods, and clinical outcomes (e.g., OS, PFS). To ensure accuracy, multiple researchers independently conduct data extraction, with any discrepancies resolved through consensus. The PIV was determined using the equation [neutrophil count (103/ml) multiplied by platelet count (103/ml) multiplied by monocyte count (103/ml)]. The lymphocyte count is measured in thousands per milliliter (103/ml). The PILE scores were a composite score derived from the PIV, LDH level, and ECOG PS, determined by summing the separate values.
2.4 Quality assessment
The quality and risk of bias within individual studies are critically evaluated. Established tools such as the Newcastle-Ottawa Scale for cohort studies and the Cochrane risk of bias tool for randomized controlled trials are employed (14). Studies with inadequate methodological rigor or high risk of bias may be excluded from the final analysis to maintain the integrity of the findings.
2.5 Statistical analysis
Statistical analyses were performed using Stata 15.0 software. A meta-analysis employs appropriate statistical methods to quantitatively combine data across studies. Heterogeneity was evaluated using the chi-square test. The random-effects model was employed when the p-value was < 0.1 or the I2 statistic exceeded 50%, while the fixed-effects model was utilized otherwise. Publication bias can impact the comprehensiveness of the findings, and efforts are made to identify and mitigate its influence. Sensitivity analyses are performed to evaluate the robustness of the results. By systematically omitting one study at a time and re-analyzing the data, the influence of individual studies on the overall findings is assessed.
3 Results
3.1 Characteristics of studies
The removal of 35 duplicate studies followed the initial search. Then, 124 articles were eliminated following a thorough examination of the titles and abstracts. The full texts of the remaining seventeen articles were subsequently evaluated in greater detail. In the end, seven articles were incorporated, and a meta-analysis was conducted on these seven investigations (12, 15–20). The flowchart for the PRISMA process is shown in Figure 1. Table 1 presents the primary attributes of the studies that were incorporated. Seven studies included a total of 982 patients, and in five studies the mean age was 60 years. The NOS scores for seven articles ranged from 6 to 8, which represented a low risk of bias.
3.2 PIV and OS
Prognostic data from six studies were used to examine the association between PIV and OS. We did not observe significant heterogeneity among the included studies (I2 = 0.0%, P = 0.52, Figure 2A), so a fixed-effects model was used. We found that higher PIV was significantly associated with shorter OS (HR = 1.895, 95%CI: 1.548-2.318, P<0.001; Figure 2A).
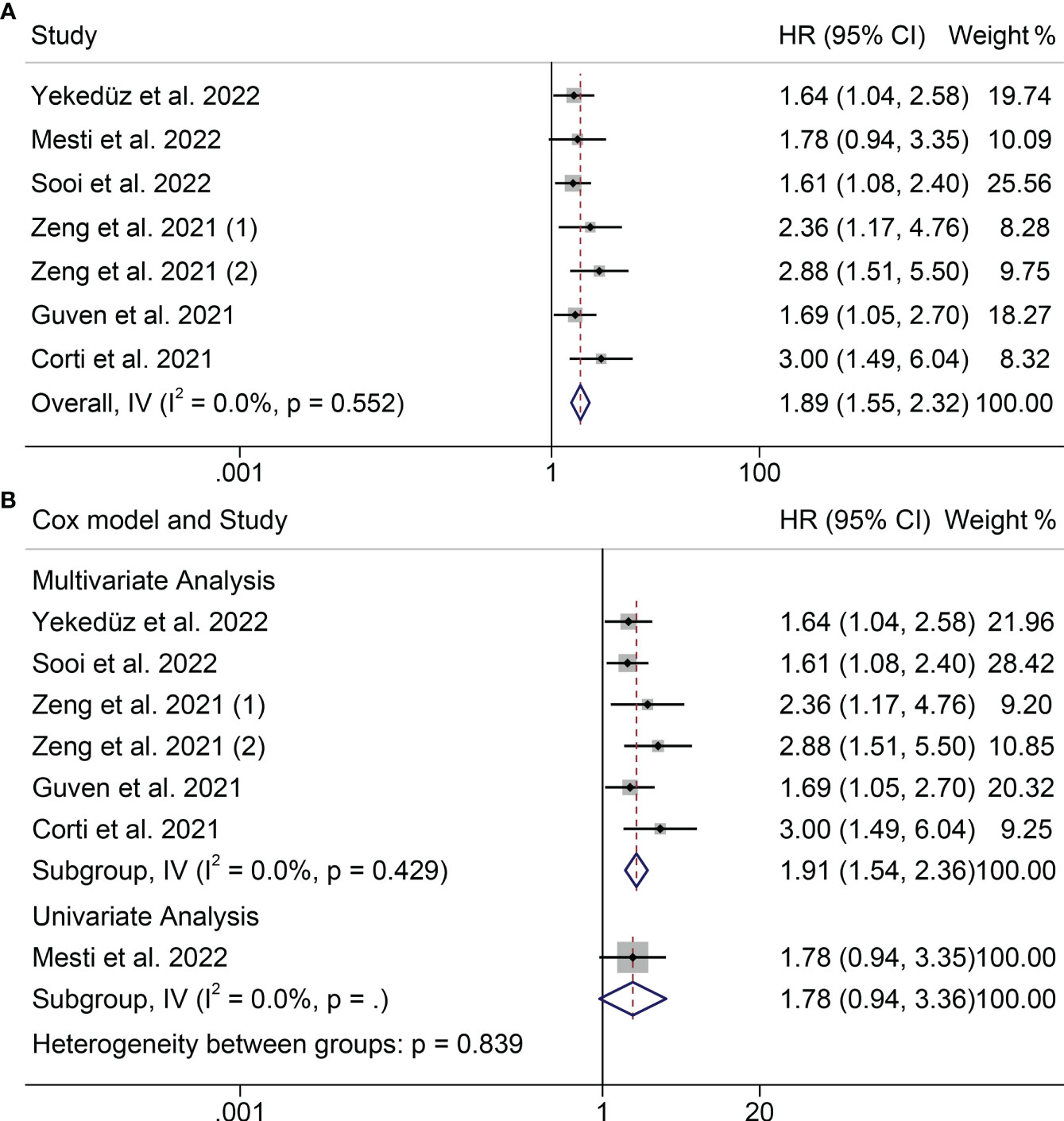
Figure 2 Forest plots of the relationship between PIV and OS (A) Meta-analysis of the overall survival. (B) Subgroup analysis of overall survival based on multivariate analysis and univariate analysis.
According to its research method to subgroup analysis. In the multivariate analysis subgroups also found similar results, patients with high PIV OS shortened (HR = 1.908, 95% CI: 1.542 2.360, P < 0.001; Figure 2B). But subgroups according to univariate analysis result is meaningless.
3.3 PIV and PFS
Seven studies with a total of 982 patients participated in the study of the relationship between PIV and PFS. Since we did not observe severe heterogeneity in these studies (I2 = 15.2%, P = 0.311; Figure 3A), we applied a fixed model. We found that patients with high PIV levels tended to have poorer survival status (HR = 1.582, 95%CI: 1.324-1.890, P<0.001; Figure 3A). In the subgroup analysis, we also found that high PIV was associated with poor survival in both multivariate and univariate analyses (multivariate analyses: HR = 1.688, 95% CI: 1.346- 2.117, P < 0.001; univariate analyses: HR = 1.426, 95% CI: 1.070- 1.900, P= 0.016; Figure 3B).
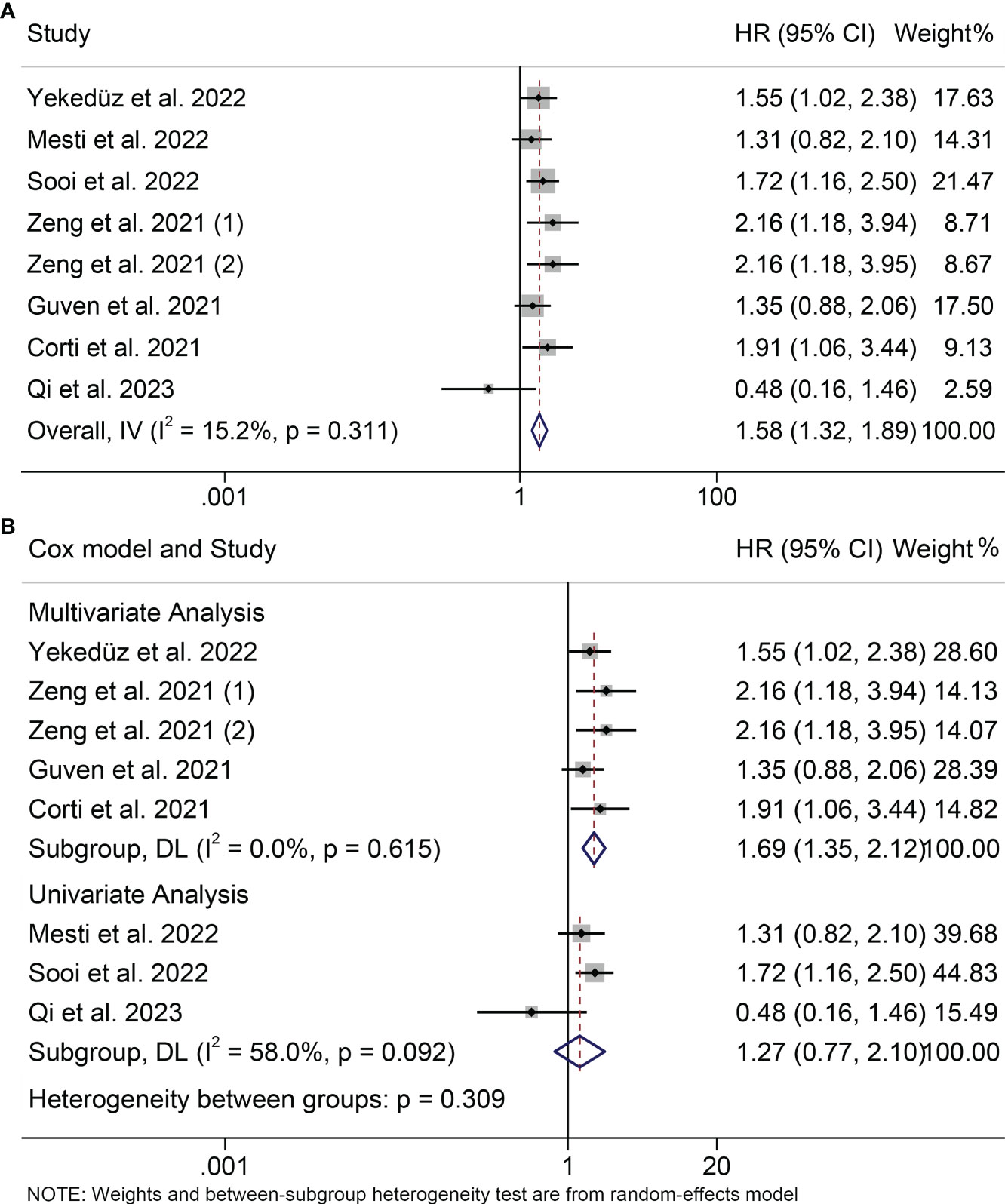
Figure 3 Forest plots of the relationship between PIV and PFS. (A) Meta-analysis of the progression-free survival. (B) Subgroup analysis of progression-free survival based on multivariate analysis and univariate analysis.
3.4 PILE and PFS or OS
Next, we investigated the relationship between PILE level and clinical outcomes (OS and PFS) in cancer patients in two studies. We did not find serious heterogeneity in the studies of these two clinical outcomes, so both were fixed models used (OS: I2 = 0.0%, P = 0.578; PFS: I2 = 0.0%, P = 0.437; Figure 4). In both studies, patients with high levels of running with worse OS and PFS (OS: HR = 2.728, 95% CI: 1.993 - 3.733, P < 0.001; PFS: HR = 2.117, 95% CI: 1.574- 2.847, P < 0.001; Figure 4).
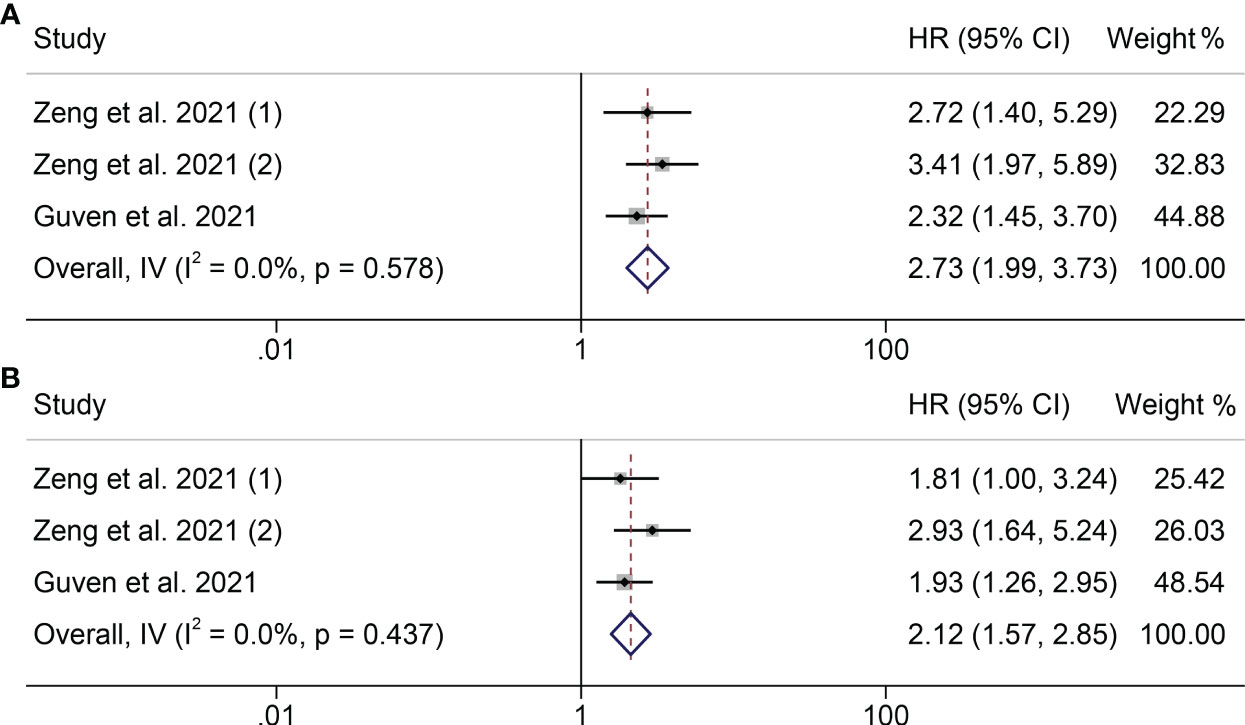
Figure 4 Forest plots of the relationship between PILE and ICI Efficacy in melanoma patients. (A) Meta-analysis of the overall survival. (B) Subgroup analysis of overall survival based on multivariate analysis and univariate analysis.
3.5 Sensitivity analysis
In order to evaluate the reliability of the results, we conducted a sensitivity analysis in which we excluded each study iteratively and assessed its effect on the overall findings. Our analysis suggests that excluding any of the studies did not have a major impact on the OS HR. Specifically, the HR estimates for OS ranged from 1.810 (95% CI: 1.464-2.239) when excluding Zeng et al., 2021 (2) to 2.003 (95% CI: 1.585-2.531) when excluding Sooi et al., (18) (Figure 5A). In addition, the sensitivity analysis indicated that the removal of any one study did not have a major impact on the overall results of PFS. The range of HR values varied from 1.535 (95% CI: 1.274-1.850) after excluding Zeng et al., 2021 (1) to 1.636 (95% CI: 1.345-1.990) after excluding Guven et al., (12) (Figure 5B).
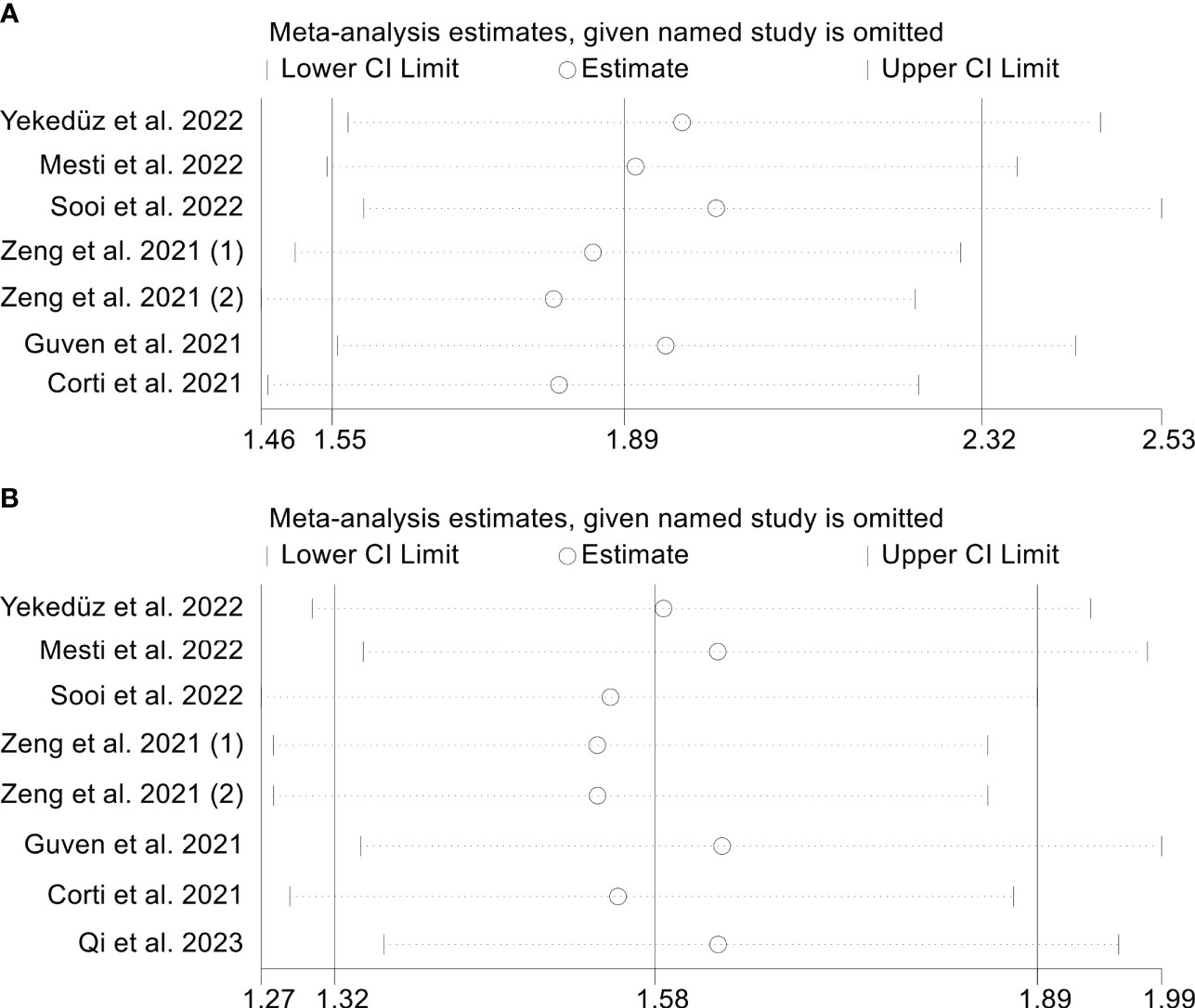
Figure 5 Sensitivity analysis. (A). PIV and overall survival (B). PIV and progression-free survival.
3.6 Publication bias
The results showed that PFS (Egger’s test: P = 0.455, Berg’s test: P = 1.000) no significant publication bias. However, we found that the OS is publication bias (Egger’s test: P = 0.015, Berg’s test: P = 0.016). To address this issue, we employed a trim and fill method to estimate the number of studies that might be missing in OS. The results showed no change in the combined HR when missing studies were not included (Figure 6).
4 Discussion
In this study, we aimed to explore the predictive significance of PIV and PILE in cancer patients, and the pooled data showed that higher PIV and PILE were significantly associated with shorter OS and PFS. Furthermore, the results remained stable even after conducting sensitivity and subgroup analyses. To our knowledge, this is the inaugural comprehensive meta-analysis to investigate the influence of PIV and PILE on the prognosis of cancer patients undergoing immunotherapy. As a readily available clinical indicator, assessing patients before immunotherapy can significantly enhance physicians’ ability to predict clinical outcomes and adjust treatment regimens promptly, ultimately leading to a reduction in mortality rates.
Although ICI has become a promising treatment for most cancer patients, the factors affecting its effectiveness are still unclear (21–23). Several biomarkers, including tumor mutation burden, microsatellite instability/mismatch repair deficiency, tertiary lymphoid structures, and tumor-infiltrating lymphocytes, have been suggested as potential predictors of response to ICI. However, the difficulties associated with acquiring specimens, the high cost, and the immaturity of detection technology impede the clinical application of these biomarkers. This prompted our focus on blood cells near tumors (24). These cells serve distinct roles in both promoting and inhibiting tumor growth. Neutrophils and platelets consistently promote tumor progression, whereas lymphocytes primarily drive anticancer responses in the tumor microenvironment (25, 26). The function of monocytes exhibits a heightened level of intricacy and context sensitivity. Particularly in the context of carcinogenesis, prevailing evidence suggests a prevalence of tumor-promoting effects. Studies have demonstrated a correlation between heightened secretion of pro-inflammatory cytokines and diminished monolayer confluence among tumor cells. Leveraging this paradigm, it becomes feasible to initially evaluate the effectiveness of Chimeric Antigen Receptor T-cell (CAR-T) therapy for solid tumors and gauge the potential risk of cytokine release syndrome development. Consequently, there exists an opportunity to enhance the efficacy of immunotherapy (27).
PIV is a recently devised scoring system that incorporates neutrophils, platelets, monocytes, and lymphocytes, among other immune-inflammatory cells, in peripheral blood. It has demonstrated significant utility as a prognostic biomarker in specific types of malignancies, including colon and breast cancer (8, 28, 29). It possesses a significant biological component as a prognostic factor in patients receiving ICI, reflecting systemic inflammation and immune activation. Within the spectrum of immune cell subsets implicated in cancer immune editing, tumor-associated neutrophils have been demonstrated to mechanistically enhance tumor cell migration and invasion, stimulate angiogenesis, and modulate the activity of other immune cell populations (30, 31). Filippo’s research indicates that elevated PIV levels at baseline and during early immunotherapy correlate with inferior OS and PFS outcomes (20). This measure has also been reported to predict outcomes in MSI-high metastatic colorectal cancer patients treated with immunotherapy (32). The PILE model derives its foundation from the scores of PIV, ECOG, and LDH. Due to its consideration of three indicators, it offers enhanced accuracy (11). A study demonstrated that the PILE model exhibited greater accuracy in predicting 12-week PFS and 24-week OS in the context of predicting immunotherapy efficacy (12).
It’s essential to acknowledge the limitations of this analysis. Firstly, the majority of the included studies were retrospective cohort studies, potentially affecting their statistical robustness. Secondly, the variation in the types of ICI utilized across studies introduces inconsistency. Consequently, further research, particularly well-designed, large-scale, multicenter prospective studies, is imperative to validate and enhance our findings.
5 Conclusion
In conclusion, cancer patients undergoing ICI treatment face diminished survival probabilities in instances characterized by elevated PIV and PILE measures. This straightforward categorization may prove beneficial in clinical application. Additional external multicenter randomized controlled trials are required to authenticate our findings on the effectiveness of the queried medication.
Data availability statement
The original contributions presented in the study are included in the article/Supplementary Material. Further inquiries can be directed to the corresponding authors.
Author contributions
TK: Writing – original draft. LZ: Software, Writing – original draft. ZQ: Writing – review & editing. KD: Writing – review & editing. WW: Writing – review & editing. KW: Writing – original draft.
Funding
The author(s) declare that no financial support was received for the research, authorship, and/or publication of this article.
Acknowledgments
The authors thank all the medical staff who contributed to the maintenance of the medical record database.
Conflict of interest
The authors declare that the research was conducted in the absence of any commercial or financial relationships that could be construed as a potential conflict of interest.
Publisher’s note
All claims expressed in this article are solely those of the authors and do not necessarily represent those of their affiliated organizations, or those of the publisher, the editors and the reviewers. Any product that may be evaluated in this article, or claim that may be made by its manufacturer, is not guaranteed or endorsed by the publisher.
Supplementary material
The Supplementary Material for this article can be found online at: https://www.frontiersin.org/articles/10.3389/fimmu.2024.1326083/full#supplementary-material
References
1. Murray CJ, Atkinson C, Bhalla K, Birbeck G, Burstein R, Chou D, et al. The state of US health, 1990-2010: burden of diseases, injuries, and risk factors. Jama. (2013) 310(6):591–608. doi: 10.1001/jama.2013.13805
2. Sordo-Bahamonde C, Lorenzo-Herrero S, Granda-Díaz R, Martínez-Pérez A, Aguilar-García C, Rodrigo JP, et al. Beyond the anti-PD-1/PD-L1 era: promising role of the BTLA/HVEM axis as a future target for cancer immunotherapy. Mol Canc (2023) 22(1):142. doi: 10.1186/s12943-023-01845-4
3. Dolton G, Rius C, Wall A, Szomolay B, Bianchi V, Galloway SAE, et al. Targeting of multiple tumor-associated antigens by individual T cell receptors during successful cancer immunotherapy. Cell. (2023) 186(16):3333–49.e27. doi: 10.1016/j.cell.2023.06.020
4. Sattiraju A, Kang S, Giotti B, Chen Z, Marallano VJ, Brusco C, et al. Hypoxic niches attract and sequester tumor-associated macrophages and cytotoxic T cells and reprogram them for immunosuppression. Immunity. (2023) 56(8):1825–43.e6. doi: 10.1016/j.immuni.2023.06.017
5. Hoos A. Development of immuno-oncology drugs - from CTLA4 to PD1 to the next generations. Nat Rev Drug Discovery (2016) 15(4):235–47. doi: 10.1038/nrd.2015.35
6. Chen Y, Hu H, Yuan X, Fan X, Zhang C. Advances in immune checkpoint inhibitors for advanced hepatocellular carcinoma. Front Immunol (2022) 13:896752. doi: 10.3389/fimmu.2022.896752
7. Le DT, Durham JN, Smith KN, Wang H, Bartlett BR, Aulakh LK, et al. Mismatch repair deficiency predicts response of solid tumors to PD-1 blockade. Science. (2017) 357(6349):409–13. doi: 10.1126/science.aan6733
8. Fucà G, Guarini V, Antoniotti C, Morano F, Moretto R, Corallo S, et al. The Pan-Immune-Inflammation Value is a new prognostic biomarker in metastatic colorectal cancer: results from a pooled-analysis of the Valentino and TRIBE first-line trials. Br J Canc (2020) 123(3):403–9. doi: 10.1038/s41416-020-0894-7
9. Lee LE, Ahn SS, Pyo JY, Song JJ, Park YB, Lee SW. Pan-immune-inflammation value at diagnosis independently predicts all-cause mortality in patients with antineutrophil cytoplasmic antibody-associated vasculitis. Clin Exp Rheumatol (2021) 39 Suppl 129(2):88–93. doi: 10.55563/clinexprheumatol/m46d0v
10. Cremolini C, Loupakis F, Antoniotti C, Lupi C, Sensi E, Lonardi S, et al. FOLFOXIRI plus bevacizumab versus FOLFIRI plus bevacizumab as first-line treatment of patients with metastatic colorectal cancer: updated overall survival and molecular subgroup analyses of the open-label, phase 3 TRIBE study. Lancet Oncol (2015) 16(13):1306–15. doi: 10.1016/S1470-2045(15)00122-9
11. Bigot F, Castanon E, Baldini C, Hollebecque A, Carmona A, Postel-Vinay S, et al. Prospective validation of a prognostic score for patients in immunotherapy phase I trials: The Gustave Roussy Immune Score (GRIm-Score). Eur J Canc (2017) 84:212–8. doi: 10.1016/j.ejca.2017.07.027
12. Guven DC, Yildirim HC, Bilgin E, Aktepe OH, Taban H, Sahin TK, et al. PILE: a candidate prognostic score in cancer patients treated with immunotherapy. Clin Transl Oncol (2021) 23(8):1630–6. doi: 10.1007/s12094-021-02560-6
13. Liberati A, Altman DG, Tetzlaff J, Mulrow C, Gøtzsche PC, Ioannidis JP, et al. The PRISMA statement for reporting systematic reviews and meta-analyses of studies that evaluate health care interventions: explanation and elaboration. PloS Med (2009) 6(7):e1000100. doi: 10.1371/journal.pmed.1000100
14. Stang A. Critical evaluation of the Newcastle-Ottawa scale for the assessment of the quality of nonrandomized studies in meta-analyses. Eur J Epidemiol (2010) 25(9):603–5. doi: 10.1007/s10654-010-9491-z
15. Qi WX, Wang X, Li C, Li S, Li H, Xu F, et al. Pretreatment absolute lymphocyte count is an independent predictor for survival outcomes for esophageal squamous cell carcinoma patients treated with neoadjuvant chemoradiotherapy and pembrolizumab: An analysis from a prospective cohort. Thorac Canc (2023) 14(17):1556–66. doi: 10.1111/1759-7714.14898
16. Mesti T, Grašič Kuhar C, Ocvirk J. Biomarkers for outcome in metastatic melanoma in first line treatment with immune checkpoint inhibitors. Biomedicines. (2023) 11(3). doi: 10.3390/biomedicines11030749
17. Yekedüz E, Tural D, Ertürk İ, Karakaya S, Erol C, Ercelep Ö, et al. The relationship between pan-immune-inflammation value and survival outcomes in patients with metastatic renal cell carcinoma treated with nivolumab in the second line and beyond: a Turkish oncology group kidney cancer consortium (TKCC) study. J Cancer Res Clin Oncol (2022) 148(12):3537–46. doi: 10.1007/s00432-022-04055-5
18. Sooi K, Li Low J, Miow QH, Kumarakulasinghe NB, Sundar R, Huang Y. Pan-immune-inflammation value as a predictive biomarker for survival in advanced non-small cell lung cancer patients treated with immunotherapy. J Clin Oncol (2022) 40(16). doi: 10.1200/JCO.2022.40.16_suppl.e21095
19. Zeng R, Liu F, Fang C, Yang J, Luo L, Yue P, et al. PIV and PILE score at baseline predict clinical outcome of anti-PD-1/PD-L1 inhibitor combined with chemotherapy in extensive-stage small cell lung cancer patients. Front Immunol (2021) 12:724443. doi: 10.3389/fimmu.2021.724443
20. Corti F, Lonardi S, Intini R, Salati M, Fenocchio E, Belli C, et al. The Pan-Immune-Inflammation Value in microsatellite instability-high metastatic colorectal cancer patients treated with immune checkpoint inhibitors. Eur J Canc (2021) 150:155–67. doi: 10.1016/j.ejca.2021.03.043
21. Suárez C, Morales-Barrera R, Ramos V, Núñez I, Valverde C, Planas J, et al. Role of immunotherapy in castration-resistant prostate cancer (CRPC). BJU Int (2014) 113(3):367–75. doi: 10.1111/bju.12110
22. Eggermont AM, Spatz A, Robert C. Cutaneous melanoma. Lancet (2014) 383(9919):816–27. doi: 10.1016/S0140-6736(13)60802-8
23. PD-1 inhibitors raise survival in NSCLC. Cancer Discovery (2014) 4(1):6. doi: 10.1158/2159-8290.CD-NB2013-164
24. Dumitru CA, Lang S, Brandau S. Modulation of neutrophil granulocytes in the tumor microenvironment: mechanisms and consequences for tumor progression. Semin Cancer Biol (2013) 23(3):141–8. doi: 10.1016/j.semcancer.2013.02.005
25. Deryugina EI, Zajac E, Juncker-Jensen A, Kupriyanova TA, Welter L, Quigley JP. Tissue-infiltrating neutrophils constitute the major in vivo source of angiogenesis-inducing MMP-9 in the tumor microenvironment. Neoplasia. (2014) 16(10):771–88. doi: 10.1016/j.neo.2014.08.013
26. Riesenberg BP, Ansa-Addo EA, Gutierrez J, Timmers CD, Liu B, Li Z. Cutting edge: targeting thrombocytes to rewire anticancer immunity in the tumor microenvironment and potentiate efficacy of PD-1 blockade. J Immunol (2019) 203(5):1105–10. doi: 10.4049/jimmunol.1900594
27. Valiullina AK, Zmievskaya EA, Ganeeva IA, Zhuravleva MN, Garanina EE, Rizvanov AA, et al. Evaluation of CAR-T cells' Cytotoxicity against modified solid tumor cell lines. Biomedicines (2023) 11(2). doi: 10.3390/biomedicines11020626
28. Ligorio F, Fucà G, Zattarin E, Lobefaro R, Zambelli L, Leporati R, et al. The pan-immune-inflammation-value predicts the survival of patients with human epidermal growth factor receptor 2 (HER2)-positive advanced breast cancer treated with first-line taxane-trastuzumab-pertuzumab. Cancers (Basel) (2021) 13(8). doi: 10.3390/cancers13081964
29. Prelaj A, Ferrara R, Rebuzzi SE, Proto C, Signorelli D, Galli G, et al. EPSILoN: A prognostic score for immunotherapy in advanced non-small-cell lung cancer: A validation cohort. Cancers (Basel) (2019) 11(12). doi: 10.3390/cancers11121954
30. Shaul ME, Fridlender ZG. Tumour-associated neutrophils in patients with cancer. Nat Rev Clin Oncol (2019) 16(10):601–20. doi: 10.1038/s41571-019-0222-4
31. Kitamura T, Qian BZ, Pollard JW. Immune cell promotion of metastasis. Nat Rev Immunol (2015) 15(2):73–86. doi: 10.1038/nri3789
Keywords: immunotherapy, meta-analysis, prognosis, pan-immune inflammation value, tumor
Citation: Kuang T, Qiu Z, Wang K, Zhang L, Dong K and Wang W (2024) Pan-immune inflammation value as a prognostic biomarker for cancer patients treated with immune checkpoint inhibitors. Front. Immunol. 15:1326083. doi: 10.3389/fimmu.2024.1326083
Received: 22 October 2023; Accepted: 24 January 2024;
Published: 12 February 2024.
Edited by:
Kanjoormana Aryan Manu, Amala Cancer Research Centre, IndiaReviewed by:
Brigida Anna Maiorano, IRCCS Casa Sollievo della Sofferenza Hospital, ItalyEmil Bulatov, Kazan Federal University, Russia
Copyright © 2024 Kuang, Qiu, Wang, Zhang, Dong and Wang. This is an open-access article distributed under the terms of the Creative Commons Attribution License (CC BY). The use, distribution or reproduction in other forums is permitted, provided the original author(s) and the copyright owner(s) are credited and that the original publication in this journal is cited, in accordance with accepted academic practice. No use, distribution or reproduction is permitted which does not comply with these terms.
*Correspondence: Keshuai Dong, dongkeshuai@whu.edu.cn; Weixing Wang, wangwx@whu.edu.cn
†These authors have contributed equally to this work